- 1Department of Rehabilitation Medicine, The Affiliated Suzhou Hospital of Nanjing Medical University, Suzhou, China
- 2Center for Excellence in Brain Science and Intelligence Technology, Institute of Neuroscience, Chinese Academy of Sciences, Shanghai, China
- 3Department of Rehabilitation Medicine, Kunshan Rehabilitation Hospital, Suzhou, China
- 4College School of Acupuncture-Moxibustion and Tuina, School of Health Preservation and Rehabilitation, Nanjing University of Chinese Medicine, Nanjing, China
Background: Electroencephalography (EEG) has become an indispensable tool in stroke research for real-time monitoring of neural activity, prognosis prediction, and rehabilitation support. In recent decades, EEG applications in stroke research have expanded, particularly in areas like brain-computer interfaces (BCI) and neurofeedback for motor recovery. However, a comprehensive analysis of research trends in this domain is currently unavailable.
Methods: The study collected data from the Web of Science Core Collection database, selecting publications related to stroke and EEG from 2005 to 2024. Visual analysis tools such as VOSviewer and CiteSpace were utilized to build knowledge maps of the research field, analyzing the distribution of publications, authors, institutions, journals, and collaboration networks. Additionally, co-occurrence, clustering, and burst detection of keywords were analyzed in detail.
Results: A total of 2,931 publications were identified, indicating a consistent increase in EEG research in stroke, with significant growth post-2017. The United States, China, and Germany emerged as the leading contributors, with high collaboration networks among Western institutions. Key research areas included signal processing advancements, EEG applications in seizure risk and consciousness disorder assessment, and EEG-driven rehabilitation techniques. Notably, recent studies have focused on integrating EEG with machine learning and multimodal data for more precise functional evaluations.
Conclusion: The findings reveal that EEG has evolved from a diagnostic tool to a therapeutic support platform in the context of stroke care. The advent of deep learning and multimodal integration has positioned EEG for expanded applications in personalized rehabilitation. It is recommended that future studies prioritize interdisciplinary collaboration and standardized EEG methodologies in order to facilitate clinical adoption and enhance translational potential in stroke management.
1 Introduction
Stroke is a leading cause of disability and mortality worldwide, classified mainly into ischemic stroke and hemorrhagic stroke (1). Stroke results in localized or widespread neurological impairment, frequently accompanied by motor, cognitive (e.g., aphasia, executive dysfunction, and memory deficits), and swallowing dysfunctions (2, 3). Specifically, cognitive impairments vary depending on the lesion location and severity, with common manifestations including visuospatial neglect (4) and attention disorder (5). These deficits not only undermine patients’ functional independence but also impose long-term challenges for rehabilitation and quality of life. With the ongoing trend of population aging, the incidence of stroke is expected to continue rising, placing an even heavier burden on healthcare systems globally (6, 7). Traditional stroke diagnosis and evaluation rely heavily on imaging techniques such as magnetic resonance imaging (MRI) and computed tomography (CT), which are highly sensitive and specific in displaying structural brain abnormalities, particularly in the acute phase (8, 9). Furthermore, functional scales such as the NIH Stroke Scale and the Fugl-Meyer Assessment are employed to quantify functional deficits (10, 11). However, these methods are primarily oriented toward the detection of structural changes and may prove inadequate for the real-time monitoring of functional dynamics in stroke patients. Functional MRI (fMRI) and positron emission tomography (PET) can be used to assess changes in brain function but are often limited by high costs, restricting broader application (12, 13). In contrast, EEG is a non-invasive tool for monitoring brain function. It provides high temporal resolution data on neural activity by recording the electrophysiological activity of the brain (14). Furthermore, EEG is a straightforward and cost-effective method of data collection. EEG is particularly advantageous in the diagnosis and evaluation of stroke, offering the dual benefit of real-time monitoring of neurological changes in stroke patients and the identification of specific patterns of brain electrical activity through quantitative EEG (qEEG). This allows for the assessment of potential functional recovery and seizure risk (15). Post-stroke patients undergoing resting-state EEG often exhibit increased delta/theta power and decreased alpha/beta power, which correlate with motor and cognitive deficits. Functional connectivity analysis further reveals disrupted network topology in the affected hemisphere, reflecting impaired inter-regional communication (4, 16, 17). Post-stroke sleep architecture often shows reduced rapid-eye-movement sleep and increased sleep fragmentation, which correlates with poor recovery. Sleep spindles and slow-wave activity may serve as biomarkers for neuroplasticity (18, 19). In recent years, the application of EEG in stroke research has extended into advanced fields such as brain-computer interfaces (BCI) and neurofeedback (20, 21). BCI technology is capable of decoding EEG signals, thereby enabling stroke patients to control external devices through brain activity (20, 22). Neurofeedback training employs real-time EEG feedback to assist patients in self-regulating brain states, thereby promoting neuroplasticity and functional restoration (23). The combination of BCI and neurofeedback has demonstrated potential as a means of providing personalized training solutions for stroke rehabilitation. In light of these substantial applications, EEG research in the context of stroke has become of considerable value. This study employs bibliometric methods to conduct a systematic analysis of EEG research in stroke over the past 20 years. The analysis utilizes VOSviewer and CiteSpace to create a comprehensive knowledge map of the field, thereby uncovering the current state, key hotspots, and future trends. This knowledge map serves to inform and guide subsequent research.
2 Materials and methods
2.1 Data source and collection
The primary data for the bibliometric analysis were obtained from the Science Citation Index Expanded (SCI-Expanded) and Social Sciences Citation Index databases (SSCI) within the Web of Science Core Collection database (WoSCC). The data retrieval strategy was summarized as follows: # 1: TS = stroke; #2: TS = (Electroencephalography OR EEG OR Electroencephalogram∗); the ultimate dataset: #1 AND #2. The utilization of a truncation symbol, “∗,” proved an effective means of preventing missed detections and enhancing retrieval efficacy. The study included only English-language studies. The time of search period was between January 1, 2005 and December 31, 2024. The search strategy is depicted in Figure 1. To minimize the potential bias from routine database updates, the literature search was conducted on a fixed date. A total of 2,931 productions were retrieved, including both reviews and research articles. To ensure the clarity and accuracy of the results and conclusions, we manually screened the 2,931 publications and categorized them into two groups: (A) “EEG in acute stroke and its early complications” and (B) “EEG in neurological rehabilitation.” Category A contained 1,207 articles, while category B included 1724 articles. The data will be stored in three separate folders: “Dataset 1” (which contains all literature), “Dataset 2” (containing only category A literature), and “Dataset 3” (containing only category B literature). Upon completion of the retrieval process, the data were saved as complete records and cited references. The articles were then extracted and exported in “Plain text file” formats.
2.2 Bibliometric analysis
The articles meeting the inclusion criteria were exported as a plain text file named “download_xxx.txt,” containing complete records and cited references. These files were imported into VOSviewer 1.6.19 and CiteSpace 6.2.R2 to construct visual knowledge maps. Additionally, Excel was used for chart creation and descriptive statistical analyses. The VOSviewer parameters were configured as follows: the normalization method was set to “association strength,” with minimum thresholds for countries/regions, institutions, authors and journals set at 5, 10, 7 and 10 publications, respectively. Keyword occurrence frequency was also considered, with a minimum threshold of 20. In CiteSpace, the analysis covered the period from January 2005 to December 2024, with a one-year time slice. Node types included keywords, and the g-index selection criteria were set to k = 25 per slice. The pruning options used were pathfinder, sliced networks, and merged networks, with all other settings left at their default values. In this study, we first analyzed the number of papers from countries, institutions, authors and journals based on Dataset 1 to summarize the current status of EEG research in stroke. We then used Dataset 2 and Dataset 3 to analyze keyword co-occurrence, keyword clustering, and emergent keywords, in order to identify current research hotspots and explore the frontiers and emerging trends in this field.
2.3 Annual publications and citations
The annual publication volume is a principal indicator for gauging research interest and predicting future dynamics in a field (24). The study encompassed 2,931 publications, comprising 2,610 original research articles (89%) and 321 review articles (11%). The total citations (TC) were 75,437. The average citation per publication (ACPP) was 25.73, and the h-index was 109. Figure 2A depicts the trajectory of annual publication volume (depicted on the left vertical axis in terms of the number of articles) and citation frequency (depicted on the right vertical axis) in the field of EEG research in stroke from 2005 to 2024. The figure illustrates an overall upward trend. The research trajectory can be delineated into two distinct phases. From 2005 to 2016, both the publication volume and the citation frequency exhibited a gradual increase. This early phase reflects the foundational work being done in the field. The moderate rise indicates a steady expansion in research and a corresponding increase in academic attention. During this period, EEG research in stroke likely laid the groundwork for more targeted clinical and experimental investigations. From 2017 onward, the field entered a phase of rapid growth, with both publication volume and citation frequency rising sharply, reaching a peak in 2024. The substantial increase in publications indicates that EEG research in stroke has gained significant traction, attracting an increasing number of researchers and funding. The rise in citations signifies a more extensive and profound integration of these studies within the broader stroke research and neurorehabilitation communities.
