- 1Department of Cardiology, Beijing Hospital, National Center of Gerontology, Beijing, China
- 2Neurology Department, Beijing Hospital, National Center of Gerontology, Beijing, China
- 3Department of Geriatric Medicine, Affiliated Hospital of Qinghai University, Xining, China
Atrial fibrillation (AF) increases the risk of ischemic stroke and systemic arterial embolism. However, the risk factors or predictors of stroke in AF patients have not been clarified. Therefore, it is necessary to find effective diagnostic and therapeutic targets. Two datasets were downloaded from the Gene Expression Omnibus (GEO) database. Differently expressed genes (DEGs) were identified between samples of atrial fibrillation without stroke and atrial fibrillation with stroke. Enrichment analysis of Gene Ontology (GO) and Kyoto Encyclopedia of Genes and Genomes (KEGG) by Gene Set Enrichment Analysis (GSEA), construction and analysis of protein-protein interaction (PPI) network and significant module, and the receiver operator characteristic (ROC) curve analysis were performed. A total of 524 DEGs were common to both datasets. Analysis of KEGG pathways indicated that the top canonical pathways associated with DEGs were ubiquitin-mediated proteolysis, endocytosis, spliceosome, and so on. Ten hub genes (SMURF2, CDC42, UBE3A, RBBP6, CDC5L, NEDD4L, UBE2D2, UBE2B, UBE2I, and MAPK1) were identified from the PPI network and were significantly associated with a diagnosis of atrial fibrillation and stroke (AFST). In summary, a total of 524 DEGs and 10 hub genes were identified between samples of atrial fibrillation without stroke and atrial fibrillation with stroke. These genes may serve as the target of early diagnosis or treatment of AF complicated by stroke.
Introduction
Atrial fibrillation (AF) is a type of supraventricular tachyarrhythmia characterized by rapid and disordered atrial electrical activity (1). The atrium loses effective contraction due to disordered electrical activity and the atrioventricular node presents diminished conduction to rapid atrial activation, resulting in an extremely irregular ventricular rhythm and a rapid or slow ventricular rate, which leads to decreased cardiac blood pumping function and mural thrombosis formation in the atria (2, 3). Stroke is an acute cerebrovascular disease, which is a group of diseases that causes brain tissue damage due to the sudden rupture of blood vessels in the brain or vascular occlusion preventing blood from flowing into the brain (4, 5). AF increases the risk of stroke, with incidence rates of 1.92% a year. Compared with non-AF-related strokes, strokes caused by AF have a worse prognosis, with a mortality rate of nearly 20% and a disability rate of nearly 60% (6). However, the molecular mechanisms of strokes caused by AF are unclear (7).
With the development of molecular biology and second-generation sequencing technology, it is possible to explore the pathogenesis of diseases on a large scale at the gene and molecular level (8). Bioinformatics analysis can obtain a large amount of gene expression information simultaneously and explore differentially expressed genes (DEGs) related to disease initiation and progression (9). Concurrently, these DEGs also provide a novel direction for the diagnosis and treatment of diseases (10). By studying the difference in gene expression profiles between AF without strokes and AF-related strokes, Allende et al. (11) suggested that Hsp70 protects AF-related stroke patients via the prevention of thrombosis without augmenting the risk of bleeding, and it might be a novel biomarker to cure patients of stroke caused by AF. Stamova et al. (12) tried to explore the distinction of gene expression in the cardioembolic stroke patients and advocated that future research should be designed to verify the role of DEGs in strokes and AF. Studying the genetic factors of stroke caused by AF is of great significance, and the research is vital to understand the pathogenesis and provide a theoretical basis of molecular genetics for the precise treatment.
Therefore, in this research, two datasets, GSE66724, and GSE58294, were downloaded from the Gene Expression Omnibus (GEO), followed by screening and enrichment of DEGs and identification of hub genes. Finally, the study reviews diagnostic and prognostic information provided by hub genes and discusses the potential value of hub genes as a new therapeutic target for patients with AF-stroke.
Materials and Methods
Access to the Data
Gene Expression Omnibus (GEO) (13) is a gene expression data warehouse and online resource for retrieving gene expression data from any species or artificial source. GEO mainly contains a variety of chip data and some sequencing data. Two datasets [GSE66724 (11) and GSE58294 (12)], which were all annotated in the platform of GPL570 [HG-U133_Plus_2] Affymetrix Human Genome U133 Plus 2.0 Array were downloaded from the GEO database. GSE66724 consisted of 16 whole blood samples, which were taken from eight patients with atrial fibrillation and without stroke (AF, n = 8), and eight patients with atrial fibrillation and stroke (AFST, n = 8). The subject characteristics of individuals in the GSE66724 were showed in the Table 1 (11). GSE58294 also included a total of 92 whole blood samples, which were taken from 23 patients with atrial fibrillation and without stroke (AF, n = 23) and 69 patients with atrial fibrillation and stroke (AFST, n = 69). However, the lack of subject characteristics of individuals in the GSE58294 was a limitation and the patients with stroke were in the hyperacute phase.
The Repeatability Test for the Intra-Group Data
The repeatability of data was verified by Pearson's correlation test. R (14) is an open language and environment for statistical computing and mapping, which was maintained by a large and active global research community. Pearson's correlation test and the mapping of heatmaps were completed by the R language. Principal component analysis (PCA) (15), a strong mathematical method, was capable of reducing the data's complexity. The PCA could capture variance in whole fields by detecting the linear combinations so that the components, which were orthogonal to and not correlated with each other, were divided. The repeatability of data was also verified by the PCA.
Screening of DEGs
GEO2R (16) is a system for online analysis of data in GEO. This tool system runs in R language based on two R packages, GEOquery and limma. The former is used for data reading, and the latter is used for calculation. DEGs between the AF blood samples and the AFST blood samples were screened by GEO2R. P ≤ 0.05 was defined as the cut-off criterion. When the DEGs were not annotated with the gene symbols, they were excluded. The DEGs were presented with volcano maps, which were drawn by a volcano plotting tool (https://shengxin.ren) based on R language. Circos (17) was one useful tool to find the overlapping genes based on their shared pathways or functions. Finding the overlapping DEGs between two datasets was performed by Circos. An online Venn tool (http://bioinformatics.psb.ugent.be/webtools/Venn/) was used to apply a VENN diagram to identify the overlap between GSE66724 and GSE58294 and obtain common DEGs.
Functional Annotation for DEGs via DAVID
Gene Ontology (GO) (18) is a database created by the Gene Ontology Consortium, which consists of a set of predefined GO terms that define and describe the functions of genes and proteins in a variety of species and can be updated as research progresses. The Gene Ontology can be divided into three parts: cellular component (CC), biological process (BP), and molecular function (MF). A protein or gene could find its corresponding GO number through ID matching or sequence annotation, and the GO number could correspond to Term, namely functional category or cell location. KEGG (Release 91.0, July 1, 2019, https://www.kegg.jp/kegg/) (19) is an open database resource for easily understanding utilities and high-level functions of biological systems in the organism, the ecosystem, and the cell from molecular-level information, especially using the large-scale datasets generated from genome sequencing technologies. The Database for Annotation, Visualization, and Integrated Discovery (DAVID) (version: v6.8, https://david.ncifcrf.gov/summary.jsp) (20, 21) provides a well-rounded set of annotation tools for function and pathway enrichment analysis so that the investigators can easily understand the biological meaning of a large list of DEGs. For the given list of DEGs, DAVID tools were able to identify enriched biological themes, particularly GO terms, and visualize genes on BioCarta and KEGG pathway maps.
Function and Pathway Enrichment Analysis by Metascape
Metascape (http://metascape.org/gp/index.html#/main/step1) (22) is a powerful annotation analysis tool for gene function that can help researchers apply popular bioinformatics analysis methods to the analysis of batch genes and proteins so as to realize the cognition of gene or protein functions. It can annotate a large number of genes or proteins, perform enrichment analysis, and construct protein-protein interaction networks. It integrates several authoritative functional databases such as GO, KEGG, and Uniprot to analyze not only human data, but also data from many other species, and to analyze not only a single data set, but also multiple gene sets simultaneously. The Matascape was used to complete the research's function and pathway enrichment analysis.
Enrichment Analysis by Gene Set Enrichment Analysis (GSEA)
Gene Set Enrichment Analysis (GSEA) (http://software.broadinstitute.org/gsea/index.jsp) (23, 24) is a powerful computational method that determines whether a priori defined set of genes manifests statistically significant differences between two states. Using a predefined set of genes that is usually derived from functional notes or results of previous experiments, GSEA can rank the genes by the degree of differential expression in the two samples, and then check to see if the predefined set of genes is enriched at the top or bottom of the list. Gene set enrichment assays detect changes in the expression of gene sets rather than individual genes and thus can include these subtle changes in expression, with better results expected. GSEA does not need to specify a clear threshold of differentially expressed genes and the algorithm analyzes the overall trend according to the actual situation. GSEA analysis was carried out to perform the GO and KEGG enrichment analysis. GSEA would be conducted on the sequenced genes of AF and AFST blood samples after importing reference function sets, gene annotation files, and all gene data of both AF and AFST blood samples.
