- 1Department of Industrial and Systems Engineering, University of Wisconsin Madison, Madison, WI, United States
- 2Department of Industrial and Systems Engineering, Texas A&M University, College Station, TX, United States
- 3Department of Civil and Coastal Engineering, University of Florida, Gainesville, FL, United States
Background: Training is essential for responder performance during emergencies, which are filled with uncertainties and stress. Virtual Reality (VR) offers a safe, repeatable, and cost-effective training tool for simulating stressful emergency scenarios.
Objective: This study aimed to evaluate firefighters’ performances in a VR-based emergency response training scenario by using a visuospatial sequence learning task to assess learning and retrieval effectiveness under stress in VR.
Methods: Forty firefighters from the local community were randomly assigned to either a control group or a stress training group, and they completed the VR-based visuospatial learning task, followed by two sets of retrieval tasks (one in a routine condition and the other in an emergency situation). Eye-tracking measures (such as gaze behavior and pupillometry), perceptions of workload and anxiety, and task performance were collected from both groups.
Results: While the stress training group exhibited poorer performance scores and longer operation times than the control group, the retrieval of learned information was similar. These findings were associated with lower gaze entropy, larger pupil dilation, and constriction in the stress training groups, especially during the initial training trials, along with heightened perceptions of mental demand, effort, frustration, and lower perceived performance.
Discussion: Eye-tracking data obtained from VR headsets can provide insights into individual cognitive states under various environmental stressors that may be utilized to create more adaptive training paradigms.
1 Introduction
Firefighters are emergency responders who specialize in extinguishing fire, rescuing people from dangerous situations, and performing medical assistance. They are usually the first responders who arrive at a scene of disaster or accident (Bureau of Labor Statistics, 2019; National Fire Protection Association, 2019). Firefighting is a stressful job because it requires high physical demands and the ability to make correct decisions quickly while in various emergencies (Horn et al., 2018; Michaelides et al., 2011; Williams-Bell et al., 2015). In 2018 alone, 64 firefighters lost their lives, and 58,250 injuries were reported while on duty in the United States (Campbell and Molis, 2019). To combat this, there is much emphasis on training to upskill and reskill emergency response skills (Bureau of Labor Statistics, 2019).
Hands-on physical training that mimics real-world scenarios, such as search and rescue, fire extinguishments, and triages, is effective in introducing emergency stressors of intense heat, smoke-filled environments, time pressure, and fear of possible injuries (Beaton et al., 1998; De Kloet et al., 2005). These trainings are extremely valuable as they allow firefighters to fully immerse themselves in emergency environments and effectively learn the required skills in the relevant contexts discussed above. Unfortunately, this training can also be very dangerous; for example, 8,175 cases of firefighter injuries were reported during emergency response training in 2018 (Campbell and Molis, 2019). Additionally, such real-world simulations make it more challenging for trainers to systematically and continuously measure training effectiveness. Finally, such simulations are expensive and occur infrequently due to budgetary and resource constraints (Engelbrecht et al., 2019), thereby reducing the number of times firefighters can experience and learn in such stressful environments.
Prior literature on learning and memory has established that the effectiveness of information retrieval post-training is state-dependent, particularly under stress (Cahill et al., 2003; Smeets et al., 2007). Learning and memory processes are interlinked, as learning is an acquisition of new knowledge, and memory highlights the capacity to hold and retrieve acquired knowledge (Baddeley, 1992; Zimmerman, 1990). Stress can impact both these processes and has either facilitative or detrimental effects on learning. These effects are dependent on when the stressor is applied–stress is facilitative during encoding and detrimental during consolidation (King et al., 2017; Vogel and Schwabe, 2016). Due to the nature of firefighters’ work and the conditions in which they operate during firefighting, firefighters need to be able to retrieve their knowledge gained under stress; therefore, it is essential to test learning and retrieval under stress for firefighters.
Virtual Reality (VR) is an emerging training tool for firefighters and other emergency responders (Cha et al., 2012; Narciso et al., 2020; Xu et al., 2014). VR-based learning supports firefighters to train in all types of real-world simulated stressful emergencies while in a physically safe environment (Freina and Ott, 2015). VR-based learning also allows for repetitions, a critical feature of skill acquisition that has shown a positive impact on memory recognition and retrieval (Buchsbaum et al., 2015; Hintzman, 1976). Hands-on live fire training, however, is not easily repeatable due to its extensive setup time and the inability to replicate an exact scenario (Kinateder et al., 2014). In contrast, there is almost no setup time required in VR and scenarios can be replicated on an as-needed basis (Kinateder et al., 2014). A VR-based training platform allows for online (or real-time) assessment of learning via integrated bioinstrumentation (such as eye tracking, motion capture, and physiological sensing) that is difficult to deploy in live-fire training drills (Engelbrecht et al., 2019). Many studies compared the effectiveness of VR-based firefighter training to existing real-world training or examined the stress levels of participants after they went through VR-based occupational training. For instance, Bliss et al. (1997) investigated the effectiveness of VR-based navigation training by comparing it with route memorization with blueprints and with no training at all. The study found that VR can be an effective training method, as both VR and blueprint groups performed better than those without training, but performance was comparable between the two groups. According to Narciso et al. (2020), virtual environments were effective at causing a high level of spatial presence, but not at provoking a similar level of physiological response to real environments. Studies found that VR-based occupational training designed for police officers (i.e., chasing a suspect and school shooting) induced stress on police officers (Groer et al., 2010; Strahler and Ziegert, 2015). While VR-based training systems offer a multitude of advantages over real-world training scenarios, there has been little work that examined the efficacy of state- and context-dependent learning, particularly under stress, in virtual simulations (Mehta et al., 2022).
Thus, two knowledge bases are well established: (1) VR-based occupational training can successfully induce stress, and (2) stress exposure training impacts learning. However, it is not clear if the impact of stress exposure training outcomes is similarly observed in VR environments that simulate stressors and whether these outcomes are accompanied by eye-tracking metrics of learners’ cognitive (i.e., learning) states. Addressing this knowledge gap is important for further evaluating if VR is an effective training medium for emergency responders to learn under stress. This study aimed to measure firefighters’ performances in a VR-based emergency response training scenario by adopting a visuospatial sequence learning task to determine the effectiveness of learning and retrieval under stress in VR. Two alternative emergency response training scenarios in VR were developed, one for routine (control) and one for emergency (stress) power plant shutdown maintenance. In the emergency state, stress was intended to be induced during the learning and retrieval phases. Participants’ training was evaluated based on their ability to retain information over time using task performance metrics (e.g., accuracy and time to complete). Based on prior literature on state-dependent learning (King et al., 2017; Vogel and Schwabe, 2016), we hypothesized that, in the VR environment, (a) the group trained in the routine (i.e., control), scenario would exhibit better performance than the group trained in the emergency (i.e., stress) scenario, and that (b) the group trained under stress will perform better than the group trained without stress during retrieval under stress.
2 Materials and methods
2.1 Participants
A total of 40 male firefighters from the local fire department volunteered to participate in this study, with a mean (SD) of age, Body Mass Index (BMI), and work experience of 30.74 (4.19) years, 29.43 (4.16) kg/m2, and 6.9 (3.99) years, respectively. While efforts were made to recruit a gender-balanced study pool, due to the lack of women in the response community, the study was limited to recruiting male firefighters. For the study, all firefighters were eligible for the experiment, and firefighters were randomly assigned to either a control (i.e., routine) training group or a stress (i.e., emergency) training group. Only one participant had prior experience with VR. However, he had VR sickness and discontinued the experiment. A total of six firefighters were unable to finish the experiment task due to VR sickness during the experiment, and the final 34 participants did not have any prior experience. This study was approved by the University’s Institutional Review Board.
2.2 Virtual environment and the experimental task
Emergencies in industrial settings, such as power plants and chemical plants, have additional dangerous components like combustible chemicals and other hazardous materials (Tsai et al., 2018). Firefighters need to shut down main valves to prevent these materials from feeding the fire or causing an explosion (Ben-Daya et al., 2009). The experimental task required that participants memorize a predetermined sequence to turn/close the valves to shut down the system. One minute was given to each participant to provide urgency as they needed to memorize the sequence in a short period and perform the shutdown procedure as quickly as possible to prevent the release of toxic materials, loss of fire containment, or additional explosions. This specific training was chosen as visuospatial working memory capacity is critical during emergency response, particularly for wayfinding and emergency shutdown procedures (Hund, 2016). Often, firefighters are given blueprints of buildings or structural/assembly information of complex structures at the emergency site. Developing visuospatial working memory capacity is thus critical for them to quickly understand, learn, and use information given to them at a rapid pace (Du et al., 2019). Participants were instructed to memorize an 8-step sequence, as shown in Figure 1 in three trials. The sequence of turning the virtual valves was developed based on the operation instruction manual of Alfa Laval plate heat exchangers (AlfaLaval, 2016).
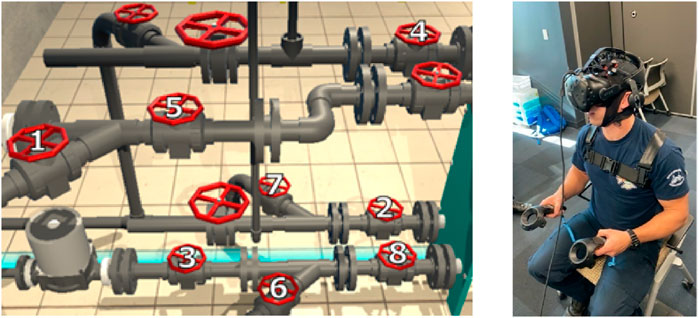
Figure 1. The order of the 8-step sequence (left) and participant during baseline and calibration (right).
