- 1Biomedical and Translational Informatics Institute, Geisinger, Danville, PA, United States
- 2Department of Genetics, Perelman School of Medicine, University of Pennsylvania, Philadelphia, PA, United States
- 3Weis Center for Research, Geisinger Clinic, Danville, PA, United States
- 4Department of Biostatistics, Epidemiology and Informatics, Perelman School of Medicine, University of Pennsylvania, Philadelphia, PA, United States
- 5Institute for Biomedical Informatics, University of Pennsylvania, Philadelphia, PA, United States
Endometrial cancer is the fourth most commonly diagnosed cancer in women. Family history is a known risk factor for endometrial cancer. The incidence of endometrial cancer in a first-degree relative elevates the relative risk to range between 1.3 and 2.8. It is unclear to what extent or what other novel germline variants are at play in endometrial cancer. We aim to address this question by utilizing whole exome sequencing as a means to identify novel, rare variant associations between exonic regions and endometrial cancer. The MyCode community health initiative is an excellent resource for this study with germline whole exome data for 60,000 patients available in the first phase, and further 30,000 patients independently sequenced in the second phase as part of DiscovEHR study. We conducted exome-wide rare variant association using 472 cases and 4,110 controls in 60,000 patients (discovery cohort); and 261 cases and 1,531 controls from 30,000 patients (replication cohort). After binning rare germline variants into genes, case-control association tests performed using Optimal Unified Approach for Rare-Variant Association, SKAT-O. Seven genes, including RBM12, NDUFB6, ATP6V1A, RECK, SLC35E1, RFX3 (Bonferroni-corrected P < 0.05) and ATP8A1 (suggestive P < 10−5), and one long non-coding RNA, DLGAP4-AS1 (Bonferroni-corrected P < 0.05), were associated with endometrial cancer. Notably, RECK, and ATP8A1 were replicated from the replication cohort (suggestive threshold P < 0.05). Additionally, a pathway-based rare variant analysis, using pathogenic and likely pathogenic variants, identified two significant pathways, pyrimidine metabolism and protein processing in the endoplasmic reticulum (Bonferroni-corrected P < 0.05). In conclusion, our results using the single-source electronic health records (EHR) linked to genomic data highlights candidate genes and pathways associated with endometrial cancer and indicates rare variants involvement in endometrial cancer predisposition, which could help in personalized prognosis and also further our understanding of its genetic etiology.
Introduction
MyCode Community Health initiative is a precision medicine initiative by Geisinger Health System (1). As part of the initiative, blood and other samples are collected from the patients who have consented to participate in the MyCode Community Health initiative. The samples are stored in a systemwide biobank and are sequenced at Regeneron Genetics Center as part of the DiscovEHR project. The high-throughput sequencing data coupled with longitudinal electronic health records (EHR) has been used for genetic research. The genetic data from DiscovEHR has been successfully used to detect various disease-causing variants, which confer increased risk to develop one or more of 21 conditions including hereditary breast and ovarian cancer, familial hypercholesterolemia, cardiomyopathy, Marfan syndrome, and Lynch syndrome (2). The clinically actionable pathogenic variants identified are delivered to the patients through the return of results program at Geisinger Health System and their EHR is updated with the information, which can be readily accessed by their provider (1). As of February, 2019, the results for 1,048 MyCode participants who have one or more of clinically actionable conditions were delivered through the return of results program (2). One of the primary conditions diagnosed as part of the MyCode initiative is Lynch syndrome, and 99 patients out of 1,048 were diagnosed with Lynch Syndrome. Lynch syndrome is caused by germline mutations in one of several DNA mismatch repair genes. Among Lynch syndrome patients, endometrial cancer is the second most diagnosed condition (3). Women with Lynch syndrome have about a 50% chance of developing endometrial cancer (3, 4). Familial risk of endometrial cancer increased by ~2 fold with a first-degree female relative with endometrial cancer (5). However, the germline abnormalities associated with Lynch syndrome only explain a small fraction of heritability of endometrial cancer, which suggests there are other rare germline variants that can help explain the heritability of the disease.
The germline variants have been studied for finding associations with various diseases on a genome-wide scale. Many successful genome-wide association studies (GWAS) have identified common variants associated with multiple complex diseases, including cancer. The NHGRI-EBI catalog has almost 60,000 unique SNP-trait associations (6). Many GWAS have been also conducted on endometrial cancer. One of the recent meta-analysis studies using 7,737 endometrial cancer cases and 37,144 controls identified seven loci associated with endometrial cancer (7). However, to date, all the GWAS using common variants are only able to explain <6% of the familial relative risk for endometrial cancer (7–10). Since common variants discovered to be associated with endometrial cancer have only modest effect size, the missing heritability could be further explained by rare variants.
Rare variants are known to play an essential role in human diseases. Due to the evolutionary purifying selection, deleterious alleles are likely to be rare (11). Moreover, many rare variant analysis studies have found variants to be associated with various cancer types, such as prostate cancer (12), melanoma (13), colorectal cancer (14), urinary tract cancer (15), etc. Notably, rare pathogenic germline variants in various cancers have been linked to functional consequences (16). To the best of our knowledge, rare variants have not been widely studied in endometrial cancer. An exome-wide rare variant association study was conducted in 2014, but they could not find any variants significantly associated with endometrial cancer (17). Therefore, there is a need to further investigate rare variants that are responsible for the endometrial cancer susceptibility. Identifying cancer predisposition genes based on pathogenic rare variants could give novel insight into the genetic basis of endometrial cancer and could be valuable for preventive markers and precision medicine.
In summary, we set out to discover rare variants associated with endometrial cancer using whole exome data where 472 endometrial cancer patients and 4,110 non-cancer patients were pulled from a single hospital system. Their phenotypes and demographics were derived from their EHRs stored in system-wide EPIC database. The rare variants were binned into genes and pathways, and subsequently, statistical tests were run to test the association of biologically-informed units with endometrial cancer. Moreover, the significant results from the discovery phase were validated using an independent DiscovEHR replication cohort with 261 endometrial cancer patients and 1,531 non-cancer patients, also pulled from the same hospital system.
Results
Study Design and Quality Control
Whole exome sequencing was performed on 60,000 samples as part of DiscovEHR study. From 60,000 samples all patients with endometrial cancer (N = 481) and matched controls (N = 4,403) were selected. Further, an independent cohort of 30,000 samples was also sequenced as part of DiscovEHR study, and endometrial cancer (N = 263) and controls (N = 1,586) was pulled as a replication dataset. The controls consisted of women with no diagnosis of cancer and were retrieved by matching the age and body mass index (BMI) with all cancer patients in the cancer registry. Age and BMI were calculated as described in the Methods section. For case population, all patients diagnosed with any type of cancer were retrieved from the cancer registry and then a subset of patients with international classification of diseases for oncology (ICD-O) site codes relevant to endometrial cancer, specifically C54.0: Isthmus uteri, C54.1: Endometrium, C54.3: Fundus uteri, and C54.9: Corpus uteri were selected.