The Construction of Protein–Protein Interaction (PPI) Network, Screening of the Significant Module, and Identification of Hub Genes
An online database, the Search Tool for the Retrieval of Interacting Genes (STRING) (25) (http://string-db.org), can trace and predict the protein–protein interaction (PPI) network after the common DEGs are imported into the database. The STRING was used to construct a PPI network of DEGs. The Cytoscape (version 2.8) (26), an open visualization software tool, was used to visualize this network. The Molecular Complex Detection tool (MCODE) (version 1.5.1) (27), a plug-in of Cytoscape, could screen and identify the most significant module in the PPI network, and the criteria was that MCODE scores >5, the degree of cut-off = 2, node score cut-off = 0.2, k-score = 2, and maximum depth = 100. Furthermore, once the degree was more than 10, the cytoHubba (28), a plug-in of Cytoscape, could identify the hub genes.
The Correlation Analysis Between the Hub Genes and AFST
Pearson's correlation test was performed to complete the correlation analysis of the hub genes. The mapping of heatmaps, which could present the correlation among the hub genes, was completed by the R language. Spearman's correlation and multiple linear regression analyses between AFST and relevant gene expression were also carried out.
Enrichment Analysis, Expression Analysis, and Diagnostic Analysis of Hub Genes
The GO and KEGG enrichment analyses for hub genes were completed via the DAVID tool, and the bubble diagrams were drawn by R language. Two heatmaps hub genes' expression level were visualized with R language. Finally, the receiver operator characteristic (ROC) curve analysis was performed to determine the usefulness of these hub genes in predicting AFST. The SPSS software (version 21.0; IBM; New York; America) was used to conduct a statistical analysis. A P ≤ 0.05 was considered statistically significant.
Identification of Hub Genes Associated With Cardiovascular and Nervous Diseases
The comparative toxicogenomics database (http://ctdbase.org/) (29) was used to identify the integrated chemical–disease, chemical–gene, and gene–disease interactions to predict novel associations and generate expanded networks. The relationships between gene products and cardiovascular and nervous diseases were analyzed by the comparative toxicogenomics database.
Results
Validation of Data
Pearson's correlation test shows that there are powerful correlations among samples in the AF group, and that there are also powerful correlations among samples in AFST group in the GSE66724 dataset (Figure 1A). After performing the principal component analysis, the repeatability of data in GSE66724 was fine (Figure 1B). Pearson's correlation test shows that there are powerful correlations among samples in the AF group, and that there are also powerful correlations among samples in AFST group in the GSE58294 dataset (Figure 1C). After performing the principal component analysis, the repeatability of data in GSE58294 was fine (Figure 1D).
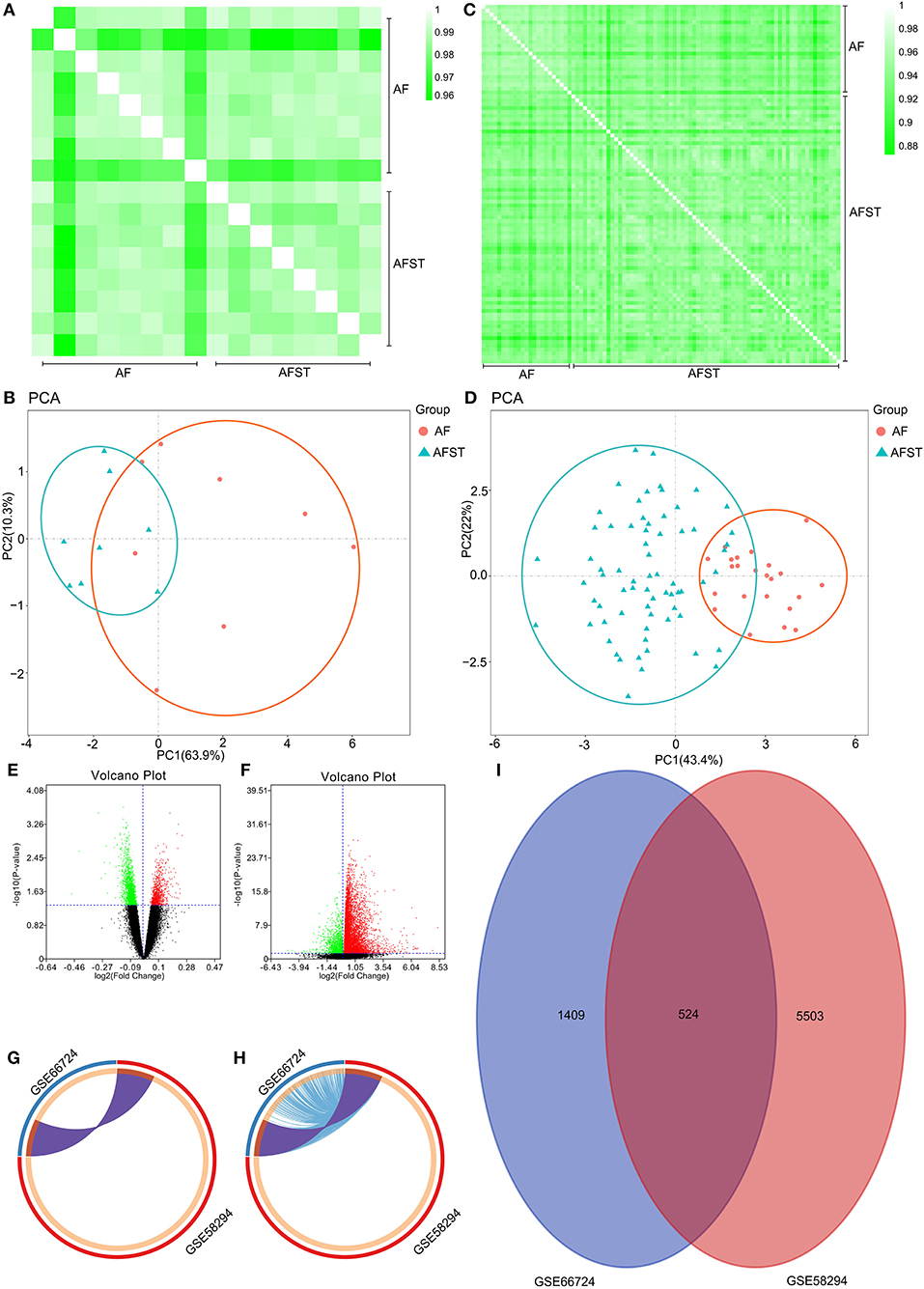
Figure 1. Validation of data, and the identification of DEGs between AF and AFST samples. (A) Pearson's correlation test for GSE66724 dataset. (B) The principal component analysis for GSE66724. (C) Pearson's correlation test for GSE58294 dataset. (D) After performing the principal component analysis, the repeatability of data in GSE58294 was fine. (E) The DEGs between AF and AFST blood samples in the GSE66724 were presented in the volcano plots. (F) The DEGs in the GSE58294 were presented in the volcano plots. (G) The overlapping DEGs between GSE66724 and GSE58294 were presented by Circos at gene level. (H) The overlapping DEGs between GSE66724 and GSE58294 were presented by Circos at the shared term level. (I) The VENN diagram manifested 524 DEGs common to both datasets.
The Identification of DEGs Between AF and AFST Samples
Through the GEO2R analysis, the DEGs between AF and AFST blood samples in the GSE66724 were presented in the volcano plot (Figure 1E) along with DEGs in the GSE58294 (Figure 1F). The overlapping DEGs between GSE66724 and GSE58294 were presented by Circos not only at gene level (Figure 1G), but also at the shared term level (Figure 1H). The VENN diagram showed that there was a total of 524 DEGs common to both datasets (Figure 1I).
GO and KEGG Functional Annotation for DEGs via DAVID and Metascape
Through DAVID analysis, the results of the GO analysis showed that variations in DEGs linked with CC were mainly enriched in nucleoplasm and ubiquitin ligase complex (Figure 2A). Variations in DEGs linked with BP were significantly enriched in protein ubiquitination and ubiquitin-dependent protein catabolic process (Figure 2B). With regard to MF, DEGs were significantly enriched in ubiquitin-protein transferase activity, ubiquitin protein ligase activity, and ubiquitin protein ligase binding (Figure 2C). Analysis of KEGG pathways indicated that the top canonical pathways associated with DEGs was ubiquitin mediated proteolysis (Figure 2D).
