- 1College of Pharmaceutical Sciences, Zhejiang University, Hangzhou, China
- 2School of Pharmaceutical Sciences and Collaborative Innovation Center for Brain Science, Chongqing University, Chongqing, China
- 3Key Laboratory of Elemene Class Anti-cancer Chinese Medicine of Zhejiang Province, School of Medicine, Hangzhou Normal University, Hangzhou, China
One of the most challenging puzzles in drug discovery is the identification and characterization of candidate drug of well-balanced profile between efficacy and safety. So far, extensive efforts have been made to evaluate this balance by estimating the quantitative structure–therapeutic relationship and exploring target profile of adverse drug reaction. Particularly, the therapeutic index (TI) has emerged as a key indicator illustrating this delicate balance, and a clinically successful agent requires a sufficient TI suitable for it corresponding indication. However, the TI information are largely unknown for most drugs, and the mechanism underlying the drugs with narrow TI (NTI drugs) is still elusive. In this study, the collective effects of human protein–protein interaction (PPI) network and biological system profile on the drugs' efficacy–safety balance were systematically evaluated. First, a comprehensive literature review of the FDA approved drugs confirmed their NTI status. Second, a popular feature selection algorithm based on artificial intelligence (AI) was adopted to identify key factors differencing the target mechanism between NTI and non-NTI drugs. Finally, this work revealed that the targets of NTI drugs were highly centralized and connected in human PPI network, and the number of similarity proteins and affiliated signaling pathways of the corresponding targets was much higher than those of non-NTI drugs. These findings together with the newly discovered features or feature groups clarified the key factors indicating drug's narrow TI, and could thus provide a novel direction for determining the delicate drug efficacy-safety balance.
Introduction
One of the most challenging puzzles in drug discovery is the identification and characterization of candidate drugs of well-balanced profile between efficacy and safety (Muller and Milton, 2012; Li et al., 2018; Xue et al., 2018b). In other words, apart from extensive effort made to optimize drug affinity and selectivity (Wang et al., 2017a; Zheng et al., 2017), considerable investments should be devoted to detect adverse drug reactions (Huang et al., 2018) and reveal drug likeness (Benet et al., 2016; Yang et al., 2018). So far, the identification of drug toxicities in preclinical or clinical developments has been accelerated by a variety of technological advances (Badders et al., 2018) including biomarker-guided safety assessment (Muller and Dieterle, 2009; Rzepecki et al., 2018), OMICs techniques (Iloro et al., 2013; Fu J. et al., 2018), breakthrough in computing capacity and bioinformatics method (Zhu et al., 2011; Tao et al., 2015; Chen et al., 2016), and so on. To measure the level of correlation between drug maximum efficacy and confined safety in given disorder, the therapeutic index (TI typically considered as the ratio of the highest non-toxic drug exposure to the exposure producing the desired efficacy) has emerged as a key indicator illustrating that delicate balance (Zaykov et al., 2016). The TI is essential for life-threatening diseases (such as cardiovascular and oncological disease) with limited treatment options (Zhu et al., 2008b; Kimmelman and Federico, 2017). Particularly, tiny variation in the dosage of drugs with narrow TI (NTI drugs, TI ≤3) may result in therapeutic failure or serious adverse drug reactions (Tao et al., 2014; Ewer and Ewer, 2015; Zheng et al., 2016), and is only acceptable for the treatment of life-threatening diseases (Yu et al., 2015). Therefore, successful therapeutic agents require sufficient TI (NNTI drugs, TI >3) suitable for it corresponding indication (Abernethy et al., 2011).
However, TI characterization is too complicated to be achieved for many drugs (Yu et al., 2015), and TI is highly susceptible to the subject variations of drug responses (Jiang et al., 2015; Yang et al., 2017). To enhance the determination and interpretation of TI, a variety of in-silico studies have been performed to reveal the mechanism underlying NTI drugs (Muller and Milton, 2012). In particular, the prediction models based on quantitative structure–activity (QSAR), structure–toxicity (QSTR), and structure–index (QSIR) relationship have been constructed to enable early assessment of TI (Zhu H. et al., 2008; Rodgers et al., 2010; Zhu et al., 2012a; Chen et al., 2016; Fu T. et al., 2018). These models are primarily constructed and exert their prediction capacity based on structures of the studied drugs, which thus demonstrate great limitations in coping with TI's vulnerability to the subject variation of drug responses (Jiang et al., 2015). Compared with the approaches based on drug structure, target-based approach turns out to be the one of enhanced effectiveness for characterizing confined toxicity behind the drug efficacy (Muller and Milton, 2012; Huang et al., 2018), since the population variation of drug target is capable of reflecting, to some extent, the subject variations of drug responses (Fujimoto et al., 2014; Jiang et al., 2015). But target-based method is sophisticated due to the involvement of target in complex protein–protein interaction (PPI) network (Rao et al., 2011; Li et al., 2016b; Xu et al., 2016; Wang et al., 2017b) and the necessity of considering target biological system profiles (Zhu F. et al., 2009; Xue et al., 2016).
So far, the PPI network properties (Ragusa et al., 2010; Guo et al., 2018) and biological system profiles (Zheng et al., 2006) have been adopted to analyze the drug likeness of candidate agents. On one hand, the target–protein interaction network has been constructed and the corresponding network features can be calculated for discovering the differential properties indicating disease status (Ragusa et al., 2010) and identifying candidate drug targets for a given indication (Guo et al., 2018; Xue et al., 2018a). On the other hand, the druggability of candidate target is found significantly determined by a variety of biological system profiles, which include the number of target affiliated signaling pathways (Yang et al., 2016), the number of similarity proteins outside target's protein family (Zheng et al., 2006), the number of human tissues distributed by the studied target (Zhu F. et al., 2009), and the differential level of target expression between patient and healthy individual (Ernst et al., 2017; Li et al., 2018). Since the underlying theories of network- and biological system-based approaches are distinct from each other (Guo et al., 2018; Li et al., 2018), it is essential to simultaneously consider these two types of properties for understanding drug likeness. However, these properties have not yet been collectively considered in TI-related studies, and the mechanism underlying drugs' narrow TI is still elusive.
In this study, a comprehensive analysis on the network features and biological system profiles of the primary therapeutic targets of all FDA approved drugs was conducted, and various features differentiating drugs of narrow TI (NTI drugs) from those of sufficient TI (NNTI drugs) were identified. First, due to the limited information of both NTI and NNTI drugs, a systematic literature review was conducted to collect the TI data for all approved drugs. Then, the primary therapeutic targets of these drugs were classified into four groups based on collected TI data. These four target groups include (a) targets of NTI drugs, (b) targets of both NTI and NNTI drugs, (c) targets of drugs without reported TI, and (d) targets of NNTI drugs. Third, a comparative analysis between target group (a) and (d) identified several key features able to differentiate two groups, and further study revealed three feature groups indicating the mechanisms underlying NTI drugs. In summary, these findings together with the newly discovered features or feature groups clarified key factors indicating drug's narrow TI, which gave a new direction for determining the delicate balance between drugs' maximum efficacy and confined safety.
Materials and Methods
Systematic Collection of Drugs and Their Corresponding Targets and TI Data
The TI data of FDA approved drugs were obtained by four steps. First, FDA approved drugs were collected from the official website of FDA (Drugs@FDA), and their corresponding diseases were carefully confirmed. In total, 1,762 drugs were collected. Second, the primary therapeutic targets of these drugs were identified from the TTD database (https://db.idrblab.org/ttd/; Li et al., 2018), and 418 primary therapeutic targets of these 1,762 drugs were discovered (detail information was provided in the following paragraphs). Third, TI data of these drugs were systematically collected by a comprehensive literature review. Particularly, various keyword combinations were searched in PubMed and other academic resources, which included “drug name + therapeutic index,” “drug name + therapeutic window,” “drug name + critical dose,” “drug name + therapeutic ranges,” and “drug name + therapeutic ratio.” As a result, 161 NTI and 29 NNTI drugs confirmed by the clinical evaluations or experiments were identified, which aimed at 60 and 28 human targets, respectively. Supplementary Table S1 provided a full list of 161 NTI and 29 NNTI drugs together with their approved disease indication and corresponding targets. To the best of our knowledge, it is the first comprehensive literature review on the TI data of all drugs approved by FDA and Supplementary Table S1 provided the most completed information of the FDA approved drugs with available TI data. Moreover, the primary therapeutic targets of all FDA approved drugs were classified into four groups based on their TI: (a) 20 targets of NTI drugs, (b) 40 targets of both NTI and NNTI drugs, (c) 339 targets of drugs without reported TI, and (d) 19 targets of NNTI drugs. Moreover, among those drugs listed in Supplementary Table S1, four multi-target drugs were found with NTI data available, which included regorafenib (hepatocellular and colorectal cancer), sorafenib (renal cell and hepatocellular carcinoma), sunitinib (gastrointestinal cancer), and vandetanib (medullary thyroid cancer). All these drugs are multi-kinases inhibitors for the treatment of cancer.
