- 1Department of Research and Development, Department of Mental Health, Norwegian University of Science and Technology, Trondheim, Norway
- 2Department of Østmarka, Division of Mental Health Care, St. Olavs Hospital, Trondheim University Hospital, Trondheim, Norway
- 3Norwegian Centre for Mental Disorders Research, KG Jebsen Centre for Psychosis Research, Institute of Clinical Medicine, University of Oslo, Oslo, Norway
- 4Norwegian Centre for Mental Disorders Research, Division of Mental Health and Addiction, Oslo University Hospital, Oslo, Norway
- 5Department of Brøset, Division of Mental Health Care, St. Olavs Hospital, Trondheim University Hospital, Trondheim, Norway
- 6Department of Medical Genetics, Oslo University Hospital, Oslo, Norway
- 7Norwegian Centre for Mental Disorders Research, KG Jebsen Centre for Psychosis Research, Department of Clinical Science, University of Bergen, Bergen, Norway
- 8Center for Multimodal Imaging and Genetics, Department of Radiology, University of California, San Diego, La Jolla, CA, United States
- 9Department of Neurosciences, University of California, San Diego, La Jolla, CA, United States
- 10Department of Psychiatry, University of California, San Diego, La Jolla, CA, United States
Background: Alzheimer’s disease (AD) and bipolar disorder (BIP) are complex traits influenced by numerous common genetic variants, most of which remain to be detected. Clinical and epidemiological evidence suggest that AD and BIP are related. However, it is not established if this relation is of genetic origin. Here, we applied statistical methods based on the conditional false discovery rate (FDR) framework to detect genetic overlap between AD and BIP and utilized this overlap to increase the power to identify common genetic variants associated with either or both traits.
Methods: We obtained genome wide association studies data from the International Genomics of Alzheimer’s Project part 1 (17,008 AD cases and 37,154 controls) and the Psychiatric Genetic Consortium Bipolar Disorder Working Group (20,352 BIP cases and 31,358 controls). We used conditional QQ-plots to assess overlap in common genetic variants between AD and BIP. We exploited the genetic overlap to re-rank test-statistics for AD and BIP and improve detection of genetic variants using the conditional FDR framework.
Results: Conditional QQ-plots demonstrated a polygenic overlap between AD and BIP. Using conditional FDR, we identified one novel genomic locus associated with AD, and nine novel loci associated with BIP. Further, we identified two novel loci jointly associated with AD and BIP implicating the MARK2 gene (lead SNP rs10792421, conjunctional FDR = 0.030, same direction of effect) and the VAC14 gene (lead SNP rs11649476, conjunctional FDR = 0.022, opposite direction of effect).
Conclusion: We found polygenic overlap between AD and BIP and identified novel loci for each trait and two jointly associated loci. Further studies should examine if the shared loci implicating the MARK2 and VAC14 genes could explain parts of the shared and distinct features of AD and BIP.
Introduction
About a century ago, Alois Alzheimer and Emil Kraepelin described the historical equivalents of AD and BIP (Alzheimer, 1907; Kraepelin, 1921). Still their etiologies are incompletely understood and no curative treatments exist (Grande et al., 2016; Scheltens et al., 2016). The Global Burden of Disease study ranks AD and BIP among the top thirty causes of years lived with disability worldwide (Vos et al., 2016).
Alzheimer’s disease is a neurodegenerative disorder (Jack et al., 2013) usually presenting in late adult life (Koedam et al., 2010), while BIP is considered a neurodevelopmental disorder (Sanches et al., 2008; O’Shea and McInnis, 2016) with average age at onset in early adult life (Baldessarini et al., 2010). Yet, epidemiological, pathophysiological, and clinical data suggest that AD and BIP could be related. A recent meta-analysis reports an odds ratio of 2.4 (95% CI 1.4–4.1) for dementia of all causes among patients with BIP (Diniz et al., 2017). The risk of dementia is higher among patients with BIP compared to patients with arthritis, diabetes, and schizophrenia (Kessing et al., 1999; Kessing and Nilsson, 2003). Among patients with BIP, treatment with lithium is associated with a reduced risk of dementia (Kessing et al., 2010; Gerhard et al., 2015) and AD (Nunes et al., 2007) in most, but not all (Cheng et al., 2017), observational studies. Among patients with AD or mild cognitive impairment, a meta-analysis of randomized controlled studies found that lithium decreased cognitive decline (Matsunaga et al., 2015). Shared pathophysiological processes between AD and BIP are reported in the kynurenine pathway (Miller et al., 2006; Myint et al., 2007; Rahman et al., 2009; Gulaj et al., 2010; Maddison and Giorgini, 2015; Savitz et al., 2015). There is also evidence of inflammatory processes in both conditions (Goldstein et al., 2009; Antonio et al., 2015; Heneka et al., 2015). Further, euthymic patients with BIP have impairments of episodic memory (Torres et al., 2007) and executive dysfunction (Torres et al., 2007; Martino et al., 2015), which are also core symptoms of AD (Gold and Budson, 2008; Godefroy et al., 2016).
Despite several lines of evidence suggesting a relation between AD and BIP, it is not established if the conditions have a shared genetic basis. AD and BIP are in most cases complex traits, i.e., they are influenced by several genetic and environmental factors. Twin studies estimate the heritability of AD and BIP to 60% or higher (McGuffin et al., 2003; Kieseppä and Partonen, 2004; Gatz et al., 2006; Lichtenstein et al., 2009). Genome wide association studies (GWASs) are the gold standard for hypothesis-free assessment of associations between complex traits and common genetic variants (Corvin et al., 2010). The common variants refer to single nucleotide polymorphisms (SNPs) with minor allele frequencies > 1–5%. The power of a GWAS is a function of study sample size and the genetic architecture of the trait (i.e., the narrow-sense heritability, the number of causal variants, their effect sizes, and population frequencies) (Schork et al., 2016; Frei et al., 2018). AD and BIP are considered highly polygenic (Purcell et al., 2009; Escott-Price et al., 2015), and ∼1/3 of their heritability can be explained by SNPs with tiny effect sizes that are not individually detectable given the power of current GWASs (Lee et al., 2011, 2013; Ridge et al., 2013, 2016).
With the current sample sizes, however, the power of GWASs can be boosted by leveraging polygenic overlap between complex traits (Andreassen et al., 2013a,b, 2015). Shared genetic influences are common among complex traits (Visscher et al., 2017). Statistical methods based on the conditional FDR framework can detect polygenic overlap between complex traits and utilize this polygenic overlap to increase the power to identify common genetic variants associated with each trait and jointly with two or more traits (Andreassen et al., 2013a,b, 2015). We aimed to use these methods to identify the shared genetic basis between AD and BIP.
Materials and Methods
Data Sources
We obtained summary statistics (i.e., effect sizes and corresponding p-values for all SNPs) from the IGAP (Lambert et al., 2013) and the PGC2-BIP (Stahl et al., 2019).
International Genomics of Alzheimer’s Project
The IGAP is a two-stage study. We used data from stage 1 of the study, which is based upon four previously published GWASs [The European Alzheimer’s Disease Initiative (Dreses-Werringloer et al., 2008; Heath et al., 2008), the Alzheimer Disease Genetics Consortium (Jun et al., 2010), The Cohorts for Heart and Aging Research in Genomic Epidemiology consortium (Psaty et al., 2009), The Genetic and Environmental Risk in AD consortium (Harold et al., 2009)] on 17,008 AD cases and 37,154 controls of European ancestry. The IMPUTE2 (Howie et al., 2009) or MaCH/Minimac (Li et al., 2010) software were used to impute SNPs from the European ancestry haplotypes in the 1000 Genome Project (Altshuler et al., 2010). In stage 2 of the study, SNPs with p-values < 1 × 10-3 from stage 1 were selected for genotyping in independent samples. We did not use data from stage 2 of the study since the conditional FDR method require genome-wide summary statistics which are not inflated.
Diagnoses of AD in the sub-studies of IGAP were in most cases made clinically according to the National Institute of Neurological and Communicative Disorders and Stroke and the Alzheimer’s disease and Related Disorders Association criteria (McKhann et al., 1984) or the Diagnostic and Statistical Manual of Mental Disorders (American Psychiatric Association, 1994) criteria, or post mortem according to the National Institute of Ageing-Regan criteria (Newell et al., 1999).
Informed consents were provided from all participants, or, in the case of substantial cognitive impairment, from caregivers, legal guardians, or other proxies. The sub-studies were approved by local ethic committees.
For further details, we refer to the original publication (Lambert et al., 2013).
Psychiatric Genetic Consortium 2 Bipolar Disorder Working Group
The PGC2-BIP is a GWAS based upon 32 sub-studies on 20,352 BIP cases and 31,358 controls of European ancestry. Arrays for genotyping were chosen by each sub-study. The Ricopoli pipeline1 was used to standardize quality control, imputation, and analyses of genotypic data from all samples except one. SNPs were excluded by the following criteria: missingness in > 5 (before sample removal) or 2% (after sample removal), p-value for Hardy–Weinberg equilibrium < 1 × 10-10 in cases or <1 × 10-6 in controls, missingness difference between cases and controls > 2%, or autosomal heterozygosity deviation (|Fhet| > 0.2). Individuals with > 2% missing genotypes were also excluded. The IMPUTE2 (Howie et al., 2009) and SHAPEIT2 (Delaneau et al., 2012) software were used for imputation.
Diagnoses of BIP were established by clinical interviews or obtained from hospital record data according to the Diagnostic and Statistical Manual of Mental Disorders 4th edition (American Psychiatric Association, 1994), the International Classification of Diseases 9th revision (World Health Organization, 1977), or the International Classification of Diseases 10th revision (World Health Organization, 1992).
Informed consents were provided from all participants. The sub-studies were approved by local ethical committees.
