- 1Department of Hepatobiliary Surgery, The First People's Hospital of Fuyang Hangzhou, Hangzhou, China
- 2Department of Breast Surgery, The First Affiliated Hospital of Zhejiang University, College of Medicine, Zhejiang University, Hangzhou, China
Growing evidence has illustrated critical roles of competing endogenous RNA (ceRNA) regulatory network in human cancers including hepatocellular carcinoma. In this study, we aimed to find promising diagnostic and prognostic biomarkers for patients with hepatocellular carcinoma. Three novel unfavorable prognosis-associated genes (CELSR3, GPSM2, and CHEK1) was first identified. We also demonstrated that these genes were significantly upregulated in hepatocellular carcinoma cell lines and tissues. Next, 154 potential miRNAs of CELSR3, GPSM2, and CHEK1 were predicted. CHEK1-hsa-mir-195-5p/hsa-mir-497-5p and GPSM2-hsa-mir-122-5p axes were defined as two key pathways in carcinogenesis of hepatocellular carcinoma by combination of in silico analysis and experimental validation. Subsequently, lncRNAs binding to hsa-mir-195-5p, hsa-mir-497-5p, and hsa-mir-122-5p were predicted via starBase and miRNet databases. After performing expression analysis and survival analysis for these predicted lncRNAs, we showed that nine lncRNAs (SNHG1, SNHG12, LINC00511, HCG18, FGD5-AS1, CERS6-AS1, NUTM2A-AS1, SNHG16, and ASB16-AS1) were markedly increased in hepatocellular carcinoma and their upregulation indicated poor prognosis. Moreover, a similar mRNA-miRNA-lncRNA analysis for six “known” genes (CLEC3B, DNASE1L3, PTTG1, KIF2C, XPO5, and UBE2S) was performed. Subsequently, a comprehensive mRNA-miRNA-lncRNA triple ceRNA network linked to prognosis of patients with hepatocellular carcinoma was established. Moreover, all RNAs in this network exhibited significantly diagnostic values for patients with hepatocellular carcinoma. In summary, the current study constructed a mRNA-miRNA-lncRNA ceRNA network associated with diagnosis and prognosis of hepatocellular carcinoma.
Introduction
Hepatocellular carcinoma is one of the most common types of cancer, with nearly 600,000 newly-diagnosed cases of patients with hepatocellular carcinoma (1–3).
Although great advances in the therapeutic approaches have been achieved in the past decades, the prognosis of patients with hepatocellular carcinoma remains dismal, with five-year survival rates less than 20% (4). Most of patients diagnosed at advanced stage of hepatocellular carcinoma and high incidence of recurrence and metastasis after curative therapies may account for this poor prognosis (5). Therefore, exploring detailed mechanisms of pathogenesis of hepatocellular carcinoma and identifying promising diagnostic and prognostic biomarkers of hepatocellular carcinoma may be helpful for providing effective therapeutic targets and improving patients' outcome. A hypothesis, namely competing endogenous RNA (ceRNA), that non-coding RNA (ncRNA), including long non-coding RNA (lncRNA), can cross-talk with messenger RNA (mRNA) by competitively binding to shared miRNAs was proposed by Salmena et al. (6). In recent years, a variety of studies regarding roles of competing endogenous RNA (ceRNA) regulatory network in human cancers have been launched and lots of attractive findings have been obtained. For example, Li et al. (7) identified prognostic signatures associated with long-term overall survival of thyroid cancer patients based on a competing endogenous RNA network; Wang et al. (8) found some prognostic markers for glioblastoma by ceRNA network analysis; Wang et al. (9) constructed a mRNA-miRNA-lncRNA competing endogenous RNA triple sub-network associated with prognosis of pancreatic cancer. However, current knowledge about ceRNA regulatory network in hepatocellular carcinoma remains extremely limited and need to be further probed. In this study, by employing “mRNA-miRNA-lncRNA” order pattern instead of “lncRNA-miRNA-mRNA” order pattern, we constructed a comprehensive ceRNA sub-network, in which all RNAs possess significant diagnostic and prognostic values for patients with hepatocellular carcinoma.
Materials and Methods
GEPIA Database Analysis
GEPIA (Gene Expression Profiling Interactive Analysis, http://gepia.cancer-pku.cn/detail.php) is a newly developed interactive web server for analyzing the RNA sequencing expression data from The Cancer Genome Atlas (TCGA) and the Genotype-Tissue Expression (GTEx) projects (10). GEPIA was employed to obtain the genes most associated with overall survival and disease-free survival of patients with hepatocellular carcinoma. Logrank P < 0.05 was considered as statistically significant. GEPIA was also used to perform expression analysis and survival analysis of lncRNA. The expression correlation between potential mRNA-lncRNA pairs was also determined by GEPIA. For expression analysis, P < 0.05 was considered as statistically significant. For correlation analysis, R > 0.1 and P < 0.05 were set as the criteria for identifying significant mRNA-lncRNA interactive pairs.
UALCAN Database Analysis
mRNA expression slevels of three candidate genes (CELSR3, GPSM2, and CHEK1) in hepatocellular carcinoma were further detected using UALCAN database (http://ualcan.path.uab.edu/index.html), which is a comprehensive, user-friendly, and interactive web resource for analyzing cancer data (11). P < 0.05 was considered as statistically significant.
Oncomine Database Analysis
Oncomine (https://www.oncomine.org/) is a cancer microarray database and an integrated data-mining platform (12). In this study, Oncomine was utilized to analyze mRNA expression of CELSR2, GPSM2, and CHEK1 in hepatocellular carcinoma by conducting a meta-analysis of datasets as we previously described (13). P < 0.05 and |fold change| > 1.5 were set as the thresholds for selecting included datasets.
HumanProteinAtlas Database Analysis
HumanProteinAtlas (https://www.proteinatlas.org/) is a Swedish-based database, which was initiated in 2003 with the aim to map all the human proteins in cells, tissues and organs (14–16). CELSR2, GPSM2, and CHEK1 protein expression levels in hepatocellular carcinoma were assessed using HumanProteinAtlas database.
miRNet Database Analysis
miRNet (http://www.mirnet.ca/), an easy-to-use online tool for miRNA-associated studies, was used to predict potential miRNAs binding to mRNAs (17, 18). Besides, it was also employed to predict potential lncRNAs binding to miRNAs. mRNA-miRNA and miRNA-lncRNA regulatory networks were subsequently established by Cytoscape software.
StarBase Database Analysis
starBase (http://starbase.sysu.edu.cn/) is an open-source database for investigating non-coding RNA interactions from CLIP-seq, degradome-seq and RNA-RNA interactome data (19, 20). starBase was introduced to perform expression correlation analysis for mRNA-miRNA and miRNA-lncRNA pairs in hepatocellular carcinoma. R < −0.1 and P < 0.05 were set as the criteria for identifying significant interactions. miRNA expression values in hepatocellular carcinoma were also determined using starBase. P < 0.05 was considered as statistically significant. Besides, starBase was employed to predict potential lncRNAs binding to miRNAs.
Kaplan-Meier Plotter Analysis
Kaplan-Meier plotter database is capable to assess the effect of miRNAs and genes on survival in 21 cancer types, including hepatocellular carcinoma (21). The prognostic values of potential miRNAs in hepatocellular carcinoma was evaluated using Kaplan-Meier plotter (http://kmplot.com/analysis/) as we previously described (22, 23). In brief, each miRNA of interest was first entered into this database. According to median expression value, all cases were classified into a low expression group and a high expression group. Subsequently, Kaplan-Meier survival plots were generated, and statistical indices containing hazard ratio (HR), 95% confidence interval (CI), logrank P-value were automatically calculated and directly displayed on the webpage. Logrank P < 0.05 was considered as statistically significant.
Cell Culture and Clinical Tissues
Human hepatocellular carcinoma cell lines hepG2 and LM3 and normal hepatic cell line HL7702 used in this study were kindly provided by the First Affiliated Hospital of Medical College, Zhejiang University (Hangzhou, China). All cell lines were cultured in Dulbecco's modified Eagle's medium (DMEM; Gibco, 12430047) supplemented with 10% fetal bovine serum (FBS; Biological Industries, 04-0101-1, Cromwell, CT, USA) under a humidified atmosphere of 5% CO2 at 37°C. Twenty-two clinical hepatocellular carcinoma tissues and matched adjacent normal hepatic tissues were collected from hepatocellular carcinoma patients who underwent surgery at the First Affiliated Hospital of Medical College, Zhejiang University (Hangzhou, China). All procedures performed in this study involving human participants were conducted in accordance with the ethical standards of the First Affiliated Hospital of Medical College, Zhejiang University. The written informed consent from every participant was obtained.
RNA Extraction, Reverse Transcription PCR and Quantitative Real-Time PCR (qRT-PCR)
RNA extraction, reverse transcription PCR and quantitative real-time fluorescence PCR were conducted as previously described (24, 25). Total RNA was first extracted from cells and clinical samples using RNAiso plus Reagent (TaKaRa, Kusatsu, Japan), after which total RNA was reversely transcribed into complementary DNA (cDNA) by the PrimeScriptTM RT Reagent Kit (TaKaRa, RR037A). Then, qRT-PCR was performed using SYBR Premix Ex Taq (TaKaRa, RR420A) in a Roche LightCycle480 II Real-Time PCR Detection System. GAPDH was employed as the internal control for gene expression analysis. Gene expression was normalized to GAPDH and calculated through the comparative threshold method of 2−ΔΔCT. All primers used in this study were listed Table S1.
Cell Transfection
20 × 104 of hepG2 and LM3 cells were seeded into 6-well plates and cultured for 12 h. Then, miRNA mimics and negative controls were transfected into these cells using Opti-MEM and Lipofectamine 3000 reagents (Invitrogen, Shanghai, China) according to the manufacturer's instruction. At 12 h post-transfection, the medium was replaced with fresh DMEM. The miRNA mimics and negative controls were synthesized and purchased from RiboBio Co. Ltd. (Guangzhou, China).
Statistical Analysis
Most of the statistical analyses were done by the bioinformatic online tools as mentioned above. P-values from GEPIA expression analysis, logrank P-values from GEPIA and Kaplan-Meier plotter survival analysis were corrected by false discovery rate and other reported P-values by online tools were not adjusted for false discovery rate correction. The statistical analyses of experimental data were conducted by GraphPad Prism software (version 7.0.3). Experiments were performed in triplicates and shown as mean ± standard deviation (SD) from at least three independent times.
Student's t-test (two tailed) were employed to do comparisons between two groups. ROC curve was utilized to assess diagnostic effect. Logrank P < 0.05 or P < 0.05 was considered as statistically significant.
Results
CELSR3, GPSM2, and CHEK1 Were Identified as Three Novel Prognosis-Associated Genes in Hepatocellular Carcinoma
To obtain the genes most associated with patient survival in hepatocellular carcinoma, GEPIA database was first utilized. In this study, two indices regarding to patients' outcome, overall survival (OS) and disease-free survival (RFS), were included. The top 100 OS-associated genes and the top 100 RFS-associated genes were identified as listed in Tables 1, 2, respectively. By intersecting OS-associated genes and RFS-associated genes, 9 genes (CLEC3B, DNASE1L3, PTTG1, CELSR3, GPSM2, KIF2C, XPO5, UBE2S, and CHEK1) were defined as candidate genes, which were commonly appeared in OS-associated gene set and RFS-associated gene set (Figure 1A). After reviewing the published literatures and previous studies, we found that 6 of 9 genes [CLEC3B (26), DNASE1L3 (27), PTTG1 (28, 29), KIF2C (30), XPO5 (31, 32), and UBE2S (33, 34)] have been demonstrated to act as promising prognostic biomarkers for hepatocellular carcinoma. The rest three genes (CELSR3, GPSM2, and CHEK1) have not been studied for their prognostic values in hepatocellular carcinoma so far. Therefore, CELSR3, GPSM2 and CHEK1 were considered as three novel potential prognostic biomarkers for hepatocellular carcinoma. The prognostic values (OS and RFS) of CELSR3, GPSM2, and CHEK1 were presented in Figures 1B–G. The results suggested that high expression of CELSR3, GPSM2, or CHEK1 indicated poor prognosis in patients with hepatocellular carcinoma.
