- 1Department of Pharmaceutical Engineering, Inje University, Gimhae, Republic of Korea
- 2Department of Science, Technology and Society, Bar-Ilan University, Ramat Gan, Israel
- 3Pharmacomicrobiomics Research Center and College of Pharmacy, Hanyang University, Ansan, Gyeonggi-Do, Republic of Korea
- 4Equine Clinic, Jeju Stud Farm, Korea Racing Authority, Jeju-si, Jeju, Republic of Korea
Introduction: Red ginseng (RG), a traditional herbal remedy, has garnered attention owing to its diverse health benefits resulting from its complex composition. However, extensive research is needed to substantiate the efficacy of RG and understand the underlying mechanisms supporting these benefits. This study aimed to identify potential biomarkers and investigate the impact of RG on related metabolic pathways in horse plasma using liquid chromatography–mass spectrometry (LC–MS)-based metabolomics.
Methods: Ten horses were divided into control and RG groups, with the latter administered RG at a dose of 600 mg⋅kg−1⋅day−1 for 3 weeks. Subsequently, the plasma samples were collected and analyzed using LC–MS. Multivariate statistical analysis, volcano plots, and feature-based molecular networking were employed.
Results: The analysis identified 16 metabolites that substantially decreased and 21 metabolites that substantially increased following RG consumption. Among the identified metabolites were oleanolic acid, ursolic acid, and ginsenoside Rb1, which are known for their antioxidant and anti-inflammatory properties, as well as lipid species that influence sphingolipid and glycerophospholipid metabolism. Additionally, potential biomarkers, including major RG components, demonstrated distinct group clustering in principal component analysis and partial least squares-discriminant analysis, indicating their utility in assessing the physiological effects of RG consumption.
Discussion: This study contributes to a comprehensive understanding of the effects of RG on health.
1 Introduction
Red ginseng (RG) is a medicinal herb traditionally used as a supplement for centuries in various treatments, owing to its composition of polyacetylenes, polysaccharides, sesquiterpenes, peptidoglycans, nitrogen-containing compounds, phenolic compounds, and vitamins (1). RG has been the subject of extensive scientific research due to its potential health benefits. The active components of RG exhibit diverse biological effects, including anti-inflammatory, antitumor, antidiabetic, antioxidant, and anti-stress activities in various tissues (2–5). These properties underscore the potential of RG as an alternative medicine for preventing and treating a range of diseases, including cardiovascular, metabolic, neurological, and hepatic disorders (2–5).
However, there are various perspectives on the precise mechanisms and metabolic pathways underlying the diverse effects of RG (6). To comprehensively understand these effects, a systematic investigation into the molecular mechanisms of its constituents is essential. Mass spectrometry offers a comprehensive and sensitive approach for substance detection, and liquid chromatography–mass spectrometry (LC–MS)-based metabolomics provides new insights into the efficacy of RG (7–12). A systematic analysis of metabolic responses of RG, along with a detailed examination of its mechanisms (13), is crucial to elucidate the synergistic effects of its different components, assess its safety, and gain a deeper understanding of complex molecular mechanisms of RG (14).
Current efforts to evaluate the health effects of RG often rely on studying changes in blood metabolites, predominantly using rodent models (1, 15). However, to understand benefits of RG in humans, it is crucial to consider interspecies specificity. Therefore, experimental evidence from diverse species is imperative to align these results with human outcomes (16).
Studying the effects of RG consumption in healthy horses and evaluating specific biomarkers can provide valuable information. First, it can validate the effects of RG on health. Second, it can assess individual physiological responses, enabling personalized health management (17). Third, specific biomarkers can be monitored to confirm the safety of long-term RG consumption and assess any potential side effects (18). Fourth, it can aid in the early prediction and prevention of potential health issues (19). Lastly, studying changes in specific biomarkers can elucidate the physiological effects of RG, contributing to a more comprehensive understanding of its mechanisms of action (20).
This study aimed to investigate, for the first time, the effect of RG consumption on horse plasma metabolites and to identify potential biomarkers associated with RG intake. Using LC–MS-based metabolomics, this study explored alterations in metabolic pathways and the potential physiological effects of RG. These findings suggest that specific metabolites, including ginsenosides, May serve as potential biomarkers to assess the effects of RG on health.
2 Materials and methods
2.1 Red ginseng consumption in horses
Ten-adult Thoroughbred horses were randomly divided into two groups of five horses each. All horses in the study were bred and supervised under the same conditions on a farm for a minimum of 1 year. The trial group received ground RG (600 mg⋅kg−1⋅day−1) mixed with molasses as a carrier every morning for 3 weeks, while the control group received only the carrier. All horses ad libitum access to food and water throughout the study period. In the third week, venipuncture was performed in the morning following overnight fasting. Twenty milliliters of blood were drawn into heparin tubes (BD Vacutainer®). Animal protocols were approved by the Institutional Animal Care and Use Committee of the Korea Racing Authority (KRA IACUC-2207-AEC-2207).
2.2 Data mining and preprocessing
The materials, sample preparation, and analysis methods are described in Supplementary material S1. The workflow chart for the study is shown in Supplementary Figure S1. Excel files (including features such as m/z values, retention times, and peak area information) for omics analysis, along with MGF format files for metabolite annotation and feature-based molecular networking (FBMN), were generated and exported from MZmine 3 (version 3.9.0). The data were calibrated using internal standards. Outliers were removed using a filter based on the QC function, and the dataset was preprocessed through log-transformation and Pareto-normalization in MetaboAnalyst 5.0 (21).
2.3 Peak annotation using in silico methods
Peak annotation was performed using SIRIUS version 5.8.3 software (22). MS1 and MS2 peak fragmentation patterns were interpreted with ZODIAC, CSI: FingerID, and CANOPUS using the ClassyFire and NPClassifier modules. All the datasets were used for tentative identification. For the potential identification of biomarkers, COSMIC, ZODIAC, and confidence scores were comprehensively considered. Candidates with a ZODIAC score of 50% or lower or a SIRIUS score of <50% without an accompanying ZODIAC score were excluded from the analysis. A comprehensive approach was employed to verify additional biomarkers using the Human Metabolome Database (HMDB, https://hmdb.ca/), mzCloud (https://www.mzcloud.org), and Lipid Maps (https://www.lipidmaps.org) databases, and a literature review. To facilitate pattern verification and data visualization, FBMN was employed (23). MGF files were uploaded to the Global Natural Product Social Molecular Networking (GNPS) platform (23).1 FBMN was conducted under the following conditions: minimum pair cosine score, 0.5; network topology, 10; and maximum connected component size, 100. The FBMN data were visualized and annotated using Cytoscape 3.10.1 software (San Diego, CA, United States).