Identification of the Primary Therapeutic Target(S) of FDA Approved Drugs
The primary therapeutic target of each FDA approved drug was strictly determined by considering (1) the experimentally determined potency of drugs against their primary target or targets (Zhu et al., 2010), (2) the observed potency or effects of drugs against disease models (cell lines, ex-vivo, in-vivo models) linking to their primary drug targets (Zhu et al., 2012b), and (3) the observed effect of target knockout, knockdown, transgenetic, RNA interference, antibody or antisense-treated in vivo models (Zhu et al., 2012b). Taking the confirmation of CDK4 as the primary therapeutic target of FDA approved Palbociclib as an example, it was determined by considering: (1) experimentally defined high potency (IC50 = 11 nM) of Palbociclib against CDK4 (Fry et al., 2004), (2) the clearly observed development of multiple tumors by a point mutation (R24C) in the first coding exon of locus encoding CDK4 in the mice models (Sotillo et al., 2001), and (3) Palbociclib-induced G1-G2 arrest and apoptosis in breast tumor cell lines (IC50 <400 nM) and tumor growth reduction in human breast tumor xenograft (Lapenna and Giordano, 2009). In conclusion, only the targets with complete target determination data (including all three types of information above) were defined as the primary therapeutic targets of the corresponding FDA approved drugs.
Deriving the Human PPI Network Properties for Each Studied Target
The human protein–protein interaction (PPI) network analyzed here included 15,554 proteins and 642,304 PPIs, which was constructed using the data provided in STRING (Szklarczyk et al., 2015). In order to ensure the reliability of the analyzed data, only those PPIs with high confidence score (>0.95) were collected for the subsequent analyses (Ghosh et al., 2015; Wang S. et al., 2015). As a result, a sub-network with 8,509 proteins and 40,468 PPIs were generated and adopted for further analyses in this study. Moreover, the network properties for each studied target were generated by the PROFEAT (Zhang et al., 2017a) and the tool NetworkAnalyzer of Cytoscape (Shannon et al., 2003; Thomas and Bonchev, 2010).
In total, 32 network properties were calculated and adopted in subsequent analysis. These properties were popular for analyzing a complex biological network, which included: (1) Average Closeness Centrality: the average number of steps required to reach the studied node from any node in a network (Ma et al., 2016); (2) Average Shortest Path Length: the average length of shortest paths between the studied node and all other ones (Zhang et al., 2014); (3) Betweenness Centrality: the number of times the studied node serving as a linking bridge along shortest path between any two nodes (Zeidán-Chuliá et al., 2015); (4) Bridging Centrality: the product of the bridging coefficient and betweenness centrality (Hwang et al., 2008); (5) Bridging Coefficient: the extent of the studied node lying between any other densely connected nodes in the network (Paladugu et al., 2008); (6) Closeness Centrality Sum: the reciprocal of the sum of the shortest paths between the studied node and all other nodes in the network (Costenbader and ValenteFontanesi, 2003); (7) Clustering Coefficient: the number of the connected pairs between all neighbors of node (Watts and Strogatz, 1998); (8) Current Flow Betweenness: a centrality index measuring the level of information travels along all possible paths within network (Paladugu et al., 2008); (9) Current Flow Closeness: the variant of current flow betweenness (Zhang et al., 2017b); (10) Degree: the number of edges linked to a node (Braeuning, 2013); (11) Degree Centrality: the number of links incident upon a studied node (Batool and Niazi, 2014); (12) Deviation: the variation between sum of node distances and network unipolarity (Zhang et al., 2017a); (13) Distance Deviation: the absolute difference between nodes' distance sum and network's average distance (Rogelj et al., 2013); (14) Distance Sum: the sum of all shortest paths starting from the studied node (Bolser et al., 2003); (15) Eccentric: the absolute difference between nodes' eccentricities and network's average eccentricity (Zhang et al., 2017a); (16) Eccentricity: the maximum non-infinite shortest path length between the studied node and all other nodes in the network (Bolser et al., 2003); (17) Eccentricity Centrality: the largest geodesic distance between the node and any other node (Batool and Niazi, 2014); (18) Eigenvector Centrality: the sum of its neighbors' centrality values (Solá et al., 2013); (19) Harmonic Closeness Centrality: the sum of the reciprocals of the average shortest path lengths of each node in network (Zhang et al., 2017b); (20) Interconnectivity: a connectivity index indicating the quality of the studied nodes being connected together (Emig et al., 2013); (21) Load Centrality: the fraction of all the shortest paths that pass through the studied node (Kivimäki et al., 2016); (22) Neighborhood Connectivity: the average connectivity of all neighbors (Carson and Lu, 2015); (23) Normalized Betweenness: the fraction of network shortest paths that a given protein lies on (Paladugu et al., 2008); (24) Number of Self Loops: the number of edges starting and ending at the same node (Garlaschelli and Loffredo, 2004); (25) Number of Triangles: the number of triangles that include the studied node as a vertex (Rubinov and Sporns, 2010); (26) Page Rank Centrality: an adjustment of Katz by considering the diluted issue (Li et al., 2013); (27) Radiality: the level of reachability of a studied node via various shortest paths within the entire network (Koschützki and Schreiber, 2008); (28) Residual Closeness Centrality: the closeness measured by removing the studied node (Dangalchev, 2006); (29) Scaled Degree: the degree of a studied node relative to the most connected node within the same module (Sormani, 2012); (30) Stress: the number of shortest paths passing through a given node (Shannon et al., 2003); (31) Topological Coefficient: the extent to which a node in network shares interaction partners with other nodes (Zhu M. et al., 2009); (32) Z Score: a connectivity index based on degree distribution of a network (Rubinov and Sporns, 2010).
Assessing the Biological System Profile for Each Studied Target
The biological system profile for each studied target included: (1) the number of target-affiliated and target immediate-downstream signaling pathways in KEGG database (Kanehisa et al., 2017). The target-affiliated pathways were determined by considering that (a) the pathways of the studied target should be life-essential in both patients and healthy people and (b) the studied target should be in the pathway upstream with the capacity of regulating the biological function of the pathways. (2) The number of human tissues each target distributed in, assessed by the TissueDistributionDBs (Kogenaru et al., 2010) and Uniprot (UniProt Consortium, 2018) databases. A target was assumed to distribute in a given tissue if >5% of the total proteins are distributed in that tissue or the target concentration is higher than the average concentration of proteins in that tissue. (3) The number of human similarity proteins of a target outside the corresponding target family for probing off-target collateral effect (Zheng et al., 2006; Zhu F. et al., 2009). This was determined by BLAST similarity screening of human proteome in Uniprot database (UniProt Consortium, 2018) with a cutoff (E-value < 0.005; Song et al., 2006; Singh et al., 2007). (4) The differential expressions of the studied target in the disease-specific tissue between patients and healthy individuals (Li et al., 2018). The relevant data were collected directly from TTD (Li et al., 2018) and calculated based on the human gene expression raw data of Affymetrix U133 Plus 2.0 platform in GEO (Barrett et al., 2013).