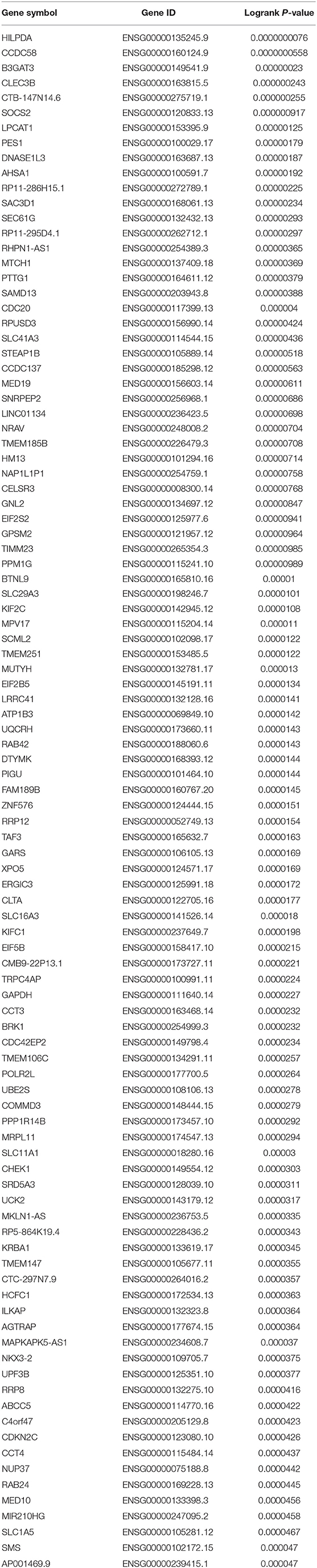
Table 1. The genes most associated with overall survival (OS) of patients with hepatocellular carcinoma determined by GEPIA database.
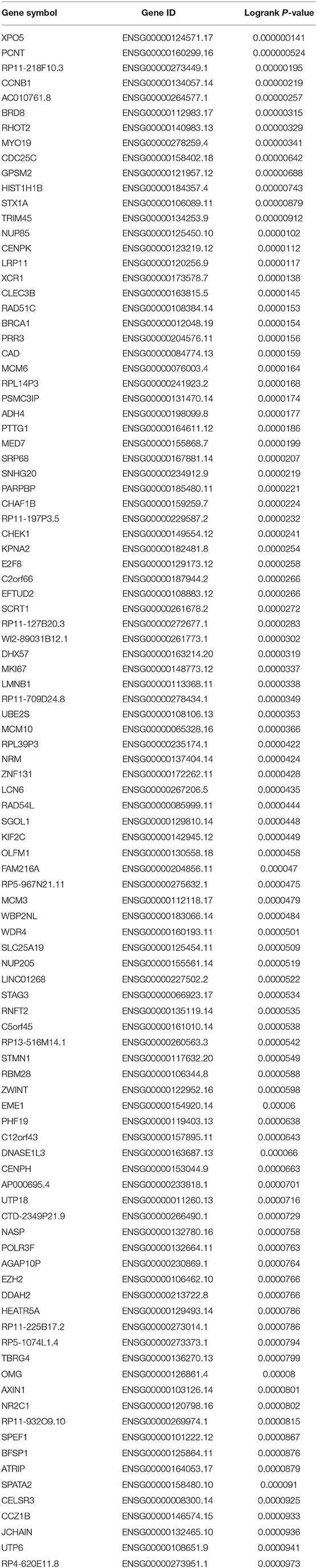
Table 2. The genes most associated with disease-free survival (RFS) of patients with hepatocellular carcinoma determined by GEPIA database.
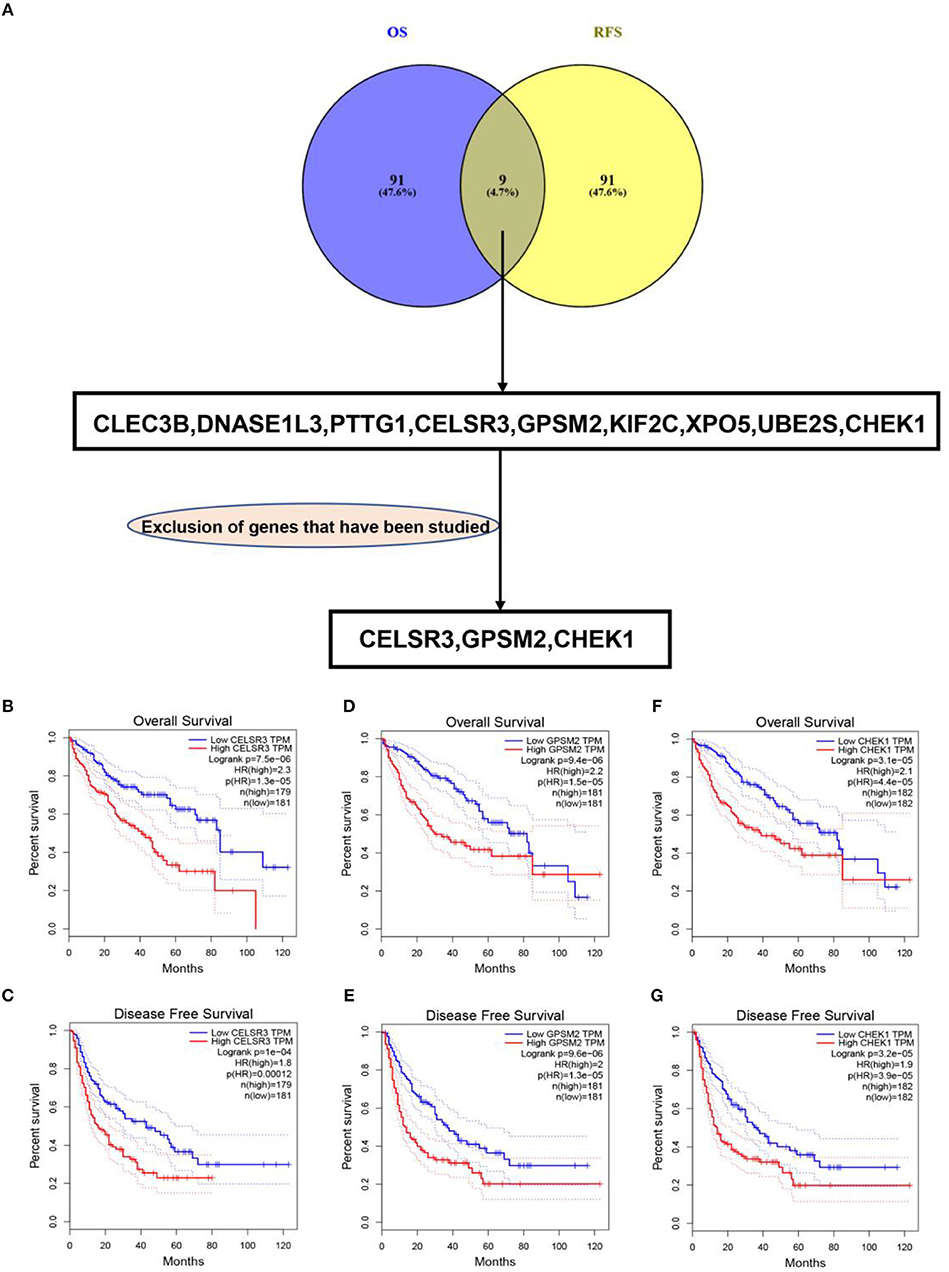
Figure 1. Identification of novel genes significantly associated with prognosis of hepatocellular carcinoma. (A) Identification of CELSR3, GPSM2, and CHEK1 as three novel genes linked to prognosis of hepatocellular carcinoma by intersecting the genes significantly associated with overall survival (OS) and disease-free survival (RFS) and excluding those genes previously studied; high expression of CELSR3 indicated poor OS (B) and RFS (C) of hepatocellular carcinoma; high expression of GPSM2 indicated poor OS (D) and RFS (E) of hepatocellular carcinoma; high expression of CHEK1 indicated poor OS (F) and RFS (G) of hepatocellular carcinoma. Logrank P < 0.05 was considered as statistically significant.
CELSR3, GPSM2, and CHEK1 Were Upregulated in Hepatocellular Carcinoma
Next, we intended to determine expression levels of three novel prognosis-associated genes in hepatocellular carcinoma by bioinformatic analysis and experimental validation. Firstly, we detected their expression in TCGA hepatocellular carcinoma tissues and normal tissues using UALCAN database. CELSR3, GPSM2, and CHEK1 were significantly upregulated in tumor samples compared with normal samples as shown in Figures 2A–C, respectively. Next, Oncomine database was further introduced to analyze CELSR3, GPSM2, and CHEK1 expression in hepatocellular carcinoma. The datasets met the thresholds of P < 0.05 and |fold change| > 1.5 were included for conducting meta-analysis. As presented in Figures 2D–F, CELSR3, GPSM2, and CHEK1 expression were markedly higher in hepatocellular carcinoma than that in normal controls. Protein expression levels of CELSR3, GPSM2, and CHEK1 were evaluated using HumanProteinAtlas database. Figures S1A,B suggested that both CELSR3 and GPSM2 protein expression levels were increased in tumor tissue compared to normal tissue. However, CHEK1 protein value was not included in HumanProteinAtlas database. Subsequently, using qRT-PCR, we found that expression levels of CELSR3 (Figure 2G), GPSM2 (Figure 2H), and CHEK1 (Figure 2I) were obviously increased in two hepatocellular carcinoma cell lines (hepG2 and LM3) when compared with normal hepatic cell line (HL7702). Moreover, compared to normal hepatic tissues, CELSR3, GPSM2, and CHEK1 expression levels were significantly upregulated in collected hepatocellular carcinoma clinical tissues as shown in Figures 2J–L, respectively. All these findings demonstrate that CELSR3, GPSM2, and CHEK1 were upregulated in hepatocellular carcinoma and linked to prognosis of patients with hepatocellular carcinoma.
