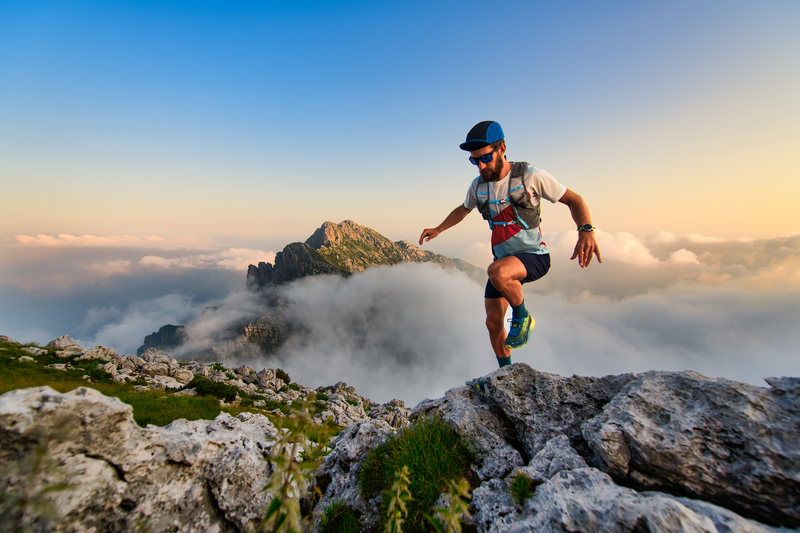
95% of researchers rate our articles as excellent or good
Learn more about the work of our research integrity team to safeguard the quality of each article we publish.
Find out more
ORIGINAL RESEARCH article
Front. Neurosci. , 11 September 2024
Sec. Translational Neuroscience
Volume 18 - 2024 | https://doi.org/10.3389/fnins.2024.1411538
Background: Stroke stands as a prominent cause of mortality and disability worldwide, posing a major public health concern. Recent years have witnessed rapid advancements in artificial intelligence (AI). Studies have explored the utilization of AI in imaging analysis, assistive rehabilitation, treatment, clinical decision-making, and outcome and risk prediction concerning stroke. However, there is still a lack of systematic bibliometric analysis to discern the current research status, hotspots, and possible future development trends of AI applications in stroke.
Methods: The publications on the application of AI in stroke were retrieved from the Web of Science Core Collection, spanning 2004–2024. Only articles or reviews published in English were included in this study. Subsequently, a manual screening process was employed to eliminate literature not pertinent to the topic. Visualization diagrams for comprehensive and in-depth analysis of the included literature were generated using CiteSpace, VOSviewer, and Charticulator.
Results: This bibliometric analysis included a total of 2,447 papers, and the annual publication volume shows a notable upward trajectory. The most prolific authors, countries, and institutions are Dukelow, Sean P., China, and the University of Calgary, respectively, making significant contributions to the advancement of this field. Notably, stable collaborative networks among authors and institutions have formed. Through clustering and citation burst analysis of keywords and references, the current research hotspots have been identified, including machine learning, deep learning, and AI applications in stroke rehabilitation and imaging for early diagnosis. Moreover, emerging research trends focus on machine learning as well as stroke outcomes and risk prediction.
Conclusion: This study provides a comprehensive and in-depth analysis of the literature regarding AI in stroke, facilitating a rapid comprehension of the development status, cooperative networks, and research priorities within the field. Furthermore, our analysis may provide a certain reference and guidance for future research endeavors.
Stroke stands as a prominent cause of death and disability worldwide, presenting a significant public health threat. In 2016, there were 13.7 million new stroke incidents worldwide, with approximately 87% being ischemic strokes (IS) (Saini et al., 2021). Through the analysis of data from 1,599 hospitals across China in 2020, it was found that IS comprised 81.9% of the total stroke cases, while intracerebral hemorrhage and subarachnoid hemorrhage strokes accounted for 14.9 and 3.1%, respectively (Tu et al., 2023). A study predicted that mortalities of stroke worldwide will surge from 6.6 million in 2020 to 9.7 million by 2050 (Feigin et al., 2023). The incidence of stroke and its sequelae severely impacts patients’ quality of life and imposes a substantial economic burden. It was estimated that the annual direct costs (such as treatment and rehabilitation) and indirect costs (productivity loss) associated with global stroke exceed $891 billion (Feigin et al., 2023). Therefore, it is necessary to explore effective approaches to enhance diagnostic accuracy and efficiency, guide clinical treatment, rehabilitation, and acute care methods, and predict stroke prognosis.
Artificial intelligence (AI), a branch within computer science, emulates thinking processes, learning abilities, and knowledge management (Sak and Suchodolska, 2021). Its integration into healthcare has experienced rapid growth in recent years, with numerous research teams exploring its application in stroke imaging analysis, assistive rehabilitation, treatment, clinical decision-making, as well as outcome and risk prediction for stroke (Heo et al., 2019; Mouridsen et al., 2020; Yedavalli et al., 2021; Lip et al., 2022; Liu et al., 2022). Studies have demonstrated that AI facilitates decreasing inter-rater divergence, thereby enhancing the standardization of stroke patient assessment. Furthermore, it enables the rapid identification of valuable imaging data for aiding in the selection of treatment schemes and bolstering clinical decision-making (Bivard et al., 2020; Akay et al., 2023). Nonetheless, a systematic bibliometric analysis is still lacking to identify the current research status, hotspots, and potential future trends in AI applications for stroke.
