- 1Biological Psychology, TU Dresden, Dresden, Germany
- 2Biomedical Cybernetics Group, Biotechnology Center (BIOTEC), Center for Molecular and Cellular Bioengineering (CMCB), Center for Systems Biology Dresden (CSBD), Department of Physics, TU Dresden, Dresden, Germany
- 3Brain Bio-Inspired Computing (BBC) Lab, IRCCS Centro Neurolesi “Bonino Pulejo”, Messina, Italy
- 4Lipotype GmbH, Dresden, Germany
Omic sciences coupled with novel computational approaches such as machine intelligence offer completely new approaches to major depressive disorder (MDD) research. The complexity of MDD's pathophysiology is being integrated into studies examining MDD's biology within the omic fields. Lipidomics, as a late-comer among other omic fields, is increasingly being recognized in psychiatric research because it has allowed the investigation of global lipid perturbations in patients suffering from MDD and indicated a crucial role of specific patterns of lipid alterations in the development and progression of MDD. Combinatorial lipid-markers with high classification power are being developed in order to assist MDD diagnosis, while rodent models of depression reveal lipidome changes and thereby unveil novel treatment targets for depression. In this systematic review, we provide an overview of current breakthroughs and future trends in the field of lipidomics in MDD research and thereby paving the way for precision medicine in MDD.
Introduction
Major depressive disorder (MDD) is a psychiatric illness with devastating consequences with regard to personal and social functioning as well as physical health (1, 2). According to the Diagnostic and Statistical Manual of Mental Disorders (DSM-5), MDD is a heterogeneous disorder, defined and diagnosed on the basis of its core symptoms of a depressed mood or anhedonia and a combination of four of nine other symptoms, such as changes in appetite, sleep, fatigue, concentration, feelings of worthlessness and suicidal ideations persisting for the majority of the day over at least a 2 week period (3). The World Health Organization has declared MDD the leading cause of disability with over 300 million people being affected worldwide (4). Regrettably, no sizeable improvement in population mental health for MDD has been achieved during the last decade (5). Diagnosing and treating MDD is complicated due to its heterogeneous illness presentation exemplified by anhedonia, considered a cardinal feature of MDD, but is truly present in only up to 50% of patients (6). The subjective nature of the patients' report of symptoms makes clinical judgment based on a person's history and cultural norms a difficult task. The high non-responder rates to standard antidepressant treatment present further challenges to clinical practice (7, 8). These factors contribute to low diagnostic reliability and the potential for misdiagnosis (9, 10).
Therefore, during the last few decades, tremendous efforts have been undertaken in order to identify a diagnostic biomarker for MDD, enabling a reliable diagnosis and potentially identifying treatment targets for novel therapeutic approaches. Although advancements in elucidating the pathophysiology of MDD have been achieved (11), one needs to acknowledge that the reductionist single biomarker approach employed during the last decades has been a failure (12). It has become increasingly clear that the pathophysiology of MDD depends on a wide array of biological parameters that cannot be measured by a single biomarker and that only a systems approach might be successful in identifying diagnostic bio-signatures for MDD.
Newly developed high-throughput screening technologies, which facilitate the quantification of omics data, offer new approaches for systems analyses with regard to MDD (13). New computational methods are currently revolutionizing biomarker research by using unsupervised data driven approaches such as machine intelligence or deep learning; these provide increased analytical power in order to come to grips with the complex pathophysiology of MDD (14–16). These computational approaches are capable of delivering multi-parametric disease signatures based on different omics data sets that can be used to identify functional networks (17).
Although studies using omics approaches (e.g., genomic, proteomic, brain connectomics, metabolomics) have increased exponentially during the last 18 years, achieving breakthroughs for example in the field of genome-wide or neurophysiological classification of MDD (18, 19), reproducibility has proved challenging the field. Lipidomics is no exception (12). However, this situation is now improving with the developments of new lipidomics platforms (20) that provide reliable absolute quantitation and inter-site reproducibility (21), which are the basis for clinical applications (12). In this systematic review we will provide an up-to-date overview of existing studies in lipidomics and MDD or rodent models of depression.
The Lipidome in MDD
The lipidome—the complete lipid profile of an organism—has central roles in most aspects of cell biology (22). The human organism invests substantial resources for the production of thousands of molecular species attributable to eight different lipid categories with multiple classes and sub-classes (23). These classes are strongly interconnected and perturbations in a lipid species often result in global lipidome changes (23, 24). An enormous variety of different functions is carried out by lipids, such as energy storage by triacylglycerol (TAG) in lipid droplets or membrane formation and trafficking by amphipathic lipids such as glycerophospholipids (e.g., phosphatidylcholines [PC], phosphatidylethanolamines [PE], and phosphatidylinositols [PI]) (25). Lipids also determine the localization and function of proteins in the cell membranes of neurons and may further act as neurotransmitters (26). Many lipid structures have been shown to be essential for neuronal signaling and survival (27–29), and thereby critically influence an individuals' mood and behavior (26). Lipids also function to sub-compartmentalize cell membranes, forming functional platforms (lipid rafts) that operate in signaling and many other membrane activities (30). Finally, lipids act as second messengers in signal transduction, also regulating glucocorticoid action as well as inflammatory processes (25). Therefore, there is a plethora of lipid markers potentially related to MDD. Reviews and meta-analyses investigating the action of single lipids in MDD suggest that polyunsaturated fatty acids (e.g., omega-3 and omega-6) (31), cholesterol (32), as well as lipids of the sphingomyelin-ceramide (SM-Cer) pathway could be crucially related to mood disorders (33, 34). However, as outlined above, lipids are strongly interconnected and hierarchically organized so that a systems approach based on networks will be an indispensable tool to reveal the role and mechanisms of the lipidome in MDD. Since lipid nomenclature is not yet standardized, in the following, we will report lipid species as in original reports (35).
