- 1Department of Neurosurgery, The Second Affiliated Hospital of Chongqing Medical University, Chongqing, China
- 2Department of Emergency, The Second Affiliated Hospital of Chongqing Medical University, Chongqing, China
Objective: This study aims to develop and validate an automated machine learning model to predict perioperative ischemic stroke (PIS) risk in endovascularly treated patients with ruptured intracranial aneurysms (RIAs), with the goal of establishing a clinical decision-support tool.
Methods: In this retrospective cohort study, we analyzed RIA patients undergoing endovascular treatment at our neurosurgical center (December 2013–February 2024). The least absolute shrinkage and selection operator (LASSO) method was used to screen essential features associated with PIS. Based on these features, nine machine learning models were constructed using a training set (75% of participants) and assessed on a test set (25% of participants). Through comparative analysis, using metrics such as area under the receiver operating characteristic curve (ROCAUC) and Brier score, we identified the optimal model—random forest (RF)—for predicting PIS. To interpret the RF models, we utilized the Shapley Additive exPlanations (SHAP).
Results: The final cohort comprised 647 consecutive RIA patients who underwent endovascular intervention. LASSO regression identified 13 clinically actionable predictors of PIS from the initial variables. These predictors encompassed: vascular risk factors (hyperlipidemia, arteriosclerosis); neuroimaging indicators of severity (modified Fisher scale, aneurysm location, and neck-to-diameter ratio); clinical status (Glasgow Coma Scale score, Hunt-Hess grade, age, sex); procedural complications (intraprocedural rupture, periprocedural re-rupture); and therapeutic determinants (therapy method and history of ischemic comorbidities). Nine machine learning algorithms were evaluated using stratified 10-fold cross-validation. Among them, the RF model demonstrated the best performance, with the ROCAUC of 92.11% (95%CI: 89.74–94.48%) on the test set and 87.08% (95%CI: 81.23–92.93%) on the training set. Finally, in a prospective validation cohort, the RF predictive model demonstrated an accuracy of 88.23% in forecasting the incidence of PIS. Additionally, based on this predictive model, this study developed a highly convenient web-based calculator. Clinicians only need to input the patient’s key factors into this calculator to predict the postoperative incidence of PIS and provide individualized treatment plans for the patient.
Conclusion: We successfully developed and validated an interpretable machine learning framework, integrated with a clinical decision-support system, for predicting postprocedural PIS in endovascularly treated RIAs patients. This tool effectively predicted the likelihood of PIS, enabling high-risk patients to promptly take specific preventive and therapeutic measures.
1 Introduction
Intracranial aneurysms (IAs) are abnormal dilations of blood vessels within the brain, characterized by degenerative changes across all layers of the vessel wall, leading to vasodilation (1). In adults over the age of 50, the prevalence of IAs is estimated to be approximately 2% (2). The onset of IAs is typically insidious, as they rarely present with obvious symptoms or clinical signs prior to rupture. The most critical risk posed by IAs is rupture, which can result in catastrophic hemorrhage. Ruptured intracranial aneurysms (RIAs) constitute a severe neurosurgical emergency, with an annual incidence of 6–9 cases per 100,000 individuals. The associated mortality rate approaches 35%, while a significant proportion of survivors sustain permanent neurological deficits, leading to long-term disability (3). Currently, treatment options for aneurysms primarily consist of surgical clipping and endovascular treatment. Endovascular treatment has emerged as the preferred choice due to its minimally invasive nature and quick recovery time. Consequently, its role in the treatment of RIAs has become increasingly prominent in recent years (4, 5).
Despite the advantages of endovascular treatment—namely its minimally invasive nature and rapid recovery—the occurrence of perioperative complications cannot be ignored. Each year, 310 million people worldwide undergo surgery, with more than 600,000 experiencing perioperative strokes. The majority of studies focus on ischemic stroke, which accounts for approximately 95% of perioperative strokes, compared to approximately 5% for hemorrhagic stroke (6, 7). Studies demonstrate that perioperative ischemic stroke (PIS) is the primary cause of disability and death following RIA treatment (8–10). The expert consensus of the American Society of Neurosurgical Anesthesiology and Critical Care Sciences defines PIS as an ischemic cerebral infarction that occurs during or within 30 days after the operation (11). With the growing number of patients undergoing endovascular surgery, the occurrence of PIS has attracted increasing attention (12–14). Relevant studies have shown that multiple factors (such as hypertension, previous stroke, diabetes, and aneurysm morphology) can increase the risk of PIS. However, determining which of these risk factors require clinical intervention or prevention strategies remains a major challenge (15–17). Recent studies suggest that integrating machine learning techniques into predictive models can substantially improve the accuracy of risk stratification (18–20). Therefore, a systematic synthesis of risk factors associated with PIS following endovascular treatment is essential. By developing robust machine learning-based predictive models, we can enhance the application scope and practicality of risk prediction models in clinical practice. This will enable clinicians to make decisions with more sufficient data support, thereby reducing the incidence of PIS in RIA patients after treatment and achieving the goal of improving long-term prognosis.
In this study, nine distinct prediction models for PIS were developed using machine learning methods, leveraging data from 647 patients who underwent procedures. Through comparative analysis, the most effective predictive model for identifying high-risk patients was identified, facilitating its clinical application. To assist healthcare professionals in effectively utilizing this model, we have also developed a web-based calculator using the collected data. This tool enables the identification of high-risk patients and provides them with more effective treatment options.
2 Methods
2.1 Source and extraction of data
The design and execution of this retrospective investigation followed the STROBE Guidelines (21, 22). Two investigators independently collected data from patients with RIAs who underwent endovascular treatment at the Second Affiliated Hospital of Chongqing Medical University from 25 December 2013 to 1 February 2024. The data included basic information (age, gender, drinking history, smoking history, hypertension, coronary heart disease, etc.), aneurysm information (size, location, etc.), and treatment method. Approval for this study was obtained from the Ethics Committee of our hospital, and all patient data were anonymized. No patient-specific information was retained, and consent was obtained from the patients.
To further verify the accuracy of the models, prospective data were collected at the Second Affiliated Hospital of Chongqing Medical University from 12 February 2024 to 1 May 2024, focusing on RIA patients who underwent endovascular treatment data.
Inclusion criteria:
Patients were included in the study if they met the following conditions:
1. The aneurysms were saccular;
2. Patients with aneurysms treated with endovascular coiling (EC), stent-assisted coiling (SAC), or flow-diverted (FD) stents;
3. Patients aged 18–85 years, not pregnant, and fully competent to act.
Exclusion criteria:
Patients were excluded from the study if they met any of the following:
1. The aneurysms were non-saccular (e.g., dissecting, fusiform, traumatic, and blood blister-like aneurysms);
2. Patients treated with hybrid clipping and endovascular procedures;
3. Patients who were adolescents, aged 85 years or older, pregnant, lacking voluntary capacity, or had limited decision-making capacity.
2.2 Endovascular procedures
The neurovascular team decided on specific endovascular therapy plans tailored to each patient. Procedures involved endovascular surgery following general anesthesia. To avoid embolic occurrences, systemic intravenous heparin systemic intravenous heparin was administered during the procedure. For patients with RIAs, oral antiplatelet agents were not administered after surgery if EC was selected. If SAC was chosen, patients received 300 mg of aspirin and 300 mg of clopidogrel after surgery. Starting the next day, the regimen was changed to aspirin 100 mg/d and clopidogrel 75 mg/d. The patient was advised to take clopidogrel 75 mg/d for 3 weeks and aspirin 100 mg/d for 6 months. If FD treatment was selected, patients were given 300 mg of aspirin and 300 mg of clopidogrel postoperatively, and the next day, clopidogrel 75 mg/d for 6 months and long-term aspirin 100 mg/d.
2.3 Outcome measures
We define PIS as any adverse neurological events that occur during or within 30 days after surgery in RIA patients receiving endovascular treatment. Computerized tomography (CT) examinations were conducted directly after the operation, and repeated examinations were performed for cases with deteriorated neurological function. When CT images do not confirm suspected new-onset cerebral infarction, magnetic resonance imaging (MRI) examination is performed (16, 23). Patients confirmed by both clinical symptoms and imaging were included in the complication group, and those without were included in the control group.
