- 1National Institute of Mental Health, Klecany, Czechia
- 2Third Faculty of Medicine, Charles University, Prague, Czechia
Repetitive transcranial magnetic stimulation (rTMS) is an effective treatment for depressive disorder, with outcomes approaching 45–55% response and 30–40% remission. Eligible predictors of treatment outcome, however, are still lacking. Few studies have investigated quantitative electroencephalography (QEEG) parameters as predictors of rTMS treatment outcome and none of them have addressed the source localization techniques to predict the response to low-frequency rTMS (LF rTMS). We investigated electrophysiological differences based on scalp EEG data and inverse solution method, exact low-resolution brain electromagnetic tomography (eLORETA), between responders and non-responders to LF rTMS in resting brain activity recorded prior to the treatment. Twenty-five unmedicated depressive patients (mean age of 45.7 years, 20 females) received a 4-week treatment of LF rTMS (1 Hz; 20 sessions per 600 pulses; 100% of the motor threshold) over the right dorsolateral prefrontal cortex. Comparisons between responders (≥50% reduction in Montgomery-Åsberg Depression Rating Scale score) and non-responders were made at baseline for measures of eLORETA current density, spectral absolute power, and inter-hemispheric and intra-hemispheric EEG asymmetry. Responders were found to have lower current source densities in the alpha-2 and beta-1 frequency bands bilaterally (with predominance on the left side) in the inferior, medial, and middle frontal gyrus, precentral gyrus, cingulate gyrus, anterior cingulate, and insula. The most pronounced difference was found in the left middle frontal gyrus for alpha-2 and beta-1 bands (p < 0.05). Using a spectral absolute power analysis, we found a negative correlation between the absolute power in beta and theta frequency bands on the left frontal electrode F7 and the change in depressive symptomatology. None of the selected asymmetries significantly differentiated responders from non-responders in any frequency band. Pre-treatment reduction of alpha-2 and beta-1 sources, but not QEEG asymmetry, was found in patients with major depressive disorder who responded to LF rTMS treatment. Prospective trials with larger groups of subjects are needed to further validate these findings.
Introduction
Major depressive disorder (MDD) is one of the most common psychiatric disorders resulting in a lifetime disability. Despite recent advances in psychopharmacology, up to 30% of patients do not respond to antidepressant medications (1). Due to this limitation, several other therapeutic potentials have been introduced. Repetitive transcranial magnetic stimulation (rTMS) is a minimally invasive method that delivers magnetic pulses through a coil placed over the scalp. These pulses induce an electric field in the target cortex regions associated with alterations in neurotransmitters’ release and metabolism or gene expression (2, 3). Up to 20–40% of pharmaco-resistant patients with MDD respond well to rTMS (4). rTMS in MDD treatment has mostly been applied by delivering stimulation at a high frequency (5-20 Hz; HF rTMS) to the left dorsolateral prefrontal cortex (DLPFC) for a period of 2 to 9 weeks (5). A similar effect may be achieved with a low-frequency (1 Hz, LF rTMS) stimulation over the right DLPFC (6). In order to improve clinical outcomes for patients, it is important to assess the potential response to rTMS treatment prior to its application.
Antidepressant rTMS therapy usually utilizes the left-sided DLPFC high-frequency stimulation and rarely the right-sided DLPFC low-frequency stimulation. The rationale being that the former mainly has an excitatory net effect while the latter, an inhibitory net effect. The theoretical reasoning behind the effort to enhance the activity or inhibition of one of the key neuronal limbic structure (based on its connectivity profile to a sub-system including the anterior cingulum, hippocampus, or amygdala), is based on several findings that show that the left-sided DLPFC activity is increased after antidepressant therapy (7–10). In terms of inter-hemispheric connectivity, the ascertainment for post-treatment increase in left-sided activity is consistent with findings that confirm the reduced right-sided DLPFC activity after treatment for the depressive disease (11, 12).
In order to improve personalized treatment for MDD, studies have attempted to detect biomarkers derived from quantitative electroencephalography (QEEG)—for in-depth review see (13, 14). The individual findings can be broken down according to the QEEG variables detected and their frequency band. Typical QEEG variables (alpha, beta, and theta bands) with a predictive value include: relative or absolute alpha, beta and theta power, hemispheral alpha asymmetry, frontal or parietal current source density, beta or theta cordance, coherence, and connectivity (14–22). As long as delays in finding effective treatments through trial and error continue to pose a burden on patients, studies that focus not only on discriminating patients from the healthy subjects but also predict treatment outcomes, are of particular importance. A recent study showed that patients responding to treatment with escitalopram exhibited elevated absolute alpha power in their left hemisphere while non-responders demonstrated the opposite (23). Greater right frontal alpha is associated with response to escitalopram and sertraline, but only for females (24, 25) while less alpha current source density in posterior areas has been associated with nonresponse in unmedicated depressed patients (26, 27). Moreover, successful antidepressant treatment of patients with more severe depression is accompanied by an increase in the left DLPFC and amygdala upper alpha EEG activity (28). Initially, larger beta spectral power values of EEG were associated with the high manifestation of residual depressive symptoms and non-responsivity after the treatment (29, 30), and the smaller beta activity at the frontotemporal region was associated with greater reductions in depressive psychopathology after paroxetine treatment (15).
Several recent QEEG studies have partially demonstrated the ability to predict rTMS treatment response (14, 31). Among the findings, a non-response or poorer response to treatment is characterized as: a change of power in theta (31–33), alpha (34), and beta (30) bands in the frontal cortex, prefrontal beta or theta cordance; decrease/unchanged between the baseline and first week of rTMS application (31, 35, 36); slowing of the anterior alpha peak or a decrease in its frequency (31); larger amplitude of evoked potential P300 (31); no increase in the Lempel-Ziv complex (from minute 1 to 2) in the alpha band (37); and a change in the beta phase-locking values (38).
Limited clinical data are available for the QEEG predictors of LF rTMS (1 Hz) treatment in MDD. For example, Valiulis et al. (39) found a marked shift of frontal alpha power asymmetry toward the right hemisphere, while no major effects were detected in the basic EEG band power. In our previous study, we found that responders to LF rTMS had a significantly reduced prefrontal theta cordance value after one week (40). However, measuring the reduction in cordance value relies on the observable change, once the therapy has begun. Therefore, it does not reflect the pre-treatment difference that indicates an antidepressant response to LF rTMS therapy. Due to the lack of studies on the pre-treatment difference between the responders and the non-responders for antidepressant treatment with LF-rTMS, we analyzed the baseline difference of EEG for selected frequency bands in the interhemispheric pairs of electrodes. We also aimed to identify the brain regions that distinguish responders from non-responders at baseline, before the LF rTMS treatment. For this purpose, we used an exact low-resolution brain electromagnetic tomography (eLORETA) in order to acquire the three-dimensional tomography of brain electrical activity (current densities) and localized multiple distributed cortical sources in different frequency bands from the resting scalp-recorded EEG data.
Methods
Subjects
For this study, we used data of 25 adult inpatients aged 18–65, that had participated in previously published studies (40, 41) (EudraCT no. 2005–000826-22) and were allocated to the rTMS arm. Patients included were with a positive diagnosis of MDD (recurrent or single episode) without psychotic symptoms according to the DSM IV criteria (42), confirmed using the Czech version 5.0.0 of the Mini-International Neuropsychiatric Interview (43) and had fulfilled at least stage I criteria for resistant depression (≥1 adequate antidepressant treatment in current episode) according to Thase and Rush (44). Only subjects who scored at least 20 points on the Montgomery-Åsberg Depression Rating Scale (MADRS) (45) and four points on the Clinical Global Impression (CGI) (46), were recruited. The exclusion criteria included suicidal risk, severe, and unstable medical illness (cardiovascular disease, neoplasms, endocrinology disorders, etc.), and neurological disorders (epilepsy and head trauma with loss of consciousness). We did not include any subjects who received electroconvulsive therapy within the 3 months prior to the start of the study and any subjects taking clozapine, olanzapine, lithium, carbamazepine, or valproate, which may affect EEG recordings. Evaluation of the adequacy of previous medication in the index episode was based on the Antidepressant Treatment History Form (47) with a score of at least 3 (more than 4 weeks of treatment inadequate dose). Standard physical examination, medical history evaluation, psychiatric examination, biochemistry, and EEG were performed in order to exclude risks and somatic or psychiatric comorbidities.
Prior to the study, the purpose and procedures of the research were carefully explained to the patients after which each participant provided his/her informed consent. The study was approved by the ethical committee of the Prague Psychiatric Centre/National Institute of Mental Health. The design and all procedures adhered to the latest version of the Declaration of Helsinki and ICH/Good Clinical Practice guidelines.
Treatment and Clinical Assessment
Following an initial washout period (5–9 days of the antidepressant-free period), eligible subjects received a 4-week treatment with low-frequency rTMS (1 Hz) consisting of twenty 10-min sessions (600 pulses per session) with a 100% stimulation intensity of the resting motor threshold. The rTMS was applied to the right DLPFC at a point 5 cm anterior in a parasagittal line to the motor threshold location (the left abductor pollicis brevis muscle) with the coil held tangentially to the scalp and its handle pointing back and away from the midline at 45°. The rTMS was delivered by a Magstim Super Rapid stimulator (Magstim, Whitland, UK) with an air-cooled, figure-eight 70-mm coil.
Anxiolytics and hypnotics, taken before the start of the rTMS treatment, were permitted in stable doses and not allowed eight hours prior to an EEG recording. No antidepressants, antipsychotics or anticonvulsants were permitted over the study period and at least five days before EEG recording and the first rTMS session. In case of severe anxiety and insomnia, zolpidem, and hydroxyzine were used.
Depressive symptoms were assessed using MADRS and CGI at baseline, week 1, and week 4. Treatment response was defined as a ≥50% reduction of MADRS total score at week four.
EEG Apparatus and Recording
The EEG examination was carried out regularly between 8 a.m. and 10 a.m. during the baseline visit, shortly preceding the first rTMS session. We used a standard 32-channel digital EEG amplifier BrainScope (M&I, Prague) with 21 Ag/AgCl surface electrodes placed according to the international 10/20 system and cross-referenced with the electrode situated between electrodes Fz and Cz in the midline (FCz). All scalp electrode impedances were below five kΩ. The EEG recording system acquired the data with 16-bit depth, 7.63 nV/bit resolution (i.e., ∼130 bit/μV), and a dynamic range of ±250 μV. The data-sampling rate was 250 Hz and the acquired signals were filtered with digital high- and low-pass filters at 0.15 and 70 Hz, respectively. The EEG was recorded for 10 min in a sound-attenuated room with subdued lighting, with patients in a semi-recumbent position and eyes closed in a maximally alert state. During the recording, the alertness was controlled. If the patterns of drowsiness appeared in the EEG, the subjects were aroused by acoustic stimuli. The data, 10 min in duration, were collected with an on-line computer system and were stored for further computer off-line analysis.
