- 1Department of Rehabilitation Medicine, The Affiliated Suzhou Hospital of Nanjing Medical University, Suzhou, China
- 2The Fourth Rehabilitation Hospital of Shanghai, Shanghai, China
Background: Parkinson’s disease (PD) is a prevalent neurodegenerative disorder affecting millions globally. It encompasses both motor and non-motor symptoms, with a notable impact on patients’ quality of life. Electroencephalogram (EEG) is a non-invasive tool that is increasingly utilized to investigate neural mechanisms in PD, identify early diagnostic markers, and assess therapeutic responses.
Methods: The data were sourced from the Science Citation Index Expanded within the Web of Science Core Collection database, focusing on publications related to EEG research in PD from 2004 to 2023. A comprehensive bibliometric analysis was conducted using CiteSpace and VOSviewer software. The analysis began with an evaluation of the selected publications, identifying leading countries, institutions, authors, and journals, as well as co-cited references, to summarize the current state of EEG research in PD. Keywords are employed to identify research topics that are currently of interest in this field through the analysis of high-frequency keyword co-occurrence and cluster analysis. Finally, burst keywords were identified to uncover emerging trends and research frontiers in the field, highlighting shifts in interest and identifying future research directions.
Results: A total of 1,559 publications on EEG research in PD were identified. The United States, Germany, and England have made notable contributions to the field. The University of London is the leading institution in terms of publication output, with the University of California closely following. The most prolific authors are Brown P, Fuhr P, and Stam C In terms of total citations and per-article citations, Stam C has the highest number of citations, while Brown P has the highest H-index. In terms of the total number of publications, Clinical Neurophysiology is the leading journal, while Brain is the most highly cited. The most frequently cited articles pertain to software toolboxes for EEG analysis, neural oscillations, and PD pathophysiology. Through analyzing the keywords, four research hotspots were identified: research on the neural oscillations and connectivity, research on the innovations in EEG Analysis, impact of therapies on EEG, and research on cognitive and emotional assessments.
Conclusion: This bibliometric analysis demonstrates a growing global interest in EEG research in PD. The investigation of neural oscillations and connectivity remains a primary focus of research. The application of machine learning, deep learning, and task analysis techniques offers promising avenues for future research in EEG and PD, suggesting the potential for advancements in this field. This study offers valuable insights into the major research trends, influential contributors, and evolving themes in this field, providing a roadmap for future exploration.
1 Introduction
Parkinson’s disease (PD) is the second most common central neurodegenerative disorder globally. It primarily affects the nigrostriatal dopaminergic system and is accompanied by degeneration of other non-dopaminergic neural circuits (Hayes, 2019). The primary clinical manifestations of this disease encompass both motor and non-motor symptoms, which significantly impact the quality of life and survival time of patients (Reich and Savitt, 2019). The diagnosis of dyskinesia is primarily based on clinical assessment, which involves the identification of characteristic motor symptoms such as bradykinesia, tremor, rigidity, postural instability, and gait disturbances (Bloem et al., 2021). The integration of genetic markers with imaging techniques, such as MRI and DAT-SPECT, facilitates the differentiation of PD from other comparable movement disorders. Moreover, non-motor symptoms like decreased olfaction and rapid eye movement sleep behavior disorder present diagnostic value in early-stage PD. Electroencephalography (EEG) is a pivotal non-invasive methodology for evaluating cerebral activity with high temporal resolution, enabling the capture of rapid dynamic alterations in cerebral processes (Waninger et al., 2020). It is particularly suitable for studying neural oscillations, brain network connections, and the effects of various stimuli or cognitive tasks on brain function. In addition, in comparison to MRI and DAT-SPECT, EEG is relatively cost-effective, straightforward to utilize, and applicable to a diverse range of clinical and research settings. These properties render EEG an invaluable tool for basic neuroscience research and clinical diagnosis. In PD, motor control is critically dependent on the synchronization and functional connectivity among cortical regions, which are substantially influenced by the dynamics within the nigrostriatal-thalamic circuitry (Lalo et al., 2008). This implies that EEG can be employed as a non-invasive and dynamic instrument for the detection of synchronization and functional connectivity between cortical areas in PD patients, with the objective of monitoring treatment response and potential disease progression. Bibliometrics analysis represents a novel approach to research outcome statistics, furnishing researchers with qualitative and quantitative insights into literature characteristics through the analysis of variables including countries, institutions, authors, journals, references and keywords (Durieux and Gevenois, 2010). The predominant bibliometric software, such as VOSviewer and CiteSpace, facilitates the visualization of extensive publication datasets. Bibliometric analysis also helps to identify leading authors, prolific research institutions, and influential and high-quality publications, which can be used to develop guidelines, pinpoint research hotspots, and predict research trends (Chen et al., 2024). To date, bibliometric studies focusing on EEG in PD have not been reported in the literature. Consequently, this research employs bibliometric analysis tools to delineate and track the evolution of research hotspots in this field from 2004 to 2023. Furthermore, it outlines prospective avenues for future investigation.
2 Materials and methods
2.1 Data source and collection
The data for the bibliometric analysis were obtained from the Science Citation Index Expanded (SCI-Expanded) within the Web of Science Core Collection database (WoSCC). The data retrieval strategy was summarized as follows: #1:TS = (“Parkinson Disease” OR Parkinson* OR “Idiopathic Parkinson’s Disease” OR “Lewy Body Parkinson’s Disease” OR “Parkinson’s Disease, Lewy Body” OR “Parkinson Disease, Idiopathic” OR “Parkinson’s Disease, Idiopathic” OR “Idiopathic Parkinson Disease” OR “Lewy Body Parkinson Disease” OR “Parkinsonism, Primary” OR “Primary Parkinsonism” OR “Paralysis Agitans”); #2:TS = (Electroencephalography OR EEG OR Electroencephalogram∗); the ultimate dataset: #1 AND #2. The use of a truncation symbol “∗” prevented missed detections and improved retrieval effects. The study included only English-language studies. The time of search period was between 1 January 2004 and 31 December 2023. The search strategy is depicted in Figure 1. In order to minimize the potential for bias resulting from routine database updates, the literature search was conducted on a specific date. A total of 1,559 documents were retrieved in the search, including reviews and articles. Upon completion of the retrieval process, the data were saved as complete records and cited references. Articles were extracted and exported in either “Plain text file” or “Tab-delimited file” formats.
2.2 Bibliometric analysis
The literature that met the inclusion criteria was exported as a plain text file named “download_xxx.txt,” which comprised full records and cited references. Subsequently, the file was imported into VOSviewer 1.6.19 and CiteSpace 6.2.R2 software in order to construct knowledge maps and perform statistical analysis. Concurrently, the documents were exported in tab-delimited file formats and uploaded to an online analysis platform for document metrology, with the objective of generating a knowledge map of national/regional collaborative networks. VOSviewer software was employed with the following parameter settings: association strength was selected as the normalization method, and minimum thresholds for country/region, institution, and author were set at 5, 10, and 6, respectively, based on publication counts. Additionally, the minimum thresholds of 100 citations were set for authors, journals, and literature. Furthermore, the frequency of occurrence for keywords was considered, with a minimum threshold of 10. The CiteSpace software was configured with the following parameter settings: the time span was set from January 2004 to December 2023, with a time slice of 1 year. Node types were set to include keyword and reference, and the selection criteria were established to identify the g-index k = 25 for each slice. The pruning options employed included pathfinder, sliced networks pruning, and merged network pruning, while all other settings were retained at their default values. In this study, we initially analyze the quantity of literature, countries, institutions, authors, journals and co-cited references to summarize the current research status of EEG in PD. Subsequently, we employ co-occurrence and cluster map of keywords to identify research hotspots. Finally, we explore research frontiers and trends in this field by examining burst keywords.