We will describe the systematic search process for studies applying lipidomic approaches in MDD or rodent models of depression (see Figure 1). Subsequently, we will review the identified studies with regard to their biological interpretation and discriminative power for MDD classification (see Table 1) and compare identified lipid species between studies (see Supplementary Table 1). Finally, we will integrate the findings into a model in which the relationship between the lipidome and MDD is mediated via dysregulated processes in the hypothalamus-pituitary-adrenal (HPA) axis and the immune system (see Figure 2).
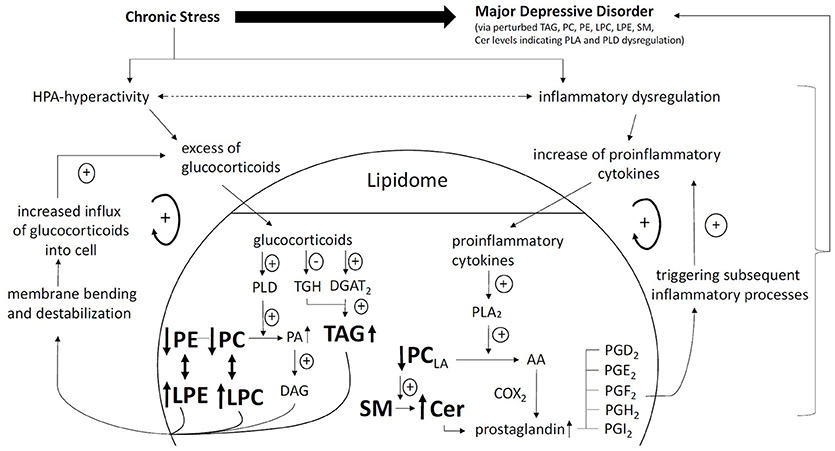
Figure 2. Pathway linking chronic stress, HPA hyperactivity, increased inflammation, lipidomic dysregulation, and development of MDD. Glucocorticoid positive spiral pathway A: Chronic stress leads to HPA hyperactivity. Increased levels of glucocorticoids increase PLD activity (48). Increased PLD activity leads to increased turnover of phosphatidylcholines (PC) and phosphatidylethanolamine (PE) to phosphatidic acid (PA) and also lysophosphatidylcholine (LPC) and lysophatidylethanolamine (LPE). Due to is chemical properties, PA is swiftly converted to diacylglycerol (DAG). DAG, LPC, and LPE cause membrane bending and destabilization allowing more influx of glucocorticoids into the cell. Glucocorticoid positive spiral pathway B: Increased levels of glucocorticoids decrease triacylglycerol hydrolase (TGH) expression. Increased levels of glucocorticoids increase triacylglycerol (TAG) biosynthesis via increase of (diacylglycerol acyltransferase 2) DGAT2. Reduced TGH expression and increased TAG biosynthesis lead to increased levels of TAG (49). TAG in turn is related to increased levels of glucocorticoids. Inflammatory positive spiral pathway: Chronic stress leads to inflammatory dysregulation. An excess of proinflammatory cytokines and phase reactants increase phospholipase A2 (PLA2) (50). Increased PLA2 activity leads to increased turnover of linoleic acid (LA) containing phosphatidylcholines (PCLA) to arachidonic acid (AA). AA is subsequently converted to prostaglandins (PG) of which the series 2 is proinflammatory (e.g., PGA2, PGD2, PGE2, PGF2, PGH2, PGI2). PG further increase inflammatory reactions (40). PC degradation is associated with SM-Cer turn over further increasing PG levels (22). Lipid Nomenclature: following annotations are used: Lipid class <sum of carbon atoms>: <sum of double bonds>; <sum of hydroxyl groups>, e.g., SM 34:1;2 signifies a SM species with 34 carbon atoms, 1 double bond and 2 hydroxyl groups in the ceramide backbone. Lipid molecular subspecies annotation (35) contains additional information about the exact identity of their fatty acids, the exact position of which cannot be discriminated in relation to the glycerol backbone (sn-1 or sn-2). This is indicated by a dash: “-.” For example, PC 18:1;0-16:0;0 denotes a phosphatidylcholine with a 18:1;0 (18 carbon atoms, 1 double bond, 0 hydroxylation) and a 16:0;0 fatty acid. CE 18:1;0 denotes a cholesteryl ester with a 18:1;0 fatty acid.
Search Strategy and Study Selection
Candidate studies were identified via PubMed/Medline, EMBASE and PsycINFO using the following search strategy, whereby separate searches were performed for human and rodent studies respectively. The applied key terms for human studies were [(depressive mood OR depressed mood OR depression OR major depression) AND (lipidomics OR lipidome)], whereas for rodent studies the key terms [(depression OR depressive behavior OR depressive-like behavior OR depressed mood OR depressive mood OR antidepressant OR chronic unpredictable stress OR chronic unpredictable mild stress OR learned helplessness OR inescapable foot shock OR pain-induced depression OR social defeat) AND (rodents OR rats OR mice) AND (lipidomics OR lipidome)] were used for searching in the title, abstract and/or any other field registered in the database. The search was restricted to English language journal articles published between database inception and 25th April 2018. The final set of articles was cross-validated and further completed based on prior reviews. In sum, 123 records were extracted. The set was refined by removing (i) duplicate entries (n = 46). Titles and abstracts were then screened for relevance, removing (ii) reviews, meta-analyses, case studies, meeting abstracts, study protocols, practical guidelines and books (n = 27). In a next step, the full text of the remaining 50 articles was assessed for eligibility, subsequently excluding all studies that did not deal with (iii) lipidomics (n = 6) or (iv) MDD or behavioral mouse models of depression (n = 29). In a final step, studies (v) with a special focus on bio-technologies (n = 3) were eliminated. Figure 1 shows the sample development throughout the selection process, reaching the final set of 12 studies for review including 7 human and 5 rodent studies (see Table 1). The study selection and eligibility screening were conducted according to the PRISMA guidelines (47).