2.4 Model input features
We considered 24 potential factors, encompassing age, gender, alcohol and tobacco use history, hypertension, arteriosclerosis, hyperlipidemia, diabetes, previous cerebral ischemia, Glasgow Coma Scale (GCS), modified Fisher scale, Hunt and Hess grade, oculomotor nerve palsy, aneurysm location, neck, length, maximum diameter, ratio of aneurysm neck to maximum diameter, multiple aneurysms, perioperative re-rupture, preoperative intracranial hematoma, intraprocedural rupture (IPR), time from symptom onset to surgery, treatment method (EC, SAC, or FD), and the occurrence of PIS. Subsequently, we used the least absolute shrinkage and selection operator (LASSO) to identify factors associated with PIS (24).
2.5 Statistical analysis
The missing data were treated in the following ways: groups were eliminated if the percentage of missing values was less than 5%, random forest (RF) regression was used for interpolation if the percentage of missing values was between 5 and 20%, and if the percentage of missing values was greater than 20%, the group was removed from the final complete dataset. It can be seen from Supplementary Figure 1 that there are no missing values in our data, so no special processing has been carried out. R software, version 4.3.2, was used for all analyses. The distribution of continuous variables, which were reported as mean ± standard deviation (SD), was tested for normality using the Shapiro–Wilk test; the independent sample t-test was used for comparison. The continuous variables with a skewed distribution were represented by the quartile and median (IQR). Frequencies and percentages were utilized to represent the categorical variables, and Fisher’s exact probability method or the chi-squared test was applied for statistical analysis. Nine models were created based on the feature set of the most significant characteristics after the most significant features were filtered out using LASSO regression analysis.
Nine learning models—Decision Tree (DT), Light Gradient Boosting Machine (LightGBM), eXtreme Gradient Boosting (XGBOOST) algorithm, Logistic Regression (LR), RF, Elastic Net (ENet), Multi-layer Perceptron (MLP), Support Vector Machine (SVM), and K-Nearest Neighbor machine (KNN)—were constructed in order to achieve the best predictive performance. Area under the receiver operating characteristic curve (ROCAUC) and Brier scores were used to assess the model’s performance. Clinical decision curve analysis (DCA) evaluation model’s clinical viability (25). The relationship between each attribute and PIS was shown using R’s Shapley Additive package (SHAP) tool once the optimal model had been obtained. The best model will finally be built on a web-based calculator using the shiny package (26, 27).
3 Results
3.1 Patient characteristics
This study enrolled 647 patients with RIAs treated with endovascular therapy (details shown in Table 1), comprising 424 females (65.5%) and 223 males (34.5%) with a median age of 54.9 years. Treatment modalities included EC (n = 199, 30.8%), SAC (n = 381, 58.9%), and FD stents (n = 67, 10.3%). Aneurysms were predominantly located in the anterior communicating artery (AcomA, 27.5%) and the posterior communicating artery (PcomA, 27.5%), followed by the middle cerebral artery (MCA, 15.8%), internal carotid artery (ICA, 11.1%), anterior cerebral artery (ACA, 4.9%), vertebral artery (VA, 3.2%), posterior inferior cerebellar artery (PICA, 2.3%), posterior cerebral artery (PCA, 2.3%), choroidal artery (ChoA, 2.6%), basilar artery (BA, 1.4%), and ophthalmic artery (OA, 1.2%).
A total of 166 patients (25.66%) experienced PIS within 30 days after endovascular therapy. Following feature screening, all variables exhibited complete data. To develop and validate the prediction model, the study population was randomly divided into a training group (75%, n = 485) and a validation set (25%, n = 162). Patients were further classified into the complication group (n = 166) and the control group (n = 481). As depicted in Figure 1, this allocation adhered to a predefined study flowchart. There were no statistically significant differences in patient characteristics between the training and testing datasets.
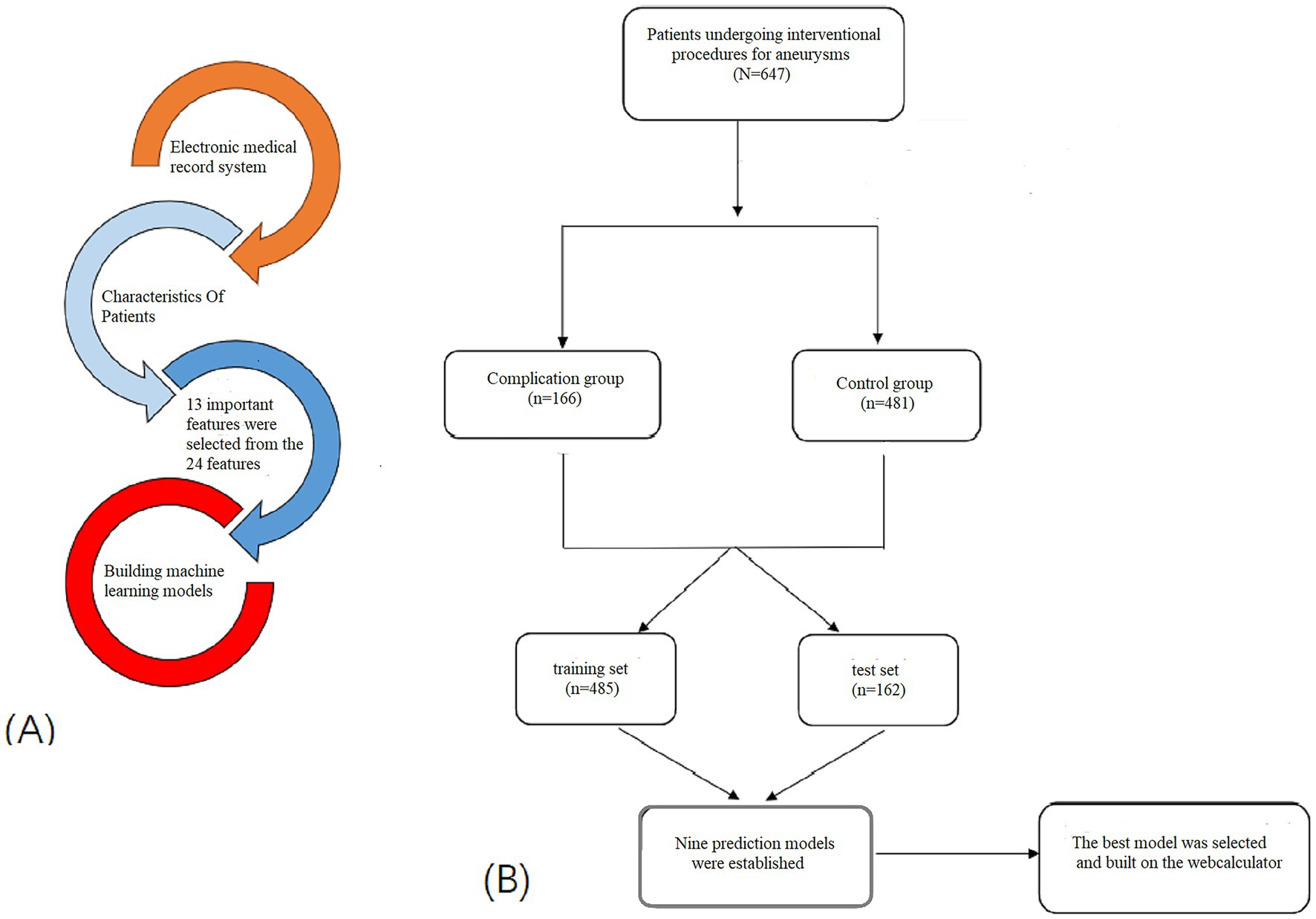
Figure 1. Flow chart of model making process and research procedure. (A) The figure shows how to obtain data from the electronic medical record system. A total of 23 variables were collected, and 13 variables were selected to build the machine learning model. (B) Study flow chart.
