- 1Department of Nursing Science, Research Institute of Nursing Science, Chungbuk National University, Cheongju, Chungcheongbuk-do, Republic of Korea
- 2Department of Information Statistics, Gyeongsang National University, Jinju, Gyeongsangnam-do, Republic of Korea
- 3Department of Radiation Oncology, Seoul National University College of Medicine, Seoul, Republic of Korea
- 4Division of Healthcare Planning, Bigdata Research Institute, Kangbuk Samsung Hospital, Sungkyunkwan University School of Medicine, Seoul, Republic of Korea
- 5Department of Family Medicine, Kangbuk Samsung Hospital, Sungkyunkwan University School of Medicine, Seoul, Republic of Korea
- 6Institute of Radiation Medicine, Medical Research Center, Seoul National University, Seoul, Republic of Korea
Background: During the height of the COVID-19 pandemic, the Korean government temporarily allowed full scale telehealth care for safety and usability. However, limited studies have evaluated the impact of telehealth by analyzing the physical and/or mental health data of patients with COVID-19 diagnosis collected through telehealth targeting Korean population.
Objective: This study aimed to identify subgroup of depressive symptom trajectories in patients with clinically mild COVID-19 using collected longitudinal data from a telehealth-based contactless clinical trial.
Methods: A total of 199 patients with COVID-19 were accrued for contactless clinical trial using telehealth from March 23 to July 20, 2022. Depressive symptoms were measured using the patient health questionnaire-9 on the start day of quarantine, on the final day of quarantine, and 1 month after release from quarantine. Additionally, acute COVID-19 symptoms were assessed every day during quarantine. This study used a latent class mixed model to differentiate subgroups of depressive symptom trajectories and a logistic regression model with Firth’s correction to identify associations between acute COVID-19 symptoms and the subgroups.
Results: Two latent classes were identified: class 1 with declining linearity at a slow rate and class 2 with increasing linearity. Among COVID-19 symptoms, fever, chest pain, and brain fog 1 month after release from quarantine showed strong associations with class 2 (fever: OR, 19.43, 95% CI, 2.30–165.42; chest pain: OR, 6.55, 95% CI, 1.15–34.61; brain fog: OR, 7.03, 95% CI 2.57–20.95). Sleeping difficulty and gastrointestinal symptoms were also associated with class 2 (gastrointestinal symptoms: OR, 4.76, 95% CI, 1.71–14.21; sleeping difficulty: OR, 3.12, 95% CI, 1.71–14.21).
Conclusion: These findings emphasize the need for the early detection of depressive symptoms in patients in the acute phase of COVID-19 using telemedicine. Active intervention, including digital therapeutics, may help patients with aggravated depressive symptoms.
1 Introduction
As the COVID-19 outbreak transition into a pandemic, the role and the use of telehealth is expanding and gaining significant background. Many countries have introduced remote patient monitoring systems that use telehealth to manage patients at remote locations (1–3). Telehealth has been shown to be an excellent method for delivering care as it allows not only patients but also health care providers to protect themselves from the risk of infection (1, 4). Since the outbreak of the COVID-19 pandemic, telemedicine, which had been under limited pilot phase in Korea, was temporarily allowed in full scale for patients diagnosed with COVID-19. Until May 2023, all patients with COVID-19 were obligated to quarantine at home for 5–7 days during the acute clinical phase. Patients with COVID-19 received prescriptions for related acute respiratory symptoms via contactless consultations during the quarantine period.
Meanwhile, the impact of COVID-19 on mental health has been continuously reported. The prevalence of depression symptoms in adults in the US during the COVID-19 pandemic was more than three times that of pre-pandemic era (5). In the UK, the prevalence of depression increased to 32% from 4.12% in the pre-pandemic period (6). In China, the prevalence of depression has been moderately high (7). South Korea likewise saw an increase in manifestation of depression. During the COVID-19 pandemic, 30.7% of 2,288 adult residents reported a Patient Health Questionnaire-2 score of 3, indicating a high prevalence of depression (8).
