- 1Laboratory of Applied Thermodynamics, Aristotle University of Thessaloniki, Thessaloniki, Greece
- 2Seidor Consulting S.L., Barcelona, Spain
- 3Department of Mechanical Engineering, Yildiz Technical University, Istanbul, Turkey
- 4Joint Research Centre of European Commission, Ispra, Italy
Heavy-duty vehicles constitute a significant contributor to road CO2 emissions, despite accounting for only a low share of the vehicle fleet. CO2 Emissions certification and monitoring are performed using vehicle simulation software designed for the purpose (VECTO). The European Union currently regulates rigid truck and tractor-trailer CO2 emissions and subsequently will proceed to buses and other heavy-duty vehicle categories. The current study investigated the use of VECTO on a city bus by modeling the on-road operating conditions of a vehicle in an urban route in Istanbul. The simulation results for constant auxiliary load showed a difference with the on-road measurements in the range of −1.6 to 3.2%, depending on the direction of the route. The difference was attributed to the influence of the total elevation change, and the use of auxiliaries. The latter comprise a significant part of energy consumption in buses, and for this reason, VECTO includes a dedicated bus auxiliary module. The use of the module was also explored, and it was found to improve the results in some cases. The findings highlight the need to assess the operation of auxiliary components in city buses accurately, and to consider the provision of more precise, auxiliary-component specific, information when running actual real-world CO2 simulations of these vehicles.
Introduction
Heavy-Duty Vehicles (HDVs) contribute 30% of road CO2 emissions despite comprising only 4% of the vehicle fleet (Muncrief and Sharpe, 2015). In this context, the European Commission has committed to reducing emissions to 60% of the 1990 levels by 2050 (European Commission, 2016) by setting up a monitoring and reporting mechanism of CO2 emissions, which also includes HDVs (Regulation (EU) 2018/956, 2018). HDV CO2 Emissions certification is performed using vehicle simulation software designed for the purpose, the Vehicle Energy and Consumption calculation Tool (VECTO). The European Union currently regulates rigid truck and tractor-trailer CO2 emissions and subsequently will proceed to buses and other heavy-duty vehicle categories. A key element to monitor and report HDV CO2 emissions is vehicle simulation, which was found to be an acceptable alternative to laboratory testing (Fontaras et al., 2014). Laboratory testing, as realized in light-duty vehicles, was considered to be not realistic due to the high customizability of the HDV models, the high testing cost and the relatively low number of total vehicles (Savvidis, 2015). In this way, a series of studies have already focused on trucks (White et al., 2017; TNO et al., 2018; Tansini et al., 2019), which were also the first to be regulated. Gradually focus has also started to extend to buses and coaches. As the environmental and economic focus on public transportation increases, the overall system optimization becomes the main topic for engineers and researchers. This optimization process usually focuses on vehicle and driveline besides the diesel engine. However, a complete approach is needed for assessing the energy demand of bus auxiliaries used in city buses for passenger comfort, vehicle safety, and improved driveability. The current study focused on the assessment of the operation of a city bus by performing a series of on-road measurements and subsequently comparing the measured results to the CO2 emissions simulated by VECTO. The respective vehicle models were built in VECTO in order to assess the potential of adequately simulating on-road emissions. It should also be taken into consideration that buses present a higher complexity in auxiliary use than trucks. In order to account for this, VECTO accounts for this by providing a bus auxiliary module that provides a more sophisticated approach for simulating these auxiliaries. The study initially approached the auxiliary usage by applying a constant auxiliary load, but in order to further investigate the issue, it also took a second approach by making use of the bus auxiliary module.
Methods
The Istanbul city rapid bus transport system-Metrobus was chosen for VECTO tool analyses. The Metrobus transportation line exhibits one of the longest public transportation axles in the world with 52 km length, where 550 buses operate at 24 h service, transferring daily over ~1 million passengers. The route of this axle consists of three main parts, and this research focused on the Beylikdüzü (B)-Avcilar (A) part. The tests were realized in both directions at two different payload conditions of 6,500 and 13,000 kg, which, respectively, corresponded to half and full allowable passenger loading capacity. However, taking into consideration safety issues, the passenger load was simulated with sandbags instead of real passengers. In order to simulate realistic driving conditions, the test vehicle was following—from a safe distance, a vehicle—that was actually in service. The relevant fuel consumption, emission and Controller Area Network (CAN) data was collected for validation and further analyses purposes. A part of the measurement results including the other pollutant emissions (NOx, CO, Soot) were evaluated and analyzed and they have been published in previous papers (Özener, 2017; Özener and Özkan, 2018). An expanded paper will be published about the measurement campaign that will describe in-detail the respective methodology, the safety concerns and the encountered difficulties.
In the next step, the relative VECTO vehicle models were developed and run on a cycle that derived based on the recorded route data. The following sections describe in detail the performed on-road measurements and the modeling process.