Lipidomic Studies in MDD and Rodent Models of Depression
Human Studies
In humans, a pioneering study by Demirkan et al. using unsupervised lipidomic analysis of 148 plasma phospho- and sphingolipid species in a sample of 742 individuals identified significant associations with a psychometric depression measure (Center for Epidemiological Studies-Depression Scale: CES-D) for the ratio sphingomyelin (SM) 23:1 to SM 16:0 and the absolute amount of PC (alkyl subclass) O 36:4, as well as the ratio of PC O 36:4 to Cer 20:0 (37). However, subsequent analysis of an independent replication dataset (N = 753) only revealed absolute levels of PC O 36:4 to be robustly inversely related to depressive symptoms. Though being robustly associated to the CES-D in this study, this lipid structure alone did not provide sufficient discriminating power to adequately differentiate between individuals suffering from MDD and healthy controls. The authors of the study further highlighted that PC O 36:4 is a potential target of phospholipase A2 (PLA2), which converts PC O 36:4 to lysophosphatidylcholines (LPCs). When PC O 36:4 is hydrolyzed by PLA2, arachidonic acid (AA) is produced, which is subsequently rapidly converted into inflammatory mediators (prostaglandins), potentially leading to increased neuro-inflammation, which has consistently been related to MDD (51). AA itself, however, is known to directly modulate neural cell function via different processes (e.g., membrane fluidity and polarization) and thereby AA could negatively affect brain function and contribute directly to depressive symptomatology (37) [see also the study by Knowles et al. section Lipidome associations with glucocorticoids and inflammatory markers. for lipid-inflammation dependent pathways in MDD (40)].
Liu et al., based on prior work (52), conducted a non-targeted lipidomic approach comparing 60 patients with diagnosed MDD with 60 healthy controls (HCs) in a discovery set and a validation set of a similar size (42). The authors reported that several differential lipid species were significantly correlated with depression severity measured by the Hamilton Depression Scale (HAMD) (42). Total levels of the following lipid classes were elevated in depressed individuals LPC, lysophosphatidylethanolamine (LPE), PC, PE, phosphatidylinositol (PI), DAG, and TAG, while total PE O and several SM species were decreased in depressed individuals. The authors, however, did not elaborate on the molecular mechanisms linking these lipid classes with MDD. Yet, negative associations between specific SM species and depressive symptoms were also reported by Demirkan et al. (37). An experimental study further investigated the effects of acid sphingomyelinase (Asm), which releases ceramides (Cer) from SMs (33). In this study, it was shown that therapeutic concentrations of two different antidepressants reduced Asm activity and Cer levels, while increased neuroplasticity and non-depressive behavior was observed (33). This suggests that SM levels and the turnover to Cer are dysregulated in MDD and restored with antidepressant medication. Furthermore, using unsupervised statistical approaches, Liu et al. extracted a combinatorial lipid marker including five lipids as diagnostic biomarker with an area under the curve (AUC) between 0.855 and 0.931 suggesting a good discriminative power for MDD and HCs. However, the identified five lipids LPE 20:4, PC 34:1, PI 40:4, SM 39:1, and TAG 44:2 were not further validated in subsequent lipidomic studies on MDD. Unfortunately, the authors did not provide values for the area under the precision/recall curve (AUPR), although unbalanced analysis was performed when comparing HCs (N = 111) to either moderately depressed (N = 78) or severely depressed individuals (N = 57). Here, it is important to note that AUC alone is not a good measure of performance in unbalanced data sets as represented by the comparison of moderately or severely depressed vs. HCs. Thus, combined reporting of AUC and AUPR is required. AUC alone can present an overly optimistic view of the algorithm's performance when there is a skew in the class distribution (53). Comparing these results, a metabolomics/lipidomic study reports an accuracy for their extracted combinatorial biomarker of 72.2% for the discrimination of MDD and HCs, which is significantly lower (54). The authors subsequently sub-classified MDD individuals into anxious depressed (N = 58) and melancholic depressed (N = 21) and compared these groups to HC (N = 97). Using this approach, an accuracy of 83.8% for melancholic depressed individuals (72% for anxious depressed individuals) was achieved. However, the authors neither reported AUC nor AUPR levels (54). This might therefore still overestimate the actual discriminative power since the false positive rate was not considered within the accuracy measure. In this study, of the lipids, PC and SM levels were decreased and Cer levels were increased, again indicating increased SM to Cer turnover by Asm hyperactivity (54).
A study by Kim et al. including 25 currently affected MDD cases, 25 remitted MDD cases, and 25 HCs, identified a lipid marker consisting of lysophosphatidic acid (LPA) 16:1, TAG 44:0, and TAG 54:8, discriminating between HCs and MDD cases with 76% accuracy (HCs vs remitted with 60% accuracy; LPA16:1, TAG52:6, TAG54:8, TAG58:10) (39). With regard to differentially elevated lipids in the three groups, an inconsistent picture emerged with no differential PC and PE species, while TAG and DAG species showed the most differentiating power and were significantly increased in MDD patients compared to controls (39). TAGs are the most abundant lipid species in the human organism and constitute a major source of energy, while lipoprotein lipase (LPL) enzymes such as adipose triglyceride lipase (ATGL) and hormone sensitive lipase (HSL) are responsible for the breakdown of TAG into DAG and free fatty acids (FFA) (55). In the brain, TAG degradation by LPL informs neurons and astrocytes about energy expenditure (56), while a dysfunction of these enzymes leading to increased TAG levels may underlie the increased fatigue observed in depressive disorders.
