- 1State Key Laboratory of Genetic Engineering and Collaborative Innovation Center for Genetics and Development, School of Life Sciences, Fudan University, Shanghai, China
- 2Fudan University Taizhou Institute of Health Sciences, Taizhou, China
- 3Key Laboratory of Public Health Safety of Ministry of Education, Department of Epidemiology, School of Public Health, Fudan University, Shanghai, China
- 4Institute of Neurology, Huashan Hospital, Fudan University, Shanghai, China
- 5State Key Laboratory of Genetic Engineering, Metabonomics and Systems Biology Laboratory, School of Life Sciences, Fudan University, Shanghai, China
- 6International Peace Maternity and Child Health Hospital, School of Medicine, Shanghai Jiao Tong University, Shanghai, China
- 7Human Phenome Institute, Fudan University, Shanghai, China
- 8Department of Medical Epidemiology and Biostatistics, Karolinska Institutet, Stockholm, Sweden
Dementia has become a major global public health challenge with a heavy economic burden. It is urgently necessary to understand dementia pathogenesis and to identify biomarkers predicting risk of dementia in the preclinical stage for prevention, monitoring, and treatment. Metabolomics provides a novel approach for the identification of biomarkers of dementia. This systematic review aimed to examine and summarize recent retrospective cohort human studies assessing circulating metabolite markers, detected using high-throughput metabolomics, in the context of disease progression to dementia, including incident mild cognitive impairment, all-cause dementia, and cognitive decline. We systematically searched the PubMed, Embase, and Cochrane databases for retrospective cohort human studies assessing associations between blood (plasma or serum) metabolomics profile and cognitive decline and risk of dementia from inception through October 15, 2018. We identified 16 studies reporting circulating metabolites and risk of dementia, and six regarding cognitive performance change. Concentrations of several blood metabolites, including lipids (higher phosphatidylcholines, sphingomyelins, and lysophophatidylcholine, and lower docosahexaenoic acid and high-density lipoprotein subfractions), amino acids (lower branched-chain amino acids, creatinine, and taurine, and higher glutamate, glutamine, and anthranilic acid), and steroids were associated with cognitive decline and the incidence or progression of dementia. Circulating metabolites appear to be associated with the risk of dementia. Metabolomics could be a promising tool in dementia biomarker discovery. However, standardization and consensus guidelines for study design and analytical techniques require future development.
Introduction
Dementia has become a major global public health challenge, and brings a heavy economic burden to the patients, their families, and society. More than 46 million people worldwide suffered from dementia in 2015; this figure is predicted to increase to 131.5 million by 2050 (Prince et al., 2015). The annual global cost for dementia care was estimated to be one trillion dollars by 2018, which is more than 1.1% of the global gross domestic product (Wimo et al., 2017). There is currently no effective disease-modifying treatment for dementia. Because of the long preclinical phase, in which there are early pathogenic changes but no cognitive impairment, prevention of dementia through management of modifiable risk factors is likely a better therapeutic strategy than developing a cure (Livingston et al., 2017). Therefore, there is an urgent need to understand the pathophysiological process of dementia and to identify biomarkers predicting risk of dementia in the preclinical stage, which could facilitate disease prevention, monitoring, and treatment (Livingston et al., 2017).
The definition of accurate preclinical biomarkers is essential to the early recognition of dementia. Clinical methods currently in use for the detection of dementia include evaluation of structural imaging (CT or MRI) for brain atrophy, functional MRI (fMRI) for brain connectivity, positron emission tomography (PET) for amyloid deposits, proton magnetic resonance spectroscopy (1H-MRS) for brain metabolites, and levels of cerebrospinal fluid (CSF) β-amyloid (Aβ) and tau (Ahmed et al., 2014). However, these means are limited because they are costly, partly invasive, time-consuming, and are not suitable for community-based population screening for early detection. Extensive deposition of Aβ was observed when MRI changes occurred, and targeting of Aβ failed to improve cognition in patients with Alzheimer's disease (AD) in phase III trials of anti-amyloid antibody treatments (Mullard, 2016), which highlighted the importance of early identification of dementia and discovery of additional biomarkers. Determination of reliable blood- or urine-based biomarkers could be an attractive development (Thambisetty and Lovestone, 2010; Ahmed et al., 2014; Fiandaca et al., 2014; Henriksen et al., 2014).
Metabolomics, an “omics” science, is based on high-throughput identification and quantification of small molecule metabolites in cells, tissues, and biofluids, and is a powerful tool for mapping global biochemical changes and discovery of new biomarkers in disease (German et al., 2005). Recently, metabolomics in animal models and human participants (in postmortem brain tissue, CSF, or blood) have attracted interest for the understanding of pathophysiology and identification of biomarkers of dementia (Kaddurah-Daouk et al., 2008; Xu et al., 2012; Fiandaca et al., 2014). Many metabolic changes in the brain or CSF could be reflected in blood profiles; metabolites in blood (serum or plasma) are therefore promising candidates for biomarkers of dementia (Fiandaca et al., 2014; Varma et al., 2018). Using proton (1H) nuclear magnetic resonance (NMR) or mass spectrometry (MS) metabolomics platforms, blood concentrations of many small molecule metabolites and lipids were found to be altered in patients with dementia relative to healthy controls (Xu et al., 2012; Wang et al., 2014; Proitsi et al., 2015; Li et al., 2016). However, these studies primarily aimed at differentiating patients with cognitive impairment from controls. This kind of cross-sectional design ignores the long preclinical phase of dementia that may be presented as a reverse causation, since the progression of dementia and many chronic complications associated with dementia could alter metabolite concentrations in peripheral blood (Thambisetty and Lovestone, 2010). Longitudinal studies allow direct assessment of pathobiology during transition periods, and identification of biomarkers for prediction of dementia. Several studies have fueled speculation of the discovery of circulating metabolites that influence cognition and the future development of dementia (Mapstone et al., 2014; Casanova et al., 2016; Bressler et al., 2017; Chouraki et al., 2017; Li et al., 2017; van der Lee et al., 2018; Varma et al., 2018). Identifying serum metabolic biomarkers of dementia in the preclinical stage would be a significant step forward, as such non-invasive blood-based biomarkers would reflect the disease-related pathophysiological process, and could be easily performed to identify individuals at risk, thus prevention and treatments could be initiated (Mielke et al., 2010; Oresic et al., 2011). However, no systematic review or meta-analysis of the use of metabolomics in prospective studies on dementia progression has been published.
Given the heterogeneity in study participants, samples (serum or plasma), and metabolite profiling analytical platforms, the aim of this systematic review is to examine and summarize recent human cohort studies assessing circulating metabolite markers, detected using high-throughput metabolomics, in the context of disease progression to dementia, including incident mild cognitive impairment (MCI), all-cause dementia, and cognitive decline (i.e., decline in neuropsychological test scores).
Methods
Data Sources and Search Strategy
The systematic search followed the Cochrane Handbook of Systematic Reviews (https://training.cochrane.org/handbook) and used the MOOSE (Meta-analysis Of Observational Studies in Epidemiology) checklist (Stroup et al., 2000). The review protocol has been registered in the PROSPERO International prospective register of systematic reviews (https://www.crd.york.ac.uk/PROSPERO/display_record.php?RecordID=111004). A comprehensive search of published literature in English was conducted in three databases: PubMed, Embase, and the Cochrane Library, from inception through October 15, 2018. The complete search strategy is listed in Supplementary Table 1. We also manually searched references of relevant articles and reviews during screening.