Selecting the Differential Features Indicating NTI Drugs by Artificial Intelligence
The artificial intelligence (AI) has been recently proposed as a powerful technique for drug target discovery (Xu and Wang, 2014; Zhu et al., 2018), protein function prediction (Li et al., 2016a; Seo et al., 2018; Yu et al., 2018) and biomarker identification (Li B. et al., 2016; Li et al., 2017) through mimicking the human thinking procedures, learning processes and information extractions, which included the machine learning algorithm (Zhu et al., 2008a; Wang P. et al., 2015), the deep learning method (van der Burgh et al., 2017; Seo et al., 2018), and the cognitive-computing (Krittanawong et al., 2017). As one of the most popular machine learning algorithms, the Boruta algorithm based on wrapper method built around a random forest classifier (Kursa, 2014) was selected and adopted in this study. It is an extension to determine the relevance via comparing the relevance of the real features to that of the random probes (Pan et al., 2018). Since Boruta was constructed by an AI-based technique (machine learning), it was considered to be the most powerful approach with the stability in the variable selection, especially suitable for the low-dimensional dataset among other available strategies (Degenhardt et al., 2017). In this study, the differential features between NTI and NNTI drugs were therefore identified by R package Boruta (Shang et al., 2017). Particularly, human PPI network properties and biological system features of each target were first calculated, and the results of feature selection were then acquired using R package Boruta by setting the p-value < 0.05, maxRuns = 100, and doTrace = 2. In the meantime, the getImp was set to “getImpRfZ,” and the mcAdj and holdHistory were set to “TRUE.”
Results and Discussion
Network Properties and Biological System Profile of NTI and NNTI Drugs
As reported, the human PPI network properties and biological system profile were key factors determining efficacy-safety balance (Zheng et al., 2006; Ragusa et al., 2010; Guo et al., 2018). Network properties were inherent feature of a target in the human PPI network, while biological system profile could reflect both the on-target and off-target pharmacology (Bender et al., 2007; Han et al., 2018; Zhu et al., 2018). Herein, 32 features of human PPI network together with 4 biological system properties were therefore adopted and calculated for further analyses. To the best of our knowledge, these were the most comprehensive sets of features ever applied for TI-related analysis. Table 1 listed the calculated values of ten properties based on the connectivity and adjacency in human PPI network. These connectivity/adjacency-based network properties were designed to describe the level of connectivity among human proteins or the neighborhood features of the studied proteins (Chen et al., 2016). The properties included bridging coefficient, clustering coefficient, degree, degree centrality, interconnectivity, neighbor connectivity, number of triangles, scaled degree, topological coefficient, and Z-score (corresponding definitions were provided in section Materials and Methods). As shown in Table 1, 8 (80.0%) out of 10 properties were significantly different (p-value < 0.05, highlighted by bold font) between the targets of NTI and NNTI drugs, and half of those 10 properties were with the most significant differences (p-value < 0.01, highlighted by bold-underline).
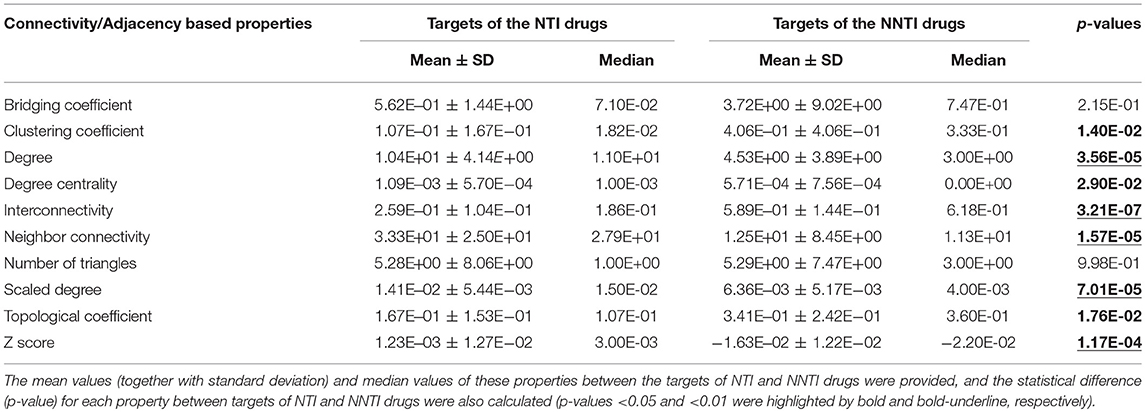
Table 1. The calculated values of 10 properties based on the connectivity and adjacency in the human PPI network.
Similar to the connectivity/adjacency-based network property, the calculated values of 16 properties based on the shortest path length in the human PPI network were provided in Table 2 (corresponding definitions of these properties were provided in section Materials and Methods). As shown in Table 2, all properties were found to be significantly different (p-values < 0.05, in bold font) between the targets of NTI and NNTI drug, and 14 (87.5%) of the 16 properties were with the most significant difference (p-value < 0.01, bold-underline). Moreover, the calculated values of 4 human biological system properties were shown in Table 3 (definition of these properties was given in section Materials and Methods). As reported, these properties were frequently adopted to analyze the druggability of therapeutic targets for not only approved drugs but also the drugs in clinical trial development or withdrawn from market (Li et al., 2018). Herein, two properties were identified as significantly different (p-value < 0.01, bold-underline) between targets of NTI and NNTI drugs, which included the number of pathways affiliated by the targets of the studied drugs and the number of similarity proteins outside target's functional family. One thing needed to be emphasized was that the standard deviation of many properties was even larger than their mean value (such as bridging coefficient, clustering coefficient, and Z-score). These deviations indicated that the corresponding p-value may not be enough to measure the difference between the targets of NTI and NNTI drug. Moreover, any of the individual feature (p-value < 0.05 shown in Tables 1–3) could not be used to satisfactorily differentiate the targets of NTI drugs from that of the NNTI ones. Thus, this finding inspired us to discover the differential features using more advanced computational algorithm and collectively considering multiple properties.
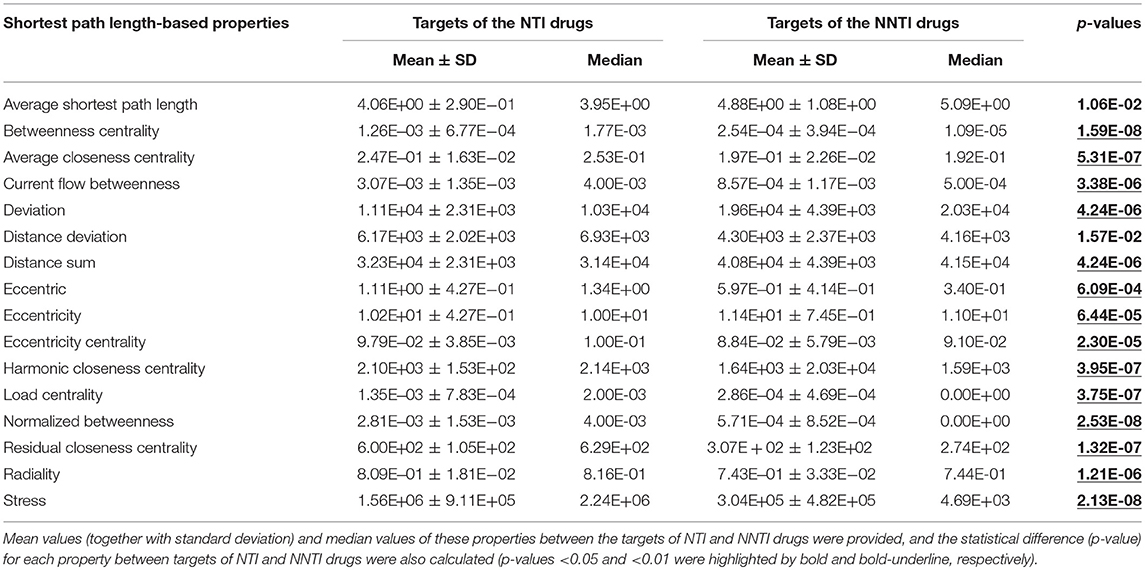
Table 2. The calculated values of 16 properties based on the shortest path length in human PPI network.
Discovering the Key Features of NTI Drug Targets by Artificial Intelligence
Based on the in-depth investigation of 36 properties in Tables 1–3, several properties were found to be not fully independent or even duplicate in their descriptions (like degree vs. scaled degree). In this study, all 36 properties were systematically reviewed, and 19 of these 36 were identified to be substantially overlapped with some other properties (Table 4). Since there was significant dependence among the 19 properties, the use of all 36 properties for statistical feature selection may introduce strong biases. Thus, the 19 properties were grouped based on their innate mutual dependence. As shown in Table 4, five property groups were generated by considering equation and description of these 19 properties, and each group was named by the first property (ordered alphabetically) in the corresponding group. As a result, these five groups included: the average closeness centrality, average shortest path length, betweenness centrality, degree, eccentricity. To minimize the possible bias induced by the innate mutual dependence among properties, only these five properties were considered in subsequent feature selection analysis, instead of investigating all 19 properties. Taking the remaining 17 relatively independent properties into consideration, 22 properties in total of each target were selected for subsequent feature selection.
