- Queensland University of Technology, Brisbane, QLD, Australia
Conversational interaction is a complex, interactive, dynamic and multimodal activity, allowing us to transmit and receive information, to form and maintain social ties, and also perform many other cultural and ritualistic functions. Methodologies for studying conversation, and human discourse in general, vary across many levels of abstraction, with everything from the qualitative manual coding methods of Conversation Analysis, through to the quantitative processing and analysis of vocal qualities based on frequency spectra. In this review we focus on how the paired techniques of recurrence plotting and recurrence quantification analysis (RQA) have been utilized in the study of human discourse. We focus on recurrence analyses that have examined communication behaviors and activities in various institutional and social settings. Our review focusses along several key dimensions: discourse type, the role of time in the encoding of input data, and the challenges that multi-modality place on recurrence-based discourse analysis methods. We argue that recurrence analysis holds much promise for the ongoing study of discourse, and that its full potential has yet to be realized within this domain of application.
Introduction
Conversational interaction is an incredible human joint accomplishment, requiring us to make use of not only our own semantic resources, but also the resources of our conversational partners. Conversation is dynamic and multimodal, allowing us to transmit and receive information, to form and maintain social ties, and also perform many other cultural and ritualistic functions.
Due to its inherent complexity, most studies of conversation tend to locate at a particular level of abstraction (e.g., phonetics, phonology, morphology, syntax, semantics, and pragmatics). Methodologies for studying conversation, and language in general, vary across these levels of abstraction, with everything from the qualitative manual coding methods of Conversation Analysis [1], through to the quantitative processing and analysis of vocal qualities based on frequency spectra. Each perspective offers different insights, but each also brings specific assumptions about the forms and functions of discourse.
The focus of this position piece is on how the paired techniques of recurrence plotting and recurrence quantification analysis (RQA) [2–5] have been utilized in the study of human discourse, framed from a Communication Studies perspective. Some of the most prominent, and indeed promising, applications of recurrence methods to language data have been in the arenas of psycholinguistics, human coordination and joint attention domains [6]. Rather than rehashing Fusaroli et al.'s [6] comprehensive review, we focus on more general studies of communication behaviors and activities and respond to some of the provocations and recommendations made therein. Our positions focus along several key dimensions: discourse type, the role of time in the encoding of input data, and the challenges that multi-modality place on recurrence-based discourse analysis methods.
Recurrence Plotting and Recurrence Quantification Analysis
The recurrence plotting technique was initially invented as a technique to display and identify patterns from time series data, specifically data from high-dimensional dynamical systems [5]. The recurrence plot is a 2D plot where the horizontal and vertical axes represent time series data, and individual elements of the plot indicate times where the phase space trajectory of the system visits the same region of phase space, expressed as:
Where is a phase space trajectory, i is shown on a horizontal axis, and j is shown on a vertical axis.
Given n points in a time series, there are n2 − n recurrence values, or n(n − 1)/2 recurrence values if only one half of the diagonal is used (this is the case with conceptual recurrence plots). Recurrence plots tend to be rendered as dot-plots, where recurrences are expressed in a Boolean fashion, often using a threshold to determine if two points in the underlying time series are sufficiently close to indicate a match, however some applications use gray or color shading to indicate the strength of recurrence.
While visual inspection of recurrence plots is useful for revealing the structure and dynamics of dynamical systems, Recurrence Quantification Analysis (RQA) extends this technique by specifying a set of metrics designed to capture specific features of recurrence plots [3, 7]. In the 25 years following the original work of Eckmann et al. [5], recurrence analysis has been applied across diverse areas including financial analysis, neural recordings, engineering, earth science, and chemistry [2].
Recurrence Plots Applied to Language
One of the first applications of recurrence plotting to language was the work of Orsucci et al. [8], analyzing poems from a variety of languages by plotting the recurrence of three letter word stems, and also applying RQA metrics to determine the underlying structures and rhythms of poetry. Their analysis, like other recurrence-based analyses of written texts that have focussed specifically on the written form rely on the presence (and absence) of lexical coherences, and grammatical patterns that are present in structured texts such as poetry or other ordered prose. In their work they showed how analysis of recurrence could reveal a prosaic signature of specific authors. This notion of textual structure is a theme that we will return to later as it is particularly important when distinguishing natural (spoken) language from written language forms.
Webber and Zbilut [9] were amongst the first to use recurrence to analyse transcribed speech, examining monologs by people with schizophrenia against control cases to locate differences in RQA measures between these groups. The results compared transcripts from a control group (scientists) who they postulated would be speaking with intent and purpose, vs. transcripts from the schizophrenia case that they postulated would be more abstract and less coherent. The resulting plots and RQA measures did reveal differences between these groups that aligned with these pre-held hypotheses.
Dale and Spivey [10] were also early pioneers of RQA for conversation, analyzing child and care provider conversations at the lexical level to reveal structural features that are indicative of language learning by children. Dale and Spivey [11] later extended their initial 2005 study by codifying the child and care provider data according to the grammatical class of each word. They used the RQA measure, Recurrence Rate, to track grammatical coordination between speakers postulating that such coordination may be further evidence of language learning. These studies were particularly important given that they included spontaneous conversations, revealing how RQA could be used to analyse conversation in its natural context.
Over the past decade, recurrence plotting and RQA for analysis of discourse have been further adapted through the Conceptual Recurrence Plotting approach [12, 13]. This recurrence method creates a conceptual coding of each turn in a conversation using a topic model or other appropriate vector representation of language, enabling comparisons and the construction of a recurrence plot on the conceptual similarity between all turn-pairs [14].