After retrieving the patient IDs for case and control populations, their whole exome sequencing data was retrieved from DiscovEHR. Further, quality filters were applied to the data before performing a rare variant analysis. Any loci with <90% call rate in the exome data were removed, and all patients were confirmed to have genotype call rate >90%. All third-degree relatives (Identity by descent (IBD) > 0.125) were removed. Additionally, principal components were calculated to adjust for population stratification using EIGENSOFT (18). There were 299 related patients with IBD <0.125 with 9 cases and 290 controls in discovery dataset and 55 related patients with two cases and 53 controls in replication dataset that were removed. Further, 10 controls from discovery and replication datasets were removed because of missing BMI in their EHR. After the marker quality control (QC) step, the whole exome sequence had 2,431,845 rare variants with minor allele frequency (MAF) <0.05, and the rare variants were binned into gene bins determined by Entrez Gene annotations. The schematic overview of the study is shown in Figure 1.
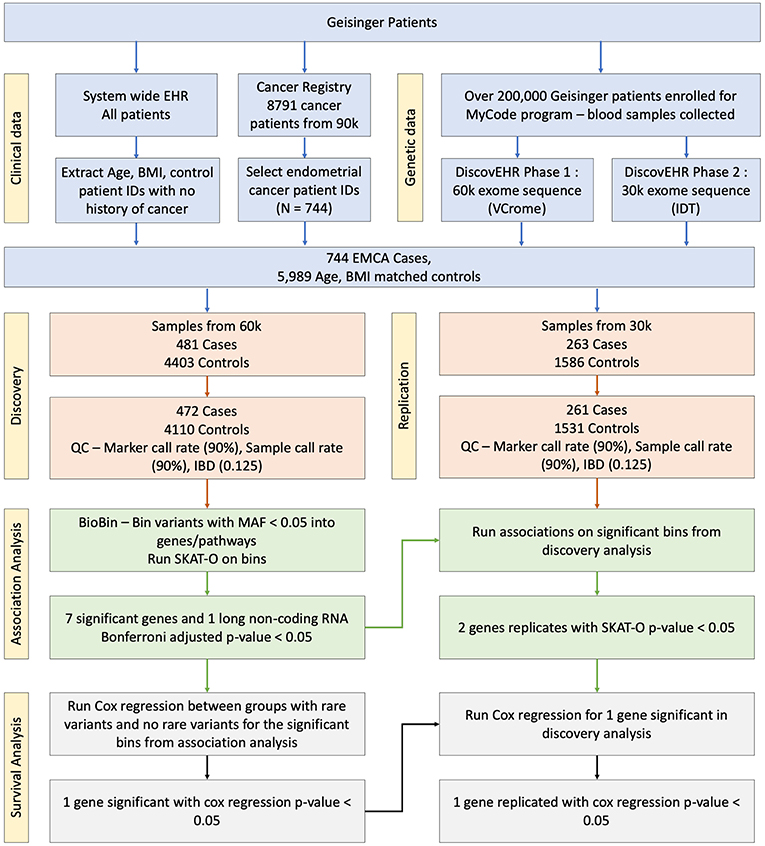
Figure 1. Schematic overview of the association study. The blood samples were collected and sequenced as part of MyCode and DiscovEHR projects. The phenotype information was pulled from the cancer registry and EHR.
The population characteristics for case and control populations after QC steps are summarized in Table 1. The average age of endometrial cancer patients was 60 years with a standard deviation of 11.69 years and control population had an average age of 58 with a standard deviation of 14.45. Further, in case population 64 were deceased, and 408 were still alive as of May 2017, when the data was retrieved from the cancer registry. The control population had 222 deceased and 3,888 patients alive. The table also provides the American Joint Committee on Cancer (AJCC) stage information and the number of patients with a history of any cancer in the family. The two tailed t-test statistics for age and BMI between case and control group in discovery and replication are listed in Supplementary Table 1.
Gene-Based Rare Variant Analysis
BioBin (19–21), a rare variant analysis tool, was used to bin rare variants into genes, and SKAT-O (22) was used to identify associations between genes and phenotype of interest. Any variant below MAF of 5% was considered rare. In the discovery analysis, 2,431,845 rare variant loci were binned into 22,126 genes. Further, any genes with <20 minor allele counts (MAC) were filtered out, leaving only 20,385 genes. Both burden and dispersion tests can be applied to test for association. However, SKAT-O is a unified approach that optimally combines the burden and non-burden sequence kernel association test (SKAT) and increases statistical power to detect associations (22). After running SKAT-O on 20,385 genes, the association p-values were further adjusted for multiple testing using Bonferroni correction and any adjusted p-value below 0.05 was considered significant. We identified six genes and one long non-coding RNA that reached global significance—RBM12, NDUFB6, DLGAP4-AS1, ATP6V1A, RECK, SLC35E1, and RFX3 as shown in Manhattan plot in Figure 2.
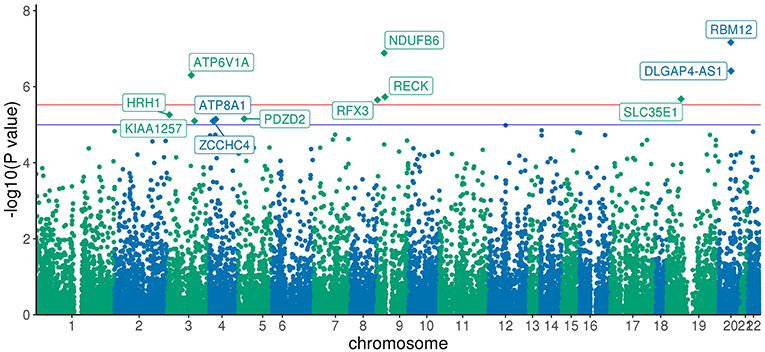
Figure 2. Manhattan Plot of SKAT-O association results: Each point represents one of the 20,385 genes plotted against x-axis being chromosome position and y-axis log transformed SKAT-O p-value. All the genes above the red line are Bonferroni significant, and genes above the blue line have a p-value < 1 × 10−5.
The RECK gene was found to be associated with endometrial cancer and was replicated (p-value < 0.05). ATP8A1 showed a suggestive association (p-value < 10−5) in the discovery analysis and was also replicated (p-value < 0.05). Table 2 shows the number of loci, allele counts, SKAT-O p-value, and Bonferroni corrected p-value of the six protein-coding genes, one long non-coding RNA as well as additional suggestive gene, ATP8A1 from discovery and replication analysis. Table 2 also summarizes the distribution of MAC between case and control populations.