On-Road Measurements
The on-road measurements were realized with an articulated diesel fueled public bus, whose properties are presented in Table 1. The passenger load was simulated with sandbags, which were distributed homogenously in the bus as payload. Regarding the testing equipment, the bus was equipped with AVL KMA Mobile fuel consumption meter (FCM), and AVL MOVE portable emission measurement system (PEMS). The FCM was directly connected to the vehicles fuel system, and it used a servo controlled positive displacement meter, which was working with Pierburg Luftfahrtgeräte Union (PLU) measuring principle. It's measurement uncertainty (according to DIN 1319; confidence level P = 95%) accuracy of measurement value for volumetric measurement is ± 0.1 %, and accuracy of measurement value for density measurement for density ± 0.03 %, and accuracy of measurement value for mass measurement ± 0.1 % (AVL, 2013). The pressure difference created on fuel line arousing from FCM is ΔP = 0, with a measurement uncertainty of FCM of ±0.1%. The PEMS was used for recording real driving condition CO2 measurements, and the device was connected to exhaust pipe using non-dispersive infrared analyzers for CO2 analyses. The measurement sensitivity for CO2 measurement was ±0.1%. The selected vehicle data was logged from CAN of the bus with using a CAN logging tool, with the logged channels being Vehicle Speed, Current Gear, Engine Speed. Additionally, in order to retrieve vehicle position, a 2 Hz GPS device was used during on-road measurements for logging latitude, longitude, and GPS based altitude. All data flow was logged with AVL MOVE system controller computer synchronously. CO2 was measured by AVL M.O.V.E Gas PEMS that has got NDIR (Non-dispersive) analyzer. It's measurement range between 0 and 20% and display resolution 0.01% as volumetric. Measurement accuracy of analyzer are ±0.1 vol% absolute (for 0… 9.99% vol.) and ±2% relative (for 10… 20% vol.) (AVL, 2010a). The measurement system commissioning is given in Figure 1. Other emissions like carbon monoxide (CO), nitric oxides (NOx), and soot were also logged during measurements besides CO2, but they were not used for research purposes in the current work.
The calculation of the exhaust mass was realized based on the C balance method according to ISO16183 (AVL, 2010b). The method relies on the input of the fuel mass flow and on the measured exhaust gas composition. No CAN data was used for this calculation as the fuel mass flow was taken from the on-board fuel consumption meter, while the exhaust gas composition data was provided by the PEMS.
The AVL PEMS Post Processing Software working on AVL Concerto Program (AVL, 2019) was used for harmonizing the logged data and speed up post-processing. The logged data was filtered by downsampling the recording rate from 10 to 1 Hz with the moving average method and a drift correction was applied if required. Subsequently, the relative alignment of the data to the ECU and GPS data was applied.
Considering that under real world operation, the passenger load is continuously changing throughout the route in a crowded dedicated line and the shift in the overall vehicle has a direct impact on fuel consumption and CO2 emissions. Future public transportation Real Driving Emissions (RDE) tests should take into consideration the mass shift, as for safety reasons the current campaign made use of sandbags to simulate vehicle load instead of real passengers. In this context, the passenger load after each stop should be derived from CAN line (or with additional sensor added on pneumatic suspension system), but attention should be payed that any devices and instruments to be isolated and out of reach of passengers.
Test Route
The tests were carried on Istanbul Metrobus Line that consists of 3 main parts. The part Beylikdüzü (B)-Avcilar (A) with 12 stops was chosen for on-road measurements. The chosen part also has the highest altitude change (Δh = 173 m) of this dedicated line and the respective test route is presented in Figure 2.
Modeling
VECTO can run in Declaration or Engineering mode with the first being used for official certification purposes by using the predefined setting for a series of parameters according to the regulation (Regulation (EU) 2017/2400, 2018). Currently, the Declaration mode is targeted only for the regulated truck class of HDV class 4, 5, 9, and 10. The Engineering mode is used for providing more freedom to the user to test different vehicle configurations and types such as buses and coaches. The Engineering mode was used for the needs of the current investigation.
VECTO separates input in three major categories that correspond to the engine, vehicle and axle/gearbox and they contain the respective technical characteristics. The study retrieved the gathered on-road data and proceeded by converting the traveled route into a driving cycle and the measured data into VECTO compatible input format. The following paragraphs describe the approach to generate the related input data by VECTO categories.
In addition to the three major categories, the study included a fourth category that focuses on bus auxiliaries. Taking into consideration that buses have high energy auxiliary demand (Muncrief et al., 2012), VECTO offers a specific module that is targeted specifically for buses and describe in detail the auxiliaries that are deployed for proper vehicle operation. There was not enough on-road data to produce the exact auxiliary operation condition, and for this reason, the study had to rely on VECTO generic values.
The model was created in VECTO version 3.3.2.1548, and the tool was set into Engineering mode.