Bibliometric analysis utilizes mathematical and statistical methods to conduct scientific quantitative analysis of literature within a certain field. This approach enables the examination of collaborative relationships among authors, institutions, and countries, thus obtaining directions for further cooperation and academic communication. Through a thorough analysis of keywords and references extracted from pertinent literature, we can better comprehend the knowledge foundation and development context regarding the application of AI in stroke. Commonly utilized software for bibliometric analysis, such as VOSviewer and CiteSpace, generates visualization graphs to elucidate research hotspots and trends more clearly and intuitively. Consequently, we perform this bibliometric analysis of relevant publications spanning 2004–2024, aiming to provide useful information for subsequent studies.
The Web of Science Core Collection (WoSCC) is a crucial database for accessing global academic information, comprising a substantial collection of influential and high-quality publications. We selected this database for our analysis due to its ability to extensively retrieve relevant literature and its widespread use in bibliometric analysis (Jiang et al., 2023; Song et al., 2024; Yang et al., 2024). In this study, we conducted a comprehensive retrieval of the WoSCC database from January 1, 2004 to March 1, 2024 to identify pertinent literature on the application of AI in stroke. The search formula was TS = (“artificial intelligence” OR “AI” OR “machine intelligence” OR “computational intelligence” OR “robot*” OR “computer reasoning” OR “computer vision system” OR “neural network*” OR “machine learning” OR “deep learning” OR “natural language processing”) AND TS = (“stroke” OR “cerebrovascular accident” OR “CVA” OR “cerebrovascular apoplexy” OR “brain vascular accident” OR “cerebrovascular stroke” OR “cerebral stroke” OR “apoplexy” OR “acute stroke” OR “acute cerebrovascular accident”). We included reviews and articles in our study, restricting the language of publications to English. After the screening of the titles and abstracts of the retrieved literature, incomplete, duplicate, and irrelevant studies were excluded. Subsequently, the remaining publications were exported with full records and cited references in TXT form.
This bibliometric analysis primarily employed CiteSpace 6.3.R1 (Chen, 2006) and VOSviewer V1.6.19 tools (van Eck and Waltman, 2010) to analyze pertinent information and references from the included literature, thus identifying research foci and frontiers. VOSviewer generates visualization network maps of journals and keywords, along with illustrating collaborative relationships among core authors and institutions. Node size corresponds to publication frequency, while the lines depict the intensity of the relationship between nodes. Additionally, a chord diagram was created using Charticulator to visualize the collaboration between countries or regions. CiteSpace was applied to conduct the dual-map overlay of journals and clustering of references. Besides, it generated citation bursts of keywords and references, providing valuable information for exploring the development status and trends of research. The flow chart for screening literature and analysis is displayed in Figure 1.
Through the search and screening of pertinent literature spanning from 2004 to 2024, a total of 2,447 publications are included, comprising 2,215 articles and 232 reviews. The annual publication trend regarding AI application in stroke is depicted in Figure 2, revealing a consistent increase over time. This suggests a growing scholarly interest in applying AI to stroke research. Notably, there was a peak of 462 articles published in 2023 and 68 articles published in the first 2 months of 2024.
A total of 11,883 authors have contributed to the literature concerning the application of AI to stroke. According to Price’s law, the minimum publication volume of core authors in a certain field is m = 0.749× , where nmax represents the publication counts of the most prolific author. Therefore, a core author should have at least published five papers. Figure 3 illustrates the collaboration network map of core authors. It can be seen that several stable author collaboration groups have been formed. Table 1 lists the top 10 most productive authors concerning the application of AI to stroke. Notably, Dukelow, Sean P. leads with 35 articles. His studies primarily focused on using AI to assess proprioceptive impairment and visuomotor adaptation impairment after stroke, as well as promoting stroke rehabilitation through robotic interventions (Dukelow, 2017; Keeling et al., 2021; Moore et al., 2022; Chilvers et al., 2023; Hossain et al., 2023). Additionally, Scott, Stephen H. gained a relatively higher average citation, indicating his publications are influential and recognized by scholars.
A total of 84 countries/regions are involved in AI applications for stroke, and Table 2 displays the top 10 countries/regions in terms of frequency. China was the leading country with 688 articles, followed by the USA (653 articles) and Italy (284 articles). The total link strength (TLS) reflects collaboration intensity with other nations, with the United States demonstrating the highest TLS, signifying robust international cooperation in AI application to stroke. Figure 4 shows a chord diagram of national cooperation, with colored areas representing publications from different countries. The size of each area manifests publication volume, while the line thickness between areas signifies cooperation strength. Notably, the USA exhibits close relations with China, England, and Italy. Moreover, collaboration between other countries still needs further strengthening.
Figure 4. The chord diagram of cooperation between countries/regions about the application of AI in stroke from 2004 to 2024.
Table 3 outlines the top 10 institutions by publication frequency. The University of Calgary emerges as the most productive organization with 70 papers, while the Massachusetts Institute of Technology boasts the largest TLS. Figure 5 manifests the cooperation network map of institutions, revealing several collaborative clusters headed by the University of Calgary, Capital Medical University, Queen’s University, and National Taiwan University. In addition, we found that cooperation between institutions predominantly occurs between adjacent regions, and academic exchange across different regions requires enhancement.
Tables 4, 5 delineates the top 10 journals and co-cited journals pertaining to the application of AI in stroke. The Journal of Neuroengineering and Rehabilitation leads with the highest number of articles on this topic, followed by Frontiers in Neurology and IEEE Transactions on Neural Systems and Rehabilitation Engineering. The impact factor (IF) of these top 10 journals ranges from 1.7 to 7.8. Figure 6A presents a network visualization map of journals, categorized into different colored clusters. The largest red cluster primarily focuses on neuroimaging, the blue cluster concentrates on rehabilitation, and the green cluster involves neuroscience and neurorehabilitation. Moreover, the journals most frequently co-cited were Stroke (7,925 times), Neurorehabilitation and Neural Repair (3,263 times), and Archives of Physical Medicine and Rehabilitation (3,057 times).