For further details, we refer to the original publication (Stahl et al., 2019).
Data Availability
Data from the IGAP2 and PGC2-BIP3 studies are publicly available for download.
Statistical Analyses
Conditional Quantile–Quantile (QQ)-Plots
We used conditional QQ-plots to visually assess pleiotropic enrichment. A conditional QQ-plot displays the distribution of p-values for the first trait, e.g., AD, conditioned on association levels for the second trait, e.g., BIP. Pleiotropic enrichment is present if the degree of leftward shift from the expected null line for the first trait is dependent on the degree of association with the second trait. For further details, we refer to previous studies (Andreassen et al., 2013a,b, 2015) and Supplementary Methods 1.1.
Conditional False Discovery Rate (condFDR)
The enrichment observed in conditional QQ-plots can be translated to FDR for each SNP. We used the conditional false discovery rate (condFDR) to improve power to detect SNPs associated with AD given associations with BIP and vice versa. condFDR is defined as “the posterior probability that a given SNP is null for the first trait given that the p-values for both traits are as small or smaller than the observed p-values” (Andreassen et al., 2015). We denoted condFDR for AD given associations with BIP as condFDR(ADjBIP) and for BIP given association with AD as condFDR(BIPj AD) and considered values < 0.01 significant. For further details, we refer to previous studies (Andreassen et al., 2013a,b, 2015) and Supplementary Methods 1.2.
Conjunctional False Discovery Rate (conjFDR)
We used conjunctional FDR (conjFDR) to identify SNPs jointly associated with AD and BIP. conjFDR is defined as “the posterior probability that a SNP is null for either phenotype or both simultaneously, given the p-values for both traits are as small or smaller than the observed p-values” (Andreassen et al., 2015). After repeating the condFDR procedure for both traits, we identified shared loci at conjFDR < 0.05, which is given by the maximum between the condFDRs for both traits. Hence, the conjFDR analysis is a conservative approach requiring that loci exceed a condFDR significance threshold for two traits simultaneously. For further details, we refer to previous studies (Andreassen et al., 2013a,b, 2015) and Supplementary Methods 1.3.
Conditional and Conjunctional Manhattan Plots
We constructed conditional Manhattan plots to visualize the chromosomal location of SNPs with condFDR(ADjBIP) (Supplementary Figure 1) and condFDR(BIPjAD) < 0.01 (Supplementary Figure 2). We constructed a similar plot for SNPs jointly associated with AD and BIP at a conjFDR < 0.05 (Figure 2).
Assessment of Novelty
To determine if a locus was novel, we first checked that the p-value(s) for the implicating variant was > 5 × 10-8 in the original GWAS(s). Further, we used LDlink (Machiela and Chanock, 2015) to exclude variants which are in LD (r2> 0.1) with any of the genome-wide significant hits in the original GWAS(s). Finally, we conducted a search on PubMed using the term (“SNP id” OR “gene name”) AND (“Bipolar Disorder”[Mesh] OR “Alzheimer Disease”[Mesh]) to exclude that the variants or implicated genes have been associated with AD or BIP at genome-wide significance in previous GWASs.
Cerebral Gene Expression Across Lifespan of the Implicated Loci
The Human Brain Transcriptome (HBT) project4 used postmortem brain tissue from over 1,340 samples to provide genome-wide exon-level transcriptome data in 16 cerebral regions (Kang et al., 2011). We obtained figures from the HBT project on gene expression in different cerebral areas as a function of age (i.e., from embryonic life through late adulthood) for the nearest genes to the loci jointly associated with AD and BIP.
Control of Spurious Enrichment
We randomly chose one SNP in each LD block (r2> 0.1), and calculated the average empirical cumulative distribution function (ecdf) by using the p-values obtained through 200 iterations. SNPs within the major histocompatibility complex region (defined as chr6:25652429–33368333) and the apolipoprotein E (APOE) gene (chr19:44909039–45912650), and SNPs in LD (r2> 0.1) with these SNPs, were excluded from the analyses due to their complex LD structure (de Bakker and Raychaudhuri, 2012) and known association to AD (Lambert et al., 2010; Scheltens et al., 2016), which could bias the estimates of enrichment. Further, we used LD-independent (r2< 0.1) intergenic SNPs, which are depleted of true associations, to calculate an inflation factor value (Wang et al., 2016a). We divided all test statistics on this value to control for genomic inflation.
Cross-Trait Linkage Disequilibrium Score Regression (LDSR)
We calculated the degree of genetic correlation between AD and BIP using cross-trait LD score regression (LDSR) (Bulik-Sullivan et al., 2015). For details, we refer to Supplementary Materials 1.4.
Ethics Statement
All GWASs performed and investigated in the present study were approved by the local ethics committees, and informed consent was obtained from all participants. Furthermore, the Norwegian Institutional Review Board for the South-East Norway Region has evaluated the methods used in the current study and found that no additional institutional review board approval was needed because no individual data were used (ref. 2011/1980).
Results
Pleiotropic Enrichment
In the conditional QQ-plots, we observed enrichment of associations with AD given increasing SNP associations with BIP, and vice versa (Figure 1). These findings indicate polygenic overlap between AD and BIP across common genetic variants.
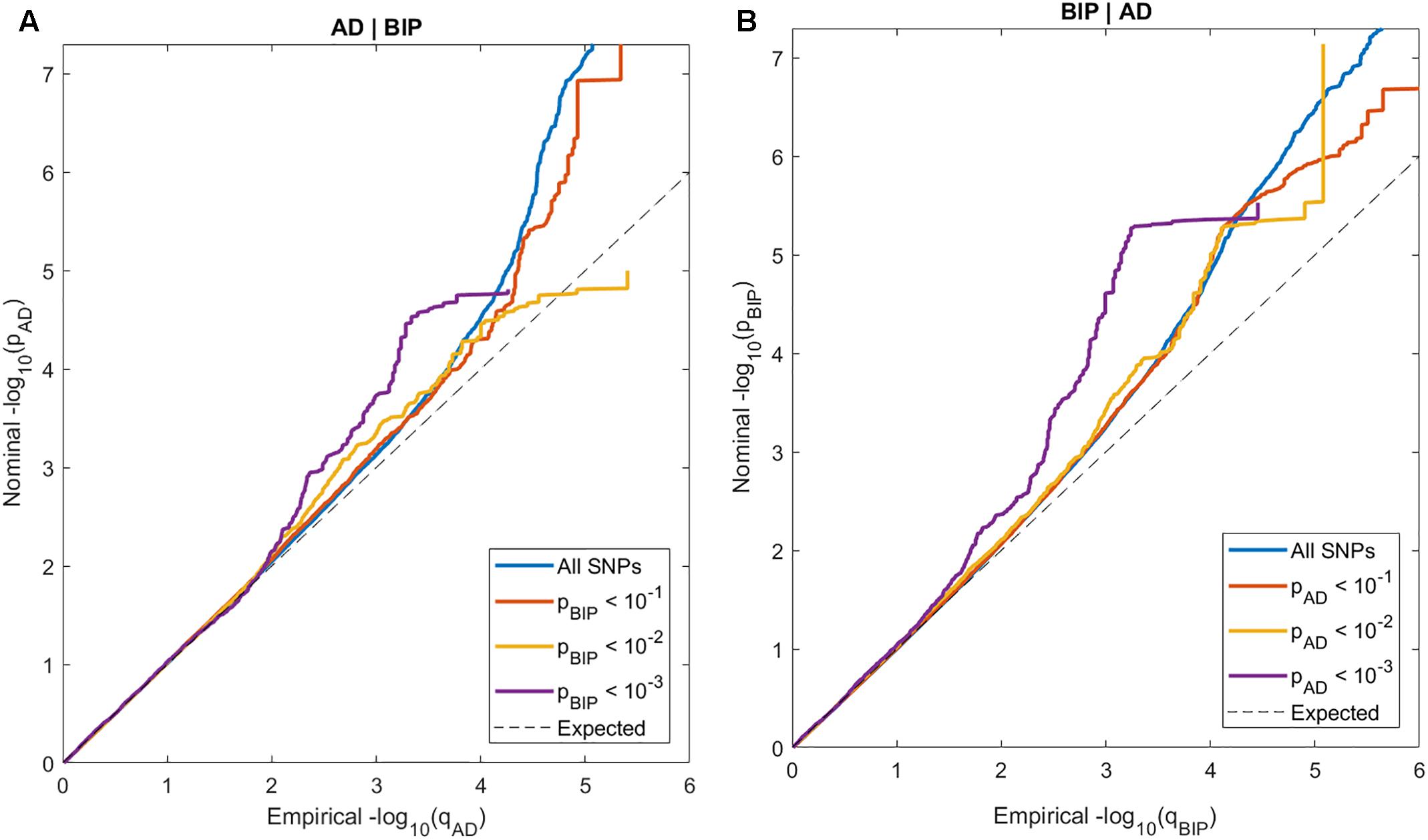
Figure 1. Conditional QQ-plots of nominal p-values at y-axis and 1 - empirical cumulative distribution function on x-axis for (A) Alzheimer’s disease (AD) with lines representing strata of SNPs according to their degree of association with bipolar disorder (BIP) and (B) BIP with lines representing strata of SNPs according to their degree of association with AD.
Improving Genetic Discovery Using Conditional FDR
We then leveraged the pleiotropic enrichment observed in conditional QQ-plots to boost SNP discovery in both traits using condFDR.
We identified 22 SNPs clumped into 19 independent loci at condFDR(ADjBIP) < 0.01 (Supplementary Table 1). The chromosomal locations of the nearest genes are visualized in a conditional Manhattan plot (Supplementary Figure 1). Red annotations represent the four loci with a lower conditional than unconditional FDR. Of these four loci, two loci have uncorrected p-values > 5 × 10-8 in the original GWAS and are thus not identified by traditional methods; NDUFS3 (rs71475924, intron variant) and MTSS1L (rs12597717, intron variant). The signal in NDUFS3 was driven by one single SNP and is thus probably a spurious association.