Study Selection
All titles and abstracts were screened by two independent reviewers (Y.F.J. and Z.Z.); disagreements between them were resolved by full-text review and consensus. Studies were considered eligible for inclusion based on the following criteria: (1) cohort studies or other original studies in humans (e.g., cohort studies, case-cohort studies, nested case-control studies, or clinical trials); (2) metabolites in blood (plasma or serum) detected by high-throughput MS or NMR analytical platforms; (3) report of incident cognitive impairment (MCI or all-cause dementia) or cognitive decline (i.e., decline in neuropsychological test scores). Metabolomics studies in CSF, saliva, and urine samples and those using MRS for the quantification of brain metabolites were excluded. We also excluded cross-sectional studies, studies in unrelated patients, non-original studies (e.g., case reports, editorials, letter to editors, commentaries, expert opinions, and any type of reviews), experimental studies in animals, and duplicate publication of the same data.
Data Extraction
Study characteristics (Table 1), including first author, year of publication and journal, study population and location, participant characteristics, sample size, duration of follow-up, biospecimens (plasma or serum), analytical technique and metabolite targets, outcome and ascertainment, number of observed events, statistical method, covariates included in the fully adjusted model, and a summary of key findings (analyzed metabolites and effect estimates), were extracted from each included study independently by two investigators (Y.F.J. and Z.Z.), and cross-checked by a third investigator (J.S.).
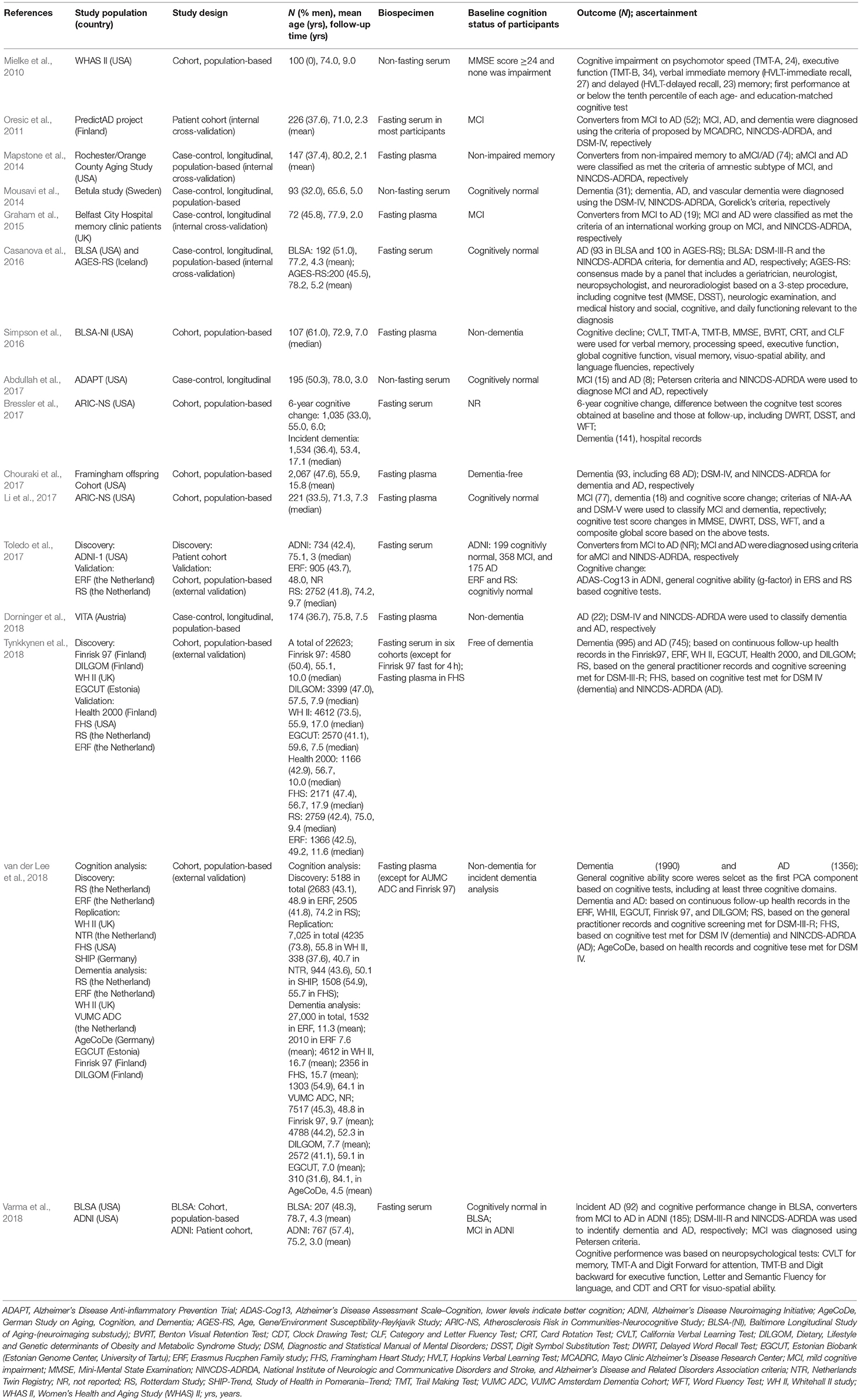
Table 1. Characteristics of studies investigating longitudinal associations between metabolites and dementia risk.
Quality Assessment
The quality of the studies were assessed and scored independently by two researchers (Z.Z. and J.S.) by using a modified Newcastle-Ottawa Scale (Wells et al., 2014). Cohort studies were scored on the following criteria: (1) Selection, including representativeness of source population (exposed and non-exposed cohort), ascertainment of exposure and definition of controls (1 point per criterion); (2) Comparability−2 points if the analysis was adjusted for most potential confounders, and 1 point if the analysis was only adjusted for age and sex (or age and sex matched); (3) Outcome, including assessment of outcome and duration of follow-up (1 point per criterion). Since all studies involved participants with baseline metabolic profiling and cognitive follow-up status, we removed attrition from the criteria. Case-control studies were rated on the following criteria: (1) Selection, including adequate definition of cases, representativeness of cases, and selection and definition of controls (1 point per criterion); (2) Comparability, same as cohort studies; (3) Exposure, including assessment of exposure, and same method of ascertainment for cases and controls (1 point per criterion). Points were summed and ranged from 0 to 8; studies with summed scores ≤4 were considered of low quality, while summed scores 5–8 were considered of high quality. Details of these criteria and score of each study are presented in Supplementary Tables 2, 3. Disagreements between reviewers in quality assessment were resolved through consensus.
Results
Literature Retrieval
In the initial search, 1,693 unique studies were identified after removal of duplicates (Figure 1). Following the review of titles and abstracts, 263 articles were selected for careful full-text screening. Fourteen were eligible. Most of the 249 studies excluded were excluded because of unrelated scope, cross-sectional design, or they were conducted in patients or in animals, or were non-original studies. Six studies were conducted using other types of biological samples (i.e., CSF and brain in vivo). We also excluded a sub-analysis study (Fiandaca et al., 2015) using the same participants as a previous publication (Mapstone et al., 2014). In total, 16 full-text articles were eligible, with an additional two included after a hand search, for inclusion in this qualitative systematic review (Mielke et al., 2010; Oresic et al., 2011; Mapstone et al., 2014; Mousavi et al., 2014; Graham et al., 2015; Casanova et al., 2016; Simpson et al., 2016; Abdullah et al., 2017; Bressler et al., 2017; Chouraki et al., 2017; Li et al., 2017; Toledo et al., 2017; Dorninger et al., 2018; Tynkkynen et al., 2018; van der Lee et al., 2018; Varma et al., 2018).