EEG Data Reduction and Analyses
Prior to the data analysis, artifact detection was visually performed to exclude all epochs containing eye blink, eye-rolling artifact, head movements, muscle artifacts, and decrease in alertness or epoch in which any channel that had a voltage deflection greater than ± 100 μV. EEG reviewer was blind to the treatment outcome. In addition, remaining EEG segments were subjected to drowsiness-detection and artifact removal function using the Neuroguide software (Neuroguide© NG-2.8.1, Applied Neuroscience Inc., St. Petersburg, FL), followed by the editing procedures where a template of “clean” artifact-free EEG (at least 10 sec in total duration) was selected. This template was then used to compute the matching amplitudes of EEG using flexible criteria of equal amplitudes to amplitudes that were ≤1.25 times larger. The decision as to which clean EEG sample multiplier is used was determined by the length of the sample, 60 sec as a minimum, visual inspection of the digital EEG, and when split-half reliability and test-retest reliability measurements were ≥0.95. Split-half reliability is the ratio of variance between the odd and even seconds of the time series of selected digital EEG while test re-test reliability is the ratio of variance between the first half vs. the second half of the selected EEG segments (variance=sum of the square of the deviation of each time point from the mean of the time points). Test-retest reliability >0.90 is commonly accepted in scientific literature and for a detailed description of editing, procedures see Thatcher et al. (48). Thus, from each EEG at least 60 sec (whole group mean 71.6 ± 14.9 sec); of vigilance-controlled, artifact-free, and highly reliable data were subjected to further analysis. The number of epochs, as well as the length of the samples processed, did not differ between the responders (69.1 ± 11.6 sec) and the non-responders (73.0 ± 16.7 sec) (p = 0.54). Fast Fourier transform (FFT) was used to calculate the absolute and relative power in each standard frequency bands (49). The power band analysis was based on the square rooted normalized values for the following bands: theta (4–8 Hz), alpha-1 (8–10 Hz), alpha-2 (10–12 Hz), and beta (12–20 Hz) obtained from each of the 19 electrodes (FP1/2, F3/4, F7/8, Fz, C3/4, Cz, T3/4, T5/6, P3/4, Pz, O1/2). Power asymmetry in each frequency band was calculated for inter-hemispheric (frontal F3-F4, central C3-C4, temporal T3-T4, parietal P3-P4, occipital O1-O2) and intra-hemispheric electrode pairs (on the left: frontotemporal F3-T3, F7-T3, fronto-parietal F3-P3, F7-P3; and analogously on the right: F4-T4, F8-T4, F4-P4, F8-P4). The values of asymmetry were calculated in the Neuroguide© NG-2.8.1 software using the formula “power asymmetry = (A - B)/(A + B) x 200”, where A = EEG channel 1 and B = EEG channel 2 (50).
eLORETA Calculation
Data analysis was performed using the exact low-resolution electromagnetic tomography – eLORETA (51, 52), an inverse solution technique that estimates the intracranial distribution of electrical activity (current density) in the cortex based on a three shell spherical head model co-registered with Talairach coordinates (53). We used the eLORETA-Key software (Key Institute for Brain-Mind Research, Zurich, Switzerland), available at http://www.uzh.ch/keyinst/loreta.htm. Using the eLORETA transformation matrix, cross spectra of each subject and for each frequency band were transformed to eLORETA files. This resulted in a corresponding 3D cortical distribution of the electrical neuronal generators for each subject. The computed eLORETA images reflect the cortical current density distribution in 6,239 voxels with a spatial resolution of 5 × 5 × 5 mm (52). The eLORETA algorithm has no localization of bias even in the presence of structured noise, which allows us to increase the localization accuracy, compared to the previous version of sLORETA (54). The advantage of eLORETA is that it belongs to a reference-free method of EEG analysis, therefore, determining the source distribution for EEG data is not affected by the selected electrode reference (51). LORETA is a well-proven inverse method as evidenced by its usage in numerous peer-reviewed publications (55). It has received considerable validation from studies that have combined LORETA with other more established localization methods such as the functional Magnetic Resonance Imaging (fMRI) (56–59), structural MRI (60), and Positron Emission Tomography (PET) (61–65). Furthermore, LORETA validation has been based on accepting as ground truth, the localization findings obtained from invasively implanted depth electrodes, as evident by several studies on epilepsy (66–68) and cognitive ERPs (69). Current density values were computed in eight frequency bands delta (0.5–3.5 Hz), theta (4–8 Hz), alpha-1 (8.5–10 Hz), alpha-2 (10.5–12 Hz), beta-1 (12.5–18 Hz), beta-2 (18.5–21 Hz), beta-3 (21.5–30 Hz), and gamma (35–45 Hz). These frequency bands were defined on the basis of factorial analysis of EEG records and are part of the guidelines and recommendations for the analysis of EEG records in the field of medical electrophysiological research (70).
Statistical Analyses
The response to rTMS was defined as a ≥50% reduction of the baseline MADRS total score. Responder’s and non-responder’s demographic and clinical data were compared using the Mann-Whitney U test and Fisher exact test, as appropriate. EEG power values were transformed by square root transformation and tested using the Kolmogorov-Smirnov test. After verifying that the data was normalized, the EEG power values and asymmetries were examined using repeated-measures analysis of variance (RM-ANOVA), separately for each power band (theta, alpha-1, alpha-2, beta) with the response status as a between-subject factor (responders, non-responders) and electrode site (nineteen electrodes) or electrode pair (13 asymmetries) as a within-subject factor. Once ANOVA was significant, an unpaired t-test was applied for each electrode or electrode pair between responders and non-responders, with correction for multiple comparisons following the Benjamini-Hochberg procedure with false discovery rate q of 0.20 (71). A similar correction was applied when calculating a Pearson correlation coefficient between the pre- vs post-treatment changes in the MADRS score and normalized power values from each electrode for each frequency band.
In the eLORETA analyses, the localization of the differences in baseline activity between the group of responders and non-responders was assessed using a voxel-by-voxel unpaired t-test of the eLORETA images, based on the power of estimated electric current density. In the resulting statistical three-dimensional images, cortical voxels showing significant differences were identified using a nonparametric approach (statistical nonparametric mapping or SnPM) via randomizations. This randomization strategy (72) determined the critical probability threshold values for the actual observed t-values, with correction for multiple comparisons across all voxels and all frequencies. A total of 5,000 permutations were used to determine significance for each randomization test. All bands were treated simultaneously in the t-between two-sided max-statistics test guaranteeing that the family-wise type-I error did not exceed the nominal level (0.05).
Results
Demographic Characteristics and Clinical Measures
In our study, 9 out of 25 patients (36%) responded to the rTMS treatment. Non-responders and responders were comparable in demographic and clinical characteristics except for the baseline severity of depression (expressed by MADRS total score), which was slightly more pronounced in the non-responder group. For numerical details, see Table 1.
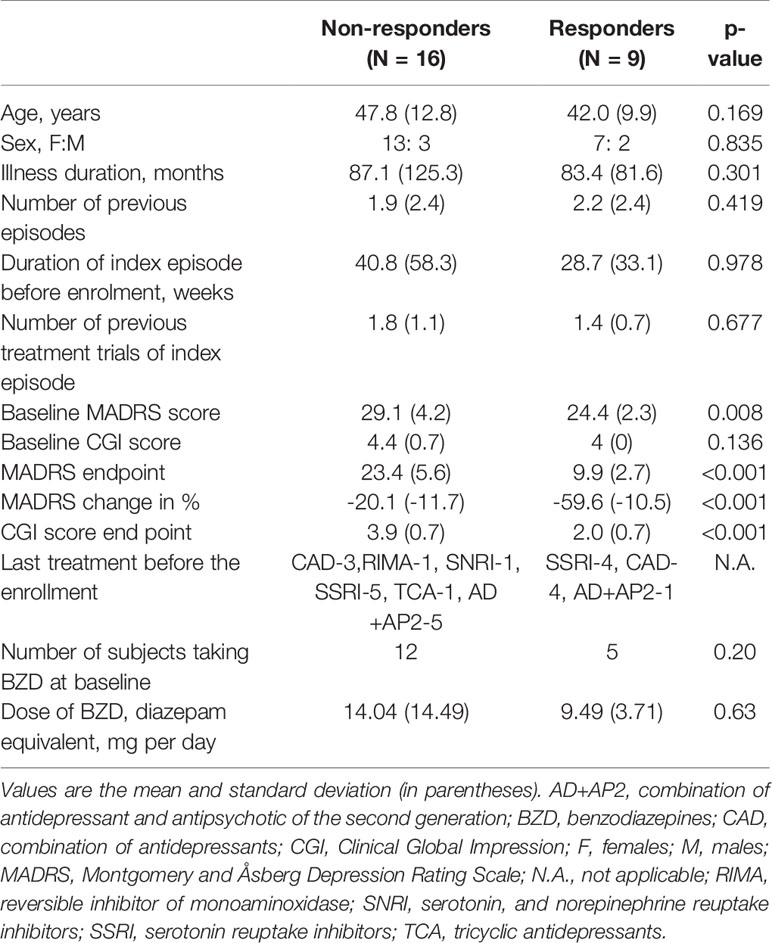
Table 1 Demographic and clinical characteristics of non-responders and responders to LF rTMS treatment.
Five out of 25 patients had experienced a first episode of MDD (responders: n = 1, non-responders: n = 4, p = 0.62, entire group: n = 25). Number of previous episodes was not statistically significant between the responders (min - max range: 0–8) and non-responders (min - max range: 0–8) (p = 0.42).
Majority of subjects (n = 16, 64%) underwent only one treatment trial prior to enrollment (no difference between groups, p = 1.0). Using a more stringent definition of resistance to treatment (≥2 previous antidepressant trials), nine subjects (36% of the sample) were considered as treatment-resistant with no differences between the groups (p = 1.0).
Spectral Power and Asymmetry
During initial data inspection, one subject from the responder's group demonstrated extremely distant (i.e., >3 standard deviations) power and asymmetry values and therefore was omitted as an outlier. Results of the repeated measures ANOVA did not reveal any significant interactions between the electrode absolute power and response status for the theta (F(18,396) = 1.32, p = 0.17), alpha-1 (F(18,396) = 1.21, p = 0.25), alpha-2 (F(18,396) = 0.79, p = 0.71), or beta (F(18,396) = 0.57, p = 0.92) frequency bands. Similarly, no significant interactions were observed for EEG asymmetries in the theta (F(12,276) = 0.95, p = 0.50), alpha-1 (F(12,276) = 0.73, p = 0.72), alpha-2 (F(12,276) = 1,11, p = 0,35), or beta (F(12,276) = 1,27, p = 0,23) frequency bands. However, a statistically significant negative correlation was observed between the change in the MADRS score (from baseline to week four) and the EEG absolute power at electrode F7 in theta (r = -0.57, p = 0.004) and beta (r = -0.60, p = 0.002) frequency bands. Scatterplots of these two significant correlations are presented in Figure 1.