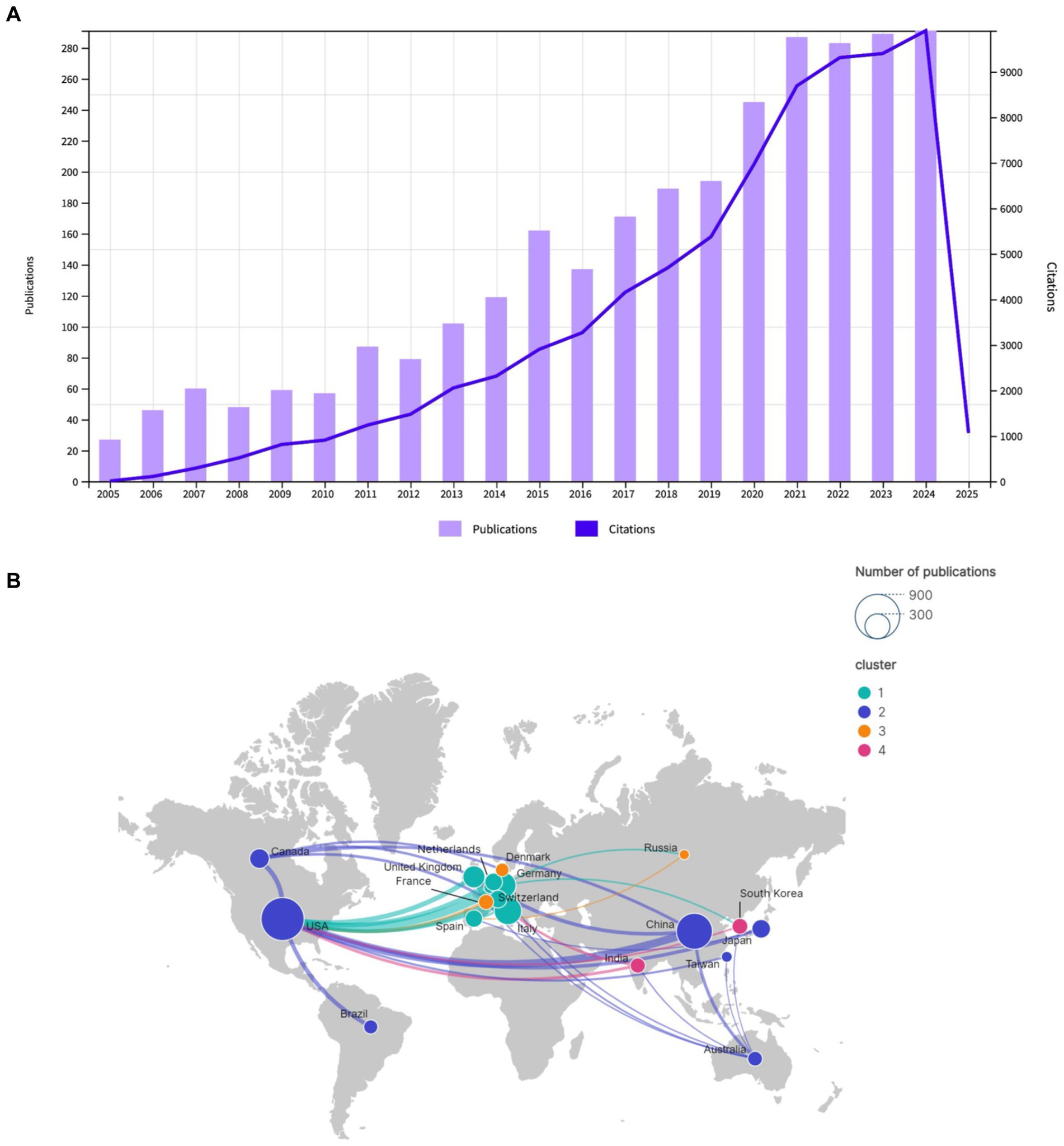
Figure 2. (A) Annual publications and citations trend chart for Dataset 1. (B) Geographical distribution and cooperation of publications Dataset 1.
2.4 Distribution of countries/regions and institutions
A total of 2,931 publications were published by 92 countries and 3,539 institutions. A total of 57 countries and 139 institutions published at least five and ten articles, respectively, in this field. In terms of publication volume, the top five countries were the United States (778 publications), China (545 publications), Italy (316 publications), Germany (273 publications), and England (184 publications), as illustrated in Table 1. As illustrated in Figure 2B, the geographical distribution of cooperative endeavors among nations is depicted. The nodes, which represent countries, are sized according to the number of publications they have received. The upper right corner of the figure demonstrates the node sizes for publication counts of 300 and 900. The presence of lines connecting the nodes signifies cooperative interactions between countries, with the thickness of the lines denoting the frequency of collaboration. The map is color-coded into clusters and includes both the number of publications from each country as well as the strength of their collaborations. The map divides countries into four different clusters based on their collaborative relationships. Cluster 1: This cluster primarily includes countries/regions from Europe, such as Germany, England, Spain, Switzerland, Italy, and Netherlands. Cluster 2: This cluster includes countries/regions such as the United States, China, Canada, Japan, Australia, Brazil, and Taiwan. Cluster 3: This cluster includes countries/regions like France, Denmark, and Russia. Cluster 4: This cluster includes countries/regions like South Korea and India.
Figure 3A depicts the institutional collaboration map. Table 2 provides a detailed overview of the top institutions involved in EEG research in stroke, showing their total number of publications, TC, TLS, ACPP, and country of origin. The top 10 institutions by publication volume in EEG research in stroke show a mix of global leaders from the United States, China, and Europe, reflecting the international prominence of these universities in advancing research in this field. As illustrated in Table 2, the University of Tübingen in Germany occupies the preeminent position with 64 publications, closely followed by institutions from China, including Capital Medical University (52 publications), Fudan University (42 publications), and Shanghai Jiao Tong University (41 publications). This observation highlights the substantial contributions of Chinese institutions to the scholarly landscape. Other prominent players include Northwestern University, University of Pittsburgh, and Harvard Medical School, all from the United States, with 42, 40, and 35 publications, respectively. These institutions from the United States are widely recognized for their impactful research and often lead the field in terms of citation frequency. Notably, Aalborg University demonstrates a particularly high citation impact, with 1,205 citations, indicating that their research has gained significant academic recognition.
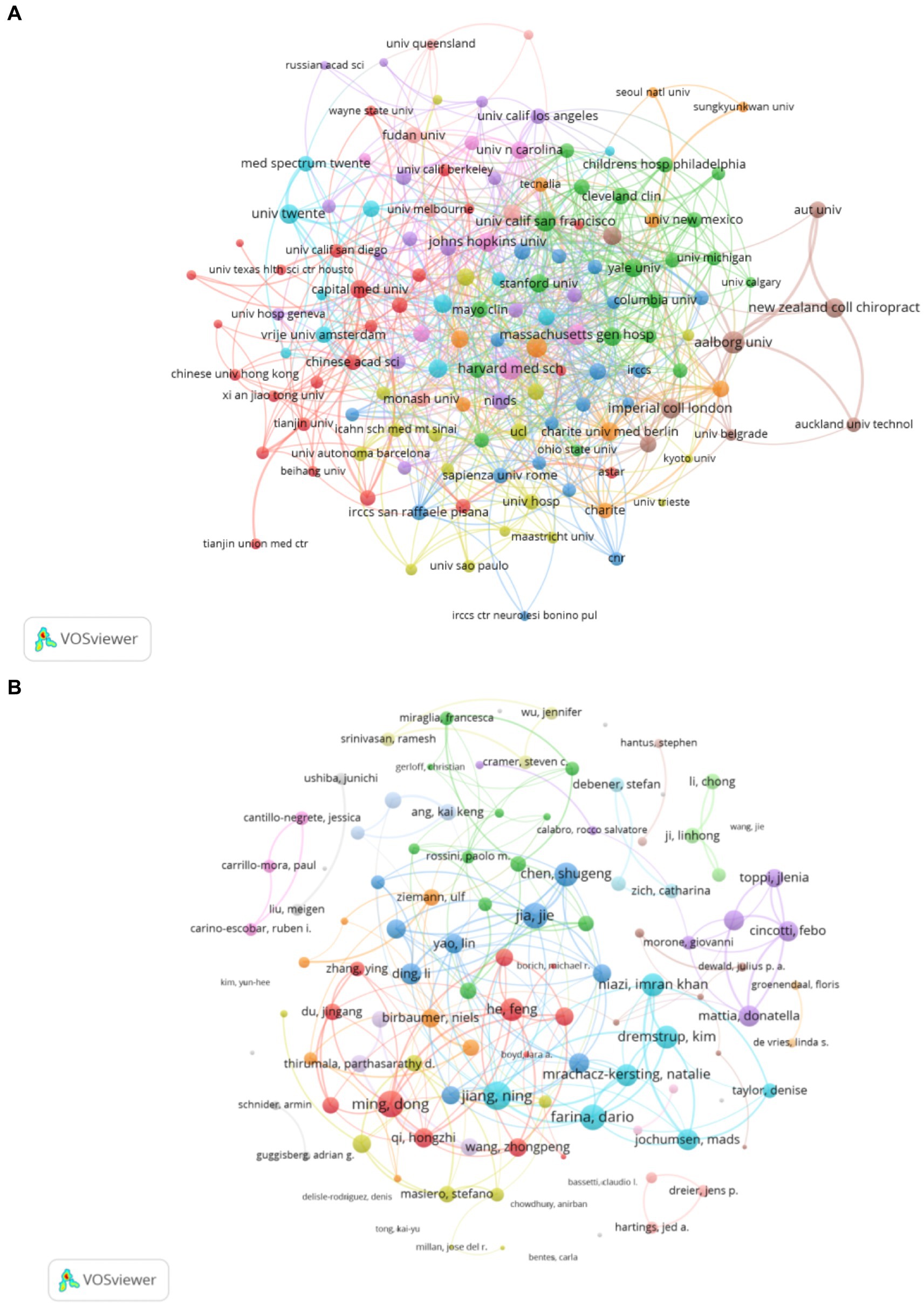
Figure 3. (A) Collaborative network knowledge map of institution Dataset 1. (B) Collaborative network knowledge map of author Dataset 1.