Study Characteristics
The characteristics of the 16 included studies are summarized in Table 1. All included studies were conducted in European or the US populations, and more than half (9/16) were published after 2016. The total number of participants was 61,345, ranging from 72 to 27,000, with mean age at baseline ranging from 48.0 to 84.1 years, and studies generally including more women than men (overall: 52.3% women).
Of the 16 longitudinal analyses, 10 studies used a retrospective cohort design (Mielke et al., 2010; Oresic et al., 2011; Simpson et al., 2016; Bressler et al., 2017; Chouraki et al., 2017; Li et al., 2017; Toledo et al., 2017; Tynkkynen et al., 2018; van der Lee et al., 2018; Varma et al., 2018), four were case-control designs embedded in population-based cohort studies (Mapstone et al., 2014; Mousavi et al., 2014; Casanova et al., 2016; Dorninger et al., 2018), and the other two analyses were based on an outpatient cohort (Graham et al., 2015) and a placebo-controlled primary prevention trial (Abdullah et al., 2017), respectively. Validations in external independent cohorts were conducted in only three of the studies (Table 1). Four studies included an internal replication analysis in randomly selected samples in the same population (Oresic et al., 2011; Mapstone et al., 2014; Graham et al., 2015; Casanova et al., 2016). Two articles aimed at replicating previously reported findings by Mapstone et al. (2014) using the same metabolite panels (Casanova et al., 2016; Li et al., 2017). Only three studies calculated and reported the sample size and expected statistical power (Mapstone et al., 2014; Casanova et al., 2016; Bressler et al., 2017), six articles acknowledged their small sample size as a limitation (Mielke et al., 2010; Oresic et al., 2011; Simpson et al., 2016; Abdullah et al., 2017; Toledo et al., 2017; Varma et al., 2018), and three analyses had a relatively large sample size or listed it as their strength (2,067, 22,623, and 27,000 respectively; Chouraki et al., 2017; Tynkkynen et al., 2018; van der Lee et al., 2018). The other four studies did not explicitly mention sample size (Mousavi et al., 2014; Graham et al., 2015; Li et al., 2017; Dorninger et al., 2018).
Quality Assessment of Studies
The results of quality assessment of the included studies using a modified version of the Newcastle-Ottawa Scale are shown in Supplementary Tables 2, 3. Most studies (14/16) were considered high quality, with a total score ≥6. Low-quality studies with a total score of 4, mainly due to the representativeness of cases and controls, comparability, or not long enough duration of follow-up (<5 years), were present among the cohort and case-control studies.
Biological Samples and Analytical Platform
Seven studies analyzed fasting plasma (except one non-fasting) and nine serum samples [five fasting and four non-fasting, while one study also used fasting plasma in the validation cohort (Tynkkynen et al., 2018)]. Variations of MS platforms were used in 13 studies, and three used both MS and NMR platforms (Table 2). More than half the studies used untargeted metabolomics analysis (n = 8). Targeted approaches were adopted in eight studies, and the BIOCRATES AbsoluteIDQ p180 platform was used in five of these analyses (Mapstone et al., 2014; Casanova et al., 2016; Li et al., 2017; Toledo et al., 2017; Varma et al., 2018).
Outcome and Ascertainment
Six studies evaluated the relationship between baseline metabolites and cognitive performance changes, relying on a number of neuropsychological tests (Mielke et al., 2010; Simpson et al., 2016; Bressler et al., 2017; Li et al., 2017; Toledo et al., 2017; Varma et al., 2018). Conversion from MCI to AD/dementia was assessed in four of these studies (Oresic et al., 2011; Graham et al., 2015; Toledo et al., 2017; Varma et al., 2018), and 14 studies determined risk of MCI and/or all-cause dementia in cognitively normal populations, mainly nested in prospective population-based cohorts (Mapstone et al., 2014; Mousavi et al., 2014; Casanova et al., 2016; Abdullah et al., 2017; Bressler et al., 2017; Chouraki et al., 2017; Li et al., 2017; Dorninger et al., 2018; Tynkkynen et al., 2018; van der Lee et al., 2018; Varma et al., 2018). The neuropsychological test battery (Table 1) used in these studies included Mini-Mental State Examination (MMSE), California Verbal Learning Test (CVLT), Delayed Word Recall Test (DWRT), and Trail Making Test A/B (TMT-A/B). Various criteria were used to classify MCI based on cognitive tests, such as the one proposed by Petersen (Petersen et al., 1999). Assessment of dementia varied across studies from examination based on the Diagnostic and Statistical Manual of Mental Disorders (DSM, 4 III-R, 5 IV, and 1 V; Association, 1987, 1994, 2013) criteria only, examination with complementary medical records (Tynkkynen et al., 2018; van der Lee et al., 2018), or health records only (Bressler et al., 2017). All the classifications of AD were based on the National Institute of Neurological and Communication Disorders and Stroke-Alzheimer's Disease and Related Disorders Association (NINCDS-ADRDA) criteria (McKhann et al., 1984).
Participants in 13 studies were free of dementia at baseline (Mielke et al., 2010; Mapstone et al., 2014; Mousavi et al., 2014; Casanova et al., 2016; Simpson et al., 2016; Abdullah et al., 2017; Bressler et al., 2017; Chouraki et al., 2017; Li et al., 2017; Dorninger et al., 2018; Tynkkynen et al., 2018; van der Lee et al., 2018; Varma et al., 2018), while one of them contained a validation analysis in converters from MCI to AD (Varma et al., 2018). Individuals were diagnosed with MCI at baseline in three studies, which aimed to assess the baseline metabolites associated with progression to AD (Oresic et al., 2011; Graham et al., 2015; Toledo et al., 2017). The mean or median follow-up time after sample collection ranged from 2.0 to 17.9 years. The number of converters from MCI to AD, MCI, AD, and all-cause dementia in these studies ranged from 19 to 185, 15 to 77, 8 to 1356, and 18 to 1990, respectively (Table 1).
Statistical Approaches
Cox proportional hazards regression models were applied in eight analyses to estimate the association between metabolites and the risk of incident all-cause dementia (Mielke et al., 2010; Abdullah et al., 2017; Bressler et al., 2017; Chouraki et al., 2017; Tynkkynen et al., 2018; van der Lee et al., 2018) or progression from MCI to AD (Toledo et al., 2017; Varma et al., 2018). This relationship was evaluated using logistic regression models in another four studies (Oresic et al., 2011; Mapstone et al., 2014; Casanova et al., 2016; Li et al., 2017). Linear models were typically used to assess the association of baseline circulating metabolites with changes in cognitive performance (Casanova et al., 2016; Bressler et al., 2017; Li et al., 2017; Toledo et al., 2017). Casanova et al. (2016) and Varma et al. (2018) additionally used several machine-learning methods, such as support vector machine (SVM) and random forest (RF), to evaluate the use of metabolites in discriminating between patients with cognitive impairment and healthy controls. Age and sex were adjusted or matched as covariates in all analyses except in Dorninger et al. (2018); some studies further adjusted for education, apolipoprotein E (APOE ε4), vascular risk factors (e.g., smoking, alcohol drinking, body mass index [BMI], hypertension or blood pressure, diabetes mellitus, and triglycerides), cardiovascular diseases, and lipid-lowering medication (Table 2).