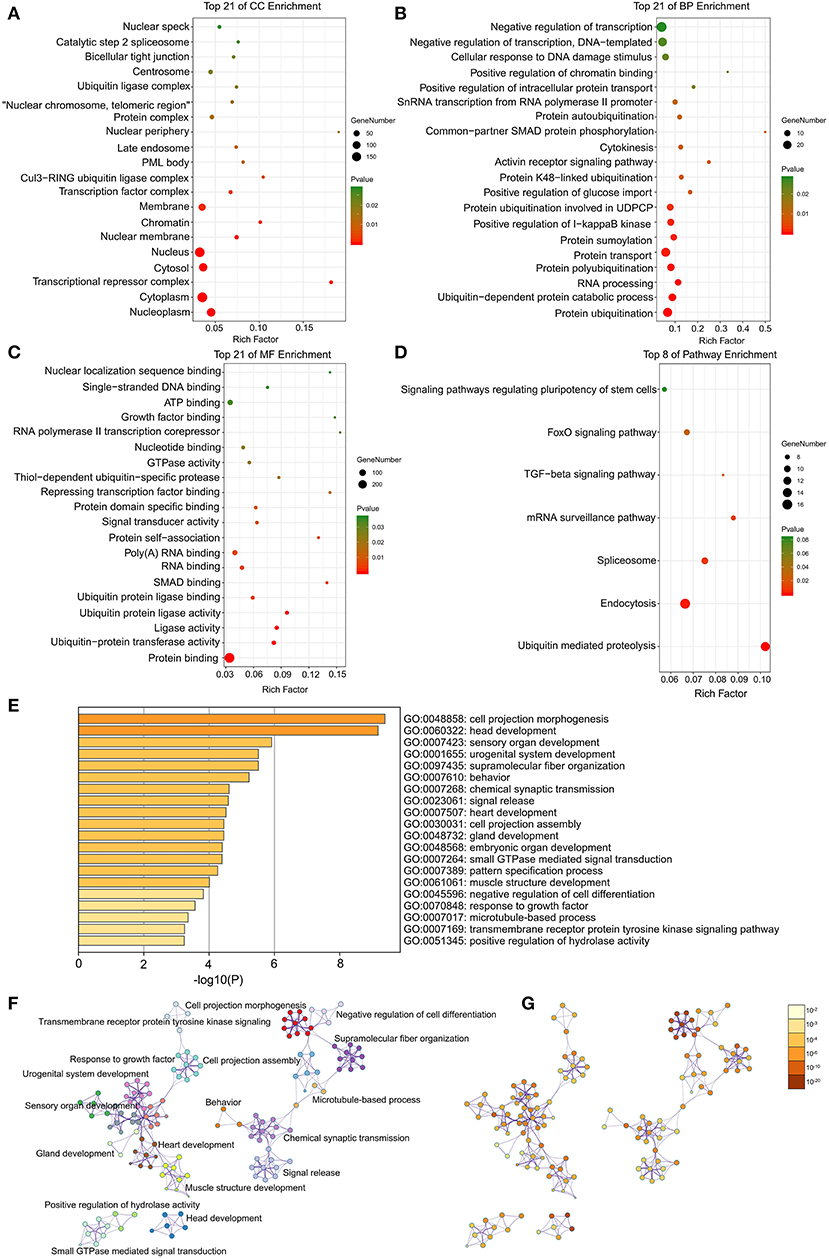
Figure 2. The enrichment analysis of DEGs by DAVID and Metascape. Detailed information relating to changes in the (A) CC, (B) BP, (C) MF, and (D) KEGG analysis for hub genes. (E) Heatmap of enriched terms across the input differently expressed gene lists, colored by p-values, via the Metascape. (F) Network of enriched terms colored by cluster identity, where nodes that share the same cluster identity are typically close to each other. (G) Network of enriched terms colored by p-value, where terms containing more genes tend to have a more significant p-value.
Furthermore, the functional enrichment analysis with Metascape found that the DEGs between AFST and AF blood samples were significantly enriched in the head development and heart development (P < 0.05, Figures 2E–G).
Enrichment Analysis Through GSEA
After implementing the GSEA, the enrichments for upregulated gene sets in the significant order (size of NES) are “GO_REGULATION_OF_CARDIAC_MUSCLE_CONTRACTION_BY_REGULATION_OF_THE_RELEASE_OF_SEQUESTERED_CALCIUM_ION” (Figure 3A), “GO_PROTEIN_K63_LINKED_UBIQUITINATION” (Figure 3B), “KEGG_ P53_SIGNALING_PATHWAY” (Figure 3C), and “KEGG_APOPTOSIS” (Figure 3D), and the enrichments for downregulated gene sets in the significant order (size of NES) are “GO_REGULATION_OF_HEART_MORPHOGENESIS” (Figure 3E) and “KEGG_NEUROACTIVE_LIGAND_RECEPTOR_INTERACTION” (Figure 3F). GSEA also showed that the enrichment gene sets in AFST were mainly related to cardiac muscle, ubiquitination, apoptosis, heart, and neuroactive ligand receptor interaction (Table 2).
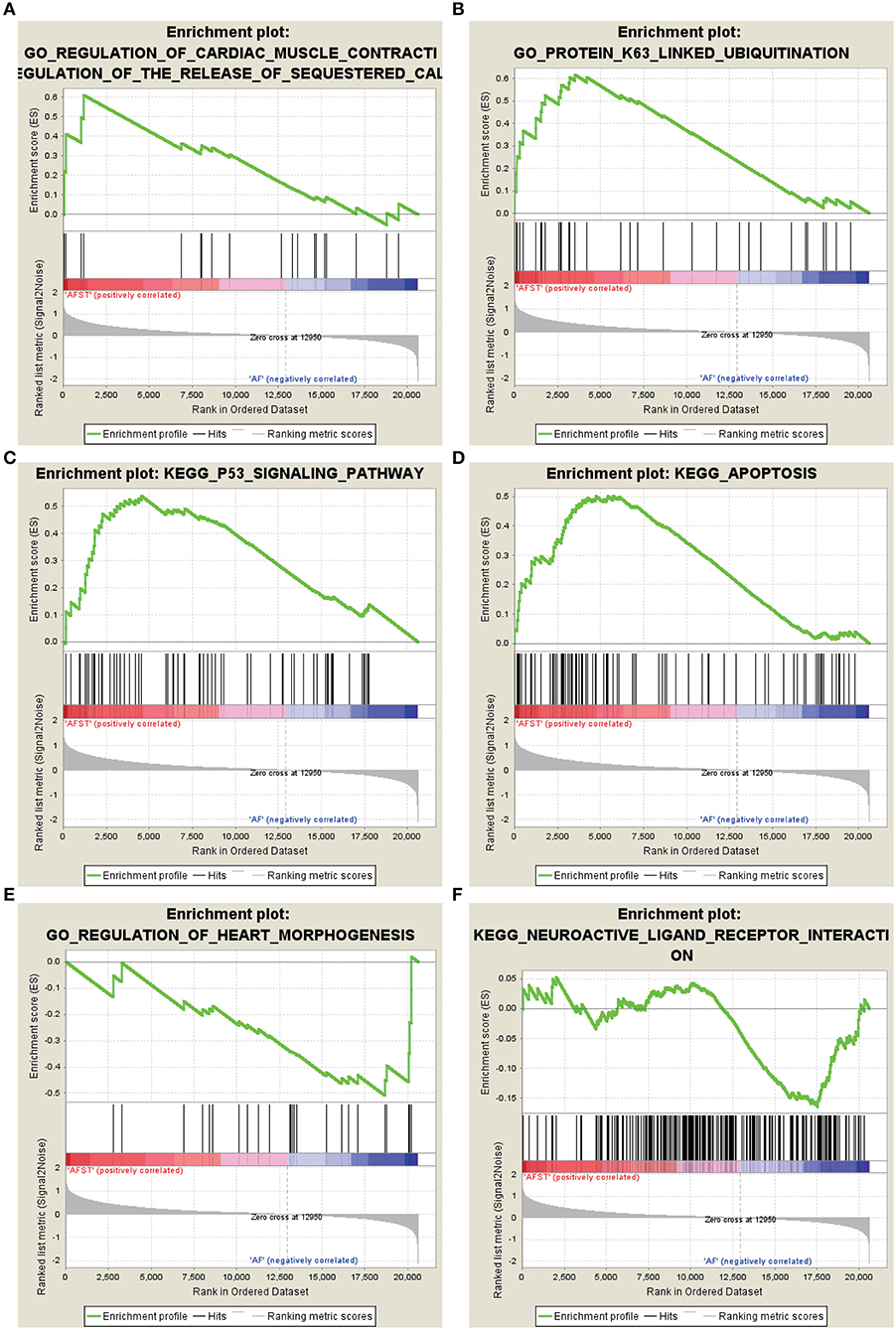
Figure 3. The six enrichments for up-regulated and down-regulated gene sets in the significant order (size of NES). (A) Enrichment plot: GO_REGULATION_OF_CARDIAC_MUSCLE_CONTRACTION_BY_REGULATION_OF_THE_RELEASE_OF_SEQUESTERED_CALCIUM_ION. (B) Enrichment plot: GO_PROTEIN_K63_LINKED_UBIQUITINATION. (C) Enrichment plot: KEGG_P53_SIGNALING_PATHWAY. (D) Enrichment plot: KEGG_APOPTOSIS. (E) Enrichment plot: GO_REGULATION_OF_HEART_MORPHOGENESIS. (F) Enrichment plot: KEGG_NEUROACTIVE_LIGAND_RECEPTOR_INTERACTION.