3 Results
3.1 Annual publications and citations
The study encompassed 1,559 related publications, comprising 1,354 original research articles (87%) and 205 review articles (13%). The total citations (TC) were 33,318. The average citation per publication (ACPP) was 31.25, and the h-index was 96. The dual-axis trend analysis in Figure 2 spanned 20 years, from 2004 to 2023, with time depicted on the horizontal axis. The left vertical axis denotes the number of publications, while the right vertical axis corresponds to the citation frequency. The bar graph in Figure 2 depicts the annual publication volume, which exhibits a clear upward trend over the period. The number of publications increased from 16 in 2004 to 175 in 2022, representing an almost elevenfold increase. The considerable expansion in the volume of publications is indicative of the sustained growth in interest and scholarly output in this field. The line graph depicted the citation frequency, rising from 12 in 2004 to 6,170 by 2023, signifying a remarkable increase of over 500 times. The upward trend in citation frequency became particularly evident around 2018, suggesting a significant period of growth. The interaction between the two metrics within the chart reflects the symbiotic relationship between the research output and its subsequent impact. Initially, the growth in both the number of publications and the frequency of citations was gradual, indicating a process of gradual accumulation and recognition of knowledge within the domain. The recent surge in citation numbers may reflect pioneering research breakthroughs gaining traction, indicating an increasing integration into the broader scientific discourse. Alternatively, it may signify the maturation of the domain, with earlier research findings being expanded upon with renewed vigor.
3.2 Distribution of countries/regions and institutions
This study examined 1,559 articles produced by 2,114 institutions across 75 different countries. Among these, 218 institutions and 50 countries had published more than 5 publications in this field. As illustrated in Table 1, the United States, Germany, England, and Italy are the primary contributors of research, with the United States accounting for 26.62% (415 publications), Germany for 15.19% (412 publications), England for 12.44% (194 publications), and Italy for 11.15% (174 publications). The United States also leads in TC, h-index, and centrality, which serves to underscore its clear leadership in this field. It is noteworthy that the Netherlands has the highest ACPP despite not ranking in the top 5 for publication volume, which signifies its academic influence and high research quality. Figure 3A maps the global collaboration networks between different countries and regions, with different colored blocks representing each country or region. The size of the blocks reflects the number of articles published, while the connecting lines represent the strength of collaboration. Thicker lines indicate a stronger relationship. The United States, England, and Italy have actively collaborated with other countries, forming the core of the global research network (Lyu et al., 2024). Figure 3B illustrates that each node represents a country or region, and the lines between the nodes indicate the presence of a cooperative relationship. The thickness of the lines reflects the closeness of the collaboration. Nodes that are blue-purple indicate an earlier publication date, while yellow-green indicates a more recent publication date. Figure 3C shows that in the early timeline, a few dominant countries, represented by a limited variety of colors, contributed the majority of publications (Zhao et al., 2023). Over time, the bars have become more diverse and evenly distributed among nations, indicating a significant increase in global participation. By 2021, China had ascended to second place behind the United States in publication count, demonstrating a substantial increase in research investment and output in recent years.
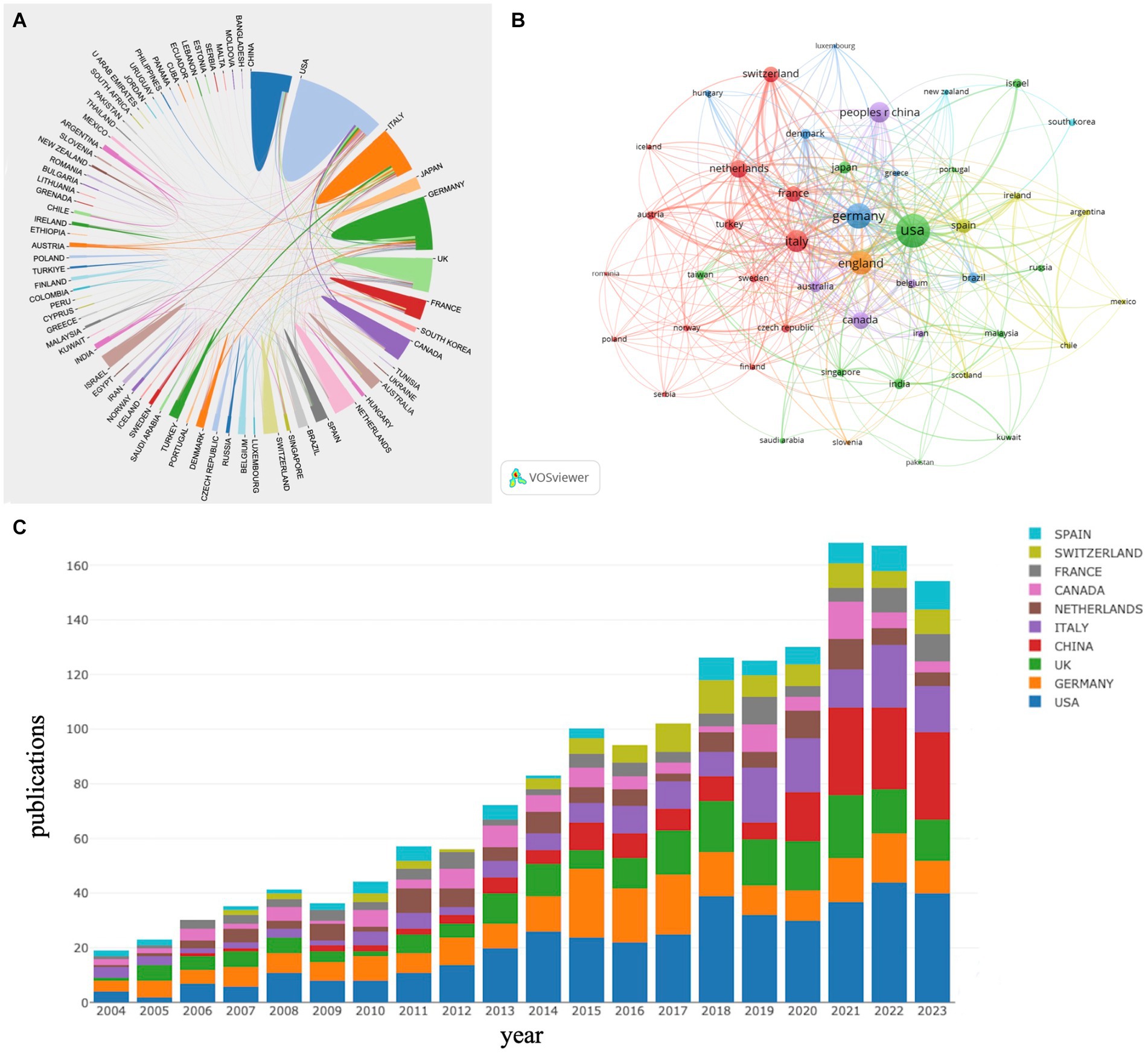
Figure 3. (A) National/regional collaborative network knowledge map. (B) Temporal map of national/regional cooperation network. (C) Stack bar plot of top 10 countries/regions in publication.
Figure 4 presents the cooperation visualization network map of institutions, created using VOSviewer, depicting collaborative relationships among institutions that have published over 10 publications in the field. The network comprises a total of 75 institutions, which have been categorized into five clusters based on the extent of their collaboration. Table 2 presents a summary of the top 10 institutions based on the number of publications. A total of 472 articles were published by these institutions, representing 30.28% of the total number of documents included in the study. In terms of the total number of publications, the University of London and the University of California have the highest number of publications. With regard to TC, the University of London is at the vanguard of this field with the highest TC (6,216), followed by the Vrije University Amsterdam in second place with TC (5,028). Among the top 10 institutions, three are from England, while the remainder are situated in the US, France, the Netherlands, Germany, and Canada. It is notable that the Vrije University Amsterdam has the highest ACPP, ranking second only to the University of London in total citations and h-index.
3.3 Analysis of authors
A total of 6,635 authors have contributed to this research area. Table 3 presents the top 10 authors, who collectively produced 280 publications, representing approximately 17.96% of the total output. Among the most prolific researchers are Brown P, Fuhr P, and Stam C, each of whom contributed 31 publications. Notably, Stam C holds the highest TC and ACPP in this field, while Brown P has the highest h-index and total link strength (TLS), which serves to highlight his substantial influence. Interestingly, although Berendse H is not among the top 5 in publication count, he ranks third for both TC and ACPP, which serves to demonstrate the significant reach and impact of his research. This reflects the profound influence of his work within the scholarly community. Figures 5A,B present a collaborative network and a time overlay graph of authors who have published 6 or more articles. They reveal that collaborative efforts are mainly concentrated among high-impact scholars who work closely within their groups. However, direct collaborations among high-impact scholars are limited.