Metabolites Associated With Dementia Risk
Table 2 shows the results of analyses associating metabolites with dementia risk. Metabolites related to future cognitive decline were estimated individually in two studies (Mielke et al., 2010; Simpson et al., 2016) and nested in four other included studies (Bressler et al., 2017; Li et al., 2017; Toledo et al., 2017; Varma et al., 2018). Faster global cognitive decline risk was observed in participants with lower concentrations of acylcarnitine (Li et al., 2017), alpha-aminoadipic acid (α-AAA) (Li et al., 2017; Toledo et al., 2017), valine (Toledo et al., 2017), or higher concentrations of sphingomyelins (SM) and phosphatidylcholines (PC) (Toledo et al., 2017) at baseline. In terms of different cognitive domains, higher levels of SM, PC, and lysophophatidylcholine (lysoPC) were associated with deterioration in attention, language, and verbal memory (Mielke et al., 2010; Varma et al., 2018). Elevated ceramides were related to memory loss (Mielke et al., 2010), and amino acids such as arginine and histidine were associated with impairment in memory (Bressler et al., 2017), language, and visuospatial skills (Varma et al., 2018). However, baseline and changes in plasma PC concentrations were not associated with 7-year cognitive performance change in the study of Simpson et al. (2016).
The majority of the studies aimed at investigating lipids, biogenic amines, acylcarnitines (AC), and amino acids in the context of risk of incident MCI or dementia (Mielke et al., 2010; Oresic et al., 2011; Mapstone et al., 2014; Mousavi et al., 2014; Graham et al., 2015; Casanova et al., 2016; Abdullah et al., 2017; Bressler et al., 2017; Chouraki et al., 2017; Li et al., 2017; Toledo et al., 2017; Dorninger et al., 2018; Tynkkynen et al., 2018; van der Lee et al., 2018; Varma et al., 2018). Of the 16 studies, 10 assessed the association between lipid metabolites and dementia. SM, PC, and lysoPC were the most commonly identified potential biomarkers, and higher baseline levels of these lipids are likely an important indicator of a higher risk of MCI, and conversion from MCI to AD/dementia (Oresic et al., 2011; Li et al., 2017; Toledo et al., 2017; Varma et al., 2018). However, Mapstone et al. reported that PC was significantly lower in participants who later showed a conversion to MCI/AD compared to cognitively normal participants (Mapstone et al., 2014). Further, docosahexaenoic acid (DHA), a well-known long-chain omega-3 polyunsaturated fatty acid involved in brain development in early life, was associated with good cognitive performance and lower risk of dementia (Abdullah et al., 2017; van der Lee et al., 2018). Moreover, van der Lee et al. (2018) found that several high-density lipoprotein (HDL) subfractions were associated with higher cognitive ability and lower risk of dementia and AD. The triglycerides-to-total-lipids ratio and other similar ratios were associated with incident dementia in the study by Tynkkynen et al. (2018). However, we did not find any metabolites that were consistently identified in all studies. Only SM C16:0 and SM (OH) C14:1 were associated with conversion from MCI to AD in an Alzheimer's disease Neuroimaging Initiative (ADNI) study (Toledo et al., 2017) and incident AD in the Baltimore Longitudinal Study of Aging (BLSA) (Varma et al., 2018).
Some essential and non-essential amino acids were significantly associated with dementia. The study by Tynkkynen et al. (2018) demonstrated that increases in branched-chain amino acids (BCAAs, i.e., isoleucine, leucine, and valine), were associated with lower dementia risk, and similar results were found by Toledo et al. (2017), concerning valine. Glutamine and glutamate were associated with higher dementia risk in two studies (Chouraki et al., 2017; van der Lee et al., 2018). Creatinine (Tynkkynen et al., 2018) and taurine (Chouraki et al., 2017) were each associated with between 10 and 26% lower risk of dementia, whereas higher plasma anthranilic acid levels were associated with greater risk of dementia (Chouraki et al., 2017). In addition, baseline blood concentrations of gamma-aminobutyric acid (GABA), ornithine, and phenylalanine were lower (Mapstone et al., 2014; Graham et al., 2015), and dihydroxybutanoic acid levels were higher (Oresic et al., 2011; Mousavi et al., 2014) in dementia converters relative to healthy controls.
Three sex steroid hormones (4- androsten-3 beta, 17 beta-diol disulfate 1; 5 alpha-androstan-3 beta, 17 beta-diol disulfate; and pregnen-diol disulfate; Bressler et al., 2017), and high arachidonic acid (AA) to DHA ratios (Abdullah et al., 2017) were associated with an increased risk of dementia, whereas hypoxanthine (Chouraki et al., 2017) decreased dementia risk. Several other circulating metabolites were also involved in the physiological processes and progression of cognitive disorders (Table 2).
Predictive Analysis
Seven studies assessed whether circulating metabolites significantly associated with dementia risk could be effectively used to distinguish or/and improve the power to predict MCI/dementia in a cognitively normal population (Oresic et al., 2011; Mapstone et al., 2014; Mousavi et al., 2014; Graham et al., 2015; Casanova et al., 2016; Abdullah et al., 2017; Li et al., 2017). Three studies reported a significant improvement in dementia prediction using combinations of metabolites relative to only traditional risk dementia factors (e.g., age, education, APOE ε4, Aβ, etc.; Oresic et al., 2011; Mapstone et al., 2014; Abdullah et al., 2017). However, only a small improvement in MCI/dementia prediction was observed in the study of Li et al, with only 0.03 increase in the C statistic (a measure of predictive ability; Li et al., 2017).
Using both untargeted and targeted MS-based platform analysis of 4600 features, Mapstone et al. (2014) identified a set of 10 lipids, after least absolute shrinkage and selection operator (LASSO) selection, that predicted MCI/AD reasonably well with an accuracy of >90% within a 3-year period. However, with the same metabolomics platform, Casanova et al. (2016) and Li et al. (2017) failed to replicate this 10-metabolite panel as predictor of incident MCI/dementia in three cohorts (i.e., BLSA, Age, Gene/Environment Susceptibility-Reykjavik Study [AGES-RS], and Atherosclerosis Risk in Communities-Neurocognitive Study [ARIC-NS]), with a C statistic of 0.642, 0.395, and 0.609, respectively.
Discussion
Through our comprehensive systematic review, we identified 16 articles, including 22 analyses, which prospectively evaluated the association between circulating metabolites and risk of dementia, three of which reported good diagnostic or predictive performance. All the included studies were conducted in western populations, and most were population-based cohort studies. MS-based approaches were predominantly applied to profile metabolite features in these analyses. Generally, elevated concentrations of some metabolites such as SM, PC, and glutamate were associated with a higher risk of dementia, whereas other metabolites (e.g., DHA and BCAAs) were associated with a lower risk. Most of the studies focused on lipids in blood samples as promising biomarker candidates. However, the same set of metabolites was rarely found in different populations, making it difficult to draw consistent conclusions from these studies.
Variations in lipid, energy, amino acid, purine, and neurotransmitter metabolism, and oxidative stress were associated with dementia pathogenesis in many previous cross-sectional animal models and human patient metabolomics studies (Seshadri et al., 2002; Thambisetty and Lovestone, 2010; Xu et al., 2012; Ahmed et al., 2014; Jove et al., 2014; Trushina and Mielke, 2014; Gonzalez-Dominguez et al., 2017; Thambisetty, 2017; Yi et al., 2017). In this systematic review, we confirmed that alterations in lipids, amino acids, hormones, and some other circulating metabolites were associated with risk of dementia, and could serve as potential biomarkers for screening of individuals at risk of dementia and in the development of future therapeutic strategies.