2.4 Statistical and enrichment analysis
Principal component analysis (PCA) and partial least squares-discriminant analysis (PLS-DA) were employed to chemometrically visualize the discrimination between the RG and control groups and to identify the variables contributing to the separation between these groups using the MetaboAnalyst 5.0 platform.2 A volcano plot was used to display the results of the fold change and independent t-tests (p < 0.01). The Lipid Pathway Enrichment Analysis platform3 and pathway analysis in MetaboAnalyst were used for functional enrichment analysis of the identified metabolites. To assess the sensitivity and specificity of the potential biomarkers, receiver operating characteristic (ROC) analyses were performed using MetaboAnalyst 5.0.
3 Results
3.1 Metabolomics method validation
QC samples were generated by combining equal volumes of extracts from all samples and were processed and tested identically to that of the analyzed samples. During the instrumental analysis, each QC sample was subjected to both positive and negative ion mode detection. The total ion chromatography spectra of the QC samples exhibited well-defined peaks and a consistently uniform distribution under these detection conditions, validating the robustness of the system (Supplementary Figure S2).
3.2 Multivariate statistical analysis and metabolic profile changes
Metabolic profiling and comprehensive analysis of metabolites between the RG and control groups using MZmine 3 detected 854 ions (positive mode) and 306 ions (negative mode) in the plasma samples. A total of 1,160 ions detected in both modes were integrated for statistical analysis. PCA was initially employed for an unsupervised comprehensive overview of the plasma samples. However, PCA did not reveal significant differences between the control and RG groups (data not shown). Subsequently, a supervised approach, PLS-DA, was employed to assess the differences between the groups. As shown in Figure 1A, the PLS-DA score plot revealed a clear distinction between the control and RG groups, suggesting substantial alterations in the metabolic profile of horse plasma following RG consumption (Figure 1B). Volcano plots were used to visualize the metabolites influencing the intergroup variations in PLS-DA. Comparing the RG-fed group with the control group, metabolites with log2 (fold change) values ≥2 and -log10 (p-value) values ≥2 were considered statistically significant. As a result, 16 metabolites exhibited a statistically significant decrease, whereas 21 metabolites showed a statistically significant increase in response to RG (Figure 1C). The red and blue dots indicate characteristic metabolites in the direction of comparison (RG consumption/control, adjusted p-value <0.01, fold change >2). Gray dots indicate the metabolites that did not differ between the two groups. Table 1 lists the numbers identified in the figure.
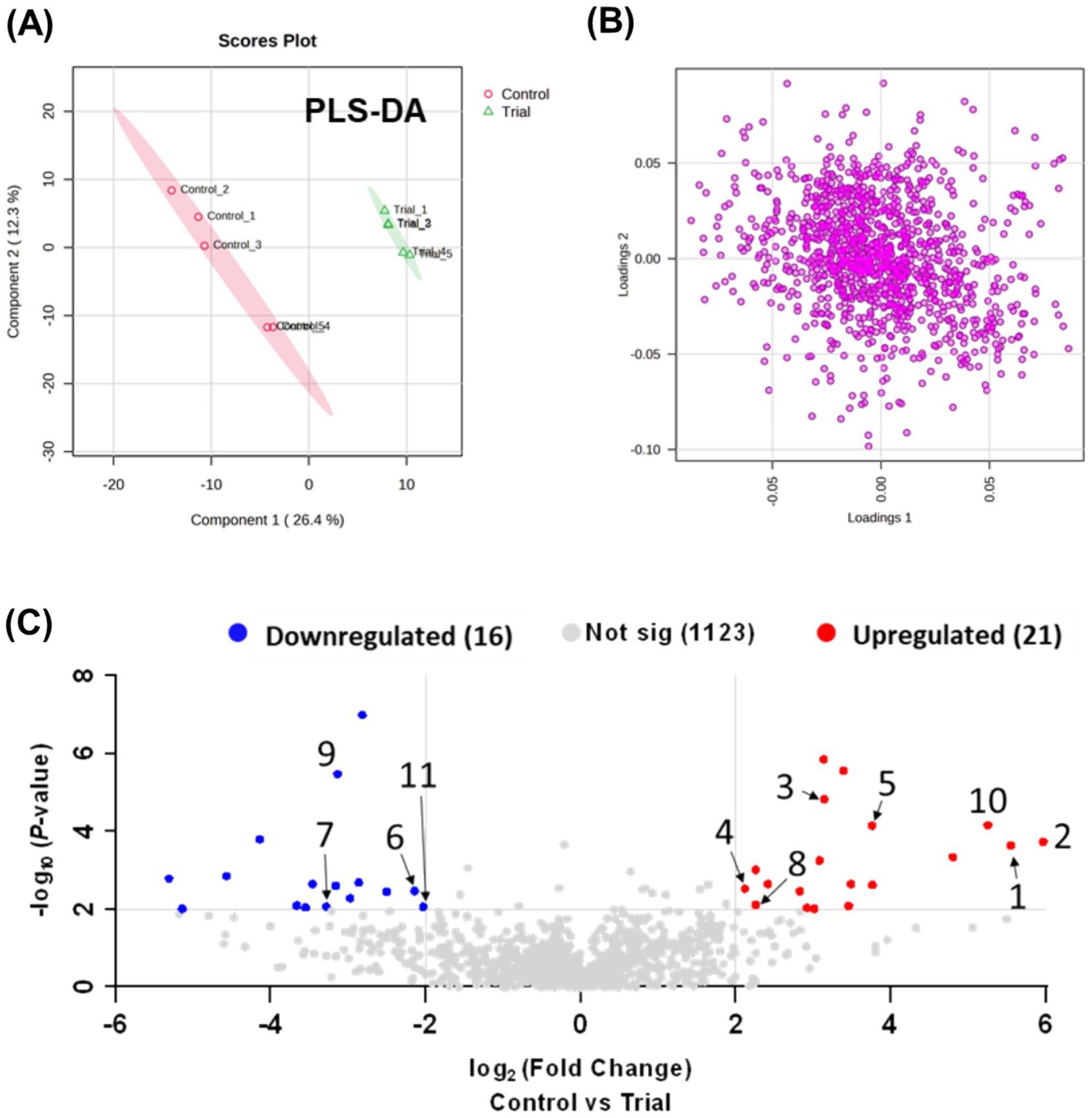
Figure 1. (A) Partial least squares-discriminant analysis plot based on LC-Q exactive data processed using Metaboanalyst 5.0, showing component 2 versus component 1. (B) Loading plot. (C) Volcano plot analysis.