2.5 Analysis of authors
A total of 13,234 authors contributed to this field between 2005 and 2024. Of these, 112 authors published at least seven articles with over 100 citations. The three most prolific authors in terms of publication volume were Birbaumer, N (29 publications), Jia, J (27 publications), and Ming, D (25 publications), as illustrated in Table 3. Professor Birbaumer, N from Germany is the most prolific researcher in the field, with the highest number of publications, TC, ACPP, and H-index. His research output is concentrated in the early period of his career, making him the founder and most influential scholar in the field. Among the top 10 high-impact authors, three are from China. Their publications are concentrated in recent years, and their collaborations are limited, with close collaboration within their teams but relatively few collaborations with teams outside their institutions, particularly across borders, as shown in Figure 3B. Notably, Van Putten, MJAM, a researcher from the Netherlands, has attained a commendable ACPP score of 49.85, underscoring the substantial academic recognition of his contributions to the field of EEG research in stroke.
2.6 Analysis of journals
A total of 2,931 publications were retrieved and published across 641 journals. Among the retrieved publications, 60 journals had at least 10 publications and over 100 citations, as illustrated in Figure 4. Table 4 presents the 10 journals with the highest TC. The top five journals in terms of citation frequency are Clinical Neurophysiology (3,191 citations), Journal of Neural Engineering (3,033 citations), Neuroimage (2,676 citations), Frontiers in Neuroscience (2,325 citations), and Sensors (2,251 citations). These journals are all ranked in the first and second quartiles by the Journal Citation Reports (JCR), which indicates that they are of high research quality and influence. Among the top 10 journals, Brain has the highest impact factor (IF), and despite a relatively lower publication count, it has the highest ACPP, which serves to underscore its academic prestige and broad influence in neuroscience.
2.7 Analysis of keywords
2.7.1 Analysis of keyword co-occurrence
Keywords are a high-level summary of the topic and content of the article. An analysis of keyword co-occurrence can reflect the hotspot and trend of research in the field (25). This study analyzes the keyword co-occurrence patterns across two distinct categories of EEG research in stroke. Table 5 shows the top 20 keywords from both Dataset 2 and Dataset 3 ranked by TLS. In Dataset 2 which includes 1,207 articles focused on “EEG in acute stroke and its early complications,” the keyword co-occurrence network (Figure 5A) highlights terms like “stroke,” “EEG,” and “epilepsy” as central nodes emphasizing the focus on EEG applications for managing acute stroke complications such as seizures and non-convulsive status epilepticus. Figure 1 showcases a total of 83 keywords with a minimum co-occurrence frequency of seven. Other significant terms such as “ischemic stroke,” “MRI,” and “intracerebral hemorrhage” reflect the intersection of EEG studies with imaging and other neurological complications. This network illustrates how EEG is used to monitor and manage the early consequences of stroke indicating the importance of EEG in understanding and addressing stroke-induced brain changes during the acute phase. Dataset 3 which includes 1,724 articles focusing on “EEG in neurological rehabilitation,” shows a different pattern in its keyword co-occurrence network (Figure 5B). This figure displays a network of 137 keywords all of which appear with a minimum frequency of seven. These central terms like “brain-computer interface (BCI),” “motor imagery (MI),” and “neurorehabilitation” are prominent reflecting the shift in focus toward using EEG in stroke recovery particularly in enhancing rehabilitation strategies through BCI systems. Keywords such as “functional connectivity,” “training,” and “rehabilitation” are tightly linked indicating the growing interest in leveraging EEG to promote motor recovery and brain plasticity in the chronic phase of stroke. Moreover terms like “virtual reality (VR)” suggest an expanding interest in integrating advanced technologies with EEG-based rehabilitation. Comparing the keyword co-occurrence networks of the two categories reveals clear differences: acute stroke research is primarily concerned with monitoring and managing immediate stroke-related complications while the rehabilitation category emphasizes long-term recovery and functional improvement through EEG-based interventions.
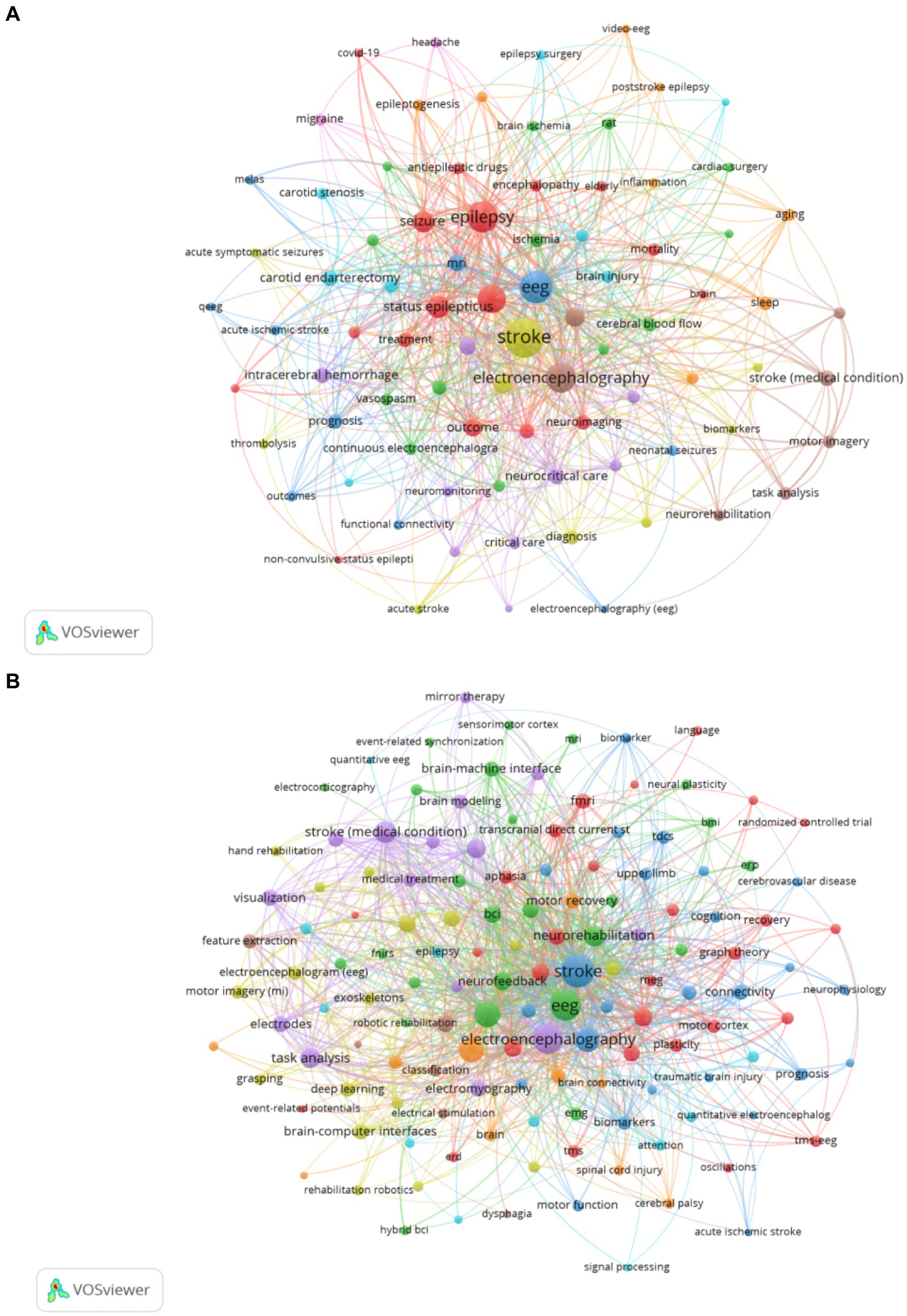
Figure 5. (A) Keyword co-occurrence network knowledge map for Dataset 2. (B) Keyword co-occurrence network knowledge map for Dataset 3.