3.2 Important features and model performance
Through 10-fold cross-validation, the optimal lambda value for LASSO feature selection was determined, resulting in the identification of 10 features with non-zero coefficients (Figures 2A,B). Subsequently, a machine learning model was developed to predict PIS following endovascular procedures using these 13 variables. The performance of the predictive model was assessed using DCA, precision-recall area under the curve (PRAUC), ROCAUC, and Brier score shown in Figures 3, 4. The RF model demonstrated higher ROCAUC and PRAUC values and a lower Brier score compared to other models. Ten cross-validations were performed on all nine models. Figure 5 displays the performance metrics for the training set and test data. The RF model outperformed the other eight models. Additionally, DCA confirmed that RF is the best diagnostic tool for predicting PIS (Figure 3A). The Youden index indicates that a cutoff probability of 36.66% is ideal for the RF. The performance of the established RF model was evaluated using data from the test set (n = 162), with the following results: ROCAUC = 87.08% (95% CI: 81.23–92.93%), accuracy = 0.78, balanced accuracy = 0.79, index = 0.58, kap = 0.55, sensitivity = 0.84, specificity = 0.75, and F1 Score = 0.73.
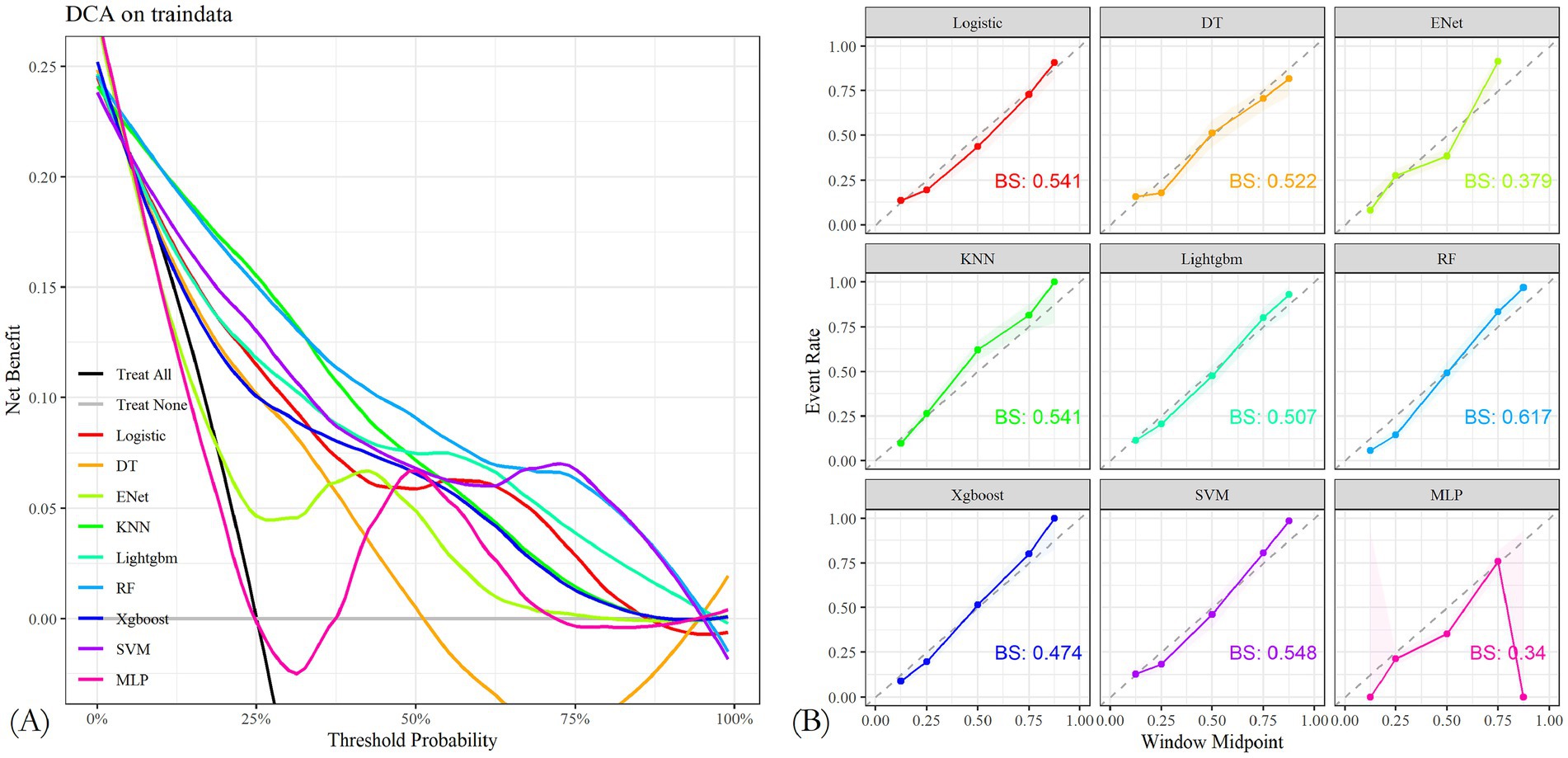
Figure 3. (A) Nine machine learning models were analyzed using decision curves. The most effective diagnostic method for perioperative ischemic stroke is the random forest model. (B) Calibration plots of the nine models. The random forest model obtained lower Brier scores than the other models.
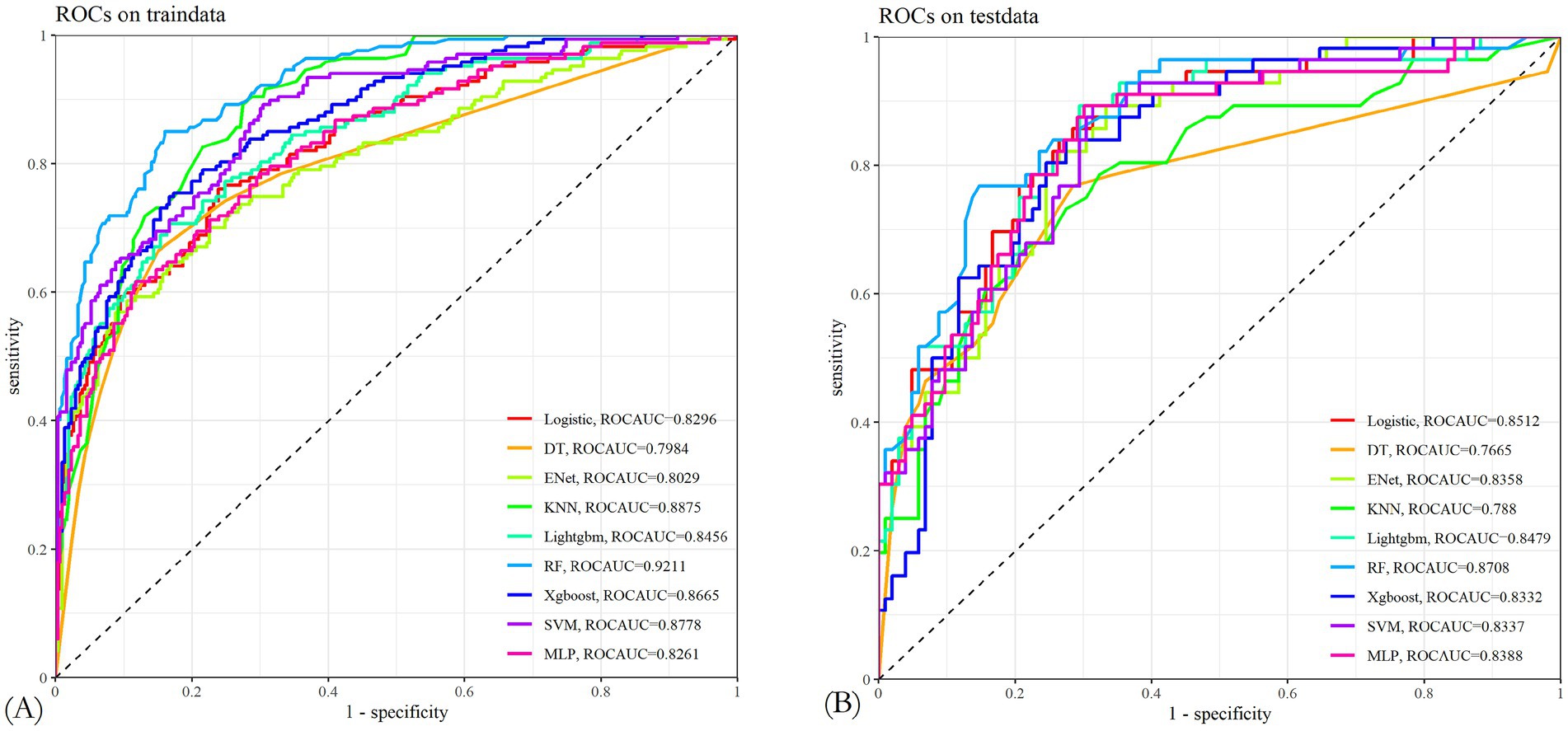
Figure 4. (A,B) Characteristic curves of nine machine learning models in the training and test sets. The random forest model had better AUROC.