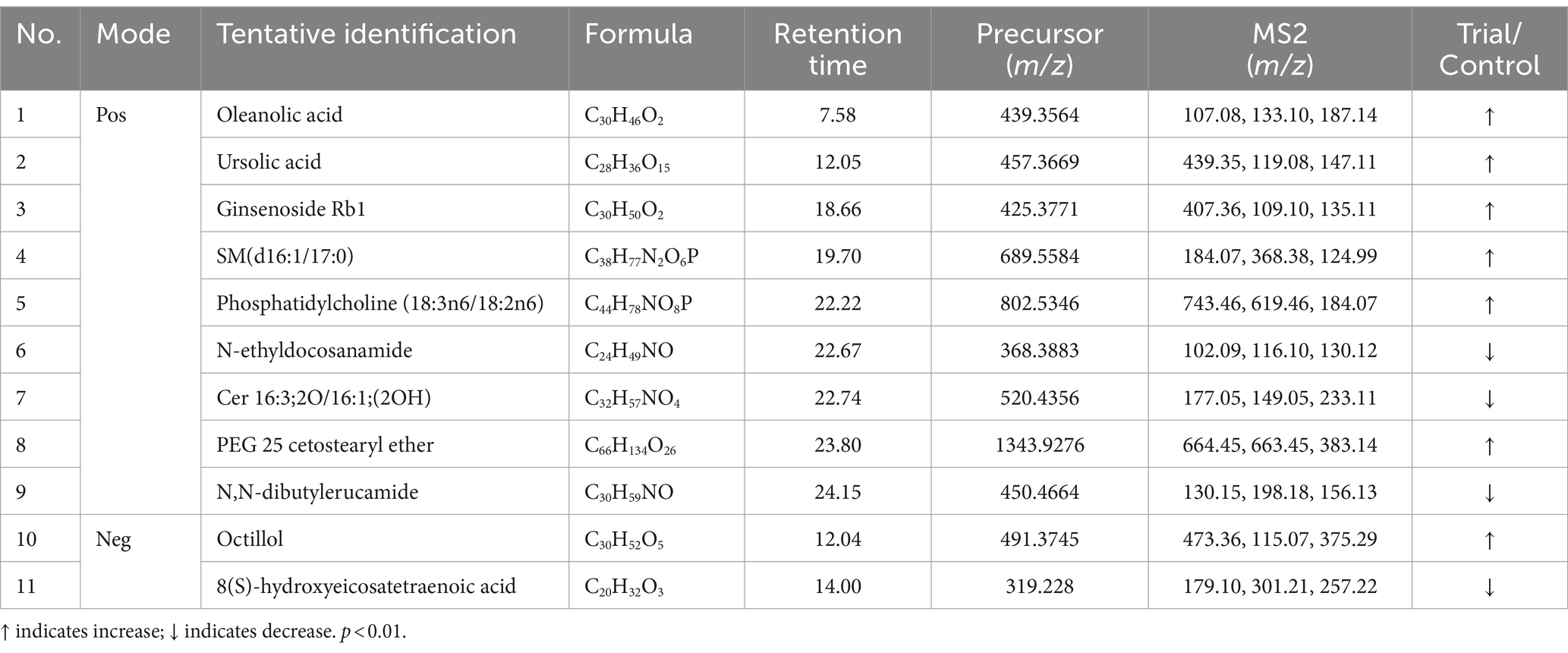
Table 1. Potential biomarkers associated with RG consumption in equine plasma (positive and negative mode).
3.3 Identification of metabolites
We annotated the significantly altered metabolites, and among the annotated metabolites, 11 out of 37 (approximately 29%) were identified based on MS1 and MS2 fragmentation data (Table 1). The identified metabolites included three major components–oleanolic acid, ursolic acid, and ginsenoside Rb1–as well as various lipid components. For the 26 peaks for which significant identification results were not obtained using the MS1 and MS2 spectra, the substances predicted using MS1 information were verified through the HMDB (Supplementary material S2, molecular weight tolerance < ±10 ppm). Among the potential metabolites identified, representative substances included phosphatidylethanolamine, oxidized ceramide, and cardiolipin.
3.4 ClassyFire information
Using ClassyFire to categorize the compounds identified in equine plasma, the majority of the compounds were summarized according to the kingdom (K), superclass (SC), and class (C) levels. Specifically, in the SC classification, most compounds were lipids and lipid-like molecules (47.2%), organic acids and their derivatives (22.5%), and benzenoids (12.8%) (Figure 2). FBMN allowed the visualization of connections between compounds based on the integration of ClassyFire information and the significance of peaks (Figure 2).
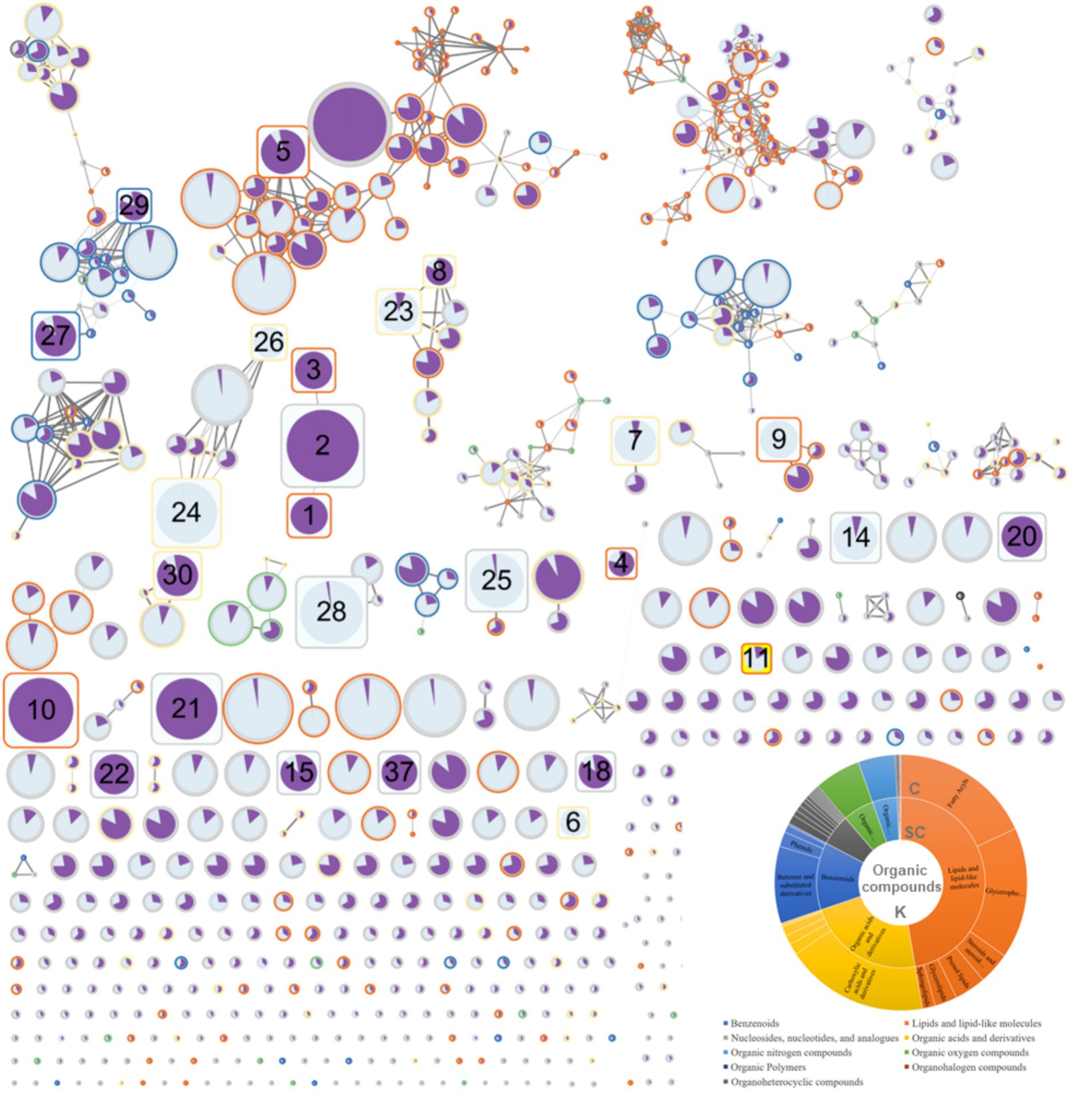
Figure 2. Results of feature-based molecular networking between the red ginseng (RG) consumption group and the control group.