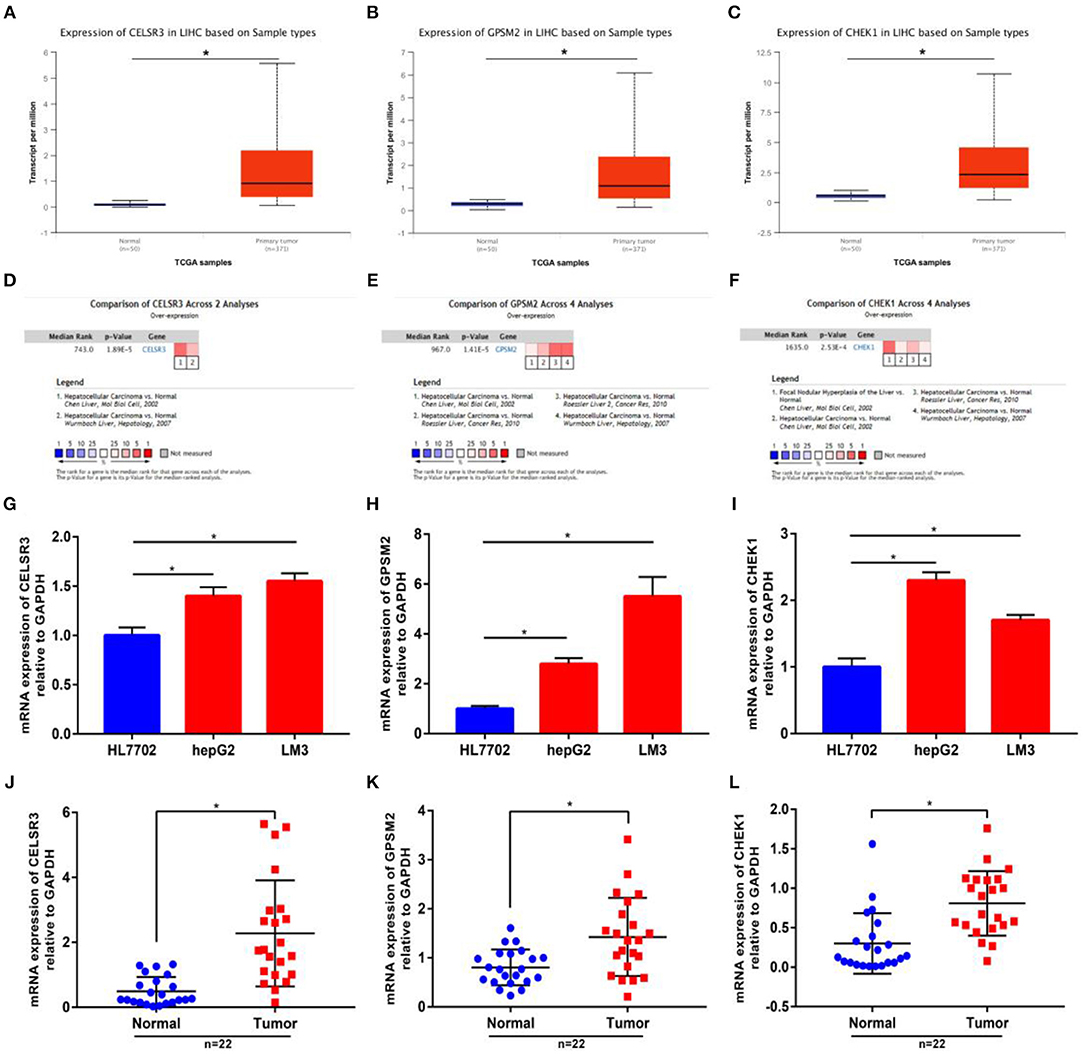
Figure 2. The mRNA expression levels of CELSR3, GPSM2, and CHEK1 in hepatocellular carcinoma. mRNA expression of CELSR3 (A), GPSM2 (B), and CHEK1 (C) were significantly increased in tumor tissues compared to normal controls determined by UALCAN database; mRNA expression of CELSR3 (D), GPSM2 (E), and CHEK1 (F) were significantly increased in tumor tissues compared to normal controls determined by Oncomine database. CELSR3 (G), GPSM2 (H), and CHEK1 (I) were markedly upregulated in hepG2 and LM3 when compared with HL7702; mRNA expression of CELSR3 (J), GPSM2 (K), and CHEK1 (L) were obviously higher in tumor tissues than that in adjacent normal tissues; normalization was done relative to GAPDH. *P < 0.05.
Prediction and Validation of Potential miRNAs Binding to CELSR3, GPSM2, and CHEK1
Next, we predicted upstream regulatory miRNAs of CELSR3, GPSM2, and CHEK1 through a comprehensive miRNA study-associated database, miRNet. A total of 156 mRNA-miRNA pairs, including 71 CELSR3-miRNA pairs, 52 GPSM2-miRNA pairs and 33 CHEK1-miRNA pairs, were acquired. For better visualization, mRNA-miRNA interactive network was constructed using Cytoscape software as presented in Figure 3. According to the classic action mechanism of miRNA in negative regulation of gene expression, there should be inverse expression relationship between the predicted mRNA-miRNA interactions. Thus, we employed starBase database to perform expression correlation analysis for these mRNA-miRNA interactions in hepatocellular carcinoma. The analytic results were shown in Table S2. Those mRNA-miRNA pairs with R < −0.1 and P < 0.05 were considered as significant interactions. Among the 156 interactions, only 8 mRNA-miRNA pairs, including CELSR3-hsa-mir-30a-5p, CELSR3-hsa-mir-4646-3p, CHEK1-hsa-mir-195-5p, CHEK1-hsa-mir-193b-3p, CHEK1-hsa-mir-497-5p, CHEK1-hsa-mir-139-3p, GPSM2-hsa-mir-122-5p, and GPSM2-hsa-mir-378a-5p, were identified as significant interactions (Figure 4). Theoretically, miRNAs that potentially bind to oncogenic CELSR3, GPSM2, and CHEK1 should be downregulated in hepatocellular carcinoma and display favorable prognostic roles. The expression levels of these potential miRNAs and their prognostic roles in hepatocellular carcinoma were determined using starBase database and Kaplan-Meier plotter database, respectively. By combination of expression analysis and survival analysis, hsa-mir-195-5p, hsa-mir-497-5p, hsa-mir-139-3p, and hsa-mir-122-5p were the potential miRNAs in hepatocellular carcinoma (Figures 5A–H). Subsequently, we further determined expression change of target gene (CHEK1 or GPSM2) after overexpression of hsa-mir-195-5p, hsa-mir-497-5p, hsa-mir-139-3p, or hsa-mir-122-5p in hepG2 and LM3 cell lines. Figures 5I,J showed a significant reduction of CHEK1 expression after overexpression of hsa-mir-195-5p and hsa-mir-497-5p; Figure 5K revealed that upregulation of hsa-mir-139-3p did not influence CHEK1 expression; Figure 5L demonstrated that GPSM2 expression was significantly downregulated after overexpression of hsa-mir-122-5p. All these results together indicate that CHEK1-hsa-mir-195-5p/hsa-mir-497-5p and GPSM2-hsa-mir-122-5p may be key pathways in mediating progression of hepatocellular carcinoma and that link to patients' prognosis.
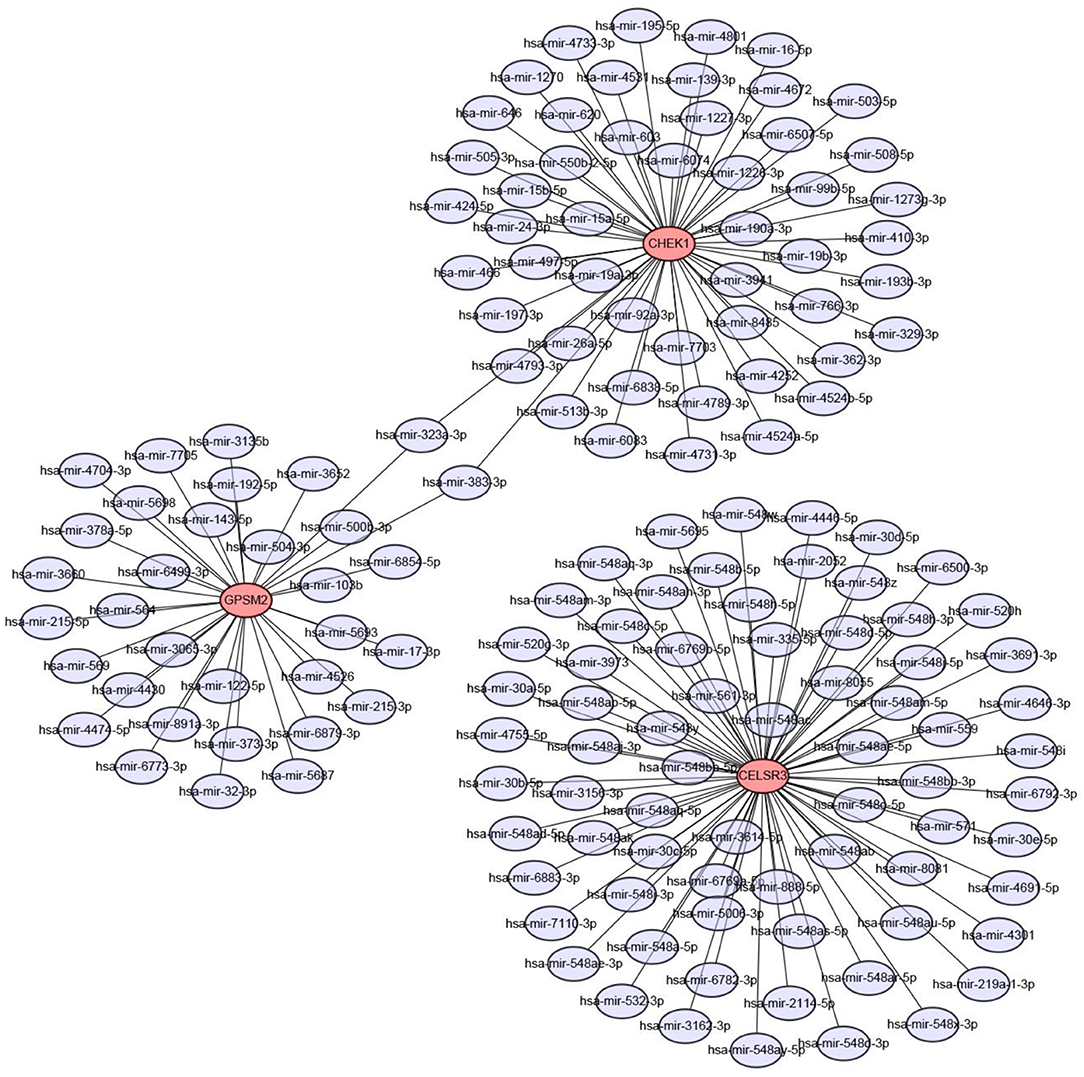
Figure 3. Construction of CELSR3/GPSM2/CHEK1-miRNA network by miRNet database and Cytoscape software.
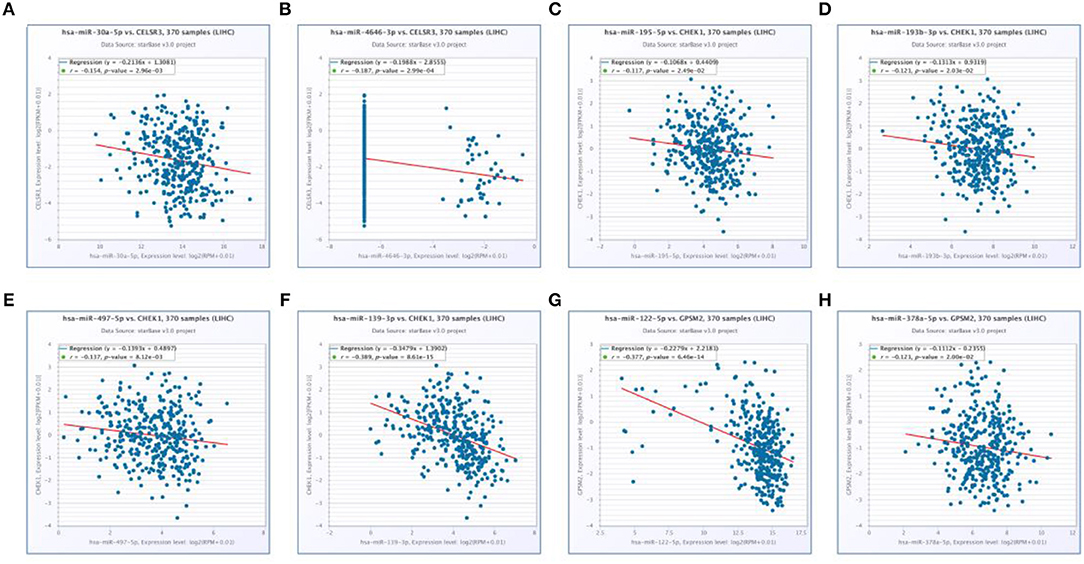
Figure 4. Significantly correlated mRNA-miRNA pairs determined by starBase database. (A) Expression of hsa-mir-30a-5p was negatively associated with CELSR3 expression; (B) expression of hsa-mir-4646-3p was negatively associated with CELSR3 expression; (C) expression of hsa-mir-195-5p was negatively associated with CHEK1 expression; (D) expression of hsa-mir-193b-3p was negatively associated with CHEK1 expression; (E) expression of hsa-mir-497-5p was negatively associated with CHEK1 expression; (F) expression of hsa-mir-139-3p was negatively associated with CHEK1 expression; (G) expression of hsa-mir-122-5p was negatively associated with GPSM2 expression; (H) expression of hsa-mir-378a-5p was negatively associated with GPSM2 expression.