Lipids play critical roles in central nervous system homeostasis, including formation of lipid rafts, maintenance of cell membrane structure, and involvement in signal transduction (Trushina and Mielke, 2014; Han, 2016; Yi et al., 2017). Deficiencies of several lipid classes, such as phospholipid, PC, phosphatidylinositol, sphingolipid, lysophospholipid, SM, and sterols, were found in brain tissue, CSF, and blood in patients with dementia (Oresic et al., 2011; Xu et al., 2012; Trushina and Mielke, 2014; Yi et al., 2017). High levels of PC, SM, and lysoPC were associated with dementia risk in our systematic review (Oresic et al., 2011; Li et al., 2017; Toledo et al., 2017; Varma et al., 2018). Toledo et al. (2017) reported that PC and SM were associated with abnormal CSF Aβ1−42 values, supporting the theory that lipid rafts could facilitate the aggregation of Aβ, which may indicate loss of membrane function and neurodegeneration in early stage cognitive dysfunction (Rushworth and Hooper, 2010). Thus, SM- and PC- containing lipids varied with time with the onset of dementia, with higher levels presymptomatically and lower levels post-impairment (Mielke et al., 2010). DHA needs to be supplied by diet, can cross the blood-brain barrier via Mfsd2a (Nguyen et al., 2014), a component of the neuronal cell membrane and anti-inflammatory factor (Cardoso et al., 2016), showed low concentrations in brains of patients with AD (Soderberg et al., 1991), and is associated with cognitive decline (Cardoso et al., 2016). High blood levels of DHA, which are raised by eating fish, were beneficial for cognition and reduced the risk of dementia (van der Lee et al., 2018). Moreover, subclasses of HDL were related to good cognitive performance and lower dementia risk in 11 cohorts (van der Lee et al., 2018). Different HDL subspecies are involved in different functions, including repairing damaged membranes, lipid metabolism, cell signaling, and anti-oxidation, which lead to protective effects on the brain (Shah et al., 2013; van der Lee et al., 2018). Therefore, replication of blood lipid classes might be a more useful endeavor for understanding the basic biology of the preclinical state of dementia in the future.
Several amino acid variations, such as reduced BCAAs (Toledo et al., 2017; Tynkkynen et al., 2018), creatinine (Tynkkynen et al., 2018), and taurine (Chouraki et al., 2017), and higher glutamate (Chouraki et al., 2017), glutamine (van der Lee et al., 2018), and anthranilic acid (Chouraki et al., 2017), are associated with dementia. Alterations in BCAAs were observed in AD-like animal models (Salek et al., 2010; Pan et al., 2016) and patients with AD (Gonzalez-Dominguez et al., 2015; Yi et al., 2017). Essential amino acids could be an energy source whose disruption could indicate nutritional deficiencies and result in lipid membrane changes (Orsitto et al., 2009; Toledo et al., 2017; Tynkkynen et al., 2018). Further, aging caused changes in amino acid metabolism, which was a risk factor for dementia (Kodaira et al., 2017). Notably, as key factors of the glutamine-glutamate/GABA cycle, glutamine, and glutamate were associated with increased risk of dementia (Chouraki et al., 2017; van der Lee et al., 2018). Elevated plasma levels of anthranilic acid and glutamate, and lower levels of taurine were associated with a higher risk of dementia via the tryptophan-kynurenine pathway, which was linked to glutamate excitotoxicity in the pathogenesis of dementia in the Framingham Heart Study (Chouraki et al., 2017). Excess glutamate in brain is likely harmful whereas glutamine is beneficial, since the latter is a non-neuroactive intermediate in the recycling of amino acid neurotransmitters in the brain (Zhou and Danbolt, 2014). All the glutamine in brain is converted from glutamate in astrocytes; therefore, the glutamate and glutamine complex (Glx) represents the integrated metabolism and neurotransmitter functions of glutamate in the brain (Zhou and Danbolt, 2014; Jahng et al., 2016; Huang et al., 2017). Decreased glutamine was found in the brain in animal models of AD (Trushina and Mielke, 2014) and in the serum of patients with AD (Gonzalez-Dominguez et al., 2015; Yi et al., 2017), whereas elevated glutamine was associated with dementia risk in the analyses by van der Lee et al. (2018); this correlation and the underlying mechanisms require further confirmation.
Our systematic review revealed the diversity and complexity of current applications of metabolomics in dementia. Several putative metabolites have been proposed as potential biomarkers for preclinical dementia. However, it is difficult to reach a consistent conclusion, since biomarker validation in different populations is a major challenge.
Several aspects should be taken into consideration. First, differences among articles in study design, populations, cognitive testing, and outcome definitions introduce heterogeneity and affect evaluations and replications in independent populations. Most of these studies used cohort designs and recruited participants from the community, while two analyses included participants selected from memory clinics or prevention trials (Graham et al., 2015; Abdullah et al., 2017). However, nearly all of the relevant studies were based on retrospective analysis of samples collected for other outcomes or samples that were collected “just in case.” Prospective studies designed from conception to look specifically at metabolomics in dementia with appropriate participant recruitment, biological sample collection, processing, and storage are warranted. Moreover, the baseline cognitive status of participants [participants with MCI in three analyses (Oresic et al., 2011; Graham et al., 2015; Toledo et al., 2017)], methods used to identify outcomes, and durations of follow-up were different across all the included studies, and the metabolic profile may be different in different phases of disease (Trushina and Mielke, 2014). Further, age, sex, and race may also contribute to disparities in metabolite profile and prevalence of dementia (Jove et al., 2014; Trushina and Mielke, 2014; Gonzalez-Dominguez et al., 2017; Li et al., 2017), which could result in discrepancies in replication and predictive analyses.
Second, the utilization of diverse metabolomics analytical platforms and different biological samples (plasma or serum) resulted in variations in the data. MS-based methods coupled with variations in separation technique (i.e., gas chromatography [GC], liquid chromatography [LC], capillary electrophoresis [CE], etc.) were applied in all 16 studies, MS and NMR were both included in three articles (Toledo et al., 2017; Tynkkynen et al., 2018; van der Lee et al., 2018) in different cohorts, and only one cohort (the FINRISK 1997 study) used both LC-MS and NMR in serum (Tynkkynen et al., 2018). Eight analyses used untargeted approaches, while eight applied targeted approaches, which could result in differences in metabolite coverage. Each of these platforms has advantages and disadvantages, and none could capture the entire metabolomics map, which results in inconsistencies in the detected metabolites (Kaddurah-Daouk et al., 2008; Xu et al., 2012; Trushina and Mielke, 2014). Eight studies used serum, seven used plasma, and one used both (Tynkkynen et al., 2018). Samples were not collected in the same conditions. In addition, sample collection and storage were not uniform: the sample freeze time and freeze-and-thaw times were different. All these factors could cause inconsistencies and difficulties in the extrapolation of results (Breier et al., 2014). To link these variables directly to the studies in this review, for example, using the same metabolomics platform, Casanova et al. (2016) failed to replicate the metabolite panel that could predict AD found by Mapstone et al. (2014), which might largely be due to the different biological samples used in the two studies (plasma vs. serum).
Third, differences in statistical approach could be a further source of inconsistency. All the studies included in this systematic review aimed to identify individual circulating metabolites or a combination of metabolites related to cognitive decline or incident dementia (MCI or all-cause dementia). Cox (Mielke et al., 2010; Abdullah et al., 2017; Bressler et al., 2017; Chouraki et al., 2017; Tynkkynen et al., 2018; van der Lee et al., 2018) or logistic (Oresic et al., 2011; Mapstone et al., 2014; Casanova et al., 2016; Li et al., 2017) regression models were applied to evaluate the association of metabolites at baseline and incident dementia, while linear models were used in terms of cognitive decline (Casanova et al., 2016; Bressler et al., 2017; Li et al., 2017; Toledo et al., 2017). Three studies used data-driven multivariate methods, such as orthogonal to partial least squares discriminant analysis (OPLS-DA) (Mousavi et al., 2014; Graham et al., 2015) and machine learning (i.e., RF, SVM, etc.; Casanova et al., 2016; Varma et al., 2018). Different data reduction approaches and multiple testing correction were applied in some of the studies, including principal component analysis (PCA) (Tynkkynen et al., 2018), LASSO (Mapstone et al., 2014), Bayesian model-based clustering (Oresic et al., 2011), Bonferroni or false discovery rate (FDR) correction (Mapstone et al., 2014; Casanova et al., 2016; Abdullah et al., 2017; Toledo et al., 2017; Dorninger et al., 2018), and others (Bressler et al., 2017; Chouraki et al., 2017; van der Lee et al., 2018). In addition, pretreatment of metabolite data, including scaling, normalization and transformation, sample size, and covariates in adjusted models were inconsistent across different studies. All of these are critical aspects in data-mining, and could affect the selection of metabolites (Guasch-Ferre et al., 2016; Ruiz-Canela et al., 2017; Yi et al., 2017).