Figure 6. (A) The network visualization map of journals; (B) The dual-map overlay of journals about the application of AI in stroke.
Figure 6B depicts the dual-map overlay of journals. The left side of the map shows citing journals, indicating the frontiers of knowledge, while the right side displays cited journals, representing the foundation of knowledge. Colored paths denote citation links, delineating the association between various research disciplines. The most prominent pink citation lines manifest that studies in the fields of molecular/biology/genetics, health/nursing/medicine, sports/rehabilitation/sport, and psychology/education/social are frequently cited by research in neurology/sports/ophthalmology.
After analyzing keywords with a frequency of more than 15 times, Figure 7 displays a network visualization map of keywords. Notably, there are three prominent clusters, which mainly encompass stroke, machine learning, and rehabilitation. Additionally, Figure 8, generated by CiteSpace, shows the top 25 keywords with the strongest citation bursts, facilitating the exploration of research focus and frontiers. Burst strength and time span serve as significant indicators in keyword burst analysis. The top three keywords with the strongest citation burst are “arm” (2008–2017), “induced movement therapy” (2004–2016), and “upper limb” (2005–2015). Examining keyword bursts in recent years aids in predicting future research trends and topics. Hence, keywords such as “artificial intelligence,” “machine learning,” “risk,” and “risk factors” offer valuable references for future studies.
Co-citation analysis of references offers insights into the knowledge foundation of the research field and identifies influential literature. The top 10 co-cited references in terms of citation counts are shown in Table 6, among which a retrospective study by Heo J. in 2019 was the most frequently cited. This study, involving 2,604 patients, demonstrated that machine learning models contribute to predicting long-term outcomes in acute stroke patients. Notably, the deep neural network model performed better than the random forest and logistic regression models (Heo et al., 2019).
Clustering analysis of references applying CiteSpace generated 100 clusters with modularity and weighted mean silhouette values of 0.8705 and 0.9468, respectively. Figure 9A depicts the top 12 clusters, with the three largest clusters focusing on machine learning, gait, and robotics. Besides, deep learning, robotic rehabilitation, robot-assisted gait training, and brain-computer interfaces are also considered research emphases.
Figure 9. (A) The clustering analysis of co-cited references; (B) The top 25 references with citation bursts about the application of AI in stroke.
Reference bursts manifest a significant increase in the attention of articles within a certain period of time. As shown in Figure 9B, the publication with the highest burst strength (45.04) among the top 25 co-cited references is “Robot-assisted therapy for long-term upper-limb impairment after stroke.” This article revealed that robot-assisted rehabilitation for 12 weeks did not show significant advantages compared to usual care and intensive comparative treatment in patients with moderate to severe upper-limb impairment 6 months post-stroke. Nevertheless, over 36 weeks, robot-assisted treatment significantly improved the motor function of patients compared to usual care, but not compared to intensive comparative treatment (Lo et al., 2010). Furthermore, a systematic review of robot-assisted therapy for upper limb recovery post-stroke has gained the second-largest burst strength from 2009 to 2013. It highlighted the potential of robot-assisted therapy for enhancing proximal upper extremity function (Kwakkel et al., 2008). The citation bursts of the references in the lower three lines of Figure 9B extend until 2024, including a guideline on the management of acute ischemic stroke (Powers et al., 2019) and two studies on using machine learning to predict clinical outcomes in stroke patients (Heo et al., 2019; Brugnara et al., 2020). These provide information for identifying research frontiers in AI applications for stroke.
In this study, we conducted a bibliometric analysis of relevant literature concerning the application of AI in stroke retrieved from 2004 to 2024. A total of 2,447 articles were included, with a peak of 462 publications in 2023. The annual publication count remained below 100 until 2017, but it has grown rapidly after 2019. According to Table 1, the authors with the highest publications and the largest average citation are Dukelow, Sean P., and Scott, Stephen H., respectively. Their work appears to be highly influential and contributes to the development of this field. Notably, several stable collaborative groups of authors and institutions have emerged, although there remains a need to further strengthen cross-regional academic cooperation. In terms of countries, China ranks first with 688 articles and shows close cooperation with the United States. The Journal of Neuroengineering and Rehabilitation, Stroke, and Neurorehabilitation and Neural Repair appeared in the top 10 journals and co-cited journals in terms of frequency, serving as valuable references for future scholars in this field.
According to Figures 8, 9A, the largest cluster, labeled #0 machine learning, not only represents a current research hotspot but also signifies the forefront of future investigations. Machine learning (ML) stands as an important subset of AI, wherein algorithms learn from data without explicit programming. ML encompasses techniques such as linear regression, logistic regression, support vector machines, and decision trees (Mouridsen et al., 2020). It was extensively employed to determine and predict stroke onset time, enhance the efficiency and accuracy of stroke diagnosis, and predict clinical outcomes and risks (Amukotuwa et al., 2019; Heo et al., 2019; Feng et al., 2023; Hong et al., 2023). Cluster #3, deep learning (DL), is an ML approach based on artificial neural networks with multiple layers to learn complex patterns presented in the input data (Karakis et al., 2023). There are two main subtypes of neural networks, among which recurrent neural networks connect nodes that form a directed graph along a time series, while convolutional neural networks calculate spatial relationships among different pixel regions in an image. Various DL methods have been developed for the detection of acute ischemic stroke, intracranial hemorrhage (ICH), and its subtypes (Chilamkurthy et al., 2018; Meijs et al., 2020). Lesion segmentation and quantitative analysis of images are crucial for stroke diagnosis, evaluation, and treatment (Chavva et al., 2022). Clèrigues et al. (2020) illustrated that a 3D patch-based DL approach using the U-Net architecture yielded superior lesion segmentation, facilitating quick quantitative estimation of the extent and location of the penumbra area. Therefore, ML and DL play an increasingly significant role in stroke management with relatively high efficiency, reliability, and repeatability.