Further, we identified 24 SNPs within 24 loci at a condFDR(BIPjAD) < 0.01 (Supplementary Table 2). As visualized in the conditional Manhatton plot (Supplementary Figure 2), 17 loci had a lower conditional than unconditional FDR. Of these 17 loci, 10 variants have uncorrected p-values > 5 × 10-8 in the original GWAS and are thus not identified by traditional methods; LOC105378763 (rs1889778, intron variant), CNTNAP5 (rs13011184, intron variant), KIAA1109 (rs45605540, intron variant), SSBP2 (rs7707981, intron variant), AK091365 (rs2388334, no genic locational annotation), RCOR2 (rs4980532, intron variant), STARD9 (rs4447398, intron variant), GRIN2A (rs11647445, intron variant), THRA (rs61554907, intron variant), and PRKCA (rs7406066, intron variant). However; the CNTNAP5 gene has previously been associated with the posterior cortical atrophy variant of AD at genome-wide significance (Schott et al., 2016) and with BIP (Djurovic et al., 2010).
Identification of Shared Loci
Finally, we applied conjFDR to assess for SNPs jointly associated with AD and BIP. We used effect sizes from the original data sources to determine the allelic direction of effects in both traits.
We identified two SNPs at two loci at a conjFDR(AD&BIP) < 0.05 (Table 1 and Figure 2). A 2 kb upstream variant at MARK2 (rs10792421) was associated with AD and BIP with the same direction of effect on AD and BIP [conjFDR(AD&BIP) = 0.030, z-score(AD) = 3.99, z-score(BIP) = 4.74]. MARK2 is widely expressed in the developing and adult human brain (Supplementary Figure 3). An intronic variant within VAC14 (rs11649476) was associated with AD and BIP with opposite directions of effect in AD and BIP [conjFDR(AD&BIP) = 0.022, z-score(AD) = -4.35, z-score(BIP) = 4.18]. VAC14 is also widely expressed in the developing and adult human brain (Supplementary Figure 4). Both SNPs have p-values > 5 × 10-8 for both traits in the original GWASs and are thus not identified by traditional methods.

Table 1. SNPs with related genes jointly associated with Alzheimer’s disease (AD) and bipolar disorder (BIP) at a conjunctional false discovery rate (conjFDR(AD&BIP)) < 0.05.
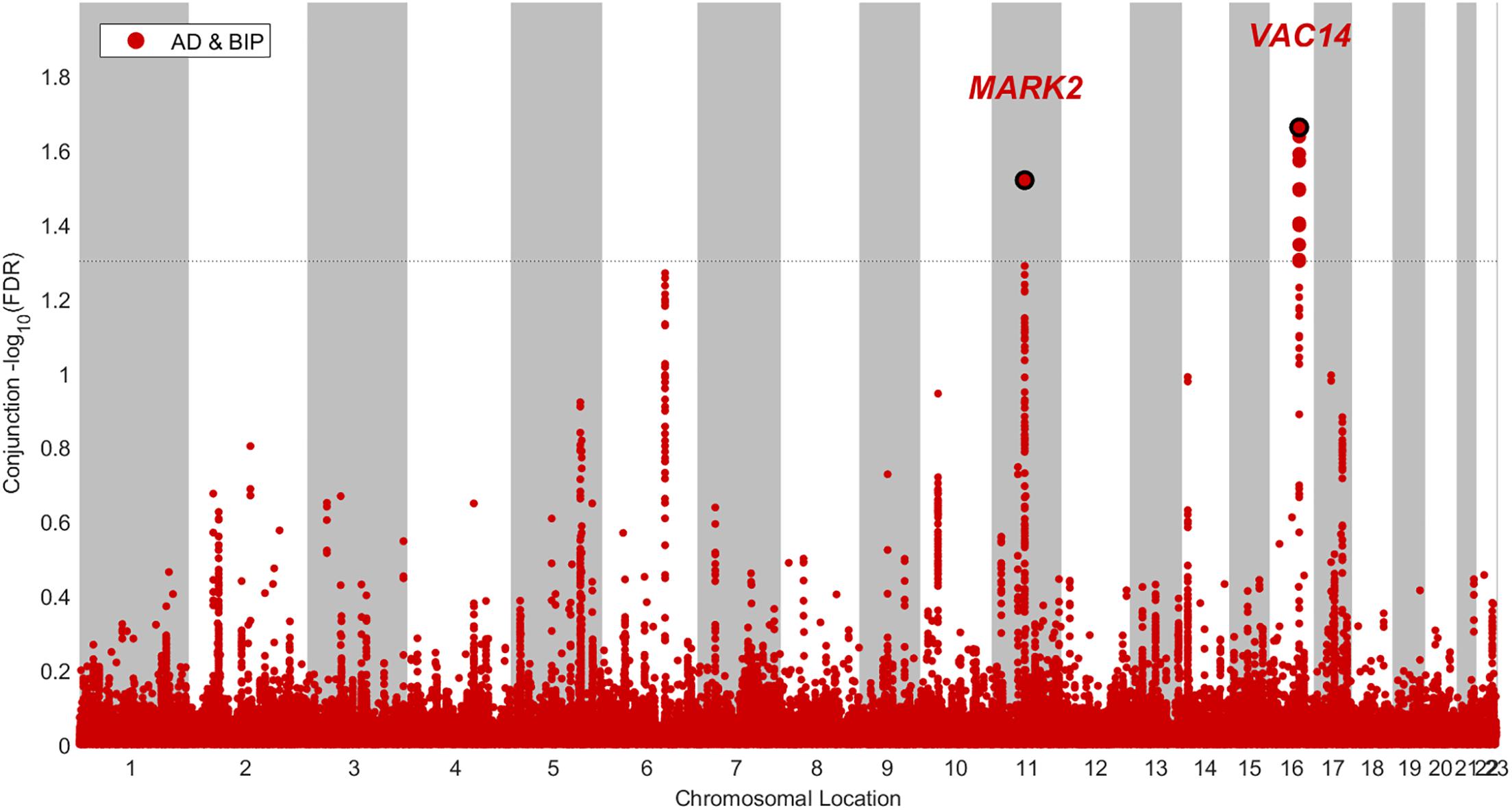
Figure 2. Conjunctional Manhatton plot of loci jointly associated with Alzheimer’s disease (AD) and bipolar disorder (BIP) at a conjuntional false discovery rate < 0.05.
Genetic Correlation
We estimated that there is no overall genetic correlation between AD and BIP according to LDSR (rg = -0.0222, SE = 0.0519, p = 0.669).
Discussion
We used statistical methods based on the condFDR framework and showed that AD and BIP have a shared genetic basis. Our study adds new insights into the relation between AD and BIP by finding polygenic overlap, one novel locus associated with AD and nine novel loci associated with BIP when conditioned on associations with the other trait, and two novel loci jointly associated with both traits.
A polygenic overlap between AD and BIP could implicate shared genetic influences as a part of the explanation to the epidemiological (Diniz et al., 2017), pathophysiological (Goldstein et al., 2009; Heneka et al., 2015), and clinical (Gold and Budson, 2008; Martino et al., 2015; Godefroy et al., 2016) links between the diseases. However, we do not find an overall genetic correlation as assessed with cross-trait LDSR (Bulik-Sullivan et al., 2015). Also, one of the two jointly associated SNPs demonstrates effects in opposite directions. These findings are compatible with a scenario where the polygenic overlap between AD and BIP involves a mixed direction of effects of the implicated SNPs yielding no genome-wide correlation (Frei et al., 2018). Thus, absence of an overall genetic correlation between brain disorders, as evident for several traits (including AD and BIP) in the study of Anttila et al. (2018), does not imply lack of genetic overlap.
The loci implicating the MARK2 and VAC14 genes were jointly associated with AD and BIP (Table 1). Both genes are widely expressed in the human brain throughout life (Supplementary Figures 3, 4), which implies a spatial and temporal relation to both neurodevelopmental and neurodegenerative processes. The locus implicating the MARK2 gene (rs10792421) had a concordant direction of effect in both traits (Table 1). The MARK2 gene encodes the microtubule affinity regulating kinase 2 (MARK2). The kinase is involved in a diversity of neuronal cellular processes, including neuronal migration, and tau phosphorylation (Matenia and Mandelkow, 2009). Migration of immature neurons is necessary for corticogenesis (Kon et al., 2017). BIP is considered a neurodevelopmental disorder partly because of previous findings of cortical cell migration abnormalities (Sanches et al., 2008; O’Shea and McInnis, 2016). Abnormal neuronal migration might also be involved in later stages of life among patients with AD (Reiner et al., 2009). Tauopathy is one of the pathophysiological hallmarks of AD (Jack et al., 2013). Gu G.J. et al. (2013) demonstrated that MARK2 increases the phosphorylation of tau in situ and found interactions between MARK2 and tau in postmortem human AD brain tissue. The role of tauopathy has also been explored in BIP. A study of cerebrospinal fluid among younger patients with BIP (Jakobsson et al., 2013) and a similar study of elderly patients with BIP and mild cognitive impairment (Forlenza et al., 2016) did not find any evidence of tauopathy. However, in another study, the total to phosphorylated tau ratio was reduced among patients with BIP carrying the risk allele of a common variant related to the previously discovered BIP risk gene CACNA1C (Jakobsson et al., 2016). A similar reduction was not found among healthy controls carrying the same risk allele. These findings suggest an alteration in the regulation of tau phosphorylation in carriers of the risk allele that is restricted to patients with BIP. Further studies should explore whether interactions with other genes involved in regulation of tau phosphorylation, like the MARK2 gene, could explain the specificity of the finding to patients with BIP. Lithium has several molecular targets including inhibition of glycogen synthase kinase 3β (Freland and Beaulieu, 2012). Evidence is conflicting on whether glycogen synthase kinase 3β in turn inhibits or activates MARK2 (Kosuga et al., 2005; Timm et al., 2008). Consequently, it is unknown whether treatment with lithium could result in reduced or increased phosphorylation of tau among carriers of the common variant related to the MARK2 gene.