The first Conceptual Recurrence Plots [12, 13] used a Bayes-inspired natural language processing engine. Prior to the construction of the conceptual recurrence plot an input document (text transcript) was used to construct a Bayes statistical model using word frequency and co-occurrence data. This language model is then used to conceptually tag each turn or paragraph break in the input text (usually along ~100 unique conceptual dimensions). A cosine similarity is then applied between the concept vectors of all text segment pairs to construct the recurrence plot. Unlike a traditional recurrence plot construction process, no threshold is applied to these similarities; instead recurrences are retained as floating point numbers between 0.0 and 1.0 and displayed using varying visual intensity to represent the strength of recurrence between each pair of text segments.
The conceptual recurrence plot was initially designed for conversation transcripts and many of its visual affordances are designed to support such inputs. In the basic visual output conversational turns sequentially according to their order in the input file. Each turn is displayed as colored squares along a diagonal line. The next deviation from traditional recurrence plotting is that the length of each turn (word count, or real timing data if known) is reflected through the size of the square, and the color of each diagonal square is determined by the channel, usually attributed as individuals, or groups of conversational participants. Recurrences appearing below the diagonal are colored according to whether they correspond to a recurrence by the same channel or between different channels, using the same color for a single channel, and splitting the recurrence element rectangle into two triangles for recurrences between different channels, using each of these different channels' colors (For an example see Figure 1).
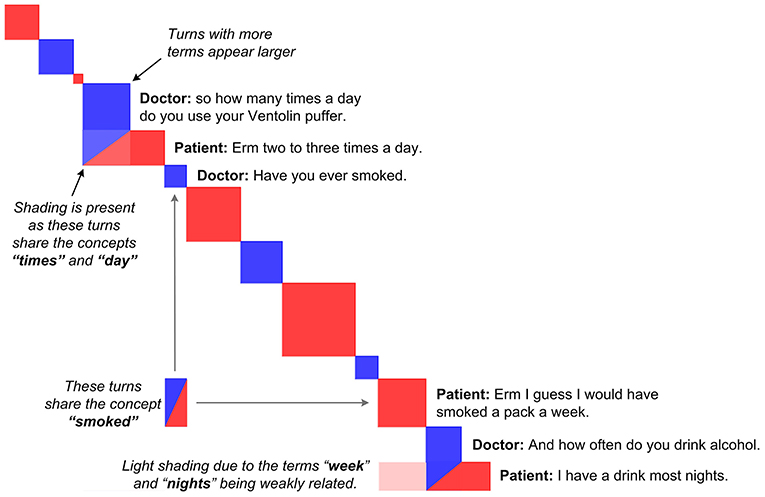
Figure 1. An example conceptual recurrence plot of an interaction between a doctor and patient. Reproduced from Angus et al. [15] with permission.
The use of color and non-binary recurrence values is informed from the intended use as an analytical support tool for conversation and discourse analysists. Color allows analysts to quickly obtain useful information such as turn ordering and dominant recurrence patterns indicating speakers who are repeating themselves perhaps more than other speakers. The use of varied recurrence block sizing helps to reveal periods where participants are engaging in longer turn structures, a point we will return to in discussing time and encoding.
Conceptual Recurrence Plots were later extended by Angus et al. [13] to generate a range of RQA-inspired metrics for the quantification of communicative interaction within a given dataset. They designed 12 primitives to measure patterns of conceptual recurrence throughout a conversational dataset, derived from all possible combinations of the following three principal dimensions: time scale (short, medium, or long range), direction (forward or backward) and type (self or other). As one example, the primitive “Long Backward Self” provides a measure of the degree to which turns of a given speaker include concepts that the same speaker (type: self) has mentioned previously (direction: backward) when examined across the entire conversation (time scale: long). Another example is “Short Forward Other”, which captures the degree to which concepts mentioned by a given speaker have been shared by the conversation partner (type: other) in the turn immediately (time scale: short) following (direction: forward).
Selections of these 12 conceptual recurrence primitives were also combined to generate eight metrics that provide specific measures for concept usage, such as topic repetition, introduction, reiteration, consistency, and novelty by one or more participants in the short- medium-, and long-term range for their own utterances and, if applicable, those of the conversation partner. As an example, the “Longterm Topic Consistency Other” metric measures the degree to which utterances of a given speaker repeat concepts brought up by their conversation partner over the entire conversation. These metrics and primitives were introduced under the banner of Multiple Participant Recurrence (MPR) metrics and have been used in a range of studies, but mostly in health communication [16–18].
Tolston et al. [19] further refined the RQA measures proposed by Angus et al. [13] through creative combinations of these RQA metrics that they revealed offered strong correlative power to observed communicative states. A multiscale (hierarchical) approach to measuring recurrence between individuals and groups of speakers in multiparty discourse was also introduced [20].
In addition to studies of the lexicosemantics of transcribed speech and written texts, there have been many studies across other important discursive modalities including gaze [21, 22], bodily movements [23], gesture [24], prosody [25], and speech acoustics [26]. Many of these studies have tended toward dyads (conversations or interactions between two people), and much of the focus has been on shared attention or mirroring of specific conversational behaviors, for example shared gaze, or mimicry of gestures. We will return to this later when discussing issues regarding non-lexicosemantic modalities, and also on the difference between dyads and multiparty conversation.
A summary and synthesis of many of the aforementioned approaches was offered by Leonardi [27]. In addition, the availability of practical instruction on the design and implementation of recurrence analyses of language data have also been made available by Wallot [28] and, Wallot and Leonardi [29].
The Discursis Software
As mentioned above, conceptual recurrence plots were first proposed by Angus et al. [12], and soon after extended to include a range of bespoke recurrence quantification metrics tailored specifically to multi-party conversation [13]. These recurrence approaches were packaged together within a standalone software toolkit known as Discursis.