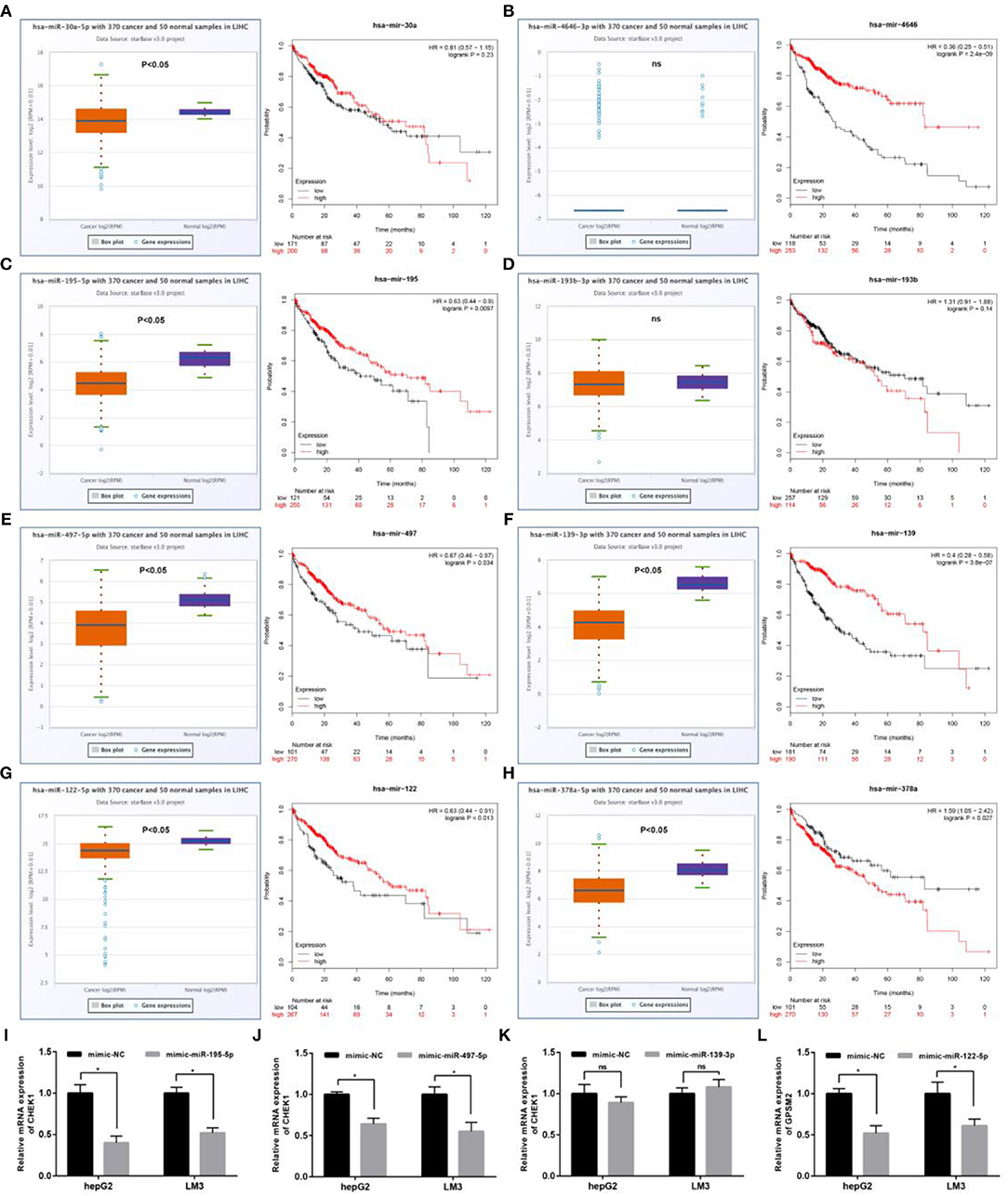
Figure 5. Identification of the most potential miRNAs associated with prognosis of hepatocellular carcinoma. Expression and prognostic value of hsa-mir-30a-5p (A), hsa-mir-4646-3p (B), hsa-mir-195-5p (C), hsa-mir-193b-3p (D), hsa-mir-497-5p (E), hsa-mir-139-3p (F), hsa-mir-122-5p (G), and hsa-mir-378a-5p (H) in hepatocellular carcinoma; (I) after overexpression of hsa-mir-195-5p, mRNA expression of CHEK1 was significantly decreased in hepG2 and LM3; (J) after overexpression of hsa-mir-497-5p, mRNA expression of CHEK1 was significantly decreased in hepG2 and LM3; (K) after overexpression of hsa-mir-139-3p, no significant downregulation of CHEK1 mRNA expression was observed in hepG2 and LM3; (L) after overexpression of hsa-mir-122-5p, mRNA expression of GPSM2 was significantly decreased in hepG2 and LM3. *P < 0.05.
Prediction and Validation of Key lncRNAs Binding to Potential miRNAs
Previous studies have suggested that lncRNAs can bind to miRNA, and mediate regulation of target gene expression and play biological roles (35, 36). Thus, two databases, miRNet and starBase, were used to predict potential lncRNAs that may bind to hsa-mir-195-5p, hsa-mir-497-5p and hsa-mir-122-5p. One hundred and forty five and Two hundred and eighty four lncRNAs were predicted to target hsa-mir-195-5p by miRNet and starBase, respectively; 146 and 284 lncRNAs were predicted to target hsa-mir-497-5p by miRNet and starBase, respectively; 21 and 92 lncRNAs were predicted to target hsa-mir-122-5p by miRNet and starBase, respectively (Data were not shown). As shown in Figures 6A–C, 33, 33, and 6 lncRNAs binding to hsa-mir-195-5p, hsa-mir-497-5p, and hsa-mir-122-5p were commonly appeared in both miRNet and starBase databases. These lncRNAs were selected for subsequent analysis. For better visualization, the miRNA-lncRNA regulatory network was established by Cytoscape software (Figure 6D). Based on ceRNA hypothesis, lncRNAs targeting to hsa-mir-195-5p, hsa-mir-497-5p, and hsa-mir-122-5p should be oncogenic lncRNAs in hepatocellular carcinoma. By combination of expression analysis and survival analysis (Figure 7A), we found that nine lncRNAs, including SNHG1 (Figure 7B), SNHG12 (Figure 7C), LINC00511 (Figure 7D), HCG18 (Figure 7E), FGD5-AS1 (Figure 7F), CERS6-AS1 (Figure 7G), NUTM2A-AS1 (Figure 7H), SNHG16 (Figure 7I), and ASB16-AS1 (Figure 7J), were significantly upregulated in hepatocellular carcinoma, and their upregulation linked to poor prognosis of patients with hepatocellular carcinoma. The current findings support that SNHG1, SNHG12, LINC00511, HCG18, FGD5-AS1, CERS6-AS1, NUTM2A-AS1, SNHG16, and ASB16-AS1, upregulated and linked to poor prognosis in hepatocellular carcinoma, might be the most potential lncRNAs that bind to previous identified miRNAs, hsa-mir-195-5p, hsa-mir-497-5p, and hsa-mir-122-5p.
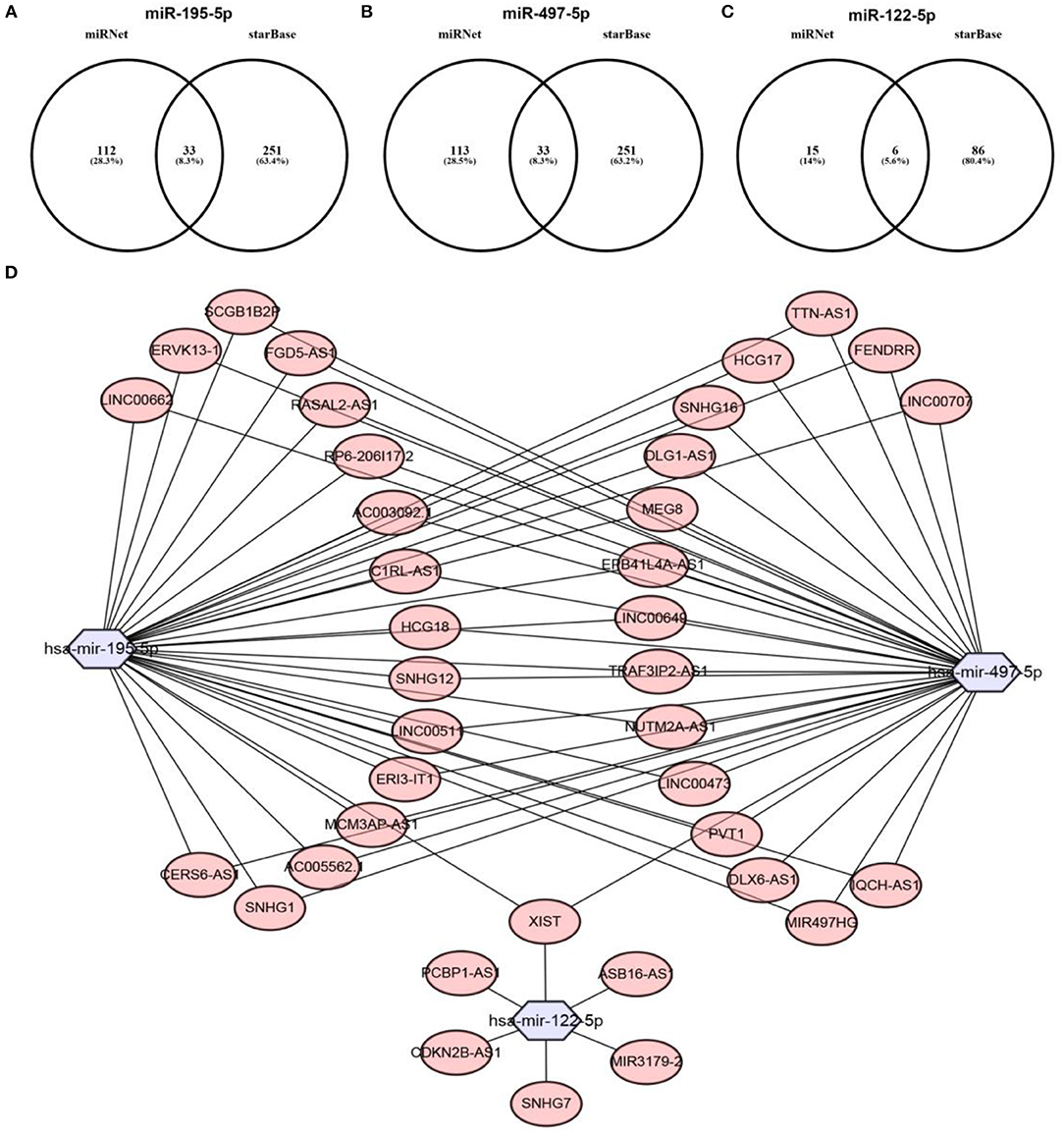
Figure 6. Prediction of upstream lncRNAs potentially binding to hsa-mir-195-5p, hsa-mir-497-5p, and hsa-mir-122-5p. (A) Potential lncRNAs of hsa-mir-195-5p predicted by miRNet and starBase databases; (B) potential lncRNAs of hsa-mir-497-5p predicted by miRNet and starBase databases; (C) potential lncRNAs of hsa-mir-122-5p predicted by miRNet and starBase databases; (D) establishment of miRNA-lncRNA network using Cytoscape software.