The intronic variant within VAC14 (rs11649476) was related to AD and BIP with opposite directions of effects. The same variant was shared between BIP and intelligence with concordant direction of effects in a recent study using conjunctional FDR (Smeland et al., 2019). VAC14 encodes a part of the PIKfyve protein kinase complex, which phosphorylates phosphatidylinositol 3-phosphate [PI(3)P] to phosphatidylinositol 3,5-bisphosphate [PI(3,5)P2] (McCartney et al., 2014). PI(3,5)P2 is involved in endosomal homeostasis (Di Paolo and De Camilli, 2006). A null mutation of VAC14 in a mouse model resulted in perinatal death and massive neurodegeneration with vacuolated neurons (Zhang et al., 2007). Amyloid precursor protein (APP) is a transmembrane protein involved in the pathophysiology of AD (O’Brien and Wong, 2011). Balklava et al. (2015) found that APP interacts with the PIKfyve complex to maintain endosomal homeostasis in C. elegans. They postulated that aberrant processing of APP contributes to the pathophysiology of AD through a cascade of reduced activation of PIKfyve, reduced levels of PI(3,5)P2, endosomal dysfunction, and reduced clearance of beta amyloid. Another example of the relationship between the processing of phosphoinositides and APP comes from a study of Miranda et al. (2018). They found that inhibition of Vps34, a kinase phosphorylating phosphatidylinositol (PI) to PI(3)P, causes endolysosomal dysfunction with secretion of exosomes containing APP C-terminal fragments. Knowles et al. (2017) recently reported that serum levels of PI, the precursor of phosphoinositides like PI(3)P and PI(3,5)P2, is negatively associated with a proxy of genetic susceptibility to BIP.
Some of the genes implicated by the novel loci identified by conditional FDR analyses (Supplementary Tables 1, 2 and Supplementary Figures 1, 2) also relate to known pathophysiological and clinical features of AD and BIP. The PRKCA gene encodes the protein kinase C alpha (PKCa). PKCa is described in amyloid plaque of patients with AD (Clark et al., 1991) where it could contribute to reduced synaptic activity (Alfonso et al., 2016). The PRKCA gene is higher expressed in bipolar mania compared to unipolar depression (Wang et al., 1999), and is lower expressed in fibroblasts of patients with BIP treated with lithium compared to those treated with other medications (Kittel-Schneider et al., 2016). Common genetic variants implicating the PRKCA gene are in healthy individuals associated to impairment of episodic memory (MacLeod and Donaldson, 2014). Variants within the KIAA1109 gene are in family studies associated with multi-system syndromes characterized by impaired neurodevelopment (Alazami et al., 2015; Gueneau et al., 2018), while the MTSS1L gene is associated with neurodegeneration in a consanguineous family study (Alazami et al., 2015). The STARD9 gene is necessary for spindle assembly during cell division in human development, and a mutation in the gene might cause a syndrome with intellectual disability (Okamoto et al., 2017). The locus implicating the AK091365 gene was previously associated with general cognitive function when conditioned on association with schizophrenia (Smeland et al., 2017), which in turn has a high genetic correlation with BIP (Bulik-Sullivan et al., 2015). The SSBP2 gene encodes the single strand DNA binding protein 2, which protects telomeres in a mouse model (Gu p. et al., 2013). In a Mendelian randomization study, Zhan et al. (2015) found that telomere length is causally related to AD. Telomere length is probably not reduced in most patients with BIP (Colpo et al., 2015; Darrow et al., 2016), however; one study found that patients with BIP treated with lithium had longer telomeres compared to patients not receiving lithium (Powell et al., 2017). The RCOR2 gene product is related to cortical development (Wang et al., 2016b) and inflammation (Alvarez-López et al., 2014) in mice. The GRIN2A gene encodes the GluN2A subunit of the N-methyl-D-aspartate (NMDA) receptor. The NMDA receptor is central for synaptic plasticity and learning (Li and Tsien, 2009). Memantine, an NMDA receptor antagonist, probably reduces cognitive decline (Reisberg et al., 2003; Howard et al., 2012) and neuropsychiatric symptoms (Maidment et al., 2008) in AD. Ketamine, another NMDA receptor antagonist, can give short term remission of depression in BIP when used as an add-on to mood stabilizers (Diazgranados et al., 2010; Zarate et al., 2012). Mutations in GRIN2A are previously associated with a range of neuropsychiatric phenotypes including mental retardation, epilepsy, schizophrenia, and BIP (Itokawa et al., 2003; Yuan et al., 2015).
Some of the genes implicated both at genome-wide significance in previously GWASs and by conditional FDR in the present study also have pathophysiological and clinical plausibility. The expression of TRANK1 is decreased in induced pluripotent stem cells derived neurons carrying the common variant found in our study (rs9834970). Decreased expression of TRANK1 alters the expression of other genes related to neuronal development and differentiation (Jiang et al., 2018). Chronic treatment with sodium valproate, a mood stabilizer used in BIP (Macritchie et al., 2001), normalizes the expression of TRANK1 (Jiang et al., 2018). The CNTNAP5 gene encodes a transmembrane protein of the neurexin family, which is related to cellular adhesion and intercellular communication (Traut et al., 2006). Common variants implicating CNTNAP5 have previously been associated with the posterior cortical atrophy variant of AD (Schott et al., 2016), BIP (Djurovic et al., 2010), and response to antipsychotic treatment in schizophrenia (Yu et al., 2018), while rare variants within CNTNAP5 have previously been associated with autism spectrum disorders (Pagnamenta et al., 2010). The NCAN gene is involved in neuronal adhesion and migration (Raum et al., 2015). Common variants implicating NCAN are associated with cognitive performance (Raum et al., 2015) and limbic gray matter volumes (Dannlowski et al., 2015) in healthy individuals, while a rare variant is associated with dyslexia (Einarsdottir et al., 2017).
Further experimental studies should examine the implications of our findings. It is unknown if the loci implicated by condFDR and conjFDR relate to altered levels of gene expression, pathophysiological processes (e.g., impaired neuronal migration, tauopathy, and disturbed endosomal homeostasis), clinical features (e.g., cognitive and affective symptoms), and treatment response to lithium among patients with AD and BIP. Further, it is unknown if the loci interact with environmental risk factors and other genes implicated in AD and BIP.
Our results should be interpreted in light of the following limitations. We can neither exclude that some of the patients with AD have had BIP, nor that some of the patients with BIP will develop AD, which could have confounded our results. However; this could not explain the finding in the conjunctional FDR analyses of one locus implicated in AD and BIP with opposite directions of effect. Due to linkage disequilibrium among SNPs, our findings do not necessarily reflect causal variants, or that the same causal variants are involved in both traits. Although we found indications of modest polygenic overlap using conditional QQ-plots (Figure 1), we only detected two genetic loci jointly associated with both AD and BIP (Figure 2). However, the observed enrichment suggests that more shared SNPs will be identified when GWAS sample sizes increase (Schork et al., 2016). Further, we have only assessed the shared common genetic variants between AD and BIP. Other genetic variations, like rare structural variants, are also shown to increase the risk of AD and BIP (Lord et al., 2014; Cruceanu et al., 2017). Lastly, most participants in the data used in our study are of European ancestry. The generalizability of our findings to samples dominated by participants of other ancestries is unknown.
Conclusion
We find polygenic overlap between AD and BIP and identify novel loci associated with each trait and jointly with both traits, providing new insights into their genetic architecture. The genes MARK2 and VAC14 jointly implicated in AD and BIP are previously described to be involved in neuronal migration, tau phosphorylation, and endosomal homeostasis. Further experimental studies should examine if our findings translate to altered levels of transcription, pathophysiological processes, clinical features, and treatment response to lithium among patients with AD and BIP.
Author Contributions
OD, PF, SH, and OA designed the protocol of the study. OA and OS obtained funding. AS, OS, OF, SH, and YW conducted the analyses. OS, AS, AW, OF, YW, SH, and OA interpreted the results. OD, OS, and AS drafted the manuscript. All authors contributed with the further writing of the manuscript and approved the final manuscript.
Funding
The study was supported by grants from by NIH (NS057198 and EB00790), the Research Council of Norway (229129, 213837, 223273, 251134, and 226971), the South-East Norway Regional Health Authority (2013–123, 2016–064), and KG Jebsen Foundation (SKGJ-2011–36).
Conflict of Interest Statement
OA has received a speaker’s honorarium from Lundbeck and has a patent application (US 20150356243) pending. AD also applied for this patent application and assigned it to UC San Diego. AD has additional disclosures outside the present work: founder, equity holder, and advisory board member for CorTechs Labs, advisory board member of Human Longevity, recipient of non-financial research support from General Electric Healthcare.
The remaining authors declare that the research was conducted in the absence of any commercial or financial relationships that could be construed as a potential conflict of interest.