Discursis was developed primarily for communication researchers as an analytical support tool that can process text transcripts of temporally organized texts, including conversation, social media, or other discourse data. The creators of the technique made their intention clear that as a technique it does not replace the need for human analysts, “rather they are tools to help analysts perform and draw greater insight from their data” [14]. This responds to a key point of methodological friction, as one of the more active disciplines that is involved in the study of discourse, Conversation Analysis (CA) [1], is fiercely defensive of the use of qualitative close reading of conversation as a foundation of any analysis. Many adherents of CA are skeptical of computational methods, or anything that they deem moves them as an analyst “away from the data”.
Despite the skepticism above, for the past two decades, computer-aided qualitative data analysis software (CAQDAS) have been used to provide semi-automated analysis of conversational data [30–35], enhancing traditional analysis methods through informative and interactive graphical interfaces. CAQDAS systems build data relationships based on user coding which allows users to input variables and visualize the relationships between them. Such systems include the use of text, audio, and video data to measure various features associated with discourse analysis, including but not limited to: time that participants spend talking; voice pitch and prosody; and identification of participant dominance during discourse. Examples of well-established CAQDAS systems include NVivo [36], ATLAS.ti [37], and MAXQDA [38].
Discursis has been positioned as a CAQDAS system (direct reference being made in [14]), despite it also allowing more quantitative RQA analysis to be performed. Discursis' user interface allows analysts to quickly load a conversation transcript and visualize a recurrence plot, and also export metric data that can be used directly, or further processed to derive RQA measures.
Discursis' analytical process starts with the loading of a conversation text. The software accepts input text transcripts as comma separated value formatted text, where each line represents a “turn” in a conversation. The only mark-up required in the transcript is the lexical information. This text is first used to inform the construction of a statistical language model using word occurrence and co-occurrence statistics derived from only this input text under analysis (facility does exist though to pre-seed this model with other texts). The software then tags each line in the input transcript based on each segment's conceptual content. As an example, if the words dog, ball, pet, and food, are strong evidence for the concept dog, and enough of these terms appear in a text segment the concept dog will be tagged against that segment. The more evidence, and more selectively words appear together, the stronger such associations become.
While Discursis has most commonly employed the aforementioned bag-of-words natural language processing approach, it is entirely plausible to substitute other language processing approaches. The key principle of using recurrence plotting to compare utterances for their semantic overlap is able to be achieved using a number of conventional language processing toolkits. Although unpublished, a new prototype of Discursis is currently being developed using the top-down ontologically-driven Paraphrase model [39].
“Natural” Language
Recurrence plotting of language focussing on the analysis of semantics has done so primarily using lexical, symbolic and syntactic approaches that measure recurrence of alphabetic characters, single words, and higher order concepts [8–11, 13, 40]. RQA measures have also been used in similar cases [8–11, 13].
As many of the prior examples demonstrate, the use of recurrence-based approaches to study language is gaining traction, and as part of this growth in interest we have also seen the field grapple more with different forms of linguistic data. While language data is often transcribed into text, transcribed spontaneous speech and written text (prose) are two very different phenomena. And even within these classes, there is an incredible degree of diversity in the forms of speech and text data to be found, i.e., not all conversation can be considered the same.
The diverse nature of text and language data seems self-evident, however there can be a tendency in practice to allow data formats dictate the choice of methodology, rather than considering the data type and source context be the primary guiding mechanisms behind methodological decision making. In practical terms, rather than asking “I have conversation data that is in a text-based format, what methods allow me to process text?” researchers would be better to frame decision making around “I have conversation data from a specific domain that is formatted as text, what methods help me to answer my research questions?” This speaks closely to points made by Fusaroli et al. [6] on the need for theory to enrich recurrence analyses of language, in this case how theory can bring important domain-specific considerations that go deeper than surface-level considerations around data formats.
An example of how communication theory and recurrence plotting can work together can be found in studies that have combined Communication Accommodation Theory (CAT) [41] with conceptual recurrence plots [15, 18, 42]. CAT argues that conversational participants adjust their speech, vocal patterns, physical gestures, and posture, to accommodate (or not accommodate) other participants. One accommodation process described by CAT is convergence, characterized by participants' attempts to align (accommodate) the behaviors of their interlocutors, often as an attempt to minimize social distance. Using conceptual recurrence approaches, the physical manifestations of these convergence behaviors using transcribed speech can be quantified using a recurrence analysis approaches.
Theories such as CAT allow researchers to deal with the messy, and context-sensitive nature of communication data. Within the domains of speech and literature, data is complicated due to the many varied norms and practices that are dependent on contexts that may be informed by social traditions, technological affordances, limits of biology, or other conventions. As Moerman put it “Unless we know how occasions of speech are socially organized, we can neither fully understand nor properly evaluate our data. We collect cultural artifacts that come mounted in a context that gives them their momentary enlivened meaning. We must preserve their interactional matric, not pretend to scoop nuggets from a swamp” [43]. Communication norms are shaped by various exogenous and endogenous social forces, and are neither devoid of context, nor static, as the example from Dale and Spivey [11] indicates with regard to the changing speech patterns and language learning by children. While conversations are defined by many layers of complexity, this does not mean that recurrence plotting can't help in revealing interesting and informative aspects of them. In their study of 101 television talk show “Andrew Denton's Enough Rope” interviews, Angus et al. [44] revealed how communication norms are shaped by particular speaker roles, evidenced by distinctive recurrence patterns that aligned to these expectations (see Figure 2).