Taken together, there is a need to standardize the workflow for study design, participant selection, sample collection and storage, and experimental and data procedures (Lindon et al., 2005; Yi et al., 2017). Application of combined analytical platforms is an attractive prospect for future metabolomics studies (Xu et al., 2012; Yi et al., 2017). Moreover, it is imperative to conduct studies in larger, well-phenotyped, serial biospecimens, with more genetic background cohorts to enhance the identification of metabolites in the prediction of dementia, especially in stages before MCI.
Several limitations of this systematic review should be acknowledged. First, our search was limited to English, which excluded studies that were published in other languages. However, most articles are published and indexed in English in PubMed, Embase, and the Cochrane Library. Second, we only included blood metabolites measured by high-throughput analytical approaches, which would exclude studies evaluating only one or several metabolites before the development of metabolomics, or conducted in other types of biospecimens related to risk of dementia. However, we aimed at identifying circulating metabolites in metabolomics profiles associated with cognitive decline or progression to dementia, which could be promising biomarkers of preclinical dementia. Third, we were unable to conduct a quantitative meta-analysis, owing to the inconsistency of the results of the included articles.
Conclusion
Metabolomics is a promising novel approach to understand the pathophysiology of dementia and identify biomarkers for the risk of dementia. This systematic review provides evidence that several metabolites, including lipids (mainly PC, lyso PC, SM, and HDL subfractions), amino acids (BCAAs, glutamate, etc.), and steroids are associated with cognitive performance change and risk of dementia. These findings highlight the translational strength of metabolomics and heterogeneities among current studies. To improve the identification of potential biomarkers of dementia, standardized designs, and experimental protocols for metabolomics studies in larger number of participants, longer follow-up time, and serial blood samples in diverse populations are warranted.
Author Contributions
XC, MC, and YJ designed this review. YJ, ZZ, and JS searched literature and assessed their quality. YJ wrote the initial manuscript. LJ and WY supervised and provided critical comments on the manuscript. All the authors (YJ, ZZ, JS, YA, KZ, YW, SL, LJ, WY, MC, and XC) read, amend, and discussed the article.
Funding
This study was supported by the National Key Research and Development program of China (grant numbers: 2017YFC0907002, 2017YFC0907501/4, 2017YFC0907201), the International Science and Technology Cooperation Program of China (grant number: 2014DFA32830), the Key Research and Development Plans of Jiangsu Province, China (grant number: BE2016726), the Key Basic Research Grants from Science and Technology Commission of Shanghai Municipality, China (grant number: 16JC1400501), Shanghai Municipal Science and Technology Major Project (grant number: 2017SHZDZX01), and the Interdisciplinary Program of Shanghai Jiao Tong University (grant number: YG2016MS40).
Conflict of Interest Statement
The authors declare that the research was conducted in the absence of any commercial or financial relationships that could be construed as a potential conflict of interest.
Supplementary Material
The Supplementary Material for this article can be found online at: https://www.frontiersin.org/articles/10.3389/fnins.2019.00343/full#supplementary-material
References
Abdullah, L., Evans, J. E., Emmerich, T., Crynen, G., Shackleton, B., Keegan, A. P., et al. (2017). APOE ε4 specific imbalance of arachidonic acid and docosahexaenoic acid in serum phospholipids identifies individuals with preclinical Mild Cognitive Impairment/Alzheimer's Disease. Aging 9, 964–985. doi: 10.18632/aging.101203
Ahmed, R. M., Paterson, R. W., Warren, J. D., Zetterberg, H., O'Brien, J. T., Fox, N. C., et al. (2014). Biomarkers in dementia: clinical utility and new directions. J. Neurol. Neurosurg. Psychiatry 85, 1426–1434. doi: 10.1136/jnnp-2014-307662
Association, A. P. (1987). Diagnostic and Statistical Manual of Mental Disorders: DSM-III-R. Washington, DC: American Psychiatric Association.
Association, A. P. (1994). Diagnostic and Statistical Manual of Mental Disorders: DSM-IV. Washington, DC: American Psychiatric Association.
Association, A. P. (2013). Diagnostic and Statistical Manual of Mental Disorders: DSM-5. Washington, DC: American Psychiatric Association.
Breier, M., Wahl, S., Prehn, C., Fugmann, M., Ferrari, U., Weise, M., et al. (2014). Targeted metabolomics identifies reliable and stable metabolites in human serum and plasma samples. PLoS ONE 9:e89728. doi: 10.1371/journal.pone.0089728
Bressler, J., Yu, B., Mosley, T. H., Knopman, D. S., Gottesman, R. F., Alonso, A., et al. (2017). Metabolomics and cognition in African American adults in midlife: the atherosclerosis risk in communities study. Transl. Psychiatry 7:e1173. doi: 10.3233/jad-170485
Cardoso, C., Afonso, C., and Bandarra, N. M. (2016). Dietary DHA and health: cognitive function ageing. Nutr. Res. Rev. 29, 281–294. doi: 10.1017/s0954422416000184
Casanova, R., Varma, S., Simpson, B., Kim, M., An, Y., Saldana, S., et al. (2016). Blood metabolite markers of preclinical Alzheimer's disease in two longitudinally followed cohorts of older individuals. Alzheimers Dement. 12, 815–822. doi: 10.1016/j.jalz.2015.12.008
Chouraki, V., Preis, S. R., Yang, Q., Beiser, A., Li, S., Larson, M. G., et al. (2017). Association of amine biomarkers with incident dementia and Alzheimer's disease in the Framingham Study. Alzheimers Dement. 13, 1327–1336. doi: 10.1007/s00415-017-8647-0
Dorninger, F., Moser, A. B., Kou, J., Wiesinger, C., Forss-Petter, S., Gleiss, A., et al. (2018). Alterations in the plasma levels of specific choline phospholipids in Alzheimer's disease mimic accelerated aging. J. Alzheimers Dis. 62, 841–854. doi: 10.3233/jad-171036
Fiandaca, M. S., Mapstone, M. E., Cheema, A. K., and Federoff, H. J. (2014). The critical need for defining preclinical biomarkers in Alzheimer's disease. Alzheimers Dement. 10, S196–212. doi: 10.1016/j.jalz.2014.04.015
Fiandaca, M. S., Zhong, X., Cheema, A. K., Orquiza, M. H., Chidambaram, S., Tan, M. T., et al. (2015). Plasma 24-metabolite panel predicts preclinical transition to clinical stages of Alzheimer's disease. Front. Neurol. 6:237. doi: 10.3389/fneur.2015.00237