The experiment was conducted in an immersive virtual environment, and both the control and stress training groups were provided with the same plate heat exchanger in a virtual operation room. Participants could see the limited space boundary and were told not to go beyond the boundary of the operating room. The participant could interact freely with the virtual valves and plate heat exchanger using the HTC controllers. In the stress training group, 1 out of the trials in Familiarization and 3 out of 8 trials in the Training Phase did not contain any stressors. The participants performed Trial 3 in Familiarization and Trial 1, 5, and 8 in the Training Phase without any stressors. The stressors included simulated smoke gradually occluding the vision, virtual fire propagation, virtual smoke propagation, sudden structural collapse sound, and fire burning sound in the distance. The purpose of adding these stressors in the virtual environment was to simulate the emergency shutdown scenario as realistically as possible. The control group performed their trials in the absence of any sudden stressors.
2.3 VR system with eye-tracking function
Owing to the difficulty of simulating emergency pipe operations in the real world, we developed a VR system integrated with an eye-tracking function based on our previously well-validated VR systems (Shi et al., 2019; Shi et al., 2016; Shi et al., 2020a; Shi et al., 2020c). To collect high-precision and high-resolution gaze movement data, the Tobii Pro eye tracker integrated with the HTC VIVE Head Mounted Display (HMD) (Tobii, 2019b) was used in this study. The VR HMD has a field of view of 110° and a display resolution of 1,440 × 1,600 pixels per eye for the dual displays. The Tobii Pro VR integration eye-tracker has an accuracy of 0.5°, and the maximum gaze data output frequency is 120 Hz. We developed several custom C# scripts based on the Tobii Pro Software Development Kit (SDK) (Tobii, 2019a) and the application programming interface in Unity to achieve the eye-tracking and playback functions in the virtual environment. The Unity 3D-5.6.3f1 version was used to create the VR environment, and the valve block was created based on the plate heat exchanger model from SketchUp. A computer with an Intel Xeon CPU at 2.60 GHz with 64 GB of RAM and a graphic card NVIDIA GTX 1080 was used to run the program. In the virtual environment, the system collected participants’ gaze movement data, head movement data, hand movement data, and pupil diameter data with a frequency of 90 Hz. After each VR experimental trial, the developed VR system automatically generated a CSV file with all the raw data.
2.4 Measurements
2.4.1 Task performance
Task performances during the training and retrieval phases were quantified based on the valve sequence accuracy score and operation time (s). The accuracy score was computed as the number of consecutive correct valves closed within each trial, and the score ranged from 1 to 8 (8 being the best performance). Incorrect actions included selecting the wrong valve or skipping the correct valve. Operation time was recorded when the participants entered the VR environment and ended when they touched the last valve. If the participants were not able to complete the sequence within the preset time (1 min), then the participants exited the VR environment, and the operation time was recorded as 1 min. Operation time per correct valve closure was calculated to account for different accuracies displayed by participants in the training and retrieval phases.
2.4.2 Stationary gaze entropy
Stationary gaze entropy (SGE) -
where
2.4.3 Pupillometry
Pupil dilation is a well-established physiological phenomenon associated with increased cognitive load and working memory development, and pupil constriction is a physiological phenomenon associated with distraction (Goldinger and Papesh, 2012; Kucewicz et al., 2018; Papesh et al., 2012; Peinkhofer et al., 2019). However, existing literature does not provide a readily available approach for pupillary data analysis under different light response effects and individual variability. We developed a novel pupil diameter analysis approach to evaluate participants’ cognitive status based on their pupil diameter data collected by eye trackers and by ruling out the influence of the light emitted from the lens of the VR headset based on our previous study (Shi et al., 2020b). This approach allows us to capture participants’ pupil dilation and constriction related to working memory development in real-time in dynamically changing scenarios, particularly those associated with varying light intensities such as fires and explosions. We selected two pupil dilation and constriction features, which are average and maximum pupil dilation and constriction (mm). The baseline data of pupil diameter was collected at the beginning of the study. Participants’ pupil diameter data were collected as soon as they entered the VR environment and stopped as soon as they touched the last valve. The average pupil dilation was calculated as the mean value of pupil dilation, and the maximum pupil dilation was calculated as the maximum pupil dilation against the baseline per each trial. An increase in pupil dilation is linked to higher cognitive load, associated with increased task difficulty or added decision-making requirements of a task (Einhauser et al., 2010; Hyönä et al., 1995). Pupil constriction has been associated with mind-wandering and distraction, and increased pupil constriction can be related to an increase in distraction level (Franklin et al., 2013; Huijser et al., 2018).
2.4.4 Mental workload
The NASA Task Load Index (TLX) was used to measure each participant’s subjective workload (Hart and Staveland, 1988). The NASA-TLX is scored from 1 to 21 for six dimensions (mental demand, physical demand, temporal demand, performance, effort, and frustration), and the higher score connects to a perceived workload increase (Hart and Staveland, 1988). There is a negative correlation between workload and performance when learning a new task, and workload can be used as indicative of learning (Yurko et al., 2010). The NASA-TLX was measured during the baseline, familiarization, and training phase and executed verbally as participants were wearing the VR headset.
2.4.5 Anxiety
The State-Trait Anxiety Inventory (STAI) was used for measuring the participants’ anxiety in both state anxiety (at any moment) and general trait anxiety (Spielberger, 1983). The STAI contains 20 questions for each state and each question is scored from 1 to 4, where a high score represents a high anxiety level (Spielberger, 1983). State anxiety is shown to negatively impact learning, which leads to poor performance (Eysenck and Calvo, 1992; Macher et al., 2012). The STAI was measured during the baseline, familiarization, and training phase and executed verbally as participants were wearing the VR headset.
2.5 Experimental protocol
Participants in both groups completed the entire experimental protocol, described in Figure 2. Upon consent, firefighters were familiarized with the study protocol, questionnaires (e.g., explanation and differentiation between various questions in the NASA TLX and STAI forms), VR equipment (headset and controllers), and emergency scenarios. During the study, the participants were required to keep the VR headset on the entire time to stay immersed in the VR environment, thus requiring NASA TLX and STAI questionnaires to be administered verbally (Hart, 2006; Kennedy and Hamilton, 1997; Mastro et al., 1985). To remain consistent with this verbal administration of questionnaires throughout the study, the baseline NASA TLX and STAI questionnaires were also conducted verbally post-consent and experiment familiarization. The participants were given adequate time to experience the VR environment and learn how to use the controllers. The participants were told to only use the right-hand controller as only the right-hand controller activated the triggers while performing tasks in the VR. The participants had to physically move the controller in their right hand and touch the valves in VR to activate them before moving on to the next valve in the sequence. When performing the tasks in the VR, participants were instructed to touch the valves with the controller, which would then turn the valves in the virtual environment. No physical motion of turning the valves was needed to complete the task. At this time, six firefighters reported VR sickness and, thus, were unable to participate in the experimental session.
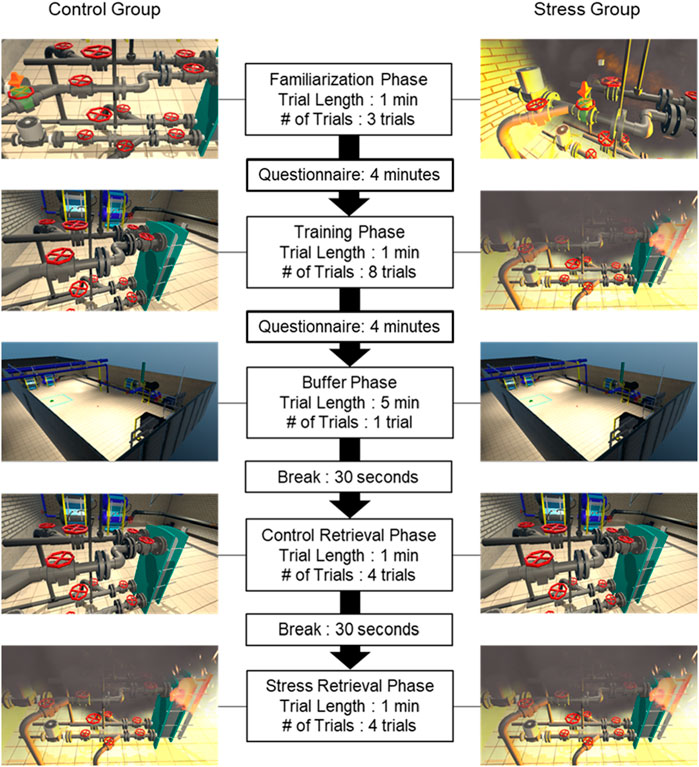
Figure 2. VR-training protocol and exemplar VR scenarios were presented to the control (left) and stress (right) training groups. The order of control and stress retrieval phases were counterbalanced within each training group.
Participants completed four experimental phases in a sequential manner: Familiarization, Training, Buffer, and Retrieval, as shown in Figure 2. Once the participants entered the VR environment, a series of pipes and a valve block were placed in front of them, and a five-point eye calibration was conducted while standing up before the experiment began.
In the Familiarization phase, virtual learning cues, such as arrows, were placed above the valves to let the participants know which valves to turn, and their goal was to follow the cues to memorize an eight-step valve closing sequence. The participants performed this trial a total of three times at their own pace, with a 1-min rest in between. After the Familiarization phase, both the NASA-TLX and STAI questionnaires were administered verbally, and the participants answered verbally while seated.
In the Training phase, the learning cues were removed from the valves, and participants were instructed to perform the eight-step sequence trial from memory as fast and as accurately as they could. Performance feedback was provided to the participants in the form of “You got everything correct” at the end of the trial or “That is incorrect” as soon as they selected the wrong valve. If they performed the sequence correctly, they repeated the trial without the arrows being present. If they performed the sequence incorrectly, the next trial included the learning cues (i.e., the virtual arrows). The time between trials was kept constant at 1 min. Each trial was set at an upper time limit of 1 min, and participants were able to relax until the next trial if they finished early. A total of eight training trials were provided, irrespective of whether they included a training or a familiarization trial. Note that throughout the familiarization and training phases, participants assigned to the control group experienced no stressors in the virtual operation room, while those assigned to the stress (emergency) training group performed the trials with different stressors as described earlier. After the Training phase, both the NASA-TLX and STAI questionnaires were administered verbally.