Neuroimaging is widely used in stroke research, and the common preferred examination for suspected stroke patients is computed tomography (CT) scanning. It identifies cerebral hemorrhage or ischemia and evaluates the extent and severity of lesions (Sarmento et al., 2020). However, interpreting subtle image changes often relies on the expertise of radiologists, which can vary among practitioners. To address this variability, AI has been applied to quickly and accurately analyze imaging results (Ding et al., 2020). A study processed CT angiography on 477 patients using an automatic detection algorithm for large vessel occlusion, achieving a high diagnostic sensitivity of 94% and a negative predictive value of 98% within 5 min (Amukotuwa et al., 2019). AI enhanced diagnostic efficiency, particularly for patients requiring transfer to comprehensive stroke centers for thrombectomy, thereby mitigating further brain damage and improving prognosis (Leslie-Mazwi and Lev, 2020). The Alberta Stroke Program Early CT Score (ASPECTS) is an approach for assessing the severity of acute ischemic stroke by employing non-contrast CT (Pacchiano et al., 2024). Maegerlein et al. (2019) indicated that ASPECTS assessments calculated by ML-based automatic software tools exhibit greater consistency with predefined consensus standards than those of experienced neuroradiologists in cases of middle cerebral artery occlusion. Furthermore, the software performed better in detecting infarction regions at a 1–4 h interval between symptom onset and imaging, underscoring its potential for early intervention. In summary, AI applications facilitate more prompt and sensitive stroke identification to guide clinical decision-making and improve care.
The primary neurological impairment following a stroke is hemiplegia, which affects the patient’s ability to engage in daily activities and causes inconvenience. Rehabilitation plays a pivotal role in stroke management, facilitating the recovery of impaired functions and enhancing overall quality of life (Hwang and Song, 2023). Cluster #7 Robotic rehabilitation is a research hotspot that encompasses assistive robots and exoskeletons (Rahman et al., 2023). A recent meta-analysis highlighted the significant enhancement of upper extremity motor function and activities of daily living in stroke patients undergoing robot-assisted rehabilitation training (Yang X. et al., 2023). However, a study published in Lancet in 2019 revealed no significant improvements in patients with moderate or severe upper limb functional impairment after stroke when compared with routine care (Rodgers et al., 2019). This may result from potential disparities in patient characteristics and treatment protocols. Singh et al. (2021) demonstrated that robotic hand exoskeleton training improved motor ability and enhanced cortical excitability of the ipsilesional hemisphere in stroke patients, which may be attributed to plastic reorganization and use-dependent plasticity.
Notably, approximately one-third of stroke survivors fail to regain the ability to walk independently, underscoring the significance of the rehabilitation of post-stroke gait impairment (Benjamin et al., 2018; Calabrò et al., 2021). Cluster #8 Robot-assisted gait training is constantly evolving, aiming to enhance limb coordination and neuroplasticity through specific repetitive motor coordination exercises. Studies have indicated the effectiveness of robotic exoskeletons in ameliorating gait disorders among both subacute and chronic stroke survivors (Calafiore et al., 2022; Yang J. et al., 2023). Cluster #11 brain-computer interface (BCI) technology, which directly connects the human brain and external devices, has shown promise in stroke rehabilitation. Zhao et al. (2022) found that 4 weeks of BCI-controlled robot training in subacute stroke patients led to the recovery of motor function in the lower extremities, increased serum BDNF levels, and improved cognitive function. In conclusion, AI has posed great potential in stroke rehabilitation. Further clinical trials are warranted to validate its efficacy and stability, with the ultimate goal of developing personalized and optimized rehabilitation plans.
Through the analysis of citation bursts in keywords and references, we found that stroke outcomes and risk prediction may be emerging research trends in this field. In clinical practice, physicians are often required to provide estimates of patient mortality, the incidence of complications, and the degree of functional dependence to guide care and treatment (Mainali et al., 2021). A retrospective study of 11,775 patients from the Swedish Stroke Register, utilizing logistic regression, revealed that 75.2% of stroke patients had comorbidities, such as atrial fibrillation and hypertension. High comorbidities (≥4) increased mortality rates from 27.3% at 12 months post-stroke to 64.6% at 5 years post-stroke. And patients without comorbidities had mortality rates of 7.3% at 12 months and 19.4% at 5 years post-stroke (Sennfält et al., 2020). After reviewing the prognostic scores of patients with acute ischemic stroke, Matsumoto et al. found that ensemble models of decision trees outperformed linear regression models in predicting adverse functional outcomes (Matsumoto et al., 2020). In terms of ICH, a study utilized machine learning algorithms to evaluate CT imaging in patients with spontaneous ICH, demonstrating high accuracy in predicting functional outcomes (evaluated by the modified Rankin Scale). Importantly, the addition of the ICH score improved predictive efficacy (Nawabi et al., 2021).
Guidelines recommend the use of risk predictive tools to promote stroke prevention, screening, and therapeutic interventions (Meschia et al., 2014). The original Framingham Stroke Risk Profile (FSRP) predicted 10-year stroke risk based on crucial risk factors identified in epidemiological studies. Since then, various predictive models have been developed, such as the QStroke algorithm and the revised FSRP (Wolf et al., 1991; Hippisley-Cox et al., 2013; Dufouil et al., 2017). Lip et al., 2022 conducted a study on a prospective US cohort of 3,435,224 patients, demonstrating that ML-based algorithms could predict stroke risk by analyzing complex relationships among various comorbidities, both cardiovascular and non-cardiovascular. It also provided automated methods for dynamic risk stratification. Given population differences, 10-year stroke risk equations were introduced to assess both 10-year and lifetime stroke risk in Chinese adults, showing superior predictive capability compared to the revised FSRP (Xing et al., 2019). Another study identified age, sex, hypertension, and low-density lipoprotein cholesterol as risk predictors for ischemic stroke, while age, sex, hypertension, body mass index, and high-density lipoprotein cholesterol as important risk factors for hemorrhagic stroke (Yu et al., 2021). As AI technology advances, future research should aim to include diverse populations from broader databases and explore additional predictive factors to enhance prediction accuracy, prevent stroke occurrence and progression, and improve patient prognosis.