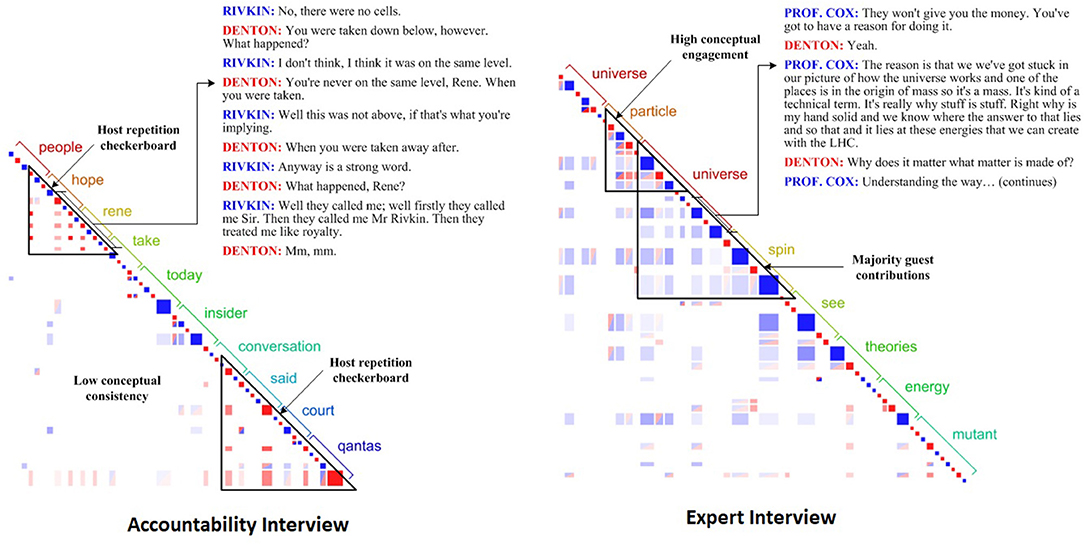
Figure 2. Conceptual recurrence plots of accountability and expert interviews from the Enough Rope television program. Reproduced from Angus et al. [44] with permission.
Television (TV) talk shows are considered to be profoundly different to spontaneous conversations unfolding in everyday life [45]. TV talk shows are based on conversations between a host and their interview partners. The norms that guide conversational behavior throughout these interviews will typically arise from obligations to fulfill the interests of media networks, sponsors, audiences, and those of the respective institutions represented by the host and, if applicable, the guest. As talk show conversations unfold, hosts and guests interact via asking and answering questions, making statements, and so forth, thereby “talking content into being”, or “mutually creating an account” of a given topic, event, or experience [45]. This process is characterized by a continuous negotiation of both the interview content and the roles of the interviewer (host) and interviewee (guest) while they interact for the benefit of an “overhearing audience” [45–47]. This complex host-controlled, guest-shaped, audience-oriented environment affects any speech act occurring within the talk show interview, making it non-commensurate with other “ordinary” spontaneous private conversations.
Recurrence plots rather than RQA were key to the analysis of Enough Rope [44], principally due to their ability to be used as highly salient visual evidence of specific communication practices. Returning to points made earlier with regard to the use (and higher preference in some cases) of qualitative methods within the field of discourse analysis, the recurrence plot allow connections to be made between known communicative repertoires and visual motifs. The task then is to educate a reader on how to read such a plot and interpret its findings by link those motifs with their existing highly nuanced understanding of communication norms and behaviors. This is not a new theme in recurrence analysis, with many practitioners being highly adept at recognizing specific recurrence motifs, and being able to link them to known functions. The challenge is in convincing a field that has historically shunned the use of visualization and numerical methods to find value in these new approaches.
Back to the point raised on the specificity of conversation though, and how discourse is shaped by various factors that situate outside of the conversation as it is recorded and transcribed. The situated nature of conversation and language should not be used to dampen enthusiasm for translating methods across domains, or used to suggest that methods or insights gained from studying one genre are wholly unsuitable for another. This said, care must be taken. In searching for patterns of recurrence the most informative aspects of these differing forms of communication can vary substantially. To return to example of poetry, we can find that word orders, repetition, syntactic rules, pagination, and grammars are all fair game for a poet to mix and recast in their own creative way. And that in the case of poetry recurrence analysis is a useful way to reveal signature styles and (a)symmetries [8]. However, it may not be true that word order choices are as informative in a study of doctor/patient communication.
The need for context sensitivity was strongly evident in work that we performed (and that is still ongoing) on the analysis of Dementia conversation. Having previously applied conceptual recurrence plotting to the analysis of doctor/patient communication [15], in our first application of the technique to conversations between care providers and people living with dementia [48] we were startled by the relatively low level of recurrence displayed in plots generated from transcribed conversations. In working with our collaborators (speech pathologists and social psychologists with decades of combined experience in the linguistics and communicative effects of dementia), we soon learned that what we (computer scientists) had considered to be low levels of recurrence, were from their standards very high levels of within and between speaker recurrence, given the severity of the impact of dementia on speech and language processing in the cases under analysis. Without domain expertise, and insight into the nature of the recordings, the results of this analysis may have been easily dismissed as inconclusive. This is also a cautionary point on the sharing of data, and of conversation data in particular, for what value does this data have if it is not also accompanied by insight?
Wallot [28] captured many of the points raised above through their analysis of the spoken performance of written texts. Wallot's analysis showed how rhythms of the spoken production by readers of the text varied across a cohort, and looked to key demographic information to hypothesize what may impact this variation. Although never intended to be part of their analysis, it is clear how an analysis of the simple lexical transcripts of these monologs alone, without considering the prosody of the speech would have yielded little insight.