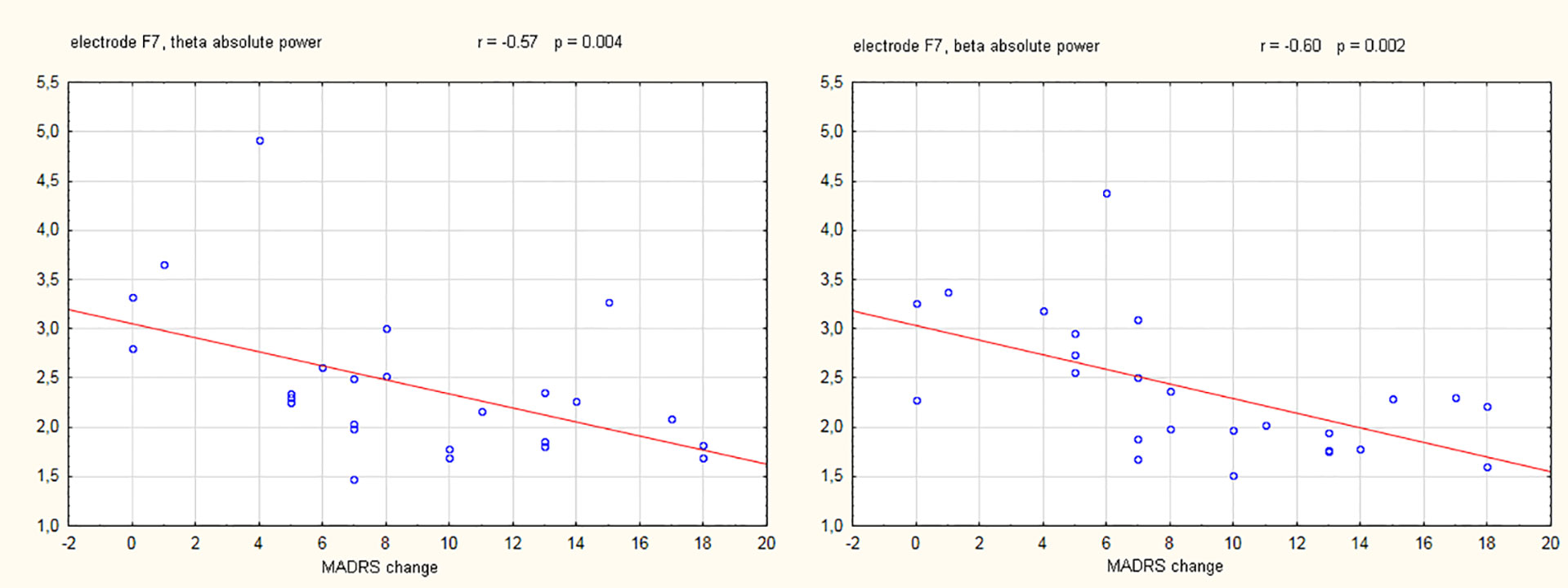
Figure 1 Scatterplots showing electroencephalography (EEG) absolute power values (µV2) in the theta and beta frequency band against the change of Montgomery-Åsberg depression rating scale (MADRS) score (baseline - week 4). The Pearson correlation coefficients (with corresponding p-values) for the relation between MADRS change and EEG power are also shown.
eLORETA
eLORETA analysis demonstrated that responders compared to non-responders, had significantly lower alpha-2 sources in the frontal lobe [Brodmann area (BA) 6, 8, 9, 32, 44, 45, 46], limbic lobe (BA 24, 33), and the insula (BA 13) (Figure 2 and Table 2). Similarly, responders showed significantly lower beta-1 current densities in the frontal lobe (BA 6, 8, 9, 44), limbic lobe (BA 24), and the insula (BA 13) (Figure 3 and Table 3). Most pronounced difference was found in the left middle frontal gyrus for the alpha-2 (t = -4.72, p < 0.05) and beta-1 frequency bands (t = -4.48, p < 0.05). Hemispheric distribution of the observed decreased activity in both frequency bands was localized more to the left and this asymmetry was substantially more noticeable for the beta-1 power (alpha-2: left hemisphere = 255 voxels, right hemisphere = 232 voxels; beta-1: left hemisphere = 344 voxels, right hemisphere = 53 voxels). To determine the clinical significance, we calculated the effect size based on Cohen's d for voxels with a maximum t-value for the alpha-2 (d = 1.79) and beta-1 (d = 1.74) frequency bands. To check if the abovementioned findings may reflect an unspecific improvement of depressive symptomatology or are related (and more specific) for rTMS, we calculated the correlation between the baseline MADRS and baseline eLORETA, where we did not observe any significance. However, the change in depressive symptomatology, expressed by the change in MADRS score, correlated negatively with the alpha-2 sources, mainly in the left hemisphere, with the highest negative correlation (r = -0.637; p < 0.05) localized in the anterior cingulate voxel (BA 24; x = 5, y = 30, z = 12). For more details see Figure 4 and Table 4.
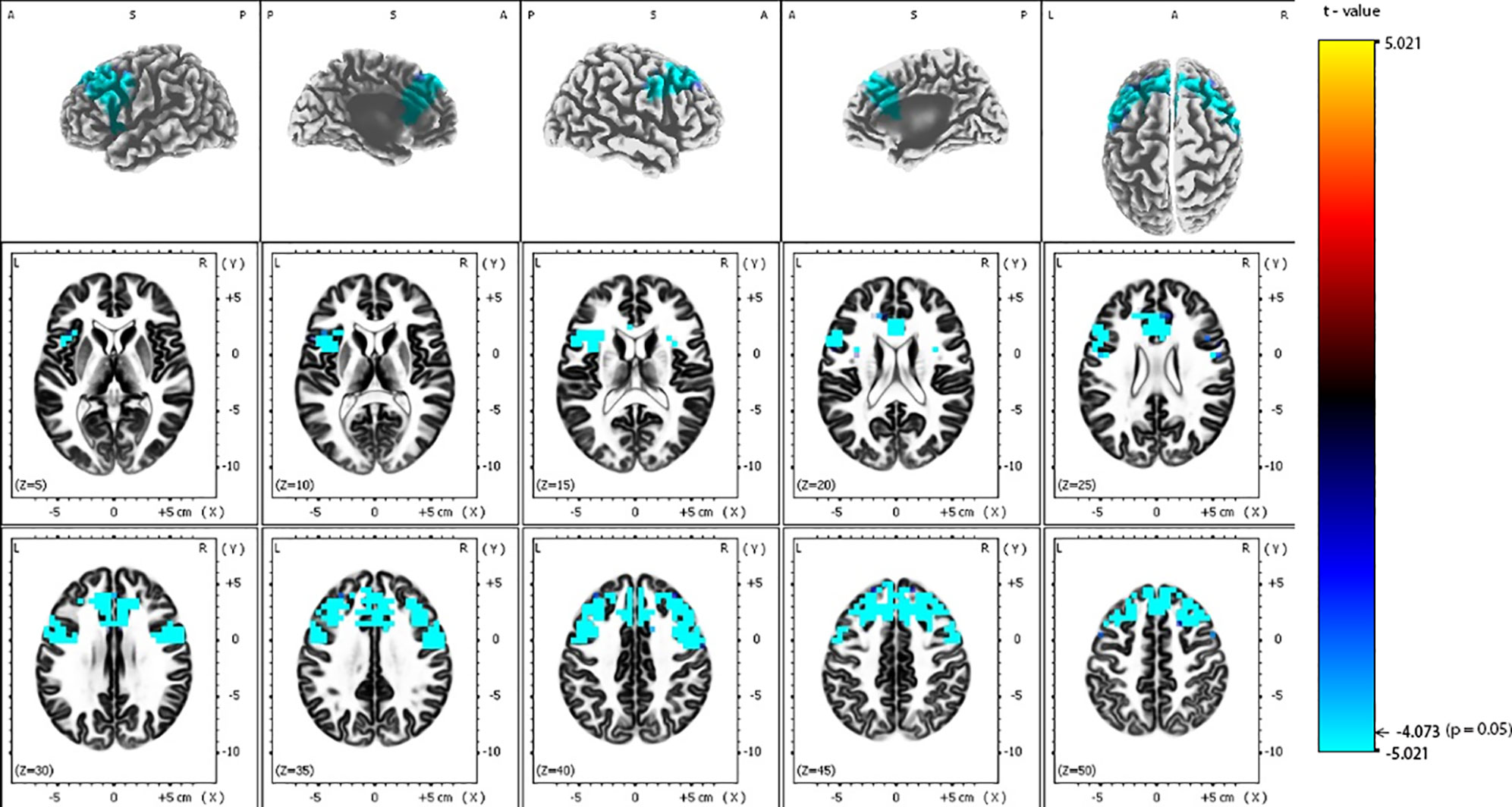
Figure 2 Comparison of baseline exact low-resolution brain electromagnetic tomography (eLORETA) current density for alpha-2 frequency band (10.5–12 Hz) between responders and non-responders to repetitive transcranial magnetic stimulation (rTMS) treatment. Surface-rendered (upper row) and axial sliced (middle and lower row) images depicting statistical nonparametric maps (SnPMs) are based on voxel-by-voxel independent t-tests of the eLORETA images based on the log-transformed power of the estimated electric current density. A significant decrease (p < 0.05) in the baseline alpha-2 eLORETA current density for rTMS responders is shown in the bilateral frontal lobes, insula and anterior cingulate. Structural anatomy is shown in grayscale (A, anterior; P, posterior; S, superior; L, left; R, right); x, y, z = Talairach coordinates. The threshold value (t = -4.073) for statistical significance (corresponding to p = 0.05) is reported at the color scale on the right side of the figure.