Acknowledgments
We would like to thank the International Genomics of Alzheimer’s Project (IGAP) for providing summary results data for these analyses. The investigators within IGAP contributed to the design and implementation of IGAP and/or provided data but did not participate in analysis or writing of this report. IGAP was made possible by the generous participation of the control subjects, the patients, and their families. The i–Select chips was funded by the French National Foundation on Alzheimer’s disease and related disorders. EADI was supported by the LABEX (laboratory of excellence program investment for the future) DISTALZ grant, Inserm, Institut Pasteur de Lille, Université de Lille 2 and the Lille University Hospital. GERAD was supported by the Medical Research Council (Grant n∘ 503480), Alzheimer’s Research UK (Grant n∘ 503176), the Wellcome Trust (Grant n∘ 082604/2/07/Z) and German Federal Ministry of Education and Research (BMBF): Competence Network Dementia (CND) grant n∘ 01GI0102, 01GI0711, 01GI0420. CHARGE was partly supported by the NIH/NIA grant R01 AG033193 and the NIA AG081220 and AGES contract N01–AG–12100, the NHLBI grant R01 HL105756, the Icelandic Heart Association, and the Erasmus Medical Center and Erasmus University. ADGC was supported by the NIH/NIA grants: U01 AG032984, U24 AG021886, U01 AG016976, and the Alzheimer’s Association grant ADGC–10–196728. We would like to thank the Psychiatric Genetic Consortium 2 Bipolar Disorder Working Group (PGC2-BIP) for providing summary statistics for the study. The authors are listed in the Supplementary Methods 1.5. We would also like to thank the Human Brain Transcriptome atlas for giving permission to use their figures on spatio-temporal cerebral expression of the genes identified in our study.
Supplementary Material
The Supplementary Material for this article can be found online at: https://www.frontiersin.org/articles/10.3389/fnins.2019.00220/full#supplementary-material
Abbreviations
AD, Alzheimer’s disease; BIP, bipolar disorder; FDR, false discovery rate; GWAS, genome wide association study; IGAP, International Genomics of Alzheimer’s Project; LD, linkage disequilibrium; LDSR, Linkage disequilibrium score regression; PGC2-BIP, Psychiatric Genetic Consortium 2 Bipolar Disorder Working Group; SNP single nucleotide polymorphism; QQ, quantile-quantile.
Footnotes
- ^ https://github.com/Nealelab/ricopili/wiki
- ^ http://web.pasteur-lille.fr/en/recherche/u744/igap/igap_download.php
- ^ https://www.med.unc.edu/pgc/results-and-downloads
- ^ http://hbatlas.org
References
Alazami, A. M., Patel, N., Shamseldin, H. E., Anazi, S., Al-dosari, M. S., Alzahrani, F., et al. (2015). Accelerating novel candidate gene discovery in neurogenetic disorders via whole-exome sequencing of prescreened multiplex consanguineous families. Cell Rep. 10, 148–161. doi: 10.1016/j.celrep.2014.12.015
Alfonso, S. I., Callender, J. A., Hooli, B., Antal, C. E., Mullin, K., Sherman, M. A., et al. (2016). Gain-of-function mutations in protein kinase Ca (PKCa) may promote synaptic defects in alzheimer’s disease. Sci. Signal. 9, 1–7. doi: 10.1126/scisignal.aaf6209
Altshuler, D. L., Durbin, R. M., Abecasis, G. R., Bentley, D. R., Chakravarti, A., Clark, A. G., et al. (2010). A map of human genome variation from population-scale sequencing. Nature 467, 1061–1073. doi: 10.1038/nature09534
Alvarez-López, M. J., Molina-Martínez, P., Castro-Freire, M., Cosín-Tomás, M., Cristòfol, R., Párrizas, M., et al. (2014). Rcor2 underexpression in senescent mice: a target for inflammaging? J. Neuroinflamm. 11, 1–10. doi: 10.1186/1742-2094-11-126
Alzheimer, A. (1907). Uber eine eigenartige Erkrankung der Hirnrinde. Allg. Zeitschrift Fur Psychiatr. Und Phychish-Gerichtliche Medizin 64, 146–148.
American Psychiatric Association. (1994). Diagnostic and Statistical Manual of Mental Disorders 4th ed. Washington, DC: American Psychiatric Association.
Andreassen, O. A., Desikan, R. S., Wang, Y., Thompson, W. K., Schork, A. J., Zuber, V., et al. (2015). Abundant genetic overlap between blood lipids and immune-mediated diseases indicates shared molecular genetic mechanisms. PLoS One 10:e0128048. doi: 10.1371/journal.pone.0123057
Andreassen, O. A., Djurovic, S., Thompson, W. K., Schork, A. J., Kendler, K. S., O’Donovan, M. C., et al. (2013a). Improved detection of common variants associated with schizophrenia by leveraging pleiotropy with cardiovascular-disease risk factors. Am. J. Hum. Genet. 92, 197–209. doi: 10.1016/j.ajhg.2013.01.001
Andreassen, O. A., Thompson, W. K., Schork, A. J., Ripke, S., Mattingsdal, M., Kelsoe, J. R., et al. (2013b). Improved detection of common variants associated with schizophrenia and bipolar disorder using pleiotropy-informed conditional false discovery rate. PLoS Genet. 9:e1005544. doi: 10.1371/journal.pgen.1003455
Antonio, D., Concetta, C., Marco, C., and Alessandro, S. (2015). Enrichment pathway analysis. the inflammatory genetic background in bipolar disorder. . J. Affect. Disord. 179, 88–94. doi: 10.1016/j.jad.2015.03.032
Anttila, V., Bulik-Sullivan, B., Finucane, H. K., Walters, R. K., Bras, J., Duncan, L., et al. (2018). Analysis of shared heritability in common disorders of the brain. Science 360:6395. doi: 10.1126/science.aap8757
Baldessarini, R. J., Bolzani, L., Cruz, N., Jones, P. B., Lai, M., Lepri, B., et al. (2010). Onset-age of bipolar disorders at six international sites. J. Affect. Disord. 121, 143–146. doi: 10.1016/j.jad.2009.05.030
Balklava, Z., Niehage, C., Currinn, H., Mellor, L., Guscott, B., Poulin, G., et al. (2015). The amyloid precursor protein controls PIKfyve function. PLoS One 10:e0130485. doi: 10.1371/journal.pone.0130485
Bulik-Sullivan, B., Finucane, H. K., Anttila, V., Gusev, A., Day, F. R., Loh, P.-R., et al. (2015). An atlas of genetic correlations across human diseases and traits. Nat. Genet. 47, 1236–1241. doi: 10.1038/ng.3406
Cheng, C., Zandi, P., Stuart, E., Lin, C., Su, P., Alexander, G., et al. (2017). Association between lithium use and risk of alzheimer’s disease. J. Clin. Psychiatry 78, e139–e145. doi: 10.4088/JCP.15m10304
Clark, E. A., Leach, K. L., Trojanowski, J. Q., and Lee, V. M. (1991). Characterization and differential distribution of the three major human protein kinase C isozymes (PKC alpha, PKC beta, and PKC gamma) of the central nervous system in normal and alzheimer’s disease brains. Lab. Invest.64, 35–44.
Colpo, G. D., Leffa, D. D., Köhler, C. A., Kapczinski, F., Quevedo, J., and Carvalho, A. F. (2015). Is bipolar disorder associated with accelerating aging? A meta-analysis of telomere length studies. J. Affect. Disord. 186, 241–248. doi: 10.1016/j.jad.2015.06.034
Corvin, A., Craddock, N., and Sullivan, P. F. (2010). Genome-wide association studies: a primer. Psychol. Med. 40, 1063–1077. doi: 10.1017/S0033291709991723
Cruceanu, C., Schmouth, J.-F., Torres-Platas, S. G., Lopez, J. P., Ambalavanan, A., Darcq, E., et al. (2017). Rare susceptibility variants for bipolar disorder suggest a role for G protein-coupled receptors. Mol. Psychiatry 23, 2050–2056. doi: 10.1038/mp.2017.223
Dannlowski, U., Kugel, H., Grotegerd, D., Redlich, R., Suchy, J., Opel, N., et al. (2015). NCAN cross-disorder risk variant is associated with limbic gray matter deficits in healthy subjects and major depression. Neuropsychopharmacology 40, 2510–2516. doi: 10.1038/npp.2015.86
Darrow, S. M., Verhoeven, J. E., Révész, D., Lindqvist, D., Penninx, B. W., Delucchi, K. L., et al. (2016). The association between psychiatric disorders and telomere length: a Meta-analysis involving 14,827 persons. Psychosom. Med. 78, 776–787. doi: 10.1097/PSY.0000000000000356.The