In this section we have considered how conversations are more than simply information passing, and how context can shape the process of human interaction. In many ways this is the greatest strength of dynamical systems approaches, as they consider systems under analysis to be complex. What is clear though is that language data is often messy, and choices that we make with regard to the recording, encoding and transcription of data can have a profound impact on downstream analyses.
Time and Encoding
Time is a central factor in the study of language. Within studies of conversation the organization of talk and pause have been studied extensively [49, 50] where much of the work has been on describing turn-taking and sequence organization, and psychosocial correlates to talk organization and timing.
Recurrence analyses of language tend to begin from two dominant starting points, one being to organize input discourse along a regular sampling interval, such as the case with recurrence of symbols (letters), words, or those of non-lexical features such as speech acoustics. The other starting point is to sample from time in a non-uniform manner, such as the case of conceptual recurrence plots that discretize speech into turns. Where a turn can constitute something as simple as a single barely audible grunt, to a long monolog.
The choice of sampling strategy can largely impact the visibility of micro and macro visual patterns in recurrence plots of discourse. Particularly when it comes to approaches such as the conceptual recurrence plotting method, a long turn will often contain a more diverse vocabulary, and therefore be more likely to be encoded with a denser conceptual vector (leading to higher chance of recurrence with other turns), whereas, small turns are less likely to have dense concept vectors leading to lower recurrence scores. To illustrate this case, we present two conceptual recurrence plots of an Enough Rope conversation where Prof Brian Cox, a well-known physicist and science communicator, is interviewed by Andrew Denton. These plots are both generated using the same conceptual mapping, the only difference is that one uses the length of turns to scale the recurrence elements, the other uses a uniform mapping where every turn is the same size (see Figure 3). In this example the use of uniform non-proportionately-sized squares fails to visually capture Prof Cox's turn-taking and interaction dynamics as well as the proportionately-sized squares (that he takes longer turns, and repeats his own concepts preferentially).
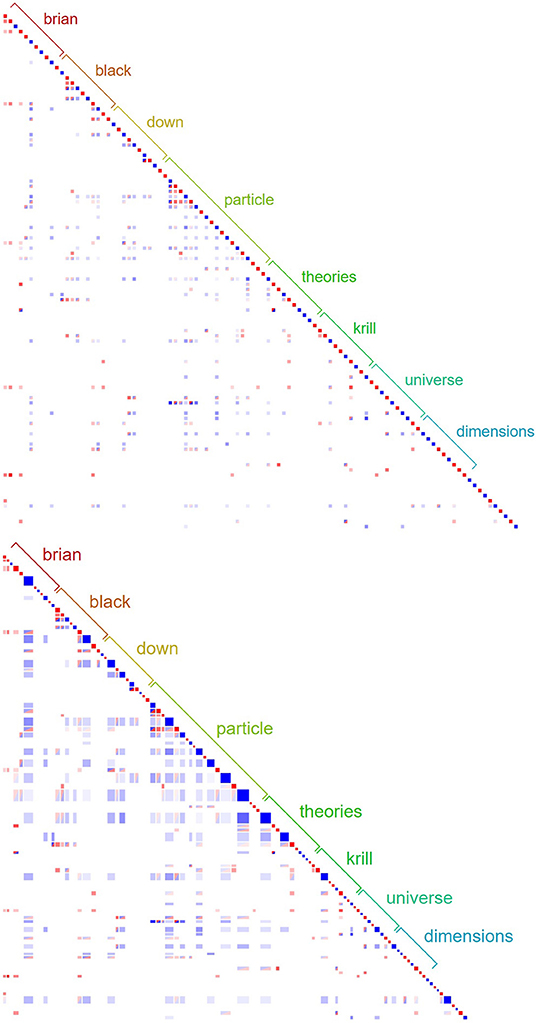
Figure 3. Comparing conceptual recurrence plots with regular vs. proportionally sized recurrence elements (proportional size is based on length of conversation turns).
Time as a critical dimension in the study of discourse is further complicated due to the messy nature of talk and interaction. Speakers in a conversation do not necessarily wait to speak in an orderly fashion, rather turn structure includes overlaps, pause, and delays that can vary according to the many contextual and cultural factors spoken of previously. This is addressed by Fusaroli and Tylén [25] in the development of a multi-modal RQA approach which specifically models pause/speech by individual speakers. Another approach to this issue is that of PauseCode [51], an open source speech/pause coding toolkit designed to encode multi-party speech data into discrete codes that indicate state transitions in patterns of speaker talk as a pre-cursor to recurrence or other statistical analysis.
Many of these issues with regard to the temporal encoding in conversation are brought about by the presence of multiple speakers. Fusaroli et al. [6] observe how the majority of conversational recurrence studies have tended toward dyads, or monologs. The challenge they identify is how to preserve group dynamics when analyzing multi-party conversation, pointing to techniques from joint recurrence, network theory, or probabilistic graphical models as potential avenues for exploration. One solution that emerged in response is that of pyramid of conceptual recurrence metrics [20].
The pyramid of conceptual recurrence creates a hierarchy of group membership amongst conversational participants to accumulate recurrence between participants based on group (non)membership (see Figure 4). A set of RQA-inspired metrics are included that seek to capture interaction across whole conversation, group-group, person-person, and turn-turn time scales. Conceptual recurrence rate (CRR) seeks to determine the overall amount of conceptual recurrence for a given interaction:
Where N is the total number of conversation turns (utterances), and Ri,j is the recurrence element at time points i and j.