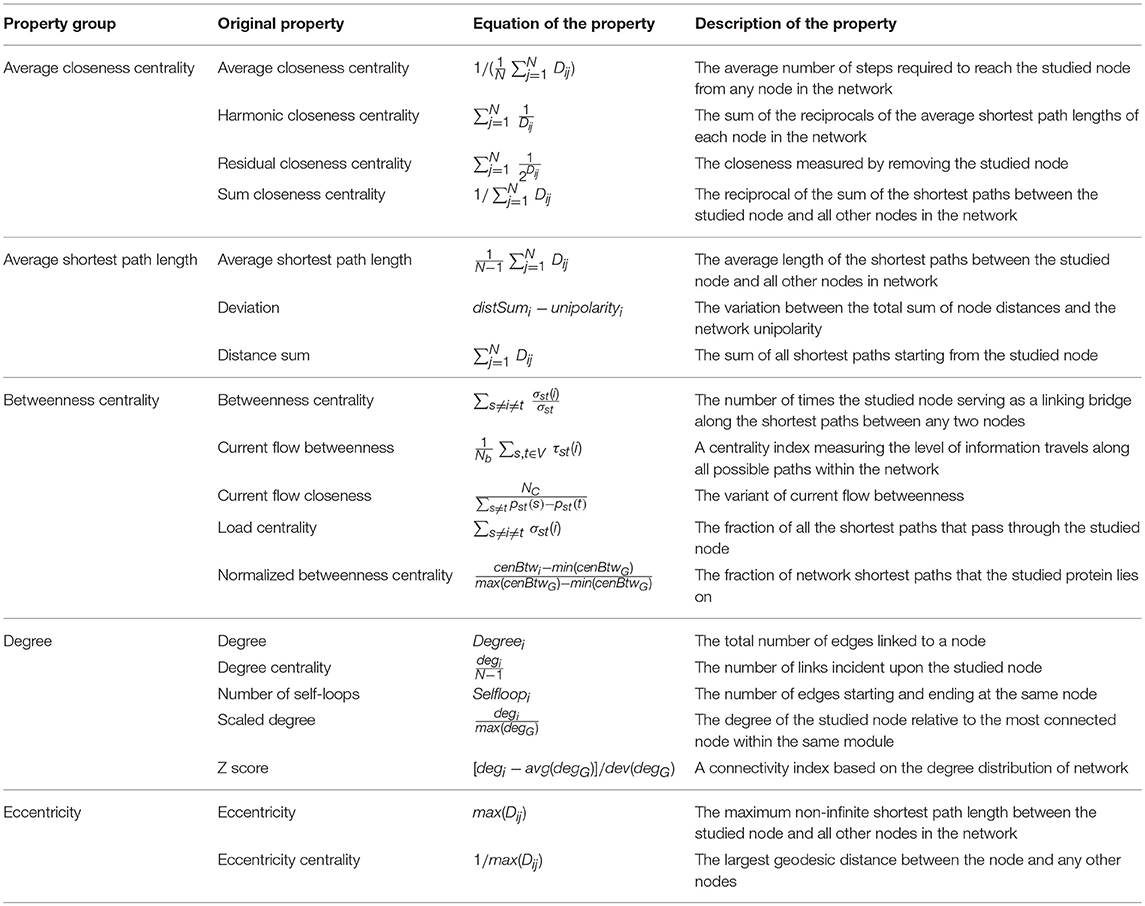
Table 4. 19 substantially overlapped network properties grouped into 5 property groups based on their innate mutual dependence.
As one of the most popular feature selection strategies based on AI, the Boruta algorithm based on a wrapper method built around a random forest classifier (Kursa, 2014) was adopted in this study. Boruta was considered the most powerful method with the stability in variable selection, especially suitable for the low-dimensional dataset among other reported strategies (Degenhardt et al., 2017). In this study, the key differential features were thus selected from 22 properties using R package Boruta by setting the p-value < 0.05. As a result, eight properties were selected as able to collectively reflect the target's mechanism underlying NTI drugs. As illustrated in Figure 1, the boxplots colored in red and green referred to the targets of NTI and NNTI drugs, respectively. Some key features increased from the targets of NTI drug to that of NNTI one (such as average shortest path length), while others demonstrated a decrease (such as average closeness centrality). Based on the comprehensive literature review, some of those 8 key features had been reported to be indirectly relevant to drugs' efficacy-safety balances. For example, the lower value of average closeness centrality of target was reported to demonstrate a less lethality risk (Chen et al., 2011), which was consistent with the findings of this study (a much higher average closeness centrality of the targets of NTI drugs was observed compared with that of NNTI ones, shown in Figure 1). Moreover, the higher level (lower value) of interconnectivity was frequently observed in lethal diseases such as cardiovascular disorder and cancer (Muhammd et al., 2018). Oncological and cardiovascular disorder had been recognized as life-threatening diseases, and the majority of their drugs were reported to be NTI ones (Muller and Milton, 2012; Yu et al., 2015). Thus, the result of interconnectivity in Figure 1 was consistent with these previous reports, which further validated the effectiveness of applied algorithm in identifying key target features underlying NTI drugs.
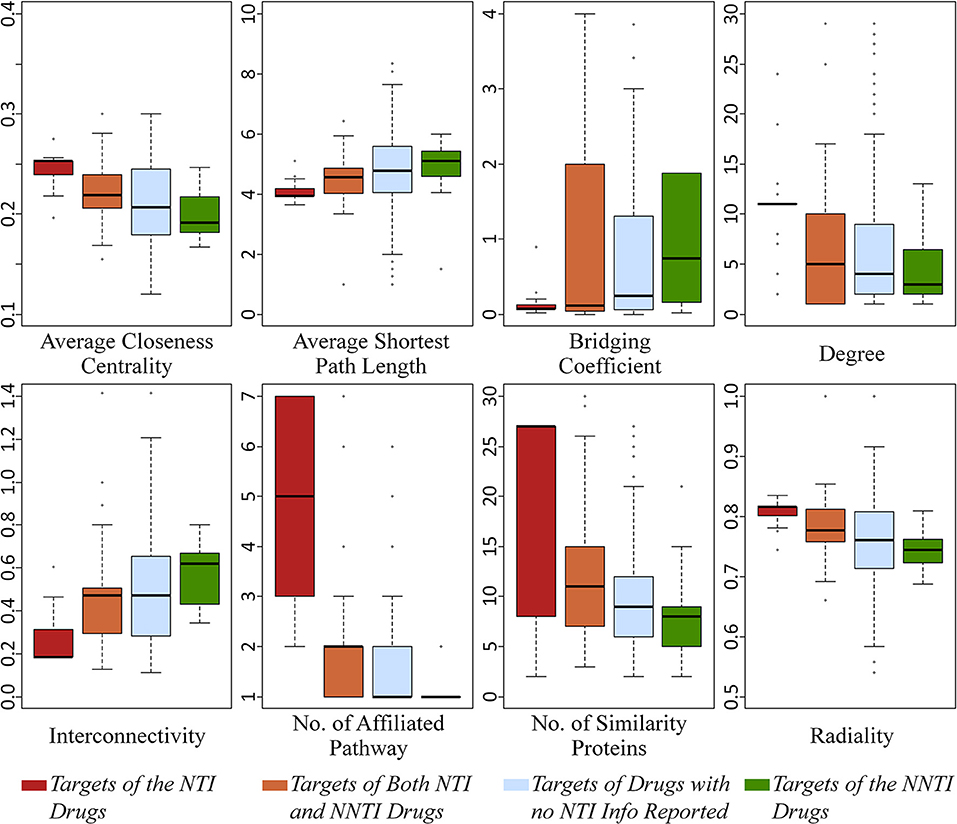
Figure 1. Boxplots of eight key features identified in this study. For each feature, there were four plots colored in red, orange, light blue and green which indicated the targets of NTI drugs, both NTI and NNTI drugs, drugs with no NTI data reported and NNTI drugs, respectively.