2.7.2 Analysis of keyword clustering
Analysis of keywords clustering is to categorize closely related keywords, which can reveal the hotspot of research in the field (26). The collected data were imported into CiteSpace for keyword clustering analysis, the smaller the cluster number, the more keywords the cluster contains. Modularity Q is a measure of the efficacy of clustering, with a range from 0 to 1. A value approaching 1 indicates a high degree of connectivity within clusters (14). For Dataset 2, which focuses on acute stroke and its early complications, the analysis revealed a Modularity Q of 0.7094, indicating substantial network modularity and high clustering quality, as shown in Figure 6A. The LLR clustering method identified 19 distinct clusters, each representing a distinct area of research, which were subsequently labeled with descriptive terms, including #0 carotid endarterectomy, #1 animal models, #2 cardiac surgery, #3 antiepileptic drug, #4 cerebrovascular disease, #5 stroke, #6 functional connectivity, #7 status epilepticus, #8 delayed cerebral ischemia, #9 spreading depression, #10 cognition, #11 biomedical signal processing, #12 stroke-related seizures, #13 temporal lobe epilepsy, #14 cerebral blood flow, #15 cortical excitability, #16 medulla-oblongata, #17 stroke-like episodes, #18 cortical infarction. Figure 6B presents the clustering results for Dataset 3, which is centered around EEG research in neurorehabilitation of stroke, also exhibited strong clustering results with a Modularity Q of 0.7791. The LLR clustering method was employed to identify distinct 19 clusters, including #0 quantitative electroencephalography, #1 stroke, #2 transcranial magnetic stimulation, #3 brain-computer interface, #4 functional connectivity, #5 ischemic stroke, #6 motor imagery, #7 carotid endarterectomy, #8 upper extremity, #9 feature extraction, #10 traumatic brain injury, #11 case report, #12 sensorimotor integration, #13 brain activity, #14 transcranial direct current stimulation, #15 cerebrovascular accident, #16 corticomuscular coherence, #17 brain plasticity, #18 seizures.
2.7.3 Analysis of keyword burst
Keyword burst analysis has been demonstrated to reveal the areas that have received the most attention within a specific timeframe thereby identifying the emerging research frontiers (26). Figure 7 illustrates the top 25 burst keywords. The “Begin” and “End” columns indicate the timeframe of the keyword burst while “strength” denotes the intensity of the burst. For Dataset 2 (analyzed with a 1-year duration parameter) Figure 7 displays the top 25 burst keywords. The earliest detected burst corresponds to “blood-flow,” which also exhibits the longest sustained burst period. Notably “therapeutic hypothermia” demonstrates the highest burst strength. The keywords “functional connectivity,” “guidelines,” “acute symptomatic seizures,” “patterns,” “score,” “connectivity,” “acute ischemic stroke,” and “outcm” are still experiencing bursts. For Dataset 3 Figure 8 reveals distinct patterns: “activation” emerges as the earliest burst keyword while “cortex” maintains the most prolonged burst duration and the keyword “task analysis” registers the strongest burst intensity. The following keywords are still experiencing bursts: “upper limb,” “feature extraction,” “stroke,” “task analysis,” “machine learning,” “deep learning,” “network,” and “stimulation.”
3 Discussion
3.1 Analysis of current research status
This study is the first to conduct a comprehensive bibliometric and visual analysis of EEG research in the field of stroke from 2005 to 2024, revealing key trends and developments. Over the past two decades, the number of publications and the frequency of citations in this area have exhibited a gradual increase. It is noteworthy that from 2000 to 2024, the annual publication volume remained above 200 publications, indicating a sustained growth trajectory in the field. This surge is indicative of the growing recognition of EEG as an essential tool in both the acute management of stroke and neurorehabilitation. The rising number of publications and the increased citation impact serve as evidence of this growing recognition.
At the national level, the analysis of publication volume and collaboration strength highlights the leading role of the United States in EEG research in stroke. Its high number of publications, citations, and collaborations with a range of countries position it as a central hub in this field. This global leadership reflects the United States’ substantial investment in neuroscience and neurotechnology, fostering an environment that supports innovation and widespread dissemination of research findings. The robust international collaboration network, particularly with countries like Germany, Italy, and England, strengthens the global impact of the United States research, accelerating scientific progress in EEG applications for stroke. China’s large volume of publications indicates a growing presence in the filed, but its relatively lower citation count and international collaboration strength suggest that its research may be more domestically focused. Countries such as Germany, Italy, and England also play pivotal roles in advancing EEG research. Germany’s high ACPP indicates that its research is impactful, shaping key innovations in the field. Meanwhile, Switzerland and Canada, though publishing fewer papers, have demonstrated strong citation impacts, reinforcing the idea that quality research can have a disproportionate effect on the global scientific community. Switzerland’s high publication count per million people further emphasizes the significant contributions of smaller nations in advancing specialized fields. As the field of EEG research in stroke continues to evolve, fostering deeper international collaboration, especially between countries with differing research capacities, will be essential for accelerating advancements.
At the institutional level, the majority of research institutions are situated in developed Western countries, with universities representing the primary contributors. This reflects the field’s reliance on economic support and experimental facilities. The University of Tübingen in Germany is the leading institution in terms of publication volume and citation frequency, exerting considerable influence, particularly in the field of BCI research. One of their most highly cited studies provides a comprehensive overview of the clinical applications of invasive and non-invasive EEG-based BCI technologies in direct brain communication and post-stroke motor recovery for paralyzed patients, demonstrating significant potential in both animal and human models (27, 28). The University of Twente in the Netherlands, despite publishing fewer papers, has a high citation frequency, indicating that their research is widely recognized for its quality. Their research has focused on qEEG technology, which has advanced the monitoring of prognosis and therapeutic responses in patients who have suffered a stroke or anoxic coma. This has established a robust foundation for the application of EEG in neuroscience (29–31). While the international collaboration network is robust (as shown in Figure 2B), our analysis shows that many research institutions collaborate predominantly with national partners (as shown in Figure 3A), with fewer instances of extensive cross-border collaboration. This suggests that geographical proximity remains an important factor influencing patterns of collaboration EEG research in stroke.
At the level of the author, Professor Birbaumer, N from Germany is one of the most prominent scholars in the field of BCI. His team has developed techniques for direct communication between the brain and external devices via EEG and other neural signals, with the objective of assisting individuals with severe paralysis and atresia syndrome to communicate with the outside world. Patients are able to control computer cursors, letter boards, or robotic arms through brain signals, thereby significantly advancing the field of BCI technology (32–34). In recent years, Professor Jia, J has been at the forefront of research utilizing BCI technology to enhance stroke rehabilitation. By integrating connectivity network patterns with spatiotemporal analysis, she has optimized EEG feature selection, thereby enhancing the efficacy of BCI applications in rehabilitation training (35, 36). Moreover, she has utilized a combination of BCI and functional electrical stimulation to markedly enhance motor function in individuals who have experienced a chronic stroke (37). Her research also encompasses traditional Chinese medicine techniques, such as electroacupuncture, and their regulatory effects on resting-state networks in stroke patients, as well as the potential clinical benefits of such techniques in rehabilitation (38). Professor Jiang N, another Chinese scholar, has also made significant contributions to the development of a single-trial detection system based on movement-related cortical potentials for BCI applications in gait initiation through extensive international collaboration (39). By interpreting patients’ motor intentions in real time through EEG signals, his work provides a basis for rehabilitative interventions for gait-impaired patients.
In terms of journal distribution, most of the journals with high publication volume and citation frequency are high-quality journals, such as Clinical Neurophysiology, Neurology and Brain. These journals not only provide theoretical and experimental support for EEG research in the field of stroke, but also demonstrate the academic maturity of research results in this field. As research continues to progress, future publications may increasingly be concentrated in these high-impact journals, thus creating a virtuous cycle. The platform role of these journals not only facilitates the dissemination of research findings, but also encourages further innovation within the field.
3.2 Analysis of research hotspots and trends
This study provides a comprehensive analysis of the keyword trends in EEG research in stroke, revealing critical insights into the evolving landscape of this field. Through the analysis of keyword co-occurrence, clustering, and burst patterns, we have identified key research hotspots, emerging trends, and shifts in the focus of EEG applications for acute stroke management and neurological rehabilitation. These findings not only highlight the current state of research but also offer directions for future investigation.