Chan et al. further investigated the lipidome in patients with coronary artery disease (CAD) and identified 10 phospholipids, which showed good discriminative power (84%) to differentiate between CAD patients with substantial depressive burden (CES-D ≥ 16) and those without (36). The identified phospholipids contained several proinflammatory compounds (e.g., linoleic acid [LA] or AA), which are released by PLA2 and are crucially implicated in proinflammatory processes (57, 58). This finding further underlines a lipid-inflammation-dependent pathway in MDD. In line with this, Hennebelle et al. report that in seasonal affective disorders (SAD), four oxylipins, which are oxidation products of polyunsaturated fatty acids, were increased in winter (38). Since oxylipins are involved in regulating proinflammatory processes, this suggests that season-dependent changes in omega-3/-6 metabolism underlie inflammatory states in SAD. However, it is important to mention that a smaller study by Kuwano et al. failed to identify any significant differences in the plasma lipidome of first-episode drug-naïve MDD patients (N = 15) compared to HCs (N = 19), but identifed two metabolites (tryptophan and kynurenine) that were significantly reduced in patients (41).
Therefore, due to the plethora of potential lipid species and inconsistent results of lipidomic studies in MDD, uncertainty prevails about which lipids are implicated in MDD pathophysiology and which lipids best segregate between MDD and HCs. Furthermore, no data on the time course of these processes exist in humans, while rodent studies provide some insight into the causal relation between the lipidome and depression models.
Rodent Studies
Important insights emerged from recent rodent studies investigating the lipidome in models of depression. One of the first rodent studies examining the lipidome in rodents used a 4 week antidepressant treatment and compared the prefrontal cortex of the rats with regard to changes in the lipidome (45). In the prefrontal cortex, robust significant reduction in the relative abundance of PC species (PC36:1, PC38:3, PC40:2, PC40:6, PC40:5, PC42:7, PC42:6, and PC42:5) and increases of LPC species (LPC16:0, LPC18:0, and LPC18:2) were identified in response to maprotiline and paroxetine, but not fluoxetine treatment indicating an increase in PLA2 activity and possible release of long-chain fatty acids on the rat brain lipidome (45). For maprotiline and paroxetine, an increase in Cer levels (Cer18:1/18:0, Cer18:1/20:0, Cer18:1/22:0,) was also observed, as well as a decrease in PE levels (PE36:4, PE36:5) and mixed changes with regard to the direction of change in SM levels (increase: SM18/16:0; decrease: SM18/24:0, SM18/24:1). PCs are the major lipids distributed in the cell membrane, while LPCs directly exert effects on membrane permeability, and SMs and Cers are also critically integrated in the membrane formation process. These results indicate that antidepressant treatments influence membrane formation, structure and permeability. Here, it is important to mention that the authors did not experimentally induce depression in the rodents and then treat them with antidepressant medication, but instead directly treated the rodents with antidepressant medication. Although this might be beneficial on a behavioral level, altering an organism's homeostasis might be related to adverse consequences with regard to its lipidome. However, the same group added behavioral testing to the reported lipidome changes for maprotiline and showed increased non-depressive behavior for maprotiline treatment (46). Interestingly, when rodents concomitantly experienced PLA2 suppression, increased depressive behavior could be observed indicating a crucial role of PLA2 activity with regard to antidepressant treatment (46). PLA2 hydrolyses the fatty acid from the sn-2 position of PCs, rendering the cleavage products available for subsequent biological actions increasing LPC, DAG, and TAG levels (59). Contrasting these results in a study using chronic restraint stress, the depressed rodents exhibited increased plasma levels of LPC (LPC18:1, LPC20:1, LPC-O16:2, LPC-O18:3) and decreased levels of PC (PC32:1, PC36:4, PC37:4, PC38:4, PC40:6, PC-O36:4, PC-O38:5) compared to control animals (43). However, a rodent study of chronic unpredictable stress (CUS; a commonly used paradigm in preclinical depression research) showed significant increases on brain lipid class level in the relative content of PC and PE levels in the stressed mice. A significant decrease in the relative content of PI levels could also be observed indicating membrane structure and function change due to chronic stress (44). Another study which used the CUS model revealed somewhat opposing changes in the rat brain lipidome and mainly in the prefrontal cortex, with increased LPC, LPE, and Cer levels and decreased PE, ether phosphatidylcholines (PC E), and SM levels (34). Interestingly, with regard to serotonin deficiency investigated in a genetic knockout model TPH2-/-, serotonin-deficient mice expressed corresponding differences in the lipid profile, with reduced levels in several PC, PE, PI species and increased levels in several LPC, LPE, and Cer species (60). Taken together, the rodent studies, unlike the human studies, form a more consistent picture, showing that experimentally induced depressive states reduced PC, PE, PI, and increased LPC, LPE, Cer, DAG and TAG levels.
Lipidome Associations With Glucocorticoids and Inflammatory Markers
After more than 30 years of biomarker research in MDD, hypercortisolism and chronic low grade inflammation are considered to be the two most consistent findings and the most salient biomarkers in MDD research (11). In the following, we provide an overview of the functional cross-talk between glucocorticoid secretion, inflammatory processes and the lipidome.
Studies by Mocking and colleagues have shown that in patients with recurrent MDD, salivary cortisol was negatively associated to fatty acid-metabolism in three fatty acids (arachidonic acid (AA), eicosapentaenoic acid (EPA), docosahexaenoic acid (DHA) (61). Furthermore, in a longitudinal study including 70 MDD patients and 51 matched controls, fatty acid-metabolism showed a significantly stronger negative relationship to salivary cortisol in patients than in the controls, while a stronger negative relationship between fatty acid-metabolism and cortisol levels was predictive for non-response to antidepressants in patients (62). Importantly, a rodent study examined the lipidome after exogeneous corticosterone administration and several brain lipid alterations were observed [decrease in: PCs, PE; increase in: LPC, Cer, PA, phosphatidylglycerol (PG)], revealing a tight interconnectedness between glucocorticoids and brain lipids (34). However, other studies reported contradictory results with regard to chronic stress (44), or found that there was no effect of corticosterone administration on Cer levels or acid sphingomyelinase (Asm), which metabolizes SM to Cer (33). However, in these contradictory studies, differing forms of application and dosages were used (oral 0.25 mg mL−1 via drinking water vs. subcutaneous injections of 40 mg kg−1) suggesting that higher doses of corticosterone administration reveal stronger changes in the rat brain lipidome. In vitro examinations further showed that dexamethasone-treated M-1 cells (a mammalian cell line from the cortical collecting duct) express higher phospholipase D (PLD) activity, which hydrolyzes PC to PA, subsequently leading to vesicle formation, budding, and fission from neurons (48). Increased PLD activity has also been related to neuro-inflammatory states via astrogliosis (63).
