- 1 Institute of Medical Psychology and Behavioural Neurobiology, University of Tübingen, Tübingen, Germany
- 2 Human Media Interaction, University of Twente, Enschede, Netherlands
- 3 Ospedale San Camillo Venezia, Istituto di Ricovero e Cura a Carattere Scientifico, Venice, Italy
- 4 Department of Psychology I, University of Würzburg, Würzburg, Germany
The current study investigated the effects of psychological well-being measured as quality of life (QoL), depression, current mood and motivation on brain–computer interface (BCI) performance in amyotrophic lateral sclerosis (ALS). Six participants with most advanced ALS were trained either for a block of 20 sessions with a BCI based on sensorimotor rhythms (SMR) or a block of 10 sessions with a BCI based on event-related potentials, or both. Questionnaires assessed QoL and severity of depressive symptoms before each training block and mood and motivation before each training session. The SMR-BCI required more training than the P300-BCI. The information transfer rate was higher with the P300-BCI (3.25 bits/min) than with the SMR-BCI (1.16 bits/min). Mood and motivation were related to the number of BCI sessions. Motivational factors, specifically challenge and mastery confidence, were positively related to BCI performance (controlled for the number of sessions) in tow participants, while incompetence fear was negatively related with performance in one participant. BCI performance was not related to motivational factors in three other participants nor to mood in any of the six participants. We conclude that motivational factors may be related to BCI performance in individual subjects and suggest that motivational factors and well-being should be assessed in standard BCI protocols. We also recommend using P300-based BCI as first choice in severely paralyzed patients who present with a P300 evoked potential.
Introduction
Brain–computer interfaces (BCI) may provide severely paralyzed users communication and control without motor responses (Kübler and Birbaumer, 2008). A BCI consists of several functional components: the input for a BCI originates from a user who “produces” brain signals (e.g., electroencephalogram, EEG; electrocorticogram, EcoG), the output of a BCI (e.g., commands for a spelling program) and the translation of input into output (signal detection, feature extraction, classification) (Kübler et al., 2001a; Mason and Birch, 2003; Kübler and Neumann, 2005).
Different EEG signals can be used to control a BCI, e.g., sensorimotor rhythms (SMR; (Pfurtscheller et al., 2000; Kübler et al., 2005a), slow cortical potentials (SCP; (Birbaumer et al., 1999; Kübler et al., 1999) and the P300 event-related potential (ERP; (Farwell and Donchin, 1988; Sellers and Donchin, 2006; Nijboer et al., 2008b; Furdea et al., 2009).
Sensorimotor or mu-rhythm (SMR) refers to 8–15 Hz EEG activity which can be recorded over primary sensory and motor cortical areas (Niedermeyer, 2004). It is usually accompanied by 18–26 Hz beta-rhythms. It decreases or desynchronizes with movement, preparation for, or imagery of movement (event-related desynchronization – ERD) and increases or synchronizes (event-related synchronization – ERS) in the post-movement period or during relaxation (Pfurtscheller and Aranibar, 1979; Pfurtscheller et al., 1999; Pfurtscheller and Neuper, 2010). BCIs based on SMR are also referred to as ERD/S-BCIs (Pfurtscheller and Neuper, 2010). In a study by Kübler et al. (2005a,b,c) all four participating amyotrophic lateral sclerosis (ALS) patients were able to achieve SMR-regulation of more than 75% accuracy within less than 20 training sessions. Performance was around chance level during the first 10 sessions, but increased significantly during the last 10 sessions.
The P300 component of the ERP is a positive deflection in the EEG time-locked to auditory or visual stimuli. It is typically seen when participants are required to attend to a stream of sensory standard stimuli interspersed with rare deviant stimuli, typically referred to as targets (Squires et al., 1977). This experimental design is often referred to as an oddball paradigm (Fabiani et al., 1987). The rare deviant stimulus will elicit different ERPs, the most typical component being the P300. A study by Nijboer et al. (2008a,b) revealed that five out of six individuals with ALS could use the P300-BCI for communication in an initial BCI training phase. Four users continued using the P300-BCI for communication (the fifth passed away) in a second phase of the study and were all able to spell messages of considerable length with the P300-BCI when more features were extracted from the EEG (Nijboer et al., 2008b).
In near future, research aims at providing severely paralyzed users with low-cost, low-risk BCIs that can be used in daily life (Kübler et al., 2006). The development of BCIs is mostly focused on improvements in signal acquisition, feature extraction, feature classification, and algorithms. Still, for yet unknown reasons, some people seem to have more difficulty in using a BCI than others and some seem unable to use a BCI (Guger et al., 2003; Kübler et al., 2004; Nijboer et al., 2008a,b). It is often hypothesized that psychological factors, for example mood and motivation of the participant, might explain some of these inter individual differences. However, few studies have systematically investigated how such non-EEG related, indirect aspects might influence and predict BCI performance (Neumann and Birbaumer, 2003; Kübler et al., 2004; Leeb et al., 2007; Nijboer et al., 2008a,b). The implementation, data analysis and maintenance of most currently available BCIs still require excessive supervision by psychologist, engineers, computer scientists, and neurologists (Kübler et al., 2006). Finding predictors for BCI performance might lead to more efficient BCI implementation (Blankertz et al., 2010).
In a first attempt to determine how psychological factors may influence BCI performance Neumann found that mood, quality of caregiving, headache, sleep, and even room temperature were related to BCI performance in some users, albeit unsystematically (Neumann, 2001). To continue this line of investigation, Nijboer et al. (2008a) related mood and motivation of the participants (N = 16 healthy subjects) measured before each training session to self-regulation of the SMR amplitude by means of visual or auditory feedback (N = 8 healthy subjects for each modality) during three daily training sessions. Four motivational factors were distinguished according to a cognitive-motivational model (Schiefele and Rheinberg, 1997; Vollmeyer and Rheinberg, 2000). First, participants can vary in their belief that they will succeed in mastering the task (mastery confidence). Second, participants indicate different levels of anxiety about failing in the task (incompetence fear). Third, participants may be challenged by the task in various degrees. And finally, the task may or may not evoke the interest of the participant.
In general, motivation can be defined as providing “an impetus toward a goal for all current processes” (Rheinberg, 1997). Two forms of motivation are often distinguished: Firstly, when participants are intrinsically motivated, they engage in an activity, such as learning to use a BCI, because they are interested and enjoy the activity (Eccles and Wigfield, 2002). Secondly, when extrinsically motivated, participants engage in activities for instrumental or other reasons, such as receiving a reward (Eccles and Wigfield, 2002). Patients with ALS might be more intrinsically motivated by the BCI task because of the possible future use of the BCI system in their daily life or because they would like to contribute to BCI research. On the contrary, healthy participants in BCI studies might be more extrinsically motivated because of the monetary reward for participation in the experiment.
In the study of Nijboer et al. (2008a) better mood and mastery confidence were related to better SMR-regulation provided visual feedback, whereas higher ratings of incompetence fear were related to worse SMR-regulation. Conversely, with auditory feedback higher scores of incompetence fear were related to better SMR-regulation. The authors suggested that when performance is high from the beginning of the training (as found with visual feedback), the incompetence fear in further sessions or loss of interest may hamper performance and further learning. However, when performance is low at initial training (as found with auditory feedback) moderate incompetence fear might boost performance (Nijboer et al., 2008a).
Leeb et al. (2007) came to similar conclusions after a study in which the authors trained 10 healthy people first in a cue-based (two-target) neurofeedback paradigm based on SMR and second in exploring a virtual apartment on a computer again controlled by the self-regulation of SMR. Subjects reported a motivation boost when switching from a standard cue-based feedback to feedback in the virtual apartment. In the first session in which they could navigate through the virtual world a slight drop in performance could be seen, especially in the good subjects. This drop was explained by the authors by the unfamiliar and exciting new task (Leeb et al., 2007). Contrary to that, poor performing subjects improved significantly after being confronted with the virtual apartment task. The improvement in performance could not be explained with learning effects, because in a final session subjects were changed back to a standard cue-based feedback paradigm upon which motivation was again reported to decrease and performance dropped. Authors concluded that although good subjects always perform well, especially bad subjects can increase their performance significantly after motivational intervention.
The summarized results justify to hypothesize that a person’s mood and motivation are related to BCI performance (Kübler et al., 2001a,b; Neumann and Birbaumer, 2003; Nijboer et al., 2008a).
We further hypothesize that addressing patient’s psychological well-being is important for BCI performance. As variables contributing to psychological well-being we measured quality of life (QoL) and depression as more stable and enduring components, and current mood as a more variable, state dependent component.
According to the resource allocation model by Ellis and Ashbrook (1988) both negative and positive emotional states may impair performance. For example, word recall was impaired among individuals when pleasant and unpleasant emotional states were induced (Seibert and Ellis, 1991). Likewise, symptoms of depression may impair learning and lead to deficits in attention and executive control (Farrin et al., 2003; Porter et al., 2003). Therefore, we may speculate that intense emotional states and depression hamper BCI performance.
To date, nothing is known about the influence of QoL on BCI performance. Yet, we argue that QoL defines the frame in which BCI training of severely sick people takes place and thus, may influence BCI performance. The definition of QoL is difficult and complex due to the individual and dynamic nature of the concept (O’Boyle and Waldron, 1997). A useful distinction to make is that between health-related QoL and overall QoL (Gill and Feinstein, 1994). Health-related QoL reflects a person’s health status and can be measured by instruments that focus on physical health and overall functional ability (Robbins et al., 2001). Overall QoL also takes into account other non-medical factors (Gill and Feinstein, 1994). Carr et al. (2001) consider health-related QoL to be the gap between our expectations of how our health should be and our experience of it. This idea implies three problems with measuring health-related QoL: people have different expectations; people may be on different points of their illness trajectory when QoL is measured and the reference value of their expectation may change over time (response shifts) (Calman, 1984; Sprangers and Schwartz, 1999; Carr et al., 2001). Additionally, most health-related QoL questionnaires impose a predetermined value system on the respondent (standardized questionnaires) (O’Boyle and Waldron, 1997). Neudert et al. (2004) showed that health-related QoL questionnaires do not adequately monitor individual aspects of QoL of patients with ALS. Instead, health-related QoL questionnaires seem to be “surrogate measures for physical function.” Therefore, it is important to let a patient define what QoL is to him or her and evaluate the quality of those areas (O’Boyle and Waldron, 1997). The schedule for the evaluation of individual QoL direct weighting (SEIQoL-DW) allows us such and individual definition of QoL and can therefore reflect response shifts (Hickey et al., 1996). The SEIQoL-DW was judged as more valid than health-related QoL questionnaires by ALS patients themselves (Neudert et al., 2001).