Engine
There was no OEM data available for the engine map and for this reason modeling approach was deployed to produce the engine map. In this context, the engine map was produced by taking into consideration engine technical characteristics such as engine type, capacity, fuel type, max torque, and power and it was simulated with a dedicated engine simulation software. A similar approach was deployed in previous research and it was found to deliver accurate results under transient conditions (Özener et al., 2018). The capability to simulate transient conditions properly led to setting the WHTC correction factor to 1. The engine category also requires vehicle idling speed, which was set to 560 RPM, the flywheel inertia that was set to 2.16 kg m2.
Vehicle
The vehicle category contains vehicle technical configuration that is separated in the following fields: general information, masses/loading, air resistance, dynamic tire radius, crosswind correction and axles/wheels. Table 2 presents the inputs for the general information, mass/payload, air resistance, and dynamic tire radius fields. Generic values from the literature were used for the aerodynamic drag coefficient (Holmberg et al., 2012; Wang and Rakha, 2016) in order to calculate the vehicle drag area. As city buses follow a standard body type that applies to all vehicles, it was considered that generic aerodynamic coefficient values are representative for the examined body type. The final calculation of the vehicle drag area was realized by multiplying the aerodynamic coefficient with the vehicle's frontal area. The rolling resistance was calculated principally based on the tire energy efficiency class, where a representative value was selected and it was validated with literature data (Wang and Rakha, 2017). The axle and wheel configuration was specified as in Table 3.
Axle and Gearbox
The axle and gearbox field contains information on the transmission type, gear ratio, torque converter and gear-shifting strategy. In the simulated model, the gearbox type was set to Automatic Transmission-Serial (AT-S) and the minimum gear-shifting time was set to 2 s. The gear-shifting strategy was derived from available data on the gearbox program that contained speed upshifting/downshifting speed and load signal for each gear. The data were converted to VECTO format that requires engine torque instead of the load signal. Regarding the axle and gear ratios, they were set as in Table 3.
In addition, VECTO developers are currently working on a new gearshifting strategy and as the relative on-road data were available, the current study also investigated the difference between the on-road and the VECTO gearshifting strategy. The comparison between the two maintained all the parameters the same except for the related gearshifting files.
The efficiency for the axle was set to a constant value of 0.95, which was considered a representative efficiency value based on the findings of a fleet-large analysis (Tansini et al., 2018).
Automatic transmissions are always engaged to the powertrain and for this reason, they require a torque converter. The torque converter adjusts torque transmission to the wheels at low speeds and when the vehicle is stopped. As this is an additional element in the powertrain it poses an additional source of losses, which is defined by a relative map that contains the output/input speed ratio and torque at specific reference engine speed. In order to quantify the transmission losses for both components, an in-house approach was used. The bus operated at predefined gears at various constant vehicle speed at a flat road for three payloads—no payload, 6,500 and 13,000 kg. The relevant CAN was logged during the process and it was used for developing the relative maps based on a methodology developed in a previous study (Özener et al., 2018).
Driving Cycles
The driving cycle was recreated from the recorded data from both trips and in order to accurately simulate the driving conditions it was chosen to use a time-based cycle. In a time-based cycle, the vehicle has a target speed that needs to reach at each timestep and this is achieved only if the configurations of the vehicle model and the actual vehicle match. This effectively led to the generation of two cycles, one for each tested payload valued.
The VECTO time-based cycle was generated by combing the timestamp, vehicle recorded speed and grade. The vehicle speed was derived from the CAN data, while the grade was produced from GPS altitude measurements. However, GPS altitude measurement data could contain significant noise and in order to counteract this, the following procedure was applied:
1. Create distance-based bins of 15 m.
2. Calculate median altitude value by bin.
3. Interpolate altitude values for every record for distance.
4. Calculate grade.
5. Replace outlier grade values that are over ±10% with the next closest valid value.
Figure 3 presents the GPS filtered altitude signal.
Driving Profile
VECTO has the capability of the implementing a driving profile that is based on the maximum acceleration and deceleration that the driver applies. According to VECTO terminology these are called acceleration/deceleration curves and they are defined by a vehicle speed bin for which is set a maximum acceleration/deceleration value. The foreseen VECTO vehicle speed bins are at 0, 25, 50, 60, and 120 km/h and these bins were also used to produce the relative curves. For each direction and payload, the measurements were separated into the respective bins and the acceleration/deceleration records were filtered by removing any value that was higher 2.5 times the standard deviation from the mean. Subsequently, the highest values were chosen for each vehicle speed bin.