In line with this, a recently conducted lipidomic and genetic analysis in patients with MDD identified a shared genetic etiology between MDD and PC indicating markers of the PC-inflammation pathway as diagnostic markers for MDD (40). In addition, MDD patients were shown to demonstrate higher levels of AA and C-reactive protein (CRP; a marker for systemic inflammation) than those in HCs. In patients, AA correlates positively with CRP levels (64). Pro-inflammatory markers such as CRP have been shown to feature upstream in the inflammatory process, triggering PCs containing AA, which is subsequently released from the cell membrane via PLA2. This leaves necessary fatty acids available for prostaglandin production and further triggers subsequent pro-inflammatory processes (65). Pro-inflammatory markers were shown to increase PLA2 activity up to 14-fold (50). Recently, it has been shown that linoleic acid administration causes an increase in CRP secretion providing further evidence for a lipid-inflammation dependent pathway for MDD (57).
Therefore, it is suggested that lipidomic risk-profiles seem to predispose individuals to MDD and that these at-risk individuals subsequently become ill and show intensified glucocorticoid and inflammatory dysregulations. Thus, a testable model of the pathophysiology of MDD, as presented in Figure 2, emerges, suggesting that dysregulated candidate lipid networks increase the risk of MDD via direct effects on neurosignaling and their causal influence on hyper-cortisolism and systemic low grade inflammation (64).
Conclusion
Due to the clear integration of multiple identified lipids in the pathophysiology of MDD based on our systematic review, lipidomics emerges as a powerful approach to identify a diagnostic biomarker for MDD, with promising results stemming from pioneering studies. However, prediction power is currently insufficient to extract clinically applicable lipid biomarkers for MDD. Furthermore, several differential lipid species were identified for MDD, although somewhat inconsistent between studies (see Supplementary Table 2). However, the emerging pattern of reduced PC, PE, PI, and increased LPC, LPE, Cer, TAG, and DAG levels in response to depressed states needs to be replicated in independent studies using lipidomics analysis (20). Recent advances in the field of computational psychiatry may further increase the predictive power of lipidomics for MDD (66), while quantitative analyses of identified lipid species and sub-species are needed to evaluate the most predictive lipids. However, potential pathways linking the lipidome to MDD highlight the inflammatory system as well as the glucocorticoid system as important mediators (see Figure 2). Furthermore, the one problem that all of the presented human lipidomic studies in MDD have in common is their cross-sectional nature. Not a single longitudinal lipidomic study in patients with MDD has been carried out so far. Thus, no data on possible alterations of lipid profiles with regard to changes of the disease state are available to date. Although potential molecular pathways linking MDD with lipid profiles via inflammatory and steroidal dysregulations have been suggested (34, 44), only longitudinal lipidomic studies in large human samples including classical inflammatory markers as well as glucocorticoids will provide clarification as to whether the proposed pathophysiologic model can be validated o r not. Finally, lipid pathway enrichment analyses of the identified lipids such as provided by LIPEA (67) will further shed light on the underlying mechanisms of how perturbed lipid pathways are contributing to MDD paving the way to precision medicine and thus providing novel drug targets for more effective treatments in MDD.
Author Contributions
AW designed and supported the systematic review and wrote the first draft of the manuscript. CC, KS, MG, CD, and CK contributed with important intellectual content and edited subsequent versions of the manuscript. SW supported the systematic review and created the art work.
Funding
We acknowledge support by the German Research Foundation and the Open Access Publication Funds of the TU Dresden.
Conflict of Interest Statement
KS is shareholder and CEO of Lipotype GmbH. MG is employees of Lipotype GmbH.
The remaining authors declare that the research was conducted in the absence of any commercial or financial relationships that could be construed as a potential conflict of interest.
Acknowledgments
We would like to thank B.Sc. Johannes Steffen for supporting and conducting the systematic search of lipidomic studies in MDD/rodent models of depression. We would also like to thank Laura Mugford for language editing.
Supplementary Material
The Supplementary Material for this article can be found online at: https://www.frontiersin.org/articles/10.3389/fpsyt.2018.00459/full#supplementary-material
References
1. Kessler RC. The costs of depression. Psychiatr Clin N Am. (2012) 35:1–14. doi: 10.1016/j.psc.2011.11.005
2. Merikangas K, Ames M, Cui L, Stang P, Ustun T, Von Korff M, et al. The impact of comorbidity of mental and physical conditions on role disability in the us adult household population. Arch Gen Psychiatry (2007) 64:1180–8. doi: 10.1001/archpsyc.64.10.1180
3. American Psychiatric Association. Diagnostic and Statistical Manual of Mental Disorders (DSM-5®), 5th Edn. Arlington, VA: American Psychiatric Pub (2013).
4. World Health Organisation (WHO). Depression and Other Common Mental Disorders Global Health Estimates. Geneva: World Health Organisation (2017).