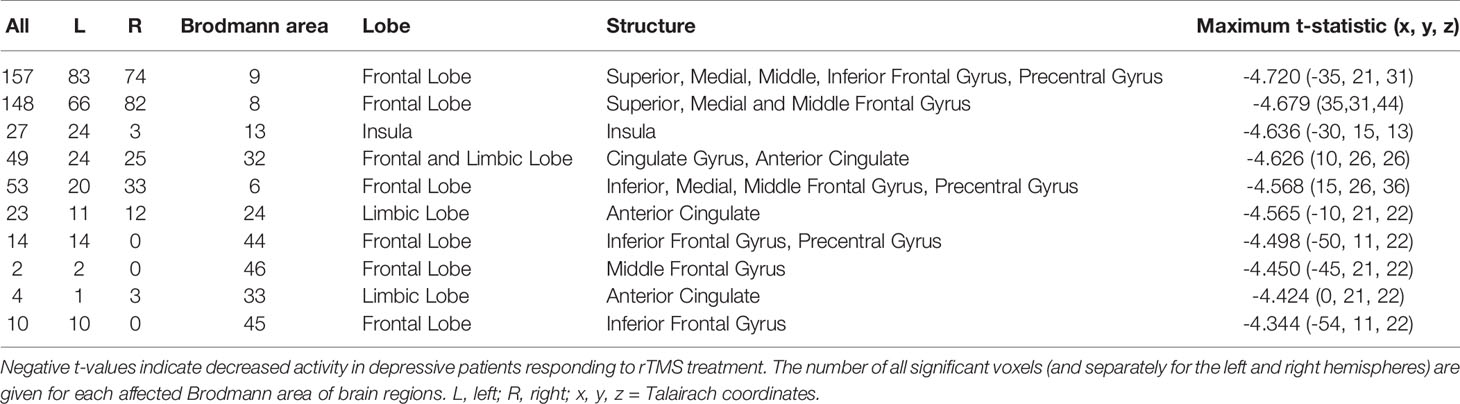
Table 2 Number of voxels, Brodmann areas and anatomical region where significant differences in baseline exact low-resolution brain electromagnetic tomography (eLORETA) current density were found for alpha-2 frequency band (10.5–12 Hz), comparing responders and non-responders to repetitive transcranial magnetic stimulation (rTMS) treatment.
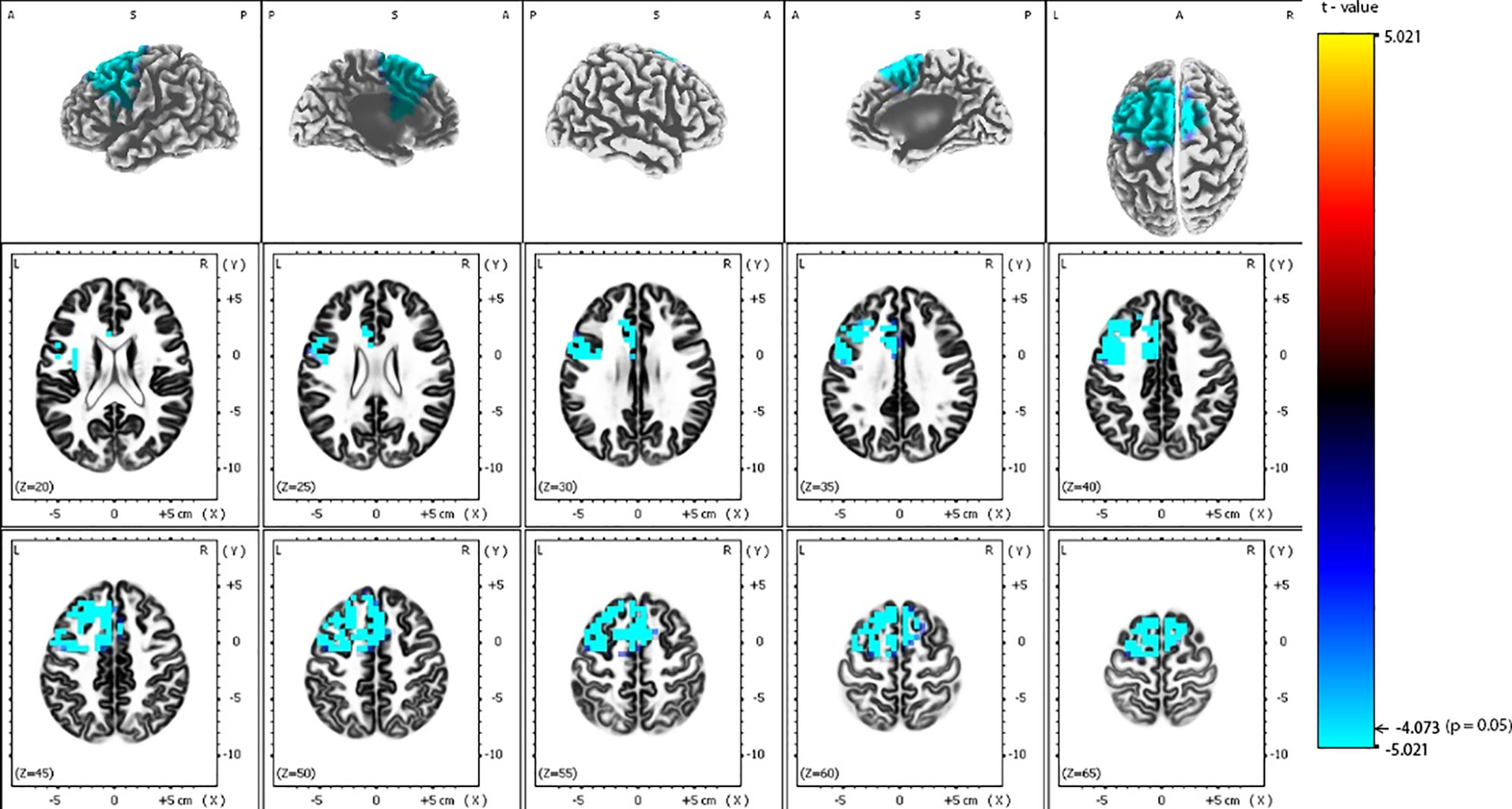
Figure 3 Comparison of baseline exact low-resolution brain electromagnetic tomography (eLORETA) current density for beta-1 frequency band (12.5–18 Hz) between responders and non-responders to repetitive transcranial magnetic stimulation (rTMS) treatment. Surface-rendered (upper row) and axial sliced (middle and lower row) images depicting statistical nonparametric maps (SnPMs) are based on voxel-by-voxel independent t-tests of the eLORETA images based on the log-transformed power of the estimated electric current density. A significant decrease (p < 0.05) in the baseline beta-1 eLORETA current density for rTMS responders is shown mainly in the left frontal and limbic lobes, left insula and left anterior cingulate. Structural anatomy is shown in grayscale (A, anterior; P, posterior, S, superior; L, left, R, right); x, y, z = Talairach coordinates. The threshold value (t = -4.073) for statistical significance (corresponding to p = 0.05) is reported at the color scale on the right side of the figure.
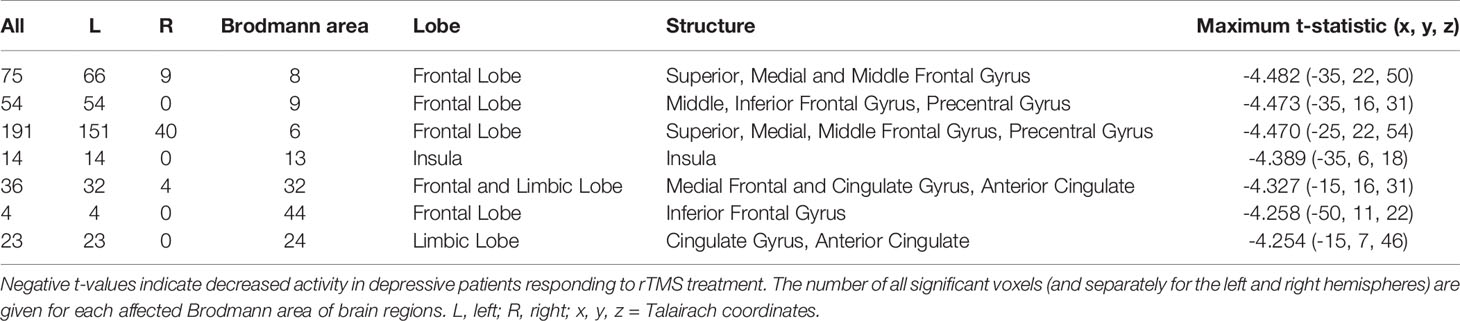
Table 3 Number of voxels, Brodmann areas and anatomical region where significant differences in baseline standardized low-resolution brain electromagnetic tomography (sLORETA) current density were found for beta-1 frequency band (12.5–18 Hz), comparing responders and non-responders to repetitive transcranial magnetic stimulation (rTMS) treatment.
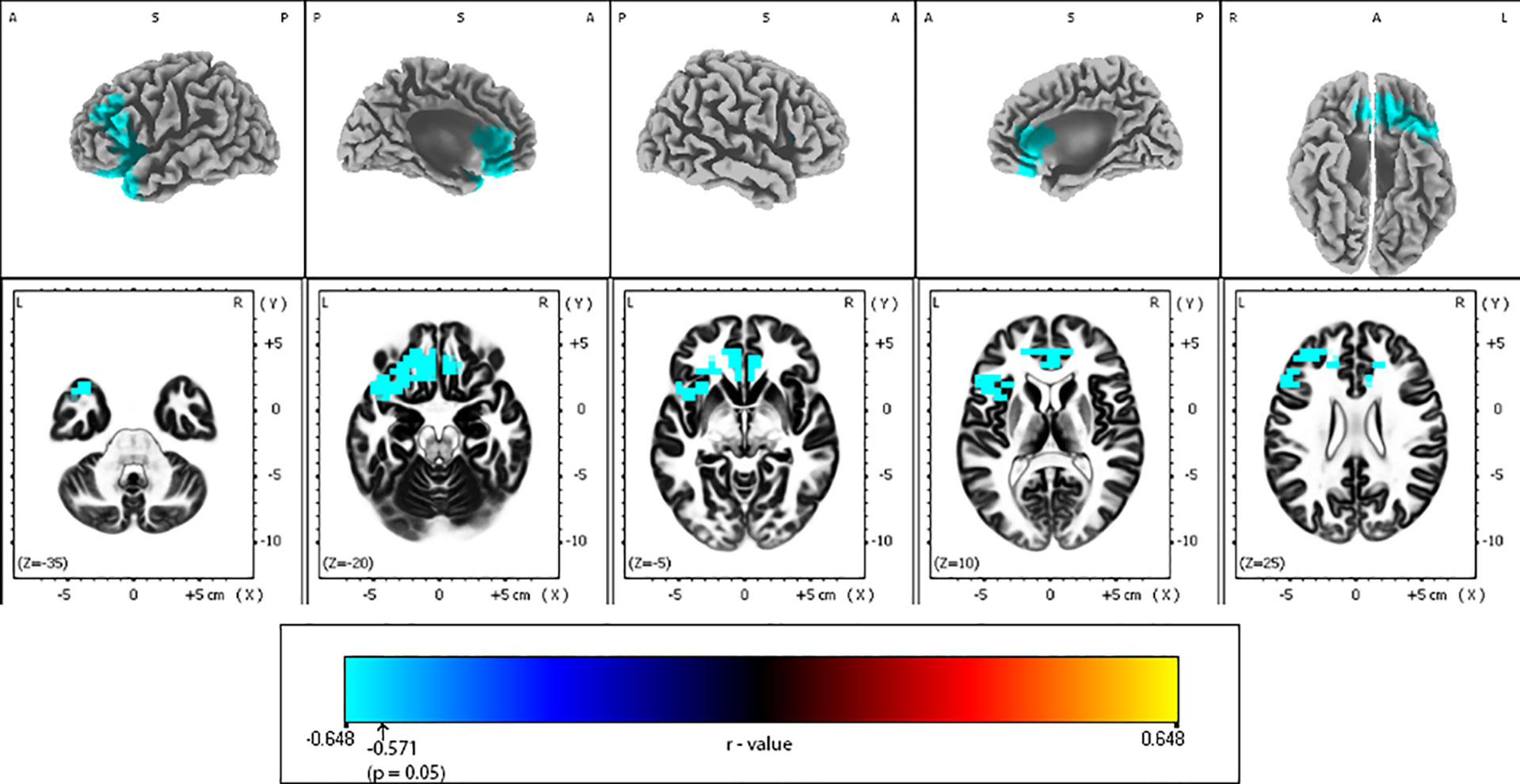
Figure 4 Correlations between Montgomery-Åsberg depression rating scale (MADRS) change and baseline alpha-2 exact low-resolution brain electromagnetic tomography (eLORETA) current density for the whole sample group (N = 25). Surface-rendered (upper row) and axial sliced (lower row) images depicting statistical nonparametric maps (SnPMs) are based on voxel-by-voxel regression analysis of MADRS score change (week 4 vs. baseline) on the baseline eLORETA current density in the alpha-2 frequency band (10.5–12 Hz). The significant negative effect (blue color; threshold: t = -0.571, p < 0.05) was observed between baseline alpha-2 eLORETA current density and the change of MADRS score may be seen in different brain regions: the less the alpha-2 current density mainly over left frontal and anterior cingulate regions, the better the treatment outcome. Structural anatomy is shown in grayscale (L, left; R, right; A, anterior; P, posterior; S, superior); x, y, z = Talairach coordinates. The threshold value (r = -0.571) for statistical significance (corresponding to p = 0.05) is reported at the color scale on the bottom side of the figure.