Auxiliaries
The auxiliaries include the required devices that ensure vehicle operation and their power requirements can either be determined based on a related map or by applying a constant load. The primary auxiliary units of a public bus can be summarized as air conditioner (AC), cooling fan, compressor, water pump, and hydraulic pumps servomotor, alternator, and 24 V system. When analyzing the auxiliary power there are several factors that should be taken into consideration for analyzing the continuous auxiliary power demand, i.e., equipment duty cycle, time of the day (operating hours), season of the year (climatic conditions.) etc. (Yang et al., 2012). Andersson (2004) indicated that the combined auxiliary system loads are <10% of the driving power when accelerating, while it can be close to 30% of the average load power. He simulated that the auxiliary load of a conventional bus 9.3 kW including steering, cooling, compressor, and 24 V electrical system and showed that it is possible to save 8.2% of this energy with using new technologies and advanced control. Khan and Clark (2010) developed an empirical approach in determining the effect of road grade on fuel consumption from transit buses and assumed a power consumption of about 7.5 kW to run the auxiliary loads. Clark et al. (2007) reported that the auxiliary load on the engine can increase ~30 kW with cooling fan operation. Also at the final report of Assessment and Reliability of Transport Emission Models and Inventory Systems (ARTEMIS) (Boulter and McCrae, 2007) set the auxiliary load assumption to 2.5% of rated power for simulations of heavy-duty vehicles after fuel consumption sensitivity tests on chassis dynamometers. Pettersson and Johansson (2006) modeled the auxiliary loads in heavy vehicles for control purposes they found the aux load as between 8 and 10 kW for two different cycles. Vepsäläinen et al. (2019) applied a model-based approach for city buses and they proposed 6 kW of the air compressor operation was used for doors operation. In addition to that usage, the hydraulic power for steering and braking systems consumed 1.5 kW continuously and they estimated average power of 1 kW for other auxiliaries. They estimated that in the best scenario the auxiliary needs were 2 kW continuously, while the worst-case scenario (frequently opening doors. etc.) required 7 kW power consumption.
The current study took the same approach by applying a constant load on the engine that applied for the auxiliaries. The supported auxiliaries are the engine fan, alternator, A/C, air compressor and steering and the applied overall constant load was considered to be at 15 kW. The increased load was derived due to the use of the A/C as the ambient temperature was ~30°C and such systems can pose an additional power demand of up to 30 kW (KB AutoTech, 2019). According to Vepsäläinen et al. (2019) an average power demand at this temperature for the A/C would be about 11 kW. The use of other auxiliaries was considered at 4 kW as a best-case scenario. This approach was justified by the fact that the vehicle operated over the designated route, with virtually a constant auxiliary load, as the vehicle maintained the doors closed at the stops to ensure smooth execution of the experiment. In this sense, there were no losses due to loss of cooling, pneumatic operation of doors and kneeling. Figure 4 presents the auxiliary power demand scheme.
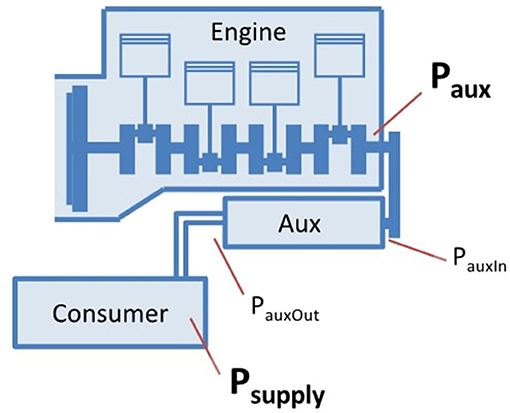
Figure 4. Auxiliary power demand scheme (European Commission TUG, 2019).
The process to calculate auxiliary power demand is as below:
1. Auxiliary speed
2. Auxiliary output power
3. Auxiliary input power
4. Auxiliary power consumption
5. Paux is added to the engine's power demand.
nEng: Calculated engine speed (1/min)
TransRatio: Speed ratio between auxiliary and engine. Defined in the Auxiliary File (-)
naux: Auxiliary speed (1/min)
Psupply: Effective supply power demand. Defined in the driving cycle (kW)
EffToSply: Consumer efficiency. Defined in the Auxiliary File (-)
PauxOut: Auxiliary output power (kW)
EffMap: Auxiliary efficiency map. Defined in the Auxiliary File (kW) = f((1/min), (kW))
PauxIn: Auxiliary input power (kW)
EffToEng: Efficiency of auxiliary (belt/gear) drive. Defined in the Auxiliary File (-)
Paux: Mechanical auxiliary power demand at the crank shaft (kW).
The study made use also of the bus auxiliary module, where the auxiliary technical characteristics were described in more detail and their usage would be closer to on-road conditions. The results of the runs with the bus auxiliary module were compared and validated to the ones with the constant load, which have derived from the relative literature and they could lay the groundwork for more sophisticated approaches in the future. Figure 5 presents the functionalities of the bus auxiliary module in comparison also to the standard auxiliaries module. The module deploys three additional submodules: electrics, pneumatics and Heat Ventilation Air Condition (HVAC) module.