In the current study we investigated the influence of motivation and psychological well-being (current mood, QoL, and depression) on the performance with an SMR- and P300-BCI. More specifically, we investigated the performance of severely paralyzed users with ALS, whom we suspect to be highly intrinsically motivated. In addition, this paper offers the first direct comparison of SMR- and P300-BCI performance of ALS patients, albeit in a small sample.
Materials and Methods
Participants
Seven participants with ALS were trained with the SMR-BCI (N = 1) or the P300-BCI (N = 3), or both (N = 3) (Kübler et al., 2005a; Nijboer et al., 2008b). All participants provided informed consent for the study which was approved by the Ethical Review Board of the Medical Faculty, University of Tübingen. Participants were trained in their home environment. Demographics, illness parameter, and type(s) of BCI are listed in Table 1. An eighth participant (H) was confronted with the P300-BCI, but because a reliable P300 could not be detected, was not included in the study. Participants H and C were locked in with increasing difficulty to move their eyes or to blink. Participant B had already been in a wheelchair due to a car accident. Her medication included morphine against phantom limb pain. Her dosage of morphine was increased during the P300-study.
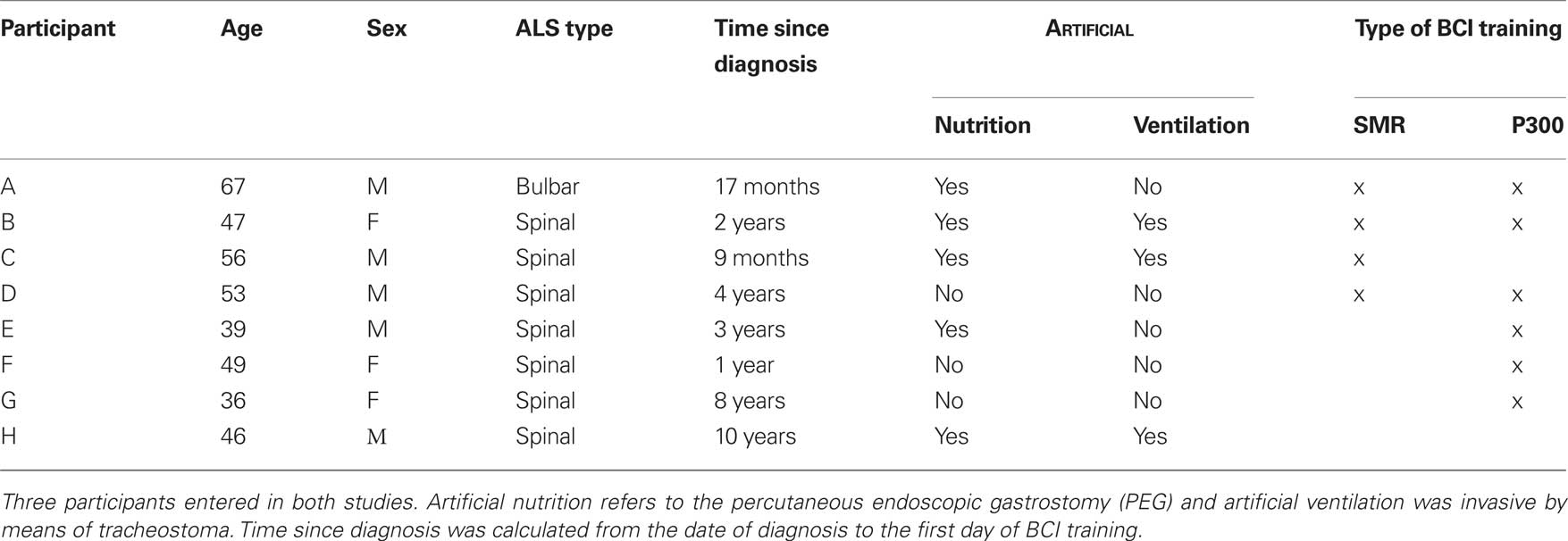
Table 1. Demographic data and illness parameters of participants, and type of BCI used for training.
BCI Training
Four participants practiced the SMR-BCI for 20 sessions over the course of 3–7 months. Six participants practiced the P300-BCI for 10 sessions over the course of 1.5–3.5 months. Those patients who entered both studies first practiced with the SMR-BCI and after 20 sessions started practicing with the P300-BCI. For an extensive description of the training paradigms see Kübler et al. (2005a) and Nijboer et al. (2008b). We will refer to all 20 SMR sessions as SMR training and to all 10 P300 sessions as P300 training.
To learn to modulate the power of SMR, patients were presented with one-dimensional cursor movement on a computer screen (Kübler et al., 2005a). During each trial, users were presented with a target consisting of a red vertical bar that occupied the top or bottom half of the right edge of the screen and a cursor on the left edge. The cursor moved steadily across the screen, with its vertical movement controlled by the SMR amplitude. The patients’ task was to move the cursor to the level of the target so that it hit the target when it reached the right edge. Low SMR amplitude following movement imagery moved the cursor to the bottom bar, thinking of nothing in particular (“relaxation”) moved the cursor toward the top bar (Kübler et al., 2005a). Each session had eight to fifteen 2- to 3-min runs comprising 20–35 trials, and separated by 1- to 5-min breaks. Thus, a typical session lasted everything from 30 to 70 min. The correct response rate (CRR) was defined as the percentage of hit targets within one session.
In the P300-BCI participants were presented with a 6 × 6 matrix where each of the 36 cells contained a character or a symbol (Farwell and Donchin, 1988; Nijboer et al., 2008b). This design becomes an oddball paradigm by first, intensifying each row and column in random order and second, by instructing participants to attend to only one of the 36 cells. Thus, in one trial of 12 flashes (6 rows and 6 columns), the target cell will flash only twice constituting a rare event compared to the 10 flashes of all other rows and columns and will therefore elicit a P300 that can be detected by the BCI (Nijboer et al., 2008b). In each session, the nine words of the German sentence “Franz jagt im komplett verwahrlosten Taxi quer durch Bayern” (translation: “Franz chases in a completely shabby taxi across Bavaria”) were each presented as separate text-to-copy runs for a total of 51 selections (Nijboer et al., 2008b). This sentence was chosen because it contains all letters of the German alphabet. Performance was defined as the percentage of correctly selected characters (CSC) of this sentence.
To compare performance it has to be taken into account that the chance level to hit a target (chance = 1/2 = 0.5) or to select a correct character (chance = 1/36 = 0.027) differs between the paradigms and depends on the number of trials per session (Kübler and Birbaumer, 2008). Therefore, the information transfer rate (bits/min) per session was calculated according to the formula suggested by Wolpaw et al. (2000).
Procedure
Before starting with SMR or P300 training blocks, physical impairment, QoL, and depression were assessed using questionnaires. The assessment lasted 1.5–3 h depending on communication abilities of the participant. All questionnaires were filled out by the participant themselves when they were able to hold a pen or otherwise by the first author. Before each training session mood and motivation were assessed using questionnaires. This assessment lasted 10–30 min.
Instruments
ALS functional rating scale
The ALS Functional Rating Scale (ALSFRS; (Cedarbaum and Stambler, 1997) was used to assess disease severity in the domains of fine motor, gross motor, bulbar, and respiratory function. The ALSFRS asks patients to rate their functioning on 10 items describing activities of daily living. Patient functioning is rated on a scale from 4 (no impairment) to 0 (unable to perform task). Scores for each item are added to a total score, ranging from 0 to 40, where lower scores refer to more severe disability.
Quality of life
The SEIQoL-DW, which is a person-centered instrument, measured individual QoL (O’Boyle and Waldron, 1997). Participants firstly nominated five life domains (cues) which were important to their QoL. Secondly, they rated their current level of satisfaction in each of these domains and finally, they were required to indicate the relative importance (weight) of each of the chosen domains. To obtain a total QoL score the so-called SEIQoL-DW index score (SIS) was calculated (0–100, with higher scores reflecting higher QoL), whereby relative weight and current satisfaction were multiplied for each cue and the results were summed up.
Severity of depressive symptoms
Severity of depressive symptoms was measured with the ALS Depression Inventory with 12 items (ADI-12; (Kübler et al., 2005b; Hammer et al., 2008). The ADI-12 takes into account the specific circumstances of progressive physical impairment culminating in severe motor paralysis, artificial feeding, and ventilation. Statements addressing activities that depend on an intact motor system are excluded. Symptoms such as weakness, change in appetite, and fatigue, which are measured with the Beck Depression Inventory (Beck et al., 1961) could be physical symptoms of the disease itself and not necessarily signs of depression (Kurt et al., 2007). Participants rate how much they agreed with a statement on a 4-point Likert-type scale. The maximum value is 48. Values equal or above 23 identify all patients with any depressive disorder including minor depression. Values equal or above 30 identify all patients with a current episode of major depression (Hammer et al., 2008).
Current mood
Mood was assessed with the subscale of the “Skalen zur Erfassung der Lebensqualität” (SEL; Scales to assess Quality of Life) (Averbeck et al., 1997) called “current mood.” Participants rate how much they agree with each of 10 statements on a 5-point Likert-type scale. A total score is obtained by averaging the scores of each item. The higher the score for “current mood,” the better the mood. Examples of statements are “Today I feel relaxed,” “Today I feel I am in a good mood,” or “Today I feel restless.”