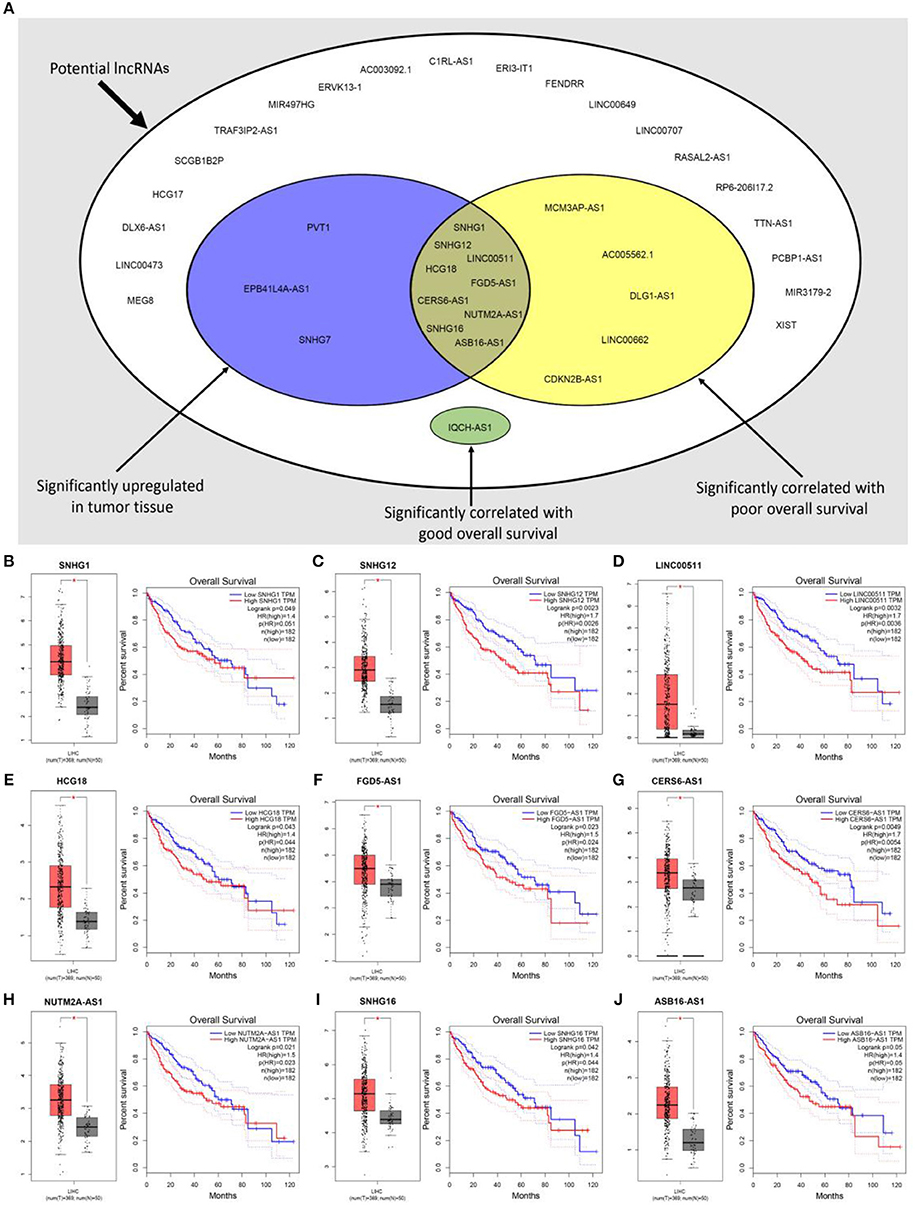
Figure 7. Expression analysis and survival analysis of potential lncRNAs in hepatocellular carcinoma. (A) SNHG1, SNHG12, LINC00511, HCG18, FGD5-AS1, CERS6-AS1, NUTM2A-AS1, SNHG16, and ASB16-AS1 were identified as key lncRNAs in hepatocellular carcinoma by combination of their expression levels and prognostic values; (B) SNHG1 was significantly upregulated in tumor tissues and linked to poor prognosis of hepatocellular carcinoma; (C) SNHG12 was significantly upregulated in tumor tissues and linked to poor prognosis of hepatocellular carcinoma; (D) LINC00511 was significantly upregulated in tumor tissues and linked to poor prognosis of hepatocellular carcinoma; (E) HCG18 was significantly upregulated in tumor tissues and linked to poor prognosis of hepatocellular carcinoma; (F) FGD5-AS1 was significantly upregulated in tumor tissues and linked to poor prognosis of hepatocellular carcinoma; (G) CERS6-AS1 was significantly upregulated in tumor tissues and linked to poor prognosis of hepatocellular carcinoma; (H) NUTM2A-AS1 was significantly upregulated in tumor tissues and linked to poor prognosis of hepatocellular carcinoma; (I) SNHG16 was significantly upregulated in tumor tissues and linked to poor prognosis of hepatocellular carcinoma; (J) ASB16-AS1 was significantly upregulated in tumor tissues and linked to poor prognosis of hepatocellular carcinoma. *P < 0.05.
Prediction and Validation of Upstream miRNA-lncRNA Network of “Known” mRNA in Hepatocellular Carcinoma
Next, based on ceRNA mechanism, we further constructed a six “known” genes-miRNA-lncRNA network in hepatocellular carcinoma. Firstly, we predicted upstream miRNAs of CLEC3B, DNASE1L3, PTTG1, KIF2C, XPO5, and UBE2S using miRNet. As shown in Table S3, a total of 165 miRNA-mRNA pairs were predicted. Then, we performed expression correlation analysis for the 165 miRNA-mRNA pairs in hepatocellular carcinoma, and found that only 13 pairs presented significantly negative relationship about their expression (Table S3). Expression and survival analyses revealed that 4 miRNAs (hsa-miR-101-3p, hsa-miR-148a-3p, hsa-miR-4524a-3p, and hsa-miR-122-5p) were significantly downregulated in hepatocellular carcinoma and correlated with favorable prognosis (Figures 8A–D). Next, we predicted the upstream lncRNAs of the 4 miRNAs through miRNet and starBase databases. As presented in Figures 8E–G, 7, 16, and 5 lncRNAs were identified to potentially bind to hsa-miR-101-3p, hsa-miR-148a-3p, and hsa-miR-122-5p, respectively. No potential lncRNAs of hsa-miR-4524a-3p were found. Expression and survival analyses demonstrated that, among these predicted lncRNAs, SNHG1 (Figure 7B), HCG18 (Figure 7E), NUTM2A-AS1 (Figure 7H) and ASB16-AS1 (Figure 7J), and SNHG6 (Figures 8H,I) were markedly upregulated in hepatocellular carcinoma and linked to poor prognosis.
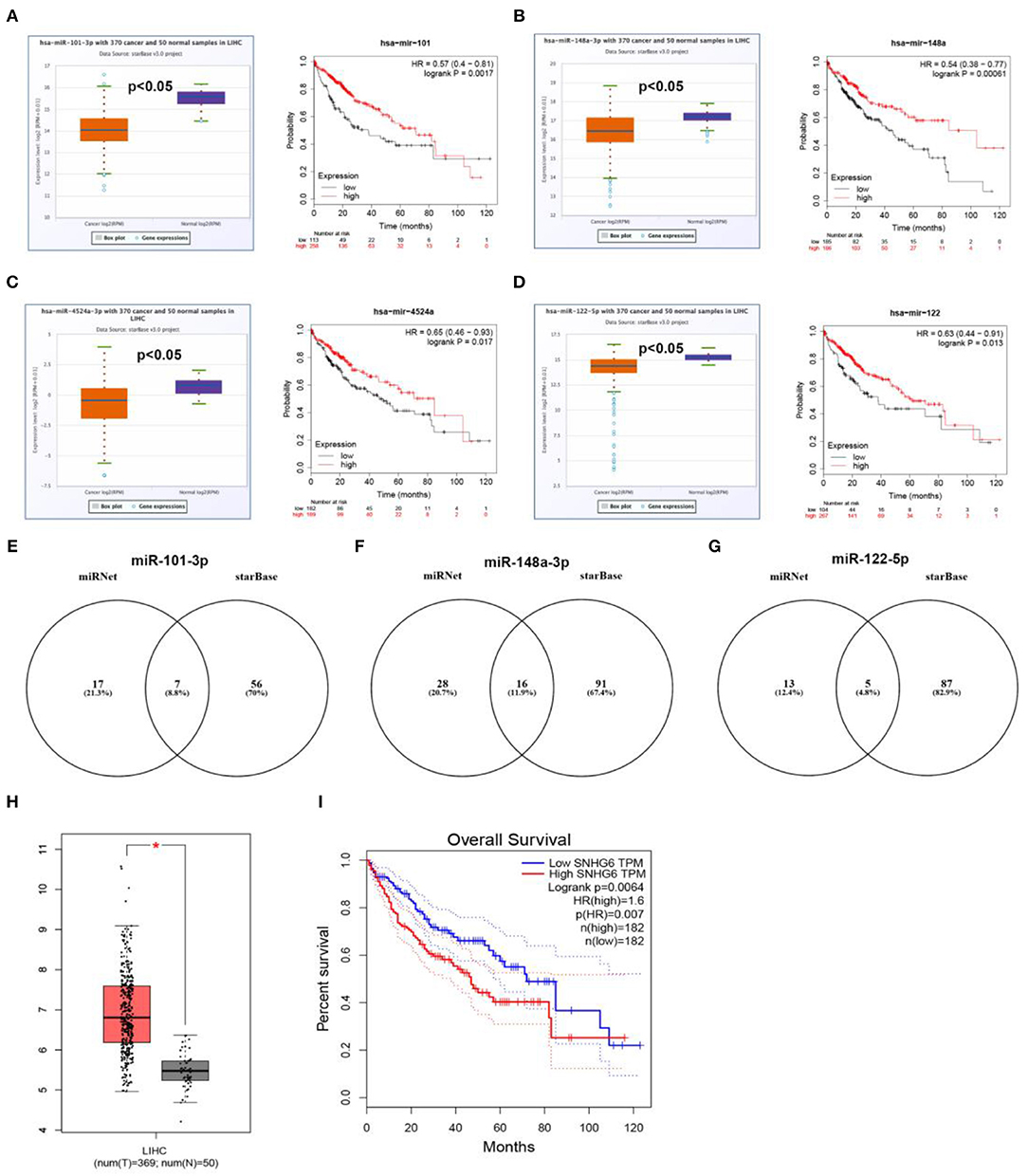
Figure 8. Prediction and validation of upstream miRNAs and lncRNAs of six “known” genes. Expression and prognostic value of hsa-mir-101-3p (A), hsa-mir-148a-3p (B), hsa-mir-4524a-3p (C), hsa-mir-122-5p (D). Potential lncRNAs of hsa-mir-101-3p (E), hsa-mir-148a-3p (F), hsa-mir-122-5p (G), predicted by miRNet and starBase. (H) Expression of SNHG6 in hepatocellular carcinoma analyzed by GEPIA. (I) The prognostic value of SNHG6 in hepatocellular carcinoma assessed by GEPIA database.