For the Buffer Phase, participants entered a scene that was similar to the room where they had been learning and training the sequence, except for a few key differences. There were no stressors for both control and stress training groups; the valve block that they had been interacting with was removed, and the participants were allowed to freely walk around the room and interact with objects that were placed in the room. Participants walked around in virtual reality by turning and swinging their arms, and most of them walked in place as this action was not controlled and left to their preferences. This buffer phase was essential to provide them with adequate time for memory consolidation (Nielson and Powless, 2007; Tse et al., 2007).
In the Retrieval phase, participants were instructed to perform the same eight-sequence trials as fast and accurately as they could. No performance feedback or learning cues were provided here. All participants, irrespective of their training group assignment, performed four trials in a control (routine) condition and four trials in a stress (emergency) condition. The order of the control and stress retrieval trial blocks were counterbalanced across the participant pool.
The control group familiarized itself with and learned the sequence without the presence of the stressors; however, the stressor training group familiarized itself with and learned the sequence in the presence of the stressors. During the retrieval phase, participants in both groups performed the sequence in both control and stress environments; therefore, the control group only experienced the stressors during the retrieval phase.
2.6 Statistical analysis
The statistical analysis tested the effects of differences between the control and the stress training groups. The presence of stressors is strictly related to the group as the control group’s familiarization and training trials did not include the stressors, but the stress training group’s trials did. The statistical analysis tested the effects of trials as trials were repeated multiple times per phase, and repetition allowed the participants to learn and perform better as the trials went on. We hypothesized that (a) the group trained in the routine (i.e., control) scenario would exhibit better performance than the group trained in the emergency (i.e., stress) scenario and that (b) the group trained under stress would perform better than the group trained without stress during retrieval under stress. While task performance metrics served as the primary dependent measures to test these hypotheses, gaze metrics and subjective responses served as secondary measures to elucidate differences in the underlying cognitive (i.e., learning) processes for the two groups. To test hypothesis (a), separate two-way mixed ANOVAs were conducted on the study-dependent measures during the familiarization and training phases (between-subjects: control, stress training group, and within-subjects: trials). To test hypothesis (b), separate two-way mixed ANOVAs were conducted on the study-dependent measures during the retrieval phases (between-subjects: control, stress training group, and within-subjects: control, stress retrieval).
The performance scores, gaze entropy, pupillometry, and subjective measures satisfied normality (Shapiro-Wilk test), homogeneity of variance (Levene’s Test), and sphericity (Mauchly’s sphericity test) assumptions. Separate two-way mixed ANOVAs were performed on the SGE (in the X, Y, and Z directions), average pupil dilation, average pupil constriction, maximum pupil dilation, and maximum pupil constriction measures during the familiarization phase to test the main and interactive effects of group (between-subjects: control, stress training group) and trials (within-subjects: first, second, third). For the training phase, these measures, along with performance scores, were subjected to two-way mixed ANOVAs to test the main and interactive effects of the group (between subjects: control, stress training group) and trials (within-subjects: early, middle, late). The early, middle, and late trials were comprised of trials 1 and 2, trials 3–6, and trials 7 and 8, respectively. Similarly, separate two-way mixed model ANOVAs were performed to compare the main and interaction effects of group (control, stress training) and retrieval type (control, stress retrieval) on performance and eye-tracking metrics. Separate two-way mixed model ANOVAs were performed to compare the main and interaction effects of group (between subjects: control, stress training) and phase (between subjects: familiarization, training) on each of the NASA-TLX sub-scales and STAI state and trait anxiety scores. Significance levels were set at α = 0.05. Post hoc analyses, corrected using Bonferroni correction, were conducted where needed.
3 Results
Statistical outputs for the performance and eye-tracking metrics across each phase are summarized in Table 1.
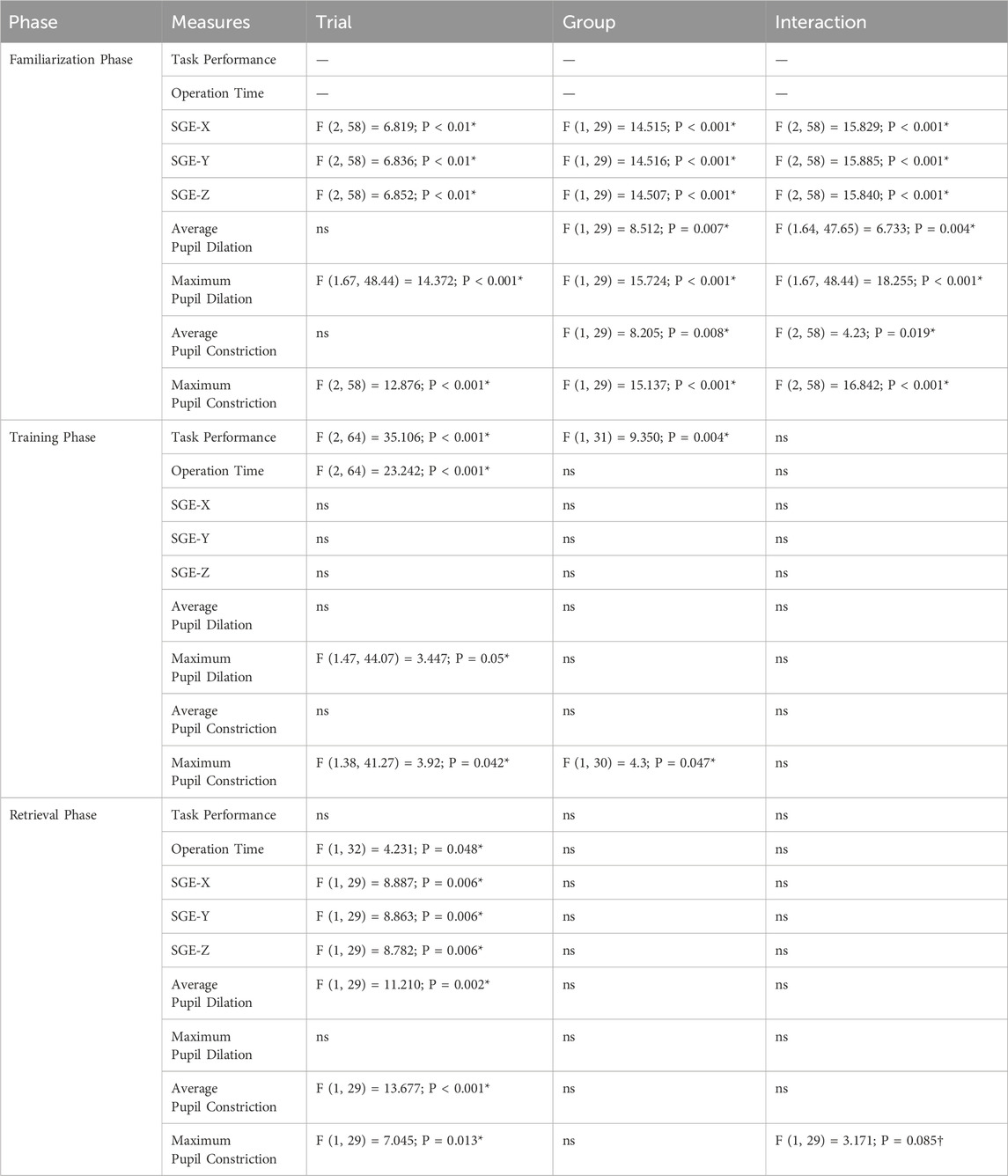
Table 1. Statistical analysis on task performance and eye-tracking metrics across each phase (*p < 0.05, †0.05 < p < 0.10, ns represents “not significant”).
3.1 Task performance
3.1.1 Training phase
Both training trial [training trial main effect: p < 0.001, η2 = 0.523] and group [group main effect: p = 0.004, η2 = 0.226] were found to significantly impact accuracy scores (Figure 3). However, training trials × group interaction was not found significant (p = 0.357). Post hoc analysis, using paired t-tests, revealed that accuracy scores were lower in the early training trials than in the middle and late training trials. In general, the control group exhibited higher scores than the stress training group. Training trial [training trial main effect: p < 0.001, η2 = 0.421] was found to significantly impact operation time per correct valve closed; however, group and training trials × group interaction were not found significant (both p’s > 0.1). Post hoc analysis, using paired t-tests, revealed that the operation times were higher in the early training trials than the middle and the late training trials.
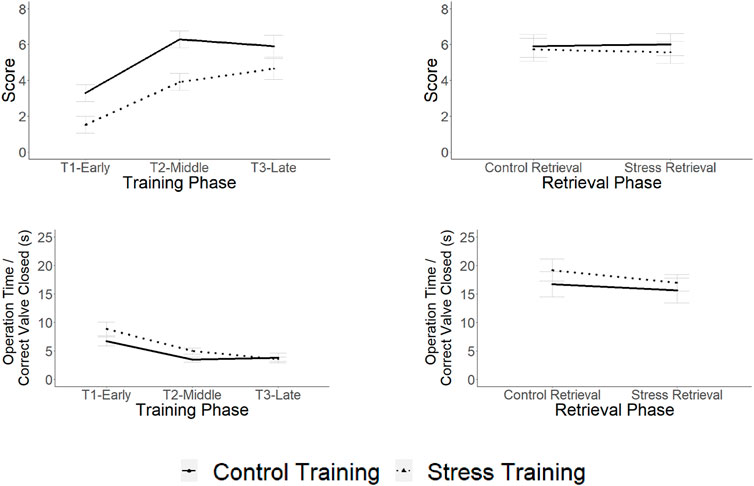
Figure 3. Performance scores and operation time per correct valve closed exhibited by the control and stress training groups during the training (left) and retrieval phases (right). Error bars denote standard error.