BCI motivation
Motivation was assessed with an adapted version of the Questionnaire for Current Motivation (QCM) developed by Vollmeyer and colleagues (Rheinberg et al., 2001). The QCM consists of 18 statements which have to be rated to which extent they apply on a 7-point Likert-type scale. Because we adapted the QCM to BCI training all items are listed in Table 2. A score for each of the four factors (mastery confidence, incompetence fear, interest, and challenge) is obtained by the average of the (reversed) scores on each statements belonging to the different factors.
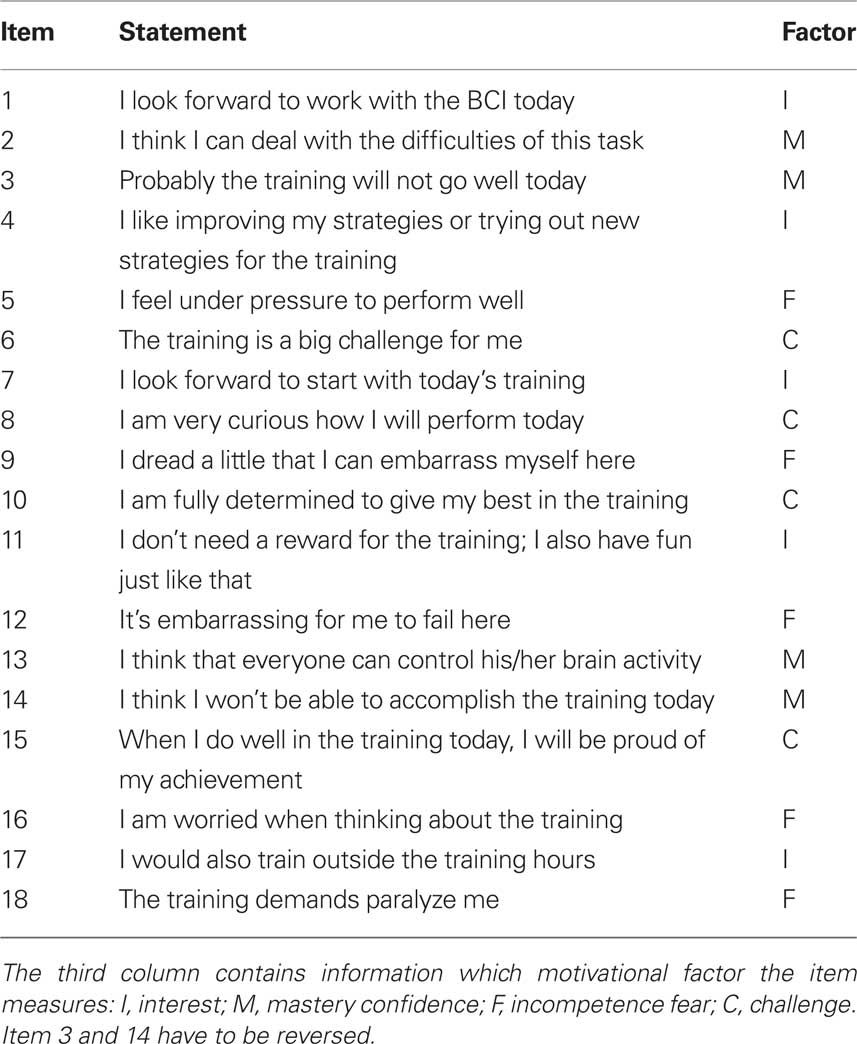
Table 2. All items of the adapted QCM are translated from German to illustrate the nature of the questionnaire (English version not validated).
Data Analysis
Considering the limited number of participants in each group, the difference between BCI performance (calculated in bits/min) during SMR- and P300-BCI training will only be reported descriptively. QoL and depression scores of patients are described and compared to results from other studies with ALS patients.
Mood and motivational factors were measured as ordinal variables, thus only non-parametric tests can be used for statistical analysis. Furthermore, SMR training consisted of 20 sessions and P300 training of 10 sessions only. To accommodate for this difference in number of sessions we used every second SMR training session (odd numbers from 1 to 19) for the correlational analysis. To address the relation between time (measured in number of sessions) and mood and motivational factors, non-parametric Spearman’s rank correlations were calculated within each individual patient. Then, the relation between BCI performance (CRR and CSC) and mood and motivational factors, was calculated using partial non-parametric (Spearman’s rank) correlations (control variable: sessions).
Results
Comparing the Information Transfer Rates
Performance data for SMR- and P300-BCI training were published in (Kübler et al., 2005a; Nijboer et al., 2008b). Figure 1 depicts the average bits per minute which were transferred during SMR-BCI sessions and during P300-BCI sessions. The information transfer rate was higher with the P300-BCI (3.25 bits/min) than with the SMR-BCI (1.16 bits/min) (Kübler et al., 2005a; Nijboer et al., 2008b). Furthermore, offline analysis, which included more spatiotemporal features in the stepwise linear discriminant analysis, showed the average information transfer rate with the P300-BCI would have been much higher (5.10 bits/min) if we had used these extra features (Krusienski et al., 2008; Nijboer et al., 2008b); it was not available during the online study. Performance increased over the SMR training (F1,18 = 82.166 p < 0.001), whereas performance remained stable during P300 training (F1,8 = 0.018; p = 0.896). (The probability of a type I error was maintained at 0.05 level for all subsequent analysis.). Those patients that entered in both the SMR as well as the P300 study all achieved their maximum information transfer rate during a session with the P300-BCI (A: 7.39 bits/min; B: 5.77 bits/min; D: 5.34 bits/min).
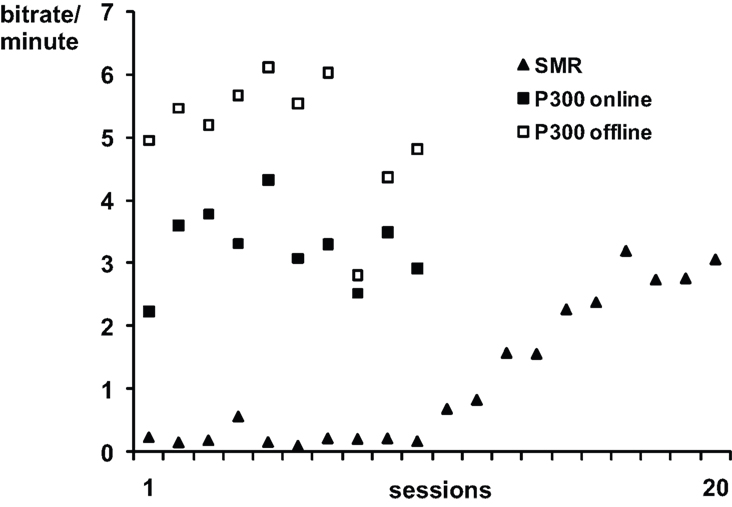
Figure 1. Average online bits per minute transferred per session in the SMR and P300-training. The offline analysis of the P300 training data is explained in Nijboer et al. (2008b). Note, that the SMR and P300 training were not conducted simultaneously, but successively.
Physical Impairment, Quality of Life, Depression, and BCI Performance
Table 3 lists physical and psychological parameters of each patient before SMR or P300 training, overall CRR/CSC (averaged over all SMR or P300 sessions), initial CRR/CSC (averaged over the first three sessions of training) and end CRR/CSC (averaged over the final three sessions of training). The average ALSFRS score of the participants was 16.5 before SMR training and 14.4 before P300 training implying increasingly severe impairment. The ADI scores indicated that participant A had a major depression disorder shortly before he started with the P300 training, which might have been a reaction to the rapid physical decline in the period between the SMR- and P300 training (ALSFRS score from 27 to 17). Nevertheless, he could spell with the highest CSC with the P300-BCI (during 7 out of 10 sessions he successfully selected more than 90% of the characters and in 2 sessions he spelled a 100% correct). With an ADI score of 23, participant D was in the range of 60% likelihood for any kind of depression before SMR- and P300-training. On average, participants were not depressed neither before SMR- (ADI = 19.8) nor P300-training (ADI = 20.7). It has to be pointed out that the ADI is a screening instrument for depression in ALS, but not a diagnostic tool. None of the participants was diagnosed with any kind of depression disorder; the reason for the lack of diagnosis and treatment of depression in ALS goes beyond the scope of this paper. Physical impairment was neither related to depression as measured with the ADI (P = 0.512, p = 0.108), nor to QoL as measured with the SEIQol-DW (P = −0.201, p = 0.554).