With the transition to the long-COVID era, many studies have explored mental health trajectories, including low, moderate, severe, and worsening mental health, using longitudinal data (9–11). Contributing social and psychological factors have also been identified. The unpredictable disease course of COVID-19 and COVID-19-related financial and social impairments have been reported to be related to the initial elevation in the level of depressive or anxiety symptoms (11–13). Moreover, the exacerbation of COVID-19 symptoms may also contribute to the development of mental health symptoms (14, 15).
Depression is strongly associated with somatic symptoms (16). Meanwhile, acute COVID-19-related somatic symptoms are associated with the exacerbation of depression and anxiety (15). In Korea, depression has been identified as the main symptom among COVID-19-related persistent symptoms (17). However, no study has explored the association between the trajectories of depression in the acute phase and acute COVID-19-related symptoms for these patients to provide a Korean perspective.
Against this background, the SMILE (Smart Monitoring solution for Infectious disease management through Lifestyle Evaluation) research team at Seoul National University Hospital established a remote patient monitoring system to effectively respond to the infectious disease. A contactless clinical trial protocol using telehealth was developed (18). Longitudinal data, including physical and mental health-related data, were prospectively collected from patients with COVID-19. Obvious next step was to demonstrate the impact of telehealth by analyzing the collected data. Thus, goal of current study was to identify subgroups of depressive symptom trajectories in patients with clinically mild COVID-19 in Korea and explore the contributing COVID-19-related symptoms to those groups using collected longitudinal data from a contactless clinical trial using telehealth.
2 Methods
2.1 Study design
This is a prospective observational study.
2.2 Participants and procedures
After institutional review board approval (IRB number: H-2107-049-1233), 199 adult patients with confirmed COVID-19 diagnosis quarantined at home were enrolled in this prospective trial from March 23 to July 20, 2022. The inclusion criteria were as follows: (1) 19 years or older; (2) confirmed COVID-19 diagnosis; (3) understand the study purpose, and (4) agree to participate in the trial.
This study was based on a published protocol developed for patients quarantined at residential treatment centers (18) with modification for home use. The study participants were recruited using convenience sampling. The research team displayed a poster that provided information on the research purpose and methods on the staff portal site and notice board at the study hospital. Patients who were hospital employees or acquaintances voluntarily contacted the research assistants and filled out an application form online through a URL or QR code in the poster.
Research assistants explained the purpose of the study to the prospective participants and oral informed consent was obtained over a phone call. Data were collected using Google Forms (Google, CA, USA). Through a mobile messenger, the study team sent the URL of the Google form containing an online questionnaire on mental health status. The participants completed online questionnaires on every quarantine day and 1 month after the release from quarantine.
2.3 Measures
Depression symptoms were measured using the patient health questionnaire-9 (PHQ-9) (19) at three time points: on the start day of quarantine (Time 1), on the final day of quarantine (Time 3), and 1 month after release from quarantine (Time 4). Participants were asked on the frequency of the nine potentially bothering symptoms. Each item was scored using the following four-point Likert scale: not at all = 0, several days a week = 1, more than half the week = 2, nearly every day = 3. Severity of depression was divided into five tiers, a score of 1–4 as none, 5–9 as mild, 10–14 as moderate, 15–19 as moderately severe, and 20–27 as severe.
General characteristics of the participants, including gender, age, past medical history, smoking status, and initial neuropsychiatric symptoms, were assessed at Time 1. Past medical history included history of diabetes mellitus, hypertension, cardiovascular disease, and respiratory disease. Initial neuropsychiatric symptoms included depression, lethargy, anxiety, sensitivity, insomnia, panic attack, and suicide attempts. Self-reported acute COVID-19 symptoms were assessed at every quarantine day (Time 2) and at Time 4. Self-reported acute COVID-19 symptoms at Time 2 included cough, sputum, fever, rhinorrhea, sore throat, dyspnea, chest pain, pain, sleeping difficulty, loss of smell, loss of taste, and various gastrointestinal symptoms, which includes nausea, vomiting, abdominal discomfort, constipation, and diarrhea.