de Bakker, P. I. W., and Raychaudhuri, S. (2012). Interrogating the major histocompatibility complex with high-throughput genomics. Hum. Mol. Genet. 21, 29–36. doi: 10.1093/hmg/dds384
Delaneau, O., Marchini, J., and Zagury, J.-F. (2012). A linear complexity phasing method for thousands of genomes. Nat. Methods 9, 179–181. doi: 10.1038/nmeth.1785
Di Paolo, G., and De Camilli, P. (2006). Phosphoinositides in cell regulation and membrane dynamics. Nature 443, 651–657. doi: 10.1038/nature05185
Diazgranados, N., Kronstein, P., Khalife, S., Kammerer, W. A., Manji, H. K., and Zarate, C. A. (2010). A randomized add-on trial of an N-methyl-D-aspartateantagonist in treatment-resistant bipolar depression. Arch. Gen. Psychiatry 67, 793–802. doi: 10.1001/archgenpsychiatry.2010.90
Diniz, B. S., Teixeira, A. L., Cao, F., Gildengers, A., Soares, J. C., Butters, M. A., et al. (2017). History of bipolar disorder and the risk of dementia: a systematic review and meta-analysis. Am. J. Geriatr. Psychiatry 25, 357–362. doi: 10.1016/j.jagp.2016.11.014
Djurovic, S., Gustafsson, O., Mattingsdal, M., Athanasiu, L., Bjella, T., Tesli, M., et al. (2010). A genome-wide association study of bipolar disorder in Norwegian individuals, followed by replication in icelandic sample. J. Affect. Disord. 126, 312–316. doi: 10.1016/j.jad.2010.04.007
Dreses-Werringloer, U., Lambert, J., Vingtdeux, V., Zhao, H., Siebert, A., Jain, A., et al. (2008). A polymorphism in CALHM1 influences Ca2+ homeostasis, aβlevels, and alzheimer’s disease risk. Cell 133, 1149–1161. doi: 10.1016/j.cell.2008.05.048
Einarsdottir, E., Peyrard-Janvid, M., Darki, F., Tuulari, J. J., Merisaari, H., Karlsson, L., et al. (2017). Identification of NCAN as a candidate gene for developmental dyslexia. Sci. Rep. 7, 1–11. doi: 10.1038/s41598-017-10175-10177
Escott-Price, V., Sims, R., Bannister, C., Harold, D., Vronskaya, M., Majounie, E., et al. (2015). Common polygenic variation enhances risk prediction for alzheimer’s disease. Brain 138, 3673–3684. doi: 10.1093/brain/awv268
Forlenza, O., Aprahamian, I., Radanovic, M., Talib, L., Camargo, M., Stella, F., et al. (2016). Cognitive impairment in late-life bipolar disorder is not associated with Alzheimer’s disease pathological signature in the cerebrospinal fluid. Bipolar Disord. 18, 63–70. doi: 10.1111/bdi.12360
Frei, O., Holland, D., Smeland, O. B., Shadrin, A. A., Chieh, C. F., Maeland, S., et al. (2018). Bivariate causal mixture model quantifies polygenic overlap between complex traits beyond genetic correlation. bioRxiv [Preprint]. doi: 10.1101/240275
Freland, L., and Beaulieu, J.-M. (2012). Inhibition of GSK3 by lithium, from single molecules to signaling networks. Front. Mol. Neurosci. 5:14. doi: 10.3389/fnmol.2012.00014
Gatz, M., Reynolds, C. A., Fratiglioni, L., Johansson, B., Mortimer, J. A., Berg, S., et al. (2006). Role of genes and environments for explaining alzheimer disease. Arch. Gen. Psychiatry 63, 168–174. doi: 10.1001/archpsyc.63.2.168
Gerhard, T., Devanand, D. P., Huang, C., Crystal, S., and Olfson, M. (2015). Lithium treatment and risk for dementia in adults with bipolar disorder: population-based cohort study. Br. J. Psychiatry 207, 46–51. doi: 10.1192/bjp.bp.114.154047
Godefroy, O., Bakchine, S., Verny, M., Delabrousse-Mayoux, J. P., and Roussel, M. (2016). Characteristics of alzheimer’s disease patients with severe executive disorders. J. Alzheimer’s Dis. 51, 815–825. doi: 10.3233/JAD-150971
Gold, C. A., and Budson, A. E. (2008). Memory loss in Alzheimer’s disease: implications for development of therapeutics. Expert Rev. Neurother. 8, 1879–1891. doi: 10.1586/14737175.8.12.1879
Goldstein, B. I., Kemp, D. E., Soczynska, J. K., and McIntyre, R. S. (2009). Inflammation and the phenomenology, pathophysiology, comorbidity, and treatment of bipolar disorder: a systematic review of the literature. J. Clin. Psychiatry 70, 1078–1090. doi: 10.4088/JCP.08r04505
Grande, I., Berk, M., Birmaher, B., and Vieta, E. (2016). Bipolar disorder. Lancet 387, 1561–1572. doi: 10.1016/S0140-6736(15)00241-X
Gu, G. J., Wu, D., Lund, H., Sunnemark, D., Kvist, A. J., Milner, R., et al. (2013). Elevated MARK2-dependent phosphorylation of tau in alzheimer’s disease. J. Alzheimer’s Dis. 33, 699–713. doi: 10.3233/JAD-2012-121357
Gu, P., Deng, W., Lei, M., and Chang, S. (2013). Single strand DNA binding proteins 1 and 2 protect newly replicated telomeres. Cell Res. 23, 705–719. doi: 10.1038/cr.2013.31
Gueneau, L., Fish, R. J., Shamseldin, H. E., Voisin, N., Mau-Them, F. T., Preiksaitiene, E., et al. (2018). KIAA1109 variants are associated with a severe disorder of brain development and arthrogryposis. Am. J. Hum. Genet. 102, 116–132. doi: 10.1016/j.ajhg.2017.12.002
Gulaj, E., Pawlak, K., Bien, B., and Pawlak, D. (2010). Kynurenine and its metabolites in alzheimer’s disease patients. Adv. Med. Sci. 55, 204–211. doi: 10.2478/v10039-010-0023-6
Harold, D., Abraham, R., Hollingworth, P., Sims, R., Gerrish, A., Hamshere, M. L., et al. (2009). Genome-wide association study identifies variants at CLU and PICALM associated with alzheimer’s disease. Nat. Genet. 41, 1088–1093. doi: 10.1038/ng.440
Heath, S. C., Gut, I. G., Brennan, P., McKay, J. D., Bencko, V., Fabianova, E., et al. (2008). Investigation of the fine structure of european populations with applications to disease association studies. Eur. J. Hum. Genet. 16, 1413–1429. doi: 10.1038/ejhg.2008.210
Heneka, M. T., Carson, M. J., Khoury, J., El Landreth, G. E., Brosseron, F., Feinstein, D. L., et al. (2015). Neuroinflammation in alzheimer’s disease. Lancet Neurol. 14, 388–405. doi: 10.1016/S1474-4422(15)70016-70015
Howard, R., McShane, R., Lindesay, J., Ritchie, C., Baldwin, A., Barber, R., et al. (2012). Donepezil and memantine for moderate-to-severe alzheimer’s disease. N. Engl. J. Med. 366, 893–903. doi: 10.1056/NEJMoa1106668
Howie, B. N., Donnelly, P., and Marchini, J. (2009). A flexible and accurate genotype imputation method for the next generation of genome-wide association studies. PLoS Genet. 5:e1000529. doi: 10.1371/journal.pgen.1000529
Itokawa, M., Yamada, K., Iwayama-Shigeno, Y., Ishitsuka, Y., Detera-Wadleigh, S., and Yoshikawa, T. (2003). Genetic analysis of a functional GRIN2A promoter (GT)n repeat in bipolar disorder pedigrees in humans. Neurosci. Lett. 345, 53–56. doi: 10.1016/S0304-3940(03)00501-509
Jack, C. R., Knopman, D. S., Jagust, W. J., Petersen, R. C., Weiner, M. W., Aisen, P. S., et al. (2013). Tracking pathophysiological processes in alzheimer’s disease: An updated hypothetical model of dynamic biomarkers. Lancet Neurol. 12, 207–216. doi: 10.1016/S1474-4422(12)70291-70290
Jakobsson, J., Palsson, E., Sellgren, C., Rydberg, F., Ekman, A., Zetterberg, H., et al. (2016). CACNA1C polymorphism and altered phosphorylation of tau in bipolar disorder. Br. J. Psychiatry 208, 195–196. doi: 10.1192/bjp.bp.114.159806
Jakobsson, J., Zetterberg, H., Blennow, K., Johan Ekman, C., Johansson, A. G. M., and Landén, M. (2013). Altered concentrations of amyloid precursor protein metabolites in the cerebrospinal fluid of patients with bipolar disorder. Neuropsychopharmacology 38, 664–672. doi: 10.1038/npp.2012.231
Jiang, X., Detera-Wadleigh, S. D., Akula, N., Mallon, B. S., Hou, L., Xiao, T., et al. (2018). Sodium valproate rescues expression of TRANK1 in iPSC-derived neural cells that carry a genetic variant associated with serious mental illness. Mol. Psychiatry doi: 10.1038/s41380-018-0207-201 [Epub ahead of print].