Although we have conducted a thorough and systematic bibliometric analysis of the application of AI in stroke, certain limitations exist. Firstly, Web of Science, a highly influential database, serves as a vital resource for academic analysis. It provides information for citation analysis and enables a deeper exploration of the research landscape within the field. However, we only employed this database for analysis, potentially neglecting valuable literature in other databases. Secondly, due to language restrictions, we only analyzed articles in English, and articles in other languages were not included in this analysis.
To our knowledge, this study represents the first comprehensive bibliometric analysis of publications from the past two decades that delves into the knowledge structure and development trajectory of AI applications in stroke. Our findings reveal a notable surge in annual publications, indicating an increasing interest in this topic. The most prolific authors, countries, and institutions are Dukelow, Sean P., China, and the University of Calgary, respectively, making substantial contributions to the advancements of this field. However, there remains a need for enhanced cross-regional and international collaboration to further bolster progress. The application of AI in stroke involves the intersection of multiple disciplines. Therefore, it is necessary to better integrate expertise across various fields to drive rapid advancements in stroke management. Our analysis highlights predominant research hotspots, which include machine learning, deep learning, and AI’s role in stroke rehabilitation and imaging for early identification and diagnosis. Moreover, emerging trends focus on machine learning as well as stroke outcomes and risk prediction. It is foreseeable that AI will increasingly play a crucial role in stroke prevention, treatment, and prognosis. This development is advantageous for optimizing the utilization of medical resources and enhancing the quality of life for patients.
The original contributions presented in the study are included in the article/supplementary material, further inquiries can be directed to the corresponding author.
FX: Conceptualization, Data curation, Formal analysis, Writing – original draft. ZD: Data curation, Formal analysis, Writing – review & editing. YY: Data curation, Formal analysis, Writing – review & editing. PH: Methodology, Supervision, Writing – review & editing. HC: Conceptualization, Funding acquisition, Supervision, Writing – review & editing.
The author(s) declare that financial support was received for the research, authorship, and/or publication of this article. This research was supported by the Anhui Clinical Medical Research Translational Project (202304295107020123) and the Key Scientific Research Project of the Anhui Health Committee (AHWJ2021a020).
The authors declare that the research was conducted in the absence of any commercial or financial relationships that could be construed as a potential conflict of interest.
All claims expressed in this article are solely those of the authors and do not necessarily represent those of their affiliated organizations, or those of the publisher, the editors and the reviewers. Any product that may be evaluated in this article, or claim that may be made by its manufacturer, is not guaranteed or endorsed by the publisher.
Akay, E. M. Z., Hilbert, A., Carlisle, B. G., Madai, V. I., Mutke, M. A., and Frey, D. (2023). Artificial intelligence for clinical decision support in acute ischemic stroke: a systematic review. Stroke 54, 1505–1516. doi: 10.1161/STROKEAHA.122.041442
Albers, G. W., Marks, M. P., Kemp, S., Christensen, S., Tsai, J. P., Ortega-Gutierrez, S., et al. (2018). Thrombectomy for stroke at 6 to 16 hours with selection by perfusion imaging. N. Engl. J. Med. 378, 708–718. doi: 10.1056/NEJMoa1713973
Amukotuwa, S. A., Straka, M., Smith, H., Chandra, R. V., Dehkharghani, S., Fischbein, N. J., et al. (2019). Automated detection of intracranial large vessel occlusions on computed tomography angiography: a single center experience. Stroke 50, 2790–2798. doi: 10.1161/STROKEAHA.119.026259
Benjamin, E. J., Virani, S. S., Callaway, C. W., Chamberlain, A. M., Chang, A. R., Cheng, S., et al. (2018). Heart disease and stroke Statistics-2018 update: a report from the American Heart Association. Circulation 137, e67–e492. doi: 10.1161/CIR.0000000000000558
Bivard, A., Churilov, L., and Parsons, M. (2020). Artificial intelligence for decision support in acute stroke - current roles and potential. Nat. Rev. Neurol. 16, 575–585. doi: 10.1038/s41582-020-0390-y
Brugnara, G., Neuberger, U., Mahmutoglu, M. A., Foltyn, M., Herweh, C., Nagel, S., et al. (2020). Multimodal predictive modeling of endovascular treatment outcome for acute ischemic stroke using machine-learning. Stroke 51, 3541–3551. doi: 10.1161/STROKEAHA.120.030287