2.4 Statistical analysis
All analyses were conducted using R version 4.2 (R Project for Statistical Computing). To identify the subgroups of depressive symptom trajectories over time, we used the latent class mixed model (LCMM), also called growth mixture modeling (20–23), subsequently selecting the models that provided the best fit for the data. To assess the clinical characteristics of the subgroups identified at Time 1, we conducted a logistic regression with subgroups identified as dependent variables and sociodemographic features, past medical history, initial psychological symptoms, and acute COVID-19 symptoms measured at Time 1 as independent variables. To support the sample size considerations for binary logistic regression analysis, we fitted the logistic regression model using a modified estimation procedure known as Firth’s correction (24). We then selected variables at Time 1 that were significantly associated with subgroup identification. Next, after selecting the clinical characteristics from Times 1, 2, and 4 associated with the subgroups of trajectories, we conducted additional logistic regression using a stepwise approach with subgroups identified as dependent variables and those variables measured at Times 2 and 4 as independent variables. In this analysis, we added previously selected significant variables at Time 1 to the logistic regression model as fixed variables. The model was also fitted using Firth’s correction (24), and statistical significance was tested at the α = 0.05 level.
3 Results
Table 1 presents the clinical characteristics of the 199 participants.
The LCMM identified two latent classes as the best fit for the data. The estimated mean trajectories of the two classes are shown in Figure 1. In class 1 (n = 163; 81.9%), the mean trajectory declined linearly at a slow rate. The PHQ-9 mean scores of class 1 at each time point were 1.64 (SD 1.89) at time 1, 2.33 (SD 2.15) at time 3, and 1.62 (SD 1.68) at time 4. In class 2 (n = 36, 18.1%), patients had a higher level of depression symptoms than those in class 1 at time 1 and showed increased linearity with the progress of the quarantine period. The PHQ-9 mean scores of class 2 at each time point were 5.0 (SD 3.58) at time 1, 5.03 (SD 2.95) at time 3, and 7.89 (SD 2.57) at time 4.
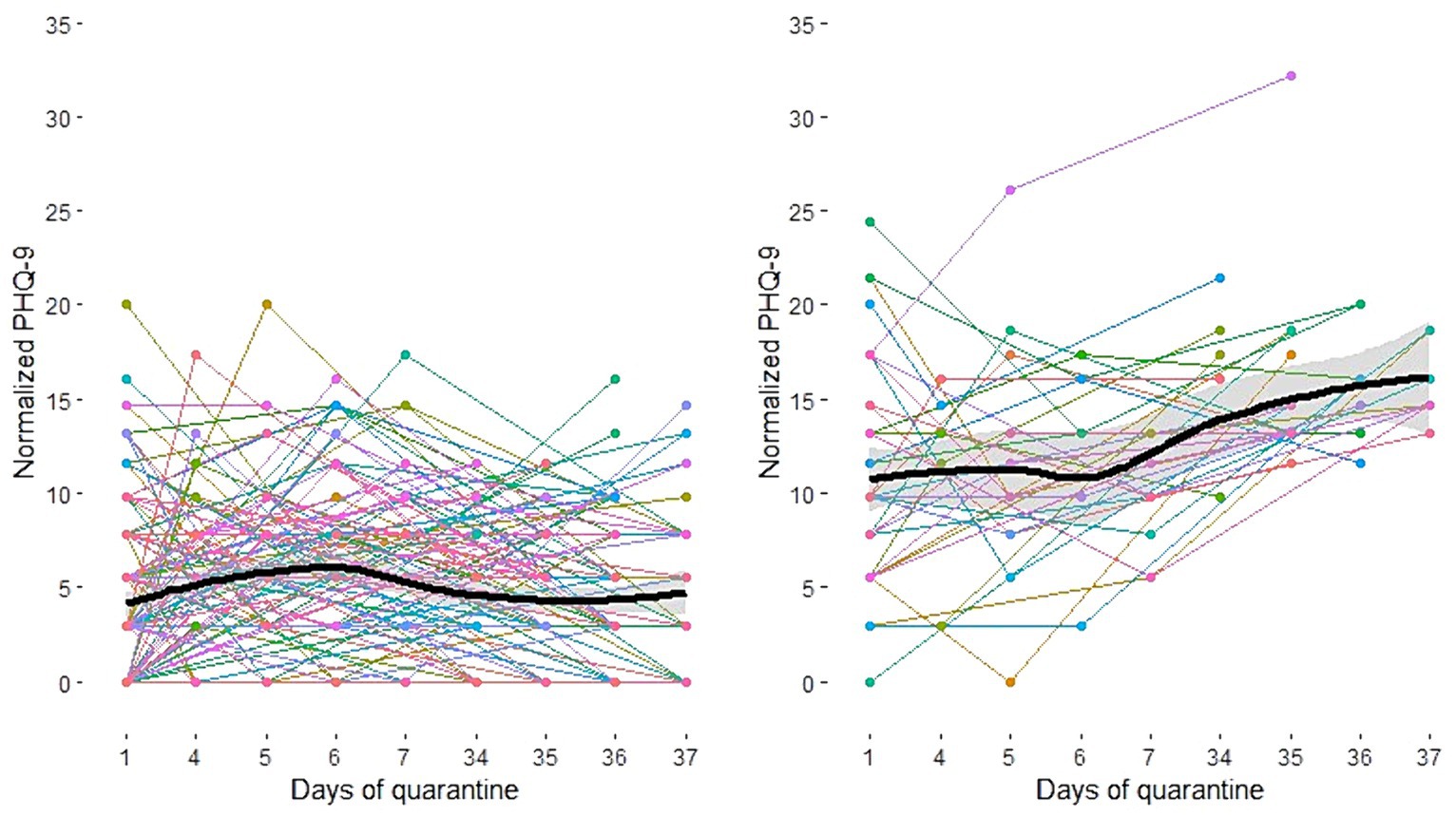
Figure 1. Estimated mean trajectories of depression symptoms in patients with COVID-19 (Left: Class 1, Right: Class 2).