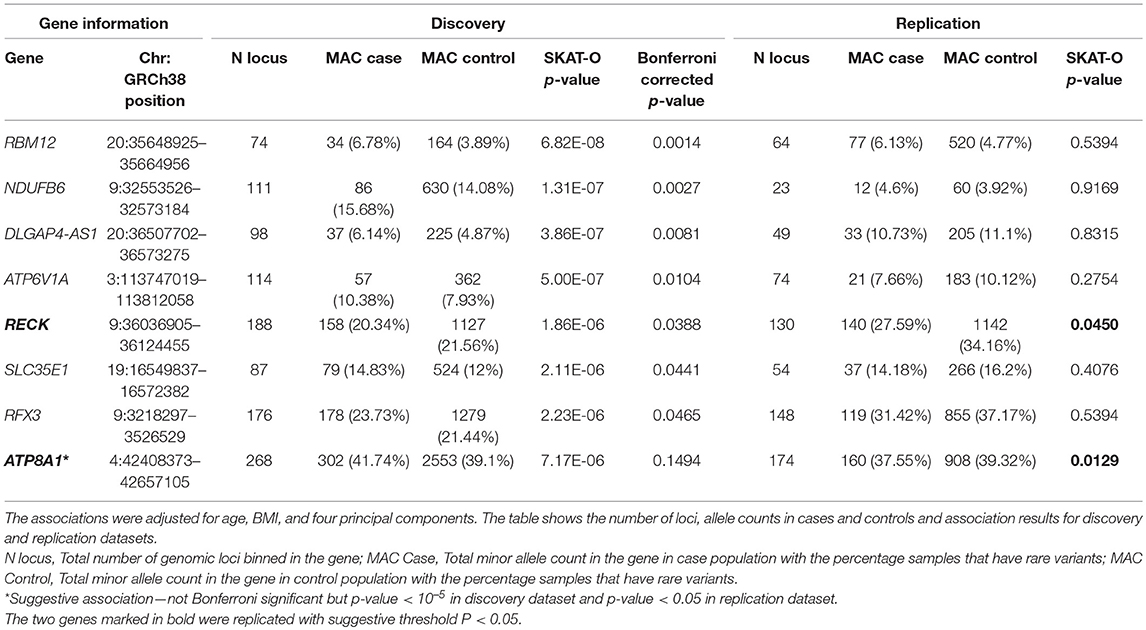
Table 2. Genes associated with endometrial cancer after Bonferroni correction in discovery analysis.
To further characterize the contribution of each locus binned in the significant genes, the association tests were rerun by removing each locus at a time from the gene/bin and p-values (Prm) were generated. A significant increase in Prm (less significant) would indicate the higher significance of the locus in the gene and no change in Prm would indicate the locus in consideration is insignificant. After running the tests, the Prm was lower for 245 loci out of 1,136 total loci binned. All the loci with positive Prm in RECK and annotated as moderate or high impact by Variant Effect Predictor (VEP), are listed in Table 3 and the corresponding plot in Figure 3. A complete list of loci and their Prm for all other significant genes can be found in Supplementary Tables 2–9.
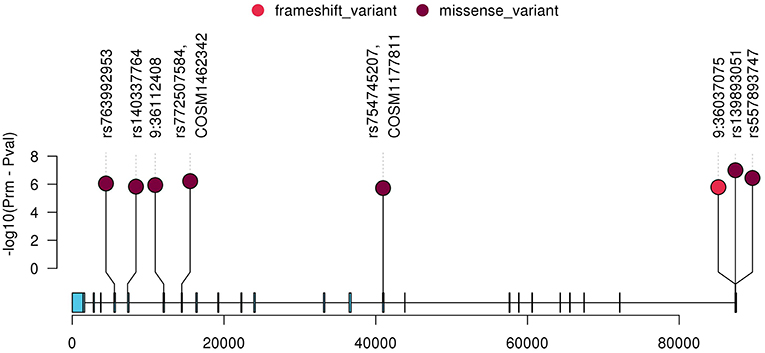
Figure 3. Plot of all variants with lower Prm in RECK which were classified as moderate or high impact by VEP. The y-axis represents negative log scaled Prm-Pval where Pval is the original SKAT-O p-value listed in Table 2, and the x-axis is relative genomic coordinate in the gene.
Variant Annotation
Variants in significant genes were annotated using ClinVar (2018-07) and Variant Effect Predictor (VEP v92) to determine the clinical significance, effect of variants on the protein and implications in human inherited diseases. ClinVar is a public archive that connects human variation to phenotypes, the clinical significance, relationship to human health, and other supporting data obtained through submissions by various groups and aggregated to reflect both consensus and conflicting assertions (23). VEP provides information about the variants' location, gene/transcript affected by variants, types of mutation (i.e., stop gained, missense, stop lost, and frameshift) and protein change scores, which indicate possible partial/complete loss of function of the protein due to amino acid substitution (24). None of the identified variants were found in ClinVar database. However, all variants were successfully annotated using VEP. The distribution of variant types across significant genes as annotated by VEP is shown in Figure 4. Majority of the variants across all significant genes are intron variants (62.0%), followed by missense (12.8%), synonymous (10.6%), and other variants (14.6%).
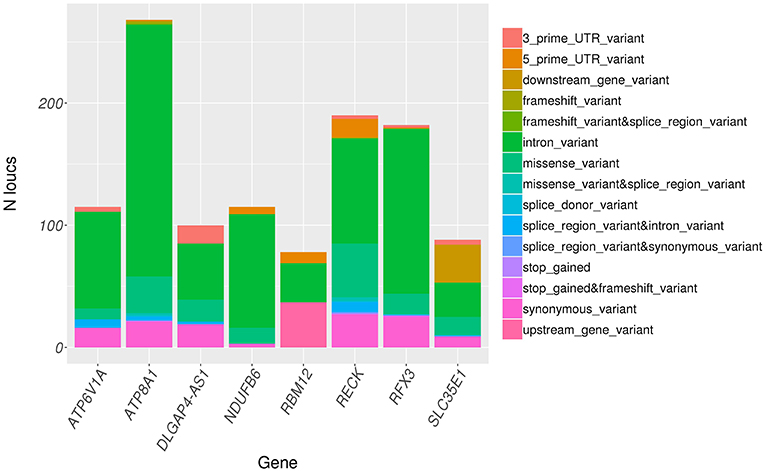
Figure 4. Distribution of variant consequence as determined by VEP for all rare variants in significant genes.