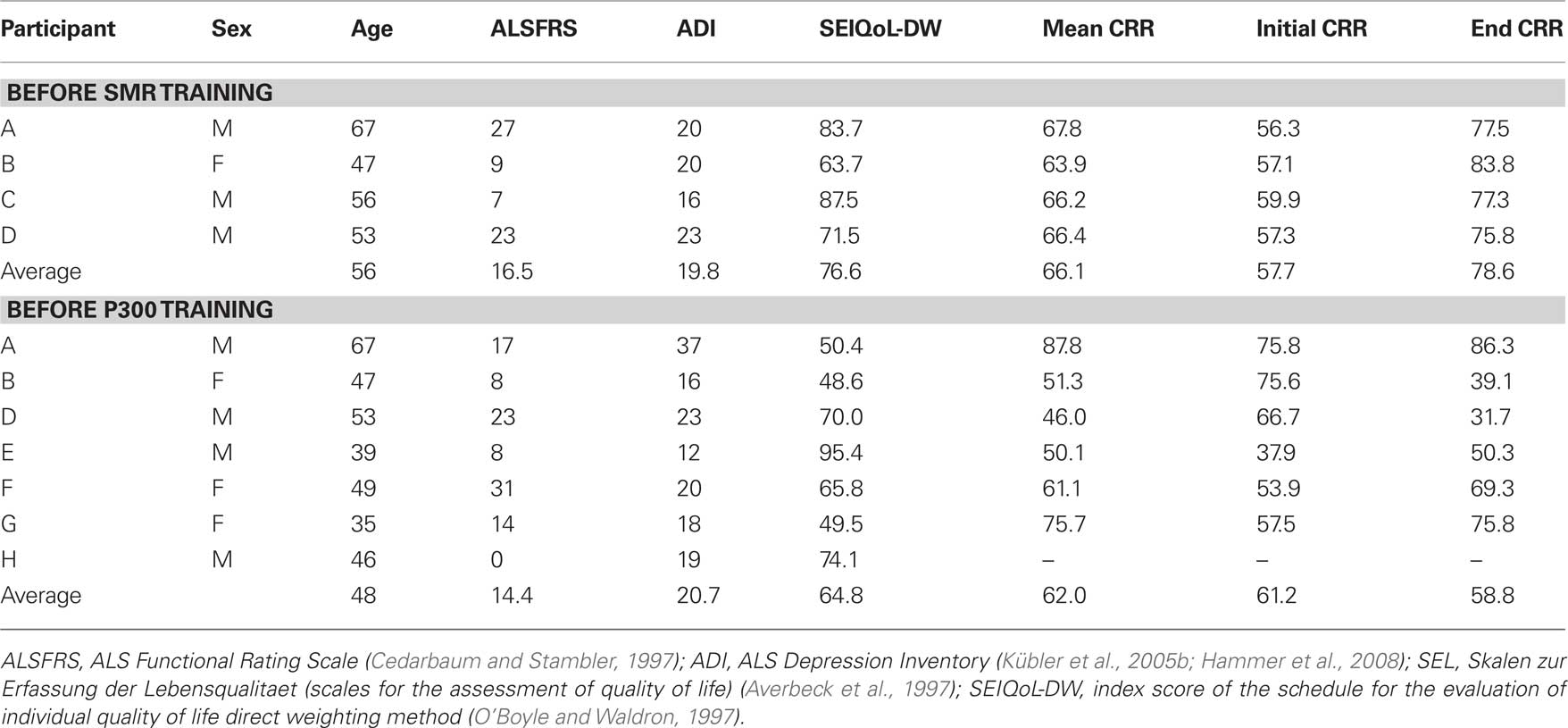
Table 3. Overview of physical and psychological parameters and the average BCI performance over all sessions (mean CRR or mean CSC), over the first three sessions (initial CRR or CSC) and over the last three sessions (end CRR or CSC).
Individual QoL, as measured with the SEIQoL-DW, was rated as satisfactory to good for most participants (SEIQoL-DW range: 48.6–95.4). The domains most often identified as determinants of QoL were: social life (12), family (10), communication (6), mobility (6), health (5), and financial security (5). All but one participant changed one or more domains of their QoL between time points 1 and 2 indicating a response shift, which is known to be adaptive when diagnosed with a chronic progressive disease (Sprangers and Schwartz, 1999).
Before P300-training the SEIQoL-DW score of participant A, B, and G indicated that their individual QoL was below average. Understanding why their subjective QoL was so low is possible by investigation of the domains they reported as determinants for their QoL (see also Quality of Life and Severity of Depression in BCI Users with ALS). Table 4 lists these domains at different time points of measurement. For participants A, B, and D, who participated in both SMR- and P300 training, time 1 reflects the SEIQoL domains before SMR training and time 2 before P300-training. For participant C, F, E, G, H time point 1 reflects the SEIQol domains before P300-training and time point 2 the SEIQoL domains immediately after P300-training (no time point 2 for participant H, because he was not included in the P300 study).
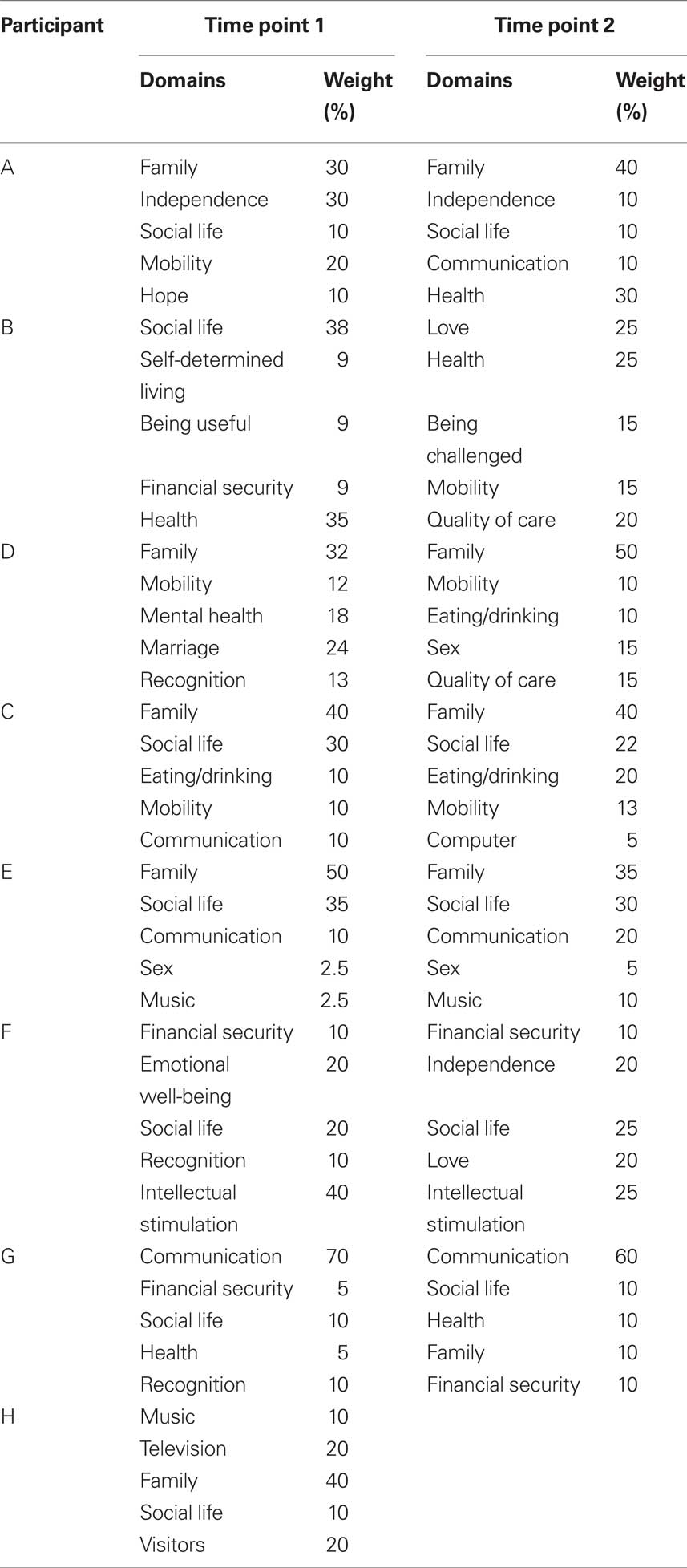
Table 4. Overview of the domains that patients indicated as determinants of their QoL at time point 1 (T1) and time point 2 (T2). It can be seen that some domains listed at T1 were no longer important at T2, and new domains were added at T2. Additionally, the weights of domains, which indicate how important these domains were for the overall QoL of the participants, changed from T1 to T2. Both, the exchange of domains and the change in weight indicate response shifts (Sprangers and Schwartz, 1999) as disease progressed. The QoL of participant H was only assessed once.
Mood, Motivation, and BCI Performance in Individual Patients
Due to the small number of participants we chose to investigate intra-individually the effects of current mood and motivation. In general, participants were highly motivated for both BCI paradigms and mood was often good before training.
To address the relation between time (measured in number of sessions) and mood and motivational factors, non-parametric Spearman’s rank correlations were calculated within each individual patient. Then, the relation between BCI performance (CRR and CSC) and mood and motivational factors, was calculated using partial non-parametric (Spearman’s rank) correlations (control variable: sessions). Table 5 provides an overview of all significant correlations between time (number of sessions), mood and motivational factors and BCI performance. In the following paragraph we will describe how time was related to BCI performance, mood and motivational factors.