The Construction of PPI and Module Networks, and Selection of Hub Genes
The PPI network consists of 1,162 edges and 413 nodes (Figure 4A). There were two significant modules screened from PPI network. One module included a total of 171 edges and 19 nodes (Figure 4B), and another module network consisted of 63 edges and 12 nodes (Figure 4C). Ten hub genes (SMURF2, CDC42, UBE3A, RBBP6, CDC5L, NEDD4L, UBE2D2, UBE2B, UBE2I, and MAPK1) were identified from the PPI network when the degrees ≥10 (Figure 4D). Summaries for the gene symbols, full names, and function of 10 hub genes are shown in Table 3.
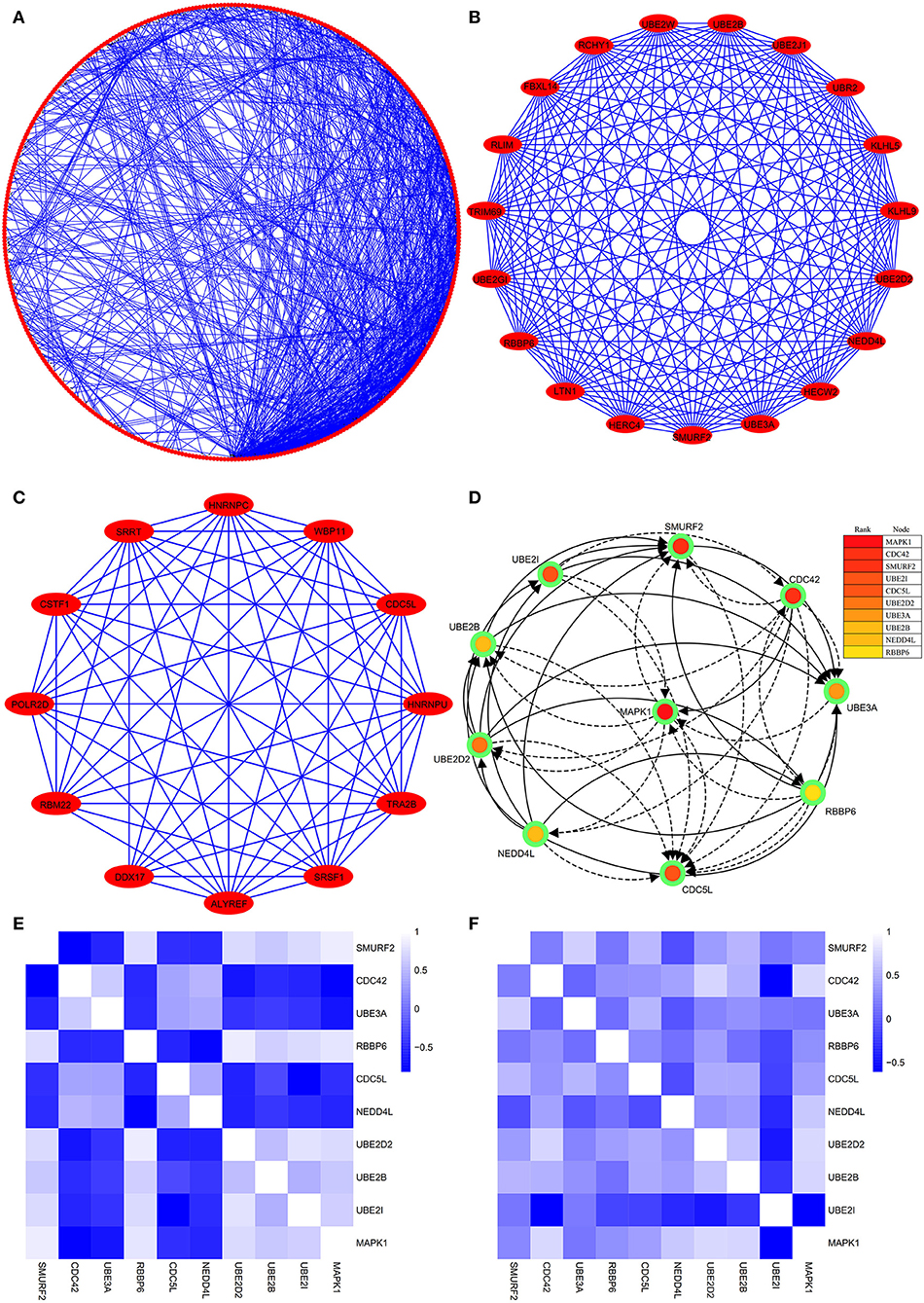
Figure 4. The PPI network of DEGs, two significant modules, hub genes network, and the correlation analysis among hub genes. (A) The PPI network consists of 1,162 edges and 413 nodes. (B) One module includes a total of 171 edges and 19 nodes. (C) Another significant module network consists of 63 edges and 12 nodes. (D) The hub genes network (SMURF2, CDC42, UBE3A, RBBP6, CDC5L, NEDD4L, UBE2D2, UBE2B, UBE2I, and MAPK1). (E) The heatmap manifests that there are strong correlations among the all hub genes in the GSE66724. (F) The strong correlations among hub genes were also verified in the GSE58294.
Strong Correlation Between the Hub Genes and AFST
Through analyzing the expression data of 10 hub genes in the GSE66724, the strong correlations among the all hub genes were found (Figure 4E). Furthermore, the strong correlations were also verified in the GSE58294 (Figure 4F). Through the Spearman correlation coefficient, MAPK1 (ρ = 0.705, p = 0.000) and UBE2D2 (ρ = 0.707, p = 0.000) were significantly correlated with AFST (Table 4). In the multivariate linear regression model, holding all other variables at a fixed value, the natural logarithmic AFST remained associated with MAPK1 and UBE2D2 (p < 0.05) (Table 4).
Functional Enrichment Analysis of Hub Genes
The results of DAVID showed that variations in CC of hub genes were mainly enriched in ubiquitin ligase complex and nuclei (Figure 5A). Variations in the BP of hub genes were significantly enriched in protein ubiquitination, protein K48-linked ubiquitination, and ubiquitin-dependent protein catabolic process (Figure 5B). Variations in the MF of hub genes were significantly enriched in ubiquitin protein ligase activity, ubiquitin–protein transferase activity, and ubiquitin conjugating enzyme activity (Figure 5C). Analysis of KEGG pathways showed that hub genes were mainly enriched in ubiquitin-mediated proteolysis (Figure 5D).
