- Department of Animal Science and Technology, Chung-Ang University, Anseong-si, South Korea
Having played important roles in human growth and development, livestock animals are regarded as integral parts of society. However, industrialization has depleted natural resources and exacerbated climate change worldwide, spurring the emergence of various diseases that reduce livestock productivity. Meanwhile, a growing human population demands sufficient food to meet their needs, necessitating innovations in veterinary sciences that increase productivity both quantitatively and qualitatively. We have been able to address various challenges facing veterinary and farm systems with new scientific and technological advances, which might open new opportunities for research. Recent breakthroughs in multi-omics platforms have produced a wealth of genetic and genomic data for livestock that must be converted into knowledge for breeding, disease prevention and management, productivity, and sustainability. Vetinformatics is regarded as a new bioinformatics research concept or approach that is revolutionizing the field of veterinary science. It employs an interdisciplinary approach to understand the complex molecular mechanisms of animal systems in order to expedite veterinary research, ensuring food and nutritional security. This review article highlights the background, recent advances, challenges, opportunities, and application of vetinformatics for quality veterinary services.
Introduction
Livestock animals are an essential part of our life. Science-led innovation in veterinary research that benefits both people and animals as individuals and populations is crucial to maintaining public health (1, 2). This encompasses research on fundamental animal biology and animal welfare, as well as disease prevention, diagnosis, and therapy. Such innovation offers several opportunities for improving animal and human health (3, 4). Currently, veterinarians face many challenges exacerbated by climate change, including the emergence of new diseases, as well as those of a rapidly growing human population that requires adequate food and nutrition. Therefore, integration of interdisciplinary approaches with veterinary science is urgently needed to decode the complex molecular mechanisms of livestock systems (5–7).
The functioning of livestock systems is an area of active, ongoing research. Advancements in mathematical science, statistical methods, computer science, and information technology help biologists learn about biological systems quantitatively and qualitatively (8). Computers are essential components of these scientific advancements, as they play a crucial role in research and development sectors and become a major tool for researchers. In the era of “omics,” we can easily handle big data using computers, but the term “bioinformatics” was not introduced until the beginning of the 1970s by Hogeweg and Ben Hesper, when DNA could not yet be sequenced (9, 10). DNA's role as genetic material was also a matter of debate before 1952. Avery et al. (11) demonstrated that a non-virulent bacterial strain could acquire virulence by absorbing purified DNA from a virulent strain (8). However, the scientific community did not immediately accept their findings. Many scientists instead believed that proteins, rather than DNA, were carriers of genetic information (8, 12). Hershey and Chase established the role of DNA as a genetic information–encoding molecule in 1952 when they demonstrated that bacteriophage-infected bacterial cells ingest and transfer DNA rather than protein (13). At this time, DNA's primary role was understood, but little was known about how the DNA molecule was arranged. It was only known that its monomers (i.e., nucleotides) were present proportionately (14). The DNA double-helix structure was finally discovered by Watson and Crick (15). Despite this achievement, it would still be another 13 years before the genetic code was cracked, and another 25 years before the first DNA sequencing techniques were made accessible (16–18). Accordingly, DNA analysis using computational tools lagged ~2 decades behind the study of proteins, whose chemical makeup was already better understood than that of DNA (8).
Due to significant improvements in the crystallographic determination of protein structures (19), protein analysis was bioinformatics' starting point in Gauthier et al. (8). Insulin's sequence, or the arrangement of its amino acids, was the first protein sequence to be published (20). Additionally, numerous improvements in determining the structure and sequence of proteins were also reported (10, 21). The first bioinformatician was an American physical chemist named Margaret Dayhoff (1925–1983) who made significant contributions and used computational approaches in the study of biochemistry and protein sciences. She is referred to as the mother and father of bioinformatics (19, 20, 22).
Needleman and Wunsch created the first dynamic programming method for pairwise protein sequence alignment in 1978 (23). Since the early 1980s, multiple sequence alignment (MSA) algorithms have been emerging, facilitated by CLUSTAL software, which was introduced to MSA in 1988 (24, 25). Further, the concept of a mathematical framework for amino acid substitution was introduced by Dayhoff with the development of a point accepted mutation matrix (26). In the 1970s, DNA became more actively researched than proteins. Additionally, parallel developments in biology and computer science took place in the 1980 and 1990. Since the establishment of the National Center for Biotechnology Information (NCBI) in 1988 and the start of the Human Genome Project in 1990, bioinformatics has received significant attention and become an integral part of the analysis of the human genome (27–29). Further, bioinformatics emerged as a separate interdisciplinary subject for research and development in different areas of science and technology (10). Its approaches are extensively utilized in biomedical and pharmaceutical research. In recent years, the veterinary science community has sought to use these approaches in their research. Therefore, the concept of vetinformatics has been introduced as a branch of bioinformatics that focuses on livestock animals for quality veterinary services (5, 30).
In veterinary science, the livestock production system is a complex process that has three interconnected basic components: animal biology, the environment, and management techniques (31). Therefore, vetinformatics approaches are required to bridge the gaps between genotype and phenotype in order to improve livestock productivity and sustainability (5, 30). Large animal datasets have been produced as a result of improvements in various omics platforms and next-generation sequencing (NGS) technologies. Several bioinformatics databases and tools are available for their management and analysis, but these databases hold information about diverse groups of organisms (7, 10), and veterinarians require species-specific databases. Additionally, they require animal-based vetinformatics tools for data analysis and integration, as well as computational and mathematical models for analyzing animal behavior (32, 33). Accordingly, vetinformatics is required as a separate interdisciplinary subject to handle livestock data. By analyzing these large data sets, it is possible to accelerate research and development by extracting crucial information that enables researchers to understand livestock systems at molecular levels (Figure 1).
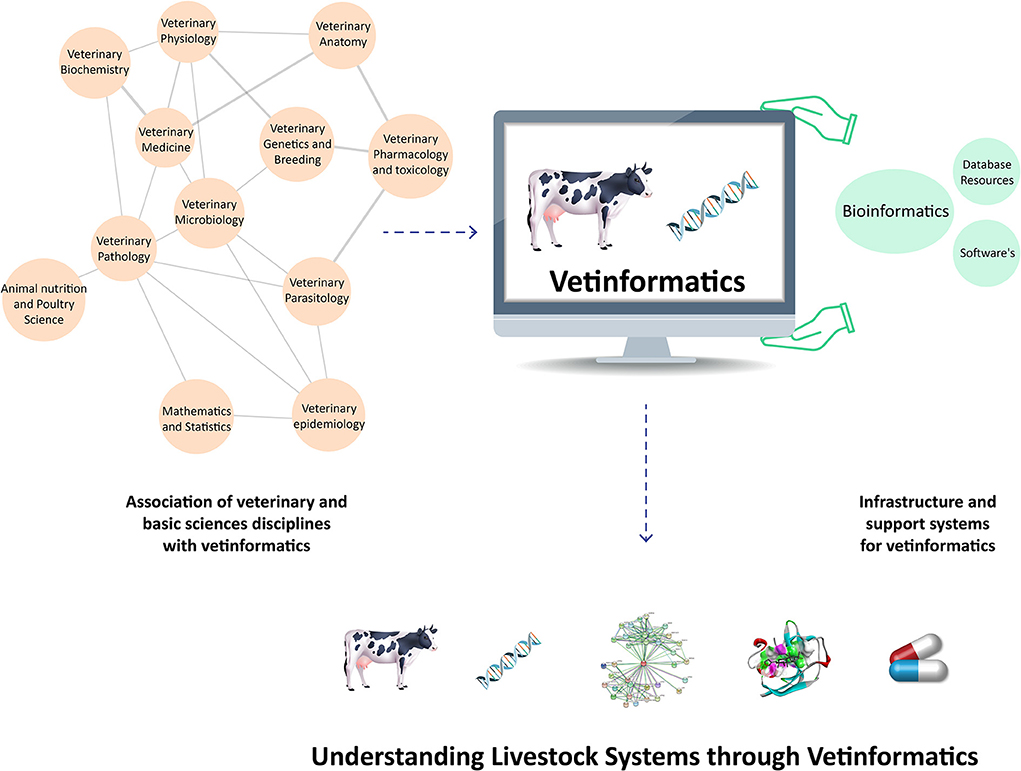
Figure 1. Integration of veterinary sciences, basic science disciplines, and support systems to create vetinformatics, enabling a better understanding of livestock systems in veterinary science.
Scientific disciplines linked with vetinformatics and their support systems
Vetinformatics is associated with the disciplines of veterinary sciences, basic sciences, and engineering; these disciplines provide infrastructure and an interdisciplinary nature to vetinformatics (5, 30, 33, 34). Several traditional and advanced subjects are associated with vetinformatics, such as veterinary physiology, biochemistry, anatomy, pharmacology and toxicology, parasitology, microbiology, pathology, epidemiology, genetics and breeding, and medicine, as well as animal nutrition and poultry science. Mathematical and statistical sciences also contribute to vetinformatics as basic-science disciplines. Computer science, information technology (IT), and computational resources serve as the foundation and support system for vetinformatics (5, 34). Accordingly, vetinformatics is created through the integration of veterinary sciences, basic sciences, and supporting disciplines. Vetinformatics uses computer science and IT to quickly provide solutions to complex challenges related to livestock systems (https://www.frontiersin.org/research-topics/33198/vetinformatics-an-insight-for-decoding-livestock-systems-through-in-silico-biology).
Needs and aims of vetinformatics
In order to better understand livestock systems, vetinformatics is expanding and has contributed to the growth of research initiatives involving high-throughput DNA sequencing data analysis and other omics fields (https://www.veterinaryirelandjournal.com/ucd-research/165-how-omics-are-contributing-to-sustainable-animal-production; accessed on 14/7/2022). Vetinformatics' aim is to decode the enormous quantity of multi-omics data produced by high-throughput technologies, structural and functional characterizations of key genes and proteins, and visualizations of key components linked with livestock productivity and sustainability (7).
In general, the goals of vetinformatics include building databases that document information on medicinal plants, particularly for the development of herbal veterinary medicines via screening of phytochemicals against molecular drug targets using molecular docking (5, 6, 35). However, vetinformatics' aims also include collecting animal genetic resources in databases, developing these databases for managing omics data sets that are species- or organism-specific, and enhancing the content of existing veterinary databases so that they can be used more effectively (36–38). In addition, developing platform-independent graphical user interface–based software for integration and analysis of multi-omics data (10, 32–34), and improving the accessibility of public software for veterinary biotechnologists, scientists, and veterinarians would further vetinformatics' missions. Finally, vetinformatics also strives to educate undergraduate students, graduate students, and faculty of veterinary and animal sciences about the use of vetinformatics for the analysis of multi-omics data [(32, 34) https://www.frontiersin.org/research-topics/33198/vetinformatics-an-insight-for-decoding-livestock-systems-through-in-silico-biology].