3.5 Feature-based molecular networking analysis
The FBMN analysis generated 780 nodes (Figure 2). In comparison, 1,160 peaks were obtained during the data mining process. The smaller number of nodes (780) was attributed to the use of the data-dependent acquisition (DDA) mode in the analysis. The DDA approach relies on MS1 data to induce a limited MS2, providing the advantage of obtaining a more reliable MS2 data for the target MS1. However, it failed to acquire all MS2 information for trace metabolites, which is crucial for FBMN analysis. Therefore, 11 of the 37 peaks that exhibited significant changes (as indicated in Table 1 and Supplementary material S2, with numbers 12, 13, 16, 17, 19, 31, 32, 33, 34, 35, and 36) were not represented as nodes. The FBMN results demonstrated that compounds with similar backbones, such as oleanolic acid, ursolic acid, and ginsenoside Rb1, were interconnected. These compounds were not detected in the control group, indicating that RG as their source. Additionally, compound 23 (Supplementary material S2), with structural similarity to compound 8, identified with high confidence (Table 1, PEG 25 cetostearyl ether), suggests the possibility of it being phosphatidylinositol-3,4,5-trisphosphate among the predicted compound candidates (momorcharaside B, notoginsenoside R9, ginsenoside M7cd, and phosphatidylinositol-3,4,5-trisphosphate). Additionally, the fold-change feature values in the RG consumption group compared to that of the control group were used to determine node sizes by incorporating omics information. The color (from white to black) and thickness of the node edges are proportionally represented by cosine values. The average intensity values between the groups were visualized within nodes using a pie chart, with the RG consumption group represented in purple and the control group represented in sky blue. The CANOPUS analysis results, conducted using ClassyFire on data mined from MZmine3, were organized into a sunburst plot. Individual peak information is highlighted by node border colors (Figure 2). Finally, nodes representing characteristic metabolites (RG-fed/control, adjusted p-value <0.01, fold change >2 times) are denoted by rounded rectangles, and specific node numbers can be cross-referenced with the information in Table 1.
3.6 Enrichment analysis
To analyze the metabolic pathways associated with the identified biomarkers after RG consumption, we used LIPEA and MetaboAnalyst 5.0 platforms. A total of 22 pathways were identified. RG administration had the greatest influence on sphingolipid (SL) and glycerophospholipid (GPL) metabolic pathways (Figure 3A). RG affected lipid metabolism by upregulating three metabolites (dihydroceramide, ceramide, and sphingomyelin) in the SL pathway and four metabolites (phosphatidylcholine, phosphatidyl-ethanolamine, phosphatidyl-L-serine, and cardiolipin) in the GPL pathway (Figure 3B).
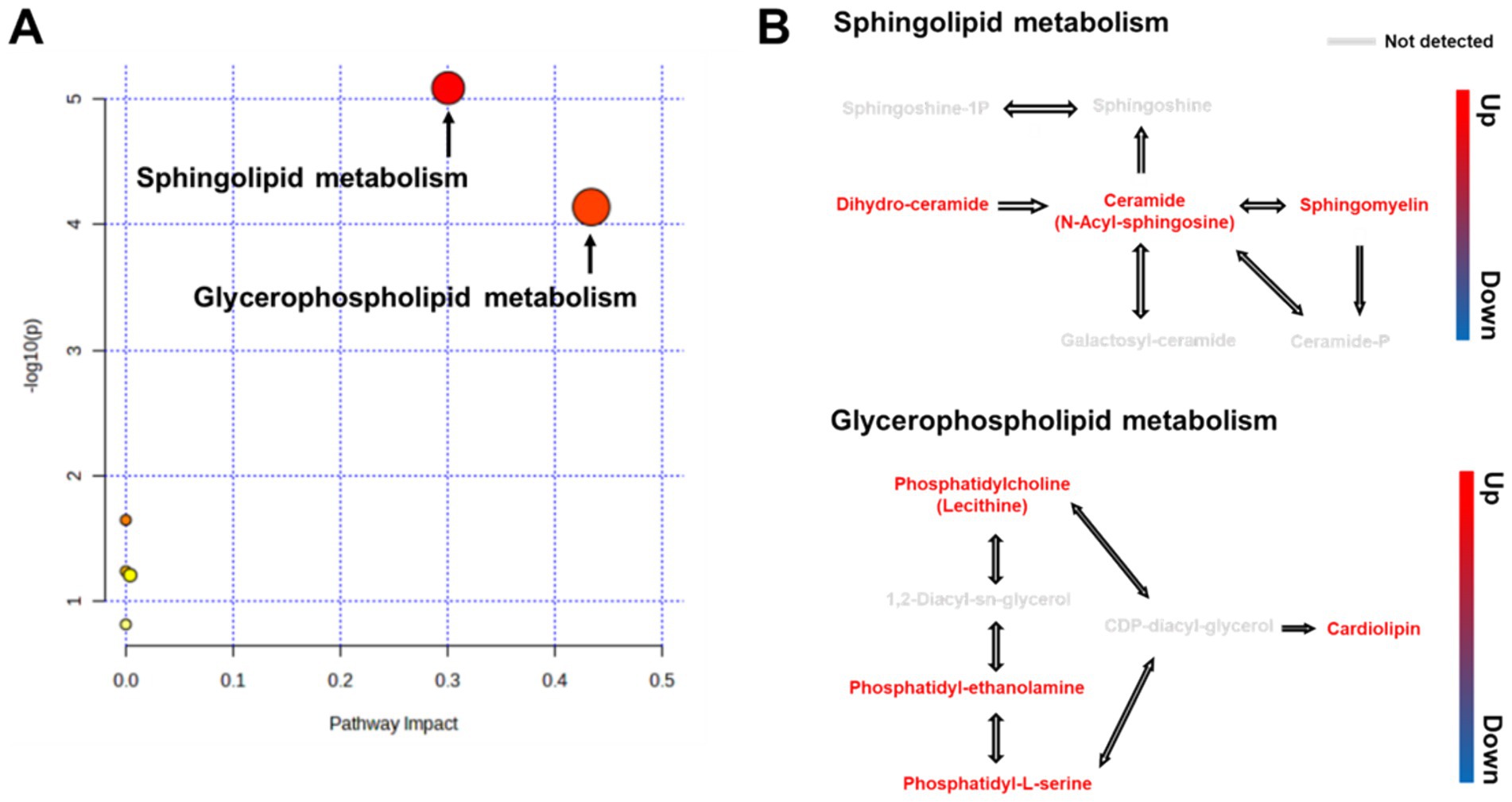
Figure 3. (A) Overview of pathway analysis for all metabolic pathways (Kyoto Encyclopedia of Genes and Genomes) for RG-fed horses. All matched pathways are classified by p-values (y-axis) from pathway enrichment analysis and pathway impact values (x-axis) from pathway topology analysis. Node size represents the impact values, while node colors indicate different p-values. (B) Pathway map of differential metabolites between RG-fed and control groups.