3.1.2 Retrieval phase
Accuracy scores during the retrieval were comparable between the two groups and across both the control and stress retrieval (all p’s > 0.4; Figure 3). Retrieval type [retrieval type main effect: p = 0.048, η2 = 0.117] was found to significantly impact operation time per correct valve closed; operation time per correct valve closed was higher in the control retrieval than the stress retrieval. Group and retrieval type × group interaction were not found significant (both p’s > 0.19).
3.2 Eye tracking metrics
3.2.1 Familiarization phase
The SGE measures, in all three directions, were significantly impacted by group, trial, and group × trial interaction effects however, only the SGE-X direction was shown as the other two directions were similar to SGE-X (Figure 4, top left panel). In general, greater SGE in X, Y, and Z directions were observed in the control than the stress training group [SGEx: p < 0.001, η2 = 0.247, SGEy: p < 0.001, η2 = 0.247, SGEz: p < 0.001, η2 = 0.247], and during the 1st compared to the 2nd or 3rd familiarization trial [SGEx: p < 0.01, η2 = 0.075, SGEy: p < 0.01, η2 = 0.075, SGEz: p < 0.01, η2 = 0.075]. The group × trial interaction effect significantly impacted SGE in all three directions [SGEx: p < 0.001, η2 = 0.158, SGEy: p < 0.001, η2 = 0.159, SGEz: p < 0.001, η2 = 0.158]. Post hoc analysis revealed that while the control training group exhibited greater SGE than the stress training group in the 1st and 2nd trials, SGE was comparable between the two groups in the 3rd trial.
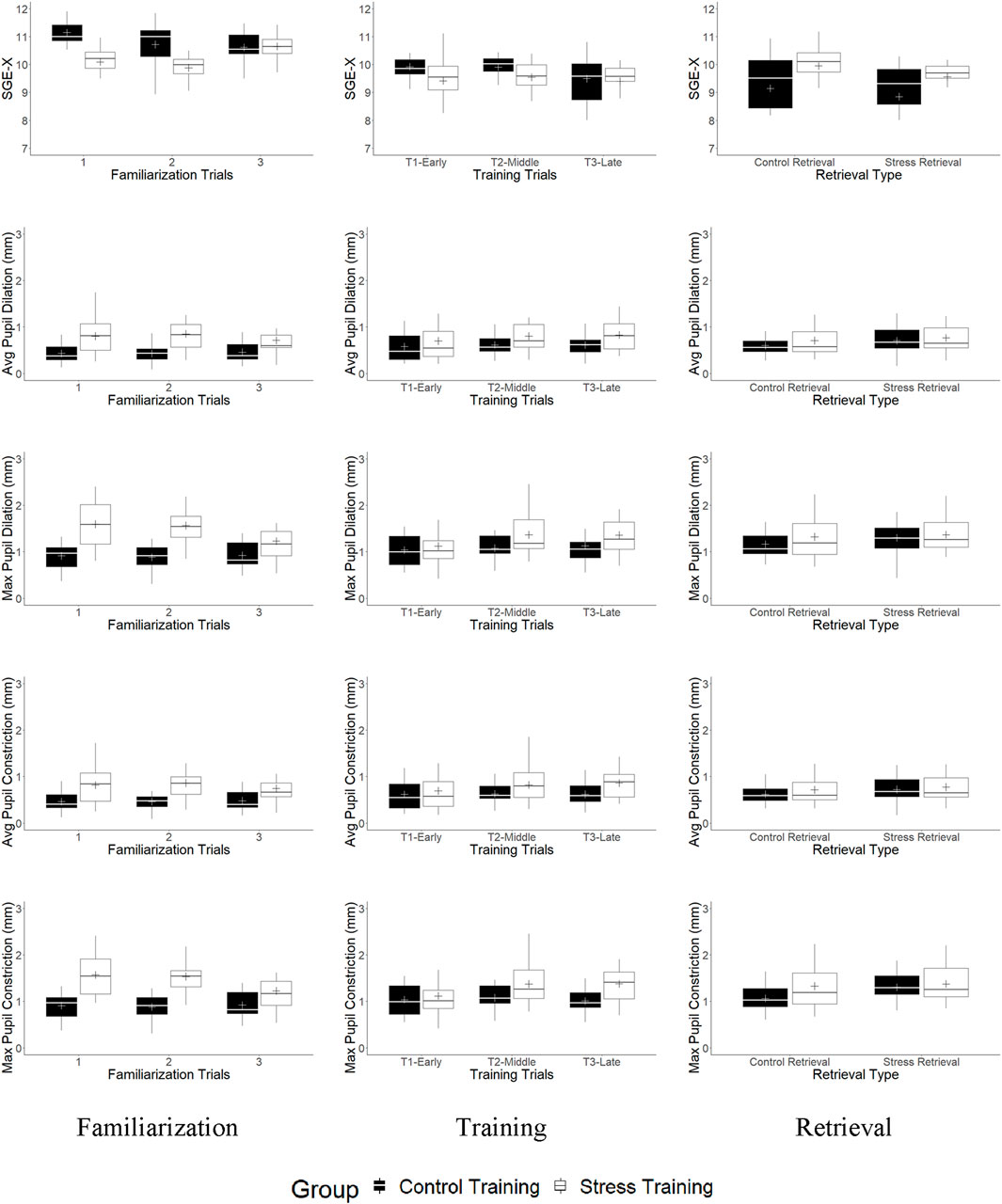
Figure 4. SGE-X, average pupil dilation, maximum pupil dilation, average pupil constriction, and maximum pupil constriction of the control (black box plots) and stress (white box plots) training group during familiarization (left column), training (middle column), and retrieval (right column) phases. The midline bar represents the median whereas + represents mean values. Upper whiskers are 75 percentiles + 1.5 IQR (Interquartile range) or maximum values and the lower whiskers are 25 percentiles - 1.5 IQR (interquartile range) or minimum values.
In general, the stress training group exhibited greater average pupil dilation than the control training group [group main effect: p = 0.007, η2 = 0.219]. While the main effect of the trial was not significant (p = 0.07), pupil dilation was significantly impacted by the group × trial interaction effect [p = 0.004, η2 = 0.011]. Post hoc analysis indicated that the stress training group exhibited larger pupil dilation than the control training group; however, this was only observed in the 1st and 2nd familiarization trials. In the 3rd trial, pupil dilation was comparable across groups. However, greater maximum pupil dilation was observed in the stress training group than in the control group [group main effect: p < 0.001, η2 = 0.333]. The trial had a significant effect on maximum pupil dilation [trial main effect: p < 0.001, η2 = 0.038]. The group × trial interaction effect [p < 0.001, η2 = 0.047] significantly affected pupil dilation, where the stress training group exhibited greater maximum pupil dilation than the control group, but only in the 1st and 2nd familiarization trials.
Similarly, greater average pupil constriction was observed in the stress training group than in the control group [group main effect: p = 0.008, η2 = 0.212]. The group × trial interaction effect [p = 0.019, η2 = 0.007] significantly affected pupil constriction, wherein the stress training group exhibited greater average pupil constriction than the control group for all three familiarization trials. Furthermore, greater maximum pupil constriction was observed in the stress training group than in the control group [group main effect: p < 0.001, η2 = 0.325]. The main effect of the trial was significant on maximum pupil constriction [trial main effect: p < 0.001, η2 = 0.034]. The group × trial interaction effect [p < 0.001, η2 = 0.044] significantly affected pupil constriction, where the stress training group exhibited greater maximum pupil constriction than the control group, but only in the 1st and 2nd familiarization trials.
3.2.2 Training phase
There were no main effects of group or trial, or their interaction, on the SGE (X, Y, Z), pupil dilation, or constriction measures (all p’s > 0.117). However, maximum pupil dilation was significantly affected by the trial [trial main effect: p = 0.05, η2 = 0.031]. Maximum pupil dilation was significantly higher in the middle and late training trials than in the early training trials. No other effects were observed on the maximum pupil dilation measure (both p’s > 0.126). Similarly, maximum pupil constriction was found to be higher for the stress training group [group main effect: p = 0.047, η2 = 0.1] and for the middle and late training trials compared to the early [training trial main effect: p = 0.042, η2 = 0.028].
3.2.3 Retrieval phase
The SGE measures, in all three directions, were significantly impacted by retrieval type; however, only the SGE-X direction was shown, as the other two directions were similar to SGE-X (Figure 4, top right panel). In general, greater SGE in X, Y, and Z directions were observed in the control retrieval than the stress retrieval for both training groups [Retrieval type main effect: SGEx: p = 0.006, η2 = 0.018, SGEy: p = 0.006, η2 = 0.018, SGEz: p = 0.006, η2 = 0.018]. No other effects were observed on the SGE measure (all p’s > 0.104).
Both groups exhibited greater average pupil dilation during the stress retrieval than the control retrieval [retrieval type main effect: p = 0.002, η2 = 0.015]. No other effects were observed on the average pupil dilation measure (both p’s > 0.332). No main effects of group or retrieval type or their interaction were observed for the maximum pupil dilation measure (both p’s > 0.230).
Similarly, both groups exhibited greater average pupil constriction during the stress retrieval than the control retrieval [retrieval type main effect: p < 0.001, η2 = 0.016]. No other effects were observed on the average pupil constriction measure (both p’s > 0.363). Likewise, both groups exhibited greater maximum pupil constriction during the stress retrieval than the control retrieval [retrieval type main effect: p = 0.013, η2 = 0.030]. However, the group x retrieval type interaction effect had a marginally significant impact [interaction effect: p = 0.085, η2 = 0.014]. There was no group main effect observed on the maximum pupil constriction measure (p = 0.249).
3.3 Subjective responses
Statistical outputs for the workload and anxiety scores are summarized in Table 2.