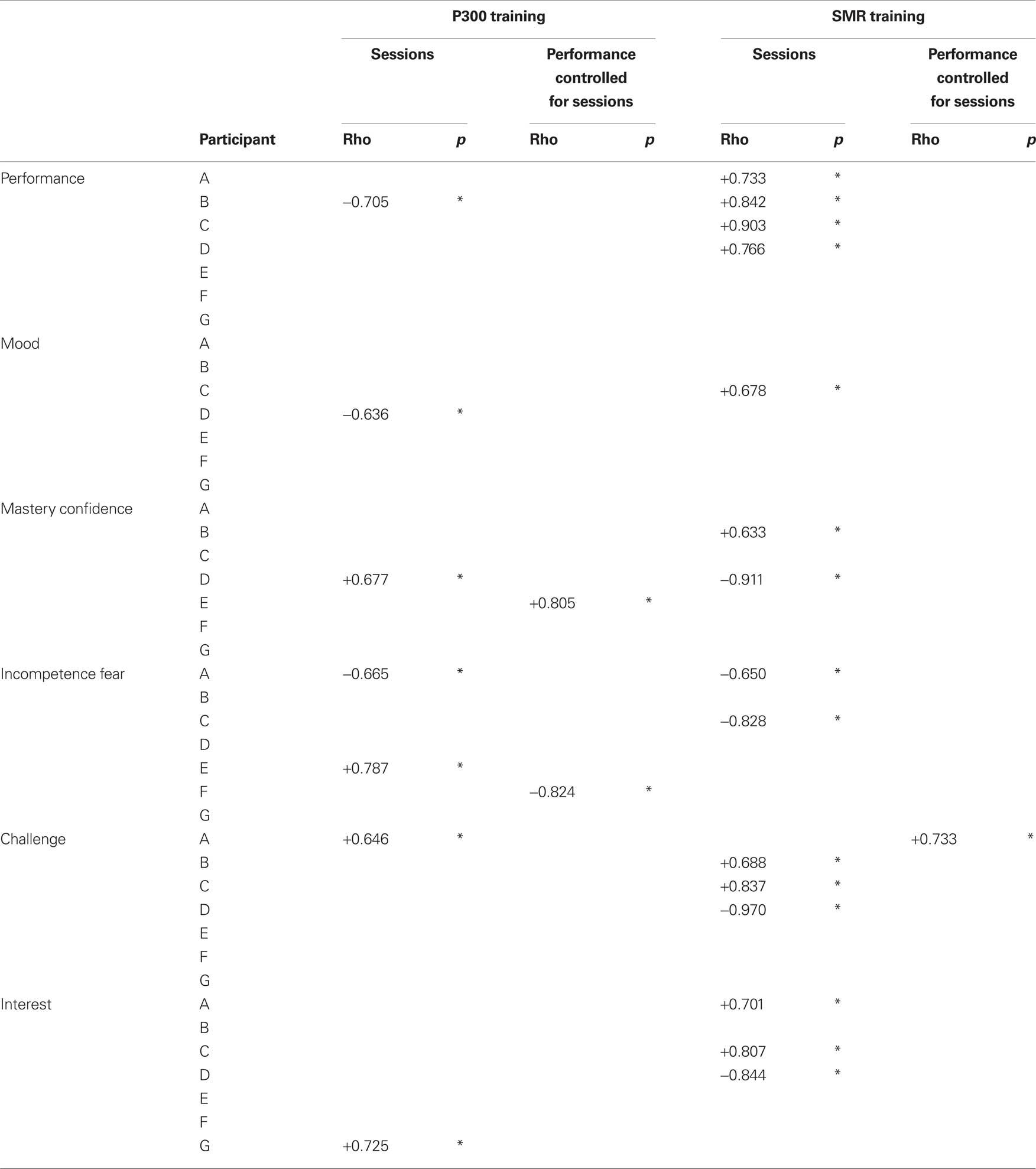
Table 5. Overview of the non-parametric correlations between number of sessions, BCI performance, mood and motivational factors in each participant. Also, partial non-parametric correlation between BCI performance, mood and motivational factors controlled for the variable sessions. Gray cells indicate that there are no data available for this participant. An asterisk (*) indicates the correlation is significant (p < 0.05).
Over the course of the P300 sessions BCI performance decreased in one participant (B), whereas BCI performance increased in all participants (A, B, C, and D) over SMR sessions. Thus, BCI performance was stable for five of six participants in the P300 training, while all four participants in the SMR training improved in performance. In contrast, mood and motivational factors changed over time in all but one (F) participants. During the SMR training mood and motivational factors were more often related to the number of sessions (11 significant correlations) than during P300 training (6 significant correlations) (see Table 5; see The Influence of Mood and Motivation on BCI Performance).
Participant C’s mood increased with number of SMR training sessions as well as the challenge he felt before the training and the interest he had in the training. Also, his incompetence fear decreased with SMR training. Participant B’s mastery confidence increased with SMR training and she felt more challenged by the training with time. Participant A was more interested in SMR training as time passed and also felt less fear that he might be incompetent in training. In contrast, participant D felt less challenged by the training and had less interest in the training. Participant D seemed to be an outlier. He also repeatedly verbally reported that the two-target paradigm in the SMR-BCI was too simple and bored him.
Over the course of the P300 sessions participant D’s mood also decreased while his mastery confidence increased. Participant A again experienced a decrease in incompetence fear over time and he felt more challenged by the task. Participant E’s incompetence fear increased over time. Finally, participant G’s interest in the P300 session increased with time.
Finally, we investigated if and how mood and motivational factors were related to BCI performance. Partial correlations (control variable was number of sessions) showed that in two participants motivational factors were related to P300-BCI performance, whereas in one participant a motivational factor was related to SMR-BCI performance. Specifically, increased mastery confidence, was related to better P300-BCI performance in participant E. The less incompetence fear participant F felt before P300 training, the better was her performance. And finally, the more challenged participant A was by SMR training, the better was his performance. Mood did not relate to BCI performance in any of the participants.
To conclude, mood and motivational factors were more often related to the number of SMR sessions than to P300 sessions. Challenge and mastery confidence were positively related to BCI performance in two participants. Incompetence fear was negatively related to BCI performance in one participant. Mood did not relate to BCI performance.
Discussion
In this study we investigated the influence of psychological well-being measured as current mood, depression and QoL, and motivation on BCI performance of severely paralyzed patients with ALS. In addition, this report provides the first comparison of two different BCI paradigms within the same participants with ALS. To summarize, firstly, the P300-BCI allowed the user higher information transfer rates after only one session compared to the SMR-BCI. Secondly, within the SMR-BCI participants needed to train for 10 sessions until performance started to increase. Thirdly and consequently, a learning effect was only detectable in the SMR-BCI. Fourthly, the participants were highly motivated for BCI training and were often in a good mood. Fifthly, QoL was rated as satisfactory to good and only one participant showed signs of a major depressive disorder. Finally, the relation between motivational factors, the state dependent component of psychological well-being current mood and BCI performance differed between individuals and BCI types and was related to the number of sessions. In particular, motivational factors were related to SMR-BCI performance and P300-BCI performance in three participants. More specifically, increased mastery confidence of participant E, was related to better P300-BCI performance. The less incompetence fear participant F felt before P300 training, the better was her performance. And finally, the more challenged participant A was by SMR training, the better was his performance. Mood did not relate to BCI performance in any of the participants. Thus, motivational factors, but not mood, were related to BCI performance in some participants.
In the following we will discuss the outcome and the relevance of the comparison between the SMR- and P300-BCI paradigms. Then, we discuss the relation between motivational factors and BCI performance and why mood and motivation may fluctuate more during SMR training. Finally, we discuss why assessing QoL, and depression rates in ALS patients is relevant for BCI research.
Comparison of SMR- vs. P300-BCI Performance
The aim to compensate for loss of motor function (e.g., inability to communicate) in severely paralyzed patients by means of a BCI asks for an empirical-based protocol. One question that needs to be addressed in this protocol is which paradigm can be used by most patients. In the here presented study participants could achieve higher information transfer rate after only one session with the P300-BCI, whereas none of the participants could use the SMR-BCI after only one training session. The use of the SMR-BCI required 10 training sessions until performance started to increase, but then all users could accurately hit more than 70% of the targets, which is considered to be the necessary level to control a communication program based on a binary spelling system (Perelmouter and Birbaumer, 2000; Kübler et al., 2001a,b).
These differing results may be explained by the nature of the paradigms. The P300-BCI relies on the exogenous triggering of brain potentials whereas the SMR-BCI as used here constitutes a neurofeedback paradigm that relies on operant conditioning of a new behavior: the ability to self-regulate SMR (Kübler et al., 2001a; Wolpaw et al., 2002). Guger et al. (2003, 2009) tested 99 healthy subjects with an SMR-BCI and 100 healthy subjects with a P300-BCI. In both studies, participants were tested with the BCI only for one session. Although 93.3% of the participants showed a control above 59% accuracy (significantly better than chance), only 6.2% of the subjects were able to learn SMR control with 100% accuracy. In contrast, 72.8% of the subjects could use an P300-BCI after this initial session. These results are compatible with the outcome of our comparison and support the hypothesis that SMR self-regulation is a skill which has to be learned by practicing, whereas P300-BCI use requires no learning. Therefore, for quick and reliable communication a P300-BCI is the BCI of choice provided a P300 is detectable. However, although all four SMR-BCI users and five of six P300-BCI users could achieve high performance, it needs to be noted that performance in persons with ALS is worse than that of healthy controls (Farwell and Donchin, 1988; Nijboer et al., 2008a). Why do patients with ALS perform so much worse? Possible explanations for the worse performance with the P300-BCI are that ALS patients have reduced P3a and P3b amplitudes compared to healthy controls (Hanagasi et al., 2002; Paulus et al., 2002) and some may use antidepressants which may further reduce P300 amplitude (d’Ardhuy et al., 1999); however, this was not the case in our participant sample. In addition, P3a latency might be prolonged (Hanagasi et al., 2002), which might imply that BCI paradigms need a longer inter-stimulus interval for patients (Kotchoubey, 2005). In addition, in late stage ALS patients may have compromised vision so that an P300-BCI with visual stimuli may not be feasible.
Blankertz et al. (2008) showed that with advanced machine-learning techniques 8 of 14 BCI novices could perform with more than 84% accuracy in the very first BCI session, and further four subjects with more than 70%. However, it remains to be demonstrated that these methods are suitable for elderly people with neurodegenerative diseases. In addition, many electrodes are needed to achieve these results and in practice it will be cumbersome if not unrealistic to use these with severely paralyzed patients. Possible other explanations for the worse performance with the SMR-BCI are that patients with ALS may have altered cortical activation during motor imagery (Lulé et al., 2007; Stanton et al., 2007) due to neuronal degeneration in motor cortical areas and that it might be difficult to imagine movements after a long period of being paralyzed.
Both BCIs, the P300 and the SMR neurofeedback paradigm, do require a substantial amount of selective and sustained attention which may be impaired in neurological patients (Hanagasi et al., 2002; Pinkhardt et al., 2008). Other general factors that may influence BCI performance are daytime sleepiness (Buhr-Schinner et al., 1995; Barthlen and Lange, 2000; Mochty et al., 2005) and noisy data due to fasciculation (Mateen et al., 2008). Additionally, BCI trials have to be interrupted more often, because ALS patients cannot suppress coughing, swallowing saliva or yawning, as a consequence of the disease, which leads to larger EMG artifacts than in healthy controls. Furthermore, patients may be in need of medication that affect attention or directly the EEG component (benzodiazepines, opioids). For example, the decline of performance with the P300-BCI in participant B corresponded to the increasing dosage of morphine against phantom limb pain. Similarly benzodiazepines to reduce anxiety affect alertness and the P300 evoked potential (Engelhardt et al., 1992; Hayashi, 2000).