4 Discussion
RG components produce diverse effects on the body, providing benefits in cardiovascular, hepatic, neurological, and metabolic disorders (2–5, 24, 25). However, the precise mechanisms and metabolic pathways underlying the effects of RG remain unclear. In this study, we aimed to identify biomarkers associated with RG administration and explore the biological pathways related to these biomarkers using systems biology approaches.
While numerous studies have investigated the health benefits of RG using rodent models (26–29), it is crucial to understand the mechanisms of action of RG components in other species. Extrapolating results from different species to humans remains uncertain. RG is commonly used as a feed additive and alternative medicine for horses to enhance performance, promote growth, and prevent diseases (30, 31). Over the past 5 years, quality test results for feed additives used in racehorses in Jeju, Korea, revealed that RG has been used extensively, accounting for 34.7% (76 out of 219 total feed additive inspections), indicating its frequent used in the equine industry. However, scientific evidence concerning its effects in horses is limited. This underscores the need to obtain experimental evidence in diverse species including horses (16). We observed changes in the plasma metabolites of horses fed RG to enhance our understanding of the impact of RG on their health. Furthermore, we statistically analyzed and visualized the plasma metabolites using PLS-DA and FBMN. Additionally, we identified metabolites potentially contributing to the observed changes through in silico methods and database analyses. The PLS-DA score plot clearly differentiated the blood samples from the control and RG groups. We also confirmed that the major representative components of RG and the plasma metabolites primarily contributed to these differences and were predominantly associated with lipid metabolism.
The potentially identified compounds–oleanolic acid, ursolic acid, and ginsenoside Rb1–are well-known representative components of RG (29, 32). Oleanolic acid and ursolic acid are structurally similar triterpenoid compounds known to inhibit inflammatory responses and reduce oxidative stress, thereby contributing to the mitigation of various chronic diseases. However, owing to structural differences, they modulate cellular signaling pathways differently, with ursolic acid reportedly exhibiting stronger anti-inflammatory effects than oleanolic acid (33, 34). Ginsenoside Rb1 exerts neuroprotective effects, improves brain function, and slows the progression of neurodegenerative diseases. It reduces stress, aids in fatigue recovery, and enhances overall physical vitality. Additionally, it contributes to blood pressure regulation and vascular function improvement, potentially reducing the risk of cardiovascular diseases (35–37).
Rb1 is the major ginsenoside in the plasma of horses (29). The findings of the current study emphasize that Rb1 is the predominant component in blood following RG consumption.
Changes in plasma metabolite levels due to RG consumption have been reported in various studies. A study administering RG daily to healthy mice for 6 weeks (3 mL, 300 mg/kg) observed an increase in plasma lipid metabolites (lysophosphatidylcholine, SL) (1). Similarly, a study conducted in a mouse model of Alzheimer’s disease showed significant changes in lipid metabolites (38). These findings strongly suggested that RG is primarily associated with lipid metabolism, reinforcing the reliability of the results obtained in this study.
We identified two significantly altered pathways following RG consumption: SL and GPL metabolism. SL and GPL are distinct lipid classes, and various lipid species within these classes participate in diverse cellular functions, including cell proliferation, signaling cascades, and apoptosis (39). The SL metabolic pathway begins with sphingosine and subsequently generates various SLs, such as ceramide and sphingomyelin. Ceramide plays a crucial role in apoptosis and cellular stress responses (40). The primary component of RG, ginsenosides, exhibit anti-inflammatory and antioxidant effects, which May contribute to the regulation of ceramide synthesis and degradation within the SL metabolic pathway. For example, ginsenosides can reduce cellular stress and promote cell survival (41, 42). GPL, consisting of a glycerol backbone attached to phosphate and fatty acids, are key components of the phospholipid bilayer. These lipids maintain cell membrane fluidity and play essential roles as secondary messengers in signaling pathways (43). RG consumption improves membrane fluidity and structural stability by regulating GPL metabolism (44). Some studies have suggested that RG consumption May positively influence blood lipid profiles and potentially improve cardiovascular health (45, 46).
Recent evidence suggests that SL and GPL pathways mutually regulate their biosynthesis, and alterations in these interactions can contribute to lipotoxicity-related impairments in various organs (47). RG exhibits adaptogenic properties in improving immunological and neurological diseases, with SL and GPL metabolism implicated in these process (48, 49). The results of this study demonstrated that RG administration in horses induced SL and GPL changes, with no relevant side effects. This strengthens our understanding of the diverse physiological effects of RG through the SL and GPL metabolic pathways.
Some studies have highlighted the role of ginsenosides in the changes in lipid composition resulting from RG consumption. Compound K plays a crucial role in regulating the biological functions of cells by increasing the expression of SL and ceramides (50). Additionally, Rb1 stimulates skin wound healing through a sphingolipid-1-phosphate-dependent mechanism and inhibits hyperlipidemia by regulating the synthesis and breakdown of phosphatidylcholine in GPL metabolism while also modulating the gut microbiota (15, 51).
However, understanding the intricate mechanisms underlying the effects of RG remains challenging, even with the evidence of lipid pathway alterations presented in this study. The effects of RG, such as the modulation of intestinal microbiota by Rb1, are influenced by various internal and external factors, resulting in enhancement or reduction of their effects. Gut microbes are closely linked to host metabolism, and SL produced by gut bacteria are subsequently transported to internal organs, affecting host lipid metabolism (52). Furthermore, RG modulates the diversity of the gut microbiota that can regulate the transformation of ginsenosides (52). Therefore, it can be inferred that RG, SL, and gut microbes intricately and reciprocally influence each other, potentially affecting the pathogenesis of various diseases. Comprehensive research on the relationship between gut microbes and RG is necessary for future studies.