German, J. B., Hammock, B. D., and Watkins, S. M. (2005). Metabolomics: building on a century of biochemistry to guide human health. Metabolomics 1, 3–9. doi: 10.1007/s11306-005-1102-8
Gonzalez-Dominguez, R., Garcia-Barrera, T., and Gomez-Ariza, J. L. (2015). Metabolite profiling for the identification of altered metabolic pathways in Alzheimer's disease. J. Pharm. Biomed. Anal. 107, 75–81. doi: 10.1016/j.jpba.2014.10.010
Gonzalez-Dominguez, R., Sayago, A., and Fernandez-Recamales, A. (2017). Metabolomics in Alzheimer's disease: the need of complementary analytical platforms for the identification of biomarkers to unravel the underlying pathology. J. Chromatogr. B Analyt. Technol. Biomed. Life Sci. 1071, 75–92. doi: 10.1016/j.jchromb.2017.02.008
Graham, S. F., Chevallier, O. P., Elliott, C. T., Holscher, C., Johnston, J., McGuinness, B., et al. (2015). Untargeted metabolomic analysis of human plasma indicates differentially affected polyamine and L-arginine metabolism in mild cognitive impairment subjects converting to Alzheimer's disease. PLoS ONE 10:e0119452. doi: 10.1371/journal.pone.0119452
Guasch-Ferre, M., Hruby, A., Toledo, E., Clish, C. B., Martinez-Gonzalez, M. A., Salas-Salvado, J., et al. (2016). Metabolomics in prediabetes and diabetes: a systematic review and meta-analysis. Diabetes Care 39, 833–846. doi: 10.2337/dc15-2251
Han, X. (2016). Lipidomics for studying metabolism. Nat. Rev. Endocrinol. 12, 668–679. doi: 10.1038/nrendo.2016.98
Henriksen, K., O'Bryant, S. E., Hampel, H., Trojanowski, J. Q., Montine, T. J., Jeromin, A., et al. (2014). The future of blood-based biomarkers for Alzheimer's disease. Alzheimers Dement. 10, 115–131. doi: 10.1016/j.jalz.2013.01.013
Huang, D., Liu, D., Yin, J., Qian, T., Shrestha, S., and Ni, H. (2017). Glutamate-glutamine and GABA in brain of normal aged and patients with cognitive impairment. Eur. Radiol. 27, 2698–2705. doi: 10.1007/s00330-016-4669-8
Jahng, G. H., Oh, J., Lee, D. W., Kim, H. G., Rhee, H. Y., Shin, W., et al. (2016). Glutamine and glutamate complex, as measured by functional magnetic resonance spectroscopy, alters during face-name association task in patients with mild cognitive impairment and Alzheimer's disease. J. Alzheimers Dis. 52, 145–159. doi: 10.3233/jad-150877
Jove, M., Portero-Otin, M., Naudi, A., Ferrer, I., and Pamplona, R. (2014). Metabolomics of human brain aging and age-related neurodegenerative diseases. J. Neuropathol. Exp. Neurol. 73, 640–657. doi: 10.1097/nen.0000000000000091
Kaddurah-Daouk, R., Kristal, B. S., and Weinshilboum, R. M. (2008). Metabolomics: a global biochemical approach to drug response and disease. Annu. Rev. Pharmacol. Toxicol. 48, 653–683. doi: 10.1146/annurev.pharmtox.48.113006.094715
Kodaira, M., Nagasawa, M., Yamaguchi, T., Ikeda, H., Minaminaka, K., Chowdhury, V. S., et al. (2017). Aging rather than stress strongly influences amino acid metabolisms in the brain and genital organs of female mice. Mech. Ageing Dev. 162, 72–79. doi: 10.1016/j.mad.2016.12.006
Li, D., Misialek, J. R., Boerwinkle, E., Gottesman, R. F., Sharrett, A. R., Mosley, T. H., et al. (2016). Plasma phospholipids and prevalence of mild cognitive impairment and/or dementia in the ARIC Neurocognitive Study (ARIC-NCS). Alzheimer's Dement. 3, 73–82. doi: 10.1016/j.dadm.2016.02.008
Li, D., Misialek, J. R., Boerwinkle, E., Gottesman, R. F., Sharrett, A. R., Mosley, T. H., et al. (2017). Prospective associations of plasma phospholipids and mild cognitive impairment/dementia among African Americans in the ARIC Neurocognitive Study. Alzheimer's Dement. 6, 1–10. doi: 10.1016/j.dadm.2016.09.003
Lindon, J. C., Nicholson, J. K., Holmes, E., Keun, H. C., Craig, A., Pearce, J. T., et al. (2005). Summary recommendations for standardization and reporting of metabolic analyses. Nat. Biotechnol. 23, 833–838. doi: 10.1038/nbt0705-833
Livingston, G., Sommerlad, A., Orgeta, V., Costafreda, S. G., Huntley, J., Ames, D., et al. (2017). Dementia prevention, intervention, and care. Lancet 390, 2673–2734. doi: 10.1016/s0140-6736(17)31363-6
Mapstone, M., Cheema, A. K., and Fiandaca, M. S. (2014). Plasma phospholipids identify antecedent memory impairment in older adults. Nat. Med. 20, 415–418. doi: 10.1038/nm.3466
McKhann, G., Drachman, D., Folstein, M., Katzman, R., Price, D., and Stadlan, E. M. (1984). Clinical diagnosis of Alzheimer's disease: report of the NINCDS-ADRDA Work Group under the auspices of Department of Health and Human Services Task Force on Alzheimer's Disease. Neurology 34, 939–944.
Mielke, M. M., Bandaru, V. V., Haughey, N. J., Rabins, P. V., Lyketsos, C. G., and Carlson, M. C. (2010). Serum sphingomyelins and ceramides are early predictors of memory impairment. Neurobiol. Aging 31, 17–24. doi: 10.1016/j.neurobiolaging.2008.03.011
Mousavi, M., Jonsson, P., Antti, H., Adolfsson, R., Nordin, A., Bergdahl, J., et al. (2014). Serum metabolomic biomarkers of dementia. Dement. Geriatr. Cogn. Disord. Extra 4, 252–262. doi: 10.1159/000364816
Mullard, A. (2016). Alzheimer amyloid hypothesis lives on. Nat. Rev. Drug Discov. 16, 3–5. doi: 10.1038/nrd.2016.281
Nguyen, L. N., Ma, D., Shui, G., Wong, P., Cazenave-Gassiot, A., Zhang, X., et al. (2014). Mfsd2a is a transporter for the essential omega-3 fatty acid docosahexaenoic acid. Nature 509, 503–506. doi: 10.1038/nature13241
Oresic, M., Hyotylainen, T., Herukka, S. K., Sysi-Aho, M., Mattila, I., Seppanan-Laakso, T., et al. (2011). Metabolome in progression to Alzheimer's disease. Transl. Psychiatry 1:e57. doi: 10.1038/tp.2011.55
Orsitto, G., Fulvio, F., Tria, D., Turi, V., Venezia, A., and Manca, C. (2009). Nutritional status in hospitalized elderly patients with mild cognitive impairment. Clin. Nutr. 28, 100–102. doi: 10.1016/j.clnu.2008.12.001
Pan, X., Nasaruddin, M. B., Elliott, C. T., McGuinness, B., Passmore, A. P., Kehoe, P. G., et al. (2016). Alzheimer's disease-like pathology has transient effects on the brain and blood metabolome. Neurobiol. Aging 38, 151–163. doi: 10.1016/j.neurobiolaging.2015.11.014
Petersen, R. C., Smith, G. E., Waring, S. C., Ivnik, R. J., Tangalos, E. G., and Kokmen, E. (1999). Mild cognitive impairment: clinical characterization and outcome. Arch. Neurol. 56, 303–308.
Prince, M., Wimo, A., Guerchet, M., Ali, G.-C., Wu, Y.-T., and Prina, M. (2015). World Alzheimer Report 2015. The Global Impact of Dementia: An Analysis of Prevalence, Incidence, Cost and Trends. Alzheimer's Disease International.