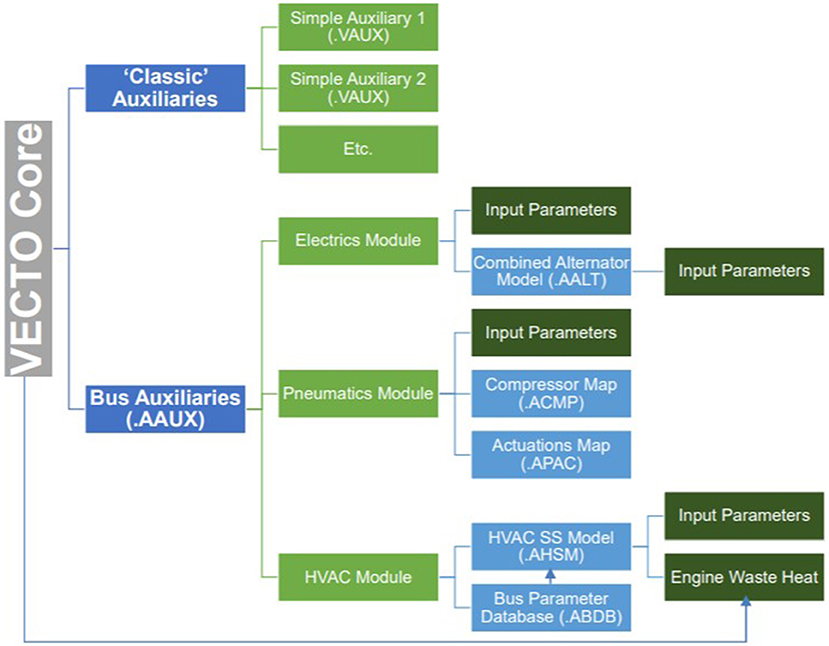
Figure 5. Bus auxiliaries functionalities scheme (Norris et al., 2016).
The bus auxiliaries require a series of parameters, which were not always readily available and in this case, the study made use of the VECTO generic input data. The modified values are as in Table 4.
Results and Discussion
Initially, the study compared the engine operating conditions between the on-road measurements and the simulation runs. Figure 6 presents a comparison of the engine operating points for the on-road measurements and for the simulation runs with constant auxiliary load-standard auxiliaries- and bus auxiliaries. The figure shows that in all cases the engine has a comparatively engine average speed. Considering only operation points with positive torque output, the average engine speed difference was ~5%. The engine speed difference presents the lowest difference at the AB direction and the highest difference at the BA direction, which can be attributed to the BA direction involving more downhill driving. In the on-road data, the driver applied more aggressive braking than in the VECTO simulations, which could result in lower engine speeds.
In the case of the 6,500 kg payload, the engine speed remains within the 1,300–1,700 RPM range for the simulation, while in the on-road measurements it was mainly within the 1,100–1,600 RPM range. However, this is not so apparent for the 13,000 kg payload, where the simulation operation points are also shifted to the right in a similar range, but most of them cluster at higher torque values along with the on-road operation points. This behavior could be attributed to the driver model and the gearshifting pattern, as the relative simulation data were generated through a process that involved filtering. In addition at a low payload, the real driver seemed to have a more dynamic driving pattern, while in the simulation the driver model maintained a more conservative profile. At a higher payload on the other hand, a dynamic driving profile in on-road conditions would be difficult to follow and it could impose also safety issues. In this sense, the driving behavior of the real driver and the VECTO driver model converge at a higher payload.
The use of the bus auxiliaries module inserts a more sophisticated simulation approach, which is reflected in the engine operation. Both runs show a similar operation at high loads, but they present a divergence at lower loads. The standard auxiliary run considered a constant auxiliary load which could be more representative at high loads and stable operating conditions. However, at lower engine loads, the auxiliary energy demand could become more complicated, which is better reflected with the use of the bus auxiliary module.
Figure 7 presents the distribution of acceleration events by run and it shows that the simulation has somewhat smoother positive acceleration events than the on-road measurements. On the other hand, the simulation also exhibits less aggressive braking. These findings confirm that the real driver had a more dynamic driving profile than the VECTO driver model. Taking into consideration only the negative acceleration, the simulation has a mean value between −0.49 m/s2 and the on-road measurements a mean value of −2.02 m/s2. The respective standard deviation values were −0.47 and −1.87. However, it should be highlighted that the on-road acceleration recordings could contain outliers as negative acceleration values at the range of −8 m/s2 seem unrealistic. Considering a filtering that would remove values that lie beyond 2.5 times the standard deviation from the mean, would remove all values that are below −6.7 m/s2.
The investigation looked into VECTO generic gearshifting and the on-road recorded gearshifting. It was found, as shown in Figure 8 that the VECTO gearshifting strategy resulted in lower fuel consumption.