This study evaluated the effects of RG supplementation in horses by analyzing both the metabolic pathways and physiological effects. However, several factors must be considered when interpreting these results. First, although RG has been used as an herbal medicine in Eastern countries for thousands of years (53), there are still no clear standards regarding its dosage and duration of administration. Previous studies in humans and rodents have used doses ranging from 100 to 600 mg/kg over periods ranging from 1 d to 27 months (54, 55). Considering these data and the resources available for our experiments, we administered RG at a dose of 600 mg/kg for 3 weeks. Furthermore, large animals like equines require more resources, cost, and specialized facilities compared to other species and considering the difficulty in collecting samples, conducting experiments involving numerous animals is challenging. Therefore, we conducted the experiment using 10 horses, which May appear relatively few compared to experiments using other animal models, such as mice. These physical and methodological limitations have contributed to the scarcity of in vivo horse data. Therefore, the data from this study warrants future large-scale experiments with rodents to build upon these findings.
5 Potential biomarkers
We assessed whether the major components of RG detected in plasma and the significantly altered plasma metabolites could potentially serve as biomarkers of RG intake. The evaluated 37 components had variable importance in projection values exceeding 1, and the area under the curve (AUC) values in the ROC analysis were 0.88 or higher (Supplementary Figure S3). PCA and PLS-DA of the 37 components revealed clear distinctions between the groups, and the heatmap results further confirmed group clustering. This suggests that these components could potentially be used as biomarkers in the future.
6 Conclusion
This study demonstrated significant alterations in the plasma metabolites of horses following RG consumption, particularly affecting lipid metabolism. The identified compounds, including oleanolic acid, ursolic acid, and ginsenoside Rb1–known for their antioxidant and anti-inflammatory properties–emerged as potential biomarkers for RG intake. This study provides insights into the metabolic effects of RG and contributes to a broader understanding of its potential health benefits. Additionally, this study serves as a foundation for tailored health management and safety assessments associated with long-term RG consumption.
Data availability statement
The original contributions presented in the study are included in the article/Supplementary material, further inquiries can be directed to the corresponding author/s.
Ethics statement
The animal study was approved by Korea Racing Authority IACUC-2207-AEC-2207. The study was conducted in accordance with the local legislation and institutional requirements.
Author contributions
YK: Conceptualization, Data curation, Formal analysis, Funding acquisition, Investigation, Methodology, Project administration, Resources, Software, Supervision, Validation, Visualization, Writing – original draft, Writing – review & editing. IS: Writing - review & editing HY: Conceptualization, Data curation, Formal analysis, Funding acquisition, Investigation, Methodology, Project administration, Resources, Software, Supervision, Validation, Visualization, Writing – original draft, Writing – review & editing. JY: Conceptualization, Data curation, Formal analysis, Funding acquisition, Investigation, Methodology, Project administration, Resources, Software, Supervision, Validation, Visualization, Writing – original draft, Writing – review & editing.
Funding
The author(s) declare that financial support was received for the research, authorship, and/or publication of this article. This study was supported by the Horse Industry Research Center of the Korea Racing Authority, the National Research Foundation of Korea (NRF) grant funded by the Korean government (2023K2A9A1A01098682 and RS-2023-00217123).
Conflict of interest
The authors declare that the research was conducted in the absence of any commercial or financial relationships that could be construed as a potential conflict of interest.
Publisher’s note
All claims expressed in this article are solely those of the authors and do not necessarily represent those of their affiliated organizations, or those of the publisher, the editors and the reviewers. Any product that may be evaluated in this article, or claim that may be made by its manufacturer, is not guaranteed or endorsed by the publisher.
Supplementary material
The Supplementary material for this article can be found online at: https://www.frontiersin.org/articles/10.3389/fvets.2024.1425089/full#supplementary-material
Footnotes
1. ^https://gnps.ucsd.edu/ProteoSAFe/static/gnps-splash.jsp
2. ^https://www.metaboanalyst.ca
3. ^LIPEA, https://hyperlipea.org
References
1. Kim, HJ, Cho, CW, Hwang, JT, Son, N, Choi, JH, Shim, GS, et al. Lc-Ms-based metabolomic analysis of serum and livers from red ginseng-fed rats. J Ginseng Res. (2013) 37:371–8. doi: 10.5142/jgr.2013.37.371
2. Lee, KJ, and Ji, GE. The effect of fermented red ginseng on depression is mediated by lipids. Nutr Neurosci. (2014) 17:7–15. doi: 10.1179/1476830513Y.0000000059
3. Kim, SH, and Park, KS. Effects of Panax ginseng extract on lipid metabolism in humans. Pharmacol Res. (2003) 48:511–3. doi: 10.1016/s1043-6618(03)00189-0
4. Keum, YS, Park, KK, Lee, JM, Chun, KS, Park, JH, Lee, SK, et al. Antioxidant and anti-tumor promoting activities of the methanol extract of heat-processed ginseng. Cancer Lett. (2000) 150:41–8. doi: 10.1016/s0304-3835(99)00369-9
5. Lee, S, and Rhee, DK. Effects of ginseng on stress-related depression, anxiety, and the hypothalamic-pituitary-adrenal axis. J Ginseng Res. (2017) 41:589–94. doi: 10.1016/j.jgr.2017.01.010
6. Fan, S, Zhang, Z, Su, H, Xu, P, Qi, H, Zhao, D, et al. Panax ginseng clinical trials: current status and future perspectives. Biomed Pharmacother. (2020) 132:110832. doi: 10.1016/j.biopha.2020.110832
7. Kwak, YB, Yu, J, Im, EJ, Jeong, BS, and Yoo, HH. Study of cobalt doping control via various routes in thoroughbred horses. Drug Test Anal. (2022) 14:718–23. doi: 10.1002/dta.3192
8. Kwak, YB, Yu, J, and Yoo, HH. Simultaneous liquid chromatography tandem mass spectrometric determination of 35 prohibited substances in equine plasma for doping control. Mass Spectrom Lett. (2022) 13:158–65. doi: 10.5478/MSL.2022.13.4.158
9. Kwak, YB, Yu, J, and Yoo, HH. A quantitative method for the simultaneous detection of Sr9009 and Sr9011 in equine plasma using liquid chromatography-electrospray ionization-tandem mass spectrometry. Drug Test Anal. (2022) 14:1532–8. doi: 10.1002/dta.3271
10. Kwak, YB, Rehman, SU, and Yoo, HH. Quantification of three prohibited anabolic-androgenic steroids in equine urine using gas chromatography-tandem mass spectrometry. Mass Spectrom Lett. (2023) 14:104–9. doi: 10.5478/MSL.2023.14.3.104
11. Kwak, YB, and Choi, MS. Identification of a metabolite for the detection of the hydrophilic drug diisopropylamine for doping control. J Pharm Biomed Anal. (2023) 234:115576. doi: 10.1016/j.jpba.2023.115576
12. Kwak, YB, Seo, JI, and Yoo, HH. Exploring metabolic pathways of anamorelin, a selective agonist of the growth hormone secretagogue receptor, via molecular networking. Pharmaceutics. (2023) 15:2700. doi: 10.3390/pharmaceutics15122700
13. Li, X, Liu, J, Zuo, TT, Hu, Y, Li, Z, Wang, HD, et al. Advances and challenges in ginseng research from 2011 to 2020: the phytochemistry, quality control, metabolism, and biosynthesis. Nat Prod Rep. (2022) 39:875–909. doi: 10.1039/d1np00071c
14. Wu, W, Jiao, C, Li, H, Ma, Y, Jiao, L, and Liu, S. Lc-Ms based metabolic and metabonomic studies of Panax ginseng. Phytochem Anal. (2018) 29:331–40. doi: 10.1002/pca.2752
15. Lianqun, J, Xing, J, Yixin, M, Si, C, Xiaoming, L, Nan, S, et al. Comprehensive multiomics analysis of the effect of ginsenoside Rb1 on hyperlipidemia. Aging (Albany NY). (2021) 13:9732–47. doi: 10.18632/aging.202728
16. Lin, JH, and Lu, AY. Role of pharmacokinetics and metabolism in drug discovery and development. Pharmacol Rev. (1997) 49:403–49.