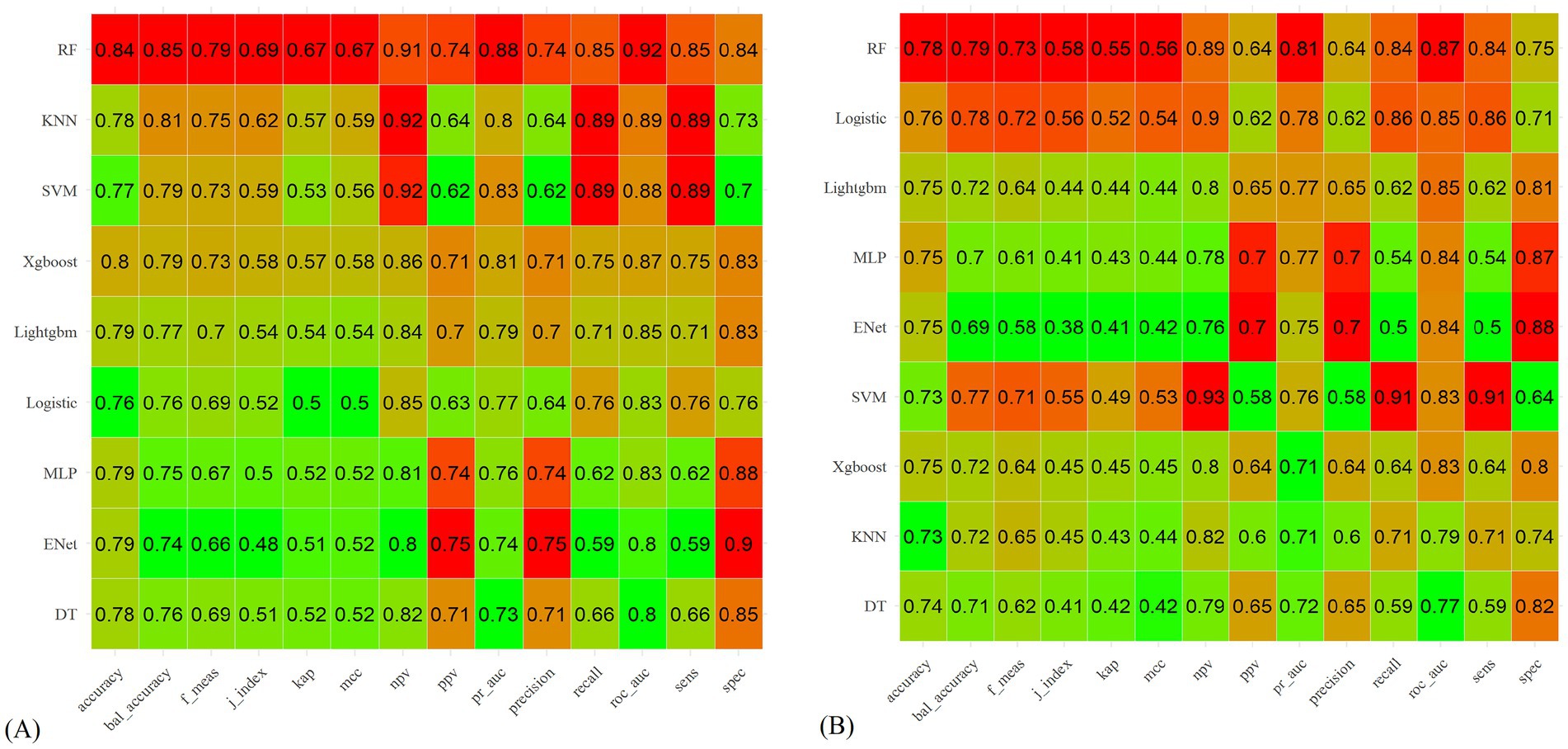
Figure 5. (A) Nine machine learning models in the training set and their performance measures. (B) Nine machine learning models in the test set and their performance measures.
Furthermore, the established RF model was analyzed using the SHAP software package, as depicted in Figure 6. This analysis illustrates the magnitude of the impact of continuous and categorical variables on PIS in the sample using hive plots and box plots, respectively, and the correlation between the size of the eigenvalues and the predicted effects using bar plots.
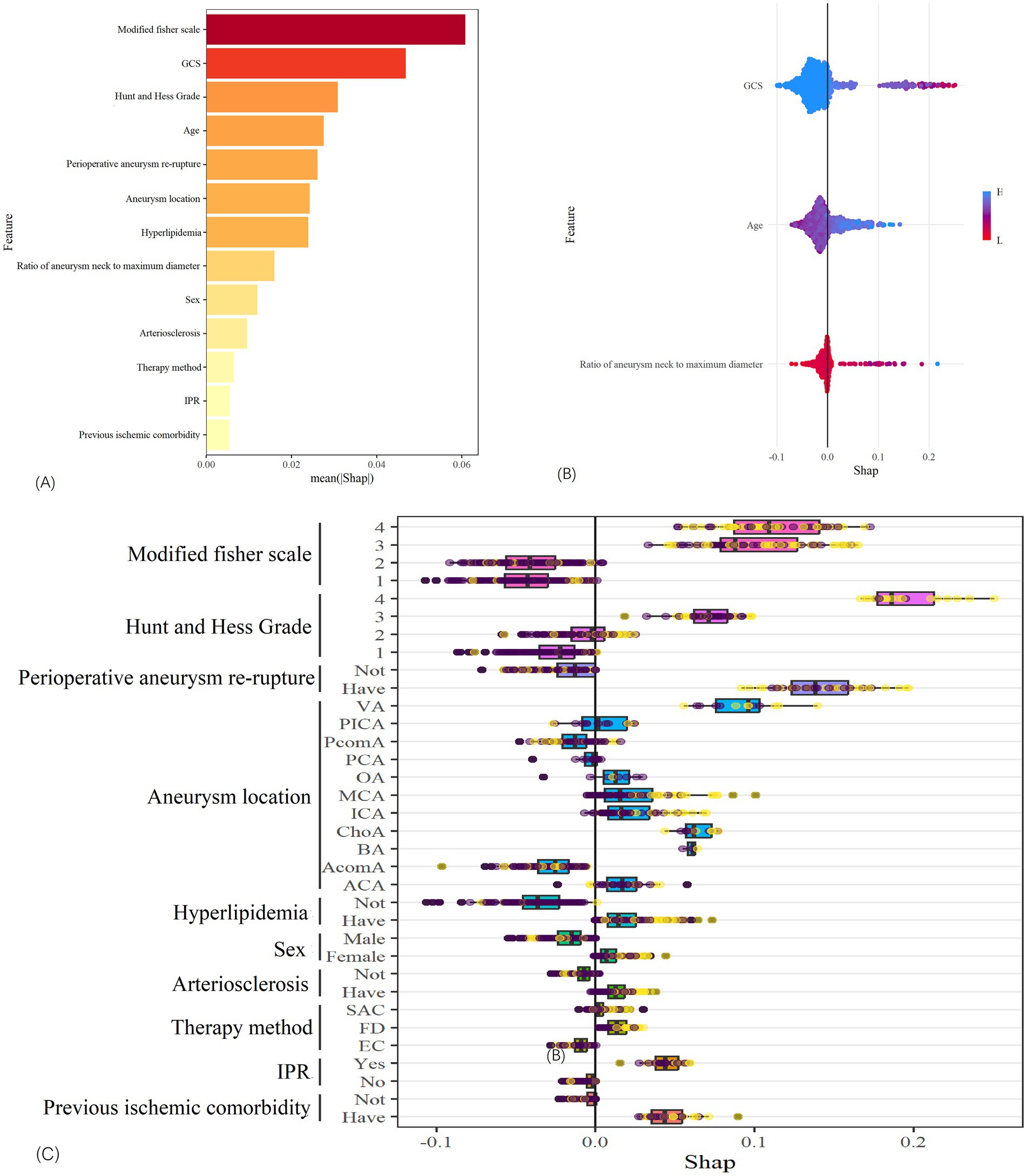
Figure 6. SHAP analysis of the random forest model. (A) Shows the relationship between the importance of each feature. (B) Shows the relationship between continuous variables and PIS. (C) Shows the relationship between classified variable and PIS.