Calabrò, R. S., Sorrentino, G., Cassio, A., Mazzoli, D., Andrenelli, E., Bizzarini, E., et al. (2021). Robotic-assisted gait rehabilitation following stroke: a systematic review of current guidelines and practical clinical recommendations. Eur. J. Phys. Rehabil. Med. 57, 460–471. doi: 10.23736/S1973-9087.21.06887-8
Calafiore, D., Negrini, F., Tottoli, N., Ferraro, F., Ozyemisci-Taskiran, O., and de Sire, A. (2022). Efficacy of robotic exoskeleton for gait rehabilitation in patients with subacute stroke: a systematic review. Eur. J. Phys. Rehabil. Med. 58, 1–8. doi: 10.23736/S1973-9087.21.06846-5
Chavva, I. R., Crawford, A. L., Mazurek, M. H., Yuen, M. M., Prabhat, A. M., Payabvash, S., et al. (2022). Deep learning applications for acute stroke management. Ann. Neurol. 92, 574–587. doi: 10.1002/ana.26435
Chen, C. (2006). Cite space II: detecting and visualizing emerging trends and transient patterns in scientific literature. J. Am. Soc. Inf. Sci. Technol. 57, 359–377. doi: 10.1002/asi.20317
Chilamkurthy, S., Ghosh, R., Tanamala, S., Biviji, M., Campeau, N. G., Venugopal, V. K., et al. (2018). Deep learning algorithms for detection of critical findings in head CT scans: a retrospective study. Lancet 392, 2388–2396. doi: 10.1016/S0140-6736(18)31645-3
Chilvers, M. J., Rajashekar, D., Low, T. A., Scott, S. H., and Dukelow, S. P. (2023). Clinical, neuroimaging and robotic measures predict long-term proprioceptive impairments following stroke. Brain Sci. 13:953. doi: 10.3390/brainsci13060953
Clèrigues, A., Valverde, S., Bernal, J., Freixenet, J., Oliver, A., and Lladó, X. (2020). Acute and sub-acute stroke lesion segmentation from multimodal MRI. Comput. Methods Prog. Biomed. 194:105521. doi: 10.1016/j.cmpb.2020.105521
Ding, L., Liu, C., Li, Z., and Wang, Y. (2020). Incorporating artificial intelligence into stroke care and research. Stroke 51, e351–e354. doi: 10.1161/STROKEAHA.120.031295
Dufouil, C., Beiser, A., McLure, L. A., Wolf, P. A., Tzourio, C., Howard, V. J., et al. (2017). Revised Framingham stroke risk profile to reflect temporal trends. Circulation 135, 1145–1159. doi: 10.1161/CIRCULATIONAHA.115.021275
Dukelow, S. P. (2017). The potential power of robotics for upper extremity stroke rehabilitation. Int. J. Stroke 12, 7–8. doi: 10.1177/1747493016654483
Feigin, V. L., and Owolabi, M. O.World Stroke Organization–Lancet Neurology Commission Stroke Collaboration Group (2023). Pragmatic solutions to reduce the global burden of stroke: a world stroke organization-lancet neurology commission. Lancet Neurol. 22, 1160–1206. doi: 10.1016/S1474-4422(23)00277-6
Feng, J., Zhang, Q., Wu, F., Peng, J., Li, Z., and Chen, Z. (2023). The value of applying machine learning in predicting the time of symptom onset in stroke patients: systematic review and Meta-analysis. J. Med. Internet Res. 25:e44895. doi: 10.2196/44895
Heo, J., Yoon, J. G., Park, H., Kim, Y. D., Nam, H. S., and Heo, J. H. (2019). Machine learning-based model for prediction of outcomes in acute stroke. Stroke 50, 1263–1265. doi: 10.1161/STROKEAHA.118.024293
Hippisley-Cox, J., Coupland, C., and Brindle, P. (2013). Derivation and validation of QStroke score for predicting risk of ischaemic stroke in primary care and comparison with other risk scores: a prospective open cohort study. BMJ 346:f2573. doi: 10.1136/bmj.f2573
Hong, C., Pencina, M. J., Wojdyla, D. M., Hall, J. L., Judd, S. E., Cary, M., et al. (2023). Predictive accuracy of stroke risk prediction models across black and white race, sex, and age groups. JAMA 329, 306–317. doi: 10.1001/jama.2022.24683
Hossain, D., Scott, S. H., Cluff, T., and Dukelow, S. P. (2023). The use of machine learning and deep learning techniques to assess proprioceptive impairments of the upper limb after stroke. J. Neuroeng. Rehabil. 20:15. doi: 10.1186/s12984-023-01140-9
Hwang, S., and Song, C.-S. (2023). Driving rehabilitation for stroke patients: a systematic review with Meta-analysis. Healthcare (Basel) 11:1637. doi: 10.3390/healthcare11111637
Jiang, S., Liu, Y., Zheng, H., Zhang, L., Zhao, H., Sang, X., et al. (2023). Evolutionary patterns and research frontiers in neoadjuvant immunotherapy: a bibliometric analysis. Int. J. Surg. 109, 2774–2783. doi: 10.1097/JS9.0000000000000492
Karakis, R., Gurkahraman, K., Mitsis, G. D., and Boudrias, M.-H. (2023). Deep learning prediction of motor performance in stroke individuals using neuroimaging data. J. Biomed. Inform. 141:104357. doi: 10.1016/j.jbi.2023.104357
Keeling, A. B., Piitz, M., Semrau, J. A., Hill, M. D., Scott, S. H., and Dukelow, S. P. (2021). Robot enhanced stroke therapy optimizes rehabilitation (RESTORE): a pilot study. J. Neuroeng. Rehabil. 18:10. doi: 10.1186/s12984-021-00804-8
Kwakkel, G., Kollen, B. J., and Krebs, H. I. (2008). Effects of robot-assisted therapy on upper limb recovery after stroke: a systematic review. Neurorehabil. Neural Repair 22, 111–121. doi: 10.1177/1545968307305457
Leslie-Mazwi, T. M., and Lev, M. H. (2020). Towards artificial intelligence for clinical stroke care. Nat. Rev. Neurol. 16, 5–6. doi: 10.1038/s41582-019-0287-9