Recent advances in vetinformatics
The integration of omics science and technology with veterinary science opens exciting opportunities to decode livestock systems (7, 36, 39). Many animal genomes have been sequenced, and others are currently under sequencing and analysis. Although multi-omics data are generated regularly, more research is still needed to increase the efficiency and standardize interpretation, analysis, and integration of these data (7, 40). Additionally, the availability of big data in veterinary science has helped in the design of innovative algorithms and improved knowledge of cellular and phenotypic mechanisms [(7) https://ivcjournal.com/ai-the-newest-tool-in-veterinary-science/; accessed July 14, 2022]. Some animal-specific databases have already been developed to provide updated information to the veterinary science community (4, 37). Further, the use of machine and deep learning approaches in livestock research is reshaping the field in unanticipated ways. Exciting, cutting-edge models that connect genotype and phenotype allow the field of vetinformatics to grow quickly in the digital era and improve livestock productivity (41, 42).
Challenges in vetinformatics
Vetinformatics is connected to veterinary sciences, basic sciences, and technology. Due to its interdisciplinary nature, vetinformatics may include people with a background in veterinary science or biology with little interest in computer programming, or people with a background in computer science who are unfamiliar with certain biological concepts. Due to the importance and application of vetinformatics in livestock research, many post-graduate programs in veterinary and animal science require exposure to the subject of vetinformatics. These programs may develop student interest in this emerging and interdisciplinary field, filling an urgent need for more researchers in vetinformatics. The major challenges faced by vetinformatics include managing big data in veterinary sciences; developing species-specific databases and tools for livestock research; improving the accuracy of available tools; developing novel algorithms and tools; analyzing and integrating multi-omics data; and identifying molecules for the development of drugs for treating livestock diseases (43, 44).
Applications of vetinformatics in health, productivity, and sustainability of livestock
The scientific community produces complex data daily by using advanced molecular biology and biotechnology-based techniques (45, 46). These techniques require statistical approaches to quickly and accurately interpret these large-scale data (7, 40). Computational studies are the only method for analysis and interpretation of genome sequencing, assembly and alignment, differentially expressed genes, biological networks, protein modeling as well as molecular docking. The integration of such data is made possible by statistical and mathematical modeling approaches (5, 6, 10, 47, 48). Vetinformatics has tremendous potential to address challenging issues in veterinary science and related fields. Today, it is a vital tool for scientists and is crucial to the study of livestock. The following sections highlight the applications of vetinformatics.
Assembly and annotation of newly sequenced genomes
Sequencing an animal's genome is necessary to understand the intricacy among them (49, 50). Aligning and combining fragments of genome sequences obtained from sequencing platforms is referred to as assembly. Depending on whether a reference genome is available or not, assembly can be divided into two categories: de novo assembly and reference-based assembly (50, 51). Genome assembly is essential for determining how gene structure and function will affect an organism's behavior. SPAdes is a highly cited genome assembler originally designed for small genomes. It was tested on microorganisms including bacteria, fungi, and other small genomes (52). Besides, it includes various assembly pipelines such as metaSPAdes, plasmidSPAdes, rnaSPAdes, truSPAdes, and dipSPAdes. These pipelines are useful for metagenomic data sets, assembly of plasmids from WGS data, de novo assembly of RNA-Seq data, barcode assembly, and highly polymorphic diploid genomes (https://cab.spbu.ru/files/release3.12.0/manual.html). In the field of genomics, annotating genomes through MAKER is convenient and easy. Genomes of eukaryotes and prokaryotes can be annotated independently and genome databases can be created using it. It is designed to identify repeats, align ESTs and proteins with the genomes, and produce ab-initio gene predictions (53).
Using high-throughput sequencing platforms, we now have sequenced genomes for many major animal species (54, 55). Zimin et al. (56) used a combination of whole-genome shotgun sequencing and hierarchical sequencing techniques to sequence the genome of the domestic cow (Bos taurus). They assembled the 35 million sequence reads to produce an improved assembly of 2.86 billion base pairs. Numerous computational tools can be used to further evaluate sequenced genomes. Several pipelines, resources, and software are available for computational assembly and study of the genome. Recent functional annotation of three domestic animal genomes (cattle, chicken, and pig) provides a useful resource for livestock research (57). The study of the genome is pertinent to many areas of research, including ancestry determination, genomic selection, and vaccine and drug design (58–60).
Transcriptome and RNA-Seq data analysis for studying gene expression
RNA-Seq has emerged as an effective approach for transcriptome analyses that will eventually make microarrays outdated for analysis of gene expression data (61). The field of research on gene expression has undergone a recent revolution. This technology has made possible the measure of simultaneous gene expression, enabling the discovery of candidate genes with potential biological significance (62). RNA-Seq analysis of porcine ovaries revealed 4,414 deferentially expressed genes and helped to discover their roles in the late metestrus and diestrus phases of the estrous cycle (48). The findings from a separate transcriptome analysis strongly suggested that IGF1, PGR, ITPR1, and CHRM3 regulate oocyte maturation and smooth muscle contraction in pigs, and provided direction for future research involving effective animal breeding programs (63).
Non-coding RNAs such as small interfering RNAs (siRNAs) and microRNAs (miRNA) play vital roles in gene regulation (64). Recent investigations have demonstrated that they are effective in treating a variety of diseases, and working as a biomarker for effective therapies (64–66). The role of miRNAs has been examined in several studies with respect to livestock diseases (66). Several candidate genes and miRNAs have been identified that could be helpful in treating mastitis disease in cows (67, 68). Vetinformatics-based approaches are useful in detection of siRNAs in host and their targets in pathogen genomes, leading to the development of novel treatments against livestock diseases (64, 69).
Metagenomic analysis for dissecting microbial communities and their role in livestock
Metagenomics allows for direct access to genetic information of whole communities by utilizing a variety of genomic technologies and computational approaches (39). It presents a considerably more comprehensive description than phylogenetic surveys because it enables access to the functional gene composition of microbial communities. Metagenomics provides information on potentially novel enzymes or biocatalysts, relationships between phylogeny and function for uncultured organisms, and evolutionary profiles of community structure and function (39). Kumar et al. (70) analyzed metagenomic data of bacterial communities in pig slurries, enhancing knowledge of how microbial abundance in swine slurries varies over time. Another microbiome analysis characterized changes in microbial community composition that resulted from feeding dairy cows one of two common diets: pasture and total mixed ration. Studies such as this one will contribute to the management of cattle feed and the study of rumen microbial ecology (71). On larger scales, metagenomics-based analyses will help to improve animal health, leading to enhanced livestock productivity and sustainability.
Sequence alignment and analysis for identification of biologically significant regions
With the availability of the BLAST tool beginning in 1990, sequence analysis has emerged as a key area of research (72). The field of sequence analysis is fairly broad, but in this section, we will focus on the analysis of nucleotide or protein sequences. A variety of sequence-alignment tools such as BLAST, FASTA, and Muscle are available to identify or compare two (pair-wise alignment) or more (multiple sequence alignment; MSA) sequences (10). Ajayi et al. (73) identified 67 genes in the bovine genome belonging to heat shock protein families using sequence alignment and analysis. Using in silico analysis, the study investigated transcription start sites and promoter regions of olfactory receptors in cattle, identifying five candidate motifs (MOR1, MOR2, MOR3, MOR4, and MOR5) important in gene regulation (74). It is also used to annotate recently discovered sequences, identify conserved and regulatory regions, and predict sequence physicochemical properties (10).
Molecular phylogeny for analyzing relationship among organisms
Another crucial area of research in vetinformatics is molecular phylogenetic analysis (75). Widely employed in evolutionary biology, molecular phylogenetic analysis can identify similarities between various animal sequences in order to infer their evolutionary relationship (76). Additionally, it facilitates the identification of critical elements in individual sequences and their association with other sequences, thereby playing an important role in drug and vaccine design (10, 76). In the field of molecular phylogeny, the Molecular Evolutionary Genetic Analysis (MEGA) (77) and PHYLIP (78) are well-known software. Besides, several other web-based tools are also available such as Clustal Omega (https://www.ebi.ac.uk/Tools/msa/clustalo/), MUSCLE (https://www.ebi.ac.uk/Tools/msa/muscle/), and T-Coffee (https://www.ebi.ac.uk/Tools/msa/tcoffee/) to perform multiple sequence alignment and building a phylogenetic tree using different methods. There has been increasing interest in reconstructing phylogenetic trees in biological science, and questions are being raised regarding the degree of confidence one should place in any given phylogenetic tree. The concept of bootstrapping and jackknifing was introduced to construct error-free phylogenetic tree (79). Phylogenetic analysis software like MEGA facilitates researchers to set a bootstrap value during phylogenetic tree reconstruction to confirm their accuracy (https://www.megasoftware.net/web_help_11/Bootstrap_Test_of_Phylogeny.htm). The Interactive Tree Of Life, i.e., iTOL (https://itol.embl.de/) and Tree View are highly cited tools facilitating phylogenetic tree visualization (80, 81). The bovine hepacivirus (BovHepV) of five positive samples that formed a separate branch from other BovHepV in a phylogenetic analysis conducted by Deng et al. based on the partial NS3 coding sequence (82). The findings suggested that these new BovHepV represent novel and emerging strains. Another study that conducted a molecular characterization and phylogenetic analysis of the lumpy skin disease virus (LSDV) that is circulating in northern Thailand revealed a relationship with other LSDVs. The LSDV that was isolated from northern Thailand shared genetic traits with the LSDVs that are currently circulating in China, Hong Kong, and Vietnam. This finding will be instrumental in developing disease control strategies against LSDVs (83).