3.3 Model application
Using the shiny package, the developed RF model has been integrated into a web-based application for practical use. Users can input patient data into the model following the instructions provided in the figure. For example, for a male patient aged 83 with an aneurysm located in an AcomA, no arteriosclerosis, no hyperlipidemia, no previous ischemic comorbidity, GCS score of 15, modified Fisher scale of 2, Hunt and Hess Grade of 2, ratio of aneurysm neck to maximum diameter of 5.8, no IPR and treated with FD stents (Figure 7), the model estimated a 28.11% probability of PIS.
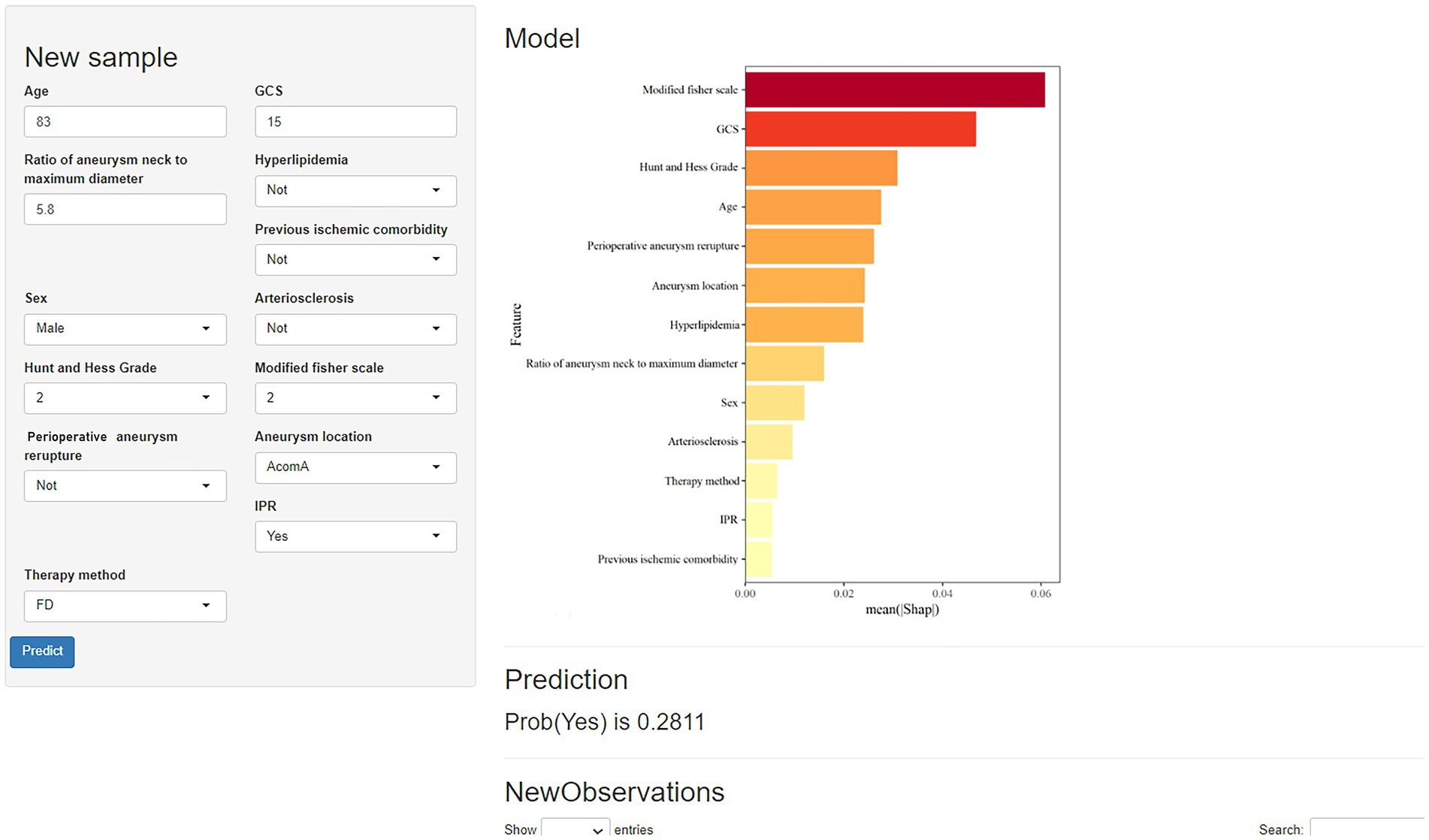
Figure 7. Prediction model of perioperative ischemic stroke in the neuroscience center of the Second Affiliated Hospital of Chongqing Medical University.
It is important to note that the best prediction probability for this model may be truncated to 19.40%. Therefore, patients with a predicted probability exceeding 36.66% are considered at higher risk for PIS. Medical professionals and caregivers should closely monitor individuals with these characteristics and take appropriate actions. Additionally, a web-based calculator based on the proposed model was developed for use by physicians. It is accessible at: Prediction model of perioperative ischemic stroke in the neuroscience center of the Second Affiliated Hospital of Chongqing Medical University (shinyapps.io).
3.4 Prospective study to validate
The data of 34 patients were prospectively collected for verification, with 23.53% (8 out of 34) of these patients developing PIS. The model achieved an accuracy of 88.23% on the validation dataset. Notably, all four patients with a predicted PIS probability above the predefined threshold subsequently developed complications, yielding a positive predictive value (PPV) of 100%. Conversely, among patients classified as low-risk, four false-negative cases were identified, while the remaining patients remained complication-free.
4 Discussion
The occurrence of PIS following endovascular therapy is a critical concern for practitioners. This study selected the best model as the RF model through the method of machine learning, which has good accuracy (84%) and sensitivity (85%) in identifying PIS. On this basis, a simple and easy-to-operate online calculator was developed, providing clinicians with an efficient and scientific method and tool for comprehensively predicting and evaluating the risk of PIS in RAI patients after endovascular therapy, and offering a preliminary theoretical basis for targeted treatment.