5. Vos T, Abajobir AA, Abbafati C, Abbas KM, Abate KH, Abd-Allah F, et al. Global, regional, and national incidence, prevalence, and years lived with disability for 328 diseases and injuries for 195 countries, 1990-2016: a systematic analysis for the Global Burden of Disease Study 2016. Lancet (2017) 390:1211–59. doi: 10.1016/S0140-6736(17)32154-2
6. Bogdan R, Nikolova YS, Pizzagalli DA. Neurogenetics of depression: a focus on reward processing and stress sensitivity. Neurobiol Dis. (2013) 52:12–23. doi: 10.1016/j.nbd.2012.05.007
7. Akil H, Gordon J, Hen R, Javitch J, Mayberg H, Mcewen B, et al. Treatment resistant depression: a multi-scale, systems biology approach. Neurosci Biobehav Rev. (2017) 84:272–88. doi: 10.1016/j.neubiorev.2017.08.019
8. Wager TD, Woo CW. Imaging biomarkers and biotypes for depression. Nat Med. (2017) 23:16–7. doi: 10.1038/nm.4264
9. Sanislow CA, Pine DS, Quinn KJ, Kozak MJ, Garvey MA, Heinssen RK, et al. Developing constructs for psychopathology research: research domain criteria. J Abnorm Psychol. (2010) 119:631–9. doi: 10.1037/a0020909
10. Regier DA, Kupfer DJ, Kuhl EA, Greiner L, Kraemer HC. DSM-5 field trials in the United States and Canada, part i: study design, sampling strategy, implementation, and analytic approaches. Am J Psychiatry (2013) 170:59–70. doi: 10.1176/appi.ajp.2012.12070999
11. Pariante CM. Why are depressed patients inflamed? A reflection on 20 years of research on depression, glucocorticoid resistance and inflammation. Eur Neuropsychopharmacol. (2017) 27:554–9. doi: 10.1016/j.euroneuro.2017.04.001
12. Simons K. How can Omic Science be improved? Proteomics (2018) 18:e1800039. doi: 10.1002/pmic.201800039
13. Shevchenko A, Simons K. Lipidomics: coming to grips with lipid diversity. Nat Rev Mol Cell Biol. (2010) 11:593–8. doi: 10.1038/nrm2934
14. Cannistraci CV, Ravasi T, Montevecchi FM, Ideker T, Alessio M. Nonlinear dimension reduction and clustering by minimum curvilinearity unfold neuropathic pain and tissue embryological classes. Bioinformatics (2010) 26:531–9. doi: 10.1093/bioinformatics/btq376
16. Ciucci S, Ge Y, Durán C, Palladini A, Jiménez-jiménez V, Martínez-sánchez LM, et al. Enlightening discriminative network functional modules behind Principal Component Analysis separation in differential-omic science studies. Sci Rep. (2017) 7:43946. doi: 10.1038/srep43946
17. Yugi K, Kubota H, Hatano A, Kuroda S. Trans-omics: how to reconstruct biochemical networks across multiple ‘Omic' layers. Trends Biotechnol. (2016) 34:276–90. doi: 10.1016/j.tibtech.2015.12.013
18. Drysdale AT, Grosenick L, Downar J, Dunlop K, Mansouri F, Meng Y, et al. Resting-state connectivity biomarkers define neurophysiological subtypes of depression. Nat Med. (2016) 23:28–38. doi: 10.1038/nm.4246
19. Hyde CL, Nagle MW, Tian C, Chen X, Paciga SA, Wendland JR, et al. Identification of 15 genetic loci associated with risk of major depression in individuals of European descent. Nat Genet. (2016) 48:1031–6. doi: 10.1038/ng.3623
20. Surma MA, Herzog R, Vasilj A, Klose C, Christinat N, Morin-Rivron D, et al. An automated shotgun lipidomics platform for high throughput, comprehensive, and quantitative analysis of blood plasma intact lipids. Eur J lipid Sci Technol. (2015) 117:1540–9. doi: 10.1002/ejlt.201500145
21. Bowden JA, Heckert A, Ulmer CZ, Jones CM, Koelmel JP, Abdullah L, et al. Harmonizing lipidomics : NIST interlaboratory comparison exercise for lipidomics using SRM 1950–metabolites in frozen human plasma. J Lipid Res. (2017) 58:2275–88. doi: 10.1194/jlr.M079012
22. Hannun YA, Obeid LM. Principles of bioactive lipid signalling: lessons from sphingolipids. Nat Rev Mol Cell Biol. (2008) 9:139–50. doi: 10.1038/nrm2329
23. Sud M, Fahy E, Cotter D, Brown A, Dennis EA, Glass CK, et al. LMSD: LIPID MAPS structure database. Nucleic Acids Res. (2007) 35:D527–32. doi: 10.1093/nar/gkl838
24. Quehenberger O, Dennis EA. The human plasma lipidome. N Engl J Med. (2011) 365:1812–23. doi: 10.1056/NEJMra1104901
25. Meer G Van, Voelker DR, Feigenson GW. Membrane lipids: where they are and how they behave. Nat Rev Mol Cell Biol. (2008) 9:112–24. doi: 10.1038/nrm2330
26. Schneider M, Levant B, Reichel M, Gulbins E, Kornhuber J, Müller CP. Lipids in psychiatric disorders and preventive medicine. Neurosci Biobehav Rev. (2017) 76:336–62. doi: 10.1016/j.neubiorev.2016.06.002
27. Tsui-Pierchala BA, Encinas M, Milbrandt J, Johnson EM. Lipid rafts in neuronal signaling and function. Trends Neurosci. (2002) 25:412–7. doi: 10.1016/S0166-2236(02)02215-4
28. Ledesma MD, Martin MG, Dotti CG. Lipid changes in the aged brain: effect on synaptic function and neuronal survival. Prog Lipid Res. (2012) 51:23–35. doi: 10.1016/j.plipres.2011.11.004
29. Tansey MG, Baloh RH, Milbrandt J, Johnson EM. GFRα-mediated localization of RET to lipid rafts is required for effective downstream signaling, differentiation, and neuronal survival. Neuron (2000) 25:611–23. doi: 10.1016/S0896-6273(00)81064-8
30. Simons K, Gerl MJ. Revitalizing membrane rafts: new tools and insights. Nat Rev Mol Cell Biol. (2010) 11:688–99. doi: 10.1038/nrm2977
31. Hallahan B, Ryan T, Hibbeln JR, Murray IT, Glynn S, Ramsden CE, et al. Efficacy of omega-3 highly unsaturated fatty acids in the treatment of depression. Br J Psychiatry (2016) 209:192–201. doi: 10.1192/bjp.bp.114.160242
32. Parekh A, Smeeth D, Milner Y, Thuret S. The Role of lipid biomarkers in major depression. (2017) 5:E5. doi: 10.3390/healthcare5010005.