Table 2 presents the clinical characteristics of the participants in the subgroups identified by the LCMM at time 1. Initial neuropsychiatric symptoms were observed in 80.6% of patients in class 2 and in 65% of patients in class 1, which showed statistically significant difference (p < 0.001). More than 70% of the patients in both classes experienced cough, sputum, and sore throat, among the COVID-19-related symptoms. Of note, there was statistically significant difference in incidence of chest pain (5.5 and 19.4% of patients in classes 1 and 2, respectively).
Table 3 presents the results of the logistic regression model for COVID-19-related symptoms at time 1. Among COVID-19 symptoms, rhinorrhea and chest pain showed positive associations with increased odds of exacerbation of depression. Although extent was not statistically significant (rhinorrhea: odds ratio [OR], 2.06, 95% CI, 0.96–4.57; chest pain: OR, 3.07, 95% CI, 0.92–10.31), these two variables were chosen as fixed variables for the final model.
Table 4 presents the association between COVID-19 symptoms and the class 2. The final model included rhinorrhea and chest pain at time 1; cough at time 2; and fever, rhinorrhea, chest pain, gastrointestinal symptoms, sleeping difficulty, headache, and brain fog at time 4. Among these symptoms, fever at time 4 showed a strong association with class 2, indicating the exacerbation of depression during quarantine (OR, 19.43; 95% CI, 2.30–165.42). Patients with chest pain or brain fog at time 4 were more likely to have exacerbated depression during quarantine (chest pain: OR, 6.55, 95% CI, 1.15–34.61; brain fog: OR, 7.03, 95% CI, 2.57–20.95). In addition, patients who experienced gastrointestinal symptoms or sleeping difficulty at time 4 were more likely to have exacerbated depression during quarantine (gastrointestinal symptoms: OR, 4.76, 95% CI, 1.71–14.21; sleeping difficulty: OR, 3.12, 95% CI, 1.71–14.21).
4 Discussion
Longitudinal data related to depressive symptoms and COVID-19-related symptoms was collected through a contactless clinical trial using telehealth targeting patients with clinically mild symptoms at the acute phase of COVID-19 in South Korea. The results showed two subgroups of depressive symptom trajectories from COVID-19 infection to 1 month after quarantine: the stable group (class 1) and the exacerbated group (class 2).