Identified variants were also annotated with a COSMIC database, which contains a manually curated list of somatically acquired mutation in various cancers. Out of 1,136 variants in the significant genes, 50 variants were also found in the COSMIC database (Supplementary Table 10). Three variants in RBM12, one variant in NDUFB6, ten variants in DLGAP4-AS1, five variants in ATPV1A, eleven variants in RECK, four variants in SLC35E1, eight variants in RFX3, and eight variants in ATP8A1 were known somatically acquired mutations in various cancers. The subset of 50 variants with the COSMIC annotation that had lower Prm is summarized in Table 4 with tissue type where the somatic mutations were observed. Two of the somatic mutations COSM1177811 in RECK and COSM1036559 in ATP6V1A were observed in Endometrium.
Pathway-Based Rare Variant Analysis
Rare variants were also binned into pathways using KEGG pathway annotations. As pathway bins would include a large number of loci with a limited sample size, only loci categorized as pathogenic or likely pathogenic with at least one star in ClinVar and loci categorized as high impact by VEP were binned. The association analysis based on pathway bins was also performed using SKAT-O. Out of 317 pathways tested, six pathways were significant with p-value < 0.1 after FDR (Table 5) and two pathways—pyrimidine metabolism and protein processing in endoplasmic reticulum were found to be significant with p-value < 0.05 after Bonferroni correction. However, none of the pathways observed to be associated with endometrial cancer were replicated.
Survival Analysis
The significant genes were further analyzed to determine their association with survival outcome of endometrial cancer patients. The survival analysis was performed using Cox regression, adjusting for age and BMI. NDUFB6 was observed to be significantly associated with survival with Cox regression p-value < 0.05 (Table 6). Out of 472 endometrial cancer patients, 74 had rare variants in NDUFB6, and they had a lower survival rate in comparison to endometrial cancer patients with no rare variants in NDUFB6 (Figure 5).
Discussion
The results from this study illustrate that a population from a single hospital system can be used to identify rare germline variants associated with endometrial cancer diagnosis. Genome-wide rare variant analysis using the DiscovEHR cohort identified seven genes and one long non-coding RNA to be associated with endometrial cancer, of which two genes RECK and ATP8A1 were replicated (suggestive threshold P < 0.05). Additionally, the significance of the variants was evaluated by the backward elimination approach. Variants were also annotated using ClinVar and VEP to examine the variant consequence and any known associations. Further, survival analysis was run to access how the presence of rare variants in a gene influenced the survival of the patient. In summary, many rare variants were discovered, which positively contributed to the association of gene, and some of them were also found to be known somatic mutations in cancer including endometrial cancer. One of the genes found to be associated with endometrial cancer, NDUFB6, was also found to be significantly associated with survival.
Several genes identified in this study have been previously implicated in endometrial cancer or other cancers. The second most significant gene found to be associated with endometrial cancer in this study, NDUFB6 is a nuclear-encoded subunit of NADH-ubiquinone oxidoreductase, also known as Complex I (CI), which is the largest complex of the electron transport chain in mitochondria. CI is known to play a role in tumorigenesis, resistance to cell death and metastasis (25). Moreover, various oncocytic cancer cells have an excessive number of mitochondria. Mutations in NDUFB6 have been observed in oncocytic thyroid tumor (26) and downregulation of NDUFB6 due to the loss in 9p24.1-p13.3 is known to be responsible for metastasis in renal cell carcinoma (27). Besides, in this study, there were 13 missense variants out of which 7 were predicted to be damaging by polyphen score that could disrupt the function of NDUFB6. Moreover, the other 99 variants in intron or UTR region could modify the gene expression. They also could result in alternative splicing of NDUFB6 as there are three distinct isoforms that are encoded by the transcript variants (RefSeq, Jan 2011). Further, one of the endometrial cancer studies investigating mitochondrial DNA mutations conducted an immunohistochemistry analysis of various types of type 1 endometrial cancer samples by staining for nuclear-coded NDUFB6 and mitochondria-coded MTND6. In a 0–4 intensity score for staining, samples with oncocytic-like foci showed about 23% (3/13) complete loss of staining, 23% (3/13) partial loss of staining with an intensity score of 2 and 23% (3/13) partial loss of staining with the intensity score of 3 for NDUFB6 (28). However, in case of endometroid samples with no specific differentiation aspects, 92% (12/13) showed complete staining (intensity 4). Thus, the evidence strongly suggests a role of NDUFB6 in oncocytic endometrial carcinoma and further studies would be required to elucidate precise mechanisms. The survival analysis performed in this study also showed endometrial cancer patients with rare variants in NDUFB6 have a significantly lower survival rate than endometrial cancer patients with no rare variants reemphasizing its possible role in endometrial cancer. Another gene found to be associated with endometrial cancer in this study, SLC35E1 is known to be upregulated in late-stage endometrial endometrioid carcinoma (29). It is also known to have differential membrane proteome expression between normal and inflammatory breast cancer cells, which is a rare and very aggressive form of breast cancer (29, 30). The SLC35E1 is not well-studied, and its mechanism of action in endometrial cancer is not well-understood.
The disruption of tumor suppressor genes and activation of oncogenes are common in cancers. The gene RECK, which was replicated in this study, is known to be a tumor suppressor (31). RECK negatively regulates some matrix metalloproteinases which are known to facilitate tumor invasion and metastasis (31). The epigenetic downregulation of RECK is known to stimulate invasion and migration in colon cancer (32), breast cancer (33), prostate cancer (34), lung cancer (35), and gastric cancer (36). Moreover, RECK is also part of KEGG pathway “MicroRNAs in cancer.” RECK has already been suggested as a promising prognostic marker, and therapeutic agent in the cancers mentioned above (37) and potentially could apply to endometrial cancer. Altogether, 189 variants were discovered in RECK, of which stop gained (N = 1), frame shift (N = 1), and missense variants (N = 44) could lead to abnormal protein product and disrupt tumor suppressor function of gene RECK. Particularly, RECK is known to produce two proteins which have opposing effects, the shorter isoform of RECK leads to faster cell migration (38). Other variants in intron, splicing region and UTR region could also promote cancer by downregulating canonical RECK isoform or alternative splicing of RECK, producing the shorter isoform. Other candidate genes found to be associated with endometrial cancer, ATP6V1A and ATP8A1 act as oncogenes. ATP6V1A is known to drive proliferation and invasion in gastric cancer (39, 40) and ATP8A1 in non-small cell lung cancer (41). The most significantly associated gene in this study, RBM12 is associated with colorectal cancer (42) and tumorigenesis of Meibomian cell carcinoma (42, 43).