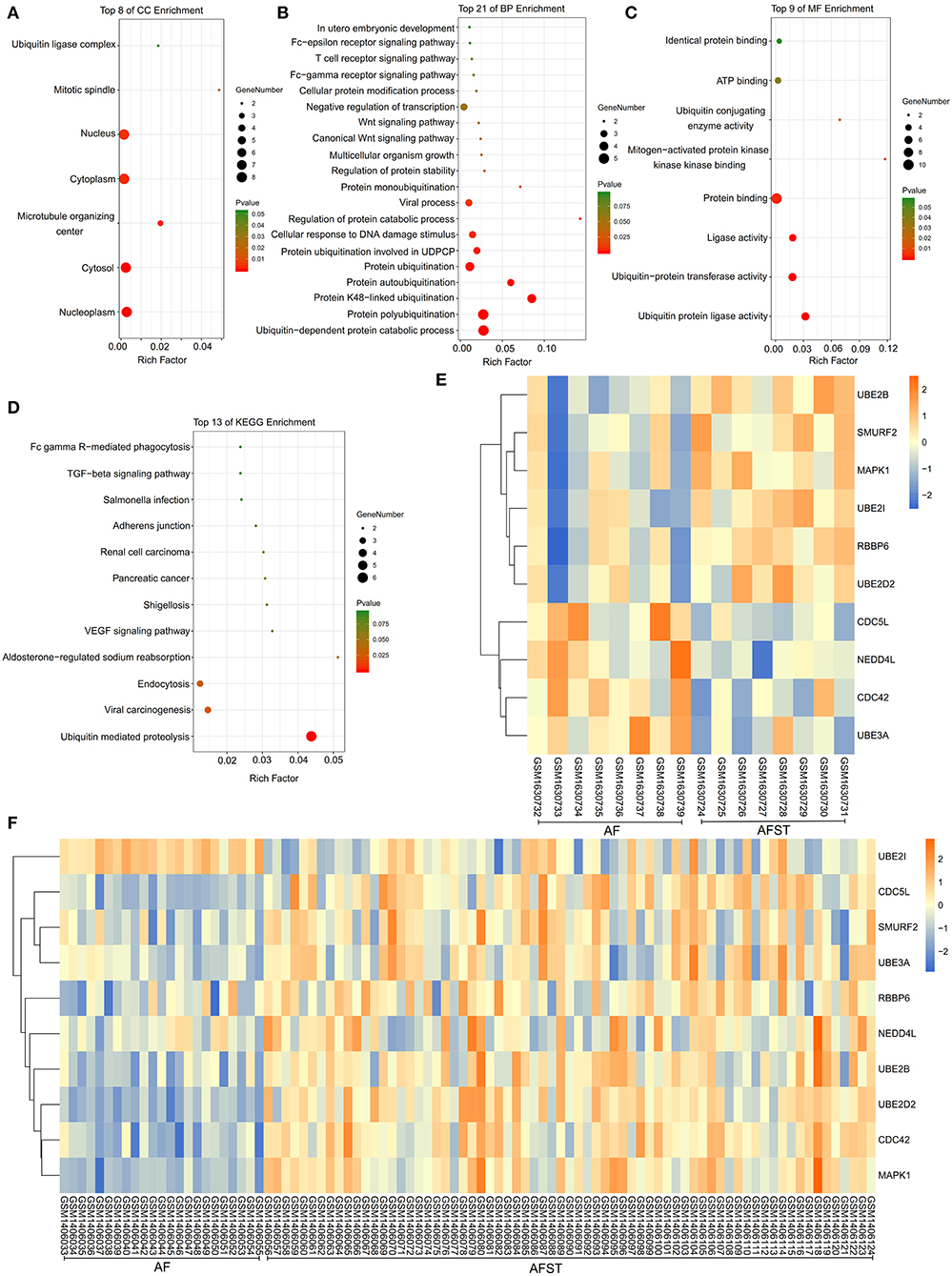
Figure 5. The enrichment analysis and expression level analysis of the hub genes. Detailed information relating to changes in the (A) CC, (B) BP, (C) MF, and (D) KEGG analysis for hub genes. (E) The comparison of expression level of hub genes between AF and AFST samples in the GSE66724. (F) The comparison of expression level of hub genes between AF and AFST samples in the GSE58294.
The Expression Level of Hub Genes in the AF and AFST Blood Samples
One heatmap showed the expression level of hub genes in the GSE66724. When compared with the AF blood samples, the expression of MAPK1 and UBE2D2 were upregulated in the AFST blood samples (Figure 5E). Another heatmap presented the expression level of hub genes in the GSE58294. When compared with the AF blood samples, the expression of MAPK1 and UBE2D2 were also upregulated in the AFST blood samples (Figure 5F).
Hub Genes Could Be Used to Predict AFST Specifically and Sensitively via the ROC Curve Analysis
To verify the accurate thresholds of hub genes to predict AFST, the ROC curves was constructed. The expression of MAPK1 and UBE2D2 were significantly associated with a diagnosis of AFST (0.7 < AUC <1, P ≤ 0.05) (Table 5, Figure 6).
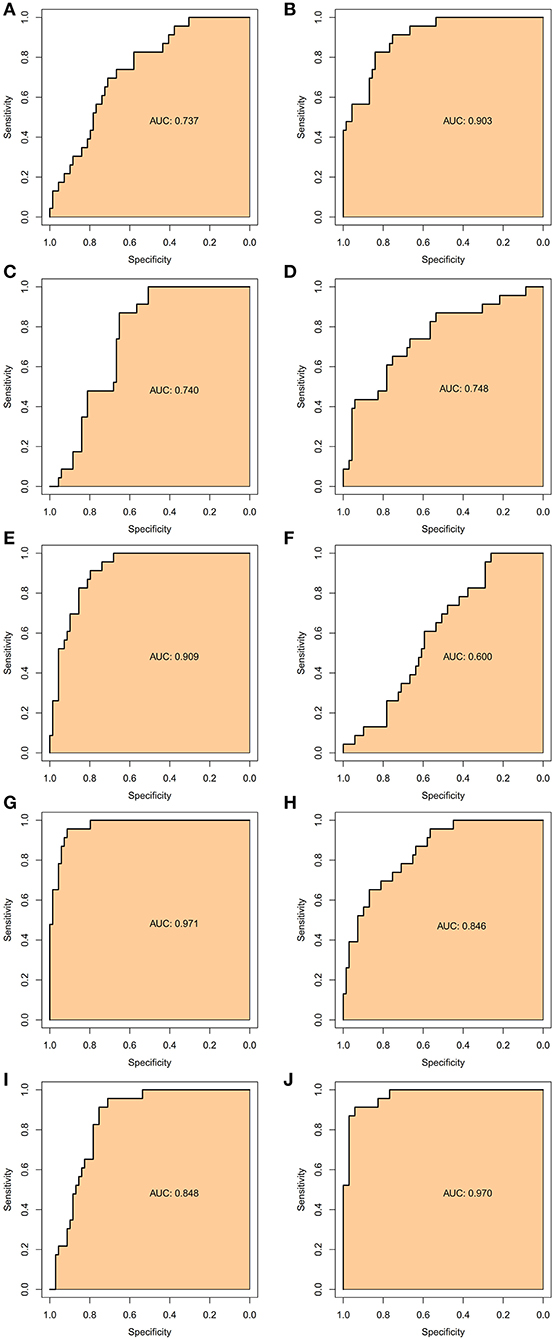
Figure 6. The receiver operator characteristic curves of the hub gene for AFST. (A) SMURF2, (B) CDC42, (C) UBE3A, (D) RBBP6, (E) CDC5L, (F) NEDD4L, (G) UBE2D2, (H) UBE2B, (I) UBE2I, and (J) MAPK1.
Identification of Hub Genes
The CTD database showed that hub genes targeted several cardiovascular and nervous diseases. This data is shown in Figure 7.
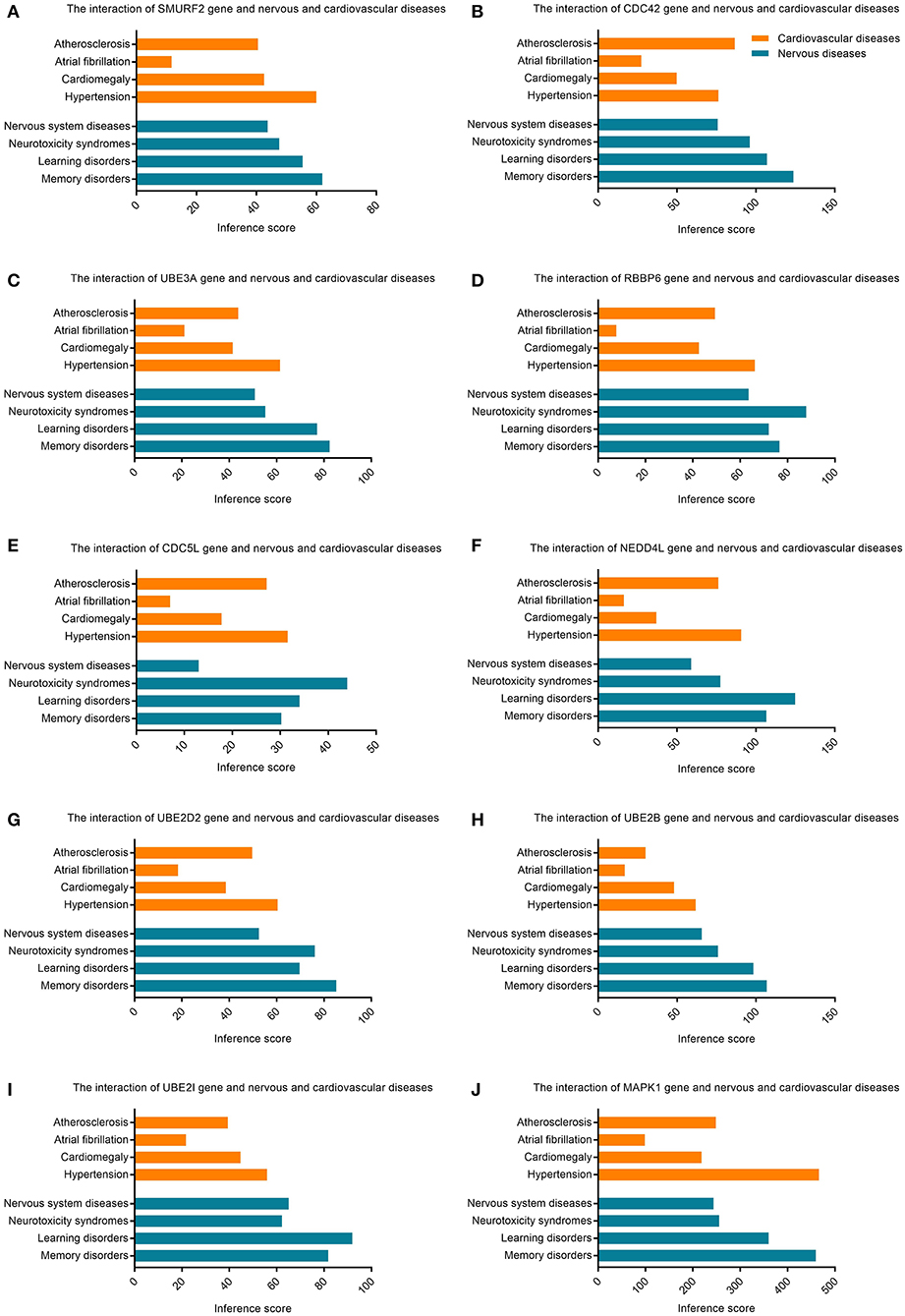
Figure 7. Relationship to cardiovascular or nervous diseases related to hub genes based on the CTD database. (A) SMURF2, (B) CDC42, (C) UBE3A, (D) RBBP6, (E) CDC5L, (F) NEDD4L, (G) UBE2D2, (H) UBE2B, (I) UBE2I, and (J) MAPK1.