Moreover, there were four groups of targets as defined in section Materials and Methods: (a) targets of NTI drugs, (b) targets of both NTI and NNTI drugs, (c) targets of drugs without reported TI, and (d) targets of NNTI drugs. Apart from the target groups (a) and (d), the remaining groups provided more complicated and informative data for illustrating the mechanism underlying NTI drugs. On one hand, the targets in group (b) were affected by both NTI and NNTI drugs, which might reflect properties from both sides, but might also be significantly affected by the properties of confirmed NTI drugs. On the other hand, no TI data of the group (c) targets was reported based on literature review. It was possible that some NTI drugs were not discovered for those targets. But considering the large number of group (c) targets (339 in total), it was highly possible that most of those group (c) targets were only aimed by NNTI drugs, and just a small fraction of which could find new NTI drug in the future. The value of 8 properties of those 4 target groups were illustrated in Figure 1. It was interesting that all properties followed a clear descending/ascending trend from the targets of group (a) to (d), which was in accordance with the analyses provided above. Thus, these findings could be another line of evidence that validated the effectiveness of the feature identification algorithm applied in this study.
Target Mechanism Underlying NTI Drugs Collectively Determined by Multiple Profiles
By collectively considering Figure 1 and Tables 1–3, seven out of those eight selected key features showed significant difference (p-value < 0.05), but it was clear that these significant differences did not guarantee the corresponding feature as the key differential one (57.7% of the features with significant difference (p-value < 0.05) were not selected as key differential ones). Moreover, significant difference was not observed for the selected key feature bridging coefficient (p-value = 0.22). This finding indicated that those eight features collectively determined the target mechanism of NTI drugs, and the TI-related mechanism might be the result of the synergistical effects among those features. Moreover, the majority of these eight key features were identified for the first time by this study, and this work was also the first analysis on the collective effects of both PPI network properties and biological system profile on the drug efficacy-safety balance.
Further analysis on these eight identified key features (shown in Figure 1) revealed that these key features were found to belong to three feature groups. These feature groups were connectivity and centrality of targets in human PPI network together with human biological system features. By combining the data in Figure 1, the key features within the same feature group (illustrated in Figure 2) followed the same ascending/descending trends, which were colored by the same background. As shown in Figure 2, the targets of NTI drugs were highly centralized and connected, and the number of similarity proteins and the number of affiliated pathways were substantially higher than those of NNTI drug. Since the number of similarity proteins and affiliated pathways was reported to be good indicator of target druggability (Zhu F. et al., 2009; Li et al., 2018), the NTI profile identified in this study was in accordance with that of reported target druggability.
Conclusion
This work is the first study conducting comprehensive review on the TI data of all FDA approved drugs (Supplementary Table S1) and revealing the collective effects of both human PPI network properties and biological system profiles on drug efficacy-safety balance. Eight key features were identified here as collectively differentiating the target mechanisms between NTI and NNTI drugs. These features revealed that the targets of NTI drugs were highly centralized and connected in human PPI network, and the numbers of similarity proteins and target-affiliated pathways were both much higher than those of NNTI drugs. These findings together with the newly discovered features/feature groups clarified the key factors indicating drug's narrow TI and could therefore provide a novel direction for determining the delicate drug efficacy-safety balance.
Author Contributions
FZ conceived the idea and supervised the work. XL, JY, and JT performed the research. XL, JY, JT, YL, QY, ZX, RZ, YW, JH, LT, and WX prepared and analyzed the data. FZ wrote the manuscript. All authors have read and approved this manuscript.
Funding
This work was funded by National Natural Science Foundation of China (81872798); Innovation Project on Industrial Generic Key Technologies of Chongqing (cstc2015zdcy-ztzx120003); and Fundamental Research Funds for Central Universities (10611CDJXZ238826, CDJZR14468801, CDJKXB14011, 2015CDJXY).
Conflict of Interest Statement
The authors declare that the research was conducted in the absence of any commercial or financial relationships that could be construed as a potential conflict of interest.
Supplementary Material
The Supplementary Material for this article can be found online at: https://www.frontiersin.org/articles/10.3389/fphar.2018.01245/full#supplementary-material
References
Abernethy, D. R., Woodcock, J., and Lesko, L. J. (2011). Pharmacological mechanism-based drug safety assessment and prediction. Clin. Pharmacol. Ther. 89, 793–797. doi: 10.1038/clpt.2011.55
Badders, N. M., Korff, A., Miranda, H. C., Vuppala, P. K., Smith, R. B., Winborn, B. J., et al. (2018). Selective modulation of the androgen receptor AF2 domain rescues degeneration in spinal bulbar muscular atrophy. Nat. Med. 24, 427–437. doi: 10.1038/nm.4500
Barrett, T., Wilhite, S. E., Ledoux, P., Evangelista, C., Kim, I. F., Tomashevsky, M., et al. (2013). NCBI GEO: archive for functional genomics data sets–update. Nucleic Acids Res. 41, D991–D995. doi: 10.1093/nar/gks1193
Batool, K., and Niazi, M. A. (2014). Towards a methodology for validation of centrality measures in complex networks. PLoS ONE 9:e90283. doi: 10.1371/journal.pone.0090283
Bender, A., Scheiber, J., Glick, M., Davies, J. W., Azzaoui, K., Hamon, J., et al. (2007). Analysis of pharmacology data and the prediction of adverse drug reactions and off-target effects from chemical structure. ChemMedChem 2, 861–873. doi: 10.1002/cmdc.200700026
Benet, L. Z., Hosey, C. M., Ursu, O., and Oprea, T. I. (2016). BDDCS, the rule of 5 and drugability. Adv. Drug Deliv. Rev. 101, 89–98. doi: 10.1016/j.addr.2016.05.007
Bolser, D., Dafas, P., Harrington, R., Park, J., and Schroeder, M. (2003). Visualisation and graph-theoretic analysis of a large-scale protein structural interactome. BMC Bioinformatics 4:45. doi: 10.1186/1471-2105-4-45
Braeuning, A. (2013). The connection of beta-catenin and phenobarbital in murine hepatocarcinogenesis: a critical discussion of Awuah et al. Arch. Toxicol. 87, 401–402. doi: 10.1007/s00204-012-1002-4
Carson, M. B., and Lu, H. (2015). Network-based prediction and knowledge mining of disease genes. BMC Med. Genomics 8(Suppl. 2):S9. doi: 10.1186/1755-8794-8-S2-S9
Chen, L., Wang, Q., Zhang, L., Tai, J., Wang, H., Li, W., et al. (2011). A novel paradigm for potential drug-targets discovery: quantifying relationships of enzymes and cascade interactions of neighboring biological processes to identify drug-targets. Mol. Biosyst. 7, 1033–1041. doi: 10.1039/c0mb00249f
Chen, S., Zhang, P., Liu, X., Qin, C., Tao, L., Zhang, C., et al. (2016). Towards cheminformatics-based estimation of drug therapeutic index: predicting the protective index of anticonvulsants using a new quantitative structure-index relationship approach. J. Mol. Graph. Model. 67, 102–110. doi: 10.1016/j.jmgm.2016.05.006
Costenbader, E., and ValenteFontanesi, T. W. (2003). The stability of centrality measures when networks are sampled. Soc. Netw. 25, 283–307. doi: 10.1016/S0378-8733(03)00012-1
Dangalchev, C. (2006). Residual closeness in networks. Phys. A 365, 556–564. doi: 10.1016/j.biortech.2018.08.122
Degenhardt, F., Seifert, S., and Szymczak, S. (2017). Evaluation of variable selection methods for random forests and omics data sets. Brief. Bioinform. doi: 10.1093/bib/bbx124. [Epub ahead of print].
Emig, D., Ivliev, A., Pustovalova, O., Lancashire, L., Bureeva, S., Nikolsky, Y., et al. (2013). Drug target prediction and repositioning using an integrated network-based approach. PLoS ONE 8:e60618. doi: 10.1371/journal.pone.0060618
Ernst, M., Du, Y., Warsow, G., Hamed, M., Endlich, N., Endlich, K., et al. (2017). FocusHeuristics - expression-data-driven network optimization and disease gene prediction. Sci. Rep. 7:42638. doi: 10.1038/srep42638
Ewer, M. S., and Ewer, S. M. (2015). Cardiotoxicity of anticancer treatments. Nat. Rev. Cardiol. 12, 547–558. doi: 10.1038/nrcardio.2015.65
Fry, D. W., Harvey, P. J., Keller, P. R., Elliott, W. L., Meade, M., Trachet, E., et al. (2004). Specific inhibition of cyclin-dependent kinase 4/6 by PD 0332991 and associated antitumor activity in human tumor xenografts. Mol. Cancer. Ther. 3, 1427–1438.