One of the pathways discovered by binning rare variants into KEGG pathways, Protein processing in the endoplasmic reticulum is associated with endometrial cancer (44, 45). Disruption in protein processing in endoplasmic reticulum could cause endoplasmic stress and activation of the unfolded protein response (UPR) and GRP78 which facilitates growth and invasion of endometrial cancer (44, 45). Another pathway, Pyrimidine metabolism was also significantly associated with endometrial cancer. Pyrimidines include cytosine, thymine, and uracil which are the basic building blocks of DNA and RNA. Disorders of purine and pyrimidine metabolism are known to increase cancer risk and even act as tumor suppressor depending upon the type and site of alterations (46).
Although we found significant associations, further studies are required to elucidate the functional and molecular mechanisms of the variants and genes in endometrial cancer. A potential limitation of our study is the replication cohort was not sufficiently powered to replicate results with genome-wide significance. Even though we found genome-wide significant genes associated with endometrial cancer in discovery dataset, the results need to be further confirmed by other independent studies, which is common practice for GWAS. Moreover, bigger sample sizes would increase statistical power and help us detect more rare variants and genes with modest effects. DiscovEHR study is ongoing, and participants/patients are still being enrolled and sequenced. Future studies could be conducted to replicate the results when more sequence data is available. Another limitation is that our data consists predominantly of patients with European ancestry due to the inherent ethnic distribution of Geisinger patients. Thus, we can only discover variants associated with European ethnicity. That being said, association analyses in a homogeneous population can be more powerful because the pool of case and controls are not divided across populations, and it can result in more robust associations. This limitation may be addressed in the future as DiscovEHR has started recruiting MyCode participants from geographical areas with a diverse ethnic population.
In conclusion, we have identified seven genes and one long non-coding RNA that are associated with endometrial cancer. At least two of the genes found have some known role in endometrial cancer. Additionally, many genes are associated with other cancers. We suggest that the genes and variants we identified in this study could help explain a fraction of the endometrial cancer heritability, facilitate personalized prognosis, and also aid in increasing our understanding of endometrial cancer etiology.
Methods
Study Population
Geisinger Health System is a health care provider in south-central and northeastern Pennsylvania and southern New Jersey. All the patients who use Geisinger health services are eligible to participate in MyCode community initiative and the study population consisted of these participants. As part of MyCode, all patients who enroll in the program are sequenced regardless of the medical conditions they have. The blood samples were collected at Geisinger and sequenced by Regeneron Genetics Center as part of DiscovEHR study. To date, whole exome sequencing has been performed on approximately 90,000 samples and are linked to their EHR under a protocol approved by Geisinger Institutional Review Board. Additionally, Geisinger also maintains a separate cancer registry, which contains information on all patients who have been diagnosed with cancer. The Geisinger cancer registry also contributes patient data to the National Cancer Database. There were 8,791 patients out of 90,000 sequenced patients diagnosed with any type of cancer from the cancer registry and 481 EMCA cases were identified among them using ICD-9 site codes—C54.3, C54.9, C54.1, and C54.0. The control population consisted of a subset of age and BMI matched patients out of 90,000 sequenced samples with no history of cancer diagnosis based on the absence of any ICD9/ICD10 code related to cancer in a problem-list entry of the diagnosis code, an inpatient hospitalization-discharge diagnosis code, or an encounter diagnosis code. The age for cancer patients was taken as age at initial diagnosis of cancer and for controls, the current age or age at death depending on whether they are alive or dead, respectively. BMI was calculated using median BMI for a year from initial diagnosis for cases. The BMI for controls were calculated using median BMI for a year from the current date for controls still alive and median BMI for a year from date of death for controls who were dead.
Sample Preparation, Sequencing, and Quality Control
The sample preparation is described in detail in Dewey et al. (47). The DNA samples were transferred using 2D matrix tubes (Thermo Scientific) logged in LIMS (Sapio Sciences) and stored in automated biobank at −80°C (LiCONiC Tubestore). The sample quality was tested by running 100 ng of sample on a 2% pre-cast agarose gel (Life Technologies). Additionally, the quantity of sample was determined by fluorescence. The exome capture was prepared through a fully automated approach developed at Regeneron using a custom reagent kit from Kapa Biosystems. The captured DNA was PCR amplified and quantified by qRT—PCR (Kapa Biosystems).
The Exome Sequencing was performed at Regeneron Genetics Center. The 60,000 samples from phase 1 were sequenced using NimbleGen probe target-capture (SeqCap VCRome), and 30,000 samples from phase 2 were sequenced using a slightly modified version of xGen capture (Integrated DNA Technologies), which had supplemental probes added to capture regions of the genome well-covered by VCRome capture reagent but poorly covered by xGen, followed by sequencing on the Illumina HiSeq 2500 platform using the same protocol previously described in detail (48, 49). In summary, the sequencing coverage depth was sufficient to provide >20x haploid depth of over 85% of targeted bases in 96% of samples, with ~80x mean haploid read depth of targeted bases. Further, the reads generated for all samples (FASTQ files) were aligned to genome reference (GRCh38) using BWA-mem (50). The duplicate reads were identified and flagged using Picard MarkDuplicates tool for exclusion in later analysis (51). The variants were called using Genome Analysis Toolkit (GATK) (52, 53). The INDEL-realigned and duplicate-marked reads were processed using GATK HaplotypeCaller to identify variations from genome reference generating genomic VCF files (gVCF). Further, both single-nucleotide variants (SNVs) and indels were identified using GATK's GenotypeGVCFs after genotyping each sample and a training set consisting of 50 randomly selected samples resulting in single-sample VCF files. The joint calling was done in batches of 200 single-sample gVCFs to create pVCF files and all pVCF files generated from joint calling were merged. This process was repeated for both datasets. Further, quality control steps were applied by filtering variant SNP sites for QualityByDepth (QD) score <3 and depth <7, and indels for QD <5 and depth <10. SNP sites and indel sites that don't carry an alternate Allele Balance (AB) ≥ 15% and AB ≥ 20%, respectively, in at least one sample were filtered out. Further, markers with a call rate <90% and samples with the call rate <90% were removed. Related samples up to a 3rd degree (IBD ≥ 0.125) were removed before running the association.
Rare Variant Gene-Based Association Test
All variants below 5% MAF were considered rare and they were binned in genes using BioBin v2.3.0 (19) (https://ritchielab.org/software/biobin-download). BioBin is a software that can collapse variants into biologically-informed bins, such as genes or pathways, and perform rare variant burden tests. BioBin uses a database called Library of Knowledge Integration (LOKI) which integrates knowledge from various disparate data sources about genomic locations of SNPs and genes, as well as known relationships among genes and proteins such as interaction pairs, pathways and ontological categories (19). The gene annotations in LOKI are derived from Entrez Gene (54). BioBin uses these annotations from LOKI as bin regions to bin the variants. After creating the bins, the variants were weighed using Madsen and Browning weights (55). All variants with MAF <5% were binned and genes with <20 variants were filtered out. SKAT-O was run using the R package (22). Additionally, age, BMI and first four principal components were used as covariates. The QQ-plot using gene based SKAT-O p-values is provided in Supplementary Figure 1. The p-values from association tests were adjusted for multiple testing using Bonferroni correction and any gene with p-value <5% was considered significant.