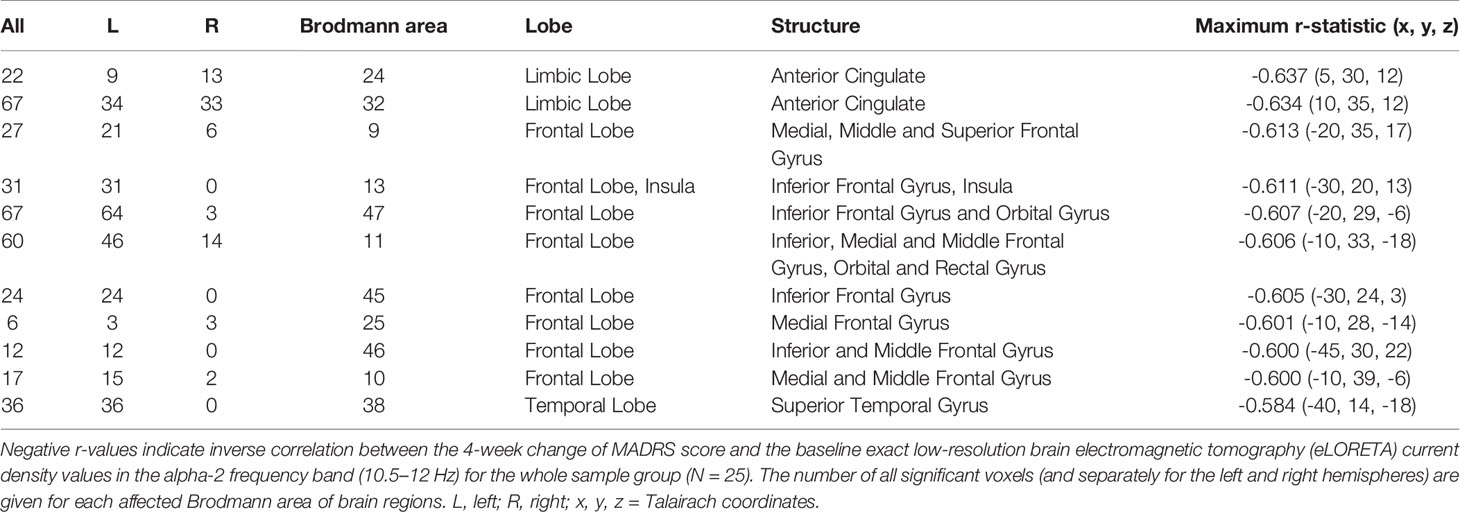
Table 4 Regional regression analysis of the Montgomery-Åsberg depression rating scale (MADRS) score change on the baseline alpha-2 eLORETA current density in depressive patients treated by repetitive transcranial magnetic stimulation (rTMS).
Discussion
Our study findings reveal that pre-treatment decrease of alpha-2 and beta-1 sources differentiate the responders and non-responders to LF rTMS treatment, when applied to patients with major depressive disorder and who have failed to respond to at least 1 adequate antidepressant treatment in their current episode. Responders to the right-side low-frequency rTMS therapy demonstrated significantly lower current source density (CSD) in the frontal gyri, anterior cingulum, and insula, i.e., regions that have previously been related with the pathophysiology of depression (73, 74). The change in depressive psychopathology correlated negatively with alpha-2 sources in the left hemisphere, with the highest negative correlation localized in the anterior cingulate. Furthermore, none of the EEG asymmetries differentiated significantly, responders from non-responders.
Studies have confirmed the association between altered activity in the aforementioned brain regions and depressive disorder (75–77). Specifically, patients compared to healthy subjects demonstrate increased CSD in several frontal regions including anterior cingulate cortex (ACC), dorsolateral prefrontal cortex (DLPFC), medial prefrontal cortex (mPFC), and the insula (78, 79). Our eLORETA analysis reported lower activity in responders’ group in alpha-2 and beta-1 bands in similar regions. The relationship between the lower beta activity in the frontal areas in responders to the antidepressant treatment as well as a negative correlation between the lower pre-treatment alpha-2 current density in the left hemisphere with psychopathology change has been observed in previous studies. For example, Knott (15) found a smaller beta activity in the frontotemporal region that was associated with greater reductions in depressive psychopathology while an opposite relationship was observed in the frontotemporal inter-hemispheric beta coherence values. Similarly, it was observed that initially, larger values of EEG beta-1and beta-2 spectral power were associated with the high manifestation of residual depressive symptoms after treatment (29). In a recent study, Hasanzadeh et al. (30) focused on finding effective EEG features for the prediction of antidepressant rTMS treatment response. Their results refer to a specific role of beta, namely, the relative power and the sum of logarithmic amplitudes of diagonal elements of bi-spectrum in beta bands that were the most discriminating features. This corroborates with the finding that rTMS can induce a significant increase in beta-band activity in the frontal areas (80). Concerning the lower left-sided alpha-2 activity, which correlates with the changes in depressive symptomatology, we find our results comparable to ones that use a source-localization technique in order to estimate neuronal correlates of approach motivation, which is altered in depression especially when it comes to reward assessment. Pizzagalli (81) found that resting alpha-2 EEG activity in the left dorsolateral PFC regions exhibit a significant inverse correlation with reward bias, suggesting that lower alpha-2 current density is associated with lower individual reward responsiveness. Another study based on a combination of EEG and fMRI found that MDD patients with more severe depression exhibited lower left DLPFC and amygdala upper alpha EEG activity, which was corrected after antidepressant intervention (28).
Therefore, responders to the rTMS therapy, demonstrate a higher potential to increase the level of absolute power in alpha-2 and beta-1 frequency bands, which may be directly associated with therapeutic physiological response. According to previous findings, the depressive disorder could be related to lower activity in the left frontal areas (82). As the increased activity in the beta frequency band is correlated positively with the regional cerebral blood flow (83, 84), one cannot rule out that a right-sided frontal stimulation might increase the deficient metabolic activity in the left frontal lobe due to a contra-lateral adaptation process. This may manifest by increasing the absolute beta power bound to the frontal area in the case of response to rTMS treatment. As observed in an earlier study, rTMS indeed can induce a significant increase in beta activity in the frontal areas (80). However, this claim is complicated by Arrubla et al. (85), who in his recent fMRI-EEG study of resting-state demonstrated that the activity of alpha-2 and beta-1 generators in posterior cingulate correlate negatively with glutamate levels, suggesting possible increased activity also in the regions highlighted in our study. The relationship between rTMS response and lower glutamate levels was recently confirmed by fMRI spectroscopy when the responders to HF-rTMS had lower baseline concentrations of DLPFC glutamate (86). Following the abovementioned studies, the lower prefrontal and cingulate beta-1 sources found in rTMS responders in our study could be interpreted in the context of higher glutamate level in these subjects (compared to non-responders) and correspond to the reduced levels of glutamate in the ACC of severely depressed patients (87). The difference observed between the responders and non-responders in the MADRS baseline may be responsible for this discrepancy. However, since we did not find any significant correlations between baseline the MADRS and baseline eLORETA values, it is possible that our findings regarding alpha-2 and beta-1 are due to the inhibitory effect of the selected treatment protocol. Some patients with severe depression thus may be more responsive to the low-frequency protocol, which may modulate GABA/glutamate imbalance in the prefrontal and temporal cortex, while others may benefit from the high-frequency protocol, which is responsible for the direct (stimulating) increase of glutamate in these areas. In order to confirm this, it will be necessary to conduct a study analyzing resting-state alpha-2 and beta-1 sources using high-resolution EEG and MR spectroscopy measurement before, during, and at the end of LF-rTMS treatment.
However, this does not explain whether the detected EEG and eLORETA profile reflects the more general index of a response outcome. Theoretically each of the source bands (alpha-2 and beta-1), in relation to certain brain areas, does not differ in their relationship to the specificity of the physiological changes that LF-rTMS can induce. This would be in line with our observations, where psychopathology change correlated with alpha-2 but not beta-1, suggesting that alpha-2 and beta-1 source generators may differ in their sensitivity to rTMS intervention. However, this was not confirmed by the above-mentioned findings where changes in both the bands occurred in different treatment interventions. Further studies with an in-depth analysis of the neuronal sources of these frequency bands in responders, non-responders, and healthy volunteers based on e.g. simultaneous EEG-fMRI assessment, and taking into account different stages of anti-depressive treatment, may provide a more comprehensive answer to this question.