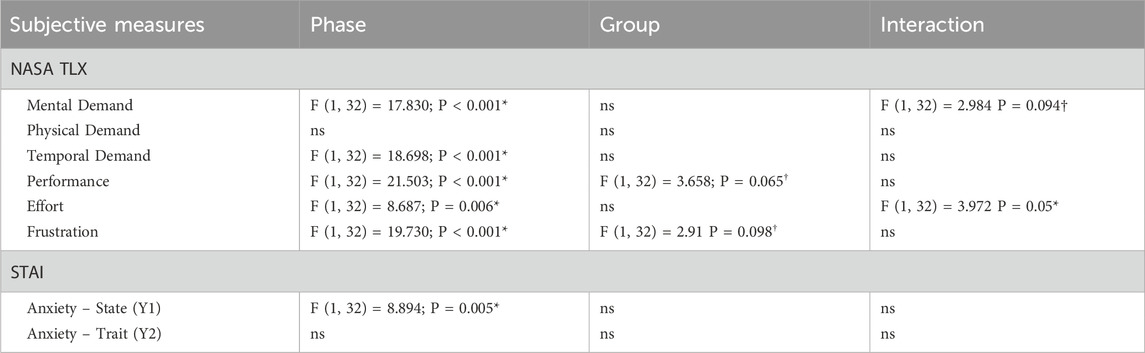
Table 2. Statistical analysis on subjective measures (*p < 0.05, †0.05 < p < 0.10, and ns represents “not significant”).
3.3.1 Mental workload
In general, both groups’ NASA-TLX scores (Figure 5) were significantly impacted by the phase main effect [Mental Demand: p < 0.001, η2 = 0.098, Temporal Demand: p < 0.001, η2 = 0.072, Performance: p < 0.001, η2 = 0.208, Effort: p = 0.006, η2 = 0.056, Frustration: p < 0.001, η2 = 0.117] except for the physical demand dimension. Furthermore, group [Performance: p = 0.065, η2 = 0.065, Frustration: p = 0.098, η2 = 0.067] and group × phase interaction [Mental Demand: p = 0.094, η2 = 0.018, Effort: p = 0.05, η2 = 0.027] effects had a marginally significant impact on the scores, whereby the stress group reported higher scores than the control group, especially during the training compared to the familiarization phase. No other effects were observed on all the other dimensions and phases (all p’s > 0.175).
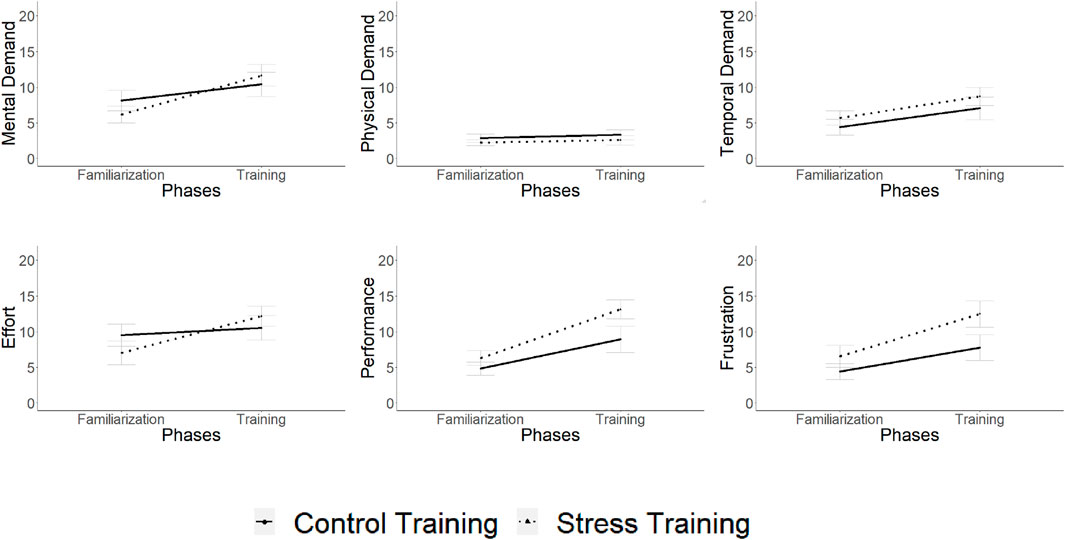
Figure 5. Mental demand, physical demand, temporal demand, performance, effort, and frustration from NASA-TLX after the familiarization and training phases. Error bars denote standard error.
3.3.2 Anxiety
There were no group differences found in the trait (Y2) anxiety scores of the STAI (M = 32.688; SE = 1.305; p = 0.926). However, both groups’ state (Y1) anxiety scores were impacted by phase [phase main effect: p = 0.005, η2 = 0.032]; participants reported greater anxiety during the training (M = 26.794; SE = 1.093) compared to the familiarization (M = 29.765; SE = 1.705) phase. Group and group × trial interaction effects on state anxiety were not found significant (both p’s > 0.430).
4 Discussion
The present study tested the overarching hypothesis that the effectiveness of VR-based emergency response training is state-dependent, meaning that training will be more effective under the specific conditions (routine vs. emergency) in which it is deployed. Key findings include: (1) while both groups showed similar improvements in operation time during the training phase, the control group achieved higher accuracy scores than the stress training group, supporting our first hypothesis; and (2) both training groups demonstrated comparable performance during the retrieval phase, which contradicted our second hypothesis. These findings were accompanied by notably different gaze behaviors, pupillometry measures, and subjective assessments, which can provide insights into VR-based learning under stress.
Both the control and stress training groups completed three trials to familiarize themselves with the 8-step sequence during the familiarization phase. The primary difference between the groups was that the first and second trials for the stress training group included environmental stressors. Performance scores for the control training group were significantly higher than those of the stress training group during the early part of the training phase. This difference in performance scores indicates that VR-simulated stressors affected the cognitive processes of the stress training group while learning the sequence, resulting in lower scores. The stress training group encountered VR-simulated stressors as soon as they entered the VR environment, and studies have shown that stress experienced before the encoding phase impairs memory formation (Elzinga et al., 2005; Vogel and Schwabe, 2016). The difference in performance scores due to learning under stress persisted throughout the training phase. While the control training group reached a performance plateau in the middle segment of training, the stress training group experienced a gradual increase in performance over the three segments. The environmental stressors encountered by the stress group likely had negative effects on learning and memory recall, even if these stressors did not entirely prevent learning (Fernandes and Moscovitch, 2000; Foerde et al., 2006). Stress has been shown to serve as a distractor during the encoding phase, which can divert attention from the primary task (Schwabe and Wolf, 2010). Although the group trained in the routine (control) scenario showed better performance than the group trained in the emergency (stress) scenario based on the performance scores during the training phase, both groups were able to achieve similar levels of learning, resulting in comparable performance in both scenarios during the retrieval phase. Ericsson (2004) reported that repeated practice often surpasses initial learning conditions, complicating the isolation of stress as a factor in retrieval performance. Future studies should control for practice effects by examining within-session performance trajectories or adjusting training trials, clarifying if learning differences stem from stress or cumulative task exposure. Both training groups exhibited significantly longer operation times during the early segment of the training phase compared to the middle and late segments. This aligns with previous work that reports shorter task completion times and greater speed in robotic surgical training tasks by novice users after just a few trials (Judkins et al., 2009). Interestingly, both training groups showed a shorter operation time per valve close under stress than routine retrieval conditions, even though both groups displayed similar retrieval performance scores.
Gaze entropy and pupillometry analyses revealed distinct eye movements among firefighters who participated in routine training compared to those who underwent stress familiarization and training. Firefighters in the stress training group demonstrated lower gaze entropy in the x, y, and z directions than those in the control training group. It is suggested that under intense focus, eye movement decreases while fixation duration increases (Hannula et al., 2010; Rayner, 1998). Thus, it is likely that to maintain their level of focus, the firefighters in the stress training group displayed more stable gaze behavior than those in the control training group during the familiarization phase. In the training phase, gaze behavior remained similar across both groups; in fact, the control group showed lower entropy during training than during familiarization. This focused attention likely led to a relatively faster learning process for this group than the stress group. Similarly, both groups demonstrated greater gaze stabilization under stress than under control retrieval.
Firefighters in the stress training group showed larger pupil dilations compared to those in the control training group, indicating a higher cognitive load in the stress training group (Kahneman and Beatty, 1966; Moresi et al., 2008; Schwalm et al., 2008; van der Wel and van Steenbergen, 2018). Interestingly, we also found that the firefighters in the stress training group showed greater pupil constriction than those in the control training group. Prior research has linked increased pupil constriction to higher levels of distraction in the field of view (Annerer-Walcher et al., 2018; Ayzenberg et al., 2018; Peinkhofer et al., 2019). Indeed, stress has also been described as a distraction during memory formation (Schwabe and Wolf, 2010). The increased pupil dilation and constriction observed in the stress training group during this study indicates a successful VR-based simulation of stressors and distractions, likely making it more applicable to the experiences firefighters face in emergencies. This is further supported by the fact that both groups showed greater pupil dilation during retrieval, but only when the retrieval trials included stressors.
It is important to note that the different gaze behaviors observed between the two groups, although statistically significant, may be influenced by how individuals react to stressors (Galatzer-Levy et al., 2013). The present study presented all the VR stressors uniformly across all emergency scenarios for the various phases (familiarization, training, and retrieval). After adjusting for light exposures, our findings can reasonably be associated with cognitive states (e.g., cognitive load and distraction), which can influence learning. Based on prior evidence (Wu et al., 2019), we anticipated that the stress training group would exhibit higher gaze entropy and greater pupillometric measures than the control training group. Interestingly, the results indicated that the stress training group had lower gaze entropy than the control group, particularly during the initial training trials. This aligns with eye-tracking findings that suggest that declines in gaze entropy with increased cognitive load serve as a strategy to enhance attentional focus on the task at hand (Schieber and Gilland, 2008; Ye et al., 2022). Alternatively, a reduction in gaze variability could reflect a narrowing of attentional scope rather than increased efficiency (Di Stasi et al., 2016). However, the stress training group showed higher pupillometric measurements than the control group during the familiarization phase, which could indicate heightened cognitive load in this group due to various VR stressors. This was also partially supported by increased perceived mental demand and frustration scores in the stress group during the training phase. It should be noted that firm conclusions cannot yet be drawn from the existing findings, as the present study did not conduct a formal analysis of the impacts of different VR stressors on gaze patterns between the two groups. Future studies are necessary to perform a task-specific analysis of this training and its effects on the different individualized gaze-based strategies that learners develop and/or adapt to. Additionally, future work may benefit from capturing potential individual learning rates (i.e., profiling or tracking performance changes over time) to address these variabilities in the pursuit of providing more personalized or adaptive training environments to enhance expertise development, particularly under stress.