LCMM was used to identify the two subgroups of patients with COVID-19 based on the trajectory of their PHQ-9 scores across multiple time points. Studies have used LCMM to successfully identify meaningful latent subgroups using longitudinal data (25). For example, it has been used to categorize trajectories of depression and anxiety symptom changes (26) and shown to perform as accurately as the traditional cutoff score approach in identifying heterogeneous subgroups in a longitudinal study on perinatal depression (27). The LCMM provided strong robustness in current analysis given the lack of a traditional or standard method for categorizing longitudinal post-COVID-19 depression changes.
Among the acute COVID-19-related symptoms at time 4, chest pain, gastrointestinal symptoms, sleeping difficulty, and brain fog, which are commonly observed in patients with depression (16), were significantly associated with class 2. The Patient Health Questionnaire 15, which is one of the most useful tools for measuring somatization in psychiatric patients, also includes the following symptoms: trouble sleeping, chest pain, and gastrointestinal symptoms, such as constipation, loose bowels, diarrhea, nausea, and indigestion (28). Indeed, 69% of patients with major depressive disorder who visited primary care facilities reported that physical symptoms were the main reason for their hospital visit (16). Ran et al. (29) found a significant correlation between depression and somatization in patients with COVID-19, which is consistent with the findings of the current study.
In addition, patients from Eastern cultures, compared to Western counterparts, tend to deny psychological symptoms and complain more about physical symptoms (16, 30–32). Therefore, early detection of depression is important for patients with persistent non-specific somatic symptoms, even after recovery from COVID-19. In Eastern cultures such as Korea, health authorities should prepare public health measures to monitor not only the progress of infectious diseases, but also the mental health.
The current study attempted to monitor and analyze acute COVID-19 somatic and depressive symptoms simultaneously using telehealth services for patients in remote locations. Results suggest that early detection of patients with depressive symptoms in the acute phase of COVID-19 using telemedicine is feasible. Digital therapeutics have been reported to be effective for patients with mental illnesses (33, 34). During the COVID-19 pandemic, even the general population without a history of mental illness preferred digital therapeutics to visiting psychiatric clinics (35). Thus, worsening of depressive symptoms may be prevented by offering digital therapeutics intervention to those with early detection during quarantine, when visit to the clinic in person can be limited (36).
4.1 Limitations
First, patient accrual done using convenience sampling may have led to the snowball sampling. Thus, the generalizability of the study results cannot be ensured. Second, the data were assessed using patient self-reports, which may be led to inaccurate estimates of symptom changes. Third, the patients could have undergone interventions, such as drug therapy for COVID-19 symptoms, that were not considered in the statistical analyses. Fourth, the PHQ-9 measures depressive symptoms over the past 2 weeks, resulting in timeline issues for Times 1 and 3 that must be considered with respect to the quarantine duration of approximately 7 days. Further study may be conducted using more specific tools such as the Hamilton depression rating scale (37) rather than PHQ-9. Fifth, current study did not collect social determinants and socio-economic status of the participants, which play an important role in mental health, but was not considered in the current analysis.
5 Conclusion
Results from current study demonstrated the potential impact of telehealth through the use of longitudinal data collected from a contactless clinical trial. We identified two subgroups of depressive symptom trajectories in Korean patients with clinically mild COVID-19: the stable group (class 1) and the worsening group (class 2). The COVID-19-related symptoms associated with these groups were fever, chest pain, brain fog, sleeping difficulty, and gastrointestinal symptoms 1 month after release from quarantine. Findings from current analysis suggested that early detection of patients with high risk may provide a chance for more effective intervention, such as digital therapeutics, prior to deterioration of mental health. In addition, further study may help to elucidate how post-COVID-19 syndrome impacts mental health of patients with COVID-19, and which of social determinants of health, such as socioeconomic status, education level, and ethnicity of these patients impact mental health to be nominated as potential risk factor.
Data availability statement
The datasets presented in this article are not readily available because hospital regulation restrictions and patient privacy concerns. Requests to access the datasets should be directed to EC, ekchie93@snu.ac.kr.
Ethics statement
The studies involving humans were approved by Institutional Review Board at Seoul National University Hospital (IRB number: H-2107-049-1233). The studies were conducted in accordance with the local legislation and institutional requirements. Written informed consent from the patients/participants was not required to participate in this study in accordance with the national legislation and the institutional requirements.