33. Gulbins E, Palmada M, Reichel M, Lüth A, Böhmer C, Amato D, et al. Acid sphingomyelinase – ceramide system mediates effects of antidepressant drugs. Nat Med. (2013) 19:2–9. doi: 10.1038/nm.3214
34. Oliveira TG, Chan RB, Bravo F V, Miranda A, Silva RR, Zhou B, et al. The impact of chronic stress on the rat brain lipidome. Mol Psychiatry (2016) 21:80–88. doi: 10.1038/mp.2015.14
35. Aimo L, Liechti R, Hyka-nouspikel N, Kuznetsov D, Niknejad A, Gleizes A, et al. The SwissLipids knowledgebase for lipid biology. Bioinformatics (2015) 31:2860–6. doi: 10.1093/bioinformatics/btv285
36. Chan P, Suridjan I, Mohammad D, Herrmann N, Mazereeuw G, Hillyer LM, et al. Novel phospholipid signature of depressive symptoms in patients with coronary artery disease. J Am Heart Assoc. (2018) 7:e008278. doi: 10.1161/JAHA.117.008278
37. Demirkan A, Isaacs A, Ugocsai P, Liebisch G, Struchalin M, Rudan I, et al. Plasma phosphatidylcholine and sphingomyelin concentrations are associated with depression and anxiety symptoms in a Dutch family-based lipidomics study Ays. J Psychiatr Res. (2013) 47:357–62. doi: 10.1016/j.jpsychires.2012.11.001
38. Hennebelle M, Otoki Y, Yang J, Hammock BD, Levitt AJ, Taha AY, et al. Altered soluble epoxide hydrolase-derived oxylipins in patients with seasonal major depression: an exploratory study. Psychiatry Res. (2017) 252:94–101. doi: 10.1016/j.psychres.2017.02.056
39. Kim EY, Lee JW, Lee MY, Kim SH, Mok HJ, Ha K, et al. Serum lipidomic analysis for the discovery of biomarkers for major depressive disorder in drug-free patients. Psychiatry Res. (2018) 265:174–82. doi: 10.1016/j.psychres.2018.04.029
40. Knowles EEM, Huynh K, Meikle PJ, Göhring HHH, Olvera RL, Mathias SR, et al. The lipidome in major depressive disorder : shared genetic influence for ether-phosphatidylcholines, a plasma-based phenotype related to inflammation, and disease risk. Eur Psychiatry (2017) 43:44–50. doi: 10.1016/j.eurpsy.2017.02.479
41. Kuwano N, Kato TA, Setoyama D, Sato-Kasai M, Shimokawa N, Hayakawa K, et al. Tryptophan-kynurenine and lipid related metabolites as blood biomarkers for first-episode drug-naïve patients with major depressive disorder: an exploratory pilot case-control study. J Affect Disord. (2018) 231:74–82. doi: 10.1016/j.jad.2018.01.014
42. Liu X, Zheng P, Zhao X, Zhang Y, Hu C, Li J, et al. Plasma lipidomics reveals potential lipid markers of major depressive disorder. Anal Bioanal Chem. (2016) 408:6497–507. doi: 10.1007/s00216-016-9768-5
43. Chen S, Wei C, Gao P, Kong H, Jia Z, Hu C, et al. Effect of Allium macrostemon on a rat model of depression studied by using plasma lipid and acylcarnitine profiles from liquid chromatography/mass spectrometry. J Pharm Biomed Anal. (2014) 89:122–9. doi: 10.1016/j.jpba.2013.10.045
44. Faria R, Santana MM, Aveleira CA, Maciel E, Melo T, Santinha D, et al. Alterations in phospholipidomic profile in the brain of mouse model of depression induced by chronic unpredictable stress. Neuroscience (2014) 273:1–11. doi: 10.1016/j.neuroscience.2014.04.042
45. Lee LHW, Shui G, Farooqui AA, Wenk MR, Tan CH, Ong WY. Lipidomic analyses of the mouse brain after antidepressant treatment: evidence for endogenous release of long-chain fatty acids? Int J Neuropsychopharmacol. (2009) 12:953–64. doi: 10.1017/S146114570900995X
46. Lee LHW, Tan CH, Shui G, Wenk MR, Ong WY. Role of prefrontal cortical calcium independent phospholipase A2 in antidepressant-like effect of maprotiline. Int J Neuropsychopharmacol. (2012) 15:1087–98. doi: 10.1017/S1461145711001234
47. Moher D, Liberati A, Tetzlaff J, Altman DG. Preferred reporting items for systematic reviews and meta-analyses: the PRISMA statement. J Clin Epidemiol. (2009) 62:1006–12. doi: 10.1016/j.jclinepi.2009.06.005
48. Kim W, Lee M, Park M, Jung J, Uhlinger DJ, Kwak J. Dexamethasone enhances phospholipase D activity in M-1 cells. Exp Mol Med. (2000) 32:170–7. doi: 10.1038/emm.2000.28
49. Dolinsky VW, Douglas DN, Lehner R, Vance DE. Regulation of the enzymes of hepatic microsomal triacylglycerol lipolysis and re-esterification by the glucocorticoid dexamethasone. Biochem J. (2004) 974:967–74. doi: 10.1042/bj20031320
50. Pruzanski W, Wilmore DW, Suffredini A, Martich GD, Hoffman AGD, Browning JL, et al. Hyperphosoholipasemia A2 in human volunteers challenged with intravenous endotoxin. Inflammation (1992) 16:561–70. doi: 10.1007/BF00918980
51. Mondelli V, Vernon AC, Turkheimer F, Dazzan P, Pariante CM. Brain microglia in psychiatric disorders. Lancet Psychiatry (2017) 4:563–72. doi: 10.1016/S2215-0366(17)30101-3