Both perceived workload and anxiety rose from the familiarization to the training phases, an anticipated outcome due to the added cognitive load from learning (Sweller, 2011). While the state anxiety scores remained similar across both groups, the stress training group reported worse performance and greater frustration than the control training group during the familiarization and training phases. These findings further support the notion that the VR simulation effectively disrupted the trainees’ stress during learning, leading to poorer performance (Eysenck and Calvo, 1992; Macher et al., 2012). The authors suggest that the increased workload and stress are interconnected, making it difficult to distinguish between the two. This is especially true since the stressors create additional workload (as intended), and individuals experience stress when they cannot manage the increased demands (Alsuraykh et al., 2019; Parent et al., 2019). The greater mental demand and effort reported by the stress group during the training phase, compared to the control group, highlight that although they remained engaged in the learning process, VR-based stressors induced stress and disrupted their learning process. Indeed, prior research has suggested that self-report measures may not fully capture individual stress responses, which may have impacted the results (Kirschbaum et al., 1993). Future studies are needed that incorporate multimodal assessments of workload through neuroimaging and physiological sensing to capture these impacts (Abujelala et al., 2021; Tyagi et al., 2023).
The lack of a retrieval advantage under stress for the stress-trained group contradicted our second hypothesis and requires further clarification. We propose several alternative cognitive explanations for future exploration. First, the stress and control groups demonstrated similar retrieval behaviors, irrespective of stressors, which may suggest a ceiling effect on performance outcomes, as depicted in Figure 3. Future research should include transfer learning assessments with varied tasks that might be better suited to evaluate this hypothesis. Second, stress negatively impacts executive function and working memory, potentially negating any advantages of synchronizing encoding and retrieval conditions (Schwabe and Wolf, 2010). We did not gather workload and anxiety data post-retrieval, making it challenging to evaluate this interpretation. Third, despite heightened pupil dilation during initial coding phases, the stress group showed similar retrieval performance to the control group, regardless of stressors. There is a continuing debate about whether pupil dilation during encoding can forecast subsequent retrieval success (Einhäuser, 2017). While we found marginally significant results showing greater pupil constriction in the control group under stress compared to control retrieval, this was not observed in the stress-trained group. Increased constriction has been linked to familiarity and recollection, highlighting the critical function of expectancy in memory encoding (Kafkas, 2024). The absence of this response in the stress-trained group during stressful retrieval indicates cognitive factors that may have impeded effective retrieval. These potential explanations highlight the necessity of considering both pupil dilation and constriction as indicators of memory encoding, as well as the need to design transfer learning tasks that can evaluate their predictive capabilities.
Several limitations of the study need to be addressed. First, there was a lack of sex representation in this experiment (40 males). Sex differences may influence participants’ task performance and stress levels in this experiment. According to previous literature, sex is known to affect both physical and cognitive task performance. Males and females differ in how they handle stress (Bonneville-Roussy et al., 2017; Matud, 2004). We acknowledge that this is a significant limitation of this study, but we were constrained by the current demographics of the firefighting population within the local community. This also restricted any power analysis that might have guided the study design. Future research must explore how diversity among the subject population—encompassing various disciplines, backgrounds, ages, and sexes—affects training outcomes and associated learning rates and utilize the findings reported here for power analysis. Additionally, this study was conducted in a laboratory setting using a virtual simulation. Real-world scenarios are often more complex and unpredictable, and human responses in physical environments may differ significantly from those in virtual platforms. Research is needed to compare the similarities and differences in outcomes between virtual and physical environments for emergency response training. For instance, the enforced VR-based interactions, such as using controllers instead of natural arm or hand movements for valve closures, likely limited the emergence of natural interactions and behaviors from participants in either training group, potentially affecting the generalizability of the study’s findings. The VR stressors applied in this study consisted of a range of emergency response-related environmental challenges, including explosions and smoke, which, while broadly applicable, lack specificity regarding how different stressors provoke physiological stress in varying ways. Although there was no difference in anxiety scores between groups, the stress group displayed poorer learning outcomes than the control group, suggesting that these stressors interfered with the learning process. More objective measures of stress induction (e.g., physiological or biological markers) could enhance stress induction protocols. Future research that systematically assesses the impact of different stressors on learning and retrieval processes will be valuable in designing personalized and adaptive training platforms based on individual learning rates. Moreover, these findings can be leveraged to investigate VR’s effectiveness in cultivating domain-specific professional skills further.
5 Conclusion
The present study examined the effectiveness of VR-based emergency response training on a visuospatial sequence task among firefighters: a control (routine) group and a stress (emergency) group. We found that while training under stress resulted in slower learning processes compared to routine conditions, the retrieval of learned information was similar across stress and routine conditions. These findings were accompanied by distinctly different gaze behaviors observed in the two groups, which may help explain the comparable retrieval performances. The results highlight the potential of eye-tracking data obtained from VR headsets as a promising indicator of a user’s cognitive state under various environmental stressors. For instance, these indicators can aid in developing personalized training protocols (Abujelala et al., 2021; Mehta et al., 2022). Our findings indicated that the stress learning group required additional trials to learn the sequence to achieve the same level of performance as the control group, as they experienced a higher cognitive load during the learning process. Given the limited number of practices and dangers associated with live-fire training, practicing emergency scenarios in a VR environment can be beneficial before real-world training. Such VR training allows firefighters to repeat the task as much as needed at their own pace, facilitating thorough learning and improved performance, as repetition is crucial to learning. As part of the firefighter training process, VR training can provide effective learning and enhance safety.
Data availability statement
The raw data supporting the conclusions of this article will be made available by the authors, without undue reservation.
Ethics statement
The studies involving humans were approved by the Texas A&M University Institutional Review Board. The studies were conducted in accordance with the local legislation and institutional requirements. The participants provided their written informed consent to participate in this study.
Author contributions
RM: Conceptualization, Methodology, Project administration, Supervision, Writing – original draft, Writing – review and editing. JK: Data curation, Formal Analysis, Methodology, Writing – original draft, Writing – review and editing. YS: Data curation, Formal Analysis, Methodology, Software, Writing – original draft, Writing – review and editing. JD: Investigation, Methodology, Supervision, Writing – original draft, Writing – review and editing.
Funding
The author(s) declare that financial support was received for the research and/or publication of this article. This project was performed as part of the LEARNER project, funded by the National Science Foundation (#2033592, #2349138).
Conflict of interest
The authors declare that the research was conducted in the absence of any commercial or financial relationships that could be construed as a potential conflict of interest.
The author(s) declared that they were an editorial board member of Frontiers, at the time of submission. This had no impact on the peer review process and the final decision.
Generative AI statement
The author(s) declare that no Generative AI was used in the creation of this manuscript.
Publisher’s note
All claims expressed in this article are solely those of the authors and do not necessarily represent those of their affiliated organizations, or those of the publisher, the editors and the reviewers. Any product that may be evaluated in this article, or claim that may be made by its manufacturer, is not guaranteed or endorsed by the publisher.
References
Abujelala, M., Karthikeyan, R., Tyagi, O., Du, J., and Mehta, R. K. (2021). Brain activity-based metrics for assessing learning states in vr under stress among firefighters: an explorative machine learning approach in neuroergonomics. Brain Sci. 11 (7), 885. doi:10.3390/brainsci11070885
AlfaLaval (2016). Instruction manual - plate Heat exchangers. Available online at: https://www.alfalaval.com/globalassets/documents/products/heat-transfer/plate-heat-exchangers/gasketed-plate-and-frame-heat-exchangers/industrial/instruction-manual-gphe-small-en.pdf.
Alsuraykh, N. H., Wilson, M. L., Tennent, P., and Sharples, S. (2019). “How stress and mental workload are connected,” in Proceedings of the 13th EAI international conference on pervasive computing technologies for healthcare.
Annerer-Walcher, S., Körner, C., and Benedek, M. (2018). Eye behavior does not adapt to expected visual distraction during internally directed cognition. PloS one 13 (9), e0204963. doi:10.1371/journal.pone.0204963
Ayzenberg, V., Hickey, M. R., and Lourenco, S. F. (2018). Pupillometry reveals the physiological underpinnings of the aversion to holes. PeerJ 6, e4185. doi:10.7717/peerj.4185
Beaton, R., Murphy, S., Johnson, C., Pike, K., and Corneil, W. (1998). Exposure to duty-related incident stressors in urban firefighters and paramedics. J. Trauma. Stress Official Publ. Int. Soc. Trauma. Stress Stud. 11 (4), 821–828. doi:10.1023/a:1024461920456
Ben-Daya, M., Duffuaa, S. O., Raouf, A., Knezevic, J., and Ait-Kadi, D. (2009). Handbook of maintenance management and engineering, 7. Springer.
Bhavsar, P., Srinivasan, B., and Srinivasan, R. (2017). Quantifying situation awareness of control room operators using eye-gaze behavior. Computers & chemical engineering, 106, 191–201.