Fu, J., Tang, J., Wang, Y., Cui, X., Yang, Q., Hong, J., et al. (2018). Discovery of the consistently well-performed analysis chain for SWATH-MS based pharmacoproteomic quantification. Front. Pharmacol. 9:681. doi: 10.3389/fphar.2018.00681
Fu, T., Zheng, G., Tu, G., Yang, F., Chen, Y., Yao, X., et al. (2018). Exploring the binding mechanism of metabotropic glutamate receptor 5 negative allosteric modulators in clinical trials by molecular dynamics simulations. ACS Chem. Neurosci. 9, 1492–1502. doi: 10.1021/acschemneuro.8b00059
Fujimoto, G. M., Monroe, M. E., Rodriguez, L., Wu, C., MacLean, B., Smith, R. D., et al. (2014). Accounting for population variation in targeted proteomics. J. Proteome Res. 13, 321–323. doi: 10.1021/pr4011052
Garlaschelli, D., and Loffredo, M. I. (2004). Patterns of link reciprocity in directed networks. Phys. Rev. Lett. 93:268701. doi: 10.1103/PhysRevLett.93.268701
Ghosh, S., Kumar, G. V., Basu, A., and Banerjee, A. (2015). Graph theoretic network analysis reveals protein pathways underlying cell death following neurotropic viral infection. Sci. Rep. 5:14438. doi: 10.1038/srep14438
Guo, R., Zhang, X., Su, J., Xu, H., Zhang, Y., Zhang, F., et al. (2018). Identifying potential quality markers of Xin-Su-Ning capsules acting on arrhythmia by integrating UHPLC-LTQ-Orbitrap, ADME prediction and network target analysis. Phytomedicine 44, 117–128. doi: 10.1016/j.phymed.2018.01.019
Han, Z. J., Xue, W. W., Tao, L., and Zhu, F. (2018). Identification of novel immune-relevant drug target genes for Alzheimer's disease by combining ontology inference with network analysis. CNS Neurosci. Ther. doi: 10.1111/cns.13051. [Epub ahead of print].
Huang, L. H., He, Q. S., Liu, K., Cheng, J., Zhong, M. D., Chen, L. S., et al. (2018). ADReCS-Target: target profiles for aiding drug safety research and application. Nucleic Acids Res. 46, D911–D917. doi: 10.1093/nar/gkx899
Hwang, W. C., Zhang, A., and Ramanathan, M. (2008). Identification of information flow-modulating drug targets: a novel bridging paradigm for drug discovery. Clin. Pharmacol. Ther. 84, 563–572. doi: 10.1038/clpt.2008.129
Iloro, I., Gonzalez, E., Gutierrez-de Juan, V., Mato, J. M., Falcon-Perez, J. M., and Elortza, F. (2013). Non-invasive detection of drug toxicity in rats by solid-phase extraction and MALDI-TOF analysis of urine samples. Anal. Bioanal. Chem. 405, 2311–2320. doi: 10.1007/s00216-012-6644-9
Jiang, X. L., Samant, S., Lesko, L. J., and Schmidt, S. (2015). Clinical pharmacokinetics and pharmacodynamics of clopidogrel. Clin. Pharmacokinet. 54, 147–166. doi: 10.1007/s40262-014-0230-6
Kanehisa, M., Furumichi, M., Tanabe, M., Sato, Y., and Morishima, K. (2017). KEGG: new perspectives on genomes, pathways, diseases and drugs. Nucleic Acids Res. 45, D353–D361. doi: 10.1093/nar/gkw1092
Kimmelman, J., and Federico, C. (2017). Consider drug efficacy before first-in-human trials. Nature 542, 25–27. doi: 10.1038/542025a
Kivimäki, I., Lebichot, B., Saramaki, J., and Saerens, M. (2016). Two betweenness centrality measures based on randomized shortest paths. Sci. Rep. 6:19668. doi: 10.1038/srep19668
Kogenaru, S., del Val, C., Hotz-Wagenblatt, A., and Glatting, K. H. (2010). TissueDistributionDBs: a repository of organism-specific tissue-distribution profiles. Theor. Chem. Acc. 125:9. doi: 10.1186/s40199-014-0080-7
Koschützki, D., and Schreiber, F. (2008). Centrality analysis methods for biological networks and their application to gene regulatory networks. Gene Regul. Syst. Biol. 2, 193–201.
Krittanawong, C., Zhang, H., Wang, Z., Aydar, M., and Kitai, T. (2017). Artificial intelligence in precision cardiovascular medicine. J. Am. Coll. Cardiol. 69, 2657–2664. doi: 10.1016/j.jacc.2017.03.571
Kursa, M. B. (2014). Robustness of random forest-based gene selection methods. BMC Bioinformatics 15:8. doi: 10.1186/1471-2105-15-8
Lapenna, S., and Giordano, A. (2009). Cell cycle kinases as therapeutic targets for cancer. Nat. Rev. Drug Discov. 8, 547–566. doi: 10.1038/nrd2907
Li, B., Tang, J., Yang, Q., Cui, X., Li, S., Chen, S., et al. (2016). Performance evaluation and online realization of data-driven normalization methods used in LC/MS based untargeted metabolomics analysis. Sci. Rep. 6:38881. doi: 10.1038/srep38881
Li, B., Tang, J., Yang, Q., Li, S., Cui, X., Li, Y., et al. (2017). NOREVA: normalization and evaluation of MS-based metabolomics data. Nucleic Acids Res. 45, W162–W170. doi: 10.1093/nar/gkx449
Li, W., Chen, L., Li, X., Jia, X., Feng, C., Zhang, L., et al. (2013). Cancer-related marketing centrality motifs acting as pivot units in the human signaling network and mediating cross-talk between biological pathways. Mol. Biosyst. 9, 3026–3035. doi: 10.1039/c3mb70289h
Li, Y. H., Wang, P. P., Li, X. X., Yu, C. Y., Yang, H., Zhou, J., et al. (2016b). The human kinome targeted by FDA approved multi-target drugs and combination products: a comparative study from the drug-target interaction network perspective. PLoS ONE 11:e0165737. doi: 10.1371/journal.pone.0165737
Li, Y. H., Xu, J. Y., Tao, L., Li, X. F., Li, S., Zeng, X., et al. (2016a). SVM-prot 2016: a web-server for machine learning prediction of protein functional families from sequence irrespective of similarity. PLoS ONE 11:e0155290. doi: 10.1371/journal.pone.0155290
Li, Y. H., Yu, C. Y., Li, X. X., Zhang, P., Tang, J., Yang, Q., et al. (2018). Therapeutic target database update 2018: enriched resource for facilitating bench-to-clinic research of targeted therapeutics. Nucleic Acids Res. 46, D1121–D1127. doi: 10.1093/nar/gkx1076
Ma, B., Wang, H., Dsouza, M., Lou, J., He, Y., Dai, Z., et al. (2016). Geographic patterns of co-occurrence network topological features for soil microbiota at continental scale in eastern China. ISME J. 10, 1891–1901. doi: 10.1038/ismej.2015.261
Muhammd, J., Khan, A., Ali, A., Fang, L., Yanjing, W., Xu, Q., et al. (2018). Network pharmacology: exploring the resources and methodologies. Curr. Top. Med. Chem. 18, 949–964. doi: 10.2174/1568026618666180330141351
Muller, P. Y., and Dieterle, F. (2009). Tissue-specific, non-invasive toxicity biomarkers: translation from preclinical safety assessment to clinical safety monitoring. Expert Opin. Drug Metab. Toxicol. 5, 1023–1038. doi: 10.1517/17425250903114174
Muller, P. Y., and Milton, M. N. (2012). The determination and interpretation of the therapeutic index in drug development. Nat. Rev. Drug Discov. 11, 751–761. doi: 10.1038/nrd3801
Paladugu, S. R., Zhao, S., Ray, A., and Raval, A. (2008). Mining protein networks for synthetic genetic interactions. BMC Bioinformatics 9:426. doi: 10.1186/1471-2105-9-426
Pan, Y., Wang, Z., Zhan, W., and Deng, L. (2018). Computational identification of binding energy hot spots in protein-RNA complexes using an ensemble approach. Bioinformatics 34, 1473–1480. doi: 10.1093/bioinformatics/btx822