17. Roussel, C, Witt, KL, Shaw, PB, and Connor, TH. Meta-analysis of chromosomal aberrations as a biomarker of exposure in healthcare workers occupationally exposed to antineoplastic drugs. Mutat Res Rev Mutat Res. (2019) 781:207–17. doi: 10.1016/j.mrrev.2017.08.002
18. Marrer, E, and Dieterle, F. Impact of biomarker development on drug safety assessment. Toxicol Appl Pharmacol. (2010) 243:167–79. doi: 10.1016/j.taap.2009.12.015
19. Fielden, MR, Brennan, R, and Gollub, J. A gene expression biomarker provides early prediction and mechanistic assessment of hepatic tumor induction by nongenotoxic chemicals. Toxicol Sci. (2007) 99:90–100. doi: 10.1093/toxsci/kfm156
20. Hook, SE, Gallagher, EP, and Batley, GE. The role of biomarkers in the assessment of aquatic ecosystem health. Integr Environ Assess Manag. (2014) 10:327–41. doi: 10.1002/ieam.1530
21. Pang, Z, Zhou, G, Ewald, J, Chang, L, Hacariz, O, Basu, N, et al. Using MetaboAnalyst 5.0 for Lc-Hrms spectra processing, multi-omics integration and covariate adjustment of global metabolomics data. Nat Protoc. (2022) 17:1735–61. doi: 10.1038/s41596-022-00710-w
22. Dührkop, K, Fleischauer, M, Ludwig, M, Aksenov, AA, Melnik, AV, Meusel, M, et al. Sirius 4: a rapid tool for turning tandem mass spectra into metabolite structure information. Nat Methods. (2019) 16:299–302. doi: 10.1038/s41592-019-0344-8
23. Nothias, LF, Petras, D, Schmid, R, Dührkop, K, Rainer, J, Sarvepalli, A, et al. Feature-based molecular networking in the Gnps analysis environment. Nat Methods. (2020) 17:905–8. doi: 10.1038/s41592-020-0933-6
24. Attele, AS, Wu, JA, and Yuan, CS. Ginseng pharmacology: multiple constituents and multiple actions. Biochem Pharmacol. (1999) 58:1685–93. doi: 10.1016/s0006-2952(99)00212-9
25. Park, TY, Hong, M, Sung, H, Kim, S, and Suk, KT. Effect of Korean red ginseng in chronic liver disease. J Ginseng Res. (2017) 41:450–5. doi: 10.1016/j.jgr.2016.11.004
26. Lim, KH, Kang, CW, Choi, JY, and Kim, JH. Korean red ginseng induced cardioprotection against myocardial ischemia in guinea pig. Korean J Physiol Pharmacol. (2013) 17:283–9. doi: 10.4196/kjpp.2013.17.4.283
27. Yang, WK, Kim, SW, Youn, SH, Hyun, SH, Han, CK, Park, YC, et al. Respiratory protective effects of Korean red ginseng in a mouse model of particulate matter 4-induced airway inflammation. J Ginseng Res. (2023) 47:81–8. doi: 10.1016/j.jgr.2022.05.008
28. Lee, B, Sur, B, and Oh, S. Neuroprotective effect of Korean red ginseng against single prolonged stress-induced memory impairments and inflammation in the rat brain associated with BDNF expression. J Ginseng Res. (2022) 46:435–43. doi: 10.1016/j.jgr.2021.08.002
29. Kwak, YB, Lee, E, Choi, H, Park, T, Kim, A, Kim, J, et al. A pharmacokinetic study on red ginseng with furosemide in equine. Front Vet Sci. (2023) 10:1319998. doi: 10.3389/fvets.2023.1319998
30. Elghandour, MMMY, Kanth Reddy, PR, Salem, AZM, Ranga Reddy, PP, Hyder, I, Barbabosa-Pliego, A, et al. Plant bioactives and extracts as feed additives in horse nutrition. J Equine Vet. (2018) 69:66–77. doi: 10.1016/j.jevs.2018.06.004
31. Pearson, W, Omar, S, and Clarke, AF. Low-dose ginseng (Panax quinquefolium) modulates the course and magnitude of the antibody response to vaccination against equid herpesvirus I in horses. Can J Vet Res. (2007) 71:213–7.