3.2.1 EEG research in acute stroke and early complications
EEG research in acute stroke primarily focuses on the early identification and management of complications such as epilepsy and disorders of consciousness. The keyword co-occurrence analysis for Dataset 2 reveals a dominant emphasis on using EEG for monitoring post-stroke complications, especially seizures and non-convulsive status epilepticus, which are crucial for early intervention. Terms such as “stroke,” “EEG,” and “epilepsy” prominently feature in the research landscape, reflecting the clinical focus on utilizing EEG to understand and manage stroke-induced brain changes.
The analysis of keywords highlights epilepsy and seizures as central themes in acute stroke research. Post-stroke epilepsy is significantly associated with adverse outcomes and elevated mortality rates (40). QEEG can assist in identifying the typical EEG patterns associated with stroke (41). Non-convulsive seizures are frequently unrecognized clinically, as standard observations may prove inadequate for detecting these anomalies. Nevertheless, EEG monitoring is capable of capturing essential electrophysiological changes. Non-convulsive seizures are frequently unrecognized clinically, as standard observations may prove inadequate for detecting these anomalies (42). However, EEG monitoring can effectively capture essential electrophysiological changes. Post-stroke epilepsy in EEG typically manifests as focal or generalized slowing, with some cases also showing lateralized periodic discharges. Bentes et al. (43) conducted a study of long-term follow-up of patients who had experienced an anterior ischemic circulation stroke, finding that 25.2% experienced seizures within the first year, with 22.7% of these acute symptomatic seizures detected only by EEG. For instance, Bentes et al. (43) demonstrated that 64-channel EEG with synchronized video-polysomnography during the first post-stroke week captured electrographic seizures in over 20% of anterior ischemic stroke patients, with 62% of these events occurring during sleep. These findings emphasize the necessity of prolonged monitoring, as 22.7% of acute symptomatic seizures are identifiable only through EEG. Beyond seizure detection, EEG’s predictive capacity extends to acute neurological deficits. Vanderschelden et al. (44) conducted a prospective study of 50 acute stroke patients evaluated by recording of EEG at rest state. The delta-theta/alpha-beta ratio (DTABR) was calculated. Multivariable modeling revealed that while age, diabetes status, and infarct volume explained 47% of NIHSS score variance, adding contralesional DTABR enhanced prediction, achieving 60% explanatory power. These seizures can contribute to worsened neurological outcomes, increased risk of mortality, and prolonged recovery time, highlighting the importance of EEG in reducing these adverse effects by enabling timely treatment (45).
Expanding beyond epilepsy, EEG provides objective biomarkers for post-stroke consciousness disorders. Reduced prefrontal-to-motor cortical information flow, measurable via transcranial magnetic stimulation coupled with high-density EEG (TMS-EEG), correlates with impaired arousal states. Bai et al. (46) reported that patients with unresponsive wakefulness syndrome exhibit ≥40% reductions in gamma band connectivity between prefrontal and motor regions, while minimally conscious patients show disrupted prefrontal-parietal alpha coherence predictive of 6-month recovery. Such electrophysiological signatures align with spectral shifts observed in consciousness research: elevated low-frequency oscillations (delta/theta) and attenuated cross-frequency coupling reflect diminished cortical integration, whereas preserved theta-gamma phase-amplitude interactions may signify recovery potential (47, 48). While EEG excels in detecting electrophysiological anomalies, its integration with advanced neuroimaging techniques enables deeper insights into structure–function relationships In a targeted investigation of thalamic stroke, researchers employed DTI-derived fractional anisotropy (FA) maps alongside qEEG to probe microstructural and functional connectivity disruptions. Correlational analyses linked theta-band EEG power reductions to FA decreases in the cingulum bundle and corpus callosum-key components of the default mode network known to modulate resting-state theta activity. Alpha-band power further correlated with FA in cortico-thalamic circuits, supporting the “thalamocortical dysrhythmia” model of stroke-induced network dysfunction. This multimodal approach also bridged behavioral deficits with neural markers: FA reductions in the right cingulum predicted impaired spatial memory, while splenium of the corpus callosum correlated with facial recognition deficits (49). Thus, EEG in acute stroke research is not only focused on identifying early stroke-related complications but also on understanding the underlying neurological processes that influence stroke recovery, especially in critical conditions like seizures and consciousness disorders. While the current study primarily focuses on EEG applications in neural monitoring and rehabilitation, we acknowledge the importance of exploring broader physiological mechanisms, including inflammation and oxidative stress (ROS), in stroke pathology (50–53). Although EEG itself does not directly measure inflammatory markers or ROS levels, emerging research highlights indirect correlations between EEG patterns and these mechanisms. For example, post-stroke neuroinflammation can disrupt cortical excitability and functional connectivity, which may manifest as altered EEG spectral power or coherence (54–57). Additionally, oxidative stress has been linked to impaired neurovascular coupling (58–60), potentially affecting EEG-derived metrics. Future studies could integrate EEG with biomarkers (e.g., serum cytokines, ROS assays) to investigate these relationships. The increasing emphasis on real-time monitoring and the integration of EEG with other diagnostic tools, such as MRI or PET scans, has great potential in enhancing the clinical management of acute stroke patients, reducing mortality and improving recovery rates.
3.2.2 Neurological rehabilitation in stroke
EEG research in neurological rehabilitation (Dataset 3) shifts its focus from immediate stroke complications to the long-term recovery process. The analysis of keywords for this dataset highlights the growing prominence of BCI technology, MI, and neurorehabilitation, reflecting the increased integration of EEG into rehabilitation efforts aimed at improving motor function and cognitive recovery. Terms such as functional connectivity, training, and rehabilitation emphasize the growing recognition of EEG’s potential in promoting neural plasticity during stroke recovery, particularly in enhancing motor recovery through non-invasive brain-computer technologies. One of the most profound advancements in neurological rehabilitation is the use of BCI systems. MI enables patients to engage motor-related brain regions by imagining limb movements without actual physical execution, thereby promoting neural plasticity, particularly in the recovery of upper limb and hand function. BCI technology further enhances the effectiveness of MI by decoding patients’ motor intentions and translating them into commands for external devices, thereby enabling hemiplegic patients to achieve indirect motor control (61). This not only improves motor function but also helps patients maintain active engagement during rehabilitation training. Benzy et al. (62) analyzed cortical activity during MI and successfully decoded the imagined hand movement direction (left/right) in stroke patients. The patients used the phase-locking value of EEG signals to decode the direction of imagined hand movement, which then controlled a motorized arm assistive device, allowing patients to move their impaired arms in the intended direction. The combination of EEG with EMG (electromyography) has also gained attention in recent years, particularly in enhancing the precision of motor control during rehabilitation. Li et al. (63) introduced EEG–EMG hybrid systems, which combine the advantages of both EEG for motor intention detection and EMG for muscle activity detection. This dual approach improves the accuracy of rehabilitation training, providing more personalized feedback to patients and potentially accelerating recovery. The hybrid system allows for more accurate decoding of patients’ movements and enhances their ability to perform motor tasks during rehabilitation. Another notable advancement in EEG-based rehabilitation is the application of VR in conjunction with EEG, offering patients immersive and interactive environments that stimulate motor recovery. As research progresses, the integration of EEG with VR systems is showing promise in fostering neuroplasticity by creating engaging and tailored rehabilitation experiences (64, 65). The development of signal processing technologies has greatly optimized EEG preprocessing, feature extraction, and classification methods. Traditional feature extraction frequently employs time-domain, frequency-domain, and time-frequency analyses, such as the utilization of power spectral density to examine patterns of brain activity across diverse frequency bands in patients (16, 66). The damaged regions of the brain in patients with stoke frequently exhibit augmented low-frequency bands and diminished high-frequency bands. The advent of deep learning, encompassing convolutional neural networks and generative adversarial networks, has facilitated the automated extraction of features. These neural network models allow for complex pattern recognition directly from raw EEG signals, facilitating a deeper understanding of post-stroke brain functions (67, 68). Tong et al. (69) constructed a deep learning model based on EEG signals for rapid detection of ischemic stroke. They gathered EEG data from 20 acute ischemic stroke patients and 19 healthy controls and introduced a fusion feature combining correlation-weighted phase lag index and sample entropy to explore inter-channel synchronization and functional connectivity. Recent studies have demonstrated that complex network analysis of EEG data can provide insights into the reorganization of brain networks after stroke. For example, one study (70) analyzing resting-state and task-state functional connectivity identified a “cognitive network” comprising nodes in the subcortical, frontoparietal, visual, and cerebellar networks. This network shows differential effective connectivity patterns that are sensitive to post-stroke cognitive impairment and improvement. Moreover, another study (71) focused on mild stroke patients compared EEG-based functional connectivity during cognitive tasks across groups with cortical infarctions, subcortical infarctions, and healthy controls. Their graph theory analysis revealed significantly reduced global and local efficiencies in patient groups, along with distinct nodal strength distributions that differed by lesion location. A systematic review (72) compared EEG-derived complex network parameters between stroke patients and healthy subjects. Although the effect sizes for parameters such as path length, clustering coefficient, and cohesion were modest, the review highlighted both structural differences and certain overlapping features between the groups. Additionally, multimodal data fusion techniques are increasingly applied to stroke EEG studies. By combining EEG with fMRI or near-infrared spectroscopy, researchers can obtain more comprehensive brain activity data, valuable for early prognosis prediction and evaluating the effectiveness of different rehabilitation interventions (73, 74). Stroke may not only damage local neural structures but also disrupt large-scale brain networks, affecting both structural and functional connectivity. Stroke-induced lesions may impair the integrity of the default mode network and the cortico-thalamic circuits, leading to reduced global efficiency and altered modular organization. Such disruptions may contribute to deficits in cognitive and motor function by impairing inter-hemispheric communication and reducing the integration of distributed neural systems (71, 75–77).