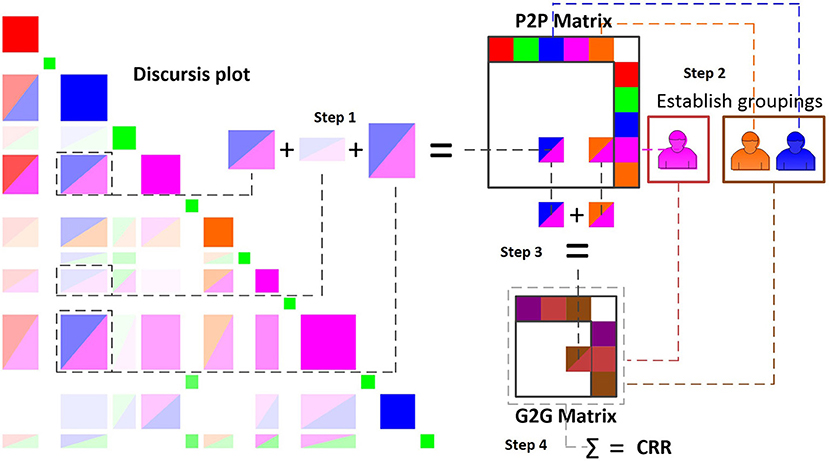
Figure 4. CRQA pyramid measures. This diagram [reproduced from Angus and Wiles [20] with permission] shows how recurrence can be aggregated over different levels, from individual interlocutors to social groups.
The Group-group (G2G) and Person-person (P2P) measures seek to determine the degree of conceptual recurrence between groups and individual conversation participants, respectively. These measures are formulated similarly, the difference between them principally being the corresponding membership vectors which determine the individual or group-based membership of each turn:
Where ga and gb are vectors that denote the time points of utterances by groups a and b respectively, and Ri,j is the recurrence element at time points i and j.
The resulting G2G and P2P metrics can be used to inform the creation of “social semantic networks” which display the degree of conceptual recurrence between participants in a network, and color nodes according to social groups to which they belong. The analysis included in Angus and Wiles [20] includes several large panel talk shows where up to 30 participants are interacting over 1 h, and reveal how experts and laypeople differ in their conversational norms and behaviors based on the values of recurrence measures.
Modality
Although the speech/verbal modality is considered to be a very robust communication channel in any social interaction, it is well-established that when available, non-verbal modalities are important in almost any spoken interaction [52, 53]. Non-verbal modalities involve observable changes such as hand and body gestures, eye movements, and facial expressions [54].
Previous research on the dynamics of non-verbal interaction indicates that while many social cues are intentional (i.e., a conscious drive to accomplish specific aim within the discussion), many of the other signals are the consequence of unintentional and unconscious processes [55]. Additionally, humans involved in an act of communication can recognize social cues quickly, unconsciously and with high accuracy. There is also a significant work related to more complex processing of these signals in social situations related to social identities, high level relationships of our social world based on deeper internal conditions of participants [56]. It is also known that social behavior and social constructs are regulated by the demonstration and recognition of non-verbal signals, in many circumstances without depending on spoken language at all [55].
While studies of single modalities are important, present there is a call for more research that can bridge different modalities. Underpinning this call is a need for methods that can bridge modalities and faithfully combine information from these various communication channels to better understand speaker behaviors. Recurrence plotting and recurrence quantification analysis are clearly suited to this roll, and the work of Fusaroli and Tylén [25] is particularly important in this regard as it offers an approach to investigate multiple aspects of communicative symmetry simultaneously, in this case prosody, lexical choice and pause/speech. Despite this excellent work, data encoding and the non-uniformity of time across different social processes still looms as a significant challenge for progress on solving the challenge of multi-modality. Cross recurrence methods require that time series information be sampled at the same rate, or at the very least be regularly sampled. However, as discussed in the previous section, social processes operate on different time scales [noted also as a key challenge by Fusaroli et al. [6]], and encodings of these processes can be non-uniform in time. This is as relevant for those using recurrence methods in other fields where processes occur across massively different time scales, yet model fidelity requires the incorporation of information from these multiple different modalities, e.g., earth sciences, or physiology.
The challenges of multi-modal communication analysis are greatly assisted through the collection and provision of open source datasets by members of the multimodal interaction community. Much of this growth is spurred on by the development of human-machine interaction systems, and the commercial interest in speech-enabled mobile devices. As one example, Czyzewski et al. [57] released a corpus containing 31 h of recordings which includes high-resolution, high-framerate stereoscopic video, multi-channel audio, and manually annotated lexical transcripts. This dataset, and others, provide diverse materials to develop and test new multi-modal recurrence approaches.
A final consideration for multi-modality relates to the decision on where along the processing pipeline information is combined or utilized. As in the case of the language recurrence methods, some studies have generated recurrence from letters, some from words, and others from concepts. Each can be considered a level of abstraction higher than the last, each incorporating and aggregating more information before subjecting discrete input units to recurrence comparison operations. For example, we could consider an option that extra modalities such as pitch, speech intensity, or other prosodic markers could be incorporated as an extra layer of abstraction prior to any similarity calculations that would be the basis of the construction of a recurrence plot. However, the risk with any approach in in reducing the complexity of the system under study and missing critical non-linearity.
Conclusion
The focus of this position piece was on how recurrence methods have been utilized in the study of human discourse, framed specifically from a Communication Studies perspective. Our positions focussed along several key dimensions: discourse type, the role of time in the encoding of input data, and the challenges that multi-modality place on recurrence-based discourse analysis methods.