RIAs often lead to subarachnoid hemorrhage (SAH), which can predispose to cerebral vasospasm (CVS), with over 50% of CVS patients being at risk of developing ischemic nerve damage (28), thereby increasing the likelihood of cerebral ischemia. However, quantifying CVS poses challenges. Frontera et al. demonstrated that the Modified Fisher scale was more accurate in predicting symptomatic vasospasm after SAH (29). Therefore, using the Modified Fisher scale as an evaluation of CVS is also a good choice. Meanwhile, studies have shown that the modified Fisher score, Hunt and Hess classification, and GCS are reliable indicators for evaluating the prognosis of patients (30). Therefore, this study investigated their relationship with PIS. As depicted in Figure 6C, the Modified Fisher scale and Hunt and Hess grade showed a strong positive correlation with the occurrence of PIS, whereas the GCS exhibited a strong negative correlation with the occurrence of PIS. In this study, the incidence of PIS was 7.97% for grade I, 23.68% for grade II, 45.00% for grade III, and 61.73% for grade IV. Different Hunt and Hess grades also demonstrated varying incidence rates of PIS: 10.75% for grade I, 30.35% for grade II, 52.38% for grade III, and 72.00% for grade IV, with statistically (p < 0.001). According to the latest guidelines (31), a higher modified Fisher scale and Hunt and Hess Grade indicate that earlier surgical intervention may be beneficial in reducing the occurrence of cerebral ischemia and improving functional outcomes. Therefore, successful operation as early as possible may be an effective method to reduce the incidence of PIS in clinical studies.
Perioperative aneurysm re-rupture is one of the most serious adverse events in the endovascular treatment of aneurysms. Existing studies have reported that it can increase the incidence of PIS in patients with RIAs (32). According to various studies, several mechanisms may contribute to perioperative re-rupture. First, aneurysm rupture may be linked to variations in vascular fragility (33). Furthermore, mechanical disruption of the aneurysmal wall may occur during therapeutic embolization procedures due to device-tissue interactions, particularly involving neurointerventional instruments such as microguidewires, microcatheters, or embolization coils. This iatrogenic trauma predisposes to IPR, particularly in cases with fragile vascular architecture (34, 35). The size of the instrument used may be related to the size of the perforation as well as the prognosis. The morbidity and mortality of small puncture wounds caused by micro-guidewires with a diameter of approximately 0.33 mm are lower than those caused by coils or microcatheters (36). Microcatheters with diameters of 0.5–1.0 mm and coils with diameters similar to those of micro-guidewires tend to produce more aneurysm wall tears (35, 36). Coils 2–20 mm in diameter lead to larger perforations and may increase PIS morbidity and mortality. Third, simple intra-aneurysm injection of contrast agent may also induce perioperative aneurysm re-rupture, and the sudden increase in arterial pressure caused by patient pain may also be an important factor in perioperative aneurysm re-rupture (33–35). Therefore, effectively preventing the occurrence of perioperative aneurysm re-rupture may be an effective preventive measure to reduce the occurrence of PIS in patients with RIAs.
Interestingly, this study also found that the location of the aneurysm was one of the most crucial factors in the RF model. The rate of PIS in aneurysms at different locations is also different: AcomA was 20.78%, PcomA was 24.15%, ACA was 31.25%, MCA was 28.43%, PCA was 13.33%, ICA was 23.61%, ChoA was 47.06%, PICA was 20.00%, VA was 57.14%, BA was 44.44%, OA was 12.50%, and the difference was statistically significant (p < 0.001). The latest research shows that the incidence of symptomatic cerebral vasospasm after surgery varies among RIA patients with aneurysms at different locations (37). Since cerebral vasospasm often leads to ischemic stroke, the probability of occurrence of PIS is different. Meanwhile, Kocur et al. found that the difficulty of establishing surgical access for aneurysms at different locations varies, thereby resulting in different probabilities of vascular injury and spasm (38). It can be seen from this that endovascular therapy should be carried out by more experienced doctors or more carefully, which may be an effective measure to reduce the occurrence of PIS.
Meanwhile, studies have shown that different endovascular treatment methods (EC, SAC, and FD) for IAs cause different ischemic events, and thus, the probability of developing PIS also varies (39–41). During EC treatment, potential sources of embolization include fragile plaques, iatrogenic dissection in the parent vessel, air bubbles, and materials such as hydrophilic coating materials on catheters and guide wires during insertion or contrast media injection (9, 42, 43). SAC and FD procedures often involve larger-diameter assisted catheters and longer procedure times, which increases the risk of vascular embolism caused by catheters and mechanical operations (44–46). Additionally, the experience of the endovascular neurosurgeon plays a crucial role; less experienced surgeons may require longer procedure times, potentially increasing the risk of intraoperative thrombosis (47). In this study, the incidence of PIS differed among treatment groups: EC (20.60%), SAC (26.25%), and FD (37.31%). Therefore, prior to aneurysm intervention, individualized selection of interventional stent types or whether to use stents for different patients, and minimizing the operation time and steps during the operation, may be an effective strategy to reduce the occurrence of PIS.
In this study, machine learning techniques were used to develop a predictive model for PIS following interventional treatment of RIAs, using nine distinct prediction models. The RF model, identified as the most effective, provides clinicians with enhanced insights into the likelihood of PIS occurrence among different patients. Identifying high-risk patients allows proactive implementation of appropriate preventive measures aimed at reducing PIS incidence and alleviating the associated medical insurance burden (48).
5 Limitations
Our study has several limitations that warrant consideration. First, despite our dataset comprising over 600 patients, the data were sourced from a single center, potentially limiting the generalizability of our findings. Furthermore, while we analyzed multiple key factors, there may be additional variables that were not included in our analysis, which could impact the predictive accuracy of our model. Second, the size of our research sample for developing the prediction model was relatively small. Larger datasets are essential for robust training and testing of predictive models to enhance reliability and applicability in clinical settings. Finally, the data used in this study were derived solely from a single center, which may not yield consistent results when applied to different institutions. Future research efforts will focus on collecting extensive datasets and incorporating diverse features from multiple centers to refine the model and optimize its suitability for broader clinical applications.
6 Conclusion
PIS stands as the most prevalent complication associated with the endovascular treatment of aneurysms. In our study, we developed nine distinct prediction models for PIS, ultimately selecting the RF model as the optimal predictor based on its superior performance across various metrics in both training and test datasets. The RF model consistently demonstrated the lowest Brier score and high accuracy compared to other classifier models evaluated. By deploying this model and creating a user-friendly web-based calculator, we anticipate it will serve as a valuable tool in clinical practice. This technology enables healthcare providers to effectively identify patients at heightened risk of PIS during endovascular treatment, facilitating the timely implementation of appropriate preventive measures. This approach can enhance the application scope and practicability of risk prediction models, which will enable clinicians to make decisions with more adequate data support, thereby improving patient prognosis and reducing the incidence of PIS.
Data availability statement
The raw data supporting the conclusions of this article will be made available by the authors without undue reservation.
Ethics statement
The studies involving humans were approved by Ethics Committee of the Second Affiliated Hospital of Chongqing Medical University. The studies were conducted in accordance with the local legislation and institutional requirements. The participants provided their written informed consent to participate in this study.
Author contributions
YP: Writing – original draft, Validation, Visualization, Data curation, Investigation, Methodology. KB: Conceptualization, Writing – original draft, Project administration, Formal Analysis. XZ: Formal Analysis, Resources, Writing – original draft, Investigation, Methodology. NH: Writing – original draft, Validation, Investigation, Funding acquisition, Supervision. XJ: Resources, Methodology, Writing – original draft. WC: Writing – original draft, Methodology, Resources. YM: Data curation, Project administration, Writing – original draft. YC: Methodology, Conceptualization, Formal analysis, Software, Writing – original draft. YJ: Methodology, Writing – review & editing, Funding acquisition, Validation. JY: Writing – review & editing, Formal Analysis, Project administration, Investigation, Visualization, Data curation.
Funding
The author(s) declare that financial support was received for the research and/or publication of this article. This work was supported by the Joint Project of Pinnacle Disciplinary Group, the Second Affiliated Hospital of Chongqing Medical University (2024310).
Conflict of interest
The authors declare that the research was conducted in the absence of any commercial or financial relationships that could be construed as a potential conflict of interest.
Generative AI statement
The authors declare that no Gen AI was used in the creation of this manuscript.
Publisher’s note
All claims expressed in this article are solely those of the authors and do not necessarily represent those of their affiliated organizations, or those of the publisher, the editors and the reviewers. Any product that may be evaluated in this article, or claim that may be made by its manufacturer, is not guaranteed or endorsed by the publisher.