4 Limitation
Firstly, this bibliometric analysis is was confined to data drawn exclusively from the WoSCC, with the exclusion of data from other databases. This limited scope might result in the omission of some critical studies, potentially affecting the comprehensiveness of the analysis. Furthermore, the study was restricted to English-language publications, which excludes relevant research in other languages, particularly domestic studies from non-English speaking regions. This limitation could affect the representation of global research progress in the field of stroke-related EEG research. Secondly, the visual mapping generated using VOSviewer and CiteSpace required specific parameter settings, including node selection, threshold settings, and clustering methods based on data availability and study requirements. These settings may introduce some level of statistical bias, which could influence the results.
5 Conclusion
This study is among the first to employ bibliometric and visual analysis techniques to examine the evolution of EEG research in the field of stroke over the past two decades. The analysis was conducted using the VOSviewer and CiteSpace software tools. The results provide a systematic illustration of the current research landscape, identifying key areas of interest and future trends in this domain. The findings demonstrate that EEG is a widely utilized tool in the monitoring of neural functions associated with stroke, the assessment of epilepsy risk, and the facilitation of rehabilitation. These observations reflect a substantial academic interest and clinical relevance. The integration of deep learning and multimodal data fusion has enabled researchers to perform more complex analyses of post-stroke electrophysiological activity, laying a solid foundation for personalized rehabilitation plans. Furthermore, the use of EEG in the assessment of epilepsy and consciousness disorders improves the accuracy of post-stroke complication detection, particularly in the early identification of non-convulsive seizures and the assessment of consciousness recovery potential. In the future, as EEG technology continues to be integrated with other imaging modalities and high-efficiency algorithms, its application in stroke rehabilitation appears to be highly promising. In this context, EEG -driven BCI technologies have evolved from basic monitoring to more advanced intervention strategies. It is recommended that future research concentrate on the promotion of interdisciplinary applications of EEG and the establishment of standardized signal processing procedures. This will ensure the consistency of study outcomes and facilitate the adoption of EEG in a broader clinical context, as well as its use in translational applications.
Data availability statement
The original contributions presented in the study are included in the article/supplementary material, further inquiries can be directed to the corresponding author/s.
Author contributions
X-YL: Writing – review & editing, Writing – original draft, Methodology. Y-EJ: Data curation, Formal analysis, Visualization, Writing – original draft, Writing – review & editing. R-JX: Validation, Writing – review & editing. T-TQ: Conceptualization, Visualization, Data curation, Writing – review & editing, Formal analysis, Writing – original draft. S-LL: Data curation, Writing – review & editing. YC: Conceptualization, Writing – original draft, Writing – review & editing.
Funding
The author(s) declare that no financial support was received for the research and/or publication of this article.
Acknowledgments
The authors thank everyone who contributed to the writing and all the publications, and their authors involved in this study.
Conflict of interest
The authors declare that the research was conducted in the absence of any commercial or financial relationships that could be construed as a potential conflict of interest.
Generative AI statement
The authors declare that no Gen AI was used in the creation of this manuscript.
Publisher’s note
All claims expressed in this article are solely those of the authors and do not necessarily represent those of their affiliated organizations, or those of the publisher, the editors and the reviewers. Any product that may be evaluated in this article, or claim that may be made by its manufacturer, is not guaranteed or endorsed by the publisher.
Abbreviations
ACPP, average citation per publication; TC, total citations; TLS, total link strength.
References
1. Ma, Q, Li, R, Wang, L, Yin, P, Wang, Y, Yan, C, et al. Temporal trend and attributable risk factors of stroke burden in China, 1990-2019: an analysis for the global burden of disease study 2019. Lancet Public Health. (2021) 6:e897–906. doi: 10.1016/s2468-2667(21)00228-0
2. Jiang, Z, Kuhnke, P, Stockert, A, Wawrzyniak, M, Halai, A, Saur, D, et al. Dynamic reorganization of task-related network interactions in post-stroke aphasia recovery. Brain. (2025). doi: 10.1093/brain/awaf036
3. Mao, L, Che, X, Wang, J, Jiang, X, Zhao, Y, Zou, L, et al. Sub-acute stroke demonstrates altered beta oscillation and connectivity pattern in working memory. J Neuroeng Rehabil. (2024) 21:212. doi: 10.1186/s12984-024-01516-5
4. Ros, T, Michela, A, Mayer, A, Bellmann, A, Vuadens, P, Zermatten, V, et al. Disruption of large-scale electrophysiological networks in stroke patients with visuospatial neglect. Netw Neurosci. (2022) 6:69–89. doi: 10.1162/netn_a_00210
5. Vidal, JPC, Danet, L, Arribarat, G, Pariente, J, Péran, P, Albucher, JF, et al. Factors behind poor cognitive outcome following a thalamic stroke. J Neurol. (2025) 272:98. doi: 10.1007/s00415-024-12777-4
6. Krishnamurthi, RV, Ikeda, T, and Feigin, VL. Global, regional and country-specific burden of Ischaemic stroke, intracerebral Haemorrhage and subarachnoid Haemorrhage: a systematic analysis of the global burden of disease study 2017. Neuroepidemiology. (2020) 54:171–9. doi: 10.1159/000506396
7. Shih, PC, Steele, CJ, Hoepfel, D, Muffel, T, Villringer, A, and Sehm, B. The impact of lesion side on bilateral upper limb coordination after stroke. J Neuroeng Rehabil. (2023) 20:166. doi: 10.1186/s12984-023-01288-4
8. Buck, BH, Akhtar, N, Alrohimi, A, Khan, K, and Shuaib, A. Stroke mimics: incidence, aetiology, clinical features and treatment. Ann Med. (2021) 53:420–36. doi: 10.1080/07853890.2021.1890205
9. Hilkens, NA, Casolla, B, Leung, TW, and de Leeuw, FE. Stroke. Lancet. (2024) 403:2820–36. doi: 10.1016/s0140-6736(24)00642-1
10. Kwah, LK, and Diong, J. National Institutes of Health stroke scale (NIHSS). J Physiother. (2014) 60:61. doi: 10.1016/j.jphys.2013.12.012
11. Shin, S, Lee, Y, Chang, WH, Sohn, MK, Lee, J, Kim, DY, et al. Multifaceted assessment of functional outcomes in survivors of first-time stroke. JAMA Netw Open. (2022) 5:e2233094. doi: 10.1001/jamanetworkopen.2022.33094
12. Czap, AL, and Sheth, SA. Overview of imaging modalities in stroke. Neurology. (2021) 97:S42–s51. doi: 10.1212/wnl.0000000000012794
13. Wang, H, Xiong, X, Zhang, K, Wang, X, Sun, C, Zhu, B, et al. Motor network reorganization after motor imagery training in stroke patients with moderate to severe upper limb impairment. CNS Neurosci Ther. (2023) 29:619–32. doi: 10.1111/cns.14065
14. Liao, XY, Gao, YX, Qian, TT, Zhou, LH, Li, LQ, Gong, Y, et al. Bibliometric analysis of electroencephalogram research in Parkinson's disease from 2004 to 2023. Front Neurosci. (2024) 18:1433583. doi: 10.3389/fnins.2024.1433583
15. Sanossian, N, and Fink, E. What will the Mobile stroke unit of the future look like, and will EEG have a role? Neurology. (2023) 101:1085–6. doi: 10.1212/wnl.0000000000208047