Discussion
Atrial fibrillation (AF) increases the risk of ischemic stroke, with an incidence of 1.92% a year. AF patients' risk of ischemic stroke is four to five times that of non-AF patients. Ischemic strokes have a nearly 20% mortality rate and 60% disability rate (30). Therefore, it is of great clinical significance to explore the mechanisms of strokes caused by AF and to search for molecular targets of diagnosis and even treatment (11). Bioinformatics technology has been widely used to find genetic changes during the occurrence and development of diseases, which is a reliable means of finding a target for the diagnosis or treatment of diseases (31).
Our own bioinformatics analysis showed that the ten genes SMURF2, CDC42, UBE3A, RBBP6, CDC5L, NEDD4L, UBE2D2, UBE2B, UBE2I, and MAPK1 were significantly and highly expressed in patients with AF complicated by stroke when compared with patients with simple AF without stroke. Therefore, we speculate that these highly expressed genes, most prominently MAPK1 and UBE2D2, are likely to be involved in incidences of AF complicated by stroke.
MAPK1 (mitogen-activated protein kinase 1) is mainly involved in affecting the activity of protein serine/threonine kinase, protein phosphorylation, regulating gene expression, and apoptosis, which can participate in the occurrence and development of various diseases (9). Through bioinformatics and microarray technology, Si et al. found that MAPK1 is involved in the chemotherapy tolerance of breast carcinoma, providing new paths for mechanism research and targeted therapy of chemotherapy tolerance (32). Through bioinformatics analysis, Xu et al. (33) found that MAPK1 is involved in the drug resistance of ovarian carcinoma. Through experiments, Xu et al. further confirmed the expression of MAPK1 in ovarian carcinoma drug-resistant cells, providing evidence for the drug resistance mechanism and targeted therapy of tumors (33). Through bioinformatics analysis, Yang et al. (34) found that MAPK1 overexpression is associated with the incidence of primary colon adenocarcinoma, suggesting that MAPK1 may be a target for early diagnosis and treatment of primary colon adenocarcinoma. Wang et al. (35) found that the MAPK1-related signaling pathway is involved in the occurrence and development of eclampsia, which may serve as a diagnostic target. Mali et al. (36) found that the stromal interacting molecule-1 may participate in the occurrence of myocardial infarction through MAPK, oxidative stress, and apoptosis, providing a new idea for the mechanism research and treatment of myocardial infarction. Through bioinformatics analysis, Zhu et al. (9) found that MAPK1 can mediate the autophagy of endothelial progenitor cells and participate in the occurrence and development of coronary atherosclerotic heart disease through the mTOR signaling pathway.
At the same time, more and more studies suggest that MAPK is an important regulator of ischemic and hemorrhagic cerebrovascular disease. Through microarray technology, Li et al. (37) found multiple biomarker molecules related to ischemic stroke and found that the MAPK signaling pathway may be involved in the occurrence and development of ischemic stroke. Huang et al. (38) found that the blood let-7e-5p may be a diagnostic target for ischemic stroke through bioinformatics analysis. Further analysis showed that MAPK is involved in the occurrence and development of ischemic stroke, suggesting that MAPK may serve as a therapeutic target. Eyileten et al. (39) summarized the possible diagnostic biomarkers of ischemic stroke and thought that MAPK1 could be a potential diagnostic and therapeutic target. Hayashi et al. (40) found that the MAPK1 signaling pathway is involved in autophagy, which is associated with myocardial infarction and AF (41, 42). We found that MAPK1 is highly expressed in patients with AF complicated by cerebral infarction compared with patients with AF alone. Therefore, we speculate that MAPK1 is involved in the onset of AF complicated by cerebral infarction through multiple mechanisms. Due to the existence of eddy currents in the atria of patients with AF, MAPK1 can promote the detachment and metastasis of atrial thrombus by regulating apoptosis, autophagy, inflammatory stress, and other elements, ultimately causing a stroke. In future studies, it is worth considering how MAPK1 may be a target for the early diagnosis and treatment of AF complicated by stroke.
UBE2D2 (ubiquitin conjugating enzyme E2 D2) is mainly involved in affecting the binding of ubiquitin protein ligases and the activity of ubiquitin protein transferase (43). UBE2D2 plays an important role in tissues and cells, where it can participate in the dissolution of tissues through the ubiquitination enzyme system (44). Geisler et al. (45) found that UBE2D2-mediated autophagy is involved in the progression of Parkinson's disease. Lee et al. (46) found that the expression of UBE2D2 is associated with the prognosis of patients with colorectal cancer (CRC), suggesting that UBE2D2 could be used as one of the prognostic indicators of CRC. Peng et al. (47, 48) argued that autophagy involved with UBE2D2 has a significant impact on the internal environment and can promote the formation and degradation of ubiquitinated aggregates through autophagy. More recent research on UBE2D2 provides new ideas for the mechanism research and targeted therapy of autophagy-related diseases.
There is also a correlation between ubiquitin protease activity, autophagy, and the onset of AF and ischemic stroke (49). Hou et al. (42) contended that moderate autophagy can remove damaged organelles, thereby protecting cells from various damages. However, excessive autophagy can cause the degradation of normal cell and tissue contents, which results in tissue and organ damage. In animal models of ischemic brain injury, autophagy can activate and participate in the death of neurons, suggesting that the regulating of autophagy may be a new treatment approach for ischemic stroke (41, 42). We found that UBE2D2 is highly expressed in patients with AF complicated by cerebral infarction, compared with patients with AF alone. We speculate that in incidences of AF complicated by cerebral infarction, UBE2D2 may be involved by affecting the activity of ubiquitin protease and autophagy. UBE2D2 can also induce the detachment of intra-atrial thrombus by regulating the activity of ubiquitin enzyme and autophagy, thereby increasing the incidence of stroke (45). We thus suggest that UBE2D2 can be a potential diagnostic and therapeutic target for patients with AF complicated by stroke. The relevant mechanism is worth further exploration.
In addition, gene expression could be different according to the stroke phase, and Oh et al. (50) researched the blood genomic profiling of the peripheral blood in the acute phase of ischemic stroke. Stamova et al. (12) studied the gene expression of the hyperacute stroke. We found that there were differences in genomic profiling between hyperacute and acute stoke. Furthermore, a stroke might cause a range of biochemical reactions in the body, from the level of genes to the level of proteins (51). Therefore, the variations in gene expression might be the consequence rather than the cause of a stroke.