Establishment of Key mRNA-miRNA-lncRNA Triple ceRNA Network in Hepatocellular Carcinoma
10 potential lncRNAs (SNHG1, SNHG12, LINC00511, HCG18, FGD5-AS1, CERS6-AS1, NUTM2A-AS1, SNHG16, ASB16-AS1, SNHG6) together with 5 potential miRNAs (hsa-miR-195-5p, hsa-miR-497-5p, hsa-miR-122-5p, hsa-miR-101-3p, and hsa-miR-148a-3p) made up a miRNA-lncRNA sub-network. After performing expression correlation analysis, we discovered that 14 of 21 interactions (hsa-miR-195-5p-SNHG1, hsa-miR-195-5p-LINC00511, hsa-miR-195-5p-HCG18, hsa-miR-195-5p-NUTM2A-AS1, hsa-miR-195-5p-SNHG16, hsa-miR-497-5p-SNHG1, hsa-miR-497-5p-HCG18, hsa-miR-497-5p-NUTM2A-AS1, hsa-miR-497-5p-SNHG16, hsa-miR-122-5p-ASB16-AS1, hsa-miR-101-3p-SNHG1, hsa-miR-101-3p-SNHG6, hsa-miR-148a-3p-HCG18, and hsa-miR-148a-3p-NUTM2A-AS1) possessed significant negative expression relationships (Table 3). The 7 lncRNAs (SNHG1, LINC00511, HCG18, NUTM2A-AS1, SNHG16, ASB16-AS1, and SNHG6) may potentially modulate CHEK1, GPSM2, XPO5, and KIF2C by competitively binding to shared miRNAs (hsa-miR-195-5p, hsa-miR-497-5p, hsa-miR-122-5p, hsa-miR-101-3p, and has-miR-148a-3p). According to ceRNA hypothesis, there should be positive association between mRNA expression and lncRNA expression. Two databases, starBase and GEPIA, were employed to analyze expression correlation of 11 mRNA-lncRNA pairs (CHEK1-SNHG1, CHEK1-LINC00511, CHEK1-HCG18, CHEK1-NUTM2A-AS1, CHEK1-SNHG16, and GPSM2-ASB16-AS1, XPO5-ASB16-AS1, KIF2C-SNHG1, KIF2C-SNHG6, KIF2C-HCG18, and KIF2C-NUTM2A-AS1). Notably, as presented in Figure 9, Figure S2, significant positive expression associations of all these mRNA-lncRNA pairs were observed in both starBase and miRNet databases. By integration of results from in silico analysis and experimental validation, we established a key mRNA-miRNA-lncRNA triple regulatory network associated with prognosis of hepatocellular carcinoma (Figure 10). Finally, we wanted to ascertain if these RNAs in the established mRNA-miRNA-lncRNA triple regulatory network possessed diagnostic roles in hepatocellular carcinoma. As shown in Figure 11, each RNA, including 4 mRNAs (CHEK1, GPSM2, KIF2C, XPO5), 5 miRNAs (hsa-miR-195-5p, hsa-miR-497-5p, hsa-miR-122-5p, hsa-miR-101-3p, hsa-miR-148a-3p) and 7 lncRNAs (SNHG1, HCG18, NUTM2A-AS1, SNHG16, LINC00511, ASB16-AS1, SNHG6), exhibited high diagnostic value for distinguishing hepatocellular carcinoma samples from normal samples.
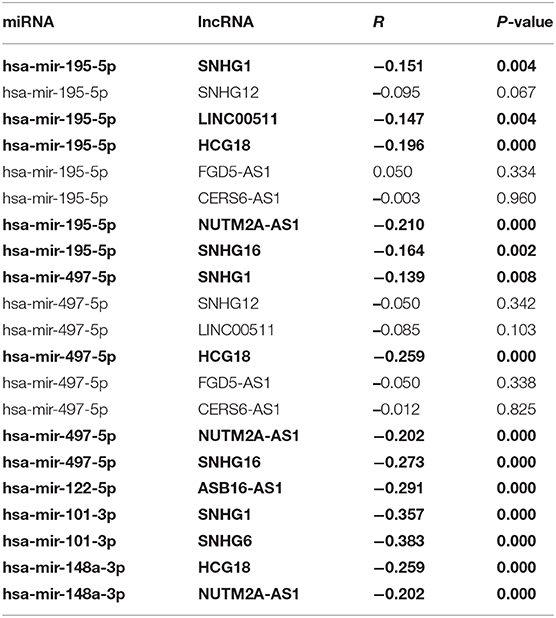
Table 3. The correlation between potential miRNA-lncRNA pairs identified by starBase (The pairs conformed to the ceRNA hypothesis are marked with Bold type).
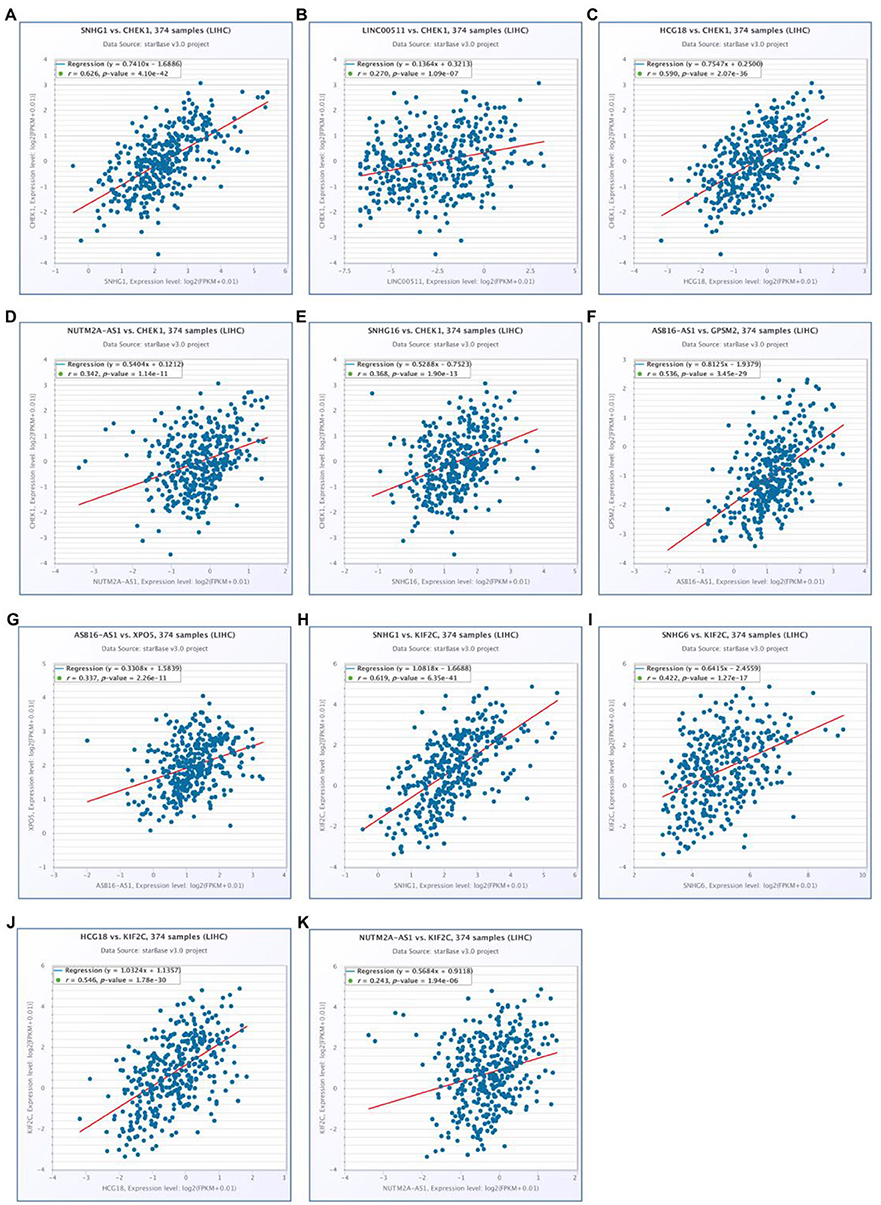
Figure 9. Correlation analysis of potential mRNA-lncRNA pairs in hepatocellular carcinoma determined by starBase. (A) SNHG1 expression was significantly positively correlated with CHEK1 expression in hepatocellular carcinoma; (B) LINC00511 expression was significantly positively correlated with CHEK1 expression in hepatocellular carcinoma; (C) HCG18 expression was significantly positively correlated with CHEK1 expression in hepatocellular carcinoma; (D) NUTM2A-AS1 expression was significantly positively correlated with CHEK1 expression in hepatocellular carcinoma; (E) SNHG16 expression was significantly positively correlated with CHEK1 expression in hepatocellular carcinoma; (F) ASB16-AS1 expression was significantly positively correlated with GPSM2 expression in hepatocellular carcinoma; (G) ASB16-AS1 expression was significantly positively correlated with XPO5 expression in hepatocellular carcinoma; (H) SNHG1 expression was significantly positively correlated with KIF2C expression in hepatocellular carcinoma; (I) SNHG6 expression was significantly positively correlated with KIF2C expression in hepatocellular carcinoma; (J) HCG18 expression was significantly positively correlated with KIF2C expression in hepatocellular carcinoma; (K) NUTM2A-AS1 expression was significantly positively correlated with KIF2C expression in hepatocellular carcinoma.
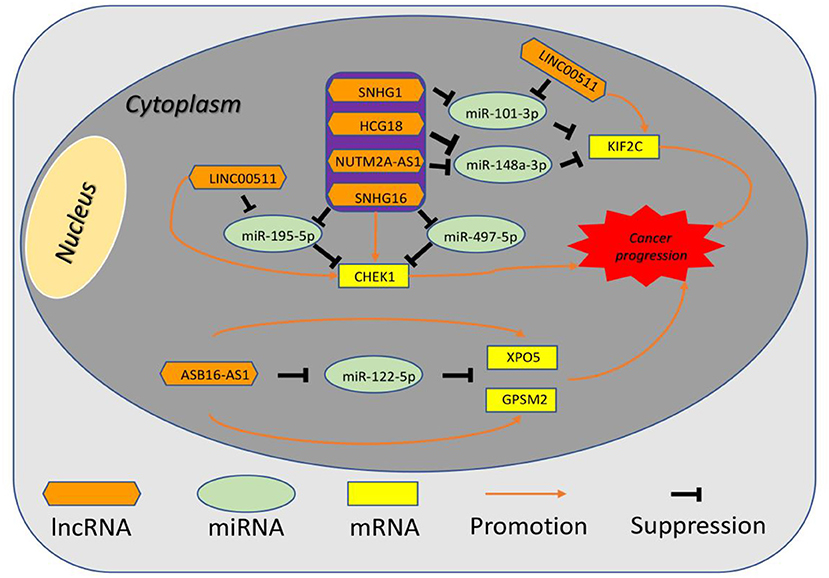
Figure 10. The established mRNA-miRNA-lncRNA competing endogenous RNA (ceRNA) triple network associated with progression and prognosis of hepatocellular carcinoma.