Limitations
The response rate in our study is relatively low. However, Berlim´s and Cao´s meta-analyses (6, 88) showed similar response rates as observed in our study. We cannot exclude the higher rate of positive results using increased motor threshold (110%–120%) and a number of stimulations (extended course to 30 stimulation) recommended in the recent guidelines (89). There are also modified or new coil positioning techniques such as 5.5 or 6 cm anterior to the motor cortex (e.g., the centimeter rule), coil placement on F3 position according to the International 10–20 system, use of stereotactic frames, and neuroimage-guided frameless positioning technologies (90) which can increase the response rate. The results of this study thus should be interpreted with caution due to its limited sample size (only 9 subjects in the responder’s group). It should be noted, however, that we experienced difficulties during the recruitment process due to the enrolment restrictions, especially the termination of medication being administered and the approval of the rTMS course application. Future studies conducted in a larger clinical sample may further support our findings. Furthermore, we did not include a placebo arm (sham stimulation without any medication) as participation of patients with MDD without any medical treatment is ethically unacceptable and not approved by the Prague Psychiatric Center Institutional Review Board, especially for treatment of patients who failed to respond to at least one adequate antidepressant treatment in their current episode. Another limitation of the EEG part of the study is the use of a relatively small number of electrodes, however, EEG assessments in a real clinical setting are usually conducted with 19 or 21 scalp electrodes. Although the number of EEG electrodes is related to the precision of source estimation, several studies indicate that a reliable LORETA/eLORETA estimation can be achieved with just 19 channels (91–96). Nevertheless, eLORETA accuracy still depends to an extent on the EEG montage density and the relatively small number of electrodes that we were limited to suggest some caution in interpreting our findings. Higher density EEG caps (e.g., at least 64 electrodes) can provide a more accurate source localization. Finally, the neuropsychological assessment had not been conducted in the patients prior to rTMS therapy. Future research conducted in a larger sample adopting initial neuropsychological testing might clarify the association between the potential benefit of rTMS therapy and the specific neuropsychological profile of depressive patients.
Conclusion
Our study revealed that the patients with major depressive disorder, responding to the right-side LF rTMS therapy, differed significantly in alpha-2 and beta-1 current density sources, which were decreased in the frontal gyri and limbic structures (anterior cingulum and insula) when compared to the non-responders. Scalp-derived QEEG measures revealed a negative correlation between the beta absolute power at the left frontal electrode F7 and the change in depressive symptomatology. However, none of the EEG asymmetries differentiated significantly the responders from non-responders. Future studies may validate our results in larger and more diverse samples, including cognitive and genetic testing related to depressive disorder.
Data Availability Statement
The datasets generated for this study are available on request to the corresponding author.
Ethics Statement
The study involving human participants was reviewed and approved by the ethical committee of the Prague Psychiatric Centre/National Institute of Mental Health, Czech Republic. The patients/participants provided their written informed consent to participate in this study.
Author Contributions
PV analyzed EEG data, managed literature searches, discussed results, and drafted the manuscript. MBa designed the study, participated in the clinical part of the project, and revised the manuscript. TN designed the study, participated in the clinical part of the project, undertook statistical analyses, and revised the manuscript. MBr designed the EEG part of the study, coordinated project activities, analyzed data, discussed results, and drafted and revised the manuscript.
Funding
The study was supported by grants from the Czech Ministry of Health, no. AZV 15-29900A and 16-31380A; by the projects PROGRES Q35 and 260388/SVV/2019 of the Third Faculty of Medicine, Charles University and by the project ““Sustainability for the National Institute of Mental Health” (NPU4NUDZ) under the grant number LO1611 with a financial support from the Ministry of Education, Youth and Sports of the Czech Republic under the NPU I program.
Conflict of Interest
The authors declare that the research was conducted in the absence of any commercial or financial relationships that could be construed as a potential conflict of interest.
Acknowledgments
The results of this study were first presented at the 20th Biennial IPEG Meeting 2018 in Zürich, Switzerland (97) and at the 5th Maudsley Mediterranean Forum 2018 in Palermo, Italy.
References
1. Rush AJ, Trivedi MH, Wisniewski SR, Nierenberg AA, Stewart JW, Warden D, et al. Acute and longer-term outcomes in depressed outpatients requiring one or several treatment steps: a STAR*D report. Am J Psychiatry (2006) 163(11):1905–17. doi: 10.1176/ajp.2006.163.11.1905
2. Tang A, Thickbroom G, Rodger J. Repetitive transcranial magnetic stimulation of the brain: mechanisms from animal and experimental models. Neuroscientist (2017) 23(1):82–94. doi: 10.1177/1073858415618897
3. Soundara Rajan T, Ghilardi MFM, Wang HY, Mazzon E, Bramanti P, Restivo D, et al. Mechanism of action for rTMS: a working hypothesis based on animal studies. Front Physiol (2017) 8:457. doi: 10.3389/fphys.2017.00457
4. Richieri R, Boyer L, Farisse J, Colavolpe C, Mundler O, Lancon C, et al. Predictive value of brain perfusion SPECT for rTMS response in pharmacoresistant depression. Eur J Nucl Med Mol Imaging (2011) 38(9):1715–22. doi: 10.1007/s00259-011-1850-9
5. Mutz J, Vipulananthan V, Carter B, Hurlemann R, Fu CHY, Young AH. Comparative efficacy and acceptability of non-surgical brain stimulation for the acute treatment of major depressive episodes in adults: systematic review and network meta-analysis. BMJ (2019) 364:l1079. doi: 10.1136/bmj.l1079
6. Berlim MT, Van den Eynde F, Jeff Daskalakis Z. Clinically meaningful efficacy and acceptability of low-frequency repetitive transcranial magnetic stimulation (rTMS) for treating primary major depression: a meta-analysis of randomized, double-blind and sham-controlled trials. Neuropsychopharmacology (2013) 38(4):543–51. doi: 10.1038/npp.2012.237
7. Fu CH, Williams SC, Cleare AJ, Brammer MJ, Walsh ND, Kim J, et al. Attenuation of the neural response to sad faces in major depression by antidepressant treatment: a prospective, event-related functional magnetic resonance imaging study. Arch Gen Psychiatry (2004) 61(9):877–89. doi: 10.1001/archpsyc.61.9.877
8. Kennedy SH, Evans KR, Kruger S, Mayberg HS, Meyer JH, McCann S, et al. Changes in regional brain glucose metabolism measured with positron emission tomography after paroxetine treatment of major depression. Am J Psychiatry (2001) 158(6):899–905. doi: 10.1176/appi.ajp.158.6.899
9. Shajahan PM, Glabus MF, Steele JD, Doris AB, Anderson K, Jenkins JA, et al. Left dorso-lateral repetitive transcranial magnetic stimulation affects cortical excitability and functional connectivity, but does not impair cognition in major depression. Prog Neuropsychopharmacol Biol Psychiatry (2002) 26(5):945–54. doi: 10.1016/s0278-5846(02)00210-5
10. Dillon DG, Pizzagalli DA. Evidence of successful modulation of brain activation and subjective experience during reappraisal of negative emotion in unmedicated depression. Psychiatry Res (2013) 212(2):99–107. doi: 10.1016/j.pscychresns.2013.01.001
11. Goldapple K, Segal Z, Garson C, Lau M, Bieling P, Kennedy S, et al. Modulation of cortical-limbic pathways in major depression: treatment-specific effects of cognitive behavior therapy. Arch Gen Psychiatry (2004) 61(1):34–41. doi: 10.1001/archpsyc.61.1.34
12. Chen MH, Lin WC, Tu PC, Li CT, Bai YM, Tsai SJ, et al. Antidepressant and antisuicidal effects of ketamine on the functional connectivity of prefrontal cortex-related circuits in treatment-resistant depression: a double-blind, placebo-controlled, randomized, longitudinal resting fMRI study. J Affect Disord (2019) 259:15–20. doi: 10.1016/j.jad.2019.08.022
13. Hunter AM, Cook IA, Leuchter AF. The promise of the quantitative electroencephalogram as a predictor of antidepressant treatment outcomes in major depressive disorder. Psychiatr Clin North Am (2007) 30(1):105–24. doi: 10.1016/j.psc.2006.12.002
14. Beuzon G, Timour Q, Saoud M. Predictors of response to repetitive transcranial magnetic stimulation (rTMS) in the treatment of major depressive disorder. Encephale (2017) 43(1):3–9. doi: 10.1016/j.encep.2016.11.002
15. Knott V, Mahoney C, Kennedy S, Evans K. Pre-treatment EEG and it's relationship to depression severity and paroxetine treatment outcome. Pharmacopsychiatry (2000) 33(6):201–5. doi: 10.1055/s-2000-8356
16. Schiller MJ. Quantitative electroencephalography in guiding treatment of major depression. Front Psychiatry (2018) 9:779. doi: 10.3389/fpsyt.2018.00779
17. Newson JJ, Thiagarajan TC. EEG frequency bands in psychiatric disorders: a review of resting state studies. Front Hum Neurosci (2018) 12:521. doi: 10.3389/fnhum.2018.00521
18. Fingelkurts AA, Fingelkurts AA. Altered structure of dynamic electroencephalogram oscillatory pattern in major depression. Biol Psychiatry (2015) 77(12):1050–60. doi: 10.1016/j.biopsych.2014.12.011
19. Baskaran A, Milev R, McIntyre RS. The neurobiology of the EEG biomarker as a predictor of treatment response in depression. Neuropharmacology (2012) 63(4):507–13. doi: 10.1016/j.neuropharm.2012.04.021
20. Palmiero M, Piccardi L. Frontal EEG asymmetry of mood: a mini-review. Front Behav Neurosci (2017) 11:224. doi: 10.3389/fnbeh.2017.00224
21. Alamian G, Hincapie AS, Combrisson E, Thiery T, Martel V, Althukov D, et al. Alterations of intrinsic brain connectivity patterns in depression and bipolar disorders: a critical assessment of magnetoencephalography-based evidence. Front Psychiatry (2017) 8:41. doi: 10.3389/fpsyt.2017.00041
22. Leuchter AF, Cook IA, Uijtdehaage SH, Dunkin J, Lufkin RB, Anderson-Hanley C, et al. Brain structure and function and the outcomes of treatment for depression. J Clin Psychiatry (1997) 58 Suppl 16:22–31.