Despite this study's rigorous bioinformatics analysis, there were still some outlooks in this study. First, the paper focused on the genomic profiling of a stroke caused by AF. However, it would also be useful to investigate gene expression in patients with strokes due to other causes than AF and it will be defined as the next step to explore the genes of stroke without AF. Second, this study lacks further mechanism validation. The reliability of the conclusion can be improved through animal experiments and clinical sample comprehensive verification. Third, there is increasing evidence that strokes may occur in the context of atrial cardiopathy, even in the absence of clinically overt AF (52, 53). It is, however, not completely understood whether atrial cardiopathy is only a marker and a surrogate of AF or if it can be an independent stroke risk factor. Further, we do not know exactly whether the pathophysiological mechanisms underlying AF and atrial cardiopathy are similar and how much they overlap. Accordingly, the analysis of gene expression should be extended to patients meeting the criteria of atrial cardiopathy, with and without stroke.
Conclusion
Bioinformatics analysis can effectively identify the differential genes in patients with AF complicated by cerebral infarction vs. patients with AF alone, especially the MAPK1 and UBE2D2 genes. These genes are involved in the incidence of AF complicated by cerebral infarction by affecting multiple signaling pathways, which may serve as the target of early diagnosis or treatment. Our study provides new evidence and ideas for further exploration of the mechanism and treatment of AF complicated by stroke.
Data Availability Statement
The datasets used and/or analyzed during the current study are available from the corresponding author upon reasonable request.
Ethics Statement
The data of this research was downloaded from the GEO database, a public website. And all institutional and national guidelines for the care and use of participants were followed.
Author Contributions
YZ and LM analyzed the data and were major contributors in writing. MH was involved in critically revising the manuscript for important intellectual content. TZ and JY made substantial contributions to research conception and designed the draft of the research process. All authors read and approved the final manuscript.
Conflict of Interest
The authors declare that the research was conducted in the absence of any commercial or financial relationships that could be construed as a potential conflict of interest.
Acknowledgments
The authors would like to thank Yong Qiu for his statistical assistance and suggestions during the submitting process.
References
1. Schotten U, Verheule S, Kirchhof P, Goette A. Pathophysiological mechanisms of atrial fibrillation: a translational appraisal. Physiol Rev. (2011) 91:265–325. doi: 10.1152/physrev.00031.2009
2. Zimetbaum P. Atrial fibrillation. Ann Intern Med. (2017) 166:ITC33–48. doi: 10.7326/AITC201703070
3. Lip GY, Fauchier L, Freedman SB, Van Gelder I, Natale A, Gianni C, et al. Atrial fibrillation. Nat Rev Dis Primers. (2016) 2:16016. doi: 10.1038/nrdp.2016.16
4. Wang W, Jiang B, Sun H, Ru X, Sun D, Wang L, et al. Prevalence, incidence, and mortality of stroke in China: results from a nationwide population-based survey of 480 687 adults. Circulation. (2017) 135:759–71. doi: 10.1161/CIRCULATIONAHA.116.025250
5. Wang D, Liu J, Liu M, Lu C, Brainin M, Zhang J. Patterns of stroke between university hospitals and nonuniversity hospitals in Mainland China: prospective multicenter hospital-based registry study. World Neurosurg. (2017) 98:258–65. doi: 10.1016/j.wneu.2016.11.006
6. Moe GK, Abildskov JA. Atrial fibrillation as a self-sustaining arrhythmia independent of focal discharge. Am Heart J. (1959) 58:59–70. doi: 10.1016/0002-8703(59)90274-1
7. Lubitz SA, Parsons OE, Anderson CD, Benjamin EJ, Malik R, Weng LC, et al. Atrial fibrillation genetic risk and ischemic stroke mechanisms. Stroke. (2017) 48:1451–6. doi: 10.1161/STROKEAHA.116.016198
8. Wang O, Chin R, Cheng X, Wu MKY, Mao Q, Tang J, et al. Efficient and unique cobarcoding of second-generation sequencing reads from long DNA molecules enabling cost-effective and accurate sequencing, haplotyping, and de novo assembly. Genome Res. (2019) 29:798–808. doi: 10.1101/gr.245126.118
9. Zhu Y, Yang T, Duan J, Mu N, Zhang T. MALAT1/miR-15b-5p/MAPK1 mediates endothelial progenitor cells autophagy and affects coronary atherosclerotic heart disease via mTOR signaling pathway. Aging. (2019) 11:1089–109. doi: 10.18632/aging.101766
10. Yang J, Hou Z, Wang C, Wang H, Zhang H. Gene expression profiles reveal key genes for early diagnosis and treatment of adamantinomatous craniopharyngioma. Cancer Gene Ther. (2018) 25:227–39. doi: 10.1038/s41417-018-0015-4
11. Allende M, Molina E, Guruceaga E, Tamayo I, Gonzalez-Porras JR, Gonzalez-Lopez TJ, et al. Hsp70 protects from stroke in atrial fibrillation patients by preventing thrombosis without increased bleeding risk. Cardiovasc Res. (2016) 110:309–18. doi: 10.1093/cvr/cvw049
12. Stamova B, Jickling GC, Ander BP, Zhan X, Liu D, Turner R, et al. Gene expression in peripheral immune cells following cardioembolic stroke is sexually dimorphic. PLoS ONE. (2014) 9:e102550. doi: 10.1371/journal.pone.0102550
13. Wang Z, Monteiro CD, Jagodnik KM, Fernandez NF, Gundersen GW, Rouillard AD, et al. Extraction and analysis of signatures from the gene expression omnibus by the crowd. Nat Commun. (2016) 7:12846. doi: 10.1038/ncomms12846
14. Lin Z, Jaberi-Douraki M, He C, Jin S, Yang RSH, Fisher JW, et al. Performance assessment and translation of physiologically based pharmacokinetic models from acslX to berkeley madonna, MATLAB, and R language: oxytetracycline and gold nanoparticles as case examples. Toxicol Sci. (2017) 158:23–35. doi: 10.1093/toxsci/kfx070
15. Ringner M. What is principal component analysis. Nat Biotechnol. (2008) 26:303–4. doi: 10.1038/nbt0308-303
16. Kameshwar AK, Qin W. Metadata analysis of phanerochaete chrysosporium gene expression data identified common CAZymes encoding gene expression profiles involved in cellulose and hemicellulose degradation. Int J Biol Sci. (2017) 13:85–99. doi: 10.7150/ijbs.17390
17. Krzywinski M, Schein J, Birol I, Connors J, Gascoyne R, Horsman D, et al. Circos: an information aesthetic for comparative genomics. Genome Res. (2009) 19:1639–45. doi: 10.1101/gr.092759.109
18. Ashburner M, Ball CA, Blake JA, Botstein D, Butler H, Cherry JM, et al. Gene ontology: tool for the unification of biology. Nat Genet. (2000) 25:25–9. doi: 10.1038/75556
19. Kanehisa M, Furumichi M, Tanabe M, Sato Y, Morishima K. KEGG: new perspectives on genomes, pathways, diseases and drugs. Nucleic Acids Res. (2017) 45:D353–61. doi: 10.1093/nar/gkw1092
20. Huang da W, Sherman BT, Lempicki RA. Systematic and integrative analysis of large gene lists using DAVID bioinformatics resources. Nat Protoc. (2009) 4:44–57. doi: 10.1038/nprot.2008.211
21. Huang da W, Sherman BT, Lempicki RA. Bioinformatics enrichment tools: paths toward the comprehensive functional analysis of large gene lists. Nucleic Acids Res. (2009) 37:1–13. doi: 10.1093/nar/gkn923
22. Zhou Y, Zhou B, Pache L, Chang M, Khodabakhshi AJ, Tanaseichuk O, et al. Metascape provides a biologist-oriented resource for the analysis of systems-level datasets. Nat Commun. (2019) 10:1523. doi: 10.1038/s41467-019-09234-6
23. Subramanian A, Tamayo P, Mootha VK, Mukherjee S, Ebert BL, Gillette MA, et al. Gene set enrichment analysis: a knowledge-based approach for interpreting genome-wide expression profiles. Proc Natl Acad Sci USA. (2005) 102:15545–50. doi: 10.1073/pnas.0506580102