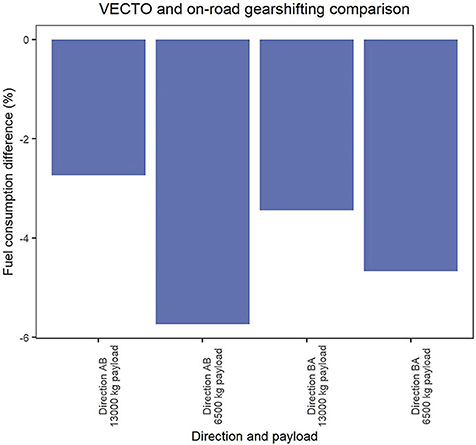
Figure 8. Comparison of fuel consumption between VECTO and on-road recorded gearshifting strategies.
The difference in driving behavior has an impact on fuel consumption, and Figure 9 presents the cumulative fuel consumption for all runs. In general the simulation run and the on-road measurements show a convergence in fuel consumption with slight oscillations that do not reveal a general trend. The difference between the overall fuel consumption was found to be between −1.6 and −0.6% for the AB direction and between 1.2 and 3.2% for the BA direction. The difference between the directions could be attributed to the elevation difference between A and B. As point A lies at a lower altitude than B, the VECTO driver model could have a more fuel-efficient driving style. Also, the simulation showed average specific CO2 emissions 716.12 g/kWh, which is in line with the values reported in another study (Grigoratos et al., 2019).
Figure 10 presents a comparison of the standard and bus auxiliaries to the on-road values for each route. The AB direction shows lower divergence for the bus auxiliaries run compared to the standard auxiliaries run. However, the BA direction showed a higher divergence for the bus auxiliaries run than the standard auxiliaries run. This could be attributed to the use of the VECTO generic auxiliary maps, as the BA is a less energy demanding route. In high energy demanding routes, the auxiliaries would work closer to their maximum power requirements (e.g., use of fan in uphill driving). However, on less energy demanding routes, the power demand could fluctuate more resulting in the need for more accurate efficiency maps.
In the next step, the analysis proceeded by performing an energy audit and compared the results of the bus and standard auxiliaries for each direction. Figure 11 compares the various loss types as shares of the total engine output. The figure confirms the results that AB direction is a more energy demanding direction as a share between 6.5 and 10% is attributed to the grade. This is reversed in the BA direction, where the respective range reaches negative values.
The energy audit confirms that the highest amount of energy is attributed to braking and highlights the significance of a representative driver model. The gearbox losses that have been calculated experimentally and could pose a level of uncertainty present a low share of the total energy losses, which is inline with other studies that calculated relative losses in the order of 2% (Zacharof et al., 2019). According to a study (Tansini et al., 2019) the highest amount of energy in HDVs is dissipated to the rolling resistance, air drag and braking. As the investigated route was mainly urban with regular stops and relatively low speed, the air drag had the lowest impact out of the three parameters, but the combined energy consumption of all three was in the in the range of 55–74%. This was loosely in line with the reported values for HDVs where the combined share of these three parameters was about 80% (Tansini et al., 2019). The difference is attributed to the auxiliary use, which is more complicated in buses than in trucks. Auxiliary use is almost the same for both runs and this is attributed to the fact that the vehicle operated under almost a constant auxiliary load. However, future research should take into consideration a more thorough investigation on the auxiliary use during the measurement phase and then proceed to a more detailed simulation approach.
Conclusions
So far the European policy regarding the CO2 emissions from HDVs has focused mainly on rigid and tractor-trailer trucks. Within this context, the development of the respective regulation has been supported by a series of studies for these HDV classes. The next step would be to focus on city buses and coaches. This investigation has been a first attempt to develop an approach for creating city buses vehicle models for VECTO simulation. The findings confirmed that the approach can achieve a high degree of accuracy, but additional work is required to further improve the results and decrease the level of uncertainty. It was recommended that future research should focus on fields that could have an significant impact on fuel consumption for city buses such as the gearshifting pattern and better implementation of the bus auxiliaries. As it was demonstrated in the energy audit, the use of auxiliaries has high energy consumption and it is a significant factor in buses and coaches. The VECTO development had foreseen this and it addressed the issue by implementing the bus auxiliaries module. The investigation showed that further research is needed for properly implementing auxiliary use. Future research could focus on individual auxiliary components and assess methods for producing the required VECTO input data.
Data Availability Statement
The datasets generated for this study are available on request to the corresponding author.
Author Contributions
OÖ and GF: conceptualization. OÖ, MÖ, GF, and NZ: methodology. NZ, MÖ, and AK: simulation runs. NZ, OÖ, MÖ, and AK: formal analysis. NZ and OÖ: data curation and writing—original draft preparation. NZ and GF: writing—revisions and final editing. OÖ and MÖ: supervision experimental tests. GF: supervision simulations and results analysis.
Funding
The experimental part of this research was supported by the Istanbul Development Agency—ISTKA, under Information Focused Economic Development Program, project No. BIL-86 with the partnership of Istanbul Public Transportation Company (IETT). The publication fees were covered by the European Commission Joint Research Centre.