32. Tang, QY, Chen, G, Song, WL, Fan, W, Wei, KH, He, SM, et al. Transcriptome analysis of Panax zingiberensis identifies genes encoding oleanolic acid glucuronosyltransferase involved in the biosynthesis of oleanane-type ginsenosides. Planta. (2019) 249:393–406. doi: 10.1007/s00425-018-2995-6
33. Liu, J . Pharmacology of oleanolic acid and ursolic acid. J Ethnopharmacol. (1995) 49:57–68. doi: 10.1016/0378-8741(95)90032-2
34. Ruszkowski, P, and Bobkiewicz-Kozlowska, T. Natural triterpenoids and their derivatives with pharmacological activity against neurodegenerative disorders. Mini Rev Org Chem. (2014) 11:307–15. doi: 10.2174/1570193X1103140915111559
35. Gong, L, Yin, J, Zhang, Y, Huang, R, Lou, Y, Jiang, H, et al. Neuroprotective mechanisms of ginsenoside Rb1 in central nervous system diseases. Front Pharmacol. (2022) 13:914352. doi: 10.3389/fphar.2022.914352
36. Shi, YH, Li, Y, Wang, Y, Xu, Z, Fu, H, and Zheng, GQ. Ginsenoside-Rb1 for ischemic stroke: a systematic review and meta-analysis of preclinical evidence and possible mechanisms. Front Pharmacol. (2020) 11:285. doi: 10.3389/fphar.2020.00285
37. Manju, BN . Exploring the potential therapeutic approach using ginsenosides for the management of neurodegenerative disorders. Mol Biotechnol. (2024) 66:1520–36. doi: 10.1007/s12033-023-00783-2
38. Li, N, Zhou, L, Li, W, Liu, Y, Wang, J, and He, P. Protective effects of ginsenosides Rg1 and Rb1 on an Alzheimer’s disease mouse model: a metabolomics study. J Chromatogr B. (2015) 985:54–61. doi: 10.1016/j.jchromb.2015.01.016
39. Ferreira, HB, Neves, B, Guerra, IM, Moreira, A, Melo, T, Paiva, A, et al. An overview of lipidomic analysis in different human matrices of multiple sclerosis. Mult Scler Relat Disord. (2020) 44:102189. doi: 10.1016/j.msard.2020.102189
40. Mullen, TD, and Obeid, LM. Ceramide and apoptosis: exploring the enigmatic connections between sphingolipid metabolism and programmed cell death. Anticancer Agents Med Chem. (2012) 12:340–63. doi: 10.2174/187152012800228661
41. Lee, Y-S, Yoo, J-M, Shin, H-W, Kim, D-H, Lee, Y-M, Yun, Y-P, et al. Protective effect of ginsenoside Rgl on H2O2-induced cell death by the decreased ceramide level in Llc-Pk1 cells. J Ginseng Res. (2006) 30:1–7. doi: 10.5142/JGR.2006.30.1.001
42. Xiao, N, Yang, LL, Yang, YL, Liu, LW, Li, J, Liu, B, et al. Ginsenoside Rg5 inhibits succinate-associated lipolysis in adipose tissue and prevents muscle insulin resistance. Front Pharmacol. (2017) 8:43. doi: 10.3389/fphar.2017.00043
43. Fisher, M . Lehninger principles of biochemistry, 3rd edition; by David L. Nelson and Michael M. Cox. Chem Educ. (2001) 6:69–70. doi: 10.1007/s00897000455a
44. Yi, J-S, Choo, H-J, Cho, B-R, Kim, H-M, Kim, Y-N, Ham, Y-M, et al. Ginsenoside Rh2 induces ligand-independent Fas activation via lipid raft disruption. Biochem Biophys Res Commun. (2009) 385:154–9. doi: 10.1016/j.bbrc.2009.05.028
45. Shin, SS, and Yoon, M. Korean red ginseng (Panax ginseng) inhibits obesity and improves lipid metabolism in high fat diet-fed castrated mice. J Ethnopharmacol. (2018) 210:80–7. doi: 10.1016/j.jep.2017.08.032
46. Li, Z, Kim, HJ, Park, MS, and Ji, GE. Effects of fermented ginseng root and ginseng berry on obesity and lipid metabolism in mice fed a high-fat diet. J Ginseng Res. (2018) 42:312–9. doi: 10.1016/j.jgr.2017.04.001
47. Rodriguez-Cuenca, S, Pellegrinelli, V, Campbell, M, Oresic, M, and Vidal-Puig, A. Sphingolipids and glycerophospholipids – the “Ying and Yang” of lipotoxicity in metabolic diseases. Prog Lipid Res. (2017) 66:14–29. doi: 10.1016/j.plipres.2017.01.002
48. Ratan, ZA, Youn, SH, Kwak, Y-S, Han, C-K, Haidere, MF, Kim, JK, et al. Adaptogenic effects of Panax ginseng on modulation of immune functions. J Ginseng Res. (2021) 45:32–40. doi: 10.1016/j.jgr.2020.09.004
49. Ye, X, Zhang, H, Li, Q, Ren, H, Xu, X, and Li, X. Structural-activity relationship of rare ginsenosides from red ginseng in the treatment of Alzheimer’s disease. Int J Mol Sci. (2023) 24:8625. doi: 10.3390/ijms24108625
50. Shin, KO, Seo, CH, Cho, HH, Oh, S, Hong, SP, Yoo, HS, et al. Ginsenoside compound K inhibits angiogenesis via regulation of sphingosine Kinase-1 in human umbilical vein endothelial cells. Arch Pharm Res. (2014) 37:1183–92. doi: 10.1007/s12272-014-0340-6
51. Shin, KO, Choe, SJ, Uchida, Y, Kim, I, Jeong, Y, and Park, K. Ginsenoside Rb1 enhances keratinocyte migration by a Sphingosine-1-phosphate-dependent mechanism. J Med Food. (2018) 21:1129–36. doi: 10.1089/jmf.2018.4246
52. Johnson, EL, Heaver, SL, Waters, JL, Kim, BI, Bretin, A, Goodman, AL, et al. Sphingolipids produced by gut bacteria enter host metabolic pathways impacting ceramide levels. Nat Commun. (2020) 11:2471. doi: 10.1038/s41467-020-16274-w
53. Choi, SH, Jung, SW, Lee, BH, Kim, HJ, Hwang, SH, Kim, HK, et al. Ginseng pharmacology: a new paradigm based on Gintonin-lysophosphatidic acid receptor interactions. Front Pharmacol. (2015) 6:245. doi: 10.3389/fphar.2015.00245
54. Shergis, JL, Zhang, AL, Zhou, W, and Xue, CC. Panax ginseng in randomised controlled trials: a systematic review. Phytother Res. (2013) 27:949–65. doi: 10.1002/ptr.4832
Keywords: red ginseng, metabolomics, biomarkers, sphingolipid metabolism, glycerophospholipid metabolism
Citation: Kwak YB, Stambler I, Yoo HH and Yoon J (2024) Metabolomic analysis of the impact of red ginseng on equine physiology. Front. Vet. Sci. 11:1425089. doi: 10.3389/fvets.2024.1425089
Edited by:
Xiangjun Qiu, Henan University of Science and Technology, ChinaCopyright © 2024 Kwak, Yoo and Yoon. This is an open-access article distributed under the terms of the Creative Commons Attribution License (CC BY). The use, distribution or reproduction in other forums is permitted, provided the original author(s) and the copyright owner(s) are credited and that the original publication in this journal is cited, in accordance with accepted academic practice. No use, distribution or reproduction is permitted which does not comply with these terms.
*Correspondence: Hye Hyun Yoo, eW9vaGhAaGFueWFuZy5hYy5rcg==; Jungho Yoon, anVuZ2hveTExQGdtYWlsLmNvbQ==