16. Saes, M, Meskers, CGM, Daffertshofer, A, de Munck, JC, Kwakkel, G, and van Wegen, EEH. How does upper extremity Fugl-Meyer motor score relate to resting-state EEG in chronic stroke? A power spectral density analysis. Clin Neurophysiol. (2019) 130:856–62. doi: 10.1016/j.clinph.2019.01.007
17. Zhang, JJ, Bai, Z, and Fong, KNK. Resting-state cortical electroencephalogram rhythms and network in patients after chronic stroke. J Neuroeng Rehabil. (2024) 21:32. doi: 10.1186/s12984-024-01328-7
18. Pace, M, Camilo, MR, Seiler, A, Duss, SB, Mathis, J, Manconi, M, et al. Rapid eye movements sleep as a predictor of functional outcome after stroke: a translational study. Sleep. (2018) 41:zsy138. doi: 10.1093/sleep/zsy138
19. Tscherpel, C, Mustin, M, Massimini, M, Paul, T, Ziemann, U, Fink, GR, et al. Local neuronal sleep after stroke: the role of cortical bistability in brain reorganization. Brain Stimul. (2024) 17:836–46. doi: 10.1016/j.brs.2024.07.008
20. Miladinović, A, Accardo, A, Jarmolowska, J, Marusic, U, and Ajčević, M. Optimizing real-time MI-BCI performance in post-stroke patients: impact of time window duration on classification accuracy and responsiveness. Sensors. (2024) 24:6125. doi: 10.3390/s24186125
21. Rustamov, N, Souders, L, Sheehan, L, Carter, A, and Leuthardt, EC. IpsiHand brain-computer Interface therapy induces broad upper extremity motor rehabilitation in chronic stroke. Neurorehabil Neural Repair. (2024) 39:74–86. doi: 10.1177/15459683241287731
22. Su, J, Wang, J, Wang, W, Wang, Y, Bunterngchit, C, Zhang, P, et al. An adaptive hybrid brain-computer Interface for hand function rehabilitation of stroke patients. IEEE Trans Neural Syst Rehabil Eng. (2024) 32:2950–60. doi: 10.1109/tnsre.2024.3431025
23. Cioffi, E, Hutber, A, Molloy, R, Murden, S, Yurkewich, A, Kirton, A, et al. EEG-based sensorimotor neurofeedback for motor neurorehabilitation in children and adults: a scoping review. Clin Neurophysiol. (2024) 167:143–66. doi: 10.1016/j.clinph.2024.08.009
24. Ba, H, Zhang, L, He, X, and Li, S. Knowledge mapping and global trends in the field of the objective structured clinical examination: bibliometric and visual analysis (2004-2023). JMIR Med Educ. (2024) 10:e57772. doi: 10.2196/57772
25. Wang, J, Cao, K, Chen, Z, Lyu, T, Xia, Q, Liu, L, et al. Research trends and hotspots of acupuncture therapy for obesity from 2004 to 2023: a bibliometric analysis. Complement Ther Med. (2024) 86:103092. doi: 10.1016/j.ctim.2024.103092
26. Xu, Y, Huang, H, Wu, M, Zhuang, Z, Liu, H, Hou, M, et al. Transcranial direct current stimulation for cognitive impairment rehabilitation: a bibliometric analysis. Arch Med Res. (2024) 56:103086. doi: 10.1016/j.arcmed.2024.103086
27. Birbaumer, N, and Cohen, LG. Brain-computer interfaces: communication and restoration of movement in paralysis. J Physiol. (2007) 579:621–36. doi: 10.1113/jphysiol.2006.125633
28. Sitaram, R, Zhang, H, Guan, C, Thulasidas, M, Hoshi, Y, Ishikawa, A, et al. Temporal classification of multichannel near-infrared spectroscopy signals of motor imagery for developing a brain-computer interface. Neuroimage. (2007) 34:1416–27. doi: 10.1016/j.neuroimage.2006.11.005
29. Hofmeijer, J, Tjepkema-Cloostermans, MC, and van Putten, MJ. Burst-suppression with identical bursts: a distinct EEG pattern with poor outcome in postanoxic coma. Clin Neurophysiol. (2014) 125:947–54. doi: 10.1016/j.clinph.2013.10.017
30. Sheorajpanday, RV, Nagels, G, Weeren, AJ, van Putten, MJ, and De Deyn, PP. Reproducibility and clinical relevance of quantitative EEG parameters in cerebral ischemia: a basic approach. Clin Neurophysiol. (2009) 120:845–55. doi: 10.1016/j.clinph.2009.02.171
31. Sheorajpanday, RV, Nagels, G, Weeren, AJ, van Putten, MJ, and De Deyn, PP. Quantitative EEG in ischemic stroke: correlation with functional status after 6 months. Clin Neurophysiol. (2011) 122:874–83. doi: 10.1016/j.clinph.2010.07.028
32. Birbaumer, N. Breaking the silence: brain-computer interfaces (BCI) for communication and motor control. Psychophysiology. (2006) 43:517–32. doi: 10.1111/j.1469-8986.2006.00456.x
33. Birbaumer, N, Weber, C, Neuper, C, Buch, E, Haapen, K, and Cohen, L. Physiological regulation of thinking: brain-computer interface (BCI) research. Prog Brain Res. (2006) 159:369–91. doi: 10.1016/s0079-6123(06)59024-7
34. Chaudhary, U, Birbaumer, N, and Curado, MR. Brain-machine interface (BMI) in paralysis. Ann Phys Rehabil Med. (2015) 58:9–13. doi: 10.1016/j.rehab.2014.11.002
35. Lin, Y, Jiang, Z, Zhan, G, Su, H, Kang, X, and Jia, J. Brain network characteristics between subacute and chronic stroke survivors in active, imagery, passive movement task: a pilot study. Front Neurol. (2023) 14:1143955. doi: 10.3389/fneur.2023.1143955
36. Shu, X, Chen, S, Yao, L, Sheng, X, Zhang, D, Jiang, N, et al. Fast recognition of BCI-inefficient users using physiological features from EEG signals: a screening study of stroke patients. Front Neurosci. (2018) 12:93. doi: 10.3389/fnins.2018.00093
37. Shaheiwola, N, Zhang, B, Jia, J, and Zhang, D. Using tDCS as an add-on treatment prior to FES therapy in improving upper limb function in severe chronic stroke patients: a randomized controlled study. Front Hum Neurosci. (2018) 12:233. doi: 10.3389/fnhum.2018.00233
38. Lin, YF, Liu, XH, Cui, ZY, Song, ZT, Zou, F, Chen, SG, et al. Weakened effective connectivity related to Electroacupuncture in stroke patients with prolonged flaccid paralysis: an EEG pilot study. Neural Plast. (2021) 2021:1–10. doi: 10.1155/2021/6641506
39. Jiang, N, Gizzi, L, Mrachacz-Kersting, N, Dremstrup, K, and Farina, D. A brain-computer interface for single-trial detection of gait initiation from movement related cortical potentials. Clin Neurophysiol. (2015) 126:154–9. doi: 10.1016/j.clinph.2014.05.003
40. Galovic, M, Ferreira-Atuesta, C, Abraira, L, Döhler, N, Sinka, L, Brigo, F, et al. Seizures and epilepsy after stroke: epidemiology, biomarkers and management. Drugs Aging. (2021) 38:285–99. doi: 10.1007/s40266-021-00837-7
41. Sinka, L, Abraira, L, Imbach, LL, Zieglgänsberger, D, Santamarina, E, Álvarez-Sabín, J, et al. Association of Mortality and Risk of epilepsy with type of acute symptomatic seizure after ischemic stroke and an updated prognostic model. JAMA Neurol. (2023) 80:605–13. doi: 10.1001/jamaneurol.2023.0611
42. Sutcliffe, L, Lumley, H, Shaw, L, Francis, R, and Price, CI. Surface electroencephalography (EEG) during the acute phase of stroke to assist with diagnosis and prediction of prognosis: a scoping review. BMC Emerg Med. (2022) 22:29. doi: 10.1186/s12873-022-00585-w
43. Bentes, C, Martins, H, Peralta, AR, Morgado, C, Casimiro, C, Franco, AC, et al. Epileptic manifestations in stroke patients treated with intravenous alteplase. Eur J Neurol. (2017) 24:755–61. doi: 10.1111/ene.13292
44. Vanderschelden, B, Erani, F, Wu, J, de Havenon, A, Srinivasan, R, and Cramer, SC. A measure of neural function provides unique insights into behavioral deficits in acute stroke. Stroke. (2023) 54:e25–9. doi: 10.1161/strokeaha.122.040841
45. Gettings, JV, Mohammad Alizadeh Chafjiri, F, Patel, AA, Shorvon, S, Goodkin, HP, and Loddenkemper, T. Diagnosis and management of status epilepticus: improving the status quo. Lancet Neurol. (2025) 24:65–76. doi: 10.1016/s1474-4422(24)00430-7
46. Bai, Y, Yang, L, Meng, X, Huang, Y, Wang, Q, Gong, A, et al. Breakdown of effective information flow in disorders of consciousness: insights from TMS-EEG. Brain Stimul. (2024) 17:533–42. doi: 10.1016/j.brs.2024.04.011