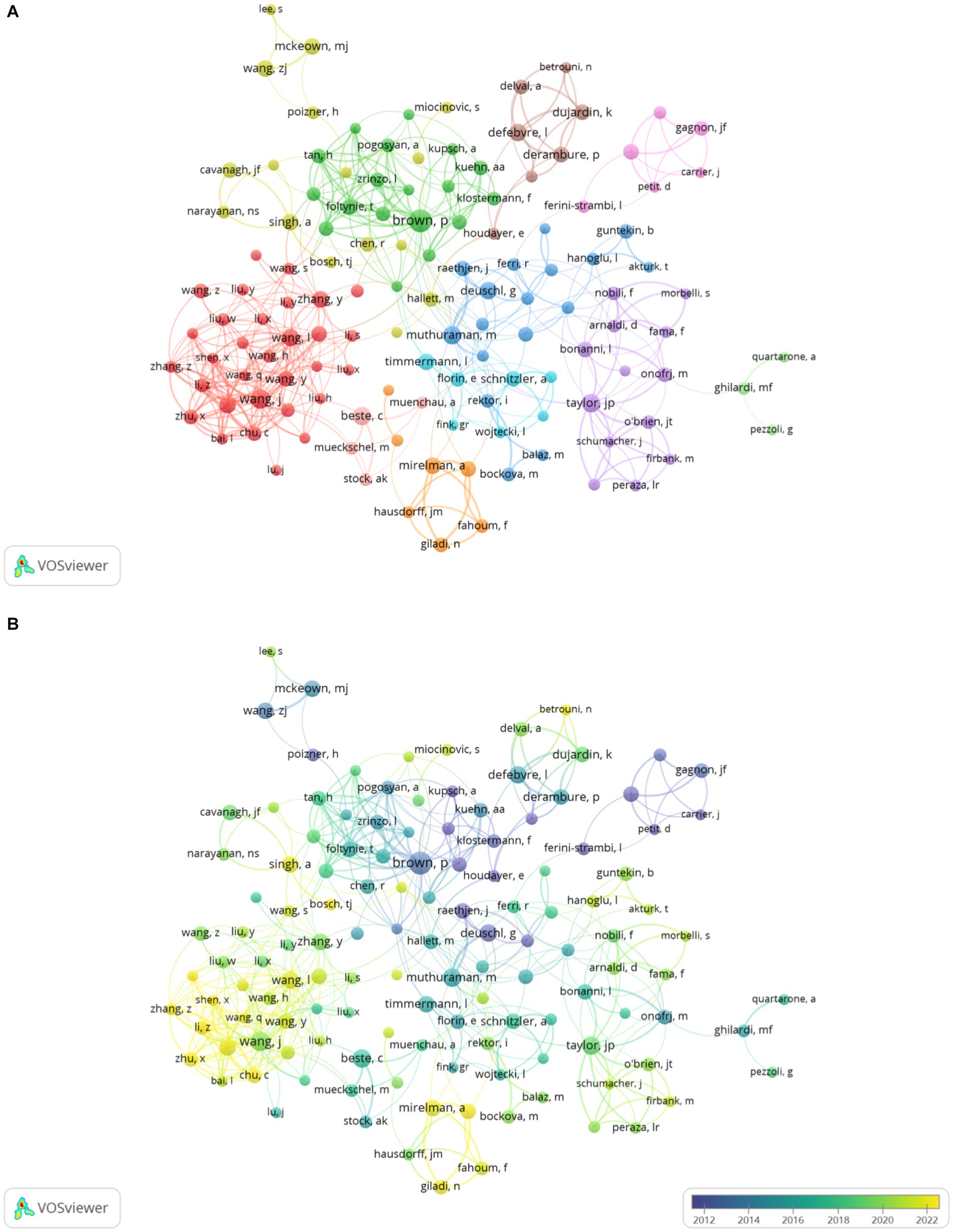
Figure 5. (A) Collaborative network knowledge map of author. (B) Temporal map of author’s collaborative network.
3.4 Analysis of journals
The articles included in this study have been published in 390 different academic journals. Table 4 illustrates that the most prolific journal is Clinical Neurophysiology, which has published 95 publications (representing 6.09% of the total), followed by Frontiers in Movement Disorders (52 publications, 3.33%), and Neuroimage (42 publications, 2.69%). The journal with the highest number of publications is Clinical Neurophysiology, while Brain has the highest ACPP, the highest H-index, and the highest impact factor (IF), indicating that it is of a high quality and authoritative in the field of study. All of the journals are classified as either Q1 or Q2, which indicates that they are of a high caliber and have a significant academic impact. Figure 6 depicts the co-citation relationships among the journals. Brain leads with the highest TC and TLS, followed by Neuroimage and Clinical Neurophysiology, as shown in Table 5. Figure 7 depicts the citation relationships among the journals. The journals on the right are cited by those on the left (Guo et al., 2023; He et al., 2023; Li F. et al., 2023). The citation relationships are indicated by colored paths between the citing and cited journals. A two-color primary citation pathway was identified through mapping. This indicates that research published in journals within the field of molecular biology and genetics is primarily cited by research published in molecular biology and immunology. Furthermore, research published in psychology, education, and social sciences is primarily cited by studies published in neurology, sports, and ophthalmology journals.
3.5 Analysis of references
Figure 8A presents the co-citation networks of publications that have been cited at least 50 times, offering a clear representation of the co-citation relationships among these articles. Table 6 provides a detailed overview of the top 10 most frequently cited publications, which serve to highlight the major research themes in this field. Three of the articles in question delve into software, toolboxes, and the core aspects of EEG processing. Two articles examine the characteristics of disease and the physiological mechanisms associated with PD. Two further articles, authored by Brown P., examine neural oscillations within cortical and basal ganglia circuits in PD. The remaining two articles address topics related to cognition. Citation bursts are defined as references that are cited frequently over time. Identifying these bursts can highlight the most active research areas within a specific time period, based on the subjects of the references (Li Z. et al., 2023). In this study, the timeline is represented by a blue line, with the periods during which citation bursts were investigated marked in red on this line. Figure 8B presents the top 25 articles exhibiting the most pronounced citation bursts. The research with the strongest citation bursts (strength: 13.78) commenced in 2020 and is focused on using a deep learning method to diagnose PD through EEG signals (Oh et al., 2020). It is noteworthy that 7 articles are currently experiencing bursts. These studies in question primarily analyze the spectral characteristics of PD with a view to supporting the diagnostic process.