Genome wide association study for identification of important genomic regions
Genome-wide association study, commonly known as GWAS, is a powerful approach used to identify genetic variants linked to increased likelihood of a certain disease or trait (84, 85). The approach requires examining a large number of individual genomes in search of genetic variants that are more prevalent in individuals with a particular disease or trait. Once such genetic variants have been discovered, they are often utilized to look for neighboring variants that directly contribute to the disease or trait (84, 85). An analysis of GWAS can be conducted using single-locus, and multi-locus models (86). The General linear model (GLM), Mixed linear model (MLM), Logistic mixed model (LMM), and Compressed mixed linear model (CMLM) are the single locus models (87–89), and multi-locus model includes Multilocus random SNP effect mixed linear models (mrMLM) and Fast multilocus random SNP effect efficient mixed-model association (FASTmrEMMA) (86, 90–92). The computer programs commonly used for GWAS include PLINK (93), GenABEL (94), GenAMap (95), and GEMMA (96). In addition, the genomic databases and genome browsers such as NCBI (https://www.ncbi.nlm.nih.gov/), Animal QTLdb (https://www.animalgenome.org/cgi-bin/QTLdb/index), NAGRP (https://www.animalgenome.org/), Ensembl (https://asia.ensembl.org/index.html), and UCSC (https://genome.ucsc.edu/) are valuable resources (86). Besides, the Genome Analysis Toolkit (GATK) pipeline is an important platform for high-throughput genomics data analysis (97). There are a variety of tools available through GATK, most of which are focused on discovering variants and used for genotyping (https://gatk.broadinstitute.org/hc/en-us). Uemoto et al. identified six significant quantitative trait loci for immune-related traits in pigs affected by mycoplasma pneumonia of swine using GWAS, revealing novel insights into the genomic elements influencing pig production, respiratory illness, and immune-related traits (98). Another GWAS-based study identified candidate genes for milk production traits in Korean Holstein cattle and individual birth weight traits in Korean Yorkshire pigs (99, 100). Therefore, GWAS-based approaches have potential to decode important and complex traits linked with livestock productivity.
Systems biology and integration of multi-omics data
Systems biology is a key subfield of vetinformatics and has made great contributions to the modeling and simulation of biological systems (101–103). The field aids in the integration of multi-omics data, including genomics, proteomics, metabolomics, and transcriptomics, in order to construct models that comprehensively characterize the behavior of biological systems under various conditions (104). In the past, researchers were forced to focus on single genes or proteins, but as omics technology and systems biology have advanced, the paradigm has changed from a reductionist approach to a holistic approach (104). Through network modeling and analysis, systems biology enables prediction of the behavior of whole systems and identification of essential components involved in various biological processes, both of which ultimately contribute to advancements in animal welfare and livestock productivity (101–104).
Network biology and analysis
In network analysis, networks represent relationships among the components of a given system (104, 105). In biological systems, these relationships have attracted significant attention in recent years, founding the new interdisciplinary area called “network biology” (105). In network biology, biological systems are illustrated in the form of nodes and edges (105). Different types of networks such as signal transduction networks, protein-protein interaction networks, gene regulatory networks, and metabolic networks contain complex information about their relevant systems (104, 105). Nodes can represent genes, proteins, or metabolites, while edges represent interactions or relationships, according to the type of network (104). Network biology approaches are highly useful in investigations of hub nodes and drug targets, as well as identification of key components involved in regulating biological systems (47, 104, 106, 107).
Protein structure modeling, visualization, and validation
It was once challenging to predict a protein's 3D structure from its amino acid sequence. Now, these predictions are facilitated by improvements in protein structure prediction methods as well as the development of AlphaFold, a deep learning–based tool for protein structure modeling (108–110). When the target protein structure cannot be elucidated by experimental techniques, computational approaches become extremely important (110). These approaches can be used to predict protein structure, and the predicted structure can be utilized in drug screening. Additionally, computational approaches are used for predicting protein-protein interactions, structural comparison, and alignment (110, 111). In addition, several tools have been developed for visualization, refinement, and validation of the predicted 3D protein model. PyMOL is most often used tool for visualization, while Swiss PDB viewer, Rampage, PROCHECK, and Structural Analysis and Verification Server are extensively used for evaluation and model validation (109, 112, 113). With use of these tools, we can improve the quality of predicted models for further research (https://saves.mbi.ucla.edu/). Pan et al. predicted the cow milk 3D structures of αs1-CA and β-CA using I-TASSER to understand its dynamics (114). Additional research has modeled protein structure using computational approaches for livestock therapeutics development (115–117).
Binding site prediction
In drug discovery and design, binding site prediction is a crucial and significant step. A protein's 3D structure must be understood to identify amino acid residues present in the binding site. In order to learn more about the binding site and other sites, such as allosteric sites, computational tools are available to measure the area and volume of cavities in proteins (110). In vetinformatics, precise knowledge of the binding site is required to elucidate receptor–ligand interactions. Some molecular modeling and docking software packages offer the capability to predict and define the binding site prior to the docking simulation. Additionally, some web-based tools like CASTp and COACH are used for binding site predictions (118, 119).
Drug discovery and design for the management of livestock disease
The emergence of novel diseases decreases livestock productivity and represents a pressing challenge in the field of veterinary science. Effective treatments are unavailable for many diseases (6). Therefore, there is an urgent need to use vetinformatics approaches to identify novel lead molecules for drug development (5). In the process of developing new drugs, computational methods act as a valuable resource (110, 120). Finding a small molecule that can geometrically and chemically fit in a cavity of a macromolecular target is the aim of computer-assisted drug discovery programs (109, 110). Recent developments in computational approaches have facilitated the estimation of receptor–ligand binding energy through molecular docking simulations, prediction of pharmacokinetics and pharmacodynamics, and optimization of lead molecules (121). Due to advancements in computer power and algorithms, the field of drug discovery and design has achieved significant progress. For developing models and tools for drug discovery and design, computational methods including the hidden markov model, artificial neural networks, support vector machines, and genetic algorithms are frequently employed (109, 110, 121). In order to accelerate the drug development process, several issues have been solved, leading to a major improvement in these approaches and tools to reduce the time and cost of drug discovery programs (5, 30, 109). Several approaches and methods that play significant roles in veterinary drug discovery programs are highlighted in the following sections (Figure 2).
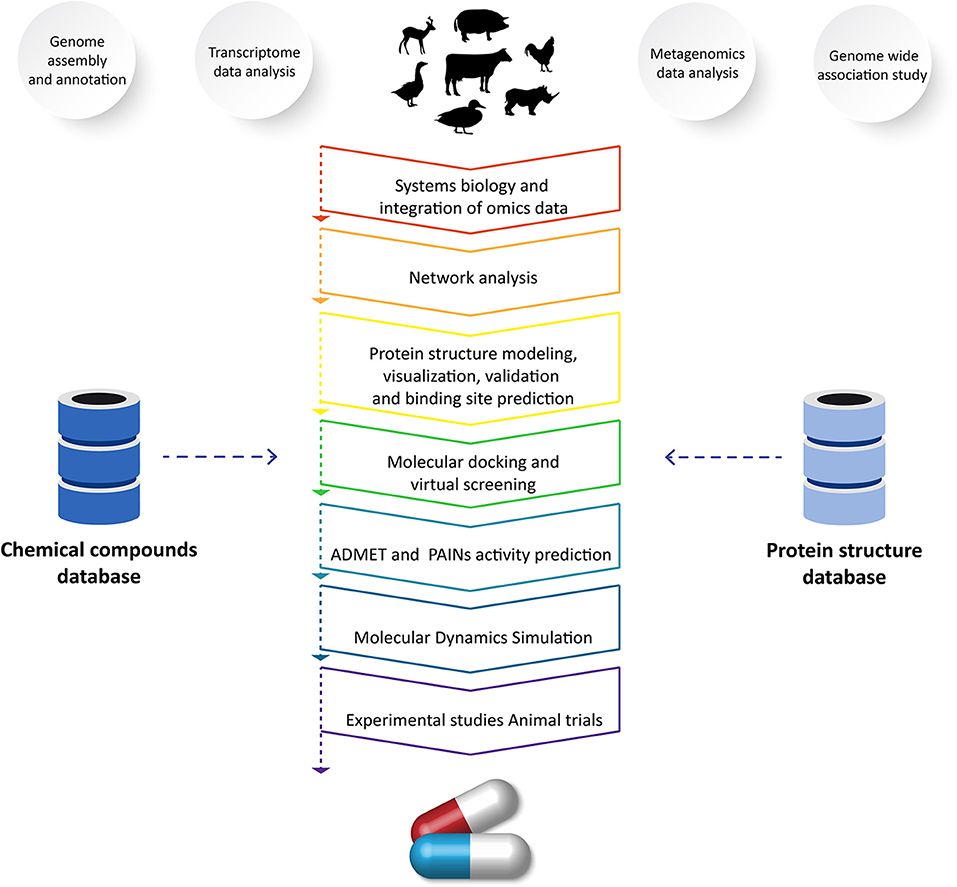
Figure 2. Application of vetinformatics to analyze high-throughput sequencing data for discovery of novel drug molecule(s) for veterinary application.
Molecular docking and virtual screening for identification of lead compounds
Recent developments in computational approaches have made it possible to predict molecular receptor–ligand interactions in the bound or complex state with perfect accuracy (110, 122, 123). To predict the interaction of small compounds with macromolecular targets, software such as AutoDock, AutoDock Vina, Glide, and Discovery studio are available (109). These programs can be used to screen a wide range of prospective compounds, look for new compounds with specific binding properties, or test available medicines with functional group alterations using molecular docking and virtual screening (109, 110, 122, 124). Recently, in silico studies predicted the lead compounds for drug development against porcine reproductive and respiratory syndrome virus (PRRSV) via the screening of 97,999 natural compounds from the ZINC database (6). The compounds 7-deacetyl-7-oxogedunin, kulactone, and nimocin were also identified as potential multi-target leads for the inhibition of porcine CD163 scavenger receptor cysteine-rich domain 5 (CD163-SRCR5), as well as non-structural protein 4 (Nsp4) and Nsp10 of PRRSV (5). The inhibitors of the imidazole glycerophosphate dehydratase protein in Staphylococcus xylosus were also identified through virtual screening (117).
ADMET and PAINs activity prediction of lead compounds
The primary criteria for sorting ligands in drug discovery programs involve its absorption, distribution, metabolism, excretion, and toxicity prediction, or ADMET (109, 121). These criteria act as a fundamental standard for testing candidate molecules. It is widely believed that every drug discovery program should consider these criteria, or Lipinski's rule of five, to evaluate orally active drugs (123, 125). In the early stages of the drug discovery process, abiding by these criteria is crucial for finding the most appropriate drug-like compounds, and it considerably reduces the late-stage failure of candidate molecules during preclinical or clinical trials (109, 110). Additionally, we can filter molecules that are related to pan-assay interference compounds (PAINS). Instead of directly affecting a specific target, PAINS typically respond non-specifically with many biological targets. In order to prevent non-specific binding and toxicity, a filter should be used (126, 127). Therefore, it is recognized as a cost-effective and time-saving approach in veterinary drug discovery program (5, 109, 110).