With regard to discourse type, we focussed on issues of the need for context sensitivity when it comes to recurrence-based analysis of discourse, and on how theoretical perspectives such as that of Communication Accommodation Theory can act as a useful anchor to generalize and contextualize findings. We then touched on issues of time and data encoding, discussing challenges regarding non-uniformity of sampling in discourse data, and also of multi-party discourse. One solution for analyzing multi-party discourse, the pyramid of conceptual recurrence approach was discussed. Lastly we touched on issues of multi-modality, and how time and encoding present extra challenges to multi-modal recurrence methods. Without diminishing the difficulties faced with regard to the required methodological and theoretical development, we also spoke of how the growth in data availability from the field of multi-modal interaction may assist in future exploration.
It is clear that recurrence analysis of communication data has come a long way over the past two decades. And that the paired approaches of visual recurrence plots, and recurrence quantification analysis each provide important entry points for scholars from both qualitative and quantitative traditions. I believe that the coming years will see exciting new advances and answers to many of the challenges identified here, and a continued growth in acceptance of these powerful methods amongst members of the discourse analysis community.
Author Contributions
The author confirms being the sole contributor of this work and has approved it for publication.
Funding
This work was supported by the Australian Research Council Centre of Excellence for the Dynamics of Language (CE140100041).
Conflict of Interest
The author declares that the research was conducted in the absence of any commercial or financial relationships that could be construed as a potential conflict of interest.
References
1. Schegloff EA. Sequence Organization in Interaction: A Primer in Conversation Analysis I. Cambridge: Cambridge University Press (2007). doi: 10.1017/CBO9780511791208
2. Marwan N, Carmen Romano M, Thiel M, Kurths J. Recurrence plots for the analysis of complex systems. Phys Rep. (2007) 438:237–329. doi: 10.1016/j.physrep.2006.11.001
3. Webber CL Jr, Zbilut JP. Dynamical assessment of physiological systems and states using recurrence plot strategies. J Appl Physiol. (1994) 76:965–73. doi: 10.1152/jappl.1994.76.2.965
4. Zbilut JP, Webber CL Jr. Embeddings and delays as derived from quantification of recurrence plots. Phys Lett A. (1992) 171:199–203. doi: 10.1016/0375-9601(92)90426-M
5. Eckmann JP, Kamphorst SO, Ruelle D. Recurrence plots of dynamical systems. Europhys Lett. (1987) 5:973–7. doi: 10.1209/0295-5075/4/9/004
6. Fusaroli R, Konvalinka I, Wallot S. Analyzing Social Interactions: The Promises and Challenges of Using Cross Recurrence Quantification Analysis. Springer International Publishing (2014). p. 137–55. doi: 10.1007/978-3-319-09531-8_9
7. Marwan N, Wessel N, Meyerfeldt U, Schirdewan A, Kurths J. Recurrence-plot-based measures of complexity and their application to heart-rate-variability data. Phys Rev E Stat Nonlin Soft Matter Phys. (2002) 66:026702. doi: 10.1103/PhysRevE.66.026702
8. Orsucci F, Walter K, Giuliani A, Webber CL, Zbilut JP. Orthographic structuring of human speech and texts: linguistic application of recurrence quantification analysis. Int J Chaos Theory Appl. (1999) 4:21–8.
9. Webber CL, Zbilut JP. Recurrence quantification analysis of nonlinear dynamical systems. In: Riley M, Van Orden G, editors. Tutorials in contemporary nonlinear methods for the behavioral sciences (2005). p. 26–94. Available online at: https://www.nsf.gov/pubs/2005/nsf05057/nmbs/preface.pdf
10. Dale R, Spivey MJ. Categorical recurrence analysis of child language. In: Proceedings of the 27th Annual Meeting of the Cognitive Science Society. (2005). p. 530–5. Retrieved from: https://escholarship.org/uc/item/0wz7s448
11. Dale R, Spivey MJ. Unraveling the Dyad: using recurrence analysis to explore patterns of syntactic coordination between children and caregivers in conversation. Lang Learn. (2006) 56:391–430. doi: 10.1111/j.1467-9922.2006.00372.x
12. Angus D, Smith A, Wiles J. Conceptual recurrence plots: revealing patterns in human discourse. IEEE Trans Vis Comput Graph. (2012) 18:988–97. doi: 10.1109/TVCG.2011.100
13. Angus D, Smith A, Wiles J. Human communication as coupled time series: quantifying multi-participant recurrence. IEEE Trans Audio Speech Lang Process. (2012) 20:1795–807. doi: 10.1109/TASL.2012.2189566
14. Angus D, Rintel S, Wiles J. Making sense of big text: a visual-first approach for analysing text data using Leximancer and Discursis. Int J Soc Res Methodol. (2013) 16:261–7. doi: 10.1080/13645579.2013.774186
15. Angus D, Watson B, Smith A, Gallois C, Wiles J. Visualising conversation structure across time: insights into effective doctor-patient consultations. PLoS ONE. (2012) 7:e38014. doi: 10.1371/journal.pone.0038014
16. Atay C, Conway ER, Angus D, Wiles J, Baker R, Chenery HJ. An automated approach to examining conversational dynamics between people with dementia and their carers. PLoS ONE. (2015) 10:e0144327. doi: 10.1371/journal.pone.0144327
17. B.-Whelan M, Angus D, Wiles J, Chenery HJ, Conway ER, Copland DA, et al. Toward the development of SMART communication technology: automating the analysis of communicative trouble and repair in dementia. Innov Aging. (2018) 2:igy034. doi: 10.1093/geroni/igy034