Proitsi, P., Kim, M., Whiley, L., Pritchard, M., Leung, R., Soininen, H., et al. (2015). Plasma lipidomics analysis finds long chain cholesteryl esters to be associated with Alzheimer's disease. Trans. Psychiatry 5:e494. doi: 10.1038/tp.2014.127
Ruiz-Canela, M., Hruby, A., Clish, C. B., Liang, L., Martinez-Gonzalez, M. A., and Hu, F. B. (2017). Comprehensive metabolomic profiling and incident cardiovascular disease: a systematic review. J Am Heart Assoc. 6:e005705. doi: 10.1161/jaha.117.005705
Rushworth, J. V., and Hooper, N. M. (2010). Lipid rafts: linking Alzheimer's amyloid-β production, aggregation, and toxicity at neuronal membranes. Int. J. Alzheimer's Dis. 2011:603052. doi: 10.4061/2011/603052
Salek, R. M., Xia, J., Innes, A., Sweatman, B. C., Adalbert, R., Randle, S., et al. (2010). A metabolomic study of the CRND8 transgenic mouse model of Alzheimer's disease. Neurochem. Int. 56, 937–947. doi: 10.1016/j.neuint.2010.04.001
Seshadri, S., Beiser, A., Selhub, J., Jacques, P. F., Rosenberg, I. H., D'Agostino, R. B., et al. (2002). Plasma homocysteine as a risk factor for dementia and Alzheimer's disease. N. Engl. J. Med. 346, 476–483. doi: 10.1056/NEJMoa011613
Shah, A. S., Tan, L., Long, J. L., and Davidson, W. S. (2013). Proteomic diversity of high density lipoproteins: our emerging understanding of its importance in lipid transport and beyond. J. Lipid Res. 54, 2575–2585. doi: 10.1194/jlr.R035725
Simpson, B. N., Kim, M., Chuang, Y. F., Beason-Held, L., Kitner-Triolo, M., Kraut, M., et al. (2016). Blood metabolite markers of cognitive performanceand brain function in aging. J. Cereb. Blood Flow Metab. 36, 1212–1223. doi: 10.1177/0271678x15611678
Soderberg, M., Edlund, C., Kristensson, K., and Dallner, G. (1991). Fatty acid composition of brain phospholipids in aging and in Alzheimer's disease. Lipids 26, 421–425.
Stroup, D. F., Berlin, J. A., Morton, S. C., Olkin, I., Williamson, G. D., Rennie, D., et al. (2000). Meta-analysis of observational studies in epidemiology: a proposal for reporting. JAMA 283, 2008–2012. doi: 10.1001/jama.283.15.2008
Thambisetty, M. (2017). Understanding mechanisms and seeking cures for Alzheimer's disease: why we must be “extraordinarily diverse”. Am. J. Physiol. Cell Physiol. 313, C353–c361. doi: 10.1152/ajpcell.00111.2017
Thambisetty, M., and Lovestone, S. (2010). Blood-based biomarkers of Alzheimer's disease: challenging but feasible. Biomark Med. 4, 65–79. doi: 10.2217/bmm.09.84
Toledo, J. B., Arnold, M., Kastenmuller, G., Chang, R., Baillie, R. A., Han, X., et al. (2017). Metabolic network failures in Alzheimer's disease: a biochemical road map. Alzheimers Dement. 13, 965–984. doi: 10.1016/j.jalz.2017.01.020
Trushina, E., and Mielke, M. M. (2014). Recent advances in the application of metabolomics to Alzheimer's Disease. Biochim. Biophys. Acta 1842, 1232–1239. doi: 10.1016/j.bbadis.2013.06.014
Tynkkynen, J., Chouraki, V., van der Lee, S. J., Hernesniemi, J., Yang, Q., Li, S., et al. (2018). Association of branched-chain amino acids and other circulating metabolites with risk of incident dementia and Alzheimer's disease: a prospective study in eight cohorts. Alzheimers Dement. 14, 723–733. doi: 10.1016/j.jalz.2018.01.003
van der Lee, S. J., Teunissen, C. E., Pool, R., Shipley, M. J., Teumer, A., Chouraki, V., et al. (2018). Circulating metabolites and general cognitive ability and dementia: evidence from 11 cohort studies. Alzheimers Dement. 14, 707–722. doi: 10.1016/j.jalz.2017.11.012
Varma, V. R., Oommen, A. M., Varma, S., Casanova, R., An, Y., Andrews, R. M., et al. (2018). Brain and blood metabolite signatures of pathology and progression in Alzheimer disease: a targeted metabolomics study. PLoS Med. 15:e1002482. doi: 10.1371/journal.pmed.1002482
Wang, G., Zhou, Y., Huang, F. J., Tang, H. D., Xu, X. H., Liu, J. J., et al. (2014). Plasma metabolite profiles of Alzheimer's disease and mild cognitive impairment. J. Proteome Res. 13, 2649–2658. doi: 10.1021/pr5000895
Wells, G. A., Shea, B. J., O'Connell, D., Peterson, J., Welch, V., Losos, M., et al. (2014). The newcastle–ottawa scale (NOS) for assessing the quality of non-randomized studies in meta-analysis. Appl. Eng. Agric. 18, 727–734.
Wimo, A., Guerchet, M., Ali, G. C., Wu, Y. T., Prina, A. M., Winblad, B., et al. (2017). The worldwide costs of dementia 2015 and comparisons with 2010. Alzheimers Dement. 13, 1–7. doi: 10.1016/j.jalz.2016.07.150
Xu, X.-H., Huang, Y., Wang, G., and Chen, S.-D. (2012). Metabolomics: a novel approach to identify potential diagnostic biomarkers and pathogenesis in Alzheimer's disease. Neurosci. Bull. 28, 641–648. doi: 10.1007/s12264-012-1272-0
Yi, L., Liu, W., Wang, Z., Ren, D., and Peng, W. (2017). Characterizing Alzheimer's disease through metabolomics and investigating anti-Alzheimer's disease effects of natural products. Ann. N. Y. Acad. Sci. 1398, 130–141. doi: 10.1111/nyas.13385
Keywords: metabolomics, lipidomics, metabolites, dementia, Alzheimer's disease, mild cognitive impairment
Citation: Jiang Y, Zhu Z, Shi J, An Y, Zhang K, Wang Y, Li S, Jin L, Ye W, Cui M and Chen X (2019) Metabolomics in the Development and Progression of Dementia: A Systematic Review. Front. Neurosci. 13:343. doi: 10.3389/fnins.2019.00343
Received: 24 January 2019; Accepted: 25 March 2019;
Published: 12 April 2019.
Edited by:
Stefano L. Sensi, Università degli Studi G. d'Annunzio Chieti e Pescara, ItalyReviewed by:
Mark Mapstone, University of California, Irvine, United StatesArianna Polverino, Università degli Studi di Napoli Parthenope, Italy
Copyright © 2019 Jiang, Zhu, Shi, An, Zhang, Wang, Li, Jin, Ye, Cui and Chen. This is an open-access article distributed under the terms of the Creative Commons Attribution License (CC BY). The use, distribution or reproduction in other forums is permitted, provided the original author(s) and the copyright owner(s) are credited and that the original publication in this journal is cited, in accordance with accepted academic practice. No use, distribution or reproduction is permitted which does not comply with these terms.
*Correspondence: Mei Cui, Y3VpbWVpQGZ1ZGFuLmVkdS5jbg==
Xingdong Chen, eGluZ2RvbmdjaGVuQGZ1ZGFuLmVkdS5jbg==