Pharmacophore and quantitative structure–activity relationship modeling
A pharmacophore is a molecular framework containing essential features of a drug's active component. Pharmacophore modeling is extensively used in the development of novel compounds (109, 110, 128). It can be used to represent and distinguish molecules at a 2D or 3D level by schematically illustrating the essential components of molecular recognition (110, 123). Relatedly, quantitative structure–activity relationship modeling is a widely used drug discovery approach that utilizes a molecule's physicochemical properties to predict its biological activity (110, 129). Both of these approaches can be used to find novel treatments for livestock diseases (5, 30).
Molecular dynamics simulation of proteins and protein–ligand complexes to determine their dynamics and behavior during interactions
Molecular dynamics simulation is used to computationally visualize the movement and behavior of a molecular system at the atomic level (110, 130). It offers a wealth of knowledge regarding the interactions between proteins and ligands and provides complex structural information on macromolecular structures (109, 110). This knowledge is crucial for understanding the structure–function relationship among the target and its dynamics during protein–ligand interactions, ultimately supporting drug discovery processes (109). As a result, it is widely utilized to characterize protein–ligand interactions in modern drug discovery programs (6). Additionally, it is used to validate predicted protein models, understand the dynamics of protein folding and unfolding and protein–ligand dynamics, examine the effects of mutations on structures, and understand binding dynamics at other sites (5). A recent study described the role of DGAT1 missense non-synonymous single nucleotide polymorphisms (SNPs) in dairy cattle using computational approaches. The DGAT1 variants (W128R, W214R, C215G, P245R, and W459G) were analyzed initially through sequence- and structure-based tools, then evaluated using molecular dynamics simulation to understand their structural and conformational dynamics compared to wild-type structures and improve milk quality in cattle (47).
Designing vaccines for livestock diseases
Emerging pathogens are a major threat to livestock productivity that requires the identification of vaccine candidates in order to ensure long-term protection of animals (33, 131). In order to provide broad-spectrum and long-term protection against different viral and bacterial diseases, new approaches to vaccine development must be created (10, 132). In the post-genome era, identifying specific antigenic regions to activate certain arms of the immune system was a major challenge (115, 133). To address this issue, computational vaccine design has been a major area of interest for researchers over the last two decades. Several tools and web-based resources have been developed that have proven useful in vaccine design (133, 134). Researchers can now utilize advanced vetinformatics approaches to design vaccines that provide protection against livestock diseases (33, 115, 131).
Machine and deep learning approaches in livestock research
Machine and deep learning approaches have received significant attention from veterinary scientists (135, 136). Computers are equipped with an adaptive mechanism that enables them to learn from examples and experiences (137). Machine and deep learning provide information-processing capabilities for handling various types of real-life information (137). In order to make predictions or conclusions about target datasets, these algorithms often build mathematical models using sample datasets, also referred to as training datasets (137, 138). The recent advancements in artificial intelligence have made it even easier to analyze animal behavior in videos using machine vision and machine learning (139). The development of predictive models such as Convolutional Neural Network (CNN) and Long Short-Term Memory (LSTM) based on modern machine techniques are helpful in livestock research (139, 140). It was shown that the development of a recurrent neural network (RNN) model with an LSTM could classify cattle behavior in a reasonable manner (141). Recently, CNN and Bidirectional Long Short-Term Memory (BiLSTM) were used for video-based identification of individual cattle (140), and C3D-ConvLSTM (Convolutional 3D- Convolutional Long Short-Term Memory) based model was used for cow behavior classification over 86% accuracy (142).
Enabled by advances in omics, an enormous amount of biological data is produced every day, and these large data sets allow researchers to build machine learning models in order to make relevant predictions and minimize the expense and duration of experiments (137, 138, 143). These approaches play important roles in different areas of vetinformatics, such as gene discovery and genome annotation, gene expression analysis, drug target prediction, protein modeling, drug discovery, text mining, digital image processing, and helpful in precision livestock farming (137, 138, 143).
Development of databases and tools for vetinformatics
Databases and tools related to veterinary science are essential for computer-based examinations of livestock data (30, 32, 33). Several databases and tools are available, but most databases contain information about many organisms (10) (Table 1). Due to recent developments in the area of vetinformatics, some animal-specific databases have been developed in recent years, but their availability is still insufficient (36–38). In the post-genomic era, large multi-omics data sets about livestock animals are urgently needed to develop species-specific databases to support veterinary science. Species-specific databases would help veterinary researchers easily find information about target animals. In addition, the availability of multi-omics data will help to improve the prediction, development, and accuracy of new algorithms that solve problems related to animal breeding, develop disease diagnostics, and offer solutions that increase livestock productivity and sustainability (138, 159–161). Some of the important software used for livestock research is highlighted in Table 2.
Future perspectives on vetinformatics
Since the beginning of the human genome project, the use of computers in biology has drawn significant interest and it is currently an essential tool in biological research. In the twenty-first century, it is difficult to imagine a novel discovery that does not rely on computational methods. Because computer software has been involved in most biological studies worldwide in the current omics era, many top research groups believe that integration of computers with biology has immense potential to decode complex biological systems, enabling the discovery of novel therapeutics and other useful information for the betterment of society. Therefore, vetinformatics will eventually become a crucial component of every veterinary science research lab. The management of big data in biology and veterinary science will also demand vetinformatics experts, who will reduce experimental work load and expense. As the human population grows, requiring commensurate increases in food production, it will be necessary to increase livestock productivity, advance animal breeding programs, improve the nutritional quality of animal products, and develop disease prevention and management strategies for animal welfare. This can be accomplished with the help of vetinformatics approaches that visualize the complexity of livestock systems in order to design solutions that meet our demands for higher livestock productivity.
Conclusion
In recent years, vetinformatics has emerged as a vital subject and a popular interdisciplinary research area in veterinary sciences. The strength of vetinformatics and the ability of its methods to tackle challenging projects in veterinary sciences were highlighted in this review. Databases and other tools available for livestock research, along with their applications and availability, were also included. Vetinformatics approaches have proven their ability to resolve a variety of problems in veterinary science. To develop vetinformatics tools and databases that successfully target livestock systems for quality veterinary services, more resources need to be developed. Therefore, a conversation is needed in the veterinary science community that encourages the implementation of vetinformatics to understand livestock systems for the enhancement of animal welfare and drug discovery.
Author contributions
J-MK and RKP developed the idea for this review article and its coverage. RKP wrote the manuscript. J-MK supervised the work and edited the manuscript. Both authors have read the final manuscript and approved the submission.
Funding
This work was supported by the National Research Foundation of Korea (NRF) grant funded by the Korean government (MSIT) (NRF-2022R1A2C1005830).
Acknowledgments
The authors thank Chung-Ang University, Anseong-si, Republic of Korea for providing high-performance computing and other necessary facilities.
Conflict of interest
The authors declare that the research was conducted in the absence of any commercial or financial relationships that could be construed as a potential conflict of interest.
Publisher's note
All claims expressed in this article are solely those of the authors and do not necessarily represent those of their affiliated organizations, or those of the publisher, the editors and the reviewers. Any product that may be evaluated in this article, or claim that may be made by its manufacturer, is not guaranteed or endorsed by the publisher.
References
1. Grandin T. Introduction: the contribution of animals to human welfare. Rev Sci Tech. (2018) 37:15–35. doi: 10.20506/rst.37.1.2737
2. Randolph TF, Schelling E, Grace D, Nicholson CF, Leroy JL, Cole DC, et al. Invited review: role of livestock in human nutrition and health for poverty reduction in developing countries. J Anim Sci. (2007) 85:2788–800. doi: 10.2527/jas.2007-0467
3. Dupjan S, Dawkins MS. Animal welfare and resistance to disease: interaction of affective states and the immune system. Front Vet Sci. (2022) 9:929805. doi: 10.3389/fvets.2022.929805
4. Rexroad C, Vallet J, Matukumalli LK, Reecy J, Bickhart D, Blackburn H, et al. Genome to phenome: improving animal health, production, and well-being - a new usda blueprint for animal genome research 2018-2027. Front Genet. (2019) 10:327. doi: 10.3389/fgene.2019.00327
5. Pathak RK, Kim D-Y, Lim B, Kim J-M. Investigating multi-target antiviral compounds by screening of phytochemicals from neem (Azadirachta indica) against PRRSV: a vetinformatics approach. Front Vet Sci. (2022) 9:854528. doi: 10.3389/fvets.2022.854528
6. Pathak RK, Seo YJ, Kim JM. Structural insights into inhibition of Prrsv Nsp4 revealed by structure-based virtual screening, molecular dynamics, and Mm-Pbsa studies. J Biol Eng. (2022) 16:4. doi: 10.1186/s13036-022-00284-x
7. Kim DY, Kim JM. Multi-Omics integration strategies for animal epigenetic studies - a review. Anim Biosci. (2021) 34:1271–82. doi: 10.5713/ab.21.0042
8. Gauthier J, Vincent AT, Charette SJ, Derome N. A brief history of bioinformatics. Brief Bioinform. (2019) 20:1981–96. doi: 10.1093/bib/bby063
9. Hogeweg P. The roots of bioinformatics in theoretical biology. PLoS Comput Biol. (2011) 7:e1002021. doi: 10.1371/journal.pcbi.1002021
10. Pathak RK, Singh DB, Singh R. Introduction to basics of bioinformatics. In:Singh DB, Pathak RK, editors. Bioinformatics. Academic Press Elsevier (2022). p. 1–15. doi: 10.1016/B978-0-323-89775-4.00006-7
11. Avery OT, MacLeod CM, McCarty M. Studies on the chemical nature of the substance inducing transformation of pneumococcal types: induction of transformation by a desoxyribonucleic acid fraction isolated from pneumococcus type III. J Exp Med. (1944) 79:137–58.