Supplementary material
The Supplementary material for this article can be found online at: https://www.frontiersin.org/articles/10.3389/fneur.2025.1599856/full#supplementary-material
References
1. Diaz, O, and Rangel-Castilla, L. Endovascular treatment of intracranial aneurysms. Handb Clin Neurol. (2016) 136:1303–9. doi: 10.1016/B978-0-444-53486-6.00067-3
2. Etminan, N, Dörfler, A, and Steinmetz, H. Unruptured intracranial aneurysms- pathogenesis and individualized management. Dtsch Arztebl Int. (2020) 117:235–42. doi: 10.3238/arztebl.2020.0235
3. Neifert, SN, Chapman, EK, Martini, ML, Shuman, WH, Schupper, AJ, Oermann, EK, et al. Aneurysmal subarachnoid hemorrhage: the last decade. Transl Stroke Res. (2021) 12:428–46. doi: 10.1007/s12975-020-00867-0
4. Molyneux, AJ, Kerr, RS, Yu, LM, Clarke, M, Sneade, M, Yarnold, JA, et al. International subarachnoid aneurysm trial (ISAT) of neurosurgical clipping versus endovascular coiling in 2143 patients with ruptured intracranial aneurysms: a randomised comparison of effects on survival, dependency, seizures, rebleeding, subgroups, and aneurysm occlusion. Lancet. (2005) 366:809–17. doi: 10.1016/S0140-6736(05)67214-5
5. Molyneux, AJ, Birks, J, Clarke, A, Sneade, M, and Kerr, RS. The durability of endovascular coiling versus neurosurgical clipping of ruptured cerebral aneurysms: 18 year follow-up of the UK cohort of the international subarachnoid aneurysm trial (ISAT). Lancet. (2015) 385:691–7. doi: 10.1016/S0140-6736(14)60975-2
6. Fanning, JP, Campbell, BCV, Bulbulia, R, Gottesman, RF, Ko, SB, Floyd, TF, et al. Perioperative stroke. Nat Rev Dis Primers. (2024) 10:3. doi: 10.1038/s41572-023-00487-6
7. Smilowitz, NR, Gupta, N, Ramakrishna, H, Guo, Y, Berger, JS, and Bangalore, S. Perioperative major adverse cardiovascular and cerebrovascular events associated with noncardiac surgery. JAMA Cardiol. (2017) 2:181–7. doi: 10.1001/jamacardio.2016.4792
8. Malinova, V, Schatlo, B, Voit, M, Suntheim, P, Rohde, V, and Mielke, D. The impact of temporary clipping during aneurysm surgery on the incidence of delayed cerebral ischemia after aneurysmal subarachnoid hemorrhage. J Neurosurg. (2018) 129:84–90. doi: 10.3171/2017.3.JNS162505
9. Ihn, YK, Shin, SH, Baik, SK, and Choi, IS. Complications of endovascular treatment for intracranial aneurysms: management and prevention. Interv Neuroradiol. (2018) 24:237–45. doi: 10.1177/1591019918758493
10. Zhang, Y, Wang, C, Tian, Z, Zhu, W, Li, W, Yang, X, et al. Risk factors for periprocedural ischemic stroke following endovascular treatment of intracranial aneurysms. Chin Neurosurg J. (2021) 7:38 2021 Aug 23. doi: 10.1186/s41016-021-00255-z
11. Vlisides, PE, Moore, LE, Whalin, MK, Robicsek, SA, Gelb, AW, Lele, AV, et al. Perioperative Care of Patients at high risk for stroke during or after non-cardiac, non-neurological surgery: 2020 guidelines from the Society for Neuroscience in anesthesiology and critical care. J Neurosurg Anesthesiol. (2020) 32:210–26. doi: 10.1097/ANA.0000000000000686
12. Świątnicki, W, Szymański, J, Szymańska, A, and Komuński, P. Predictors of intraoperative aneurysm rupture, aneurysm remnant, and brain ischemia following microsurgical clipping of intracranial aneurysms: single-center, retrospective cohort study. J Neurol Surg A Cent Eur Neurosurg. (2021) 82:410–6. doi: 10.1055/s-0040-1721004
13. Wang, JW, Li, CH, Liu, JF, Li, H, Guo, H, and Gao, BL. Endovascular treatment of multiple intracranial aneurysms. Medicine (Baltimore). (2023) 102:e36340. doi: 10.1097/MD.0000000000036340
14. Pierot, L, Gawlitza, M, and Soize, S. Unruptured intracranial aneurysms: management strategy and current endovascular treatment options. Expert Rev Neurother. (2017) 17:977–86. doi: 10.1080/14737175.2017.1371593
15. Tian, Z, Li, W, Feng, X, Sun, K, and Duan, C. Prediction and analysis of periprocedural complications associated with endovascular treatment for unruptured intracranial aneurysms using machine learning. Front Neurol. (2022) 13:1027557. Published 2022 Oct 12. doi: 10.3389/fneur.2022.1027557
16. Ma, Y, Liu, S, Zhang, F, Cong, X, Zhao, B, Sun, M, et al. Risk factor analysis and creation of an externally-validated prediction model for perioperative stroke following non-cardiac surgery: a multi-center retrospective and modeling study. PLoS Med. (2025) 22:e1004539. doi: 10.1371/journal.pmed.1004539
17. Liu, S, Wang, B, Ma, L, Yang, H, Liu, M, Song, Y, et al. The association between aspartate transaminase to alanine transaminase ratio and perioperative ischemic stroke in patients with diabetes: a retrospective cohort study. CNS Neurosci Ther. (2025) 31:e70223. doi: 10.1111/cns.70223
18. Deo, RC. Machine learning in medicine. Circulation. (2015) 132:1920–30. doi: 10.1161/CIRCULATIONAHA.115.001593
19. Handelman, GS, Kok, HK, Chandra, RV, Razavi, AH, Lee, MJ, and Asadi, H. eDoctor: machine learning and the future of medicine. J Intern Med. (2018) 284:603–19. doi: 10.1111/joim.12822
20. Erickson, BJ. Basic artificial intelligence techniques: machine learning and deep learning. Radiol Clin North Am. (2021) 59:933–40. doi: 10.1016/j.rcl.2021.06.004
21. Skrivankova, VW, Richmond, RC, Woolf, BAR, Yarmolinsky, J, Davies, NM, Swanson, SA, et al. Strengthening the reporting of observational studies in epidemiology using Mendelian randomization: the STROBE-MR statement. JAMA. (2021) 326:1614–21. doi: 10.1001/jama.2021.18236
22. Page, MJ, McKenzie, JE, Bossuyt, PM, Boutron, I, Hoffmann, TC, Mulrow, CD, et al. The PRISMA 2020 statement: an updated guideline for reporting systematic reviews. BMJ. (2021) 372:n71. doi: 10.1136/bmj.n71
23. Han, J, Liu, F, Chen, J, Tong, X, Han, M, Peng, F, et al. Periprocedural cerebrovascular complications and 30-day outcomes of endovascular treatment for intracranial vertebral artery dissecting aneurysms. J Neurosurg. (2022) 138:1503–11. doi: 10.3171/2022.10.JNS221953
24. Mullah, MAS, Hanley, JA, and Benedetti, A. LASSO type penalized spline regression for binary data. BMC Med Res Methodol. (2021) 21:83. doi: 10.1186/s12874-021-01234-9
25. Zhang, Z, Rousson, V, Lee, WC, Ferdynus, C, Chen, M, Qian, X, et al. Decision curve analysis: a technical note. Ann Transl Med. (2018) 6:308. doi: 10.21037/atm.2018.07.02
26. Ou, C, Liu, J, Qian, Y, Chong, W, Zhang, X, Liu, W, et al. Rupture risk assessment for cerebral aneurysm using interpretable machine learning on multidimensional data. Front Neurol. (2020) 11:570181. doi: 10.3389/fneur.2020.570181
27. Miller, DM, and Shalhout, SZ. Storyboardr: an R package and shiny application designed to visualize real-world data from clinical patient registries. JAMIA Open. (2023) 6:ooac109. doi: 10.1093/jamiaopen/ooac109