23. Baskaran A, Farzan F, Milev R, Brenner CA, Alturi S, Pat McAndrews M, et al. The comparative effectiveness of electroencephalographic indices in predicting response to escitalopram therapy in depression: a pilot study. J Affect Disord (2018) 227:542–9. doi: 10.1016/j.jad.2017.10.028
24. Arns M, Bruder G, Hegerl U, Spooner C, Palmer DM, Etkin A, et al. EEG alpha asymmetry as a gender-specific predictor of outcome to acute treatment with different antidepressant medications in the randomized iSPOT-D study. Clin Neurophysiol (2016) 127(1):509–19. doi: 10.1016/j.clinph.2015.05.032
25. van der Vinne N, Vollebregt MA, van Putten M, Arns M. Stability of frontal alpha asymmetry in depressed patients during antidepressant treatment. NeuroImage Clin (2019) 24:102056. doi: 10.1016/j.nicl.2019.102056
26. Smith EE, Tenke CE, Deldin PJ, Trivedi MH, Weissman MM, Auerbach RP, et al. Frontal theta and posterior alpha in resting EEG: a critical examination of convergent and discriminant validity. Psychophysiology (2019), 57(2):e13483. doi: 10.1111/psyp.13483
27. Tenke CE, Kayser J, Manna CG, Fekri S, Kroppmann CJ, Schaller JD, et al. Current source density measures of electroencephalographic alpha predict antidepressant treatment response. Biol Psychiatry (2011) 70(4):388–94. doi: 10.1016/j.biopsych.2011.02.016
28. Zotev V, Yuan H, Misaki M, Phillips R, Young KD, Feldner MT, et al. Correlation between amygdala BOLD activity and frontal EEG asymmetry during real-time fMRI neurofeedback training in patients with depression. NeuroImage Clin (2016) 11:224–38. doi: 10.1016/j.nicl.2016.02.003
29. Iznak A, Tiganov A, Iznak E, Sorokin S. EEG correlates and possible predictors of the efficacy of the treatment of endogenous depression. Hum Physiol (2013) 39(4):378–85. doi: 10.1134/S0362119713040063
30. Hasanzadeh F, Mohebbi M, Rostami R. Prediction of rTMS treatment response in major depressive disorder using machine learning techniques and nonlinear features of EEG signal. J Affect Disord (2019) 256:132–42. doi: 10.1016/j.jad.2019.05.070
31. Arns M, Drinkenburg WH, Fitzgerald PB, Kenemans JL. Neurophysiological predictors of non-response to rTMS in depression. Brain Stimul (2012) 5(4):569–76. doi: 10.1016/j.brs.2011.12.003
32. Narushima K, McCormick LM, Yamada T, Thatcher RW, Robinson RG. Subgenual cingulate theta activity predicts treatment response of repetitive transcranial magnetic stimulation in participants with vascular depression. J Neuropsychiatry Clin Neurosci (2010) 22(1):75–84. doi: 10.1176/appi.neuropsych.22.1.75
33. Bailey NW, Hoy KE, Rogasch NC, Thomson RH, McQueen S, Elliot D, et al. Responders to rTMS for depression show increased fronto-midline theta and theta connectivity compared to non-responders. Brain Stimul (2018) 11(1):190–203. doi: 10.1016/j.brs.2017.10.015
34. Price GW, Lee JW, Garvey C, Gibson N. Appraisal of sessional EEG features as a correlate of clinical changes in an rTMS treatment of depression. Clin EEG Neurosci (2008) 39(3):131–8. doi: 10.1177/155005940803900307
35. Hunter AM, Nghiem TX, Cook IA, Krantz DE, Minzenberg MJ, Leuchter AF. Change in quantitative EEG theta cordance as a potential predictor of repetitive transcranial magnetic stimulation clinical outcome in major depressive disorder. Clin EEG Neurosci (2017), 49(5):306–15. doi: 10.1177/1550059417746212
36. Ozekes S, Erguzel T, Sayar GH, Tarhan N. Analysis of brain functional changes in high-frequency repetitive transcranial magnetic stimulation in treatment-resistant depression. Clin EEG Neurosci (2014) 45(4):257–61. doi: 10.1177/1550059413515656
37. Arns M, Cerquera A, Gutierrez RM, Hasselman F, Freund JA. Non-linear EEG analyses predict non-response to rTMS treatment in major depressive disorder. Clin Neurophysiol (2014) 125(7):1392–9. doi: 10.1016/j.clinph.2013.11.022
38. Zuchowicz U, Wozniak-Kwasniewska A, Szekely D, Olejarczyk E, David O. EEG phase synchronization in persons with depression subjected to transcranial magnetic stimulation. Front Neurosci (2018) 12:1037. doi: 10.3389/fnins.2018.01037
39. Valiulis V, Gerulskis G, Dapsys K, Vistartaite G, Siurkute A, Maciulis V. Electrophysiological differences between high and low frequency rTMS protocols in depression treatment. Acta Neurobiol Exp (Wars) (2012) 72(3):283–95.
40. Bares M, Brunovsky M, Novak T, Kopecek M, Stopkova P, Sos P, et al. QEEG Theta cordance in the prediction of treatment outcome to prefrontal repetitive transcranial magnetic stimulation or venlafaxine ER in patients with major depressive disorder. Clin EEG Neurosci (2015) 46(2):73–80. doi: 10.1177/1550059413520442
41. Bares M, Kopecek M, Novak T, Stopkova P, Sos P, Kozeny J, et al. Low frequency (1-Hz), right prefrontal repetitive transcranial magnetic stimulation (rTMS) compared with venlafaxine ER in the treatment of resistant depression: a double-blind, single-centre, randomized study. J Affect Disord (2009) 118(1-3):94–100. doi: 10.1016/j.jad.2009.01.032
42. American Psychiatric Association. Diagnostic criteria from DSM-IV-TR. Washington, D.C: American Psychiatric Association (2000). p. 370.
43. Sheehan DV, Lecrubier Y, Sheehan KH, Amorim P, Janavs J, Weiller E, et al. The Mini-International Neuropsychiatric Interview (M.I.N.I.): the development and validation of a structured diagnostic psychiatric interview for DSM-IV and ICD-10. J Clin Psychiatry (1998) 59 Suppl 20:22–33;quiz 4-57.
44. Thase ME. Psychotherapy of refractory depressions. Depress Anxiety (1997) 5(4):190–201. doi: 10.1002/(SICI)1520-6394(1997)5:4<190::AID-DA5>3.0.CO;2-H
45. Montgomery SA, Asberg M. New depression scale designed to be sensitive to change. Br J Psychiatry (1979) 134:382–9. doi: 10.1192/bjp.134.4.382
46. Guy W. Clinical global impressions-ECDEU Asessment manual psychopharmacology (DHEW Publ no ADM 76–338). In: Public Health Service, Alcohol, Drug Abuse, and Mental Health Administration, NIMH. Revised. Rockville MD: US Department of Health, Education, and Welfare (1976).
47. Sackeim HA. The definition and meaning of treatment-resistant depression. J Clin Psychiatry (2001) 62 Suppl 16:10–7.
48. Thatcher RW, Biver CJ, North DM. Quantitative EEG and the Frye and Daubert standards of admissibility. Clin Electroencephalogr (2003) 34(2):39–53. doi: 10.1177/155005940303400203
49. Nuwer MR, Lehmann D, da Silva FL, Matsuoka S, Sutherling W, Vibert JF. IFCN guidelines for topographic and frequency analysis of EEGs and EPs. The international federation of clinical neurophysiology. Electroencephalogr Clin Neurophysiol Suppl (1999) 52:15–20.
50. Bendat JS, Piersol AG. Engineering applications of correlation and spectral analysis. New York Wiley-Interscience (1980) 315 p.
51. Pascual-Marqui RD, Esslen M, Kochi K, Lehmann D. Functional imaging with low-resolution brain electromagnetic tomography (LORETA): a review. Methods Find Exp Clin Pharmacol (2002) 24 Suppl C:91–5.
52. Pascual-Marqui RD. Standardized low-resolution brain electromagnetic tomography (sLORETA): technical details. Methods Find Exp Clin Pharmacol (2002) 24 Suppl D:5–12.
53. Talairach J, Tournoux P. Co-planar stereotaxic atlas of the human brain: 3-dimensional proportional system: an approach to cerebral imaging. Stuttgart; New York: Georg Thieme (1988). 122 p.
54. Pascual-Marqui RD, Lehmann D, Koukkou M, Kochi K, Anderer P, Saletu B, et al. Assessing interactions in the brain with exact low-resolution electromagnetic tomography. Philos Trans A Math Phys Eng Sci (1952) (2011), 3768–84. doi: 10.1098/rsta.2011.0081
55. Thatcher RW, North DM, Biver CJ. Diffusion spectral imaging modules correlate with EEG LORETA neuroimaging modules. Hum Brain Mapp (2012) 33(5):1062–75. doi: 10.1002/hbm.21271
56. Mulert C, Jager L, Schmitt R, Bussfeld P, Pogarell O, Moller HJ, et al. Integration of fMRI and simultaneous EEG: towards a comprehensive understanding of localization and time-course of brain activity in target detection. NeuroImage (2004) 22(1):83–94. doi: 10.1016/j.neuroimage.2003.10.051
57. Vitacco D, Brandeis D, Pascual-Marqui R, Martin E. Correspondence of event-related potential tomography and functional magnetic resonance imaging during language processing. Hum Brain Mapp (2002) 17(1):4–12. doi: 10.1002/hbm.10038
58. Olbrich S, Mulert C, Karch S, Trenner M, Leicht G, Pogarell O, et al. EEG-vigilance and BOLD effect during simultaneous EEG/fMRI measurement. NeuroImage (2009) 45(2):319–32. doi: 10.1016/j.neuroimage.2008.11.014
59. Whittingstall K, Bartels A, Singh V, Kwon S, Logothetis NK. Integration of EEG source imaging and fMRI during continuous viewing of natural movies. Magn Reson Imaging (2010) 28(8):1135–42. doi: 10.1016/j.mri.2010.03.042
60. Worrell GA, Lagerlund TD, Sharbrough FW, Brinkmann BH, Busacker NE, Cicora KM, et al. Localization of the epileptic focus by low-resolution electromagnetic tomography in patients with a lesion demonstrated by MRI. Brain Topogr (2000) 12(4):273–82. doi: 10.1023/a:1023407521772
61. Dierks T, Jelic V, Pascual-Marqui RD, Wahlund L, Julin P, Linden DE, et al. Spatial pattern of cerebral glucose metabolism (PET) correlates with localization of intracerebral EEG-generators in Alzheimer's disease. Clin Neurophysiol (2000) 111(10):1817–24. doi: 10.1016/S1388-2457(00)00427-2
62. Pizzagalli DA, Oakes TR, Fox AS, Chung MK, Larson CL, Abercrombie HC, et al. Functional but not structural subgenual prefrontal cortex abnormalities in melancholia. Mol Psychiatry (2004) 9(4):325, 93–405. doi: 10.1038/sj.mp.4001501