Conflict of Interest
NZ was employed by Seidor Consulting S.L.
The remaining authors declare that the research was conducted in the absence of any commercial or financial relationships that could be construed as a potential conflict of interest.
The reviewer FR declared a past co-authorship with one of the authors, NZ, to the handling editor.
References
Andersson, C. (2004). On auxiliary systems in commercial vehicles (Ph.D thesis). Lund University, Lund, Sweden. Available online at: https://www.iea.lth.se/publications/Theses/LTH-IEA-1039.pdf
AVL (2019). AVL concerto M.O.V.E - AVL concerto M.O.V.E - development. AVL. Available online at: https://www.avl.com (accessed July 26, 2019).
AVL List GmbH (2010a). AVL GAS PEMS Portable Emissions Measurement System Product Guide AT4291E, Rev.04. Graz: AVL List GmbH.
AVL List GmbH (2010b). AVL PEMS Post Processing Software v4.3 User's Guide AT3298E, Rev.00. Graz: AVL List GmbH.
AVL List GmbH (2013). AVL KMA MOBILE Fuel Consumption Measuring System AT2262E, Rev.03. Graz: AVL List GmbH.
Boulter, P., and McCrae, I. (2007). ARTEMIS: Assessment and Reliability of Transport Emission Models and Inventory Systems. Final Report, TRL Limited.
Clark, N., Khan, A. S., Wayne, W. S., Gautam, M., Thompson, G. J., McKain, D. L., et al. (2007). Weight effect on emissions and fuel consumption from diesel and lean-burn natural gas transit buses, in SAE 2007-01-3626, SAE Asia Pacific Automotive Engineering Conference (Hollywood, CA).
European Commission (2016). Communication From the Commission to the European Parliament, the Council, the European Economic and Social Committee and the Committee of the Regions: A European Strategy for Low-Emission Mobility. Available online at: https://eur-lex.europa.eu/legal-content/en/TXT/?uri=CELEX%3A52016DC0501 (accessed September 26, 2016).
Fontaras, G., Rexeis, M., Hausberger, S., Kies, A., Hammer, J., Schulte, L.-E., et al. (2014). Development of a CO2 Certification and Monitoring Methodology for Heavy Duty Vehicles: Proof of Concept Report. Publications Office. Available online at: http://dx.publications.europa.eu/10.2790/12582 (accessed November 28, 2016).
Grigoratos, T., Fontaras, G., Giechaskiel, B., and Zacharof, N. (2019). Real world emissions performance of heavy-duty Euro VI diesel vehicles. Atmos. Environ. 201, 348–359. doi: 10.1016/j.atmosenv.2018.12.042
Holmberg, K., Andersson, P., and Erdemir, A. (2012). Global energy consumption due to friction in passenger cars. Tribol. Int. 47, 221–234. doi: 10.1016/j.triboint.2011.11.022
KB AutoTech (2019). KB Bus Air-Conditioner. Available online at: http://www.hcvm.com.my/content/aircond_index-spec.pdf (accessed September 12, 2019).
Khan, A. S., and Clark, N. (2010). An empirical approach in determining the effect of road grade on fuel consumption from transit buses. SAE Int. J. Commer. Veh. 3, 164–180. doi: 10.4271/2010-01-1950
Muncrief, R., Cruz, M., Ng, H., and Harold, M. (2012). Impact of Auxiliary Loads on Fuel Economy and Emissions in Transit Bus Applications. SAE Technical Paper, SAE 2012 World Congress & Exhibition, University of Houston. doi: 10.4271/2012-01-1028
Muncrief, R., and Sharpe, B. (2015). Overview of the Heavy-Duty Vehicle Market and CO2 Emissions in the European Union. Available online at: http://www.theicct.org/overview-heavy-duty-vehicle-market-and-co2-emissions-european-union (accessed December 4, 2015).
Norris, J., Hill, N., Kirsch, F., Nurse, D., Revereault, P., and Preston, M. (2016). Quantifying energy consumption of HDV auxiliary components and their contribution to CO2emissions of buses and coaches. Heavy Duty Vehicles Framework Contract-Service Request 3. Final Report for DG Climate Action Ref: CLIMA.C.2/FRA/2013/0007.
Özener, O. (2017). Real driving emissions and fuel consumption characteristics of Istanbul public transportation. Therm. Sci. 21, 665–667. doi: 10.2298/TSCI161112308O
Özener, O., and Özkan, M. (2018). Assessment of real driving emissions of a bus operating on a dedicated route. Therm. Sci. 349. doi: 10.2298/TSCI180921349O
Özener, O., Özkan, M., Orak, E., and Acarbulut, G. (2018). A fuel consumption model for public transportation with 3-D road geometry approach. Therm. Sci. 22, 1505–1514. doi: 10.2298/TSCI180421158O
Pettersson, N., and Johansson, K. H. (2006). Modelling and control of auxiliary loads in heavy vehicles. Int. J. Control 79, 479–495. doi: 10.1080/00207170600587333
Regulation (EU) 2017/2400 (2018). Regulation (EU) 2017/2400 of 12 December 2017 Implementing Regulation (EC) No 595/2009 of the European Parliament and of the Council as Regards the Determination of the CO2 Emissions and Fuel Consumption of Heavy-Duty Vehicles and Amending Directive 2007/46/EC of the European Parliament and of the Council and Commission Regulation (EU) No 582/2011. Available online at: https://eur-lex.europa.eu/eli/reg/2017/2400/oj (accessed December 19, 2018).