Bliss, J. P., Tidwell, P. D., and Guest, M. A. (1997). The effectiveness of virtual reality for administering spatial navigation training to firefighters. Presence Teleoperators and Virtual Environ. 6 (1), 73–86. doi:10.1162/pres.1997.6.1.73
Bonneville-Roussy, A., Evans, P., Verner-Filion, J., Vallerand, R. J., and Bouffard, T. (2017). Motivation and coping with the stress of assessment: gender differences in outcomes for university students. Contemp. Educ. Psychol. 48, 28–42. doi:10.1016/j.cedpsych.2016.08.003
Buchsbaum, B. R., Lemire-Rodger, S., Bondad, A., and Chepesiuk, A. (2015). Recency, repetition, and the multidimensional basis of recognition memory. J. Neurosci. 35 (8), 3544–3554. doi:10.1523/jneurosci.2999-14.2015
Bureau of Labor Statistics (2019). Occupational outlook handbook. Firefighters. Available online at: https://www.bls.gov/ooh/protective-service/firefighters.htm#tab-2.
Cahill, L., Gorski, L., and Le, K. (2003). Enhanced human memory consolidation with post-learning stress: interaction with the degree of arousal at encoding. Learn. and Mem. 10 (4), 270–274. doi:10.1101/lm.62403
Campbell, R., and Molis, J. (2019). United States firefighter injuries in 2018. Available online at: https://www.nfpa.org/News-and-Research/Publications-and-media/NFPA-Journal/2019/November-December-2019/Features/FF-Injuries.
Cha, M., Han, S., Lee, J., and Choi, B. (2012). A virtual reality based fire training simulator integrated with fire dynamics data. Fire Saf. J. 50, 12–24. doi:10.1016/j.firesaf.2012.01.004
De Kloet, E. R., Joëls, M., and Holsboer, F. (2005). Stress and the brain: from adaptation to disease. Nat. Rev. Neurosci. 6 (6), 463–475. doi:10.1038/nrn1683
Diaz-Piedra, C., Sanchez-Carrion, J. M., Rieiro, H., and Di Stasi, L. L. (2017). Gaze-based technology as a tool for surgical skills assessment and training in urology. Urology 107, 26–30. doi:10.1016/j.urology.2017.06.030
Di Stasi, L. L., Diaz-Piedra, C., Rieiro, H., Sanchez Carrion, J. M., Martin Berrido, M., Olivares, G., et al. (2016). Gaze entropy reflects surgical task load. Surg. Endosc. 30 (11), 5034–5043. doi:10.1007/s00464-016-4851-8
Du, J., Wang, Q., Lin, Y., and Ahn, C. (2019). “Personalize wayfinding information for fire responders based on virtual reality training data,” in Proceedings of the 52nd Hawaii international conference on system sciences.
Einhäuser, W. (2017). “The pupil as marker of cognitive processes,”. Computational and cognitive neuroscience of vision, 141–169. Springer.
Einhauser, W., Koch, C., and Carter, O. (2010). Pupil dilation betrays the timing of decisions. Front. Hum. Neurosci. 4, 18. doi:10.3389/fnhum.2010.00018
Elzinga, B. M., Bakker, A., and Bremner, J. D. (2005). Stress-induced cortisol elevations are associated with impaired delayed, but not immediate recall. Psychiatry Res. 134 (3), 211–223. doi:10.1016/j.psychres.2004.11.007
Engelbrecht, H., Lindeman, R., and Hoermann, S. (2019). A SWOT analysis of the field of virtual reality for firefighter training. Front. Robotics AI 6, 101. doi:10.3389/frobt.2019.00101
Ericsson, K. A. (2004). Deliberate practice and the acquisition and maintenance of expert performance in medicine and related domains. Acad. Med. 79 (10), S70–S81. doi:10.1097/00001888-200410001-00022
Eysenck, M. W., and Calvo, M. G. (1992). Anxiety and performance: the processing efficiency theory. Cognition and Emot. 6 (6), 409–434. doi:10.1080/02699939208409696
Fernandes, M. A., and Moscovitch, M. (2000). Divided attention and memory: evidence of substantial interference effects at retrieval and encoding. J. Exp. Psychol. General 129 (2), 155–176. doi:10.1037/0096-3445.129.2.155
Foerde, K., Knowlton, B. J., and Poldrack, R. A. (2006). Modulation of competing memory systems by distraction. Proc. Natl. Acad. Sci. 103 (31), 11778–11783. doi:10.1073/pnas.0602659103
Franklin, M. S., Broadway, J. M., Mrazek, M. D., Smallwood, J., and Schooler, J. W. (2013). Window to the wandering mind: pupillometry of spontaneous thought while reading. London, England: SAGE Publications Sage UK.
Freina, L., and Ott, M. (2015). “A literature review on immersive virtual reality in education: state of the art and perspectives,” in The international scientific conference eLearning and software for education.
Galatzer-Levy, I. R., Bonanno, G. A., Bush, D. E., and LeDoux, J. (2013). Heterogeneity in threat extinction learning: substantive and methodological considerations for identifying individual difference in response to stress. Front. Behav. Neurosci. 7, 55. doi:10.3389/fnbeh.2013.00055
Goldinger, S. D., and Papesh, M. H. (2012). Pupil dilation reflects the creation and retrieval of memories. Curr. Dir. Psychol. Sci. 21 (2), 90–95. doi:10.1177/0963721412436811
Groer, M., Murphy, R., Bunnell, W., Salomon, K., Van Eepoel, J., Rankin, B., et al. (2010). Salivary measures of stress and immunity in police officers engaged in simulated critical incident scenarios. J. Occup. Environ. Med. 52, 595–602. doi:10.1097/jom.0b013e3181e129da
Hannula, D. E., Althoff, R. R., Warren, D. E., Riggs, L., Cohen, N. J., and Ryan, J. D. (2010). Worth a glance: using eye movements to investigate the cognitive neuroscience of memory. Front. Hum. Neurosci. 4, 166. doi:10.3389/fnhum.2010.00166
Hart, S. G. (2006). NASA-task load index (NASA-TLX); 20 years later. Proc. Hum. factors ergonomics Soc. Annu. Meet. 50, 904–908. doi:10.1177/154193120605000909
Hart, S. G., and Staveland, L. E. (1988). Development of NASA-TLX (task load index): results of empirical and theoretical research. Adv. Psychol. 52, 139–183. doi:10.1016/s0166-4115(08)62386-9
Hintzman, D. L. (1976). Repetition and memory. Psychol. Learn. motivation 10, 47–91. doi:10.1016/s0079-7421(08)60464-8
Horn, G. P., Kesler, R. M., Kerber, S., Fent, K. W., Schroeder, T. J., Scott, W. S., et al. (2018). Thermal response to firefighting activities in residential structure fires: impact of job assignment and suppression tactic. Ergonomics 61 (3), 404–419. doi:10.1080/00140139.2017.1355072
Huijser, S., van Vugt, M. K., and Taatgen, N. A. (2018). The wandering self: tracking distracting self-generated thought in a cognitively demanding context. Conscious. Cognition 58, 170–185. doi:10.1016/j.concog.2017.12.004
Hund, A. M. (2016). Visuospatial working memory facilitates indoor wayfinding and direction giving. J. Environ. Psychol. 45, 233–238. doi:10.1016/j.jenvp.2016.01.008
Hyönä, J., Tommola, J., and Alaja, A.-M. (1995). Pupil dilation as a measure of processing load in simultaneous interpretation and other language tasks. Q. J. Exp. Psychol. 48 (3), 598–612. doi:10.1080/14640749508401407
Judkins, T. N., Oleynikov, D., and Stergiou, N. (2009). Objective evaluation of expert and novice performance during robotic surgical training tasks. Surg. Endosc. 23 (3), 590–597. doi:10.1007/s00464-008-9933-9
Kafkas, A. (2024). Eyes on memory: pupillometry in encoding and retrieval. Vision 8 (2), 37. doi:10.3390/vision8020037
Kahneman, D., and Beatty, J. (1966). Pupil diameter and load on memory. Science 154 (3756), 1583–1585. doi:10.1126/science.154.3756.1583
Kennedy, P., and Hamilton, L. R. (1997). Psychological impact of the management of methicillin-resistant Staphylococcus aureus (MRSA) in patients with spinal cord injury. Spinal Cord. 35 (9), 617–619. doi:10.1038/sj.sc.3100469
Kinateder, M., Ronchi, E., Nilsson, D., Kobes, M., Müller, M., Pauli, P., et al. (2014). “Virtual reality for fire evacuation research,” in 2014 federated conference on computer science and information systems.
King, B. R., Saucier, P., Albouy, G., Fogel, S. M., Rumpf, J.-J., Klann, J., et al. (2017). Cerebral activation during initial motor learning forecasts subsequent sleep-facilitated memory consolidation in older adults. Cereb. cortex 27 (2), 1588–1601. doi:10.1093/cercor/bhv347
Kirschbaum, C., Pirke, K.-M., and Hellhammer, D. H. (1993). The ‘Trier Social Stress Test’–a tool for investigating psychobiological stress responses in a laboratory setting. Neuropsychobiology 28 (1-2), 76–81. doi:10.1159/000119004
Kucewicz, M. T., Dolezal, J., Kremen, V., Berry, B. M., Miller, L. R., Magee, A. L., et al. (2018). Pupil size reflects successful encoding and recall of memory in humans. Sci. Rep. 8 (1), 4949. doi:10.1038/s41598-018-23197-6
Macher, D., Paechter, M., Papousek, I., and Ruggeri, K. (2012). Statistics anxiety, trait anxiety, learning behavior, and academic performance. Eur. J. Psychol. Educ. 27 (4), 483–498. doi:10.1007/s10212-011-0090-5
Mastro, J., French, R., Henschen, K., and Horvat, M. (1985). Use of the state-trait anxiety inventory for visually impaired athletes. Percept. Mot. Ski. 61 (3), 775–778. doi:10.2466/pms.1985.61.3.775
Matud, M. P. (2004). Gender differences in stress and coping styles. Personality Individ. Differ. 37 (7), 1401–1415. doi:10.1016/j.paid.2004.01.010
Mehta, R., Moats, J., Karthikeyan, R., Gabbard, J., Srinivasan, D., Du, E., et al. (2022). Human-centered intelligent training for emergency responders. AI Mag. 43 (1), 83–92. doi:10.1002/aaai.12041
Michaelides, M. A., Parpa, K. M., Henry, L. J., Thompson, G. B., and Brown, B. S. (2011). Assessment of physical fitness aspects and their relationship to firefighters' job abilities. J. Strength and Cond. Res. 25 (4), 956–965. doi:10.1519/jsc.0b013e3181cc23ea
Moresi, S., Adam, J. J., Rijcken, J., and Van Gerven, P. W. (2008). Cue validity effects in response preparation: a pupillometric study. Brain Res. 1196, 94–102. doi:10.1016/j.brainres.2007.12.026
Narciso, D., Melo, M., Raposo, J. V., Cunha, J., and Bessa, M. (2020). Virtual reality in training: an experimental study with firefighters. Multimedia Tools Appl. 79 (9), 6227–6245. doi:10.1007/s11042-019-08323-4
National Fire Protection Association (2019). Fire department calls. Available online at: https://www.nfpa.org/News-and-Research/Data-research-and-tools/Emergency-Responders/Fire-department-calls.