Author contributions
SS: Conceptualization, Writing – review & editing, Formal analysis, Methodology, Writing – original draft. SK: Formal analysis, Methodology, Writing – original draft, Writing – review & editing. YK: Formal analysis, Writing – review & editing. YB: Formal analysis, Writing – original draft, Writing – review & editing, Conceptualization, Methodology. EC: Conceptualization, Writing – review & editing, Funding acquisition, Supervision.
Funding
The author(s) declare financial support was received for the research, authorship, and/or publication of this article. This work was supported by an Institute of Information & Communications Technology Planning & Evaluation (IITP) grant funded by the Korean government (MSIT) (No. 2021–0-00312, Development of Non-Face-to-face Patient Infection Activity Prediction and Protection Management SW Technology at Home and Community Treatment Centers for Effective Response to Infectious Disease).
Conflict of interest
The authors declare that the research was conducted in the absence of any commercial or financial relationships that could be construed as a potential conflict of interest.
Publisher’s note
All claims expressed in this article are solely those of the authors and do not necessarily represent those of their affiliated organizations, or those of the publisher, the editors and the reviewers. Any product that may be evaluated in this article, or claim that may be made by its manufacturer, is not guaranteed or endorsed by the publisher.
References
1. Garfan, S, Alamoodi, AH, Zaidan, B, Al-Zobbi, M, Hamid, RA, Alwan, JK, et al. Telehealth utilization during the COVID-19 pandemic: a systematic review. Comput Biol Med. (2021) 138:104878. doi: 10.1016/j.compbiomed.2021.104878
2. Doraiswamy, S, Abraham, A, Mamtani, R, and Cheema, S. Use of telehealth during the COVID-19 pandemic: scoping review. J Med Internet Res. (2020) 22:e24087. doi: 10.2196/24087
3. Annis, T, Pleasants, S, Hultman, G, Lindemann, E, Thompson, JA, Billecke, S, et al. Rapid implementation of a COVID-19 remote patient monitoring program. J Am Med Inform Assoc. (2020) 27:1326–30. doi: 10.1093/jamia/ocaa097
4. Monaghesh, E, and Hajizadeh, A. The role of telehealth during COVID-19 outbreak: a systematic review based on current evidence. BMC Public Health. (2020) 20:1193. doi: 10.1186/s12889-020-09301-4
5. Ettman, CK, Abdalla, SM, Cohen, GH, Sampson, L, Vivier, PM, and Galea, S. Prevalence of depression symptoms in US adults before and during the COVID-19 pandemic. JAMA Netw Open. (2020) 3:e2019686. doi: 10.1001/jamanetworkopen.2020.19686
6. Dettmann, LM, Adams, S, and Taylor, G. Investigating the prevalence of anxiety and depression during the first COVID-19 lockdown in the United Kingdom: systematic review and meta-analyses. Br J Clin Psychol. (2022) 61:757–80. doi: 10.1111/bjc.12360
7. Bareeqa, SB, Ahmed, SI, Samar, SS, Yasin, W, Zehra, S, Monese, GM, et al. Prevalence of depression, anxiety and stress in China during COVID-19 pandemic: a systematic review with meta-analysis. Int J Psychiatry Med. (2021) 56:210–27. doi: 10.1177/0091217420978005
8. Kim, DM, Bang, YR, Kim, JH, and Park, JH. The prevalence of depression, anxiety and associated factors among the general public during COVID-19 pandemic: a cross-sectional study in Korea. J Korean Med Sci. (2021) 36:e214. doi: 10.3346/jkms.2021.36.e214
9. Saunders, R, Buckman, JE, Fonagy, P, and Fancourt, D. Understanding different trajectories of mental health across the general population during the COVID-19 pandemic. Psychol Med. (2021) 52:4049–57. doi: 10.1017/S0033291721000957