12. Griffiths JF, Griffiths AJ, Wessler SR, Lewontin RC, Gelbart WM, Suzuki DT, et al. An Introduction to Genetic Analysis. Macmillan (2005). Available online at: https://store.macmillanlearning.com/us/product/Introduction-to-Genetic-Analysis/p/1319114784
13. Hershey AD, Chase M. Independent functions of viral protein and nucleic acid in growth of bacteriophage. J Gen Physiol. (1952) 36:39–56. doi: 10.1085/jgp.36.1.39
14. Tamm C, Shapiro HS, Lipshitz R, Chargaff E. Distribution density of nucleotides within a desoxyribonucleic acid chain. J Biol Chem. (1953) 203:673–88. doi: 10.1016/S0021-9258(19)52337-7
15. Watson JD, Crick FH. Molecular Structure of Nucleic Acids: A Structure for Deoxyribose Nucleic Acid. Nature. (1974) 248:765. doi: 10.1038/248765a0
16. Nirenberg M, Leder P. Rna codewords and protein synthesis: the effect of trinucleotides upon the binding of srna to ribosomes. Science. (1964) 145:1399–407. doi: 10.1126/science.145.3639.1399
17. Sanger F, Nicklen S, Coulson AR. DNA sequencing with chain-terminating inhibitors. Proc Natl Acad Sci USA. (1977) 74:5463–7. doi: 10.1073/pnas.74.12.5463
18. Maxam AM, Gilbert W. A new method for sequencing DNA. Proc Natl Acad Sci USA. (1977) 74:560–4. doi: 10.1073/pnas.74.2.560
19. Jaskolski M, Dauter Z, Wlodawer A. A brief history of macromolecular crystallography, illustrated by a family tree and its nobel fruits. FEBS J. (2014) 281:3985–4009. doi: 10.1111/febs.12796
20. Sanger F, Thompson EO. The amino-acid sequence in the glycyl chain of insulin. I. The identification of lower peptides from partial hydrolysates. Biochem J. (1953) 53:353–66. doi: 10.1042/bj0530353
22. Moody G. Digital Code of Life : How Bioinformatics Is Revolutionizing Science, Medicine, and Business. Hoboken, NJ: Wiley (2004) 389 p.
23. Needleman SB, Wunsch CD. A general method applicable to the search for similarities in the amino acid sequence of two proteins. J Mol Biol. (1970) 48:443–53. doi: 10.1016/0022-2836(70)90057-4
24. Higgins DG, Sharp PM. Clustal: a package for performing multiple sequence alignment on a microcomputer. Gene. (1988) 73:237–44. doi: 10.1016/0378-1119(88)90330-7
25. Ranwez V, Chantret N. Strengths and Limits of Multiple Sequence Alignment and Filtering Methods (2020). Available online at: https://hal.archives-ouvertes.fr/hal-02535389/document
26. Dayhoff M, Schwartz R, Orcutt B. A model of evolutionary change in proteins. In:Dayhoff M, editor. Atlas of Protein Sequence and Structure (1972). Available online at: https://chagall.med.cornell.edu/BioinfoCourse/PDFs/Lecture2/Dayhoff1978.pdf
27. Chial H. DNA sequencing technologies key to the human genome project. Nat Educ. (2008) 1:219. Available online at: https://www.nature.com/scitable/topicpage/dna-sequencing-technologies-key-to-the-human-828/
28. Hood L, Rowen L. The human genome project: big science transforms biology and medicine. Genome Med. (2013) 5:79. doi: 10.1186/gm483
29. Coordinators NR. Database resources of the national center for biotechnology information. Nucleic Acids Res. (2015) 43:D6–17. doi: 10.1093/nar/gku1130
30. Sujatha P, Kumarasamy P, Preetha S, Balachandran P. Vetinformatics: a new paradigm for quality veterinary services. Res Rev J Vet Sci Technol. (2018) 5:16–9. doi: 10.37591/rrjovst.v5i2.537
31. Tiwary BK. Farm animal informatics. In:Tiwary BK, editor. Bioinformatics and Computational Biology. Singapore: Springer (2022). p. 203–18. doi: 10.1007/978-981-16-4241-8_11
32. Hardy T. Animal bioinformatics. EJBI. (2021) 17:9–10. Available online at: https://www.ejbi.org/scholarly-articles/animal-bioinformatics-8691.html
33. Kaikabo A, Kalshingi H. Concepts of bioinformatics and its application in veterinary research and vaccines development. Nigerian Vet J. (2007) 28:39–46. doi: 10.4314/nvj.v28i2.3554
34. Byrne C, Logas J. The future of technology and computers in veterinary medicine. Diagnost Ther Vet Dermatol. (2021) 245–50. doi: 10.1002/9781119680642.ch26
35. Hahnke VD, Kim S, Bolton EE. Pubchem chemical structure standardization. J Cheminform. (2018) 10:36. doi: 10.1186/s13321-018-0293-8
36. Dawson HD, Chen C, Gaynor B, Shao J, Urban JF Jr. The porcine translational research database: a manually curated, genomics and proteomics-based research resource. BMC Genomics. (2017) 18:643. doi: 10.1186/s12864-017-4009-7
37. Shamimuzzaman M, Le Tourneau JJ, Unni DR, Diesh CM, Triant DA, Walsh AT, et al. Bovine genome database: new annotation tools for a new reference genome. Nucleic Acids Res. (2020) 48:D676–81. doi: 10.1093/nar/gkz944
38. Yang W, Yang Y, Zhao C, Yang K, Wang D, Yang J, et al. Animal-Imputedb: a comprehensive database with multiple animal reference panels for genotype imputation. Nucleic Acids Res. (2020) 48:D659–67. doi: 10.1093/nar/gkz854
39. Thomas T, Gilbert J, Meyer F. Metagenomics - a guide from sampling to data analysis. Microb Inform Exp. (2012) 2:3. doi: 10.1186/2042-5783-2-3
40. Guillemin N, Horvatic A, Kules J, Galan A, Mrljak V, Bhide M. Omics approaches to probe markers of disease resistance in animal sciences. Mol Biosyst. (2016) 12:2036–46. doi: 10.1039/C6MB00220J
41. Ghosh S, Dasgupta R. Machine learning in the study of animal health and veterinary sciences. In:Ghosh S, Dasgupta R, editors. Machine Learning in Biological Sciences. Singapore: Springer (2022). p. 251–9. doi: 10.1007/978-981-16-8881-2_29
42. Ezanno P, Picault S, Beaunee G, Bailly X, Munoz F, Duboz R, et al. Research perspectives on animal health in the era of artificial intelligence. Vet Res. (2021) 52:40. doi: 10.1186/s13567-021-00902-4
43. Morrison-Smith S, Boucher C, Sarcevic A, Noyes N, O'Brien C, Cuadros N, et al. Challenges in large-scale bioinformatics projects. Hum Soc Sci Commun. (2022) 9:125. doi: 10.1057/s41599-022-01141-4
44. Soetan KO, Awosanya EA. Bioinformatics and its application in animal health: a review. Trop Vet. (2015) 33:3–22. Available online at: https://www.ajol.info/index.php/tv/article/view/160158
45. Li Q, Freeman LM, Rush JE, Huggins GS, Kennedy AD, Labuda JA, et al. Veterinary medicine and multi-omics research for future nutrition targets: metabolomics and transcriptomics of the common degenerative mitral valve disease in dogs. Omics. (2015) 19:461–70. doi: 10.1089/omi.2015.0057
46. Jianghong W, Li X, Xu X. Multi-Omics approaches to study complex traits in domestic animals. Front Syst Biol. (2021) 1:771644. doi: 10.3389/fsysb.2021.771644
47. Pathak RK, Lim B, Park Y, Kim JM. Unraveling structural and conformational dynamics of Dgat1 missense Nssnps in dairy cattle. Sci Rep. (2022) 12:4873. doi: 10.1038/s41598-022-08833-6
48. Park Y, Park YB, Lim SW, Lim B, Kim JM. Time series ovarian transcriptome analyses of the porcine estrous cycle reveals gene expression changes during steroid metabolism and corpus luteum development. Animals. (2022) 12:376. doi: 10.3390/ani12030376
49. Hotaling S, Kelley JL, Frandsen PB. Toward a genome sequence for every animal: where are we now? Proc Natl Acad Sci USA. (2021) 118:e2109019118. doi: 10.1073/pnas.2109019118
50. Baker M. De novo genome assembly: what every biologist should know. Nat Methods. (2012) 9:333–7. doi: 10.1038/nmeth.1935
51. Thrash A, Hoffmann F, Perkins A. Toward a more holistic method of genome assembly assessment. BMC Bioinformatics. (2020) 21:249. doi: 10.1186/s12859-020-3382-4
52. Bankevich A, Nurk S, Antipov D, Gurevich AA, Dvorkin M, Kulikov AS, et al. Spades: a new genome assembly algorithm and its applications to single-cell sequencing. J Comput Biol. (2012) 19:455–77. doi: 10.1089/cmb.2012.0021
53. Cantarel BL, Korf I, Robb SM, Parra G, Ross E, Moore B, et al. Maker: an easy-to-use annotation pipeline designed for emerging model organism genomes. Genome Res. (2008) 18:188–96. doi: 10.1101/gr.6743907
54. Taylor JF, Whitacre LK, Hoff JL, Tizioto PC, Kim J, Decker JE, et al. Lessons for livestock genomics from genome and transcriptome sequencing in cattle and other mammals. Genet Sel Evol. (2016) 48:59. doi: 10.1186/s12711-016-0237-6
55. Talenti A, Powell J, Hemmink JD, Cook EAJ, Wragg D, Jayaraman S, et al. A cattle graph genome incorporating global breed diversity. Nat Commun. (2022) 13:910. doi: 10.1038/s41467-022-28605-0
56. Zimin AV, Delcher AL, Florea L, Kelley DR, Schatz MC, Puiu D, et al. A whole-genome assembly of the domestic cow, bos taurus. Genome Biol. (2009) 10:R42. doi: 10.1186/gb-2009-10-4-r42
57. Kern C, Wang Y, Xu X, Pan Z, Halstead M, Chanthavixay G, et al. Functional annotations of three domestic animal genomes provide vital resources for comparative and agricultural research. Nat Commun. (2021) 12:1821. doi: 10.1038/s41467-021-22100-8
58. Bovo S, Schiavo G, Bolner M, Ballan M, Fontanesi L. Mining livestock genome datasets for an unconventional characterization of animal DNA viromes. Genomics. (2022) 114:110312. doi: 10.1016/j.ygeno.2022.110312
59. Xia XH. Bioinformatics and drug discovery. Curr Top Med Chem. (2017) 17:1709–26. doi: 10.2174/1568026617666161116143440
60. Rosen BD, Bickhart DM, Schnabel RD, Koren S, Elsik CG, Tseng E, et al. De novo assembly of the cattle reference genome with single-molecule sequencing. Gigascience. (2020) 9:giaa021. doi: 10.1093/gigascience/giaa021