Taken together, we conclude that the P300-BCI is superior to the SMR-BCI for healthy persons and ALS patients alike, provided a P300 is detectable and vision is intact. The advantage of the SMR-BCI, which relies on neurofeedback, is that even if there is no modulation of SMR at the very beginning of training it can be learned by means of operant conditioning – an option which is not existent in the P300-BCI.
The Influence of Mood and Motivation on BCI Performance
Our study showed that patients with ALS are highly motivated for BCI training and tend to be in good mood. Challenge and mastery confidence were positively related to BCI performance in two participants. Incompetence fear was negatively related to BCI performance in one participant.
During SMR-BCI training incompetence fear of two participants decreased as the number of training sessions increased, whereas challenge increased in two participants. Challenge was positively correlated to performance with the SMR-BCI in one participant. We may cautiously argue that as the participants become more familiar with BCI and reach a higher level of performance, they experience more challenge which may be due to increased expectations in their performance. During the first 10 sessions of the SMR training performance of all participants was at chance level. Participants verbally reported feeling frustrated or unsatisfied about the lack of improvement during these initial sessions (e.g., one participant stated that she was “very annoyed that the cursor does not move where” she wanted it to move), but we did not systematically assess frustration or satisfaction with training. However, interest in SMR training remained constantly high across sessions and thus, possible “frustration” did not affect motivation. The results of participant D were in contrast to the results from the other participants. He explicitly stated to feel bored during the SMR training. His mood decreased during P300 training. Compared to the other participants, D was in a good physical condition (e.g., no bulbar symptoms, fully functioning upper limbs) and had a full time position as an engineer. After we finished the SMR and P300 study participant D informed us that he no longer wanted to volunteer in BCI studies, because of his tight time schedule.
Perceiving the task as challenging boosted performance in one of four participants during SMR training. Does this mean we should make BCIs more challenging? Maybe exploring a virtual apartment or playing computer games with a BCI would be more challenging than attempting to hit one of two possible targets as in a SMR-BCI (Leeb et al., 2007; Tangermann et al., 2009). However, participants reported being very motivated to do this simple task (with the exception of participant D). Furthermore, by making a task more engaging (such as with a virtual environment), the complexity of the task can also increase, which may affect the targeted brain signals. The optimal training paradigm may be to incrementally increase the complexity of the task for participants (Neumann and Kübler, 2003; Kübler et al., 2008). However, finding the optimal training protocol calls for empirical investigation.
In general, we conclude that motivational factors may be related to performance in both BCIs in some participants and should be monitored in standard BCI protocols. Moreover, mood and motivational factors are more often related to the number of SMR sessions than to the number of P300 sessions. This may be explained by the different nature of the two BCI tasks: the SMR-BCI required learning to modulate the SMR amplitude by means of neurofeedback. It is argued that learning to self-regulate SMR is like learning a motor skill (Wolpaw et al., 2000; Hinterberger et al., 2005) particularly because these skills share similar properties. Firstly, SMR modulation is highly related to execution, imagination and observation of precise movement (Muthukumaraswamy and Johnson, 2004; Muthukumaraswamy et al., 2004). Secondly, SMR modulation and motor skill learning are influenced similarly by the same factors: feedback (Proteau et al., 1992), practice (Proctor and Dutta, 1995; Ganguly and Carmena, 2009) and, at least for motor skill, sleep (Walker et al., 2002). Mental imagery not only plays an important role in SMR modulation, but also in physical motor skill learning (Warner and McNeill, 1988). In both cases it is more effective if the subject uses kinesthetic imagery (recreating an experience as if the individual was actually moving) rather than visual imagery (in which the individuals see themselves performing as if watching a movie of themselves) (Suinn, 1984; Neuper et al., 2005). Thus, in the SMR-BCI paradigm participants are “juggling” to learn how to use self-regulation of their brain signals to control a cursor. Participants receive real-time feedback of their EEG and related performance, while with the P300-BCI performance feedback is only provided at the end of each trial. In addition, participants might continue practicing motor imagery without being connected to the BCI. Participants may therefore feel a more internal locus of control during the SMR training, which may explain why psychological factors were more often related to the number of SMR sessions than the number of P300 sessions.
In contrast, the use of the P300-BCI requires users only to focus their attention on a desired character (which might be difficult, though, for participants with neurodegenerative disease or brain damage). P300s are triggered by external stimuli and one cannot be trained to produce these responses. Nevertheless, volition and cognitive processes can affect ERPs as early as 50 ms. after stimulus onset (endogenous P300 components) (Birbaumer and Schmidt, 2006). Within the P300-BCI the most important component that distinguishes targets from non-targets is the P300. The amplitude of the P300 is known to be directly related to subjective relevance of the eliciting event (Donchin and Cohen, 1967). Also in several studies, the P300 amplitude was related to monetary incentives (Homberg et al., 1981; Sato et al., 2005). However, in our participant sample the different components of motivation had no obvious effect on the P300 amplitude. One reason might be that the intrinsic motivation of participants was high from the very beginning and they reported feeling very motivated to work with the BCI. It remains to be investigated whether additional incentives (like monetary reward) could increase extrinsic motivation and if this would have an effect on BCI performance. First results from healthy subjects indicate, however, that intrinsic motivation indeed influences the P300 amplitude independent of extrinsic motivation (Kleih et al., 2010).
Quality of Life and Severity of Depression in BCI Users with ALS
Quality of life was rated as satisfactory to good and was not related to physical impairment which replicates results from other studies ( Simmons et al., 2000; Kübler et al., 2005c; Hammer et al., 2008). The SEIQoL-DW results give important insight into response shifts occurring during the course of the disease in ALS patients.
For example, one participant (A) was considered to have clinically relevant depressive symptoms. This may have been related to his low QoL before the P300-BCI study. Before he developed a depressive disorder his QoL was determined by family, independence, social life, mobility, and having hope. He was very satisfied with the quality of all of these domains. He had lost his ability to speak, but communicated perfectly by writing his messages in a notebook. However, before the P300-training the ALSFRS indicated that participant A started having problems holding a pen. His handwriting became sloppy and slow. He also needed more help, for example with getting dressed or turning around in bed. Consequently, before P300-training the most important determinants of his QoL shifted to current issues in his life: health, independence, communication, family, and friends. Apart from family and friends, he was very unsatisfied with the other domains.
Another example is provided by participant B whose low individual QoL before P300-training can be explained with the bad quality of care she was receiving at that time in which her physical state was deteriorating. Participant G indicated a low QoL, because her communication ability was very poor, yet she indicated that communication made up 70% of her overall QoL.
We suggest assessing individual QoL in the BCI target population, because the results might serve as a tool for understanding how the BCI might impact a patient’s life. If a BCI serves its purpose as an assistive technology then it adds value to the patient’s life that is reflected in the QoL score. In addition, further findings that QoL and depression do not impact BCI performance, would suggest that BCIs can be used by persons also when they have a period of depression or low QoL.
Conclusion
To conclude, this study provides the first long-term study on mood and motivation in BCI paradigms with participants with ALS. It showed that motivational factors, such as challenge, incompetence fear and mastery confidence, were related to the performance of some participants. The sample size was small and the conditions between participants varied considerably, thus it is difficult to predict how these results will translate to the future BCI user population. However, these data constitute a first long-term exploration of relations between psychological factors and BCI use. The data are of importance to the BCI community in which most studies test BCIs with young healthy participants. Knowledge of the target population and its needs will be essential for bringing the BCI from the lab to the homes of persons. We recommend to routinely assess psychological well-being and motivation in patients and healthy controls enrolled in BCI trials. We also recommend to use P300-based BCI as first choice in severely paralyzed patients who present with a P300 evoked potential and have intact vision.
Acknowledgments
We are very grateful to all participants with ALS for working with us on this project. Without their effort and enthusiasm we could not have conducted this longitudinal study. We dedicate this paper to participants B and E who passed away. Both participants showed us that life can be “always beautiful, valuable, and exciting” and that it’s all about “rock & roll.” Special thanks to Boris Kotchoubey for his helpful comments and criticism and Jürgen Mellinger for his technical support of the BCI studies. We also thank our colleagues Tamara Matuz, Miguel Jordan, Seung-Soo Lee, Slavica von Hartlieb, Jeroen Lakerveld, Boris Kleber, Tilman Gaber, and Ursula Mochty for help in data collection. We also thank our colleagues from the Wadsworth Center in Albany for their contributions to the set-up of the BCIs and the BCI protocols. This study was supported by the Deutsche Forschungsgemeinschaft (DFG) (SFB 550/TB5), the National Institutes of Health (NIH) (Grants HD30146 and EB00856), the Bernstein Center of BMBF (Tübingen and the European ICT Programme Project FP7-224631). This paper only reflects the authors’ views and funding agencies are not liable for any use that may be made of the information contained herein.
Conflict of Interests Statement
The authors declare that the research was conducted in the absence of any commercial or financial relationships that could be construed as a potential conflict of interest.
References
Averbeck, M., Leiberich, P., Grote-Kusch, M., Olbrich, E., Schröder, A., Brieger, M., and Schumacher, K. (1997). Skalen zur Erfassung der Lebensqualität (SEL) - Manual. Frankfurt: Swets & Zeitlinger B.V., Swets Test Services.
Barthlen, G. M., and Lange, D. J. (2000). Unexpectedly severe sleep and respiratory pathology in patients with amyotrophic lateral sclerosis. Eur. J. Neurol. 7, 299–302.
Beck, A. T., Ward, C. H., Mendelson, M., Mock, J., and Erbaugh, J. (1961). An inventory for measuring depression. Arch. Gen. Psychiatry 4, 561–571.
Birbaumer, N., Ghanayim, N., Hinterberger, T., Iversen, I., Kotchoubey, B., Kübler, A., Perelmouter, J., Taub, E., and Flor, H. (1999). A spelling device for the paralysed. Nature 398, 297–298.
Birbaumer, N., and Schmidt, R. F. (2006). Biologische Psychologie (Heidelberg: Springer Medizin Verlag).