47. Lee, M, Baird, B, Gosseries, O, Nieminen, JO, Boly, M, Postle, BR, et al. Connectivity differences between consciousness and unconsciousness in non-rapid eye movement sleep: a TMS-EEG study. Sci Rep. (2019) 9:5175. doi: 10.1038/s41598-019-41274-2
48. Purdon, PL, Pierce, ET, Mukamel, EA, Prerau, MJ, Walsh, JL, Wong, KF, et al. Electroencephalogram signatures of loss and recovery of consciousness from propofol. Proc Natl Acad Sci USA. (2013) 110:E1142–51. doi: 10.1073/pnas.1221180110
49. Duru, AD, Duru, DG, Yumerhodzha, S, and Bebek, N. Analysis of correlation between white matter changes and functional responses in thalamic stroke: a DTI & EEG study. Brain Imaging Behav. (2016) 10:424–36. doi: 10.1007/s11682-015-9397-1
50. Gutiérrez, M, Merino, JJ, Alonso de Leciñana, M, and Díez-Tejedor, E. Cerebral protection, brain repair, plasticity and cell therapy in ischemic stroke. Cerebrovasc Dis. (2009) 27:177–86. doi: 10.1159/000200457
51. Maida, CD, Norrito, RL, Rizzica, S, Mazzola, M, Scarantino, ER, and Tuttolomondo, A. Molecular pathogenesis of ischemic and hemorrhagic strokes: background and therapeutic approaches. Int J Mol Sci. (2024) 25:6297. doi: 10.3390/ijms25126297
52. Qin, C, Yang, S, Chu, YH, Zhang, H, Pang, XW, Chen, L, et al. Signaling pathways involved in ischemic stroke: molecular mechanisms and therapeutic interventions. Signal Transduct Target Ther. (2022) 7:215. doi: 10.1038/s41392-022-01064-1
53. Regenhardt, RW, Das, AS, Lo, EH, and Caplan, LR. Advances in understanding the pathophysiology of lacunar stroke: a review. JAMA Neurol. (2018) 75:1273–81. doi: 10.1001/jamaneurol.2018.1073
54. Chaturvedi, S, and De Marchis, GM. Inflammatory biomarkers and stroke subtype: an important new frontier. Neurology. (2024) 102:e208098. doi: 10.1212/wnl.0000000000208098
56. Shi, K, Tian, DC, Li, ZG, Ducruet, AF, Lawton, MT, and Shi, FD. Global brain inflammation in stroke. Lancet Neurol. (2019) 18:1058–66. doi: 10.1016/s1474-4422(19)30078-x
57. Zhao, Y, Li, Q, Niu, J, Guo, E, Zhao, C, Zhang, J, et al. Neutrophil membrane-camouflaged Polyprodrug Nanomedicine for inflammation suppression in ischemic stroke therapy. Adv Mater. (2024) 36:e2311803. doi: 10.1002/adma.202311803
58. Cai, W, Zhang, K, Li, P, Zhu, L, Xu, J, Yang, B, et al. Dysfunction of the neurovascular unit in ischemic stroke and neurodegenerative diseases: an aging effect. Ageing Res Rev. (2017) 34:77–87. doi: 10.1016/j.arr.2016.09.006
59. Fang, J, Wang, Z, and Miao, CY. Angiogenesis after ischemic stroke. Acta Pharmacol Sin. (2023) 44:1305–21. doi: 10.1038/s41401-023-01061-2
60. Foreman, B, and Claassen, J. Quantitative EEG for the detection of brain ischemia. Crit Care. (2012) 16:216. doi: 10.1186/cc11230
61. Liu, H, Wei, P, Wang, H, Lv, X, Duan, W, Li, M, et al. An EEG motor imagery dataset for brain computer interface in acute stroke patients. Sci Data. (2024) 11:131. doi: 10.1038/s41597-023-02787-8
62. Benzy, VK, Vinod, AP, Subasree, R, Alladi, S, and Raghavendra, K. Motor imagery hand movement direction decoding using brain computer Interface to aid stroke recovery and rehabilitation. IEEE Trans Neural Syst Rehabil Eng. (2020) 28:3051–62. doi: 10.1109/tnsre.2020.3039331
63. Li, H, Ji, H, Yu, J, Li, J, Jin, L, Liu, L, et al. A sequential learning model with GNN for EEG-EMG-based stroke rehabilitation BCI. Front Neurosci. (2023) 17:1125230. doi: 10.3389/fnins.2023.1125230
64. Shen, J, Gu, X, Fu, J, Yao, Y, Li, Y, Zeng, M, et al. Virtual reality-induced motor function of the upper extremity and brain activation in stroke: study protocol for a randomized controlled trial. Front Neurol. (2023) 14:1094617. doi: 10.3389/fneur.2023.1094617
65. Tang, Z, Wang, H, Cui, Z, Jin, X, Zhang, L, Peng, Y, et al. An upper-limb rehabilitation exoskeleton system controlled by MI recognition model with deep emphasized informative features in a VR scene. IEEE Trans Neural Syst Rehabil Eng. (2023) 31:4390–401. doi: 10.1109/tnsre.2023.3329059
66. Delcamp, C, Srinivasan, R, and Cramer, SC. EEG provides insights into motor control and neuroplasticity during stroke recovery. Stroke. (2024) 55:2579–83. doi: 10.1161/strokeaha.124.048458
67. Choi, YA, Park, SJ, Jun, JA, Pyo, CS, Cho, KH, Lee, HS, et al. Deep learning-based stroke disease prediction system using real-time bio signals. Sensors. (2021) 21:4269. doi: 10.3390/s21134269
68. Vecchio, F, Caliandro, P, Reale, G, Miraglia, F, Piludu, F, Masi, G, et al. Acute cerebellar stroke and middle cerebral artery stroke exert distinctive modifications on functional cortical connectivity: a comparative study via EEG graph theory. Clin Neurophysiol. (2019) 130:997–1007. doi: 10.1016/j.clinph.2019.03.017
69. Tong, W, Yue, W, Chen, F, Shi, W, Zhang, L, and Wan, J. MSE-VGG: a novel deep learning approach based on EEG for rapid ischemic stroke detection. Sensors. (2024) 24:4234. doi: 10.3390/s24134234
70. Zhang, J, Tang, H, Zuo, L, Liu, H, Liu, C, Li, Z, et al. Identification of a cognitive network with effective connectivity to post-stroke cognitive impairment. Cogn Neurodyn. (2024) 18:3741–56. doi: 10.1007/s11571-024-10139-4
71. Xu, M, Qian, L, Wang, S, Cai, H, Sun, Y, Thakor, N, et al. Brain network analysis reveals convergent and divergent aberrations between mild stroke patients with cortical and subcortical infarcts during cognitive task performing. Front Aging Neurosci. (2023) 15:1193292. doi: 10.3389/fnagi.2023.1193292
72. Asadi, B, Cuenca-Zaldivar, JN, Nakhostin, AN, Ibáñez, J, Herrero, P, and Calvo, S. Brain analysis with a complex network approach in stroke patients based on electroencephalography: a systematic review and Meta-analysis. Healthcare. (2023) 11:666. doi: 10.3390/healthcare11050666
73. Liang, J, Song, Y, Belkacem, AN, Li, F, Liu, S, Chen, X, et al. Prediction of balance function for stroke based on EEG and fNIRS features during ankle dorsiflexion. Front Neurosci. (2022) 16:968928. doi: 10.3389/fnins.2022.968928
74. Saj, A, Pierce, JE, Ronchi, R, Ros, T, Thomasson, M, Bernati, T, et al. Real-time fMRI and EEG neurofeedback: a perspective on applications for the rehabilitation of spatial neglect. Ann Phys Rehabil Med. (2021) 64:101561. doi: 10.1016/j.rehab.2021.101561
75. Grefkes, C, and Fink, GR. Connectivity-based approaches in stroke and recovery of function. Lancet Neurol. (2014) 13:206–16. doi: 10.1016/s1474-4422(13)70264-3
76. Siegel, JS, Ramsey, LE, Snyder, AZ, Metcalf, NV, Chacko, RV, Weinberger, K, et al. Disruptions of network connectivity predict impairment in multiple behavioral domains after stroke. Proc Natl Acad Sci USA. (2016) 113:E4367–76. doi: 10.1073/pnas.1521083113
Keywords: stroke, electroencephalogram, bibliometrix, CiteSpace, VOSviewer, visualization analysis
Citation: Liao X-Y, Jiang Y-E, Xu R-J, Qian T-T, Liu S-L and Che Y (2025) A bibliometric analysis of electroencephalogram research in stroke: current trends and future directions. Front. Neurol. 16:1539736. doi: 10.3389/fneur.2025.1539736
Edited by:
Mingming Lu, Characteristic Medical Center of Chinese People’s Armed Police Force, ChinaCopyright © 2025 Liao, Jiang, Xu, Qian, Liu and Che. This is an open-access article distributed under the terms of the Creative Commons Attribution License (CC BY). The use, distribution or reproduction in other forums is permitted, provided the original author(s) and the copyright owner(s) are credited and that the original publication in this journal is cited, in accordance with accepted academic practice. No use, distribution or reproduction is permitted which does not comply with these terms.
*Correspondence: Yi Che, NTgxMzU3NEBxcS5jb20=
†These authors share first authorship