61. Rao MS, Van Vleet TR, Ciurlionis R, Buck WR, Mittelstadt SW, Blomme EAG, et al. Comparison of Rna-Seq and microarray gene expression platforms for the toxicogenomic evaluation of liver from short-term rat toxicity studies. Front Genet. (2018) 9:636. doi: 10.3389/fgene.2018.00636
62. Wolf JB. Principles of transcriptome analysis and gene expression quantification: an Rna-Seq tutorial. Mol Ecol Resour. (2013) 13:559–72. doi: 10.1111/1755-0998.12109
63. Jang MJ, Lim C, Lim B, Kim JM. Integrated multiple transcriptomes in oviductal tissue across the porcine estrous cycle reveal functional roles in oocyte maturation and transport. J Anim Sci. (2022) 100:skab364. doi: 10.1093/jas/skab364
64. Lam JKW, Chow MYT, Zhang Y, Leung SWS. Sirna versus mirna as therapeutics for gene silencing. Mol Ther-Nucl Acids. (2015) 4:e252. doi: 10.1038/mtna.2015.23
65. Do DN, Dudemaine PL, Mathur M, Suravajhala P, Zhao X, Ibeagha-Awemu EM. Mirna regulatory functions in farm animal diseases, and biomarker potentials for effective therapies. Int J Mol Sci. (2021) 22:3080. doi: 10.3390/ijms22063080
66. Miretti S, Lecchi C, Ceciliani F, Baratta M. Micrornas as biomarkers for animal health and welfare in livestock. Front Vet Sci. (2020) 7:578193. doi: 10.3389/fvets.2020.578193
67. Li Z, Wang H, Chen L, Wang L, Liu X, Ru C, et al. Identification and characterization of novel and differentially expressed micro Rna S in peripheral blood from healthy and mastitis holstein cattle by deep sequencing. Anim Genet. (2014) 45:20–7. doi: 10.1111/age.12096
68. Chen Z, Zhou J, Wang X, Zhang Y, Lu X, Fan Y, et al. Screening candidate micror-15a-Irak2 regulatory pairs for predicting the response to staphylococcus aureus-induced mastitis in dairy cows. J Dairy Res. (2019) 86:425–31. doi: 10.1017/S0022029919000785
69. Dana H, Chalbatani GM, Mahmoodzadeh H, Karimloo R, Rezaiean O, Moradzadeh A, et al. Molecular mechanisms and biological functions of sirna. Int J Biomed Sci. (2017) 13:48–57. Available online at: https://www.ncbi.nlm.nih.gov/pmc/articles/PMC5542916/
70. Kumar H, Jang YN, Kim K, Park J, Jung MW, Park JE. Compositional and functional characteristics of swine slurry microbes through 16s Rrna metagenomic sequencing approach. Animals. (2020) 10:1372. doi: 10.3390/ani10081372
71. de Menezes AB, Lewis E, O'Donovan M, O'Neill BF, Clipson N, Doyle EM. Microbiome analysis of dairy cows fed pasture or total mixed ration diets. FEMS Microbiol Ecol. (2011) 78:256–65. doi: 10.1111/j.1574-6941.2011.01151.x
72. Altschul SF, Gish W, Miller W, Myers EW, Lipman DJ. Basic local alignment search tool. J Mol Biol. (1990) 215:403–10. doi: 10.1016/S0022-2836(05)80360-2
73. Ajayi OO, Peters SO, De Donato M, Sowande SO, Mujibi FDN, Morenikeji OB, et al. Computational genome-wide identification of heat shock protein genes in the bovine genome. F1000Res. (2018) 7:1504. doi: 10.12688/f1000research.16058.1
74. Samuel B, Dinka H. In silico analysis of the promoter region of olfactory receptors in cattle (Bos indicus) to understand its gene regulation. Nucleosides Nucleotides Nucleic Acids. (2020) 39:853–65. doi: 10.1080/15257770.2020.1711524
75. Quan JQ, Cai Y, Yang TL, Ge QY, Jiao T, Zhao SG. Phylogeny and conservation priority assessment of asian domestic chicken genetic resources. Glob Ecol Conserv. (2020) 22:e00944. doi: 10.1016/j.gecco.2020.e00944
76. Olvera A, Busquets N, Cortey M, de Deus N, Ganges L, Nunez JI, et al. Applying phylogenetic analysis to viral livestock diseases: moving beyond molecular typing. Vet J. (2010) 184:130–7. doi: 10.1016/j.tvjl.2009.02.015
77. Tamura K, Stecher G, Kumar S. Mega11: molecular evolutionary genetics analysis version 11. Mol Biol Evol. (2021) 38:3022–7. doi: 10.1093/molbev/msab120
78. Retief JD. Phylogenetic analysis using phylip. In:Misener S, Krawetz SA, editors. Bioinformatics Methods and Protocols. Humana Totowa, NJ: Springer (2000). p. 243–58. doi: 10.1385/1-59259-192-2:243
79. Soltis PS, Soltis DE. Applying the bootstrap in phylogeny reconstruction. Stat Sci. (2003) 18:256–67. doi: 10.1214/ss/1063994980
80. Letunic I, Bork P. Interactive tree of life (Itol) V5: an online tool for phylogenetic tree display and annotation. Nucleic Acids Res. (2021) 49:W293–6. doi: 10.1093/nar/gkab301
81. Page RD. Tree view: an application to display phylogenetic trees on personal computers. Bioinformatics. (1996) 12:357–8. doi: 10.1093/bioinformatics/12.4.357
82. Deng Y, Guan SH, Wang S, Hao G, Rasmussen TB. The detection and phylogenetic analysis of bovine hepacivirus in China. Biomed Res Int. (2018) 2018:6216853. doi: 10.1155/2018/6216853
83. Singhla T, Boonsri K, Kreausukon K, Modethed W, Pringproa K, Sthitmatee N, et al. Molecular characterization and phylogenetic analysis of lumpy skin disease virus collected from outbreaks in northern Thailand in 2021. Vet Sci. (2022) 9:194. doi: 10.3390/vetsci9040194
84. Uffelmann E, Huang QQ, Munung NS, De Vries J, Okada Y, Martin AR, et al. Genome-Wide association studies. Nat Rev Methods Prim. (2021) 1:1–21. doi: 10.1038/s43586-021-00056-9
85. Cheng J, Fernando R, Cheng H, Kachman SD, Lim K, Harding JCS, et al. Genome-Wide association study of disease resilience traits from a natural polymicrobial disease challenge model in pigs identifies the importance of the major histocompatibility complex region. G3. (2022) 12:jkab441. doi: 10.1093/g3journal/jkab441
86. Mkize N, Maiwashe A, Dzama K, Dube B, Mapholi N. Suitability of gwas as a tool to discover Snps associated with tick resistance in cattle: a review. Pathogens. (2021) 10:1604. doi: 10.3390/pathogens10121604
87. Chu BB, Keys KL, German CA, Zhou H, Zhou JJ, Sobel EM, et al. Iterative hard thresholding in genome-wide association studies: generalized linear models, prior weights, and double sparsity. Gigascience. (2020) 9:giaa044. doi: 10.1093/gigascience/giaa044
88. Zhang Z, Ersoz E, Lai CQ, Todhunter RJ, Tiwari HK, Gore MA, et al. Mixed linear model approach adapted for genome-wide association studies. Nat Genet. (2010) 42:355–60. doi: 10.1038/ng.546
89. Shenstone E, Cooper J, Rice B, Bohn M, Jamann TM, Lipka AE. An assessment of the performance of the logistic mixed model for analyzing binary traits in maize and sorghum diversity panels. PLoS ONE. (2018) 13:e0207752. doi: 10.1371/journal.pone.0207752
90. Wang SB, Feng JY, Ren WL, Huang B, Zhou L, Wen YJ, et al. Improving power and accuracy of genome-wide association studies via a multi-locus mixed linear model methodology. Sci Rep. (2016) 6:19444. doi: 10.1038/srep19444
91. Zhang YW, Tamba CL, Wen YJ, Li P, Ren WL, Ni YL, et al. Mrmlm V4.0.2: an R platform for multi-locus genome-wide association studies. Genomics Proteomics Bioinformatics. (2020) 18:481–7. doi: 10.1016/j.gpb.2020.06.006
92. Wen YJ, Zhang YW, Zhang J, Feng JY, Zhang YM. The improved fastmremma and Gcim algorithms for genome-wide association and linkage studies in large mapping populations. Crop J. (2020) 8:723–32. doi: 10.1016/j.cj.2020.04.008
93. Purcell S, Neale B, Todd-Brown K, Thomas L, Ferreira MA, Bender D, et al. Plink: a tool set for whole-genome association and population-based linkage analyses. Am J Hum Genet. (2007) 81:559–75. doi: 10.1086/519795
94. Aulchenko YS, Ripke S, Isaacs A, van Duijn CM. Genabel: an R library for genome-wide association analysis. Bioinformatics. (2007) 23:1294–6. doi: 10.1093/bioinformatics/btm108
95. Curtis RE, Kinnaird P, Xing EP, editors. Genamap: visualization strategies for structured association mapping. In: 2011 IEEE Symposium on Biological Data Visualization (BioVis). Providence, RI: IEEE (2011). doi: 10.1109/BioVis.2011.6094052
96. Zhou X, Stephens M. Genome-Wide efficient mixed-model analysis for association studies. Nat Genet. (2012) 44:821–4. doi: 10.1038/ng.2310
97. Liu Z, Li H, Zhong Z, Jiang S. A whole genome sequencing-based genome-wide association study reveals the potential associations of teat number in qingping pigs. Animals. (2022) 12:1057. doi: 10.3390/ani12091057
98. Uemoto Y, Ichinoseki K, Matsumoto T, Oka N, Takamori H, Kadowaki H, et al. Genome-Wide association studies for production, respiratory disease, and immune-related traits in landrace pigs. Sci Rep. (2021) 11:15823. doi: 10.1038/s41598-021-95339-2
99. Kim S, Lim B, Cho J, Lee S, Dang CG, Jeon JH, et al. Genome-Wide identification of candidate genes for milk production traits in korean holstein cattle. Animals. (2021) 11:1392. doi: 10.3390/ani11051392
100. Lee J, Lee SM, Lim B, Park J, Song KL, Jeon JH, et al. Estimation of variance components and genomic prediction for individual birth weight using three different genome-wide snp platforms in yorkshire pigs. Animals. (2020) 10:2219. doi: 10.3390/ani10122219
101. Adhil M, Agarwal M, Achutharao P, Talukder AK. Advanced computational methods, ngs tools, and software for mammalian systems biology. In:Kadarmideen HN, editor. Systems Biology in Animal Production and Health, Vol. 1. Springer (2016). p. 117–51. doi: 10.1007/978-3-319-43335-6_6