Rare Variant Pathway-Based Association Test
Rare variants were binned into gene pathways to test the association of pathways to endometrial cancer. LOKI is integrated with KEGG pathway information (56). The version of LOKI used in this study integrated the latest data on 15 April 2017 using the KEGG API. The rare variants were binned into 302 pathways and weighed using Madsen and Browning weights (55). Associations were tested using SKAT-O, adjusting for age, BMI and first four principal components. The QQ-plot using pathway based SKAT-O p-values is provided in Supplementary Figure 2. The SKAT-O p-values were adjusted for multiple testing using Bonferroni correction and any p-value <5% was considered significant.
Survival Analysis
Survival analysis was run using Cox regression adjusting for age and BMI, using the following model:
Where “x” is the input from BioBin phe-bins output file which contains Madsen-browning weighted rare variant counts for each patient and gene. The “months” were measured as the number of months from birth to death for dead patients and the number of months from birth to the last follow-up recorded in the EHR for patients alive.
Data Availability
The raw data supporting the conclusions of this manuscript will be made available by the authors to any qualified researcher subject to a data use agreement.
Ethics Statement
The DisocvEHR study cohort is derived from individuals who consented to participate in Geisinger's MyCode Community Health Initiative as described previously (1, 57). Additionally, IRB approval was obtained for this work (IRB- 2016-0119).
Author Contributions
MS, RG, and DK designed and conceived the project. MS, VD, and JM carried out the methodology and implementation. DK and RG helped to supervise the project. MS wrote the paper. All authors revised the manuscript and approved the final version prior to submission.
Funding
This project was funded, in part, under a grant with the Pennsylvania Department of Health (#SAP 4100070267). The Department specifically disclaims responsibility for any analyses, interpretations or conclusions. We gratefully acknowledge the funding support from Geisinger Medical Center (SRC-075) (RG) and Rice Women's Cancer Research Fund (RG and VD). Support for this work was also provided by NHGRI T32HG009495-01 (JM). The funders specifically disclaim responsibility for the study design, data collection, analyses, interpretation, conclusions, and writing of the manuscript.
Conflict of Interest Statement
The authors declare that the research was conducted in the absence of any commercial or financial relationships that could be construed as a potential conflict of interest.
Supplementary Material
The Supplementary Material for this article can be found online at: https://www.frontiersin.org/articles/10.3389/fonc.2019.00574/full#supplementary-material
References
1. Carey DJ, Fetterolf SN, Davis FD, Faucett WA, Kirchner HL, Mirshahi U, et al. The Geisinger MyCode community health initiative: an electronic health record–linked biobank for precision medicine research. Genet Med. (2016) 18:906–13. doi: 10.1038/gim.2015.187
2. Schwartz MLB, McCormick CZ, Lazzeri AL, Lindbuchler DAM, Hallquist MLG, Manickam K, et al. A model for genome-first care: returning secondary genomic findings to participants and their healthcare providers in a large research cohort. Am J Hum Genet. (2018) 103:328–37. doi: 10.1016/j.ajhg.2018.07.009
3. Wang Y, Wang Y, Li J, Cragun J, Hatch K, Chambers SK, et al. Lynch syndrome related endometrial cancer: clinical significance beyond the endometrium. J Hematol Oncol. (2013) 6:22. doi: 10.1186/1756-8722-6-22
4. Hampel H, Frankel W, Panescu J, Lockman J, Sotamaa K, Fix D, et al. Screening for lynch syndrome (hereditary nonpolyposis colorectal cancer) among endometrial cancer patients. Cancer Res. (2006) 66:7810–7. doi: 10.1158/0008-5472.CAN-06-1114
5. Win AK, Reece JC, Ryan S. Family history and risk of endometrial cancer: a systematic review and meta-analysis. Obstetr Gynecol. (2015) 125:89–98. doi: 10.1097/AOG.0000000000000563
6. MacArthur J, Bowler E, Cerezo M, Gil L, Hall P, Hastings E, et al. The new NHGRI-EBI Catalog of published genome-wide association studies (GWAS Catalog). Nucleic Acids Res. (2017) 45:D896–901. doi: 10.1093/nar/gkw1133
7. Cheng THT, Thompson DJ, O'Mara TA, Painter JN, Glubb DM, Flach S, et al. Five endometrial cancer risk loci identified through genome-wide association analysis. Nat Genet. (2016) 48:667–74. doi: 10.1038/ng.3562
8. Carvajal-Carmona LG, O'Mara TA, Painter JN, Lose FA, Dennis J, Michailidou K, et al. Candidate locus analysis of the TERT–CLPTM1L cancer risk region on chromosome 5p15 identifies multiple independent variants associated with endometrial cancer risk. Hum Genet. (2015) 134:231–45. doi: 10.1007/s00439-014-1515-4