Ragusa, M., Avola, G., Angelica, R., Barbagallo, D., Guglielmino, M. R., Duro, L. R., et al. (2010). Expression profile and specific network features of the apoptotic machinery explain relapse of acute myeloid leukemia after chemotherapy. BMC Cancer 10:377. doi: 10.1186/1471-2407-10-377
Rao, H. B., Zhu, F., Yang, G. B., Li, Z. R., and Chen, Y. Z. (2011). Update of PROFEAT: a web server for computing structural and physicochemical features of proteins and peptides from amino acid sequence. Nucleic Acids Res. 39, W385–W390. doi: 10.1093/nar/gkr284
Rodgers, A. D., Zhu, H., Fourches, D., Rusyn, I., and Tropsha, A. (2010). Modeling liver-related adverse effects of drugs using knearest neighbor quantitative structure-activity relationship method. Chem. Res. Toxicol. 23, 724–732. doi: 10.1021/tx900451r
Rogelj, P., Hudej, R., and Petric, P. (2013). Distance deviation measure of contouring variability. Radiol. Oncol. 47, 86–96. doi: 10.2478/raon-2013-0005
Rubinov, M., and Sporns, O. (2010). Complex network measures of brain connectivity: uses and interpretations. Neuroimage 52, 1059–1069. doi: 10.1016/j.neuroimage.2009.10.003
Rzepecki, A. K., Cheng, H., and McLellan, B. N. (2018). Cutaneous toxicity as a predictive biomarker for clinical outcome in patients receiving anticancer therapy. J. Am. Acad. Dermatol. 79, 545–555. doi: 10.1016/j.jaad.2018.04.046
Seo, S., Oh, M., Park, Y., and Kim, S. (2018). DeepFam: deep learning based alignment-free method for protein family modeling and prediction. Bioinformatics 34, i254–i262. doi: 10.1093/bioinformatics/bty275
Shang, L., Liu, C., Tomiura, Y., and Hayashi, K. (2017). Machine-learning-based olfactometer: prediction of odor perception from physicochemical features of odorant molecules. Anal. Chem. 89, 11999–12005. doi: 10.1021/acs.analchem.7b02389
Shannon, P., Markiel, A., Ozier, O., Baliga, N. S., Wang, J. T., Ramage, D., et al. (2003). Cytoscape: a software environment for integrated models of biomolecular interaction networks. Genome Res. 13, 2498–2504. doi: 10.1101/gr.1239303
Singh, S., Malik, B. K., and Sharma, D. K. (2007). Choke point analysis of metabolic pathways in E.histolytica: a computational approach for drug target identification. Bioinformation 2, 68–72. doi: 10.6026/97320630002068
Solá, L., Romance, M., Criado, R., Flores, J., Garcia del Amo, A., and Boccaletti, S. (2013). Eigenvector centrality of nodes in multiplex networks. Chaos 23:033131. doi: 10.1063/1.4818544
Song, L., Xu, W., Li, C., Li, H., Wu, L., Xiang, J., et al. (2006). Development of expressed sequence tags from the bay scallop, Argopecten irradians irradians. Mar. Biotechnol. 8, 161–169. doi: 10.1007/s10126-005-0126-4
Sormani, M. P. (2012). Modeling the distribution of new MRI cortical lesions in multiple sclerosis longitudinal studies. Mult. Scler. Relat. Disord. 1:108. doi: 10.1016/j.msard.2012.01.001
Sotillo, R., Dubus, P., Martin, J., de la Cueva, E., Ortega, S., Malumbres, M., et al. (2001). Wide spectrum of tumors in knock-in mice carrying a Cdk4 protein insensitive to INK4 inhibitors. EMBO J. 20, 6637–6647. doi: 10.1093/emboj/20.23.6637
Szklarczyk, D., Franceschini, A., Wyder, S., Forslund, K., Heller, D., Huerta-Cepas, J., et al. (2015). STRING v10: protein-protein interaction networks, integrated over the tree of life. Nucleic Acids Res. 43, D447–D452. doi: 10.1093/nar/gku1003
Tao, L., Zhu, F., Qin, C., Zhang, C., Xu, F., Tan, C. Y., et al. (2014). Nature's contribution to today's pharmacopeia. Nat. Biotechnol. 32, 979–980. doi: 10.1038/nbt.3034
Tao, L., Zhu, F., Xu, F., Chen, Z., Jiang, Y. Y., and Chen, Y. Z. (2015). Co-targeting cancer drug escape pathways confers clinical advantage for multi-target anticancer drugs. Pharmacol. Res. 102, 123–131. doi: 10.1016/j.phrs.2015.09.019
Thomas, S., and Bonchev, D. (2010). A survey of current software for network analysis in molecular biology. Hum. Genomics 4, 353–360. doi: 10.1186/1479-7364-4-5-353
UniProt Consortium, T. (2018). UniProt: the universal protein knowledgebase. Nucleic Acids Res. 46:2699. doi: 10.1093/nar/gky092
van der Burgh, H. K., Schmidt, R., Westeneng, H. J., de Reus, M. A., van den Berg, L. H., and van den Heuvel, M. P. (2017). Deep learning predictions of survival based on MRI in amyotrophic lateral sclerosis. Neuroimage Clin. 13, 361–369. doi: 10.1016/j.nicl.2016.10.008
Wang, P., Fu, T., Zhang, X., Yang, F., Zheng, G., Xue, W., et al. (2017a). Differentiating physicochemical properties between NDRIs and sNRIs clinically important for the treatment of ADHD. Biochim. Biophys. Acta 1861, 2766–2777. doi: 10.1016/j.bbagen.2017.07.022
Wang, P., Yang, F., Yang, H., Xu, X., Liu, D., Xue, W., et al. (2015). Identification of dual active agents targeting 5-HT1A and SERT by combinatorial virtual screening methods. Biomed. Mater. Eng. 26(Suppl. 1), S2233–2239. doi: 10.3233/BME-151529
Wang, P., Zhang, X., Fu, T., Li, S., Li, B., Xue, W., et al. (2017b). Differentiating physicochemical properties between addictive and nonaddictive ADHD drugs revealed by molecular dynamics simulation studies. ACS Chem. Neurosci. 8, 1416–1428. doi: 10.1021/acschemneuro.7b00173
Wang, S., Tong, Y., Ng, T. B., Lao, L., Lam, J. K., Zhang, K. Y., et al. (2015). Network pharmacological identification of active compounds and potential actions of Erxian decoction in alleviating menopause-related symptoms. Chin. Med. 10:19. doi: 10.1186/s13020-015-0051-z
Watts, D. J., and Strogatz, S. H. (1998). Collective dynamics of 'small-world' networks. Nature 393, 440–442. doi: 10.1038/30918
Xu, J., Wang, P., Yang, H., Zhou, J., Li, Y., Li, X., et al. (2016). Comparison of FDA approved kinase targets to clinical trial ones: insights from their system profiles and drug-target interaction networks. Biomed. Res. Int. 2016:2509385. doi: 10.1155/2016/2509385
Xu, R., and Wang, Q. (2014). Automatic construction of a large-scale and accurate drug-side-effect association knowledge base from biomedical literature. J. Biomed. Inform. 51, 191–199. doi: 10.1016/j.jbi.2014.05.013
Xue, W., Wang, P., Li, B., Li, Y., Xu, X., Yang, F., et al. (2016). Identification of the inhibitory mechanism of FDA approved selective serotonin reuptake inhibitors: an insight from molecular dynamics simulation study. Phys. Chem. Chem. Phys. 18, 3260–3271. doi: 10.1039/c5cp05771j
Xue, W., Wang, P., Tu, G., Yang, F., Zheng, G., Li, X., et al. (2018a). Computational identification of the binding mechanism of a triple reuptake inhibitor amitifadine for the treatment of major depressive disorder. Phys. Chem. Chem. Phys. 20, 6606–6616. doi: 10.1039/c7cp07869b
Xue, W., Yang, F., Wang, P., Zheng, G., Chen, Y., Yao, X., et al. (2018b). What contributes to serotonin-norepinephrine reuptake inhibitors' dual-targeting mechanism? The key role of transmembrane domain 6 in human serotonin and norepinephrine transporters revealed by molecular dynamics simulation. ACS Chem. Neurosci. 9, 1128–1140. doi: 10.1021/acschemneuro.7b00490