102. Headon D. Systems Biology And Livestock Production. Animal. (2013) 7:1959–63. doi: 10.1017/S1751731113000980
103. Kadarmideen HN. Genomics to systems biology in animal and veterinary sciences: progress, lessons and opportunities. Livest Sci. (2014) 166:232–48. doi: 10.1016/j.livsci.2014.04.028
104. Pathak RK, Singh DB. Systems biology approaches for food and health. In:Sharma TR, Deshmukh R, Sonah H, editors. Advances in Agri-Food Biotechnology. Singapore: Springer Nature (2020). p. 409–26. doi: 10.1007/978-981-15-2874-3_16
105. Barabasi AL, Oltvai ZN. Network biology: understanding the cell's functional organization. Nat Rev Genet. (2004) 5:101–13. doi: 10.1038/nrg1272
106. Ma J, Wang J, Ghoraie LS, Men X, Liu LN, Dai PG. Network-Based method for drug target discovery at the isoform level. Sci Rep. (2019) 9:13868. doi: 10.1038/s41598-019-50224-x
107. Lim D, Kim N-K, Park H-S, Lee S-H, Cho Y-M, Oh SJ, et al. Identification of candidate genes related to bovine marbling using protein-protein interaction networks. Int J Biol Sci. (2011) 7:992. doi: 10.7150/ijbs.7.992
108. Jumper J, Evans R, Pritzel A, Green T, Figurnov M, Ronneberger O, et al. Highly accurate protein structure prediction with alphafold. Nature. (2021) 596:583–9. doi: 10.1038/s41586-021-03819-2
109. Pathak RK, Singh DB, Sagar M, Baunthiyal M, Kumar A. Computational approaches in drug discovery and design. In:Singh DB, editor. Computer-Aided Drug Design. Singapore: Springer (2020). p. 1–21. doi: 10.1007/978-981-15-6815-2_1
110. Singh DB, Pathak RK. Computational approaches in drug designing and their applications. In:Gupta N, Gupta V, editors. Experimental Protocols in Biotechnology. Humana New York, NY: Springer (2020). p. 95–117. doi: 10.1007/978-1-0716-0607-0_6
111. Vakser IA. Protein-Protein docking: from interaction to interactome. Biophys J. (2014) 107:1785–93. doi: 10.1016/j.bpj.2014.08.033
112. DeLano WL. Pymol: an open-source molecular graphics tool. CCP4 Newsl Protein Crystallogr. (2002) 40:82–92. Available online at: https://legacy.ccp4.ac.uk/newsletters/newsletter40/11_pymol.pdf
113. Johansson MU, Zoete V, Michielin O, Guex N. Defining and searching for structural motifs using deepview/Swiss-Pdbviewer. BMC Bioinformatics. (2012) 13:173. doi: 10.1186/1471-2105-13-173
114. Pan F, Li JX, Zhao L, Tuersuntuoheti T, Mehmood A, Zhou N, et al. A Molecular docking and molecular dynamics simulation study on the interaction between cyanidin-3-O-glucoside and major proteins in cow's milk. J Food Biochem. (2021) 45:e13570. doi: 10.1111/jfbc.13570
115. Mugunthan SP, Mani Chandra H. A computational reverse vaccinology approach for the design and development of multi-epitopic vaccine against avian pathogen mycoplasma gallisepticum. Front Vet Sci. (2021) 8:721061. doi: 10.3389/fvets.2021.721061
116. Thakuria D, Khangembam VC, Pant V, Bhat RAH, Tandel RS, C S, et al. Anti-Oomycete activity of chlorhexidine gluconate: molecular docking and in vitro studies. Front Vet Sci. (2022) 9:909570. doi: 10.3389/fvets.2022.909570
117. Chen XR, Wang XT, Hao MQ, Zhou YH, Cui WQ, Xing XX, et al. Homology modeling and virtual screening to discover potent inhibitors targeting the imidazole glycerophosphate dehydratase protein in staphylococcus xylosus. Front Chem. (2017) 5:98. doi: 10.3389/fchem.2017.00098
118. Yang J, Roy A, Zhang Y. Protein–Ligand binding site recognition using complementary binding-specific substructure comparison and sequence profile alignment. Bioinformatics. (2013) 29:2588–95. doi: 10.1093/bioinformatics/btt447
119. Tian W, Chen C, Lei X, Zhao J, Liang J. Castp 3.0: computed atlas of surface topography of proteins. Nucleic Acids Res. (2018) 46:W363–7. doi: 10.1093/nar/gky473
120. Bhasme PC, Kurjogi MM, Sanakal RD, Kaliwal RB, Kaliwal BB. In silico characterization of putative drug targets in Staphylococcus saprophyticus, causing bovine mastitis. Bioinformation. (2013) 9:339–44. doi: 10.6026/97320630009339
121. Verma S, Pathak RK. Discovery and optimization of lead molecules in drug designing. In:Singh DB, Pathak RK, editors. Bioinformatics. Academic Press Elsevier (2022). p. 253–67. doi: 10.1016/B978-0-323-89775-4.00004-3
122. Agnihotry S, Pathak RK, Srivastav A, Shukla PK, Gautam B. Molecular docking and structure-based drug design. In:Computer-Aided Drug Design. Singapore: Springer (2020). p. 115–31. doi: 10.1007/978-981-15-6815-2_6
123. Pant S, Verma S, Pathak RK, Singh DB. Structure-Based drug designing. In:Singh DB, Pathak RK, editors. Bioinformatics. Academic Press Elsevier (2022). p. 219–31. doi: 10.1016/B978-0-323-89775-4.00027-4
124. Goodsell DS, Morris GM, Olson AJ. Automated docking of flexible ligands: applications of autodock. J Mol Recognit. (1996) 9:1–5. doi: 10.1002/(SICI)1099-1352(199601)9:1<1::AID-JMR241>3.0.CO;2-6
125. Lipinski CA, Lombardo F, Dominy BW, Feeney PJ. Experimental and computational approaches to estimate solubility and permeability in drug discovery and development settings. Adv Drug Deliv Rev. (2001) 46:3–26. doi: 10.1016/s0169-409x(00)00129-0
126. Baell JB, Holloway GA. New substructure filters for removal of pan assay interference compounds (pains) from screening libraries and for their exclusion in bioassays. J Med Chem. (2010) 53:2719–40. doi: 10.1021/jm901137j
127. Baell JB, Nissink JWM. Seven year itch: pan-assay interference compounds (pains) in 2017-utility and limitations. ACS Chem Biol. (2018) 13:36–44. doi: 10.1021/acschembio.7b00903
128. Qing X, Lee XY, De Raeymaecker J, Tame JR, Zhang KY, De Maeyer M, et al. Pharmacophore modeling: advances, limitations, and current utility in drug discovery. J Recept Ligand Channel Res. (2014) 7:81–92. doi: 10.2147/JRLCR.S46843
129. Tandon H, Chakraborty T, Suhag V. A concise review on the significance of qsar in drug design. Chem Biomol Eng. (2019) 4:45–51. doi: 10.11648/j.cbe.20190404.11
130. Tiwari A, Singh S. Computational approaches in drug designing. In:Singh DB, Pathak RK, editors. Bioinformatics. Academic Press Elsevier (2022). p. 207–17. doi: 10.1016/B978-0-323-89775-4.00010-9
131. Ganguly B, Rastogi SK, Prasad S. Computational designing of a poly-epitope fecundity vaccine for multiple species of livestock. Vaccine. (2013) 32:11–8. doi: 10.1016/j.vaccine.2013.10.086
132. Gebre MS, Brito LA, Tostanoski LH, Edwards DK, Carfi A, Barouch DH. Novel approaches for vaccine development. Cell. (2021) 184:1589–603. doi: 10.1016/j.cell.2021.02.030
133. Awasthi A, Sharma G, Agrawal P. Computational approaches for vaccine designing. In: Bioinformatics. Elsevier (2022) p. 317–35. doi: 10.1016/B978-0-323-89775-4.00011-0
134. Pathak RK, Lim B, Kim DY, Kim JM. Designing multi-epitope-based vaccine targeting surface immunogenic protein of Streptococcus agalactiae using immunoinformatics to control mastitis in dairy cattle. BMC Vet Res. (2022) 18:337. doi: 10.1186/s12917-022-03432-z
135. Neethirajan S. The role of sensors, big data and machine learning in modern animal farming. Sens Bio Sens Res. (2020) 29:100367. doi: 10.1016/j.sbsr.2020.100367
136. Dumortier L, Guepin F, Delignette-Muller ML, Boulocher C, Grenier T. Deep learning in veterinary medicine, an approach based on CNN to detect pulmonary abnormalities from lateral thoracic radiographs in cats. Sci Rep. (2022) 12:11418. doi: 10.1038/s41598-022-14993-2
137. Kumar I, Singh SP. Machine learning in bioinformatics. In:Singh DB, Pathak RK, editors. Bioinformatics. Academic Press Elsevier (2022). p. 443–56. doi: 10.1016/B978-0-323-89775-4.00020-1
138. Greener JG, Kandathil SM, Moffat L, Jones DT. A guide to machine learning for biologists. Nat Rev Mol Cell Biol. (2022) 23:40–55. doi: 10.1038/s41580-021-00407-0
139. Roberts H, Segev A, editors. Animal behavior prediction with long short-term memory. In: 2020 IEEE International Conference on Big Data (Big Data). Atlanta, GA: IEEE (2020). doi: 10.1109/BigData50022.2020.9378184
140. Qiao Y, Clark C, Lomax S, Kong H, Su D, Sukkarieh S. Automated individual cattle identification using video data: a unified deep learning architecture approach. Front Anim Sci. (2021) 2:759147. doi: 10.3389/fanim.2021.759147
141. Peng YQ, Kondo N, Fujiura T, Suzuki T, Wulandari, Yoshioka H, et al. Classification of multiple cattle behavior patterns using a recurrent neural network with long short-term memory and inertial measurement units. Comput Electron Agr. (2019) 157:247–53. doi: 10.1016/j.compag.2018.12.023
142. Qiao YL, Guo YY, Yu KP, He DJ. C3d-Convlstm based cow behaviour classification using video data for precision livestock farming. Comput Electron Agr. (2022) 193:106650. doi: 10.1016/j.compag.2021.106650
143. Reel PS, Reel S, Pearson E, Trucco E, Jefferson E. Using machine learning approaches for multi-omics data analysis: a review. Biotechnol Adv. (2021) 49:107739. doi: 10.1016/j.biotechadv.2021.107739
144. Benson D, Boguski M, Lipman D, Ostell J. The national center for biotechnology information. Genomics. (1990) 6:389–91. doi: 10.1016/0888-7543(90)90583-G
145. UniProt C. Uniprot: the universal protein knowledgebase in 2021. Nucleic Acids Res. (2021) 49:D480–9. doi: 10.1093/nar/gkaa1100