Jun, G., Naj, A. C., Beecham, G. W., Wang, L. S., Buros, J., Gallins, P. J., et al. (2010). Meta-analysis confirms CR1, CLU, and PICALM as Alzheimer disease risk loci and reveals interactions with APOE genotypes. Arch. Neurol. 67, 1473–1484. doi: 10.1001/archneurol.2010.201
Kang, H. J., Kawasawa, Y. I., Cheng, F., Zhu, Y., Xu, X., Li, M., et al. (2011). Spatio-temporal transcriptome of the human brain. Nature 478, 483–489. doi: 10.1038/nature10523
Kessing, L. V., Forman, J. L., and Andersen, P. K. (2010). Does lithium protect against dementia? Bipolar Disord. 12, 87–94. doi: 10.1111/j.1399-5618.2009.00788.x
Kessing, L. V., and Nilsson, F. M. (2003). Increased risk of developing dementia in patients with major affective disorders compared to patients with other medical illnesses. J. Affect. Disord. 73, 261–269. doi: 10.1016/S0165-0327(02)00004-6
Kessing, L. V., Olsen, E. W., Mortensen, P. B., and Andersen, P. K. (1999). Dementia in affective disorder: a case-register study. Acta Psychiatr. Scand. 100, 176–185. doi: 10.1111/j.1600-0447.1999.tb10843.x
Kieseppä, T., and Partonen, T. (2004). High concordance of bipolar I disorder in a nationwide sample of twins. Am. J. Psychiatry 161, 1814–1821. doi: 10.1176/appi.ajp.161.10.1814
Kittel-Schneider, S., Lorenz, C., Auer, J., Weiß, L., and Reif, A. (2016). DGKH genetic risk variant influences gene expression in bipolar affective disorder. J. Affect. Disord. 198, 148–157. doi: 10.1016/j.jad.2016.03.041
Knowles, E. E. M., Meikle, P. J., Huynh, K., Göring, H. H. H., Olvera, R. L., Mathias, S. R., et al. (2017). Serum phosphatidylinositol as a biomarker for bipolar disorder liability. Bipolar Disord. 19, 107–115. doi: 10.1111/bdi.12468
Koedam, E. L. G. E., Lauffer, V., Van Der Vlies, A. E., Van Der Flier, W. M., Scheltens, P., and Pijnenburg, Y. A. L. (2010). Early-versus late-onset alzheimer’s disease: more than age alone. J. Alzheimer’s Dis. 19, 1401–1408. doi: 10.3233/JAD-2010-1337
Kon, E., Cossard, A., and Jossin, Y. (2017). Neuronal polarity in the embryonic mammalian cerebral cortex. Front. Cell. Neurosci. 11:163. doi: 10.3389/fncel.2017.00163
Kosuga, S., Tashiro, E., Kajioka, T., Ueki, M., Shimizu, Y., and Imoto, M. (2005). GSK-3β directly phosphorylates and activates MARK2/PAR-1. J. Biol. Chem. 280, 42715–42722. doi: 10.1074/jbc.M507941200
Lambert, J.-C., Grenier-Boley, B., Chouraki, V., Heath, S., Zelenika, D., Fievet, N., et al. (2010). Implication of the immune system in alzheimer’s disease: evidence from genome-wide pathway analysis. J. Alzheimer’s Dis. 20, 1107–1118. doi: 10.3233/JAD-2010-100018
Lambert, J.-C., Ibrahim-Verbaas, C. A., Harold, D., Naj, A. C., Sims, R., Bellenguez, C., et al. (2013). Meta-analysis of 74,046 individuals identifies 11 new susceptibility loci for alzheimer’s disease. Nat. Genet. 45, 1452–1458. doi: 10.1038/ng.2802
Lee, S. H., Harold, D., Nyholt, D. R., Goddard, M. E., Zondervan, K. T., Williams, J., et al. (2013). Estimation and partitioning of polygenic variation captured by common SNPs for alzheimer’s disease, multiple sclerosis and endometriosis. Hum. Mol. Genet. 22, 832–841. doi: 10.1093/hmg/dds491
Lee, S. H., Wray, N. R., Goddard, M. E., and Visscher, P. M. (2011). Estimating missing heritability for disease from genome-wide association studies. Am. J. Hum. Genet. 88, 294–305. doi: 10.1016/j.ajhg.2011.02.002
Li, F., and Tsien, J. (2009). Clinical implications of basic research: memory and the NMDA receptors. N. Engl. J. Med. 361, 302–303. doi: 10.1056/NEJMcibr0902052
Li, Y., Willer, C. J., Ding, J., Scheet, P., and Abecasis, G. R. (2010). MaCH: using sequence and genotype data to estimate haplotypes and unobserved genotypes. Genet. Epidemiol. 34, 816–834. doi: 10.1002/gepi.20533
Lichtenstein, P., Yip, B. H., Björk, C., Pawitan, Y., Cannon, T. D., Sullivan, P. F., et al. (2009). Common genetic determinants of schizophrenia and bipolar disorder in swedish families: a population-based study. Lancet 373, 234–239. doi: 10.1016/S0140-6736(09)60072-60076
Lord, J., Lu, A. J., and Cruchaga, C. (2014). Identification of rare variants in alzheimer’s disease. Front. Genet. 5:369. doi: 10.3389/fgene.2014.00369
Machiela, M. J., and Chanock, S. J. (2015). Genetics and population analysis LDlink: a web-based application for exploring population-specific haplotype structure and linking correlated alleles of possible functional variants. Bioinformatics 31, 3555–3557. doi: 10.1093/bioinformatics/btv402
MacLeod, C. A., and Donaldson, D. I. (2014). PRKCA polymorphism changes the neural basis of episodic remembering in healthy individuals. PLoS One 9:e98018. doi: 10.1371/journal.pone.0098018
Macritchie, K. A., Geddes, J. R., Scott, J., Haslam, D. R., and Goodwin, G. M. (2001). Valproic acid, valproate and divalproex in the maintenance treatment of bipolar disorder. Cochrane Database Syst. Rev. 3:CD003196. doi: 10.1002/14651858.CD003196
Maddison, D. C., and Giorgini, F. (2015). The kynurenine pathway and neurodegenerative disease. Semin. Cell Dev. Biol. 40, 134–141. doi: 10.1016/j.semcdb.2015.03.002
Maidment, I. D., Fox, C. G., Boustani, M., Rodriguez, J., Brown, R. C., and Katona, C. L. (2008). Efficacy of memantine on behavioral and psychological symptoms related to dementia: a systematic meta-analysis. Ann. Pharmacother. 42, 32–38. doi: 10.1345/aph.1K372
Martino, D. J., Samamé, C., Ibañez, A., and Strejilevich, S. A. (2015). Neurocognitive functioning in the premorbid stage and in the first episode of bipolar disorder: a systematic review. Psychiatry Res. 226, 23–30. doi: 10.1016/j.psychres.2014.12.044
Matenia, D., and Mandelkow, E. M. (2009). The tau of MARK: a polarized view of the cytoskeleton. Trends Biochem. Sci. 34, 332–342. doi: 10.1016/j.tibs.2009.03.008
Matsunaga, S., Kishi, T., Annas, P., Basun, H., Hampel, H., and Iwata, N. (2015). Lithium as a treatment for alzheimer’s disease: a systematic review and meta-analysis. J. Alzheimer’s Dis. 48, 403–410. doi: 10.3233/JAD-150437
McCartney, A. J., Zhang, Y., and Weisman, L. S. (2014). Phosphatidylinositol 3,5-bisphosphate: low abundance, high significance. Bioessays 36, 52–64. doi: 10.1002/bies.201300012
McGuffin, P., Rijsdijk, F., Andrew, M., Sham, P., Katz, R., and Cardno, A. (2003). The heritability of bipolar affective disorder and the genetic relationship to unipolar depression. Arch. Gen. Psychiatry 60, 497–502. doi: 10.1001/archpsyc.60.5.497
McKhann, G., Drachman, D., and Folstein, M. (1984). Clinical diagnosis of alzheimer’s disease: report of the NINCDS-ADRDA work group under the auspices of department of health and human services task force on alzheimer’s disease. Neurology 34, 939–944. doi: 10.1212/WNL.34.7.939
Miller, C. L., Llenos, I. C., Dulay, J. R., and Weis, S. (2006). Upregulation of the initiating step of the kynurenine pathway in postmortem anterior cingulate cortex from individuals with schizophrenia and bipolar disorder. Brain Res. 1073–1074, 25–37. doi: 10.1016/j.brainres.2005.12.056
Miranda, A. M., Lasiecka, Z. M., Xu, Y., Neufeld, J., Shahriar, S., Simoes, S., et al. (2018). Neuronal lysosomal dysfunction releases exosomes harboring APP C-terminal fragments and unique lipid signatures. Nat. Commun. 9:291. doi: 10.1038/s41467-017-02533-w
Myint, A. M., Kim, Y. K., Verkerk, R., Park, S. H., Scharpé, S., Steinbusch, H. W. M., et al. (2007). Tryptophan breakdown pathway in bipolar mania. J. Affect. Disord. 102, 65–72. doi: 10.1016/j.jad.2006.12.008
Newell, K. L., Hyman, B. T., Growdon, J. H., and Hedley-Whyte, E. T. (1999). Application of the national institute on aging (NIA)-reagan institute criteria for the neuropathological diagnosis of alzheimer disease. J. Neuropathol. Exp. Neurol. 58, 1147–1155. doi: 10.1097/00005072-199911000-199911004
Nunes, P. V., Forlenxa, O. V., and Gattaz, W. F. (2007). Lithium and risk for alzheimer’s disease in elderly patients with bipolar disorder. Br. J. Psychiatry 190, 359–361. doi: 10.1192/bjp.bp.106.029868
O’Brien, R. J., and Wong, P. C. (2011). Amyloid precursor protein processing and alzheimer’s disease. Annu. Rev. Neurosci. 34, 185–204. doi: 10.1146/annurev-neuro-061010-113613
Okamoto, N., Tsuchiya, Y., Miya, F., Tsunoda, T., Yamashita, K., and Boroevich, K. A. (2017). A novel genetic syndrome with STARD9 mutation and abnormal spindle morphology. Am. J. Med. Genet. 173, 2690–2696. doi: 10.1002/ajmg.a.38391
O’Shea, K. S., and McInnis, M. G. (2016). Neurodevelopmental origins of bipolar disorder: iPSC models. Mol. Cell. Neurosci. 73, 63–83. doi: 10.1016/j.mcn.2015.11.006
Pagnamenta, A. T., Bacchelli, E., De Jonge, M. V., Mirza, G., Scerri, T. S., Minopoli, F., et al. (2010). Characterization of a family with rare deletions in CNTNAP5 and DOCK4 suggests novel risk loci for autism and dyslexia. Biol. Psychiatry 68, 320–328. doi: 10.1016/j.biopsych.2010.02.002
Powell, T. R., Dima, D., Frangou, S., and Breen, G. (2017). Telomere length and bipolar disorder. Neuropsychopharmacology 43, 445–453. doi: 10.1038/npp.2017.125