Blankertz, B., Losch, F., Krauledat, M., Dornhege, G., Curio, G., and Müller, K-M. (2008). The Berlin Brain–Computer Interface: accurate performance from first-session in BCI-naive subjects. IEEE Trans. Biomed. Eng. 55, 2452–2462.
Blankertz, B., Sannelli, C., Halder, S., Hammer E. M., Kübler, A., Müller, K-R., Curio, G., and Dickhaus, T. (2010). Neurophysiological predictor of SMR-based BCI performance. Neuroimage 51, 1303–1309.
Buhr-Schinner, H., Laier-Groeneveld, G., and Criee, C. P. (1995). Amyotrophic lateral sclerosis and intermittent self ventilation therapy. Indications and follow-up. Med. Klin. (Munich) 90(Suppl. 1), 49–51.
Calman, K. C. (1984). Quality of life in cancer patients – an hypothesis. J. Med. Ethics 10, 124–127.
Carr, A. J., Gibson, B., and Robinson, P. G. (2001). Measuring quality of life: is quality of life determined by expectations or experience? BMJ 322, 1240–1243.
Cedarbaum, J. M., and Stambler, N. (1997). Performance of the Amyotrophic Lateral Sclerosis Functional Rating Scale (ALSFRS) in multicenter clinical trials. J. Neurol. Sci. 152(Suppl. 1), S1–S9.
d’Ardhuy, X. L., Boeijinga, P. H., Renault, B., Luthringer, R., Rinaudo, G., Soufflet, L., Toussaint, T., and Macher, J. (1999). Effects of serotonin-selective and classical antidepressants on the auditory P300 cognitive potential. Neuropsychobiology 40, 207–213.
Donchin, E., and Cohen, L. (1967). Averaged evoked potentials and intramodality selective attention. Electroencephalogr. Clin. Neurophysiol. 22, 537–546.
Eccles, J. S., and Wigfield, A. (2002). Motivational beliefs, values, and goals. Annu. Rev. Psychol. 53, 109–132.
Ellis, H. C., and Ashbrook, P. W. (1988). “Resource allocation model of the effects of depressed mood states on memory,” in Affect, Cognition, and Social Behaviour, eds K. Fiedler and J. Forgas (Toronto: Hogrefe), 25–43.
Engelhardt, W., Friess, K., Hartung, E., Sold, M., and Dierks, T. (1992). EEG and auditory evoked potential P300 compared with psychometric tests in assessing vigilance after benzodiazepine sedation and antagonism. Br. J. Anaesth. 69, 75–80.
Fabiani, M., Gratton, G., Karis, D., and Donchin, E. (1987). Definition, identification, and reliability of measurement of the P300 component of the event-related brain potential. Adv. Psychophysiol. 2, 1–78.
Farrin, L., Hull, L., Unwin, C., Wykes, T., and David, A. (2003). Effects of depressed mood on objective and subjective measures of attention. J. Neuropsychiatry Clin. Neurosci. 15, 98–104.
Farwell, L. A., and Donchin, E. (1988). Talking off the top of your head: toward a mental prosthesis utilizing event-related brain potentials. Electroencephalogr. Clin. Neurophysiol. 70, 512–523.
Furdea, A., Halder, S., Krusienski, D. J., Bross, D., Nijboer, F., Birbaumer, N., and Kübler, A. (2009). An auditory oddball (P300) spelling system for brain–computer interfaces. Psychophysiology, 46, 617–625.
Ganguly, K., and Carmena, J. M. (2009). Emergence of a stable cortical map for neuroprosthetic control. PLoS Biol. 7, e1000153. doi:10.1371/journal.pbio.1000153.
Gill, T. M., and Feinstein, A. R. (1994). A critical appraisal of the quality of quality-of-life measurements. JAMA 272, 619–626.
Guger, C., Daban, S., Sellers, E., Holzner, C., Krausz, G., Carabalona, R., Gramatica, F., and Edlinger, G. (2009). How many people are able to control a P300-based brain–computer interface (BCI)? Neurosci. Lett. 462, 94–98.
Guger, C., Edlinger, G., Harkam, W., Niedermayer, I., and Pfurtscheller, G. (2003). How many people are able to operate an EEG-based brain–computer interface (BCI)? IEEE Trans. Neural Syst. Rehabil. Eng. 11, 145–147.
Hammer, E. M., Hacker, S., Hautzinger, M., Meyer, T. D., and Kübler, A. (2008). Validity of the ALS-Depression-Inventory (ADI-12) – a new screening instrument for depressive disorders in patients with amyotrophic lateral sclerosis. J. Affect. Disord. 109, 213–219.
Hanagasi, H. A., Gurvit, I. H., Ermutlu, N., Kaptanoglu, G., Karamursel, S., Idrisoglu, H. A., Emre, M., and Demiralp, T. (2002). Cognitive impairment in amyotrophic lateral sclerosis: evidence from neuropsychological investigation and event-related potentials. Brain Res. Cogn. Brain Res. 14, 234–244.
Hayashi, R. (2000). Correlation between coefficient of variation of choice reaction time and components of event-related potentials (P300): effect of benzodiazepine. J. Neurol. Sci. 178, 52–56.
Hickey, A. M., Bury, G., O’Boyle, C. A., Bradley, F., O’Kelly, F. D., and Shannon, W. (1996). A new short form individual quality of life measure (SEIQoL-DW): application in a cohort of individuals with HIV/AIDS. BMJ 313, 29–33.
Hinterberger, T., Veit, R., Wilhelm, B., Weiskopf, N., Vatine, J. J., and Birbaumer, N. (2005). Neuronal mechanisms underlying control of a brain–computer interface. Eur. J. Neurosci. 21, 3169–3181.
Homberg, V., Grunewald, G., and Grunewald-Zuberbier, E. (1981). The variation of p300 amplitude in a money-winning paradigm in children. Psychophysiology 18, 258–262.
Kleih, S. C., Nijboer, F., Halder, S., and Kübler, A (2010). Motivation modulates the P300 amplitude during brain–computer interface use. Clin. Neurophysiol. 121, 1003–1031.
Kotchoubey, B. (2005). Event-related potential measures of consciousness: two equations with three unknowns. Prog. Brain Res. 150, 427–444.
Krusienski, D. J., Sellers, E. W., McFarland, D. J., Vaughan, T. M., and Wolpaw, J. R. (2008). Toward enhanced P300 speller performance J. Neurosci. Methods 167, 15–21.
Kübler, A., and Birbaumer, N. (2008). Brain–computer interfaces and communication in paralysis: extinction of goal directed thinking in completely paralysed patients? Clin. Neurophysiol. 119, 2658–2666.
Kübler, A., Kotchoubey, B., Hinterberger, T., Ghanayim, N., Perelmouter, J., Schauer, M., Fritsch, C., Taub, E., and Birbaumer, N. (1999). The thought translation device: a neurophysiological approach to communication in total motor paralysis. Exp. Brain Res. 124, 223–232.
Kübler, A., Kotchoubey, B., Kaiser, J., Wolpaw, J. R., and Birbaumer, N. (2001a). Brain–computer communication: unlocking the locked in. Psychol. Bull. 127, 358–375.
Kübler, A., Neumann, N., Kaiser, J., Kotchoubey, B., Hinterberger, T., and Birbaumer, N. P. (2001b). Brain–computer communication: self-regulation of slow cortical potentials for verbal communication. Arch. Phys. Med. Rehabil. 82, 1533–1539.
Kübler, A., Mushahwar, V., Hochberg, L. R., and Donoghue, J. P. (2006). BCI meeting 2005 – workshop on clinical issues and applications. IEEE Trans. Neural Syst. Rehabil. Eng. 14, 131–134.
Kübler, A., and Neumann, N. (2005). Brain–computer interfaces – the key for the conscious brain locked into a paralyzed body. Prog. Brain Res. 150, 513–525.
Kübler, A., Neumann, N., Wilhelm, B., Hinterberger, T., and Birbaumer, N. (2004). Predictability of brain–computer communication. J. Psychophysiol. 18, 121–129.
Kübler, A., Nijboer, F., Mellinger, J., Vaughan, T. M., Pawelzik, H., Schalk, G., McFarland, D. J., Birbaumer, N., and Wolpaw, J. R. (2005a). Patients with ALS can use sensorimotor rhythms to operate a brain–computer interface. Neurology 64, 1775–1777.
Kübler, A., Winter, S., Kaiser, J., Birbaumer, N., and Hautzinger, M. (2005b). Das ALS-Depressionsinventar (ADI): Ein Fragebogen zur Messung von Depression bei degenerativen neurologischen Erkrankungen (amyotrophe Lateralsklerose). Z. Klin. Psychol. Psychother. 34, 19–26.
Kübler, A., Winter, S., Ludolph, A. C., Hautzinger, M., and Birbaumer, N. (2005c). Severity of depressive symptoms and quality of life in patients with amyotrophic lateral sclerosis. Neurorehabil. Neural. Repair 19, 182–193.
Kurt, A., Nijboer, F., Matuz, T., and Kübler, A. (2007). Depression and anxiety in individuals with amyotrophic lateral sclerosis: epidemiology and management. CNS Drugs 21, 279–291.
Leeb, R., Lee, F., Keinrath, C., Scherer, R., Bischof, H., and Pfurtscheller, G. (2007). Brain–computer communication: motivation, aim, and impact of exploring a virtual apartment. IEEE Trans. Neural Syst. Rehabil. Eng. 15, 473–482.
Lulé, D., Diekmann, V., Kassubek, J., Kurt, A., Birbaumer, N., Ludolph, A. C., and Kraft, E. (2007). Cortical plasticity in amyotrophic lateral sclerosis: motor imagery and function. Neurorehabil. Neural. Repair 21, 518–526.
Mason, S. G., and Birch, G. E. (2003). A general framework for brain–computer interface design. IEEE Trans. Neural Syst. Rehabil. Eng. 11, 70–85.
Mateen, F. J., Sorenson, E. J., and Daube, J. R. (2008). Strength, physical activity, and fasciculations in patients with ALS. Amyotroph. Lateral Scler. 9, 120–121.