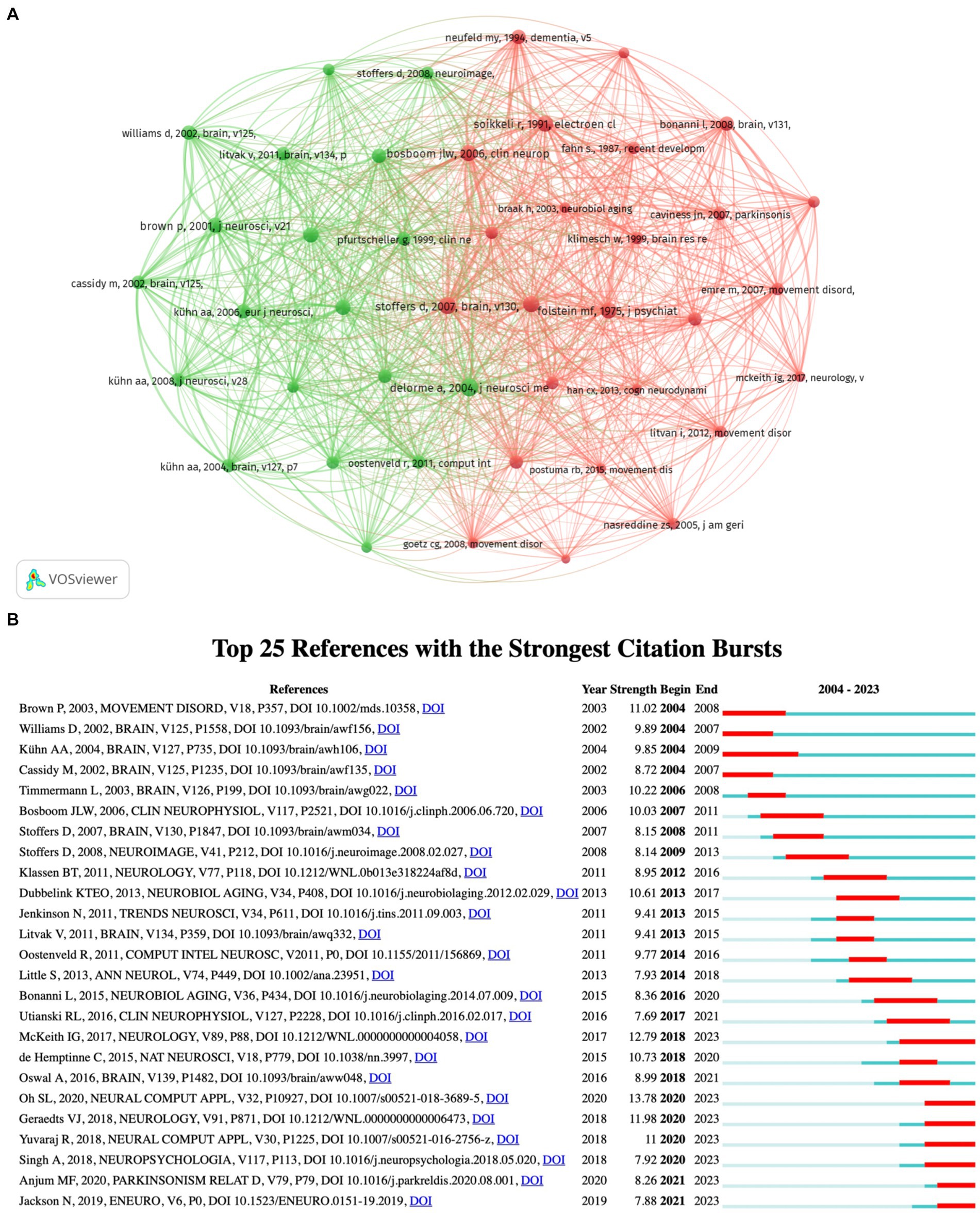
Figure 8. (A) Literature co-citation network knowledge map. (B) The top 25 references with the highest burst strength.
3.6 Analysis of keywords
Keywords are concise summaries of the research content of an article. The analysis of high-frequency keywords can reveal the main research topics in a given field. Figure 9A presents the co-occurrence relationships among keywords with at least 10 occurrences. The node size is directly proportional to the frequency of occurrence, and the thickness of the connecting lines correlates with how often the keywords co-occurrence at both ends. Different color clusters reflect the cooperative relationships among keywords. Table 7 lists the top 40 high-frequency keywords and their TLS, providing insights into the primary research topics and emerging trends in this field. Figure 9B presents a visualization cluster map of co-cited references created with CiteSpace. The modularity Q value of 0.7504 and the mean silhouette value of 0.8789 provide evidence of the clustering’s validity, as both values exceed 0.5(Tao et al., 2023). Each circle represents a keyword, and circles of the same color group together around a shared theme, revealing 24 representative clusters. By setting the duration to 1 year, the top 25 keywords with the highest burst intensity were identified and shown in Figure 9C. The keyword “L-dopa” appeared first, “cerebral-cortex” had the longest burst, and keywords like “machine learning,” “deep learning,” “prediction,” and “nonmotor symptoms” are still experiencing bursts. Analyzing these bursts helps identify research hotspots and trends for future development in this field. Particularly, keywords with ongoing bursts offer valuable insights and guidance for further research.
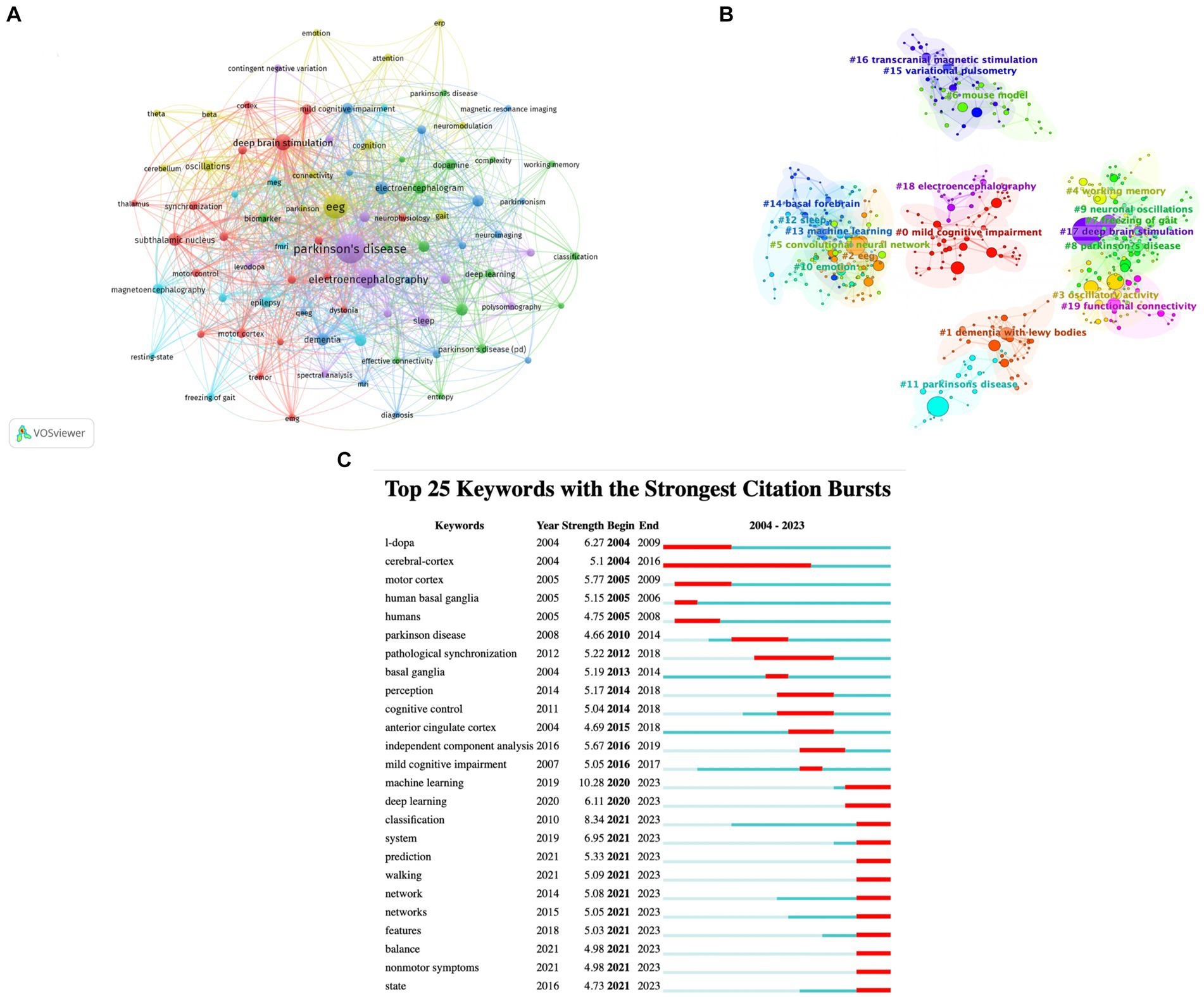
Figure 9. (A) Keyword co-occurrence network knowledge map. (B) Cluster map of keywords. (C) The top 25 keywords with the highest burst strength.
4 Discussion
The landscape of EEG research in PD has experienced a notable surge in academic output from 2004 to 2023. This surge is marked by a notable rise in publications since 2020. The sustained annual publication of over 100 articles during 2021–2023 reflects the growing and dynamic interest in this field. This trend may be attributed to the increasing recognition of PD’s complexity and the potential of EEG as a valuable tool for diagnosis and monitoring, which has spurred extensive research efforts.