9. Cheng THT, Thompson D, Painter J, O'Mara T, Gorman M, Martin L, et al. Meta-analysis of genome-wide association studies identifies common susceptibility polymorphisms for colorectal and endometrial cancer near SH2B3 and TSHZ1. Sci Rep. (2015) 5:17369. doi: 10.1038/srep17369
10. O'Mara TA, Glubb DM, Painter JN, Cheng T, Dennis J, The Australian National Endometrial Cancer Study G, et al. Comprehensive genetic assessment of the ESR1 locus identifies a risk region for endometrial cancer. Endocr Relat Cancer. (2015) 22:851–61. doi: 10.1530/ERC-15-0319
11. Lee S, Abecasis Gonçalo R, Boehnke M, Lin X. Rare-variant association analysis: study designs and statistical tests. Am J Hum Genet. (2014) 95:5–23. doi: 10.1016/j.ajhg.2014.06.009
12. Gudmundsson J, Sulem P, Gudbjartsson DF, Masson G, Agnarsson BA, Benediktsdottir KR, et al. A study based on whole-genome sequencing yields a rare variant at 8q24 associated with prostate cancer. Nat Genet. (2012) 44:1326–9. doi: 10.1038/ng.2437
13. Artomov M, Stratigos AJ, Kim I, Kumar R, Lauss M, Reddy BY, et al. Rare variant, gene-based association study of hereditary melanoma using whole-exome sequencing. J Natl Cancer Inst. (2017) 109:djx083. doi: 10.1093/jnci/djx083
14. Li J, Zou L, Zhou Y, Li L, Zhu Y, Yang Y, et al. A low-frequency variant in SMAD7 modulates TGF-β signaling and confers risk for colorectal cancer in Chinese population. Mol Carcinog. (2017) 56:1798–807. doi: 10.1002/mc.22637
15. Ge Y, Wang Y, Shao W, Jin J, Du M, Ma G, et al. Rare variants in BRCA2 and CHEK2 are associated with the risk of urinary tract cancers. Sci Rep. (2016) 6:33542. doi: 10.1038/srep33542
16. Huang K-l, Mashl RJ, Wu Y, Ritter DI, Wang J, Oh C, et al. Pathogenic germline variants in 10,389 adult cancers. Cell. (2018) 173:355–70.e14. doi: 10.1158/1538-7445.AM2018-5359
17. Chen MM, Crous-Bou M, Setiawan VW, Prescott J, Olson SH, Wentzensen N, et al. Exome-wide association study of endometrial cancer in a multiethnic population. PLoS ONE. (2014) 9:e97045. doi: 10.1371/journal.pone.0097045
18. Price AL, Patterson NJ, Plenge RM, Weinblatt ME, Shadick NA, Reich D. Principal components analysis corrects for stratification in genome-wide association studies. Nat Genet. (2006) 38:904–9. doi: 10.1038/ng1847
19. Moore CB, Wallace JR, Frase AT, Pendergrass SA, Ritchie MD. BioBin: a bioinformatics tool for automating the binning of rare variants using publicly available biological knowledge. BMC Med Genomics. (2013) 6(Suppl. 2):S6. doi: 10.1186/1755-8794-6-S2-S6
20. Basile AO, Byrska-Bishop M, Wallace J, Frase AT, Ritchie MD. Novel features and enhancements in BioBin, a tool for the biologically inspired binning and association analysis of rare variants. Bioinformatics. (2018) 34:527–9. doi: 10.1093/bioinformatics/btx559
21. Moore CCB, Basile AO, Wallace JR, Frase AT, Ritchie MD. A biologically informed method for detecting rare variant associations. BioData Mining. (2016) 9:27. doi: 10.1186/s13040-016-0107-3
22. Lee S, Emond MJ, Bamshad MJ, Barnes KC, Rieder MJ, Nickerson DA, et al. Optimal unified approach for rare-variant association testing with application to small-sample case-control whole-exome sequencing studies. Am J Hum Genet. (2012) 91:224–37. doi: 10.1016/j.ajhg.2012.06.007
23. Landrum MJ, Lee JM, Benson M, Brown G, Chao C, Chitipiralla S, et al. ClinVar: public archive of interpretations of clinically relevant variants. Nucleic Acids Res. (2016) 44:D862–8. doi: 10.1093/nar/gkv1222
24. McLaren W, Gil L, Hunt SE, Riat HS, Ritchie GRS, Thormann A, et al. The ensembl variant effect predictor. Genome Biol. (2016) 17:122. doi: 10.1186/s13059-016-0974-4
25. Urra FA, Muñoz F, Lovy A, Cárdenas C. The mitochondrial Complex(I)ty of cancer. Front Oncol. (2017) 7:118. doi: 10.3389/fonc.2017.00118
26. Evangelisti C, de Biase D, Kurelac I, Ceccarelli C, Prokisch H, Meitinger T, et al. A mutation screening of oncogenes, tumor suppressor gene TP53 and nuclear encoded mitochondrial complex I genes in oncocytic thyroid tumors. BMC Cancer. (2015) 15:157. doi: 10.1186/s12885-015-1122-3
27. Narimatsu T, Matsuura K, Nakada C, Tsukamoto Y, Hijiya N, Kai T, et al. Downregulation of NDUFB6 due to 9p24.1-p13.3 loss is implicated in metastatic clear cell renal cell carcinoma. Cancer Med. (2015) 4:112–24. doi: 10.1002/cam4.351
28. Guerra F, Kurelac I, Cormio A, Zuntini R, Amato LB, Ceccarelli C, et al. Placing mitochondrial DNA mutations within the progression model of type I endometrial carcinoma. Hum Mol Genet. (2011) 20:2394–405. doi: 10.1093/hmg/ddr146
29. Chang S-J, Wang T-Y, Tsai C-Y, Hu T-F, Chang MD-T, Wang H-W. Increased epithelial stem cell traits in advanced endometrial endometrioid carcinoma. BMC Genomics. (2009) 10:613. doi: 10.1186/1471-2164-10-613
30. Suárez-Arroyo IJ, Feliz-Mosquea YR, Pérez-Laspiur J, Arju R, Giashuddin S, Maldonado-Martínez G, et al. The proteome signature of the inflammatory breast cancer plasma membrane identifies novel molecular markers of disease. Am J Cancer Res. (2016) 6:1720–40.