Psaty, B. M., O’Donnell, C. J., Gudnason, V., Lunetta, K. L., Folsom, A. R., Rotter, J. I., et al. (2009). Cohorts for heart and aging research in genomic epidemiology (CHARGE) consortium design of prospective meta-analyses of genome-wide association studies from 5 cohorts. Circ. Cardiovasc. Genet. 2, 73–80. doi: 10.1161/CIRCGENETICS.108.829747
Purcell, S. M., Wray, N. R., Stone, J. L., Visscher, P. M., O’Donovan, M. C., Sullivan, P. F., et al. (2009). Common polygenic variation contributes to risk of schizophrenia and bipolar disorder. Nature 460, 748–752. doi: 10.1038/nature08185
Rahman, A., Ting, K., Cullen, K. M., Braidy, N., Brew, B. J., and Guillemin, G. J. (2009). The excitotoxin quinolinic acid induces tau phosphorylation in human neurons. PLoS One 4:e6344. doi: 10.1371/journal.pone.0006344
Raum, H., Dietsche, B., Nagels, A., Witt, S. H., Rietschel, M., Kircher, T., et al. (2015). A genome-wide supported psychiatric risk variant in NCAN influences brain function and cognitive performance in healthy subjects. Hum. Brain Mapp. 36, 378–390. doi: 10.1002/hbm.22635
Reiner, O., Shmueli, A., and Sapir, T. (2009). Neuronal migration and neurodegeneration: 2 Sides of the same coin. Cereb. Cortex 19, i42–i48. doi: 10.1093/cercor/bhp039
Reisberg, B., Doody, R., Stöffner, A., Schmitt, F., Ferris, S., Möbius, H. J., et al. (2003). Memantine in moderate-to-severe alzheimer’s disease. N. Engl. J. Med. 348, 1333–1341. doi: 10.1056/nejm200308073490616
Ridge, P. G., Hoyt, K. B., Boehme, K., Mukherjee, S., Crane, P. K., Haines, J. L., et al. (2016). Assessment of the genetic variance of late-onset alzheimer’s disease. Neurobiol. Aging 41, 200.e13–200.e20. doi: 10.1016/j.neurobiolaging.2016.02.024
Ridge, P. G., Mukherjee, S., Crane, P. K., and Kauwe, J. S. K. (2013). Alzheimer’s disease: analyzing the missing heritability. PLoS One 8:e79771. doi: 10.1371/journal.pone.0079771
Sanches, M., Keshavan, M. S., Brambilla, P., and Soares, J. C. (2008). Neurodevelopmental basis of bipolar disorder: A critical appraisal. Prog. Neuro Psychopharmacol. Biol. Psychiatry 32, 1617–1627. doi: 10.1016/j.pnpbp.2008.04.017
Savitz, J., Dantzer, R., Wurfel, B. E., Victor, T. A., Ford, B. N., Bodurka, J., et al. (2015). Neuroprotective kynurenine metabolite indices are abnormally reduced and positively associated with hippocampal and amygdalar volume in bipolar disorder. Psychoneuroendocrinology 52, 200–211. doi: 10.1016/j.psyneuen.2014.11.015
Scheltens, P., Blennow, K., Breteler, M. M. B., de Strooper, B., Frisoni, G. B., Salloway, S., et al. (2016). Alzheimer’s disease. Lancet 388, 505–517. doi: 10.1016/S0140-6736(15)01124-1121
Schork, A. J., Wang, Y., Thompson, W. K., Dale, A. M., and Andreassen, O. A. (2016). New statistical approaches exploit the polygenic architecture of schizophrenia - implications for the underlying neurobiology. Curr. Opin. Neurobiol. 36, 89–98. doi: 10.1016/j.conb.2015.10.008
Schott, J. M., Crutch, S. J., Carrasquillo, M. M., Uphill, J., Shakespeare, T. J., Ryan, N. S., et al. (2016). Genetic risk factors for the posterior cortical atrophy variant of alzheimer’s disease. Alzheimer’s Dement. 12, 862–871. doi: 10.1016/j.jalz.2016.01.010
Smeland, O. B., Bahrami, S., Frei, O., Shadrin, A., O’Connell, K., Savage, J., et al. (2019). Genome-wide analysis reveals extensive genetic overlap between schizophrenia, bipolar disorder, and intelligence. Mol. Psychiatry doi: 10.1038/s41380-018-0332-x [Epub ahead of print].
Smeland, O. B., Frei, O., Kauppi, K., Hill, W. D., Li, W., Wang, Y., et al. (2017). Identification of genetic loci jointly influencing schizophrenia risk and the cognitive traits of verbal-numerical reasoning, reaction time, and general cognitive function. JAMA Psychiatry 74, 1065–1075. doi: 10.1001/jamapsychiatry.2017.1986
Stahl, E., Breen, G., Forstner, A., McQuillin, A., Ripke, S., Bipolar Disorder Working Group of the Psychiatric Genomics Consortium, et al. (2019). Genomewide association study identifies 30 loci associated with bipolar disorder. bioRxiv [Preprint]. doi: 10.1101/173062
Timm, T., Balusamy, K., Li, X., Biernat, J., Mandelkow, E., and Mandelkow, E. M. (2008). Glycogen Synthase Kinase (GSK) 3β directly phosphorylates serine 212 in the regulatory loop and inhibits microtubule affinity-regulating kinase (MARK) 2. J. Biol. Chem. 283, 18873–18882. doi: 10.1074/jbc.M706596200
Torres, I. J., Boudreau, V. G., and Yatham, L. N. (2007). Neuropsychological functioning in euthymic bipolar disorder: a meta-analysis. Acta Psychiatr. Scand. 116, 17–26. doi: 10.1111/j.1600-0447.2007.01055.x
Traut, W., Weichenhan, D., Himmelbauer, H., and Winking, H. (2006). New members of the neurexin superfamily: Multiple rodent homologues of the human CASPR5 gene. Mamm. Genome 17, 723–731. doi: 10.1007/s00335-005-0157-151
Visscher, P. M., Wray, N. R., Zhang, Q., Sklar, P., Mccarthy, M. I., Brown, M. A., et al. (2017). 10 years of GWAS discovery: biology, function, and translation. Am. J. Hum. Genet. 101, 5–22. doi: 10.1016/j.ajhg.2017.06.005
Vos, T., Allen, C., Arora, M., Barber, R. M., Brown, A., Carter, A., et al. (2016). Global, regional, and national incidence, prevalence, and years lived with disability for 310 diseases and injuries, 1990–2015: a systematic analysis for the global burden of disease study 2015. Lancet 388, 1545–1602. doi: 10.1016/S0140-6736(16)31678-31676
Wang, H.-Y., Markowitz, P., Levinson, D., Undie, A. S., and Friedman, E. (1999). Increased membrane-associated protein kinase C activity and translocation in blood platelets from bipolar affective disorder patients. J. Psychiatr. Res. 33, 171–179. doi: 10.1016/S0022-3956(98)90057-90057
Wang, Y., Thompson, W. K., Schork, A. J., Holland, D., Chen, C.-H., Bettella, F., et al. (2016a). Leveraging genomic annotations and pleiotropic enrichment for improved replication rates in schizophrenia GWAS. PLoS Genet. 12:e1005803. doi: 10.1371/journal.pgen.1005803
Wang, Y., Wu, Q., Yang, P., Wang, C., Liu, J., Ding, W., et al. (2016b). LSD1 co-repressor Rcor2 orchestrates neurogenesis in the developing mouse brain. Nat. Commun. 7, 1–14. doi: 10.1038/ncomms10481
World Health Organization. (1977). International Classification of Diseases, Ninth Revision. Geneva: World Health Organization.
World Health Organization. (1992). The ICD-10 classification of mental and behavioural disorders: Clinical descriptions and diagnostic guidelines. Geneva: World Health Organization.
Yu, H., Yan, H., Wang, L., Li, J., Tan, L., Deng, W., et al. (2018). Five novel loci associated with antipsychotic treatment response in patients with schizophrenia: a genome-wide association study. Lancet Psychiatry 5, 327–338. doi: 10.1016/S2215-0366(18)30049-X
Yuan, H., Low, C.-M., Moody, O. A., Jenkins, A., and Traynelis, S. F. (2015). Ionotropic GABA and glutamate receptor mutations and human neurologic diseases. Mol. Pharmacol. 88, 203–217. doi: 10.1124/mol.115.097998
Zarate, C. Jr., Brutsche, N., Ibrahim, L., Franco-Chaves, J., Diazgranados, N., Cravchik, A., et al. (2012). Replication of ketamine’s antidepressant efficacy in bipolar depression: a randomized controlled add-on trial. Biol. Psychiatry 71, 939–946. doi: 10.1016/j.biopsych.2011.12.010
Zhan, Y., Song, C., Karlsson, R., Tillander, A., Reynolds, C. A., Pedersen, N. L., et al. (2015). Telomere length shortening and alzheimer disease - a mendelian randomization study. JAMA Neurol. 72, 1202–1203. doi: 10.1001/jamaneurol.2015.1513
Keywords: Alzheimer’s disease, bipolar disorder, GWAS, pleiotropy, cognitive symptoms, affective symptoms, MARK2, VAC14
Citation: Drange OK, Smeland OB, Shadrin AA, Finseth PI, Witoelar A, Frei O, Psychiatric Genomics Consortium Bipolar Disorder Working Group, Wang Y, Hassani S, Djurovic S, Dale AM and Andreassen OA (2019) Genetic Overlap Between Alzheimer’s Disease and Bipolar Disorder Implicates the MARK2 and VAC14 Genes. Front. Neurosci. 13:220. doi: 10.3389/fnins.2019.00220
Received: 30 January 2018; Accepted: 26 February 2019;
Published: 13 March 2019.
Edited by:
Efthimios M. C. Skoulakis, Alexander Fleming Biomedical Sciences Research Center, GreeceReviewed by:
Petr A. Slominsky, Institute of Molecular Genetics (RAS), RussiaIoannis Sotiropoulos, University of Minho, Portugal
Copyright © 2019 Drange, Smeland, Shadrin, Finseth, Witoelar, Frei, Psychiatric Genomics Consortium Bipolar Disorder Working Group, Wang, Hassani, Djurovic, Dale and Andreassen. This is an open-access article distributed under the terms of the Creative Commons Attribution License (CC BY). The use, distribution or reproduction in other forums is permitted, provided the original author(s) and the copyright owner(s) are credited and that the original publication in this journal is cited, in accordance with accepted academic practice. No use, distribution or reproduction is permitted which does not comply with these terms.
*Correspondence: Ole Kristian Drange, b2xlLmtyaXN0aWFuLmRyYW5nZUBnbWFpbC5jb20=