Lip, G. Y. H., Genaidy, A., Tran, G., Marroquin, P., Estes, C., and Sloop, S. (2022). Improving stroke risk prediction in the general population: a comparative assessment of common clinical rules, a new multimorbid index, and machine-learning-based algorithms. Thromb. Haemost. 122, 142–150. doi: 10.1055/a-1467-2993
Liu, K., Yin, M., and Cai, Z. (2022). Research and application advances in rehabilitation assessment of stroke. J Zhejiang Univ Sci B 23, 625–641. doi: 10.1631/jzus.B2100999
Lo, A. C., Guarino, P. D., Richards, L. G., Haselkorn, J. K., Wittenberg, G. F., Federman, D. G., et al. (2010). Robot-assisted therapy for long-term upper-limb impairment after stroke. N. Engl. J. Med. 362, 1772–1783. doi: 10.1056/NEJMoa0911341
Maegerlein, C., Fischer, J., Mönch, S., Berndt, M., Wunderlich, S., Seifert, C. L., et al. (2019). Automated calculation of the Alberta stroke program early CT score: feasibility and reliability. Radiology 291, 141–148. doi: 10.1148/radiol.2019181228
Mainali, S., Darsie, M. E., and Smetana, K. S. (2021). Machine learning in action: stroke diagnosis and outcome prediction. Front. Neurol. 12:734345. doi: 10.3389/fneur.2021.734345
Matsumoto, K., Nohara, Y., Soejima, H., Yonehara, T., Nakashima, N., and Kamouchi, M. (2020). Stroke prognostic scores and data-driven prediction of clinical outcomes after acute ischemic stroke. Stroke 51, 1477–1483. doi: 10.1161/STROKEAHA.119.027300
Meijs, M., Meijer, F. J. A., Prokop, M., van Ginneken, B., and Manniesing, R. (2020). Image-level detection of arterial occlusions in 4D-CTA of acute stroke patients using deep learning. Med. Image Anal. 66:101810. doi: 10.1016/j.media.2020.101810
Meschia, J. F., Bushnell, C., Boden-Albala, B., Braun, L. T., Bravata, D. M., Chaturvedi, S., et al. (2014). Guidelines for the primary prevention of stroke: a statement for healthcare professionals from the American Heart Association/American Stroke Association. Stroke 45, 3754–3832. doi: 10.1161/STR.0000000000000046
Moore, R. T., Piitz, M. A., Singh, N., Dukelow, S. P., and Cluff, T. (2022). Assessing impairments in Visuomotor adaptation after stroke. Neurorehabil. Neural Repair 36, 415–425. doi: 10.1177/15459683221095166
Mouridsen, K., Thurner, P., and Zaharchuk, G. (2020). Artificial intelligence applications in stroke. Stroke 51, 2573–2579. doi: 10.1161/STROKEAHA.119.027479
Murray, N. M., Unberath, M., Hager, G. D., and Hui, F. K. (2020). Artificial intelligence to diagnose ischemic stroke and identify large vessel occlusions: a systematic review. J. Neurointerv. Surg. 12, 156–164. doi: 10.1136/neurintsurg-2019-015135
Nawabi, J., Kniep, H., Elsayed, S., Friedrich, C., Sporns, P., Rusche, T., et al. (2021). Imaging-based outcome prediction of acute intracerebral hemorrhage. Transl. Stroke Res. 12, 958–967. doi: 10.1007/s12975-021-00891-8
Nielsen, A., Hansen, M. B., Tietze, A., and Mouridsen, K. (2018). Prediction of tissue outcome and assessment of treatment effect in acute ischemic stroke using deep learning. Stroke 49, 1394–1401. doi: 10.1161/STROKEAHA.117.019740
Nogueira, R. G., Jadhav, A. P., Haussen, D. C., Bonafe, A., Budzik, R. F., Bhuva, P., et al. (2018). Thrombectomy 6 to 24 hours after stroke with a mismatch between deficit and infarct. N. Engl. J. Med. 378, 11–21. doi: 10.1056/NEJMoa1706442
Pacchiano, F., Tortora, M., Criscuolo, S., Jaber, K., Acierno, P., De Simone, M., et al. (2024). Artificial intelligence applied in acute ischemic stroke: from child to elderly. Radiol. Med. 129, 83–92. doi: 10.1007/s11547-023-01735-1
Powers, W. J., Rabinstein, A. A., Ackerson, T., Adeoye, O. M., Bambakidis, N. C., Becker, K., et al. (2018). 2018 guidelines for the early Management of Patients with Acute Ischemic Stroke: a guideline for healthcare professionals from the American Heart Association/American Stroke Association. Stroke 49, e46–e110. doi: 10.1161/STR.0000000000000158
Powers, W. J., Rabinstein, A. A., Ackerson, T., Adeoye, O. M., Bambakidis, N. C., Becker, K., et al. (2019). Guidelines for the early Management of Patients with Acute Ischemic Stroke: 2019 update to the 2018 guidelines for the early Management of Acute Ischemic Stroke: a guideline for healthcare professionals from the American Heart Association/American Stroke Association. Stroke 50, e344–e418. doi: 10.1161/STR.0000000000000211
Rahman, S., Sarker, S., Haque, A. K. M. N., Uttsha, M. M., Islam, M. F., and Deb, S. (2023). AI-driven stroke rehabilitation systems and assessment: a systematic review. IEEE Trans. Neural Syst. Rehabil. Eng. 31, 192–207. doi: 10.1109/TNSRE.2022.3219085
Rodgers, H., Bosomworth, H., Krebs, H. I., van Wijck, F., Howel, D., Wilson, N., et al. (2019). Robot assisted training for the upper limb after stroke (RATULS): a multicentre randomised controlled trial. Lancet 394, 51–62. doi: 10.1016/S0140-6736(19)31055-4
Saini, V., Guada, L., and Yavagal, D. R. (2021). Global epidemiology of stroke and access to acute ischemic stroke interventions. Neurology 97, S6–S16. doi: 10.1212/WNL.0000000000012781