Yang, F., Zheng, G., Fu, T., Li, X., Tu, G., Li, Y. H., et al. (2018). Prediction of the binding mode and resistance profile for a dual-target pyrrolyl diketo acid scaffold against HIV-1 integrase and reverse-transcriptase-associated ribonuclease H. Phys. Chem. Chem. Phys. 20, 23873–23884. doi: 10.1039/c8cp01843j
Yang, F. Y., Fu, T. T., Zhang, X. Y., Hu, J., Xue, W. W., Zheng, G. X., et al. (2017). Comparison of computational model and X-ray crystal structure of human serotonin transporter: potential application for the pharmacology of human monoamine transporters. Mol. Simul. 43, 1089–1098. doi: 10.1080/08927022.2017.1309653
Yang, H., Qin, C., Li, Y. H., Tao, L., Zhou, J., Yu, C. Y., et al. (2016). Therapeutic target database update 2016: enriched resource for bench to clinical drug target and targeted pathway information. Nucleic Acids Res. 44, D1069–D1074. doi: 10.1093/nar/gkv1230
Yu, C. Y., Li, X. X., Yang, H., Li, Y. H., Xue, W. W., Chen, Y. Z., et al. (2018). Assessing the performances of protein function prediction algorithms from the perspectives of identification accuracy and false discovery rate. Int. J. Mol. Sci. 19:19010183. doi: 10.3390/ijms19010183
Yu, L. X., Jiang, W., Zhang, X., Lionberger, R., Makhlouf, F., Schuirmann, D. J., et al. (2015). Novel bioequivalence approach for narrow therapeutic index drugs. Clin. Pharmacol. Ther. 97, 286–291. doi: 10.1002/cpt.28
Zaykov, A. N., Mayer, J. P., and DiMarchi, R. D. (2016). Pursuit of a perfect insulin. Nat. Rev. Drug Discov. 15, 425–439. doi: 10.1038/nrd.2015.36
Zeidán-Chuliá, F., Gursoy, M., Neves de Oliveira, B. H., Ozdemir, V., Kononen, E., and Gursoy, U. K. (2015). A systems biology approach to reveal putative host-derived biomarkers of periodontitis by network topology characterization of MMP-REDOX/NO and apoptosis integrated pathways. Front. Cell. Infect. Microbiol. 5:102. doi: 10.3389/fcimb.2015.00102
Zhang, P., Tao, L., Zeng, X., Qin, C., Chen, S., Zhu, F., et al. (2017a). A protein network descriptor server and its use in studying protein, disease, metabolic and drug targeted networks. Brief. Bioinform. 18, 1057–1070. doi: 10.1093/bib/bbw071
Zhang, P., Tao, L., Zeng, X., Qin, C., Chen, S. Y., Zhu, F., et al. (2017b). PROFEAT update: a protein features web server with added facility to compute network descriptors for studying omics-derived networks. J. Mol. Biol. 429, 416–425. doi: 10.1016/j.jmb.2016.10.013
Zhang, W., Zhang, Q., Zhang, M., Zhang, Y., Li, F., and Lei, P. (2014). Network analysis in the identification of special mechanisms between small cell lung cancer and non-small cell lung cancer. Thorac. Cancer 5, 556–564. doi: 10.1111/1759-7714.12134
Zheng, C. J., Han, L. Y., Yap, C. W., Ji, Z. L., Cao, Z. W., and Chen, Y. Z. (2006). Therapeutic targets: progress of their exploration and investigation of their characteristics. Pharmacol. Rev. 58, 259–279. doi: 10.1124/pr.58.2.4
Zheng, G., Xue, W., Wang, P., Yang, F., Li, B., Li, X., et al. (2016). Exploring the inhibitory mechanism of approved selective norepinephrine reuptake inhibitors and reboxetine enantiomers by molecular dynamics study. Sci. Rep. 6:26883. doi: 10.1038/srep26883
Zheng, G., Xue, W., Yang, F., Zhang, Y., Chen, Y., Yao, X., et al. (2017). Revealing vilazodone's binding mechanism underlying its partial agonism to the 5-HT1A receptor in the treatment of major depressive disorder. Phys. Chem. Chem. Phys. 19, 28885–28896. doi: 10.1039/c7cp05688e
Zhu, F., Han, B., Kumar, P., Liu, X., Ma, X., Wei, X., et al. (2010). Update of TTD: therapeutic target database. Nucleic Acids Res. 38, D787–D791. doi: 10.1093/nar/gkp1014
Zhu, F., Han, L., Zheng, C., Xie, B., Tammi, M. T., Yang, S., et al. (2009). What are next generation innovative therapeutic targets? Clues from genetic, structural, physicochemical, and systems profiles of successful targets. J. Pharmacol. Exp. Ther. 330, 304–315. doi: 10.1124/jpet.108.149955
Zhu, F., Han, L. Y., Chen, X., Lin, H. H., Ong, S., Xie, B., et al. (2008a). Homology-free prediction of functional class of proteins and peptides by support vector machines. Curr. Protein Pept. Sci. 9, 70–95. doi: 10.2174/138920308783565697
Zhu, F., Li, X. X., Yang, S. Y., and Chen, Y. Z. (2018). Clinical success of drug targets prospectively predicted by in silico study. Trends Pharmacol. Sci. 39, 229–231. doi: 10.1016/j.tips.2017.12.002
Zhu, F., Ma, X. H., Qin, C., Tao, L., Liu, X., Shi, Z., et al. (2012a). Drug discovery prospect from untapped species: indications from approved natural product drugs. PLoS ONE 7:e39782. doi: 10.1371/journal.pone.0039782
Zhu, F., Qin, C., Tao, L., Liu, X., Shi, Z., Ma, X., et al. (2011). Clustered patterns of species origins of nature-derived drugs and clues for future bioprospecting. Proc. Natl. Acad. Sci. U. S. A. 108, 12943–12948. doi: 10.1073/pnas.1107336108
Zhu, F., Shi, Z., Qin, C., Tao, L., Liu, X., Xu, F., et al. (2012b). Therapeutic target database update 2012: a resource for facilitating target-oriented drug discovery. Nucleic Acids Res. 40, D1128–D1136. doi: 10.1093/nar/gkr797
Zhu, F., Zheng, C. J., Han, L. Y., Xie, B., Jia, J., Liu, X., et al. (2008b). Trends in the exploration of anticancer targets and strategies in enhancing the efficacy of drug targeting. Curr. Mol. Pharmacol. 1, 213–232. doi: 10.2174/1874467210801030213
Zhu, H., Tropsha, A., Fourches, D., Varnek, A., Papa, E., Gramatica, P., et al. (2008). Combinatorial QSAR modeling of chemical toxicants tested against Tetrahymena pyriformis. J. Chem. Inf. Model. 48, 766–784. doi: 10.1021/ci700443v
Keywords: drug efficacy-safety balance, therapeutic index, artificial intelligence, protein-protein interaction network, biological system profile
Citation: Li X, Yin J, Tang J, Li Y, Yang Q, Xiao Z, Zhang R, Wang Y, Hong J, Tao L, Xue W and Zhu F (2018) Determining the Balance Between Drug Efficacy and Safety by the Network and Biological System Profile of Its Therapeutic Target. Front. Pharmacol. 9:1245. doi: 10.3389/fphar.2018.01245
Received: 28 July 2018; Accepted: 12 October 2018;
Published: 31 October 2018.
Edited by:
Zhi-Liang Ji, Xiamen University, ChinaReviewed by:
Enrique Hernandez-Lemus, Instituto Nacional de Medicina Genómica (INMEGEN), MexicoQing-Chuan Zheng, Jilin University, China
Yan Li, Army Medical University, China
Copyright © 2018 Li, Yin, Tang, Li, Yang, Xiao, Zhang, Wang, Hong, Tao, Xue and Zhu. This is an open-access article distributed under the terms of the Creative Commons Attribution License (CC BY). The use, distribution or reproduction in other forums is permitted, provided the original author(s) and the copyright owner(s) are credited and that the original publication in this journal is cited, in accordance with accepted academic practice. No use, distribution or reproduction is permitted which does not comply with these terms.
*Correspondence: Feng Zhu, zhufeng@zju.edu.cn; prof.zhufeng@gmail.com