In the study of PD through EEG research, certain countries have emerged as early and influential contributors. Germany, the Netherlands, and Canada not only have an earlier average publication year but were also instrumental during the early stages of this field, providing a strong foundation for ongoing studies. The United States, England, and Germany show high centrality and h-index scores, demonstrating their significant roles within the global research community and their contribution to fostering academic partnerships. Notably, these three countries are at the heart of major international collaborative networks. The United States leads an extensive network, engaging with countries such as India, Singapore, Japan, and Israel. Similarly, England spearheads another influential network, partnering with Australia, Italy, the Netherlands, France, and Turkey. Germany plays a pivotal role in a strong collaborative framework involving Denmark, Brazil, Greece, and Hungary. These networks exemplify strategic international alliances that significantly contribute to global research initiatives. Despite ranking sixth in the total number of publications, the Netherlands has the highest ACPP, indicating the broad recognition and impact of its research. This suggests that academic achievements from the Netherlands are frequently cited and highly valued in the scholarly community. Following 2020, China’s research output has rapidly increased, positioning it as one of the largest publishing countries. However, due to its relatively late entry into the field, its global impact remains lower compared to other leading nations. This discrepancy underscores the importance of balancing research quantity with quality and global impact. The 10 countries with the highest number of publications in this field are primarily located in Europe, with two of them in North America. This distribution reflects the prominence of these countries in this field. The extent of research into PD at the national or regional level does not appear to be correlated with the prevalence of PD in a given region (Dammertz et al., 2023; Zheng et al., 2023). This suggests that economic, technological, and other factors exert a more pronounced influence on research productivity.
The University of London is the most prolific institution in the field, with the highest TC and H-index, and has made a significant impact on this field. One of their highly cited research articles investigated the impact of inter-regional cortical synchronization on PD pathophysiology and assessed how dopaminergic therapy and subthalamic nucleus (STN) high-frequency stimulation influence these dynamics. EEG recordings from patients with PD demonstrated a relationship between the 10–35 Hz coherence range and the severity of the disease. Both L-dopa and STN stimulation were found to reduce this cortical coupling, which corresponded with clinical improvements. This implies that these treatments may help restore normal cortical interactions, potentially explaining their similar therapeutic effects in PD management (Silberstein et al., 2005). After the University of London, the University of California is the second-most prolific institution in this field. One of their highly cited articles investigated the deep brain stimulation (DBS) on response inhibition in the STN of PD patients. Using scalp EEG, the researchers observed an increase in beta-band power in a stopping task when the STN was stimulated. Patients with DBS exhibited a faster response time when stimulation was active compared to when it was turned off. This effect was more pronounced in the right frontal cortex, suggesting enhanced messaging within the frontal-basal ganglia circuit (Swann et al., 2011). The number of publications produced by Vrije University Amsterdam is not particularly high, but they are ranked first in the number of citations to their articles. This reflects the high quality and wide recognition of their academic output, which holds significant academic influence in this field. In a previous published review article, they discussed how the brain communication organization is a complex network under normal conditions and how it deteriorates in neurological diseases. The review underscores the significance of contemporary network theory and sophisticated imaging techniques, such as high-density EEG and magnetoencephalography (MEG), in elucidating the intricacies of these networks. The healthy brain is characterized by the formation of “small-world networks” with optimal local and long-distance connectivity, which are vital for cognitive and intellectual functions (Stam and van Straaten, 2012). It is evident that the number of publications does not always correlate with citation metrics or the spread of research influence. For instance, some institutions with fewer publications have exhibited a high ACPP, which indicates the profound reach and recognition of their research. This suggests that while quantity is a factor, the quality and impact of research are of significant importance in determining an institution’s standing in the global research community.
Professor Brown P of Oxford University has the greatest number of published articles, H-index, and TLS, and has a significant impact on this field. His team conducted a series of studies elucidating the role of abnormal brain rhythms, particularly beta oscillations, in the basal ganglia circuits of PD patients. These abnormal oscillations are associated with the rigidity and bradykinesia characteristic of PD (Mallet et al., 2008; Litvak et al., 2011; Little et al., 2012). Furthermore, these oscillations can be influenced by treatments such as DBS and medication (Fischer et al., 2019; Muthuraman et al., 2020). They have employed EEG extensively to study these oscillatory activities, thereby providing crucial insights into their role in PD pathology and treatment efficacy. Professor Stam C from Vrije University Amsterdam is also a high-impact scholar. He is not only one of the most prolific authors in this field of PD research, but also the author with the highest TC and ACPP. His contributions have revolved around elucidating the complex patterns of brain activity associated with PD and exploring the potential for EEG as a tool in the diagnosis and understanding of the disease. He has significantly impacted the field of PD research by employing EEG analysis through the lens of nonlinear dynamical systems (Stam, 2005; Stam and van Straaten, 2012). These studies are of critical importance for the development of more effective diagnostic tools and targeted treatment strategies for PD. EEG-based biomarkers have the potential to be utilized for early diagnosis and for monitoring disease progression. It is noteworthy that a team from Tianjin University, including Zhu X, Chun C, and Liu C, among others, has been active in recent years. In their latest study, they explored the temporal and spectral variations in EEG as manifestations of cognitive impairment. By recruiting early PD patients and conducting microstate analysis in specific frequency bands identified through deep learning, they identify distinctive EEG patterns correlated with cognitive assessment scales (Liu et al., 2023). This study uncovers abnormal microstate characteristics in early PD with mild cognitive impairment (MCI) and offers potential electrophysiological markers for the early recognition of MCI in PD.
An examination of the number of journal publications and their co-citations can provide valuable insights that can assist with the selection of appropriate journals for manuscript submission. The investigation into the EEG research of PD is well-represented across a range of journals, with a concentrated core of journals that are particularly influential in this field. Among the top 10 journals in this field, Clinical Neurophysiology, Movement Disorders, and Neuroimaging are the three most prolific. These three journals have high TC, TLS and IF, with each classified as Q1. It is therefore recommended that new articles be submitted to these journals. In contrast, Brain has the highest IF, TC, and TLS among co-cited journals, functioning as central nodes in the network of scholarly communication. It is closely followed by other highly-cited journals such as Neuroimaging, Clinical Neurophysiology, Movement Disorders, and Neurology. These journals serve not only as platforms for disseminating new discoveries but also as cornerstones in constructing the academic narrative within the field. While the number of publications is a metric that can be used to assess the influence of a journal, co-citation data provides a more accurate reflection of the dissemination and recognition of research within and beyond the field. Furthermore, it is noteworthy that the annual publication of Frontiers in Neurology has experienced a notable surge, commencing in 2019. In a broader context, this bibliometric analysis informs researchers of the most appropriate journals in which to submit their manuscripts for potential publication and serves as a guide for those seeking to keep abreast of leading-edge developments. It is of the utmost importance for researchers to contribute novel findings and engage with the broader academic community through these influential platforms in order to ensure the dissemination and impact of their work.
The significance of an article within a research domain is often determined by the frequency of its citations. The top 10 cited publications included two reviews, six research articles and two editorials. These can be classified into distinct research directions, with EEG studies forming a primary category. Among these, a highly cited article by (Delorme and Makeig, 2004) introduced EEGLAB, an open-source MATLAB toolbox tailored for analyzing single-trial EEG dynamics, inclusive of independent component analysis. EEGLAB is a comprehensive tool for EEG data processing that offers robust capabilities for data importation, visualization, preprocessing, and analysis. It is accessible to both newcomers and seasoned experts in the field. In addition, a noteworthy publication by (Oostenveld et al., 2011) describes FieldTrip, another open-source MATLAB toolbox designed for the analysis of MEG, EEG, and invasive electrophysiological data. FieldTrip encompasses a range of functionalities, from preprocessing to statistical analysis, thereby supporting a diverse spectrum of neuroelectrophysiological research. These software tools have significantly advanced the field of brain neuroscience, catalysing the progress of research by enabling the detailed examination of complex electrophysiological data. The second category delves into the physiological and pathological underpinnings of PD. Hoehn and Yahr (1967) compiled data on 802 patients observed from 1949 to 1964, aiming to understand the variability and progression of the disease across a large group (Brown et al., 2001; Brown, 2003) examining the impact of dopaminergic modulation and motor tasks on neuronal discharge frequency and synchronization within the PD-affected circuits. The researchers identified specific oscillation frequencies, particularly in the beta-band and gamma-band, that are associated with motor functions. This suggests that these oscillations may have functional significance in the pathology and treatment of PD. Moreover, the researchers discovered that levodopa treatment reduced low-frequency activity while enhancing higher-frequency synchronization, indicating that dopamine plays an intricate role in regulating neural activity within the basal ganglia. The third category of research focuses on the application of EEG in the field of PD research. One study by Soikkeli et al. (1991) investigated mid-frontal theta activity during cognitive control tasks in PD patients and found a reduced modulation compared to healthy controls. The researchers examined EEG abnormalities in PD patients, with a focus on the differences between those with and without dementia. A general slowing of EEG signals was observed in PD patients, with the most marked changes occurring in those with dementia, as evidenced by increased delta and theta activity. This distinction in EEG patterns between demented and non-demented PD patients highlights the potential of EEG as a diagnostic tool for detecting neurological changes tied to cognitive decline in PD.