10. Fancourt, D, Steptoe, A, and Bu, F. Trajectories of anxiety and depressive symptoms during enforced isolation due to COVID-19 in England: a longitudinal observational study. Lancet Psychiatry. (2021) 8:141–9. doi: 10.1016/S2215-0366(20)30482-X
11. Batterham, PJ, Calear, AL, McCallum, SM, Morse, AR, Banfield, M, Farrer, LM, et al. Trajectories of depression and anxiety symptoms during the COVID-19 pandemic in a representative Australian adult cohort. Med J Aust. (2021) 214:462–8. doi: 10.5694/mja2.51043
12. Brooks, SK, Webster, RK, Smith, LE, Woodland, L, Wessely, S, Greenberg, N, et al. The psychological impact of quarantine and how to reduce it: rapid review of the evidence. Lancet. (2020) 395:912–20. doi: 10.1016/S0140-6736(20)30460-8
13. de Medeiros Carvalho, PM, Moreira, MM, de Oliveira, MNA, Landim, JMM, and Neto, MLR. The psychiatric impact of the novel coronavirus outbreak. Psychiatry Res. (2020) 286:112902. doi: 10.1016/j.psychres.2020.112902
14. Mazza, MG, De Lorenzo, R, Conte, C, Poletti, S, Vai, B, Bollettini, I, et al. Anxiety and depression in COVID-19 survivors: role of inflammatory and clinical predictors. Brain Behav Immun. (2020) 89:594–600. doi: 10.1016/j.bbi.2020.07.037
15. Sung, S, Kim, SH, Lee, C, Kim, Y, Bae, YS, and Chie, EK. The association of acute signs and symptoms of COVID-19 and exacerbation of depression and anxiety in patients with clinically mild COVID-19: retrospective observational study. JMIR Public Health Surveill. (2023) 9:e43003. doi: 10.2196/43003
16. Simon, GE, VonKorff, M, Piccinelli, M, Fullerton, C, and Ormel, J. An international study of the relation between somatic symptoms and depression. N Engl J Med. (1999) 341:1329–35. doi: 10.1056/NEJM199910283411801
17. Kim, Y, Kim, S-W, Chang, H-H, Kwon, KT, Bae, S, and Hwang, S. Post-acute COVID-19 syndrome in patients after 12 months from COVID-19 infection in Korea. BMC Infect Dis. (2022) 22:93. doi: 10.1186/s12879-022-07062-6
18. Bae, YS, Sung, S, Lee, J, Lee, H, and Chie, EK. Building and implementing a contactless clinical trial protocol for patients with COVID-19: a Korean perspective. Front Med. (2022) 9:975243. doi: 10.3389/fmed.2022.975243
19. Kroenke, K, Spitzer, RL, and Williams, JB. The PHQ-9: validity of a brief depression severity measure. J Gen Intern Med. (2001) 16:606–13. doi: 10.1046/j.1525-1497.2001.016009606.x
20. Bauer, DJ, and Curran, PJ. Distributional assumptions of growth mixture models: implications for overextraction of latent trajectory classes. Psychol Methods. (2003) 8:338–63. doi: 10.1037/1082-989X.8.3.338
21. Muthén, B, and Shedden, K. Finite mixture modeling with mixture outcomes using the EM algorithm. Biometrics. (1999) 55:463–9. doi: 10.1111/j.0006-341X.1999.00463.x
22. Verbeke, G, and Lesaffre, E. A linear mixed-effects model with heterogeneity in the random-effects population. J Am Stat Assoc. (1996) 91:217–21. doi: 10.1080/01621459.1996.10476679
23. Proust-Lima, C, Philipps, V, and Liquet, B. Estimation of Extended Mixed Models Using Latent Classes and Latent Processes: The R Package lcmm. Journal of Statistical Software. (2017) 78:1–56. doi: 10.18637/jss.v078.i02
24. Van Smeden, M, de Groot, JA, Moons, KG, Collins, GS, Altman, DG, Eijkemans, MJ, et al. No rationale for 1 variable per 10 events criterion for binary logistic regression analysis. BMC Med Res Methodol. (2016) 16:163. doi: 10.1186/s12874-016-0267-3
25. Herle, M, Micali, N, Abdulkadir, M, Loos, R, Bryant-Waugh, R, Hübel, C, et al. Identifying typical trajectories in longitudinal data: modelling strategies and interpretations. Eur J Epidemiol. (2020) 35:205–22. doi: 10.1007/s10654-020-00615-6