52. Liu X, Zheng P, Zhao X, Zhang Y, Hu C, Li J, et al. Discovery and validation of plasma biomarkers for major depressive disorder classification based on liquid chromatography–mass spectrometry. J Proteome Res. (2015) 14:2322–30. doi: 10.1021/acs.jproteome.5b00144
53. Davis J, Goadrich M. The relationship between precision-recall and roc curves. In: Proceedings of the 23rd International conference on Machine Learning. New York, NY (2006) 233–40. doi: 10.1145/1143844.1143874
54. Liu Y, Yieh L, Yang T, Drinkenburg W, Peeters P, Steckler T, et al. Metabolomic biosignature differentiates melancholic depressive patients from healthy controls. BMC Genomics (2016) 17:669–86. doi: 10.1186/s12864-016-2953-2
55. Berglund L, Sacks F, Brunzell JD. Risk factors for cardiovascular disease : renewed interest in triglycerides Risk factors for cardiovascular disease : renewed interest in triglycerides. Clin Lipidol. (2017) 8:1–4. doi: 10.2217/clp.12.81
56. Cruciani-Guglielmacci C, Magnan C. Brain lipoprotein lipase as a regulator of energy balance. Biochimie (2017) 143:51–5. doi: 10.1016/j.biochi.2017.07.012
57. Smedman A, Basu S, Jovinge S, Fredrikson GN, Vessby B. Conjugated linoleic acid increased C-reactive protein in human subjects. Br J Nutr. (2005) 94:791–5. doi: 10.1079/BJN20041419
58. Su H, Liu R, Chang M, Huang J, Wang X. Dietary linoleic acid intake and blood inflammatory markers: a systematic review and meta-analysis of randomized controlled trials. Food Funct. (2017) 8:3091–103. doi: 10.1039/C7FO00433H
59. Burke JE, Dennis EA. Phospholipase A2 structure/function, mechanism, and signaling. J Lipid Res. (2009) 50:S237–42. doi: 10.1194/jlr.R800033-JLR200
60. Weng R, Shen S, Burton C, Yang L, Nie H, Tian Y, et al. Lipidomic profiling of tryptophan hydroxylase 2 knockout mice reveals novel lipid biomarkers associated with serotonin deficiency. Anal Bioanal Chem. (2016) 408:2963–73. doi: 10.1007/s00216-015-9256-3
61. Mocking RJT, Ruhe HG, Assies J, Lok A, Koeter MWJ, Visser I, et al. Relationship between the hypothalamic–pituitary–adrenal-axis and fatty acid metabolism in recurrent depression. Psychoneuroendocrinology (2013) 38:1607–17. doi: 10.1016/j.psyneuen.2013.01.013
62. Mocking RJT, Verburg HF, Westerink AM, Assies J, Vaz FM, Koeter MWJ, et al. Fatty acid metabolism and its longitudinal relationship with the hypothalamic–pituitary–adrenal axis in major depression : associations with prospective antidepressant response. Psychoneurendocrinology (2015) 59:1–13. doi: 10.1016/j.psyneuen.2015.04.027
63. Klein J. Functions and pathophysiological roles of phospholipase D in the brain. J Neurochem. (2005) 94:1473–87. doi: 10.1111/j.1471-4159.2005.03315.x
64. Mocking RJT, Nap TS, Westerink AM, Assies J, Vaz FM, Koeter MWJ, et al. Biological profiling of prospective antidepressant response in major depressive disorder: associations with (neuro) inflammation, fatty acid metabolism, and amygdala-reactivity. Psychoneuroendocrinology (2017) 79:84–92. doi: 10.1016/j.psyneuen.2017.02.019
65. Lozanski G, Berthier F, Kushner I. The sphingomyelin–ceramide pathway participates in cytokine regulation of C-reactive protein and serum amyloid A, but not a-fibrinogen. Biochem J. (1997) 328:271–5. doi: 10.1042/bj3280271
66. Huys QJM. Advancing clinical improvements for patients using the theory-driven and data-driven branches of computational psychiatry. JAMA Psychiatry (2018) 75:225–6. doi: 10.1001/jamapsychiatry.2017.4246
Keywords: major depressive disorder, depression, lipidomics, machine learning, computational psychiatry, cortisol, inflammation, prostaglandin
Citation: Walther A, Cannistraci CV, Simons K, Durán C, Gerl MJ, Wehrli S and Kirschbaum C (2018) Lipidomics in Major Depressive Disorder. Front. Psychiatry 9:459. doi: 10.3389/fpsyt.2018.00459
Received: 07 May 2018; Accepted: 04 September 2018;
Published: 15 October 2018.
Edited by:
Stefan Borgwardt, Universität Basel, SwitzerlandReviewed by:
Jennifer C. Felger, Emory University, United StatesStefania Schiavone, University of Foggia, Italy
Copyright © 2018 Walther, Cannistraci, Simons, Durán, Gerl, Wehrli and Kirschbaum. This is an open-access article distributed under the terms of the Creative Commons Attribution License (CC BY). The use, distribution or reproduction in other forums is permitted, provided the original author(s) and the copyright owner(s) are credited and that the original publication in this journal is cited, in accordance with accepted academic practice. No use, distribution or reproduction is permitted which does not comply with these terms.
*Correspondence: Andreas Walther, YW5kcmVhcy53YWx0aGVyQHR1LWRyZXNkZW4uZGU=