The keyword is the authors’ refining and summarizing of the content of the article, which can reflect the core content of the article. The cluster analysis of keywords identifies the principal research topics in this field, including mild cognitive impairment, dementia with Lewy bodies, EEG, oscillatory activity, working memory, convolutional neural network, mouse model, freezing of gait, Parkinson’s disease, neuronal oscillations, emotion, sleep, machine learning, basal forebrain, variational pulsometry, transcranial magnetic stimulation, deep brain stimulation, functional connectivity, schizophrenia, antisaccade task, CSF examination, and frontal eye field. Based on this keyword analysis, the main research directions over the last two decades have been identified as: (1) Neural oscillations and connectivity: Studies have focused on measuring changes in specific EEG frequency bands, such as beta and gamma oscillations, which are believed to play a key role in the pathophysiology of PD. Many research studies have analyzed how these oscillations correlate with disease severity and response to treatment, providing insights into the underlying neural mechanisms of the disease (Stein and Bar-Gad, 2013; Guan et al., 2022). (2) Innovations in EEG Analysis: Techniques such as graph signal processing and graph convolutional networks have been developed with the objective of enhancing the interpretability and accuracy of EEG analysis (Betrouni et al., 2019). These innovative models integrate local and global network information to enhance the accuracy of PD diagnosis, offering high classification accuracy and detailed visualizations of EEG patterns linked to PD-specific impairments, such as speech disorders. Furthermore, other advanced machine learning techniques, including support vector machines and k-nearest neighbors, are employed to analyze resting-state EEG for cognitive profiling in PD, demonstrating the potential to identify levels of cognitive impairment (Zhao et al., 2024). (3) Impact of therapies on EEG: Research in this field assesses the impact of various therapeutic interventions on EEG dynamics, offering insights into the effects of treatment on brain function. Studies investigate the impact of medications such as levodopa and therapies like DBS on EEG patterns, with the aim of elucidating their mechanisms and optimizing treatment strategies (Tinkhauser et al., 2017; Lofredi et al., 2018; Miocinovic et al., 2018). (4) Cognitive and emotional assessments. Cozac et al. (2016) have demonstrated that quantitative EEG (QEEG) is an effective method for predicting cognitive decline and the risk of dementia in patients with PD. QEEG measures, such as background rhythm frequency and power in specific frequency bands, demonstrate a correlation with neuropsychological assessments and are capable of predicting the onset of dementia. Furthermore, EEG-based emotion classification research is advancing our understanding of how PD affects emotional recognition and expression, utilizing neural network architectures to improve the accuracy and robustness of emotion detection (Klassen et al., 2011). The identification of keyword bursts can reveal changes in the research hotspots and future development trends in this field. In recent years, the identification of burst keywords in this field has revealed a number of emerging research topics, including machine learning, deep learning, prediction, task analysis, walking, network, features, balance, and nonmotor symptoms. These research hotspots not only reflect the current development trends but also provide valuable insights for future research. The emergence of burst keywords such as machine learning and deep learning indicates the integration of advanced computational methods in PD research. In particular, these methods have been employed to enhance predictive models and to facilitate the understanding of complex datasets (Geraedts et al., 2021; Aljalal et al., 2022; Parajuli et al., 2023; Diao et al., 2024; Riasi et al., 2024). Other keywords, such as “prediction” and “task analysis,” reflect a growing focus on developing tools that can anticipate disease progression and evaluate patient responses to various tasks (Bar-On et al., 2023; Memon et al., 2023; Huang et al., 2024). These tools are designed to inform the development of personalized treatment strategies. The growing interest in “walking,” “balance,” and “nonmotor symptoms (Conti et al., 2023)” reflects a broader approach to understanding PD, emphasizing the need to address the multifaceted nature of the disease that affects both motor and cognitive functions (Bosch et al., 2021; Costa et al., 2023; Guo et al., 2024). As researchers continue to investigate these topics, integrating advanced technologies and holistic approaches promises to drive innovations in the management of PD, potentially leading to earlier detection, more effective treatments, and better patient outcomes. This convergence of technology and clinical practice paves the way for transformative advancements in the care and study of PD.
5 Limitation
Firstly, this bibliometric analysis is confined to literature indexed from the SCI-Expanded within the WoSCC, which may result in the overlooking of significant contributions from other databases or journals not covered by this collection. The primary reason for this is the utilization of an online bibliometric visualization and analysis website, which currently only supports the WoS database. Furthermore, the focus on English-language publications may exclude valuable research published in other languages, which could offer different insights or corroborate current findings. This language bias could limit the comprehensiveness of our understanding of the global research landscape in the field. In the future, translation tools or enhanced national communication may be employed to include articles in a greater number of languages. Moreover, by concentrating primarily on peer-reviewed articles and reviews, other potentially impactful works such as conference papers, dissertations, or technical reports might have been excluded, which could contribute innovative approaches or preliminary findings not yet available in journal articles. Additionally, the inherent limitations of bibliometric analysis, such as the dependence on keyword frequency and co-citation networks, might not fully capture the nuanced interrelations or emerging trends that qualitative assessments could reveal. Finally, this article includes studies from 2004 to 2023, which inevitably limits the inclusion of earlier as well as more recent studies. In conclusion, while this study provides a structured overview of EEG research in PD using bibliometric methods, it is important to interpret its findings within the context of these outlined limitations. Future research should aim to expand the scope of databases, include multilingual publications, and incorporate a broader range of document types to enhance the depth and accuracy of the portrayal of the research landscape.
6 Conclusion
From 2004 to 2023, EEG research in PD has shown a significant increase in both publication and citation counts. This reflects the growing interest and significant progress in this field. The application of bibliometrics and visualization methods has provided insights into the evolution, impact, and future direction of this area of research. This study demonstrates that countries such as the United States, Germany, and England are at the forefront of EEG research and innovation, contributing a substantial volume of research and technological advancement. The extensive collaboration between scholars and institutions around the world serves to illustrate the collective global effort to address the complexities of PD through EEG research. The identification of key research institutions, influential scholars, and significant publications illustrates the dynamic and interconnected nature of the field. Notably, the integration of EEG technology has revealed the complex neurological underpinnings of PD, providing innovative diagnostic tools and therapeutic insights. In the future, this field will continue to adopt advanced EEG technologies and machine learning models in order to deepen our understanding of the disease and improve treatments.
Data availability statement
The original contributions presented in the study are included in the article/Supplementary material, further inquiries can be directed to the corresponding authors.
Author contributions
X-YL: Conceptualization, Data curation, Formal analysis, Methodology, Visualization, Writing – original draft, Writing – review & editing. Y-XG: Investigation, Methodology, Writing – review & editing. T-TQ: Validation, Writing – review & editing. L-HZ: Visualization, Writing – review & editing. L-QL: Resources, Writing – review & editing. YG: Funding acquisition, Writing – review & editing. T-FY: Conceptualization, Writing – review & editing.
Funding
The author(s) declare that financial support was received for the research, authorship, and/or publication of this article. The study was supported by National Natural Science Foundation of China (82102664), the Gusu District Health Talent Training Project (GSWS2022073).
Acknowledgments
The authors thank everyone who contributed to the writing and all the publications, and their authors involved in this study.
Conflict of interest
The authors declare that the research was conducted in the absence of any commercial or financial relationships that could be construed as a potential conflict of interest.
Publisher’s note
All claims expressed in this article are solely those of the authors and do not necessarily represent those of their affiliated organizations, or those of the publisher, the editors and the reviewers. Any product that may be evaluated in this article, or claim that may be made by its manufacturer, is not guaranteed or endorsed by the publisher.