Mochty, U., Hobson, J. A., Birbaumer, N., and Kübler, A. (2005). Sleep and rapid eye movement activity in amyotrophic lateral sclerosis (ALS). Psychophysiology 42(Suppl. 1), 90.
Muthukumaraswamy, S. D., and Johnson, B. W. (2004). Changes in rolandic mu rhythm during observation of a precision grip. Psychophysiology 41, 152–156.
Muthukumaraswamy, S. D., Johnson, B. W., and McNair, N. A. (2004). Mu rhythm modulation during observation of an object-directed grasp. Brain Res. Cogn. Brain Res. 19, 195–201.
Neudert, C., Wasner, M., and Borasio, G. D. (2001). Patients’ assessment of quality of life instruments: a randomised study of SIP, SF-36 and SEIQoL-DW in patients with amyotrophic lateral sclerosis. J. Neurol. Sci. 191, 103–109.
Neudert, C., Wasner, M., and Borasio, G. D. (2004). Individual quality of life is not correlated with health-related quality of life or physical function in patients with amyotrophic lateral sclerosis. J. Palliat. Med. 7, 551–557.
Neumann, N. (2001). Gehirn-Computer-Kommunikation: Einflussfaktoren der Selbstregulation langsamer kortikaler Hirnpotentiale. Unpublished Dissertation, Eberhard-Karlsuniversität Tübingen, Tübingen.
Neumann, N., and Birbaumer, N. (2003). Predictors of successful self control during brain–computer communication. J. Neurol. Neurosurg. Psychiatr. 74, 1117–1121.
Neumann, N., and Kübler, A. (2003). Training locked-in patients: a challenge for the use of brain–computer interfaces. IEEE Trans. Neural Syst. Rehabil. Eng. 11, 169–172.
Neuper, C., Scherer, R., Reiner, M., and Pfurtscheller, G. (2005). Imagery of motor actions: differential effects of kinesthetic and visual-motor mode of imagery in single-trial EEG. Brain Res. Cogn. Brain Res. 25, 668–677.
Niedermeyer, E. (2004). “The normal EEG of the waking adult,” in Electroencephalography: Basic Principles, Clinical Applications and Related Fields, 5th Edn., eds E. Niedermeyer and F. H. Lopes da Silva (Baltimore: Lippincott Williams and Wilkins), 149–173.
Nijboer, F., Furdea, A., Gunst, I., Mellinger, J., McFarland, D. J., Birbaumer, N., and Kübler, A. (2008a). An auditory brain–computer interface (BCI). J. Neurosci. Methods 167, 43–50.
Nijboer, F., Sellers, E. W., Mellinger, J., Jordan, M. A., Matuz, T., Furdea, A., Halder, S., Mochty, U., Krusienski, D. J., Vaughan, T.M., Wolpaw, J. R., Birbaumer, N., and Kübler, A. (2008b). A P300-based brain–computer interface for people with amyotrophic lateral sclerosis. Clin. Neurophysiol. 119, 1909–1916.
O’Boyle, C. A., and Waldron, D. (1997). Quality of life issues in palliative medicine. J. Neurol. 244(Suppl. 4), S18–S25.
Paulus, K. S., Magnano, I., Piras, M. R., Solinas, M. A., Solinas, G., Sau, G. F., and Aiello, I. (2002). Visual and auditory event-related potentials in sporadic amyotrophic lateral sclerosis. Clin. Neurophysiol. 113, 853–861.
Perelmouter, J., and Birbaumer, N. (2000). A binary spelling interface with random errors. IEEE Trans. Neural Syst. Rehabil. Eng. 8, 227–232.
Pfurtscheller, G., and Aranibar, A. (1979). Evaluation of event-related desynchronization (ERD) preceding and following self-paced movements. Electroencephalogr. Clin. Neurophysiol. 46, 138–146.
Pfurtscheller, G., Guger, C., Müller, G., Krausz, G., and Neuper, C. (2000). Brain oscillations control hand orthosis in a tetraplegic. Neurosci. Lett. 292, 211–214.
Pfurtscheller, G., and Neuper, C. (2010). “EEG-based brain computer communication,” in The Neurophysiological Foundations of Mental and Motor Imagery, eds A. Guillot and C. Collet (Oxford: Oxford University Press), 203–212.
Pfurtscheller, G., Neuper, C., Ramoser, H., and Muller-Gerking, J. (1999). Visually guided motor imagery activates sensorimotor areas in humans. Neurosci. Lett. 269, 153–156.
Pinkhardt, E. H., Jürgens, R., Becker, W., Mölle, M., Born, J., Ludolph, A. C., and Schreiber, H. (2008). Signs of impaired selective attention in patients with amyotrophic lateral sclerosis. J. Neurol. 255, 532–538.
Porter, R. J., Gallagher, P., Thompson, J. M., and Young, A. H. (2003). Neurocognitive impairment in drug-free patients with major depressive disorder. Br. J. Psychiatry 182, 214–220.
Proctor, R., and Dutta, A. (1995). Skill Acquisition and Human Performance. Thousand Oaks, CA: Sage publications, Inc.
Proteau, L., Marteniuk, R. G., and Levesque, L. (1992). A sensorimotor basis for motor learning: evidence indicating specificity of practice. Q. J. Exp. Psychol. A. 44, 557–575.
Rheinberg, F., Vollmeyer, R., and Burns, B. (2001). FAM: Eine Fragebogen zur Erfassung Aktueller Motivation in Lern- und Leistungssituationen. Diagnostica 47, 57–66.
Robbins, R. A., Simmons, Z., Bremer, B. A., Walsh, S. M., and Fischer, S. (2001). Quality of life in ALS is maintained as physical function declines. Neurology 56, 442–444.
Sato, A., Yasuda, A., Ohira, H., Miyawaki, K., Nishikawa, M., Kumano, H., and Kuboki, T. (2005). Effects of value and reward magnitude on feedback negativity and P300. Neuroreport 16, 407–411.
Schiefele, U., and Rheinberg, F. (1997). “Motivation and knowledge acquisition: searching for mediating processes,” in Advances in Motivation and Achievement, eds P. Pintrich and L. Maehr (Greenwich, CT: JAI Press), 251–301.
Seibert, P. S., and Ellis, H. C. (1991). Irrelevant thoughts, emotional mood states, and cognitive task performance. Mem. Cogn. 19, 507–513
Sellers, E. W., and Donchin, E. (2006). A P300-based brain–computer interface: initial tests by ALS patients. Clin. Neurophysiol. 117, 538–548.
Simmons, Z., Bremer, B. A., Robbins, R. A., Walsh, S. M., and Fischer, S. (2000). Quality of life in ALS depends on factors other than strength and physical function. Neurology 55, 388–392.
Sprangers, M. A., and Schwartz, C. E. (1999). Integrating response shift into health-related quality of life research: a theoretical model. Soc. Sci. Med. 48, 1507–1515.
Squires, K. C., Donchin, E., Herning, R. I., and McCarthy, G. (1977). On the influence of task relevance and stimulus probability on event-related-potential components. Electroencephalogr. Clin. Neurophysiol. 42, 1–14.
Stanton, B. R., Williams, V. C., Leigh, P. N., Williams, S. C., Blain, C. R., Jarosz, J. M., and Simmons, A. (2007). Altered cortical activation during a motor task in ALS. Evidence for involvement of central pathways. J. Neurol. 254, 1260–1267.
Suinn, R. (1984). “Imagery and sport,” in Cognitive Sport Psychology, eds W. Straub and J. Williams (Lansing, NY: Sport Science Associates), 253–271.
Tangermann, M. W., Krauledat, M., Grzeska, K., Sagebaum, M., Blankertz, B., Vidaurre, C., Blankertz, B., and Müller, K.-R. (2009). “Playing pinball with non-invasive BCI,” in Advances in Neural Information Processing Systems, Vol. 21 (Cambridge, MA: MIT Press), 1641–1648.
Vollmeyer, R., and Rheinberg, F. (2000). Does motivation affect performance via persistence. Learn. Instr. 10, 293–309.
Walker, M. P., Brakefield, T., Morgan, A., Hobson, J. A., and Stickgold, R. (2002). Practice with sleep makes perfect: sleep-dependent motor skill learning. Neuron 35, 205–211.
Warner, L., and McNeill, M. E. (1988). Mental imagery and its potential for physical therapy. Phys. Ther. 68, 516–521.
Wolpaw, J. R., Birbaumer, N., Heetderks, W. J., McFarland, D. J., Peckham, P. H., Schalk, G., Donchin, E., Quatrano, L. A., Robinson, R. J., and Vaughan, T. M. (2000). Brain–computer interface technology: a review of the first international meeting. IEEE Trans. Rehabil. Eng. 8, 164–173.
Keywords: amyotrophic lateral sclerosis, brain–computer interface, motivation, mood, sensorimotor rhythms, P300 event-related potential
Citation: Nijboer F, Birbaumer N and Kübler A (2010) The influence of psychological state and motivation on brain–computer interface performance in patients with amyotrophic lateral sclerosis – a longitudinal study. Front. Neurosci. 4:55. doi: 10.3389/fnins.2010.00055
Received: 17 March 2010;
Paper pending published: 14 April 2010;
Accepted: 03 July 2010;
Published online: 21 July 2010
Edited by:
Gert Pfurtscheller, Graz University of Technology, AustriaReviewed by:
Dennis J. McFarland, Wadsworth Center for Laboratories and Research, USAFabio Babiloni, Sapienza University of Rome, Italy
Copyright: © 2010 Nijboer, Birbaumer and Kübler. This is an open-access article subject to an exclusive license agreement between the authors and the Frontiers Research Foundation, which permits unrestricted use, distribution, and reproduction in any medium, provided the original authors and source are credited.
*Correspondence: Femke Nijboer, Human Media Interaction, Faculty of Electrical Engineering, Mathematics and Computer Science, University of Twente, PO Box 217, 7500 AE Enschede, Netherlands. e-mail:Zi5uaWpib2VyQGV3aS51dHdlbnRlLm5s