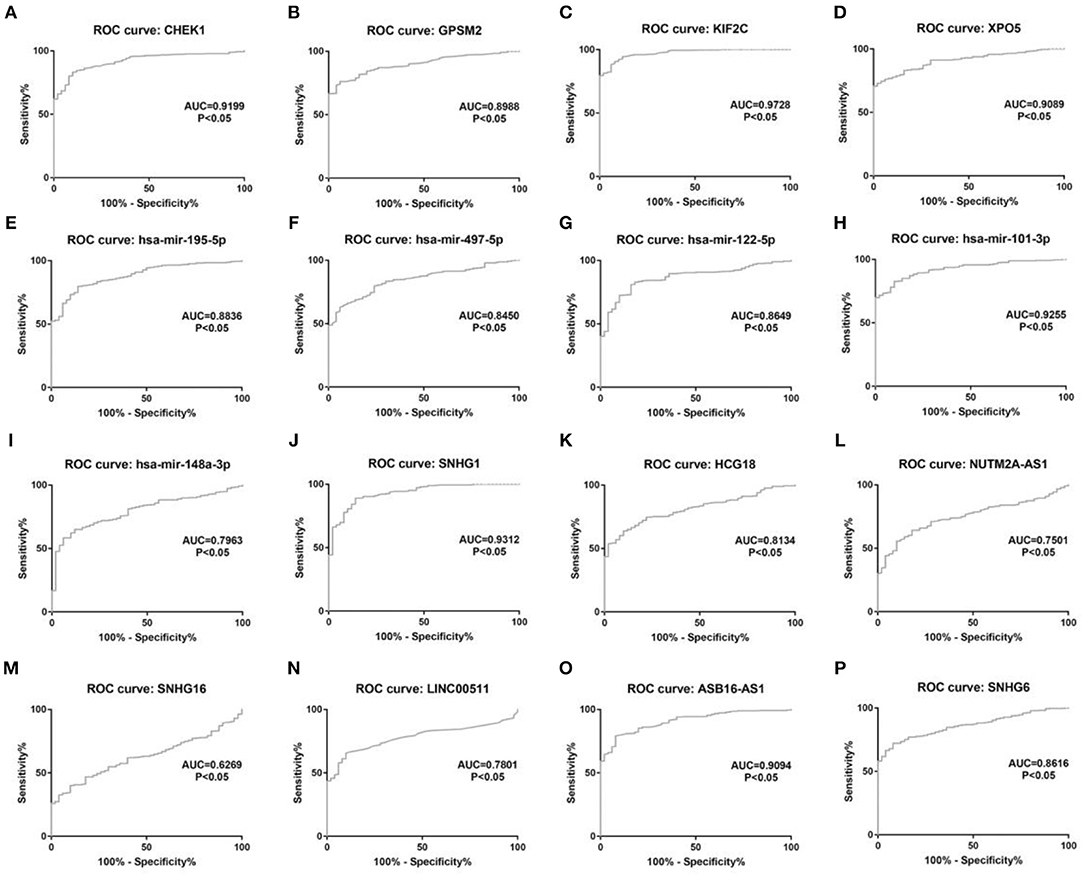
Figure 11. The diagnostic values of 16 potential molecules in hepatocellular carcinoma. (A) CHEK1 ROC analysis in hepatocellular carcinoma; (B) GPSM2 ROC analysis in hepatocellular carcinoma; (C) KIF2C ROC analysis in hepatocellular carcinoma; (D) XPO5 ROC analysis in hepatocellular carcinoma; (E) hsa-mir-195-5p ROC analysis in hepatocellular carcinoma; (F) hsa-mir-497-5p ROC analysis in hepatocellular carcinoma; (G) hsa-mir-122-5p ROC analysis in hepatocellular carcinoma; (H) hsa-mir-101-3p ROC analysis in hepatocellular carcinoma; (I) hsa-mir-148a-5p ROC analysis in hepatocellular carcinoma; (J) SNHG1 ROC analysis in hepatocellular carcinoma; (K) HCG18 ROC analysis in hepatocellular carcinoma; (L) NUTM2A-AS1 ROC analysis in hepatocellular carcinoma; (M) SNHG16 ROC analysis in hepatocellular carcinoma; (N) LINC00511 ROC analysis in hepatocellular carcinoma; (O) ASB16-AS1 ROC analysis in hepatocellular carcinoma. (P) SNHG6 ROC analysis in hepatocellular carcinoma. ROC, receiver operating characteristic. P < 0.05 was considered as statistically significant.
Discussion
Hepatocellular carcinoma is notorious for its poor prognosis and high aggressiveness. Elucidation of molecular mechanisms of hepatocellular carcinoma pathogenesis and identification of promising biomarkers for diagnosis and prognosis of hepatocellular carcinoma are critical for updating therapeutic approaches and improving patients' outcome. ceRNA regulatory network has been reported to participate in initiation and progression of human cancers. To our knowledge, a comprehensive ceRNA regulatory network based on the order model of mRNA-miRNA-lncRNA in hepatocellular carcinoma has not been constructed so far. Therefore, we tried to establish a prognosis/diagnosis-associated mRNA-miRNA-lncRNA ceRNA triple sub-network in hepatocellular carcinoma. By performing survival (OS and RFS) analysis using TCGA hepatocellular carcinoma data, nine genes including three novel genes (CELSR3, GPSM2 and CHEK1) and six “known” genes [CLEC3B (26), DNASE1L3 (27), PTTG1 (28, 29), KIF2C (30), XPO5 (31, 32) and UBE2S (33, 34)] were identified as prognosis-associated genes in hepatocellular carcinoma. The prognostic values of the three novel genes in hepatocellular carcinoma have not been investigated so far. However, they have been well-documented to function as key oncogenes and biomarkers in various types of cancer. For example, Pan et al. (37) reported that CELSR3 may be a promising prognostic gene in head and neck squamous cell carcinoma; He et al. (38) demonstrated that GPSM2 facilitated tumor growth and metastasis; CHEK1 downregulation suppressed by hsa-mir-195-5p hindered growth and metastasis of non-small cell lung cancer (39). In silico analysis and experimental validation together suggested that the three genes were significantly upregulated in hepatocellular carcinoma. All these findings indicate that high expression of CELSR3, GPSM2, or CHEK1 links to unfavorable prognosis of patients with hepatocellular carcinoma.
As mentioned above, mRNA can cross-talk with lncRNA by competitively binding to shared miRNAs (6). Therefore, potential miRNAs of CELSR3, GPSM2, and CHEK1 and lncRNAs that bind to potential miRNAs were successively predicted. 154 miRNAs of CELSR3, GPSM2, and CHEK1 were first identified using miRNet database. Based on the action mechanism of miRNA on mRNA and putative oncogenic roles of CELSR3, GPSM2, and CHEK1, potential miRNAs should be tumor suppressive miRNAs and should be negatively correlated with CELSR3, GPSM2, or CHEK1. Accordingly, we identified 8 potential pairs (CELSR3-hsa-mir-30a-5p, CELSR3-hsa-mir-4646-3p, CHEK1-hsa-mir-195-5p, CHEK1-hsa-mir-193b-3p, CHEK1-hsa-mir-497-5p, CHEK1-hsa-mir-139-3p, GPSM2-hsa-mir-122-5p, and GPSM2-hsa-mir-378a-5p) by conducting correlation analysis for these mRNA-miRNA interactions in hepatocellular carcinoma. After performing expression analysis, survival analysis and qRT-PCR, three of eight mRNA-miRNA pairs (CHEK1-hsa-mir-195-5p, CHEK1-hsa-mir-497-5p, and GPSM2-hsa-mir-122-5p) were selected for subsequent analysis. Liu et al. (39) suggested that CHEK1 was a direct target of hsa-mir-195-5p in non-small cell lung cancer; Xie et al. (40) confirmed that CHEK1 was inversely regulated by hsa-miR-497-5p in hepatocellular carcinoma. In spite of lacking of evidence that has-mir-122-5p directly binds to CHEK1, the inhibitory effect of hsa-mir-122-5p in multiple human cancers has been widely reported (41–43). Our current findings together with these reports suggest that CHEK1-hsa-mir-195-5p, CHEK1-hsa-mir-497-5p, and GPSM2-hsa-mir-122-5p may be key pathways in pathogenesis of hepatocellular carcinoma. Next, lncRNAs that bind to hsa-mir-195-5p, hsa-mir-497-5p, or hsa-mir-122-5p were predicted by miRNet and starBase databases. According to ceRNA hypothesis, potential lncRNAs of hsa-mir-195-5p, hsa-mir-497-5p, or hsa-mir-122-5p should act as oncogenic lncRNAs in hepatocellular carcinoma. Among all predicted lncRNAs, nine lncRNAs (SNHG1, SNHG12, LINC00511, HCG18, FGD5-AS1, CERS6-AS1, NUTM2A-AS1, SNHG16, and ASB16-AS1) were significantly upregulated in hepatocellular carcinoma and their upregulation linked to poor prognosis of patients with hepatocellular carcinoma. Most of them have been reported to function as oncogenic lncRNAs in hepatocellular carcinoma. For example, SNHG1 was found to promote liver cancer development through inhibiting p53 expression via binding to DNMT1 (44); Lan et al. (45) demonstrated that SNHG12 facilitated tumorigenesis and metastasis by targeting miR-199a/b-5p in hepatocellular carcinoma (45); LINC00511 contributed to cell proliferation and metastasis by modulating miR-424 in hepatocellular carcinoma (46); SNHG16 was also reported to enhance proliferation, migration and invasion of hepatocellular carcinoma (47–49). Besides, a similar mRNA-miRNA-lncRNA analysis for six “known” genes was performed.
By integrating these mRNA-miRNA and miRNA-lncRNA interactions, a potential mRNA-miRNA-lncRNA ceRNA triple sub-network associated with prognosis of hepatocellular carcinoma was constructed. Moreover, ROC curve analysis for these RNAs in the established network revealed that each of them possessed high diagnostic value for hepatocellular carcinoma, further suggesting the key roles of this mRNA-miRNA-lncRNA sub-network in hepatocellular carcinoma. Of course, more corresponding studies need to be launched in the future.
Conclusion
In conclusion, using in silico analysis and experimental validation, we established a comprehensive mRNA-miRNA-lncRNA triple ceRNA network in hepatocellular carcinoma. Each RNA in this network possesses significant expression difference between hepatocellular carcinoma and normal control and has promising diagnostic and prognostic values for patients with hepatocellular carcinoma. However, these findings need to be further confirmed by more basic experiments and larger-scale clinical trials in the future.
Data Availability Statement
The datasets analyzed in this study can be found in the GEPIA, UALCAN, and Oncomine databases.
Ethics Statement
The studies involving human participants were reviewed and approved by the Ethical Committee of First Affiliated Hospital of Zhejiang University School of Medicine. The patients/participants provided their written informed consent to participate in this study.
Author Contributions
JZ designed the study, wrote the manuscript and performed in silico analysis of the data. WL conducted experiments and revised the manuscript. JZ and WL have read and approved the final manuscript.
Conflict of Interest
The authors declare that the research was conducted in the absence of any commercial or financial relationships that could be construed as a potential conflict of interest.
Supplementary Material
The Supplementary Material for this article can be found online at: https://www.frontiersin.org/articles/10.3389/fonc.2020.00340/full#supplementary-material
References
1. Yang Z, Zhou L, Wu LM, Lai MC, Xie HY, Zhang F, et al. Overexpression of long non-coding RNA HOTAIR predicts tumor recurrence in hepatocellular carcinoma patients following liver transplantation. Ann Surg Oncol. (2011) 18:1243–50. doi: 10.1245/s10434-011-1581-y
2. Xiao J, Yu H, Ma Z. LINC00339 promotes growth and invasiveness of hepatocellular carcinoma by the miR-1182/SKA1 pathway. Onco Targets Ther. (2019) 12:4481–8. doi: 10.2147/OTT.S207397
3. Zamor PJ, deLemos AS, Russo MW. Viral hepatitis and hepatocellular carcinoma: etiology and management. J Gastrointest Oncol. (2017) 8:229–42. doi: 10.21037/jgo.2017.03.14
4. Lou W, Liu J, Gao Y, Zhong G, Ding B, Xu L, et al. MicroRNA regulation of liver cancer stem cells. Am J Cancer Res. (2018) 8:1126–41.