Supplementary material
The Supplementary material for this article can be found online at: https://www.frontiersin.org/articles/10.3389/fnins.2024.1433583/full#supplementary-material
Abbreviations
TC, Total citations; ACPP, Average citation per publication; TLS, Total link strength.
References
Aljalal, M., Aldosari, S. A., Molinas, M., AlSharabi, K., and Alturki, F. A. (2022). Detection of Parkinson's disease from EEG signals using discrete wavelet transform, different entropy measures, and machine learning techniques. Sci. Rep. 12:22547. doi: 10.1038/s41598-022-26644-7
Bar-On, M., Baharav, S., Katzir, Z., Mirelman, A., Sosnik, R., and Maidan, I. (2023). Task-related reorganization of cognitive network in Parkinson's disease using electrophysiology. Mov. Disord. 38, 2031–2040. doi: 10.1002/mds.29571
Betrouni, N., Delval, A., Chaton, L., Defebvre, L., Duits, A., Moonen, A., et al. (2019). Electroencephalography-based machine learning for cognitive profiling in Parkinson's disease: preliminary results. Mov. Disord. 34, 210–217. doi: 10.1002/mds.27528
Bloem, B. R., Okun, M. S., and Klein, C. (2021). Parkinson's disease. Lancet 397, 2284–2303. doi: 10.1016/s0140-6736(21)00218-x
Bosch, T. J., Kammermeier, S., Groth, C., Leedom, M., Hanson, E. K., Berg-Poppe, P., et al. (2021). Cortical and cerebellar oscillatory responses to postural instability in Parkinson's disease. Front. Neurol. 12:752271. doi: 10.3389/fneur.2021.752271
Brown, P. (2003). Oscillatory nature of human basal ganglia activity: relationship to the pathophysiology of Parkinson's disease. Mov. Disord. 18, 357–363. doi: 10.1002/mds.10358
Brown, P., Oliviero, A., Mazzone, P., Insola, A., Tonali, P., and Di Lazzaro, V. (2001). Dopamine dependency of oscillations between subthalamic nucleus and pallidum in Parkinson's disease. J. Neurosci. 21, 1033–1038. doi: 10.1523/jneurosci.21-03-01033.2001
Chen, L., Chen, J., Wu, M., Yan, P., and Zhou, X. (2024). Analyzing the bibliometrics of brain-gut axis and Parkinson's disease. Front. Neurol. 15:1343303. doi: 10.3389/fneur.2024.1343303
Conti, M., Guerra, A., Pierantozzi, M., Bovenzi, R., D'Onofrio, V., Simonetta, C., et al. (2023). Band-specific altered cortical connectivity in early Parkinson's disease and its clinical correlates. Mov. Disord. 38, 2197–2208. doi: 10.1002/mds.29615
Costa, T. D. C., Machado, C. B. d. S., Lemos Segundo, R. P., Silva, J. P. d. S., Silva, A. C. T., Andrade, R. d. S., et al. (2023). Are the EEG microstates correlated with motor and non-motor parameters in patients with Parkinson's disease? Neurophysiol. Clin. 53:102839. doi: 10.1016/j.neucli.2022.102839
Cozac, V. V., Gschwandtner, U., Hatz, F., Hardmeier, M., Rüegg, S., and Fuhr, P. (2016). Quantitative EEG and cognitive decline in Parkinson's disease. Parkinsons Dis 2016:9060649. doi: 10.1155/2016/9060649
Dammertz, L., Schrag, A., Bohlken, J., Heuer, J., Kohring, C., Schorlemmer, J., et al. (2023). Falling incidence of Parkinson's disease in Germany. Eur. J. Neurol. 30, 3124–3131. doi: 10.1111/ene.16000
Delorme, A., and Makeig, S. (2004). EEGLAB: an open source toolbox for analysis of single-trial EEG dynamics including independent component analysis. J. Neurosci. Methods 134, 9–21. doi: 10.1016/j.jneumeth.2003.10.009
Diao, Y., Xie, H., Wang, Y., Zhao, B., Yang, A., and Zhang, J. (2024). Individual structural covariance network predicts long-term motor improvement in Parkinson's disease with STN-DBS. AJNR Am. J. Neuroradiol. doi: 10.3174/ajnr.A8245 [Epub ahead of print].
Durieux, V., and Gevenois, P. A. (2010). Bibliometric indicators: quality measurements of scientific publication. Radiology 255, 342–351. doi: 10.1148/radiol.09090626
Fischer, P., Pogosyan, A., Green, A. L., Aziz, T. Z., Hyam, J., Foltynie, T., et al. (2019). Beta synchrony in the cortico-basal ganglia network during regulation of force control on and off dopamine. Neurobiol. Dis. 127, 253–263. doi: 10.1016/j.nbd.2019.03.004
Geraedts, V. J., Koch, M., Kuiper, R., Kefalas, M., Bäck, T. H. W., van Hilten, J. J., et al. (2021). Preoperative electroencephalography-based machine learning predicts cognitive deterioration after subthalamic deep brain stimulation. Mov. Disord. 36, 2324–2334. doi: 10.1002/mds.28661
Guan, A., Wang, S., Huang, A., Qiu, C., Li, Y., Li, X., et al. (2022). The role of gamma oscillations in central nervous system diseases: mechanism and treatment. Front. Cell. Neurosci. 16:962957. doi: 10.3389/fncel.2022.962957
Guo, B., Han, Q., Ni, J., and Yan, Z. (2023). Research hotspots and frontiers of neuromodulation techniques in disorders of consciousness: a bibliometric analysis. Front. Neurosci. 17:1343471. doi: 10.3389/fnins.2023.1343471
Guo, X., Zhao, S., Yu, L., Wang, H., Acquah, M. E. E., Chen, W., et al. (2024). Neural correlates of abnormal cortical gait control in Parkinson's disease: a whole-gait-cycle EEG study. IEEE Trans. Biomed. Eng. 71, 400–409. doi: 10.1109/tbme.2023.3301528
Hayes, M. T. (2019). Parkinson's disease and parkinsonism. Am. J. Med. 132, 802–807. doi: 10.1016/j.amjmed.2019.03.001
He, S., Liang, Q., Zhu, J., Wang, C., Lin, X., Wu, D., et al. (2023). Research landscape and emerging trends of diabetes-associated cognitive dysfunction: a bibliometric analysis. Front. Neurosci. 17:1214301. doi: 10.3389/fnins.2023.1214301
Hoehn, M. M., and Yahr, M. D. (1967). Parkinsonism: onset, progression and mortality. Neurology 17, 427–442. doi: 10.1212/wnl.17.5.427
Huang, C. Y., Chen, Y. A., Wu, R. M., and Hwang, I. S. (2024). Neural oscillations and functional significances for prioritizing dual-task walking in Parkinson's disease. J. Parkinsons Dis. 14, 283–296. doi: 10.3233/jpd-230245
Klassen, B. T., Hentz, J. G., Shill, H. A., Driver-Dunckley, E., Evidente, V. G., Sabbagh, M. N., et al. (2011). Quantitative EEG as a predictive biomarker for Parkinson disease dementia. Neurology 77, 118–124. doi: 10.1212/WNL.0b013e318224af8d
Lalo, E., Thobois, S., Sharott, A., Polo, G., Mertens, P., Pogosyan, A., et al. (2008). Patterns of bidirectional communication between cortex and basal ganglia during movement in patients with Parkinson disease. J. Neurosci. 28, 3008–3016. doi: 10.1523/jneurosci.5295-07.2008
Li, F., Zhang, D., Chen, J., Tang, K., Li, X., and Hou, Z. (2023). Research hotspots and trends of brain-computer interface technology in stroke: a bibliometric study and visualization analysis. Front. Neurosci. 17:1243151. doi: 10.3389/fnins.2023.1243151
Li, Z., Zhang, Y., Zhang, B., Guo, R., He, M., Liu, Z. L., et al. (2023). Bibliometric study of immunotherapy for hepatocellular carcinoma. Front. Immunol. 14:1210802. doi: 10.3389/fimmu.2023.1210802
Little, S., Pogosyan, A., Kuhn, A. A., and Brown, P. (2012). β band stability over time correlates with parkinsonian rigidity and bradykinesia. Exp. Neurol. 236, 383–388. doi: 10.1016/j.expneurol.2012.04.024