63. Zumsteg D, Wennberg RA, Treyer V, Buck A, Wieser HG. H2(15)O or 13NH3 PET and electromagnetic tomography (LORETA) during partial status epilepticus. Neurology (2005) 65(10):1657–60. doi: 10.1212/01.wnl.0000184516.32369.1a
64. Horacek J, Brunovsky M, Novak T, Skrdlantova L, Klirova M, Bubenikova-Valesova V, et al. Effect of low-frequency rTMS on electromagnetic tomography (LORETA) and regional brain metabolism (PET) in schizophrenia patients with auditory hallucinations. Neuropsychobiology (2007) 55(3-4):132–42. doi: 10.1159/000106055
65. Anderer P, Saletu B, Pascual-Marqui RD. Effect of the 5-HT(1A) partial agonist buspirone on regional brain electrical activity in man: a functional neuroimaging study using low-resolution electromagnetic tomography (LORETA). Psychiatry Res (2000) 100(2):81–96. doi: 10.1016/S0925-4927(00)00066-4
66. Zumsteg D, Lozano AM, Wieser HG, Wennberg RA. Cortical activation with deep brain stimulation of the anterior thalamus for epilepsy. Clin Neurophysiol (2006) 117(1):192–207. doi: 10.1016/j.clinph.2005.09.015
67. Zumsteg D, Lozano AM, Wennberg RA. Depth electrode recorded cerebral responses with deep brain stimulation of the anterior thalamus for epilepsy. Clin Neurophysiol (2006) 117(7):1602–9. doi: 10.1016/j.clinph.2006.04.008
68. Zumsteg D, Friedman A, Wieser HG, Wennberg RA. Propagation of interictal discharges in temporal lobe epilepsy: correlation of spatiotemporal mapping with intracranial foramen ovale electrode recordings. Clin Neurophysiol (2006) 117(12):2615–26. doi: 10.1016/j.clinph.2006.07.319
69. Volpe U, Mucci A, Bucci P, Merlotti E, Galderisi S, Maj M. The cortical generators of P3a and P3b: a LORETA study. Brain Res Bull (2007) 73(4-6):220–30. doi: 10.1016/j.brainresbull.2007.03.003
70. Jobert M, Wilson FJ, Ruigt GS, Brunovsky M, Prichep LS, Drinkenburg WH, et al. Guidelines for the recording and evaluation of pharmaco-EEG data in man: the International Pharmaco-EEG Society (IPEG). Neuropsychobiology (2012) 66(4):201–20. doi: 10.1159/000343478
71. Genovese CR, Lazar NA, Nichols T. Thresholding of statistical maps in functional neuroimaging using the false discovery rate. NeuroImage (2002) 15(4):870–8. doi: 10.1006/nimg.2001.1037
72. Nichols TE, Holmes AP. Nonparametric permutation tests for functional neuroimaging: a primer with examples. Hum Brain Mapp (2002) 15(1):1–25. doi: 10.1002/hbm.1058
73. Alexander L, Clarke HF, Roberts AC. A focus on the functions of area 25. Brain Sci (2019) 9(6):129. doi: 10.3390/brainsci9060129
74. Taib S, Arbus C, Sauvaget A, Sporer M, Schmitt L, Yrondi A. How does repetitive transcranial magnetic stimulation influence the brain in depressive disorders?: a review of neuroimaging magnetic resonance imaging studies. J ECT (2018) 34(2):79–86. doi: 10.1097/YCT.0000000000000477
75. Wang L, Hermens DF, Hickie IB, Lagopoulos J. A systematic review of resting-state functional-MRI studies in major depression. J Affect Disord (2012) 142(1-3):6–12. doi: 10.1016/j.jad.2012.04.013
76. Auerbach RP, Stewart JG, Stanton CH, Mueller EM, Pizzagalli DA. Emotion-processing biases and resting eeg activity in depressed adolescents. Depress Anxiety (2015) 32(9):693–701. doi: 10.1002/da.22381
77. Rogers MA, Kasai K, Koji M, Fukuda R, Iwanami A, Nakagome K, et al. Executive and prefrontal dysfunction in unipolar depression: a review of neuropsychological and imaging evidence. Neurosci Res (2004) 50(1):1–11. doi: 10.1016/j.neures.2004.05.003
78. Saletu B, Anderer P, Saletu-Zyhlarz GM. EEG topography and tomography (LORETA) in diagnosis and pharmacotherapy of depression. Clin EEG Neurosci (2010) 41(4):203–10. doi: 10.1177/155005941004100407
79. Ricardo-Garcell J, Gonzalez-Olvera JJ, Miranda E, Harmony T, Reyes E, Almeida L, et al. EEG sources in a group of patients with major depressive disorders. Int J Psychophysiol (2009) 71(1):70–4. doi: 10.1016/j.ijpsycho.2008.07.021
80. Canali P, Sarasso S, Rosanova M, Casarotto S, Sferrazza-Papa G, Gosseries O, et al. Shared reduction of oscillatory natural frequencies in bipolar disorder, major depressive disorder and schizophrenia. J Affect Disord (2015) 184:111–5. doi: 10.1016/j.jad.2015.05.043
81. Pizzagalli DA, Sherwood RJ, Henriques JB, Davidson RJ. Frontal brain asymmetry and reward responsiveness: a source-localization study. Psychol Sci (2005) 16(10):805–13. doi: 10.1111/j.1467-9280.2005.01618.x
82. Bruder GE, Stewart JW, McGrath PJ. Right brain, left brain in depressive disorders: clinical and theoretical implications of behavioral, electrophysiological and neuroimaging findings. Neurosci Biobehav Rev (2017) 78:178–91. doi: 10.1016/j.neubiorev.2017.04.021
83. Leuchter AF, Uijtdehaage SHJ, Cook IA, O'Hara R, Mandelkern M. Relationship between brain electrical activity and cortical perfusion in normal subjects. Psychiatry Res Neuroimaging (1999) 90(2):125–40. doi: 10.1016/S0925-4927(99)00006-2
84. Nakamura S, Sadato N, Oohashi T, Nishina E, Fuwamoto Y, Yonekura Y. Analysis of music-brain interaction with simultaneous measurement of regional cerebral blood flow and electroencephalogram beta rhythm in human subjects. Neurosci Lett (1999) 275(3):222–6. doi: 10.1016/S0304-3940(99)00766-1
85. Arrubla J, Farrher E, Strippelmann J, Tse DHY, Grinberg F, Shah NJ, et al. Microstructural and functional correlates of glutamate concentration in the posterior cingulate cortex. J Neurosci Res (2017) 95(9):1796–808. doi: 10.1002/jnr.24010
86. Luborzewski A, Schubert F, Seifert F, Danker-Hopfe H, Brakemeier EL, Schlattmann P, et al. Metabolic alterations in the dorsolateral prefrontal cortex after treatment with high-frequency repetitive transcranial magnetic stimulation in patients with unipolar major depression. J Psychiatr Res (2007) 41(7):606–15. doi: 10.1016/j.jpsychires.2006.02.003
87. Auer DP, Putz B, Kraft E, Lipinski B, Schill J, Holsboer F. Reduced glutamate in the anterior cingulate cortex in depression: an in vivo proton magnetic resonance spectroscopy study. Biol Psychiatry (2000) 47(4):305–13. doi: 10.1016/s0006-3223(99)00159-6
88. Cao X, Deng C, Su X, Guo Y. Response and remission rates following high-frequency vs. low-frequency repetitive transcranial magnetic stimulation (rTMS) over right DLPFC for treating Major Depressive Disorder (MDD): A meta-analysis of randomized, double-blind trials. Front Psychiatry (2018) 9:413. doi: 10.3389/fpsyt.2018.00413
89. Milev RV, Giacobbe P, Kennedy SH, Blumberger DM, Daskalakis ZJ, Downar J, et al. Canadian Network for Mood and Anxiety Treatments (CANMAT) 2016 clinical guidelines for the management of adults with major depressive disorder: section 4. neurostimulation treatments. Can J Psychiatry (2016) 61(9):561–75. doi: 10.1177/0706743716660033
90. McClintock SM, Reti IM, Carpenter LL, McDonald WM, Dubin M, Taylor SF, et al. Consensus Recommendations for the Clinical Application of Repetitive Transcranial Magnetic Stimulation (rTMS) in the treatment of depression. J Clin Psychiatry (2018) 79(1). doi: 10.4088/JCP.16cs10905
91. Thatcher RW, North DM, Biver CJ. LORETA EEG phase reset of the default mode network. Front Hum Neurosci (2014) 8:529. doi: 10.3389/fnhum.2014.00529
92. Ghaderi AH, Andevari MN, Sowman PF. Evidence for a resting state network abnormality in adults who stutter. Front Integr Neurosci (2018) 12:16. doi: 10.3389/fnint.2018.00016
93. Aoki Y, Ishii R, Pascual-Marqui RD, Canuet L, Ikeda S, Hata M, et al. Detection of EEG-resting state independent networks by eLORETA-ICA method. Front Hum Neurosci (2015) 9:31. doi: 10.3389/fnhum.2015.00031
94. Clemens B, Puskas S, Spisak T, Lajtos I, Opposits G, Besenyei M, et al. Increased resting-state EEG functional connectivity in benign childhood epilepsy with centro-temporal spikes. Seizure (2016) 35:50–5. doi: 10.1016/j.seizure.2016.01.001
95. Hata M, Kazui H, Tanaka T, Ishii R, Canuet L, Pascual-Marqui RD, et al. Functional connectivity assessed by resting state EEG correlates with cognitive decline of Alzheimer's disease - An eLORETA study. Clin Neurophysiol (2016) 127(2):1269–78. doi: 10.1016/j.clinph.2015.10.030
96. Mumtaz W, Xia L, Ali SSA, Yasin MAM, Hussain M, Malik AS. Electroencephalogram (EEG)-based computer-aided technique to diagnose major depressive disorder (MDD). Biomed Signal Process Control (2017) 31:108–15. doi: 10.1016/j.bspc.2016.07.006
Keywords: major depressive disorder, repetitive transcranial magnetic stimulation, quantitative electroencephalography, LORETA, EEG asymmetry
Citation: Vlcek P, Bares M, Novak T and Brunovsky M (2020) Baseline Difference in Quantitative Electroencephalography Variables Between Responders and Non-Responders to Low-Frequency Repetitive Transcranial Magnetic Stimulation in Depression. Front. Psychiatry 11:83. doi: 10.3389/fpsyt.2020.00083
Received: 30 August 2019; Accepted: 03 February 2020;
Published: 27 February 2020.
Edited by:
Paul Croarkin, Mayo Clinic, United StatesReviewed by:
Yoshihiro Noda, Keio University, JapanPrabhjot Dhami, Centre for Addiction and Mental Health, Canada
Fidel Vila-Rodriguez, University of British Columbia, Canada
Natalia Jaworska, University of Ottawa, Canada
Copyright © 2020 Vlcek, Bares, Novak and Brunovsky. This is an open-access article distributed under the terms of the Creative Commons Attribution License (CC BY). The use, distribution or reproduction in other forums is permitted, provided the original author(s) and the copyright owner(s) are credited and that the original publication in this journal is cited, in accordance with accepted academic practice. No use, distribution or reproduction is permitted which does not comply with these terms.
*Correspondence: Martin Brunovsky, bWFydGluLmJydW5vdnNreUBudWR6LmN6