Sak, J., and Suchodolska, M. (2021). Artificial intelligence in nutrients science research: a review. Nutrients 13:322. doi: 10.3390/nu13020322
Sarmento, R. M., Vasconcelos, F. F. X., Filho, P. P. R., Wu, W., and de Albuquerque, V. H. C. (2020). Automatic Neuroimage processing and analysis in stroke-a systematic review. IEEE Rev. Biomed. Eng. 13, 130–155. doi: 10.1109/RBME.2019.2934500
Sennfält, S., Pihlsgård, M., Petersson, J., Norrving, B., and Ullberg, T. (2020). Long-term outcome after ischemic stroke in relation to comorbidity - an observational study from the Swedish stroke register (Riksstroke). Eur. Stroke J. 5, 36–46. doi: 10.1177/2396987319883154
Singh, N., Saini, M., Kumar, N., Srivastava, M. V. P., and Mehndiratta, A. (2021). Evidence of neuroplasticity with robotic hand exoskeleton for post-stroke rehabilitation: a randomized controlled trial. J. Neuroeng. Rehabil. 18:76. doi: 10.1186/s12984-021-00867-7
Song, Y., Liu, J., Zong, C., Zhang, F., Ren, Y., Ching, Y., et al. (2024). A bibliometric study on trends in chiropractic research from 1920 to 2023. Complement. Ther. Med. 82:103038. doi: 10.1016/j.ctim.2024.103038
Tu, W., and Wang, L.Special Writing Group of China Stroke Surveillance Report (2023). China stroke surveillance report 2021. Mil. Med. Res. 10:33. doi: 10.1186/s40779-023-00463-x
van Eck, N. J., and Waltman, L. (2010). Software survey: VOSviewer, a computer program for bibliometric mapping. Scientometrics 84, 523–538. doi: 10.1007/s11192-009-0146-3
Veerbeek, J. M., Langbroek-Amersfoort, A. C., van Wegen, E. E. H., Meskers, C. G. M., and Kwakkel, G. (2017). Effects of robot-assisted therapy for the upper limb after stroke. Neurorehabil. Neural Repair 31, 107–121. doi: 10.1177/1545968316666957
Wolf, P. A., D’Agostino, R. B., Belanger, A. J., and Kannel, W. B. (1991). Probability of stroke: a risk profile from the Framingham study. Stroke 22, 312–318. doi: 10.1161/01.str.22.3.312
Xing, X., Yang, X., Liu, F., Li, J., Chen, J., Liu, X., et al. (2019). Predicting 10-year and lifetime stroke risk in Chinese population. Stroke 50, 2371–2378. doi: 10.1161/STROKEAHA.119.025553
Yang, J., Gong, Y., Yu, L., Peng, L., Cui, Y., and Huang, H. (2023). Effect of exoskeleton robot-assisted training on gait function in chronic stroke survivors: a systematic review of randomised controlled trials. BMJ Open 13:e074481. doi: 10.1136/bmjopen-2023-074481
Yang, Z., Hotterbeex, P., Marent, P.-J., Cerin, E., Thomis, M., and van Uffelen, J. (2024). Physical activity, sedentary behaviour, and cognitive function among older adults: a bibliometric analysis from 2004 to 2024. Ageing Res. Rev. 97:102283. doi: 10.1016/j.arr.2024.102283
Yang, X., Shi, X., Xue, X., and Deng, Z. (2023). Efficacy of robot-assisted training on rehabilitation of upper limb function in patients with stroke: a systematic review and Meta-analysis. Arch. Phys. Med. Rehabil. 104, 1498–1513. doi: 10.1016/j.apmr.2023.02.004
Yedavalli, V. S., Tong, E., Martin, D., Yeom, K. W., and Forkert, N. D. (2021). Artificial intelligence in stroke imaging: current and future perspectives. Clin. Imaging 69, 246–254. doi: 10.1016/j.clinimag.2020.09.005
Yu, Q., Wu, Y., Jin, Q., Chen, Y., Lin, Q., and Liu, X. (2021). Development and internal validation of a multivariable prediction model for 6-year risk of stroke: a cohort study in middle-aged and elderly Chinese population. BMJ Open 11:e048734. doi: 10.1136/bmjopen-2021-048734
Keywords: artificial intelligence, stroke, machine learning, bibliometric analysis, VOSviewer, CiteSpace
Citation: Xu F, Dai Z, Ye Y, Hu P and Cheng H (2024) Bibliometric and visualized analysis of the application of artificial intelligence in stroke. Front. Neurosci. 18:1411538. doi: 10.3389/fnins.2024.1411538
Received: 03 April 2024; Accepted: 29 August 2024;
Published: 11 September 2024.
Edited by:
Tarun Singh, University of Michigan, United StatesReviewed by:
Flavie Bompaire, Hôpital d’Instruction des Armées Percy, FranceCopyright © 2024 Xu, Dai, Ye, Hu and Cheng. This is an open-access article distributed under the terms of the Creative Commons Attribution License (CC BY). The use, distribution or reproduction in other forums is permitted, provided the original author(s) and the copyright owner(s) are credited and that the original publication in this journal is cited, in accordance with accepted academic practice. No use, distribution or reproduction is permitted which does not comply with these terms.
*Correspondence: Hongliang Cheng, Y2hsLjc1ODExQDE2My5jb20=; Peijia Hu, emp5eWhwakAxNjMuY29t
Disclaimer: All claims expressed in this article are solely those of the authors and do not necessarily represent those of their affiliated organizations, or those of the publisher, the editors and the reviewers. Any product that may be evaluated in this article or claim that may be made by its manufacturer is not guaranteed or endorsed by the publisher.
Research integrity at Frontiers
Learn more about the work of our research integrity team to safeguard the quality of each article we publish.