Nielson, K. A., and Powless, M. (2007). Positive and negative sources of emotional arousal enhance long-term word-list retention when induced as long as 30 min after learning. Neurobiol. Learn. Mem. 88 (1), 40–47. doi:10.1016/j.nlm.2007.03.005
Papesh, M. H., Goldinger, S. D., and Hout, M. C. (2012). Memory strength and specificity revealed by pupillometry. Int. J. Psychophysiol. 83 (1), 56–64. doi:10.1016/j.ijpsycho.2011.10.002
Parent, M., Peysakhovich, V., Mandrick, K., Tremblay, S., and Causse, M. (2019). The diagnosticity of psychophysiological signatures: can we disentangle mental workload from acute stress with ECG and fNIRS? Int. J. Psychophysiol. 146, 139–147. doi:10.1016/j.ijpsycho.2019.09.005
Peinkhofer, C., Knudsen, G. M., Moretti, R., and Kondziella, D. (2019). Cortical modulation of pupillary function: systematic review. PeerJ 7, e6882. doi:10.7717/peerj.6882
Rayner, K. (1998). Eye movements in reading and information processing: 20 years of research. Psychol. Bull. 124 (3), 372–422. doi:10.1037/0033-2909.124.3.372
Schieber, F., and Gilland, J. (2008). Visual entropy metric reveals differences in drivers' eye gaze complexity across variations in age and subsidiary task load. Proc. Hum. Factors Ergonomics Soc. Annu. Meet. 52, 1883–1887. doi:10.1177/154193120805202311
Schwabe, L., and Wolf, O. T. (2010). Learning under stress impairs memory formation. Neurobiol. Learn. Mem. 93 (2), 183–188. doi:10.1016/j.nlm.2009.09.009
Schwalm, M., Keinath, A., and Zimmer, H. D. (2008). “Pupillometry as a method for measuring mental workload within a simulated driving task,” in Human Factors for assistance and automation, 1–13.
Shannon, C. E. (2001). A mathematical theory of communication. ACM Sigmob. Mob. Comput. Commun. Rev. 5 (1), 3–55. doi:10.1145/584091.584093
Shi, Y., Du, J., Ahn, C. R., and Ragan, E. (2019). Impact assessment of reinforced learning methods on construction workers' fall risk behavior using virtual reality. Automation Constr. 104, 197–214. doi:10.1016/j.autcon.2019.04.015
Shi, Y., Du, J., Lavy, S., and Zhao, D. (2016). A multiuser shared virtual environment for facility management. Procedia Eng. 145, 120–127. doi:10.1016/j.proeng.2016.04.029
Shi, Y., Du, J., and Ragan, E. (2020a). Review visual attention and spatial memory in building inspection: toward a cognition-driven information system. Adv. Eng. Inf. 44, 101061. doi:10.1016/j.aei.2020.101061
Shi, Y., Du, J., and Worthy, D. A. (2020b). The impact of engineering information formats on learning and execution of construction operations: a virtual reality pipe maintenance experiment. Automation Constr. 119, 103367. doi:10.1016/j.autcon.2020.103367
Shi, Y., Zhu, Y., Mehta, R. K., and Du, J. (2020c). A neurophysiological approach to assess training outcome under stress: a virtual reality experiment of industrial shutdown maintenance using Functional Near-Infrared Spectroscopy (fNIRS). Adv. Eng. Inf. 46, 101153. doi:10.1016/j.aei.2020.101153
Shiferaw, B., Downey, L., and Crewther, D. (2019). A review of gaze entropy as a measure of visual scanning efficiency. Neurosci. and Biobehav. Rev. 96, 353–366. doi:10.1016/j.neubiorev.2018.12.007
Shiferaw, B. A., Downey, L. A., Westlake, J., Stevens, B., Rajaratnam, S. M., Berlowitz, D. J., et al. (2018). Stationary gaze entropy predicts lane departure events in sleep-deprived drivers. Sci. Rep. 8 (1), 2220–2310. doi:10.1038/s41598-018-20588-7
Smeets, T., Giesbrecht, T., Jelicic, M., and Merckelbach, H. (2007). Context-dependent enhancement of declarative memory performance following acute psychosocial stress. Biol. Psychol. 76 (1-2), 116–123. doi:10.1016/j.biopsycho.2007.07.001
Strahler, J., and Ziegert, T. (2015). Psychobiological stress response to a simulated school shooting in police officers. Psychoneuroendocrinology 51, 80–91. doi:10.1016/j.psyneuen.2014.09.016
Sweller, J. (2011). Cognitive load theory. Psychol. Learn. motivation 55, 37–76. doi:10.1016/b978-0-12-387691-1.00002-8
Tobii (2019a). Tobii Pro SDK. Available online at: https://www.tobiipro.com/product-listing/tobii-pro-sdk/.
Tobii (2019b). Tobii Pro VR integration. Available online at: https://www.tobiipro.com/product-listing/vr-integration/.
Tsai, S.-F., Huang, A.-C., and Shu, C.-M. (2018). Integrated self-assessment module for fire rescue safety in a chemical plant–A case study. J. Loss Prev. Process Industries 51, 137–149. doi:10.1016/j.jlp.2017.12.011
Tse, D., Langston, R. F., Kakeyama, M., Bethus, I., Spooner, P. A., Wood, E. R., et al. (2007). Schemas and memory consolidation. Science 316 (5821), 76–82. doi:10.1126/science.1135935
Tyagi, O., Hopko, S., Kang, J., Shi, Y., Du, J., and Mehta, R. K. (2023). Modeling brain dynamics during virtual reality-based emergency response learning under stress. Hum. Factors 65 (8), 1804–1820. doi:10.1177/00187208211054894
van der Wel, P., and van Steenbergen, H. (2018). Pupil dilation as an index of effort in cognitive control tasks: a review. Psychonomic Bull. and Rev. 25 (6), 2005–2015. doi:10.3758/s13423-018-1432-y
Vogel, S., and Schwabe, L. (2016). Learning and memory under stress: implications for the classroom. npj Sci. Learn. 1 (1), 16011–16110. doi:10.1038/npjscilearn.2016.11
Williams-Bell, F. M., Kapralos, B., Hogue, A., Murphy, B., and Weckman, E. (2015). Using serious games and virtual simulation for training in the fire service: a review. Fire Technol. 51 (3), 553–584. doi:10.1007/s10694-014-0398-1
Wu, C., Cha, J., Sulek, J., Zhou, T., Sundaram, C. P., Wachs, J., et al. (2019). Eye-tracking metrics predict perceived workload in robotic surgical skills training. Hum. Factors, 0018720819874544. doi:10.1177/0018720819874544
Xu, Z., Lu, X., Guan, H., Chen, C., and Ren, A. (2014). A virtual reality based fire training simulator with smoke hazard assessment capacity. Adv. Eng. Softw. 68, 1–8. doi:10.1016/j.advengsoft.2013.10.004
Ye, Y., Shi, Y., Xia, P., Kang, J., Tyagi, O., Mehta, R. K., et al. (2022). Cognitive characteristics in firefighter wayfinding Tasks: an Eye-Tracking analysis. Adv. Eng. Inf. 53, 101668. doi:10.1016/j.aei.2022.101668
Yurko, Y. Y., Scerbo, M. W., Prabhu, A. S., Acker, C. E., and Stefanidis, D. (2010). Higher mental workload is associated with poorer laparoscopic performance as measured by the NASA-TLX tool. Simul. Healthc. 5 (5), 267–271. doi:10.1097/sih.0b013e3181e3f329
Keywords: emergency response, virtual reality, learning, stress, eye tracking (ET)
Citation: Mehta RK, Kang J, Shi Y and Du J (2025) Effectiveness of training under stress in immersive VR: an investigation of firefighter performance, gaze entropy, and pupillometry. Front. Virtual Real. 6:1542507. doi: 10.3389/frvir.2025.1542507
Received: 09 December 2024; Accepted: 31 March 2025;
Published: 22 April 2025.
Edited by:
Laura Thomas, PARSEC Space, United KingdomReviewed by:
Kerstin Witte, Otto von Guericke University Magdeburg, GermanyJie Hao, Southeast Colorado Hospital, United States
Ruben F. Salinas, Harvard Business School, United States
Copyright © 2025 Mehta, Kang, Shi and Du. This is an open-access article distributed under the terms of the Creative Commons Attribution License (CC BY). The use, distribution or reproduction in other forums is permitted, provided the original author(s) and the copyright owner(s) are credited and that the original publication in this journal is cited, in accordance with accepted academic practice. No use, distribution or reproduction is permitted which does not comply with these terms.
*Correspondence: Ranjana K. Mehta, cm1laHRhMzhAd2lzYy5lZHU=
†Present address: Yangming Shi, Department of Civil and Environmental Engineering, Colorado School of Mines, Golden, CO, United States