146. Bateman A, Birney E, Durbin R, Eddy SR, Howe KL, Sonnhammer EL. The Pfam protein families database. Nucleic Acids Res. (2000) 28:263–6. doi: 10.1093/nar/28.1.263
147. Berman HM, Westbrook J, Feng Z, Gilliland G, Bhat TN, Weissig H, et al. The protein data bank. Nucleic Acids Res. (2000) 28:235–42. doi: 10.1093/nar/28.1.235
148. Varadi M, Anyango S, Deshpande M, Nair S, Natassia C, Yordanova G, et al. Alphafold protein structure database: massively expanding the structural coverage of protein-sequence space with high-accuracy models. Nucleic Acids Res. (2022) 50:D439–44. doi: 10.1093/nar/gkab1061
149. Irwin JJ, Shoichet BK. Zinc–a free database of commercially available compounds for virtual screening. J Chem Inf Model. (2005) 45:177–82. doi: 10.1021/ci049714+
150. Edgar R, Domrachev M, Lash AE. Gene expression omnibus: ncbi gene expression and hybridization array data repository. Nucleic Acids Res. (2002) 30:207–10. doi: 10.1093/nar/30.1.207
151. Katz K, Shutov O, Lapoint R, Kimelman M, Brister JR, O'Sullivan C. The sequence read archive: a decade more of explosive growth. Nucleic Acids Res. (2022) 50:D387–90. doi: 10.1093/nar/gkab1053
152. Kanehisa M, Goto S. Kegg: kyoto encyclopedia of genes and genomes. Nucleic Acids Res. (2000) 28:27–30. doi: 10.1093/nar/28.1.27
153. Szklarczyk D, Gable AL, Lyon D, Junge A, Wyder S, Huerta-Cepas J, et al. String V11: protein-protein association networks with increased coverage, supporting functional discovery in genome-wide experimental datasets. Nucleic Acids Res. (2019) 47:D607–13. doi: 10.1093/nar/gky1131
154. Chelliah V, Laibe C, Le Novere N. Biomodels database: a repository of mathematical models of biological processes. Methods Mol Biol. (2013) 1021:189–99. doi: 10.1007/978-1-62703-450-0_10
155. Mishra D, Yadav S, Sikka P, Jerome A, Paul S, Rao A, et al. Snprbb: economically important trait specific snp resources of buffalo (Bubalus bubalis). Conserv Genet Resourc. (2021) 13:283–9. doi: 10.1007/s12686-021-01210-x
156. Sarika, Arora V, Iquebal MA, Rai A, Kumar D. In silico mining of putative microsatellite markers from whole genome sequence of water buffalo (Bubalus Bubalis) and development of first buffsatdb. BMC Genomics. (2013) 14:43. doi: 10.1186/1471-2164-14-43
157. McCarthy FM, Pendarvis K, Cooksey AM, Gresham CR, Bomhoff M, Davey S, et al. Chickspress: a resource for chicken gene expression. Database. (2019) 2019:baz058. doi: 10.1093/database/baz058
158. Cunningham F, Allen JE, Allen J, Alvarez-Jarreta J, Amode MR, Armean IM, et al. Ensembl 2022. Nucleic Acids Res. (2022) 50:D988–95. doi: 10.1093/nar/gkab1049
159. Garcia JF, Carmo AS, Utsunomiya YT, Rezende Neves HH, Carvalheiro R, Tassell CV, et al., editors. How bioinformatics enables livestock applied sciences in the genomic era. In: Brazilian Symposium on Bioinformatics. Springer (2012). doi: 10.1007/978-3-642-31927-3_17
160. Bayat A. Science, medicine, and the future-bioinformatics. BMJ. (2002) 324:1018–22. doi: 10.1136/bmj.324.7344.1018
161. Council NR. Critical Needs for Research in Veterinary Science (2005). Washington, DC: The National Academies Press. Available online at: https://nap.nationalacademies.org/catalog/11366/critical-needs-for-research-in-veterinary-science
162. Leinonen R, Akhtar R, Birney E, Bonfield J, Bower L, Corbett M, et al. Improvements to services at the european nucleotide archive. Nucleic Acids Res. (2010) 38:D39–45. doi: 10.1093/nar/gkp998
163. Abouelkhair MA. Non-SARS-CoV-2 genome sequences identified in clinical samples from covid-19 infected patients: evidence for co-infections. PeerJ. (2020) 8:e10246. doi: 10.7717/peerj.10246
164. Simons A. A Quality Control Tool for High Throughput Sequence Data (2010). Available online at: https://www.bioinformatics.babraham.ac.uk/projects/fastqc/
165. Bolger AM, Lohse M, Usadel B. Trimmomatic: a flexible trimmer for illumina sequence data. Bioinformatics. (2014) 30:2114–20. doi: 10.1093/bioinformatics/btu170
166. Martin M. Cutadapt removes adapter sequences from high-throughput sequencing reads. EMBnet J. (2011) 17:10–2. doi: 10.14806/ej.17.1.200
167. Chen S, Zhou Y, Chen Y, Gu J. Fastp: an ultra-fast all-in-one fastq preprocessor. Bioinformatics. (2018) 34:i884–i90. doi: 10.1093/bioinformatics/bty560
168. Kim D, Langmead B, Salzberg SL. Hisat: a fast spliced aligner with low memory requirements. Nat Methods. (2015) 12:357–60. doi: 10.1038/nmeth.3317
169. Li H, Handsaker B, Wysoker A, Fennell T, Ruan J, Homer N, et al. The sequence alignment/map format and samtools. Bioinformatics. (2009) 25:2078–9. doi: 10.1093/bioinformatics/btp352
170. Langmead B, Salzberg SL. Fast gapped-read alignment with bowtie 2. Nat Methods. (2012) 9:357–9. doi: 10.1038/nmeth.1923
171. Li H, Durbin R. Fast and accurate short read alignment with burrows-wheeler transform. Bioinformatics. (2009) 25:1754–60. doi: 10.1093/bioinformatics/btp324
172. Grabherr MG, Haas BJ, Yassour M, Levin JZ, Thompson DA, Amit I, et al. Full-length transcriptome assembly from Rna-Seq data without a reference genome. Nat Biotechnol. (2011) 29:644–52. doi: 10.1038/nbt.1883
173. Robinson MD, McCarthy DJ, Smyth GK. Edger: a bioconductor package for differential expression analysis of digital gene expression data. Bioinformatics. (2010) 26:139–40. doi: 10.1093/bioinformatics/btp616
174. Love MI, Huber W, Anders S. Moderated estimation of fold change and dispersion for Rna-Seq data with Deseq2. Genome Biol. (2014) 15:550. doi: 10.1186/s13059-014-0550-8
175. Langfelder P, Horvath S. Wgcna: an R package for weighted correlation network analysis. BMC Bioinformatics. (2008) 9:559. doi: 10.1186/1471-2105-9-559
176. McKenna A, Hanna M, Banks E, Sivachenko A, Cibulskis K, Kernytsky A, et al. The genome analysis toolkit: a mapreduce framework for analyzing next-generation DNA sequencing data. Genome Res. (2010) 20:1297–303. doi: 10.1101/gr.107524.110
177. Zerbino DR, Birney E. Velvet: algorithms for de novo short read assembly using de bruijn graphs. Genome Res. (2008) 18:821–9. doi: 10.1101/gr.074492.107
178. Supek F, Bosnjak M, Skunca N, Smuc T. Revigo summarizes and visualizes long lists of gene ontology terms. PLoS ONE. (2011) 6:e21800. doi: 10.1371/journal.pone.0021800
179. Howe EA, Sinha R, Schlauch D, Quackenbush J. Rna-Seq analysis in mev. Bioinformatics. (2011) 27:3209–10. doi: 10.1093/bioinformatics/btr490
180. Subramanian A, Tamayo P, Mootha VK, Mukherjee S, Ebert BL, Gillette MA, et al. Gene Set enrichment analysis: a knowledge-based approach for interpreting genome-wide expression profiles. Proc Natl Acad Sci USA. (2005) 102:15545–50. doi: 10.1073/pnas.0506580102
181. Buchfink B, Xie C, Huson DH. Fast and sensitive protein alignment using diamond. Nat Methods. (2015) 12:59–60. doi: 10.1038/nmeth.3176
182. Conesa A, Gotz S, Garcia-Gomez JM, Terol J, Talon M, Robles M. Blast2go: a universal tool for annotation, visualization and analysis in functional genomics research. Bioinformatics. (2005) 21:3674–6. doi: 10.1093/bioinformatics/bti610
183. Shannon P, Markiel A, Ozier O, Baliga NS, Wang JT, Ramage D, et al. Cytoscape: a software environment for integrated models of biomolecular interaction networks. Genome Res. (2003) 13:2498–504. doi: 10.1101/gr.1239303
184. Pettersen EF, Goddard TD, Huang CC, Couch GS, Greenblatt DM, Meng EC, et al. Ucsf chimera–a visualization system for exploratory research and analysis. J Comput Chem. (2004) 25:1605–12. doi: 10.1002/jcc.20084
185. Choi Y, Chan AP. Provean web server: a tool to predict the functional effect of amino acid substitutions and indels. Bioinformatics. (2015) 31:2745–7. doi: 10.1093/bioinformatics/btv195
186. Csizmadia P. Marvinsketch and Marvinview: Molecule Applets for the World Wide Web. (1999). doi: 10.3390/ecsoc-3-01775
187. Daina A, Michielin O, Zoete V. Swissadme: a free web tool to evaluate pharmacokinetics, drug-likeness and medicinal chemistry friendliness of small molecules. Sci Rep. (2017) 7:42717. doi: 10.1038/srep42717
Keywords: vetinformatics, livestock systems, functional genomics, drug discovery, veterinary science
Citation: Pathak RK and Kim J-M (2022) Vetinformatics from functional genomics to drug discovery: Insights into decoding complex molecular mechanisms of livestock systems in veterinary science. Front. Vet. Sci. 9:1008728. doi: 10.3389/fvets.2022.1008728
Received: 01 August 2022; Accepted: 31 October 2022;
Published: 11 November 2022.
Edited by:
Joanna Szyda, Wroclaw University of Environmental and Life Sciences, PolandCopyright © 2022 Pathak and Kim. This is an open-access article distributed under the terms of the Creative Commons Attribution License (CC BY). The use, distribution or reproduction in other forums is permitted, provided the original author(s) and the copyright owner(s) are credited and that the original publication in this journal is cited, in accordance with accepted academic practice. No use, distribution or reproduction is permitted which does not comply with these terms.
*Correspondence: Jun-Mo Kim, anVubW9raW1AY2F1LmFjLmty