28. Diringer, MN, and Zazulia, AR. Aneurysmal subarachnoid hemorrhage: strategies for preventing vasospasm in the intensive care unit. Semin Respir Crit Care Med. (2017) 38:760–7. doi: 10.1055/s-0037-1607990
29. Frontera, JA, Claassen, J, Schmidt, JM, Wartenberg, KE, Temes, R, Connolly, ES, et al. Prediction of symptomatic vasospasm after subarachnoid hemorrhage: the modified fisher scale. Neurosurgery. (2006) 59:21–7. doi: 10.1227/01.neu.0000243277.86222.6c
30. Roquer, J, Cuadrado-Godia, E, Guimaraens, L, Conesa, G, Rodríguez-Campello, A, Capellades, J, et al. Short- and long-term outcome of patients with aneurysmal subarachnoid hemorrhage. Neurology. (2020) 95:e1819–29. doi: 10.1212/WNL.0000000000010618
31. Hoh, BL, Ko, NU, Amin-Hanjani, S, Chou, SH-Y, Cruz-Flores, S, Dangayach, NS, et al. 2023 guideline for the management of patients with aneurysmal subarachnoid hemorrhage: a guideline from the American Heart Association/American Stroke Association. Stroke. (2023) 54:e314–70. doi: 10.1161/STR.0000000000000436
32. van Lieshout, JH, Verbaan, D, Donders, R, van den Berg, R, Vandertop, PWP, Klijn, CJM, et al. Periprocedural aneurysm rerupture in relation to timing of endovascular treatment and outcome. J Neurol Neurosurg Psychiatry. (2019) 90:363–5. doi: 10.1136/jnnp-2018-318090
33. Elijovich, L, Higashida, RT, Lawton, MT, Duckwiler, G, Giannotta, S, Johnston, SC, et al. Predictors and outcomes of intraprocedural rupture in patients treated for ruptured intracranial aneurysms: the CARAT study. Stroke. (2008) 39:1501–6. doi: 10.1161/STROKEAHA.107.504670
34. Luo, CB, Mu-Huo Teng, M, Chang, FC, Lin, CJ, Guo, WY, and Chang, CY. Intraprocedure aneurysm rupture in embolization: clinical outcome with imaging correlation. J Chin Med Assoc. (2012) 75:281–5. doi: 10.1016/j.jcma.2012.04.008
35. Chen, M. A checklist for cerebral aneurysm embolization complications. J Neurointerv Surg. (2013) 5:20–7. doi: 10.1136/neurintsurg-2011-010137
36. Brisman, JL, Niimi, Y, Song, JK, and Berenstein, A. Aneurysmal rupture during coiling: low incidence and good outcomes at a single large volume center. Neurosurgery. (2005) 57:1103–9. doi: 10.1227/01.neu.0000185631.20246.1a
37. Moriya, M, Karako, K, Miyazaki, S, Minakata, S, Satoh, S, Abe, Y, et al. Interpretable machine learning model for outcome prediction in patients with aneurysmatic subarachnoid hemorrhage. Crit Care. (2025) 29:36. doi: 10.1186/s13054-024-05245-y
38. Kocur, D, Przybyłko, N, Bażowski, P, and Baron, J. Rupture during coiling of intracranial aneurysms: predictors and clinical outcome. Clin Neurol Neurosurg. (2018) 165:81–7. doi: 10.1016/j.clineuro.2018.01.006
39. Piotin, M, Blanc, R, Spelle, L, Mounayer, C, Piantino, R, Schmidt, PJ, et al. Stent-assisted coiling of intracranial aneurysms: clinical and angiographic results in 216 consecutive aneurysms. Stroke. (2010) 41:110–5. doi: 10.1161/STROKEAHA.109.558114
40. Adeeb, N, Griessenauer, CJ, Moore, JM, Foreman, PM, Shallwani, H, Motiei-Langroudi, R, et al. Ischemic stroke after treatment of Intraprocedural thrombosis during stent-assisted coiling and flow diversion. Stroke. (2017) 48:1098–100. doi: 10.1161/STROKEAHA.116.016521
41. Ge, H, Yang, H, Ren, H, Li, W, Lv, X, Jin, H, et al. Association of thrombelastographic parameters with complications in patients with intracranial aneurysm after stent placement. World Neurosurg. (2019) 127:e30–8. doi: 10.1016/j.wneu.2019.02.007
42. Platz, J, Wagner, M, Güresir, E, You, SJ, Konczalla, J, de Rochemont, RM, et al. Early diffusion-weighted MRI lesions after treatment of unruptured intracranial aneurysms: a prospective study. J Neurosurg. (2017) 126:1070–8. doi: 10.3171/2016.2.JNS152456
43. Kim, MJ, Lim, YC, Oh, SY, Kim, BM, Kim, BS, and Shin, YS. Thromboembolic events associated with electrolytic detachment of Guglielmi detachable coils and target coils: comparison with use of diffusion-weighted MR imaging. J Korean Neurosurg Soc. (2013) 54:19–24. doi: 10.3340/jkns.2013.54.1.19
44. Park, JC, Lee, DH, Kim, JK, Ahn, JS, Kwun, BD, Kim, DY, et al. Microembolism after endovascular coiling of unruptured cerebral aneurysms: incidence and risk factors. J Neurosurg. (2016) 124:777–83. doi: 10.3171/2015.3.JNS142835
45. Nakae, R, Nagaishi, M, Kawamura, Y, Tanaka, Y, Hyodo, A, and Suzuki, K. Microhemorrhagic transformation of ischemic lesions on T2*-weighted magnetic resonance imaging after pipeline embolization device treatment. J Neurosurg. (2018) 130:1997–2004. doi: 10.3171/2017.12.JNS172480
46. Pikis, S, Mantziaris, G, Mamalis, V, Barkas, K, Tsanis, A, Lyra, S, et al. Diffusion weighted image documented cerebral ischemia in the postprocedural period following pipeline embolization device with shield technology treatment of unruptured intracranial aneurysms: a prospective, single center study. J Neurointerv Surg. (2020) 12:407–11. doi: 10.1136/neurintsurg-2019-015363
47. Yue, J, Xie, Y, Zhang, X, Jiang, Y, Chen, W, Ma, Y, et al. The safety and outcomes of acutely ruptured intracranial aneurysms with incomplete occlusion after coiling: a case-control study. BMC Neurol. (2020) 20:10. doi: 10.1186/s12883-020-01991-y
Keywords: complication prediction, automated machine learning, endovascular therapy, intracranial aneurysms, perioperative ischemia stroke
Citation: Peng Y, Bi K, Zhang X, Huang N, Ji X, Chen W, Ma Y, Cheng Y, Jiang Y and Yue J (2025) Automated machine learning for predicting perioperative ischemia stroke in endovascularly treated ruptured intracranial aneurysm patients. Front. Neurol. 16:1599856. doi: 10.3389/fneur.2025.1599856
Edited by:
Guang-xian Wang, Xinqiao Hospital, ChinaReviewed by:
Jianjun Gu, People’s Hospital of Zhengzhou University, ChinaYu Zhou, Naval Military Medical University, China
Copyright © 2025 Peng, Bi, Zhang, Huang, Ji, Chen, Ma, Cheng, Jiang and Yue. This is an open-access article distributed under the terms of the Creative Commons Attribution License (CC BY). The use, distribution or reproduction in other forums is permitted, provided the original author(s) and the copyright owner(s) are credited and that the original publication in this journal is cited, in accordance with accepted academic practice. No use, distribution or reproduction is permitted which does not comply with these terms.
*Correspondence: Yongxiang Jiang, ZG9jdG9yamlhbmcyMDAzQGhvc3BpdGFsLmNxbXUuZWR1LmNu; Jianhe Yue, eXVlMjAyMEBzdHUuY3FtdS5lZHUuY24=
†These authors have contributed equally to this work and share first authorship