31. Takagi S, Simizu S, Osada H. RECK negatively regulates matrix metalloproteinase-9 transcription. Cancer Res. (2009) 69:1502–8. doi: 10.1158/0008-5472.CAN-08-2635
32. Cho C-Y, Wang J-H, Chang H-C, Chang C-K, Hung W-C. Epigenetic inactivation of the metastasis suppressor RECK enhances invasion of human colon cancer cells. J Cell Physiol. (2007) 213:65–9. doi: 10.1002/jcp.21089
33. Walsh LA, Roy DM, Reyngold M, Giri D, Snyder A, Turcan S, et al. RECK controls breast cancer metastasis by modulating a convergent, STAT3-dependent neoangiogenic switch. Oncogene. (2015) 34:2189–203. doi: 10.1038/onc.2014.175
34. Hirata H, Ueno K, Shahryari V, Deng G, Tanaka Y, Tabatabai ZL, et al. MicroRNA-182-5p promotes cell invasion and proliferation by down regulating FOXF2, RECK and MTSS1 genes in human prostate cancer. PLoS ONE. (2013) 8:e55502. doi: 10.1371/journal.pone.0055502
35. Xu L-F, Wu Z-P, Chen Y, Zhu Q-S, Hamidi S, Navab R. MicroRNA-21 (miR-21) regulates cellular proliferation, invasion, migration, and apoptosis by targeting PTEN, RECK and Bcl-2 in lung squamous carcinoma, Gejiu City, China. PLoS ONE. (2014) 9:e103698. doi: 10.1371/journal.pone.0103698
36. Xie J, Tan Z-H, Tang X, Mo M-S, Liu Y-P, Gan R-L, et al. MiR-374b-5p suppresses RECK expression and promotes gastric cancer cell invasion and metastasis. World J Gastroenterol. (2014) 20:17439–47. doi: 10.3748/wjg.v20.i46.17439
37. Clark JCM, Thomas DM, Choong PFM, Dass CR. RECK—a newly discovered inhibitor of metastasis with prognostic significance in multiple forms of cancer. Cancer Metastasis Rev. (2007) 26:675–83. doi: 10.1007/s10555-007-9093-8
38. Lee HN, Mitra M, Bosompra O, Corney DC, Johnson EL, Rashed N, et al. RECK isoforms have opposing effects on cell migration. Mol Biol Cell. (2018) 29:1825–38. doi: 10.1091/mbc.E17-12-0708
39. Liu P, Chen H, Han L, Zou X, Shen W. Expression and role of V1A subunit of V-ATPases in gastric cancer cells. Int J Clin Oncol. (2015) 20:725–35. doi: 10.1007/s10147-015-0782-y
40. Wang P, Wang L, Sha J, Lou G, Lu N, Hang B, et al. Expression and transcriptional regulation of human ATP6V1A gene in gastric cancers. Sci Rep. (2017) 7:3015. doi: 10.1038/s41598-017-03021-3
41. Dong W, Yao C, Teng X, Chai J, Yang X, Li B. MiR-140-3p suppressed cell growth and invasion by downregulating the expression of ATP8A1 in non-small cell lung cancer. Tumor Biol. (2016) 37:2973–85. doi: 10.1007/s13277-015-3452-9
42. Giannakis M, Mu XJ, Shukla SA, Qian ZR, Cohen O, Nishihara R, et al. Genomic correlates of immune-cell infiltrates in colorectal carcinoma. Cell Rep. (2016) 15:857–65. doi: 10.1016/j.celrep.2016.03.075
43. Kumar A, Kumar Dorairaj S, Prabhakaran VC, Prakash DR, Chakraborty S. Identification of genes associated with tumorigenesis of meibomian cell carcinoma by microarray analysis. Genomics. (2007) 90:559–66. doi: 10.1016/j.ygeno.2007.07.008
44. Ulianich L, Insabato L. Endoplasmic reticulum stress in endometrial cancer. Front Med. (2014) 1:55. doi: 10.3389/fmed.2014.00055
45. Rao RV, Bredesen DE. Misfolded proteins, endoplasmic reticulum stress and neurodegeneration. Curr Opin Cell Biol. (2004) 16:653–62. doi: 10.1016/j.ceb.2004.09.012
46. Buj R, Aird KM. Deoxyribonucleotide triphosphate metabolism in cancer and metabolic disease. Front Endocrinol. (2018) 9:177. doi: 10.3389/fendo.2018.00177
47. Dewey FE, Gusarova V, O'Dushlaine C, Gottesman O, Trejos J, Hunt C, et al. Inactivating variants in ANGPTL4 and risk of coronary artery disease. N Engl J Med. (2016) 374:1123–33. doi: 10.1056/NEJMoa1510926
48. Staples J, Maxwell EK, Gosalia N, Gonzaga-Jauregui C, Snyder C, Hawes A, et al. Profiling and leveraging relatedness in a precision medicine cohort of 92,455 exomes. Am J Hum Genet. (2018) 102:874–89. doi: 10.1016/j.ajhg.2018.03.012
49. Mirshahi UL, Luo JZ, Manickam K, Wardeh AH, Mirshahi T, Murray MF, et al. Trajectory of exonic variant discovery in a large clinical population: implications for variant curation. Genet Med. (2018) 21, 1417–24. doi: 10.1038/s41436-018-0353-5
50. Li H, Durbin R. Fast and accurate short read alignment with Burrows-Wheeler transform. Bioinformatics. (2009) 25:1754–60. doi: 10.1093/bioinformatics/btp324
51. “Picard Toolkit,.” Broad Institute, GitHub Repository. Broad Institute (2019). Available online at: http://broadinstitute.github.io/picard/ (accessed March 1, 2018).
52. McKenna A, Hanna M, Banks E, Sivachenko A, Cibulskis K, Kernytsky A, et al. The genome analysis toolkit: a MapReduce framework for analyzing next-generation DNA sequencing data. Genome Res. (2010) 20:1297–303. doi: 10.1101/gr.107524.110
53. Van der Auwera GA, Carneiro MO, Hartl C, Poplin R, del Angel G, Levy-Moonshine A, et al. From FastQ data to high confidence variantcalls: the Genome Analysis Toolkit best practices pipeline. Curr Protoc Bioinformatics. (2013) 11:11.0.1–33. doi: 10.1002/0471250953.bi1110s43
54. Maglott D, Ostell J, Pruitt KD, Tatusova T. Entrez Gene: gene-centered information at NCBI. Nucleic Acids Res. (2011) 39:D52–7. doi: 10.1093/nar/gkq1237
55. Madsen BE, Browning SR. A groupwise association test for rare mutations using a weighted sum statistic. PLoS Genet. (2009) 5:e1000384. doi: 10.1371/journal.pgen.1000384
56. Kanehisa M, Sato Y, Kawashima M, Furumichi M, Tanabe M. KEGG as a reference resource for gene and protein annotation. Nucleic Acids Res. (2016) 44:D457–62. doi: 10.1093/nar/gkv1070
Keywords: endometrial cancer, rare variant, cancer predisposition gene, EHR, EHR-linked Biobank, DiscovEHR cohort
Citation: Shivakumar M, Miller JE, Dasari VR, Gogoi R and Kim D (2019) Exome-Wide Rare Variant Analysis From the DiscovEHR Study Identifies Novel Candidate Predisposition Genes for Endometrial Cancer. Front. Oncol. 9:574. doi: 10.3389/fonc.2019.00574
Received: 27 February 2019; Accepted: 13 June 2019;
Published: 05 July 2019.
Edited by:
Rengyun Liu, Johns Hopkins University, United StatesReviewed by:
Shicheng Guo, Marshfield Clinic Research Institute, United StatesYanqiang Li, Houston Methodist Research Institute, United States
Copyright © 2019 Shivakumar, Miller, Dasari, Gogoi and Kim. This is an open-access article distributed under the terms of the Creative Commons Attribution License (CC BY). The use, distribution or reproduction in other forums is permitted, provided the original author(s) and the copyright owner(s) are credited and that the original publication in this journal is cited, in accordance with accepted academic practice. No use, distribution or reproduction is permitted which does not comply with these terms.
*Correspondence: Radhika Gogoi, cnBnb2dvaUBnZWlzaW5nZXIuZWR1; Dokyoon Kim, ZG9reW9vbi5raW1AcGVubm1lZGljaW5lLnVwZW5uLmVkdQ==