Regulation (EU) 2018/956 (2018). Regulation (EU) 2018/956 of the European Parliament and of the Council of 28 June 2018 on the Monitoring and Reporting of CO2 Emissions From and Fuel Consumption of New Heavy-Duty Vehicles (Text With EEA Relevance). Available online at: http://data.europa.eu/eli/reg/2018/956/oj/eng (accessed October 22, 2018).
Savvidis, D. (2015). Heavy Duty Vehicles' CO2 Legislation in Europe and VECTO Simulation Tool. Available online at: http://climate.blue/download/presentations_heavy_duty_vehicles/Savvidis_European%20Commission.pdf (accessed September 12, 2019).,
Tansini, A., Zacharof, N., Prado Rujas, I., and Fontaras, G. (2018). Analysis of VECTO data for Heavy-Duty Vehicles (HDV). Publications Office of the European Union. Available online at: http://publications.jrc.ec.europa.eu/repository/handle/JRC112015
Tansini, A., Zacharof, N., Prado Rujas, I., Fontaras, G., Ciuffo, B., and Millo, F. (2019). A method for calculating heavy-duty trucks energy and fuel consumption with the use of correlation formulas derived from VECTO simulations. SAE Technical Paper, 019-01-1278. Available online at: https://www.sae.org/publications/technical-papers/content/2019-01-1278/
TNO TUG, CE Delft, ICCT. (2018). Support for Preparation of the Impact Assessment for CO2 Emissions Standards for Heavy Duty Vehicles. Available online at: https://ec.europa.eu/clima/sites/clima/files/transport/vehicles/heavy/docs/support_impact_assessment_hdv_en.pdf (accessed February 27, 2019).
Vepsäläinen, J., Otto, K., Lajunen, A., and Tammi, K. (2019). Computationally efficient model for energy demand prediction of electric city bus in varying operating conditions. Energy 169, 433–443. doi: 10.1016/j.energy.2018.12.064
Wang, J., and Rakha, H. A. (2016). Fuel consumption model for conventional diesel buses. Appl. Energy 170, 394–402. doi: 10.1016/j.apenergy.2016.02.124
Wang, J., and Rakha, H. A. (2017). Fuel consumption model for heavy duty diesel trucks: model development and testing. Transp. Res. Part Transp. Environ. 55, 127–141. doi: 10.1016/j.trd.2017.06.011
White, B., Kirsch, F., Levin, S., and Skinner, I. (2017). Analysis of Fuel Economy and GHG Emission Reduction Measures From Heavy Duty Vehicles in Other Countries and of Options for the EU. Ricardo Energy & Environment. Available online at: https://ec.europa.eu/clima/sites/clima/files/transport/vehicles/heavy/docs/analysis_fuel_economy_hdv_en.pdf
Yang, C. X., Bibeau, E., and Molinski, T. (2012). “Fuel consumption model for diesel and electric buses considering bus route and passenger load variation,” in EV 2012 (Montreal, QC).
Keywords: city-bus, heavy duty vehicle, on-road CO2, VECTO, auxiliaries
Citation: Zacharof N, Özener O, Özkan M, Kilicaslan A and Fontaras G (2019) Simulating City-Bus On-Road Operation With VECTO. Front. Mech. Eng. 5:58. doi: 10.3389/fmech.2019.00058
Received: 23 May 2019; Accepted: 05 September 2019;
Published: 24 September 2019.
Edited by:
Evangelos G. Giakoumis, National Technical University of Athens, GreeceReviewed by:
Felipe Rodriguez, International Council on Clean Transportation, GermanyXu Ning, Tongji University, China
Copyright © 2019 Zacharof, Özener, Özkan, Kilicaslan and Fontaras. This is an open-access article distributed under the terms of the Creative Commons Attribution License (CC BY). The use, distribution or reproduction in other forums is permitted, provided the original author(s) and the copyright owner(s) are credited and that the original publication in this journal is cited, in accordance with accepted academic practice. No use, distribution or reproduction is permitted which does not comply with these terms.
*Correspondence: Orkun Özener, b296ZW5lckB5aWxkaXouZWR1LnRy; Georgios Fontaras, Z2Vvcmdpb3MuZm9udGFyYXNAZWMuZXVyb3BhLmV1