5. Zhong JH, Xiang X, Wang YY, Liu X, Qi LN, Luo CP, et al. The lncRNA SNHG16 affects prognosis in hepatocellular carcinoma by regulating p62 expression. J Cell Physiol. (2019) 235:1090–102. doi: 10.1002/jcp.29023
6. Salmena L, Poliseno L, Tay Y, Kats L, Pandolfi PP. A ceRNA hypothesis: the rosetta stone of a hidden RNA language? Cell. (2011) 146:353–8. doi: 10.1016/j.cell.2011.07.014
7. Li X, Dai D, Wang H, Wu B, Wang R. Identification of prognostic signatures associated with long-term overall survival of thyroid cancer patients based on a competing endogenous RNA network. Genomics. (2019) 112:1197–207. doi: 10.1016/j.ygeno.2019.07.005
8. Wang H, Zhang H, Zeng J, Tan Y. ceRNA network analysis reveals prognostic markers for glioblastoma. Oncol Lett. (2019) 17:5545–57. doi: 10.3892/ol.2019.10275
9. Wang W, Lou W, Ding B, Yang B, Lu H, Kong Q, Fan W. A novel mRNA-miRNA-lncRNA competing endogenous RNA triple sub-network associated with prognosis of pancreatic cancer. Aging. (2019) 11:2610–27. doi: 10.18632/aging.101933
10. Tang Z, Li C, Kang B, Gao G, Li C, Zhang Z. GEPIA: a web server for cancer and normal gene expression profiling and interactive analyses. Nucleic Acids Res. (2017) 45:W98–102. doi: 10.1093/nar/gkx247
11. Chandrashekar DS, Bashel B, Balasubramanya SAH, Creighton CJ, Ponce-Rodriguez I, Chakravarthi BVSK, et al. UALCAN: a portal for facilitating tumor subgroup gene expression and survival analyses. Neoplasia. (2017) 19:649–58. doi: 10.1016/j.neo.2017.05.002
12. Rhodes DR, Yu J, Shanker K, Deshpande N, Varambally R, Ghosh D, et al. ONCOMINE: a cancer microarray database and integrated data-mining platform. Neoplasia. (2004) 6:1–6. doi: 10.1016/S1476-5586(04)80047-2
13. Lou W, Ding B, Fan W. High expression of pseudogene PTTG3P indicates a poor prognosis in human breast cancer. Mol Ther Oncolytics. (2019) 14:15–26. doi: 10.1016/j.omto.2019.03.006
14. Uhlen M, Fagerberg L, Hallstrom BM, Lindskog C, Oksvold P, Mardinoglu A, et al. Tissue-based map of the human proteome. Science. (2015) 347:1260419. doi: 10.1126/science.1260419
15. Thul PJ, Akesson L. A subcellular map of the human proteome. (2017) 356:eaal3321. doi: 10.1126/science.aal3321
16. Uhlen M, Zhang C. A pathology atlas of the human cancer transcriptome. (2017) 357:eaan2507. doi: 10.1126/science.aan2507
17. Fan Y, Siklenka K, Arora SK, Ribeiro P, Kimmins S, Xia J. miRNet - dissecting miRNA-target interactions and functional associations through network-based visual analysis. Nucleic Acids Res. (2016) 44:W135–41. doi: 10.1093/nar/gkw288
18. Fan Y, Xia J. miRNet-Functional analysis and visual exploration of miRNA-Target interactions in a network context. Methods Mol Biol. (2018) 1819:215–33. doi: 10.1007/978-1-4939-8618-7_10
19. Li JH, Liu S, Zhou H, Qu LH, Yang JH. starBase v2.0: decoding miRNA-ceRNA, miRNA-ncRNA and protein-RNA interaction networks from large-scale CLIP-Seq data. Nucleic Acids Res. (2014). 42:D92–97. doi: 10.1093/nar/gku004
20. Yang JH, Li JH, Shao P, Zhou H, Chen YQ, Qu LH. starBase: a database for exploring microRNA-mRNA interaction maps from argonaute CLIP-Seq and degradome-Seq data. Nucleic Acids Res. (2011). 39:D202–9. doi: 10.1093/nar/gkq1056
21. Nagy A, Lanczky A, Menyhart O, Gyorffy B. Validation of miRNA prognostic power in hepatocellular carcinoma using expression data of independent datasets. Sci Rep. (2018). 8:9227. doi: 10.1038/s41598-018-27521-y
22. Lou W, Chen J, Ding B, Chen D, Zheng H, Jiang D, et al. Identification of invasion-metastasis-associated microRNAs in hepatocellular carcinoma based on bioinformatic analysis and experimental validation. J Transl Med. (2018) 16:266. doi: 10.1186/s12967-018-1639-8
23. Lou W, Liu J, Ding B, Xu L, Fan W. Identification of chemoresistance-associated miRNAs in breast cancer. Cancer Manag Res. (2018) 10:4747–57. doi: 10.2147/CMAR.S172722
24. Lou W, Liu J, Ding B, Chen D, Xu L, Ding J, et al. Identification of potential miRNA-mRNA regulatory network contributing to pathogenesis of HBV-related HCC. J Transl Med. (2019) 17:7. doi: 10.1186/s12967-018-1761-7
25. Lou W, Liu J, Ding B, Jin L, Xu L, Li X, et al. Five miRNAs-mediated PIEZO2 downregulation, accompanied with activation of Hedgehog signaling pathway, predicts poor prognosis of breast cancer. Aging. (2019) 11:2628–52. doi: 10.18632/aging.101934
26. Zhang X, Wan JX, Ke ZP, Wang F, Chai HX, Liu JQ. TMEM88, CCL14 and CLEC3B as prognostic biomarkers for prognosis and palindromia of human hepatocellular carcinoma. Tumour Biol. (2017) 39:1010428317708900. doi: 10.1177/1010428317708900
27. Xu B, Lv W, Li X, Zhang L, Lin J. Prognostic genes of hepatocellular carcinoma based on gene coexpression network analysis. J Cell Biochem. (2019) 120:11616–23. doi: 10.1002/jcb.28441
28. Kong J, Wang T, Zhang Z, Yang X, Shen S, Wang W. Five core genes related to the progression and prognosis of hepatocellular carcinoma identified by analysis of a coexpression network. DNA Cell Biol. (2019) 38:1564–76. doi: 10.1089/dna.2019.4932
29. Liang M, Chen X, Liu W, Li S, Li C, Jiang L, et al. Role of the pituitary tumor transforming gene 1 in the progression of hepatocellular carcinoma. Cancer Biol Ther. (2011) 11:337–45. doi: 10.4161/cbt.11.3.14102
30. Chen J, Li S, Zhou S, Cao S, Lou Y, Shen H, et al. Kinesin superfamily protein expression and its association with progression and prognosis in hepatocellular carcinoma. J Cancer Res Ther. (2017) 13:651–9. doi: 10.4103/jcrt.JCRT_491_17
31. Chen L, Huang Y. Prognostic roles of the transcriptional expression of exportins in hepatocellular carcinoma. Biosci Rep. (2019). 39:BSR20190827. doi: 10.1042/BSR20190827
32. Wang J, Chen D, Wei H, Huang M, Huang Y, Li Y, et al. Downregulation and tumor-suppressive role of XPO5 in hepatocellular carcinoma. Biosci Rep. (2016) 415:197–205. doi: 10.1007/s11010-016-2692-3
33. Li Q, Zhao Y, Shi X, Fan C. Ube2s expression is elevated in hepatocellular carcinoma and predicts poor prognosis of the patients. Int J Clin Exp Pathol. (2018) 11:781–7.
34. Ma Y, Li K, Li S, Liang B, Liu Q, Mo Z. Prognostic value of ubiquitin-conjugating enzyme E2 S overexpression in hepatocellular carcinoma. Int J Biol Macromol. (2018) 119:225–231. doi: 10.1016/j.ijbiomac.2018.07.136
35. Militello G, Weirick T, John D, Doring C, Dimmeler S, Uchida S. Screening and validation of lncRNAs and circRNAs as miRNA sponges. Brief Bioinform. (2017) 18:780–8. doi: 10.1093/bib/bbw053
36. Song H, Liu Y, Jin X, Liu Y, Yang Y, Li L, et al. Long non-coding RNA LINC01535 promotes cervical cancer progression via targeting the miR-214/EZH2 feedback loop. J Cell Mol Med. (2019) 23:6098–111. doi: 10.1111/jcmm.14476
37. Pan Y, Liu G, Wang D, Li Y. Analysis of lncrna-Mediated ceRNA crosstalk and identification of prognostic signature in head and neck squamous cell carcinoma. Front Pharmacol. (2019) 10:150. doi: 10.3389/fphar.2019.00150
38. He XQ, Zhang YF, Yu JJ, Gan YY, Han NN, Zhang MX, et al. High expression of G-protein signaling modulator 2 in hepatocellular carcinoma facilitates tumor growth and metastasis by activating the PI3K/AKT signaling pathway. Tumour Biol. (2017). 39:1010428317695971. doi: 10.1177/1010428317695971
39. Liu B, Qu J, Xu F, Guo Y, Wang Y, Yu H, Qian B. MiR-195 suppresses non-small cell lung cancer by targeting CHEK1. Oncotarget. (2015) 6:9445–56. doi: 10.18632/oncotarget.3255
40. Xie Y, Wei RR, Huang GL, Zhang MY, Yuan YF, Wang HY. Checkpoint kinase 1 is negatively regulated by miR-497 in hepatocellular carcinoma. Med Oncol. (2014) 31:844. doi: 10.1007/s12032-014-0844-4
41. Pei ZJ, Zhang ZG, Hu AX, Yang F, Gai Y. miR-122-5p inhibits tumor cell proliferation and induces apoptosis by targeting MYC in gastric cancer cells. Pharmazie. (2017) 72:344–7. doi: 10.1691/ph.2017.6404
42. Xu X, Gao F, Wang J, Tao L, Ye J, Ding L, et al. MiR-122-5p inhibits cell migration and invasion in gastric cancer by down-regulating DUSP4. Cancer Biol Ther. (2018) 19:427–35. doi: 10.1080/15384047.2018.1423925
43. Xu Z, Liu G, Zhang M, Zhang Z, Jia Y, Peng L, et al. miR-122-5p inhibits the proliferation, invasion growth of bile duct carcinoma cells by targeting ALDOA. Cell Physiol Biochem. (2018) 48:2596–606. doi: 10.1159/000492702
44. Li SJ, Wang L, Sun ZX, Sun SJ, Gao J, Ma RL. LncRNA SNHG1 promotes liver cancer development through inhibiting p53 expression via binding to DNMT1. Eur Rev Med Pharmacol Sci. (2019) 23:2768–76. doi: 10.26355/eurrev_201904_17550
45. Lan T, Ma W, Hong Z, Wu L, Chen X, Yuan Y. Long non-coding RNA small nucleolar RNA host gene 12 (SNHG12) promotes tumorigenesis and metastasis by targeting miR-199a/b-5p in hepatocellular carcinoma. J Exp Clin Cancer Res. (2017) 36:11. doi: 10.1186/s13046-016-0486-9
46. Wang RP, Jiang J, Jiang T, Wang Y, Chen LX. Increased long noncoding RNA LINC00511 is correlated with poor prognosis and contributes to cell proliferation and metastasis by modulating miR-424 in hepatocellular carcinoma. Eur Rev Med Pharmacol Sci. (2019) 23:3291–301. doi: 10.26355/eurrev_201904_17691
47. Chen H, Li M, Huang P. LncRNA SNHG16 promotes hepatocellular carcinoma proliferation, migration and invasion by regulating miR-186 expression. J Cancer. (2019) 10:3571–81. doi: 10.7150/jca.28428
48. Li W, Xu W, Song JS, Wu T, Wang WX. LncRNA SNHG16 promotes cell proliferation through miR-302a-3p/FGF19 axis in hepatocellular carcinoma. Neoplasma. (2019) 66:397–404. doi: 10.4149/neo_2018_180720N504
Keywords: hepatocellular carcinoma, competing endogenous RNA (ceRNA), diagnosis, prognosis, log non-coding RNA (lncRNA), microRNA (miRNA)
Citation: Zhang J and Lou W (2020) A Key mRNA-miRNA-lncRNA Competing Endogenous RNA Triple Sub-network Linked to Diagnosis and Prognosis of Hepatocellular Carcinoma. Front. Oncol. 10:340. doi: 10.3389/fonc.2020.00340
Received: 19 October 2019; Accepted: 26 February 2020;
Published: 17 March 2020.
Edited by:
Luisa Lanfrancone, European Institute of Oncology (IEO), ItalyReviewed by:
Volkhard Helms, Saarland University, GermanyAlessandra Cataldo, National Cancer Institute Foundation (IRCCS), Italy
Copyright © 2020 Zhang and Lou. This is an open-access article distributed under the terms of the Creative Commons Attribution License (CC BY). The use, distribution or reproduction in other forums is permitted, provided the original author(s) and the copyright owner(s) are credited and that the original publication in this journal is cited, in accordance with accepted academic practice. No use, distribution or reproduction is permitted which does not comply with these terms.
*Correspondence: Junjie Zhang, MzMwNzAyMDkxQHFxLmNvbQ==