26. Saunders, R, Buckman, JE, Cape, J, Fearon, P, Leibowitz, J, and Pilling, S. Trajectories of depression and anxiety symptom change during psychological therapy. J Affect Disord. (2019) 249:327–35. doi: 10.1016/j.jad.2019.02.043
27. Badiya, PK, Siddabattuni, S, Dey, D, Javvaji, SK, Nayak, SP, Hiremath, AC, et al. Identification of clinical and psychosocial characteristics associated with perinatal depression in the south Indian population. Gen Hosp Psychiatry. (2020) 66:161–70. doi: 10.1016/j.genhosppsych.2020.08.002
28. Han, C, Pae, C-U, Patkar, AA, Masand, PS, Kim, KW, Joe, S-H, et al. Psychometric properties of the patient health Questionnaire-15 (PHQ-15) for measuring the somatic symptoms of psychiatric outpatients. Psychosomatics. (2009) 50:580–5. doi: 10.1176/appi.psy.50.6.580
29. Ran, L, Wang, W, Ai, M, Kong, Y, Chen, J, and Kuang, L. Psychological resilience, depression, anxiety, and somatization symptoms in response to COVID-19: a study of the general population in China at the peak of its epidemic. Soc Sci Med. (2020) 262:113261. doi: 10.1016/j.socscimed.2020.113261
30. Mezzich, JE, and Raab, ES. Depressive symptomatology across the Americas. Arch Gen Psychiatry. (1980) 37:818–23. doi: 10.1001/archpsyc.1980.01780200096012
31. Kleinman, A . Neurasthenia and depression: a study of somatization and culture in China. Cult Med Psychiatry. (1982) 6:117–90. doi: 10.1007/BF00051427
32. Bhatt, A, Tomenson, B, and Benjamin, S. Transcultural patterns of somatization in primary care: a preliminary report. J Psychosom Res. (1989) 33:671–80. doi: 10.1016/0022-3999(89)90082-2
33. Cho, C-H, Lee, T, Lee, J-B, Seo, JY, Jee, H-J, Son, S, et al. Effectiveness of a smartphone app with a wearable activity tracker in preventing the recurrence of mood disorders: prospective case-control study. JMIR Ment Health. (2020) 7:e21283. doi: 10.2196/21283
34. Kang, Y-W, Sun, T, Kim, GY, Jung, HY, Kim, HJ, Lee, S, et al. Design and methods of a prospective smartphone app-based study for digital phenotyping of mood and anxiety symptoms mixed with centralized and decentralized research form: the search your mind (S.Y.M., 心) project. Psychiatry Investig. (2022) 19:588–94. doi: 10.30773/pi.2022.0102
35. Kwon, M, Jung, Y-C, Lee, D, and Ahn, J. Mental health problems during COVID-19 and attitudes toward digital therapeutics. Psychiatry Investig. (2023) 20:52–61. doi: 10.30773/pi.2022.0150
36. Dang, A, Dang, D, and Rane, P. The expanding role of digital therapeutics in the post-COVID-19 era. Open COVID J. (2021) 1:32–7. doi: 10.2174/2666958702101010032
Keywords: telehealth, telemedicine, depression, COVID-19, LCMM
Citation: Sung S, Kim SH, Kim Y, Bae YS and Chie EK (2024) Exploring depressive symptom trajectories in COVID-19 patients with clinically mild condition in South Korea using remote patient monitoring: longitudinal data analysis. Front. Public Health. 12:1265848. doi: 10.3389/fpubh.2024.1265848
Edited by:
Eric S. Hall, Nemours Foundation, United StatesReviewed by:
Ajay Kumar, All India Institute of Medical Sciences Raipur, IndiaAlma Nurtazina, Semey State Medical University, Kazakhstan
Copyright © 2024 Sung, Kim, Kim, Bae and Chie. This is an open-access article distributed under the terms of the Creative Commons Attribution License (CC BY). The use, distribution or reproduction in other forums is permitted, provided the original author(s) and the copyright owner(s) are credited and that the original publication in this journal is cited, in accordance with accepted academic practice. No use, distribution or reproduction is permitted which does not comply with these terms.
*Correspondence: Eui Kyu Chie, ekchie93@snu.ac.kr; Ye Seul Bae, byeye1313@gmail.com