24. Mootha VK, Lindgren CM, Eriksson KF, Subramanian A, Sihag S, Lehar J, et al. PGC-1alpha-responsive genes involved in oxidative phosphorylation are coordinately downregulated in human diabetes. Nat Genet. (2003) 34:267–73. doi: 10.1038/ng1180
25. Szklarczyk D, Franceschini A, Wyder S, Forslund K, Heller D, Huerta-Cepas J, et al. STRING v10: protein-protein interaction networks, integrated over the tree of life. Nucleic Acids Res. (2015) 43:D447–52. doi: 10.1093/nar/gku1003
26. Smoot ME, Ono K, Ruscheinski J, Wang PL, Ideker T. Cytoscape 2.8: new features for data integration and network visualization. Bioinformatics. (2011) 27:431–2. doi: 10.1093/bioinformatics/btq675
27. Bader GD, Hogue CW. An automated method for finding molecular complexes in large protein interaction networks. BMC Bioinformatics. (2003) 4:2. doi: 10.1186/1471-2105-4-2
28. Chin CH, Chen SH, Wu HH, Ho CW, Ko MT, Lin CY. cytoHubba: identifying hub objects and sub-networks from complex interactome. BMC Syst Biol. (2014) 8(Suppl. 4):S11. doi: 10.1186/1752-0509-8-S4-S11
29. Davis AP, Grondin CJ, Johnson RJ, Sciaky D, King BL, McMorran R, et al. The comparative toxicogenomics database: update (2017). Nucleic Acids Res. (2017) 45:D972–8. doi: 10.1093/nar/gkw838
30. Chiang CE, Okumura K, Zhang S, Chao TF, Siu CW, Wei LT, et al. 2017 consensus of the Asia Pacific heart rhythm society on stroke prevention in atrial fibrillation. J Arrhythm. (2017) 33:345–67. doi: 10.1016/j.joa.2017.05.004
31. Meng LB, Shan MJ, Qiu Y, Qi R, Yu ZM, Guo P, et al. TPM2 as a potential predictive biomarker for atherosclerosis. Aging. (2019) 11:6960–82. doi: 10.18632/aging.102231
32. Si W, Shen J, Du C, Chen D, Gu X, Li C, et al. A miR-20a/MAPK1/c-Myc regulatory feedback loop regulates breast carcinogenesis and chemoresistance. Cell Death Differ. (2018) 25:406–20. doi: 10.1038/cdd.2017.176
33. Xu M, Zhou K, Wu Y, Wang L, Lu S. Linc00161 regulated the drug resistance of ovarian cancer by sponging microRNA-128 and modulating MAPK1. Mol Carcinog. (2019) 58:577–87. doi: 10.1002/mc.22952
34. Yang Y, Li XJ, Li P, Guo XT. MicroRNA-145 regulates the proliferation, migration and invasion of human primary colon adenocarcinoma cells by targeting MAPK1. Int J Mol Med. (2018) 42:3171–80. doi: 10.3892/ijmm.2018.3904
35. Wang Y, Cheng K, Zhou W, Liu H, Yang T, Hou P, et al. miR-141-5p regulate ATF2 via effecting MAPK1/ERK2 signaling to promote preeclampsia. Biomed Pharmacother. (2019) 115:108953. doi: 10.1016/j.biopha.2019.108953
36. Mali V, Haddox S, Belmadani S, Matrougui K. Essential role for smooth muscle cell stromal interaction molecule-1 in myocardial infarction. J Hypertens. (2018) 36:377–86. doi: 10.1097/HJH.0000000000001518
37. Li P, Teng F, Gao F, Zhang M, Wu J, Zhang C. Identification of circulating microRNAs as potential biomarkers for detecting acute ischemic stroke. Cell Mol Neurobiol. (2015) 35:433–47. doi: 10.1007/s10571-014-0139-5
38. Huang S, Lv Z, Guo Y, Li L, Zhang Y, Zhou L, et al. Identification of blood let-7e-5p as a biomarker for ischemic stroke. PLoS ONE. (2016) 11:e0163951. doi: 10.1371/journal.pone.0163951
39. Eyileten C, Wicik Z, De Rosa S, Mirowska-Guzel D, Soplinska A, Indolfi C, et al. MicroRNAs as diagnostic and prognostic biomarkers in ischemic stroke-A comprehensive review and bioinformatic analysis. Cells. (2018) 7:249. doi: 10.3390/cells7120249
40. Hayashi Y, Toyomasu Y, Saravanaperumal SA, Bardsley MR, Smestad JA, Lorincz A, et al. Hyperglycemia increases interstitial cells of cajal via MAPK1 and MAPK3 signaling to ETV1 and KIT, leading to rapid gastric emptying. Gastroenterology. (2017) 153:521–35.e20. doi: 10.1053/j.gastro.2017.04.020
41. Yuan Y, Zhao J, Gong Y, Wang D, Wang X, Yun F, et al. Autophagy exacerbates electrical remodeling in atrial fibrillation by ubiquitin-dependent degradation of L-type calcium channel. Cell Death Dis. (2018) 9:873. doi: 10.1038/s41419-018-0860-y
42. Hou K, Xu D, Li F, Chen S, Li Y. The progress of neuronal autophagy in cerebral ischemia stroke: mechanisms, roles and research methods. J Neurol Sci. (2019) 400:72–82. doi: 10.1016/j.jns.2019.03.015
43. Wang Y, Li J, Du C, Zhang L, Zhang Y, Zhang J, et al. Upregulated circular RNA circ-UBE2D2 predicts poor prognosis and promotes breast cancer progression by sponging miR-1236 and miR-1287. Transl Oncol. (2019) 12:1305–13. doi: 10.1016/j.tranon.2019.05.016
44. Chiang MH, Chen LF, Chen H. Ubiquitin-conjugating enzyme UBE2D2 is responsible for FBXW2 (F-box and WD repeat domain containing 2)-mediated human GCM1 (glial cell missing homolog 1) ubiquitination and degradation. Biol Reprod. (2008) 79:914–20. doi: 10.1095/biolreprod.108.071407
45. Geisler S, Vollmer S, Golombek S, Kahle PJ. The ubiquitin-conjugating enzymes UBE2N, UBE2L3 and UBE2D2/3 are essential for parkin-dependent mitophagy. J Cell Sci. (2014) 127:3280–93. doi: 10.1242/jcs.146035
46. Lee JH, Jung S, Park WS, Choe EK, Kim E, Shin R, et al. Prognostic nomogram of hypoxia-related genes predicting overall survival of colorectal cancer-analysis of TCGA database. Sci Rep. (2019) 9:1803. doi: 10.1038/s41598-018-38116-y
47. Peng H, Yang J, Li G, You Q, Han W, Li T, et al. Ubiquitylation of p62/sequestosome1 activates its autophagy receptor function and controls selective autophagy upon ubiquitin stress. Cell Res. (2017) 27:657–74. doi: 10.1038/cr.2017.40
48. Peng H, Yang F, Hu Q, Sun J, Peng C, Zhao Y, et al. The ubiquitin-specific protease USP8 directly deubiquitinates SQSTM1/p62 to suppress its autophagic activity. Autophagy. (2020) 16:698–708. doi: 10.1080/15548627.2019.1635381
49. Graham SH, Liu H. Life and death in the trash heap: the ubiquitin proteasome pathway and UCHL1 in brain aging, neurodegenerative disease and cerebral ischemia. Ageing Res Rev. (2017) 34:30–8. doi: 10.1016/j.arr.2016.09.011
50. Oh SH, Kim OJ, Shin DA, Song J, Yoo H, Kim YK, et al. Alteration of immunologic responses on peripheral blood in the acute phase of ischemic stroke: blood genomic profiling study. J Neuroimmunol. (2012) 249:60–5. doi: 10.1016/j.jneuroim.2012.04.005
51. Inoue N, Okamura T, Kokubo Y, Fujita Y, Sato Y, Nakanishi M, et al. LOX index, a novel predictive biochemical marker for coronary heart disease and stroke. Clin Chem. (2010) 56:550–8. doi: 10.1373/clinchem.2009.140707
52. Lattanzi S, Cagnetti C, Pulcini A, Morelli M, Maffei S, Provinciali L, et al. The P-wave terminal force in embolic strokes of undetermined source. J Neurol Sci. (2017) 375:175–8. doi: 10.1016/j.jns.2017.01.063
Keywords: atrial fibrillation, stroke, bioinformatic technology, differentially expressed genes, hub genes
Citation: Zhang Y, Meng L, Hao M, Yang J and Zou T (2020) Identification of Co-expressed Genes Between Atrial Fibrillation and Stroke. Front. Neurol. 11:184. doi: 10.3389/fneur.2020.00184
Received: 13 December 2019; Accepted: 25 February 2020;
Published: 24 March 2020.
Edited by:
Maurizio Acampa, Siena University Hospital, ItalyReviewed by:
Simona Lattanzi, Marche Polytechnic University, ItalyJoylene Elisabeth Siland, University of Groningen, Netherlands
Copyright © 2020 Zhang, Meng, Hao, Yang and Zou. This is an open-access article distributed under the terms of the Creative Commons Attribution License (CC BY). The use, distribution or reproduction in other forums is permitted, provided the original author(s) and the copyright owner(s) are credited and that the original publication in this journal is cited, in accordance with accepted academic practice. No use, distribution or reproduction is permitted which does not comply with these terms.
*Correspondence: Tong Zou, em91dG9uZzIwMDFAMTYzLmNvbQ==; enlmMTIzNjU0Nzg5QHllYWgubmV0
†These authors have contributed equally to this work