Litvak, V., Jha, A., Eusebio, A., Oostenveld, R., Foltynie, T., Limousin, P., et al. (2011). Resting oscillatory cortico-subthalamic connectivity in patients with Parkinson's disease. Brain 134, 359–374. doi: 10.1093/brain/awq332
Liu, C., Jiang, Z., Liu, S., Chu, C., Wang, J., Liu, W., et al. (2023). Frequency-dependent microstate characteristics for mild cognitive impairment in Parkinson's disease. IEEE Trans. Neural Syst. Rehabil. Eng. 31, 4115–4124. doi: 10.1109/tnsre.2023.3324343
Lofredi, R., Neumann, W. J., Bock, A., Horn, A., Huebl, J., Siegert, S., et al. (2018). Dopamine-dependent scaling of subthalamic gamma bursts with movement velocity in patients with Parkinson's disease. eLife 7:e31895. doi: 10.7554/eLife.31895
Lyu, Y., Li, W., Guo, Q., and Wu, H. (2024). Mapping knowledge landscapes and emerging trends of Marburg virus: a text-mining study. Heliyon 10:e29691. doi: 10.1016/j.heliyon.2024.e29691
Mallet, N., Pogosyan, A., Márton, L. F., Bolam, J. P., Brown, P., and Magill, P. J. (2008). Parkinsonian beta oscillations in the external globus pallidus and their relationship with subthalamic nucleus activity. J. Neurosci. 28, 14245–14258. doi: 10.1523/jneurosci.4199-08.2008
Memon, A. A., Catiul, C., Irwin, Z., Pilkington, J., Memon, R. A., Joop, A., et al. (2023). Quantitative sleep electroencephalogram and cognitive performance in Parkinson's disease with and without rapid eye movement sleep behavior disorder. Front. Neurol. 14:1223974. doi: 10.3389/fneur.2023.1223974
Miocinovic, S., Miller, A., Swann, N. C., Ostrem, J. L., and Starr, P. A. (2018). Chronic deep brain stimulation normalizes scalp EEG activity in isolated dystonia. Clin. Neurophysiol. 129, 368–376. doi: 10.1016/j.clinph.2017.11.011
Muthuraman, M., Bange, M., Koirala, N., Ciolac, D., Pintea, B., Glaser, M., et al. (2020). Cross-frequency coupling between gamma oscillations and deep brain stimulation frequency in Parkinson's disease. Brain 143, 3393–3407. doi: 10.1093/brain/awaa297
Oh, S. L., Hagiwara, Y., Raghavendra, U., Yuvaraj, R., Arunkumar, N., Murugappan, M., et al. (2020). A deep learning approach for Parkinson's disease diagnosis from EEG signals. Neural Comput. Applic. 32, 10927–10933. doi: 10.1007/s00521-018-3689-5
Oostenveld, R., Fries, P., Maris, E., and Schoffelen, J. M. (2011). FieldTrip: open source software for advanced analysis of MEG, EEG, and invasive electrophysiological data. Comput. Intell. Neurosci. 2011:156869. doi: 10.1155/2011/156869
Parajuli, M., Amara, A. W., and Shaban, M. (2023). Deep-learning detection of mild cognitive impairment from sleep electroencephalography for patients with Parkinson's disease. PLoS One 18:e0286506. doi: 10.1371/journal.pone.0286506
Reich, S. G., and Savitt, J. M. (2019). Parkinson's disease. Med. Clin. North Am. 103, 337–350. doi: 10.1016/j.mcna.2018.10.014
Riasi, A., Delrobaei, M., and Salari, M. (2024). A decision support system based on recurrent neural networks to predict medication dosage for patients with Parkinson's disease. Sci. Rep. 14:8424. doi: 10.1038/s41598-024-59179-0
Silberstein, P., Pogosyan, A., Kühn, A. A., Hotton, G., Tisch, S., Kupsch, A., et al. (2005). Cortico-cortical coupling in Parkinson's disease and its modulation by therapy. Brain 128, 1277–1291. doi: 10.1093/brain/awh480
Soikkeli, R., Partanen, J., Soininen, H., Pääkkönen, A., and Riekkinen, P. Sr. (1991). Slowing of EEG in Parkinson's disease. Electroencephalogr. Clin. Neurophysiol. 79, 159–165. doi: 10.1016/0013-4694(91)90134-p
Stam, C. J. (2005). Nonlinear dynamical analysis of EEG and MEG: review of an emerging field. Clin. Neurophysiol. 116, 2266–2301. doi: 10.1016/j.clinph.2005.06.011
Stam, C. J., and van Straaten, E. C. (2012). The organization of physiological brain networks. Clin. Neurophysiol. 123, 1067–1087. doi: 10.1016/j.clinph.2012.01.011
Stein, E., and Bar-Gad, I. (2013). β oscillations in the cortico-basal ganglia loop during parkinsonism. Exp. Neurol. 245, 52–59. doi: 10.1016/j.expneurol.2012.07.023
Swann, N., Poizner, H., Houser, M., Gould, S., Greenhouse, I., Cai, W., et al. (2011). Deep brain stimulation of the subthalamic nucleus alters the cortical profile of response inhibition in the beta frequency band: a scalp EEG study in Parkinson's disease. J. Neurosci. 31, 5721–5729. doi: 10.1523/jneurosci.6135-10.2011
Tao, S., Tang, X., Yu, L., Li, L., Zhang, G., Zhang, L., et al. (2023). Prognosis of coronary heart disease after percutaneous coronary intervention: a bibliometric analysis over the period 2004-2022. Eur. J. Med. Res. 28:311. doi: 10.1186/s40001-023-01220-5
Tinkhauser, G., Pogosyan, A., Tan, H., Herz, D. M., Kühn, A. A., and Brown, P. (2017). Beta burst dynamics in Parkinson's disease OFF and ON dopaminergic medication. Brain 140, 2968–2981. doi: 10.1093/brain/awx252
Waninger, S., Berka, C., Stevanovic, K. M., Korszen, S., Mozley, P. D., Henchcliffe, C., et al. (2020). Neurophysiological biomarkers of Parkinson's disease. J. Parkinsons Dis. 10, 471–480. doi: 10.3233/jpd-191844
Zhao, S., Dai, G., Li, J., Zhu, X., Huang, X., Li, Y., et al. (2024). An interpretable model based on graph learning for diagnosis of Parkinson's disease with voice-related EEG. NPJ Digit. Med. 7:3. doi: 10.1038/s41746-023-00983-9
Zhao, Y., Xia, Q., Zong, H., Wang, Y., Dong, H., Zhu, L., et al. (2023). Bibliometric and visual analysis of spinal cord injury-associated macrophages from 2002 to 2023. Front. Neurol. 14:1285908. doi: 10.3389/fneur.2023.1285908
Keywords: bibliometrix, CiteSpace, VOSviewer, Parkinson’ disease, electroencephalogram, neural oscillations, visualization analysis
Citation: Liao X-Y, Gao Y-X, Qian T-T, Zhou L-H, Li L-Q, Gong Y and Ye T-F (2024) Bibliometric analysis of electroencephalogram research in Parkinson’s disease from 2004 to 2023. Front. Neurosci. 18:1433583. doi: 10.3389/fnins.2024.1433583
Edited by:
Matteo Conti, Policlinico Tor Vergata, ItalyReviewed by:
Zinovia Maria Kefalopoulou, University of Patras, GreeceMario Versaci, Mediterranea University of Reggio Calabria, Italy
Copyright © 2024 Liao, Gao, Qian, Zhou, Li, Gong and Ye. This is an open-access article distributed under the terms of the Creative Commons Attribution License (CC BY). The use, distribution or reproduction in other forums is permitted, provided the original author(s) and the copyright owner(s) are credited and that the original publication in this journal is cited, in accordance with accepted academic practice. No use, distribution or reproduction is permitted which does not comply with these terms.
*Correspondence: Li-Qin Li, bGlsaXFpbmhzQDE2My5jb20=; Yan Gong, Z29uZ3lhbjIwMDYwNUAxMjYuY29t; Tian-Fen Ye, dGlmZmFueXNvb2Nob3dAb3V0bG9vay5jb20=
†These authors share first authorship