18. Watson BM, Angus D, Gore L, Farmer J. Communication in open disclosure conversations about adverse events in hospitals. Lang Commun. (2015) 41:57–70. doi: 10.1016/j.langcom.2014.10.013
19. Tolston MT, Riley MA, Mancuso V, Finomore V, Funke GJ. Beyond frequency counts: novel conceptual recurrence analysis metrics to index semantic coordination in team communications. Behav Res Methods. (2019) 51:342–60. doi: 10.3758/s13428-018-1132-6
20. Angus D, Wiles J. Social semantic networks: measuring topic management in discourse using a pyramid of conceptual recurrence metrics. Chaos. (2018) 28:085723. doi: 10.1063/1.5024809
21. Bedford R, Wagner NJ, Rehder PD, Propper C, Willoughby MT, Mills-Koonce RW. The role of infants' mother-directed gaze, maternal sensitivity, and emotion recognition in childhood callous unemotional behaviours. Eur Child Adolesc Psychiatry. (2017) 26:947–56. doi: 10.1007/s00787-017-0967-1
22. Richardson DC, Dale R, Kirkham NZ. The Art of conversation is coordination. Psychol Sci. (2007) 18:407–13. doi: 10.1111/j.1467-9280.2007.01914.x
23. Demos AP, Chaffin R, Kant V. Toward a dynamical theory of body movement in musical performance. Front Psychol. (2014) 5:477. doi: 10.3389/fpsyg.2014.00477
24. Coco MI, Dale R. Cross-recurrence quantification analysis of categorical and continuous time series: an R package. Front Psychol. (2014) 5:510. doi: 10.3389/fpsyg.2014.00510
25. Fusaroli R, Tylén K. Investigating conversational dynamics: interactive alignment, interpersonal synergy, and collective task performance. Cogn Sci. (2016) 40:145–71. doi: 10.1111/cogs.12251
26. Schultz A. Recurrence quantification analysis of speech. J Acoust Soc Am. (2006) 119:3339. doi: 10.1121/1.4786429
27. Leonardi G. The study of language and conversation with recurrence analysis methods. Psychol Lang Commun. (2012) 16:165–83. doi: 10.2478/v10057-012-0012-x
28. Wallot S. Recurrence Quantification Analysis of Processes and Products of Discourse: A Tutorial in R. Discourse Process. (2017) 54:382–405. doi: 10.1080/0163853X.2017.1297921
29. Wallot S, Leonardi G. Analyzing multivariate dynamics using cross-recurrence quantification analysis (CRQA), diagonal-cross-recurrence profiles (DCRP), and multidimensional recurrence quantification analysis (MdRQA) – a tutorial in R. Front Psychol. (2018) 9:2232. doi: 10.3389/fpsyg.2018.02232
30. Fielding N, Warnes R. Computer-based qualitative methods in case study research. In: Byrne D, Ragin CC, editors. The Sage Handbook of Case-Based Methods. Los Angeles, CA: Sage (2009). p. 270–88.
31. Krippendorff K. Content Analysis: An Introduction to Its Methodology. 3rd Edn. Thousand Oaks, CA: Sage (2012).
32. Ronen Feldman JS. The Text Mining Handbook: Advanced Approaches in Analyzing Unstructured Data. New York, NY: Cambridge University Press (2006).
33. Schönfelder W. CAQDAS and Qualitative Syllogism Logic - NVivo 8 and MAXQDA 10 Compared. Qualitative Social Research, 12 (2011). Retrieved from: http://www.qualitative-research.net/index.php/fqs/article/viewArticle/1514
34. Seale C. Using computers to analyse qualitative data. In: Silverman D, editor. Doing Qualitative Research: A Practical Handbook. London: Sage (2010). p. 251–67.
35. Smith AE. Machine mapping of document collections: The Leximancer system. In: Paper Presented at the Proceedings of the Fifth Australasian Document Computing Symposium. Sunshine Coast, QLD (2000).
36. QSR International Pty Ltd. NVivo Qualitative Data Analysis Software [Online], Version 10. QSR International Pty Ltd. (2012). Available online at: http://www.qsrinternational.com/
37. Atlas.ti Qualitative Data Analysis Software [Online] (1993). Available online at: http://www.atlasti.com/
38. VERBI Software. MAXQDA 2018 [Computer Software]. Berlin: VERBI Software (2017). Available online at: https://www.maxqda.com
39. Pavlick E, Bos J, Nissim M, Beller C, Van Durme B, Callison-Burch C. Adding semantics to data-driven paraphrasing. In: Proceedings of the 53rd Annual Meeting of the Association for Computational Linguistics and the 7th International Joint Conference on Natural Language Processing (Volume 1: Long Papers). Beijing (2015). p. 1512–22. doi: 10.3115/v1/P15-1146
40. Church KW, Helfman JI. Dotplot: a program for exploring self-similarity in millions of lines of text and code. J Comput Graph Stat. (1993) 2:153–74. doi: 10.1080/10618600.1993.10474605
41. Gallois C, Ogay T, Giles H. Communication accommodation theory: a look back and a look ahead. In: Gudykunst W, editors. Theorizing About Intercultural Communication. Thousand Oaks, CA: Sage (2005). p. 121–48.
42. Chevalier B, Watson B, Barras M, Cottrell W, Angus D. Using discursis to enhance the qualitative analysis of hospital pharmacist-patient interactions. PLoS ONE. (2018) 13:e0197288. doi: 10.1371/journal.pone.0197288
43. Moerman M. Talking Culture: Ethnography and Conversation Analysis. University of Philadelphia, PA: Pennsylvania Press (2010).
44. Angus D, Fitzgerald R, Atay C, Wiles J. Using visual text analytics to examine broadcast interviewing. Discourse Context Media. (2016) 11:38–49. doi: 10.1016/j.dcm.2015.11.002
45. Hutchby I. Media Talk - Conversation Analysis and the Study of Broadcasting. Berkshire: Open University Press (2006).
46. Heritage J. Analysing news interview: aspects of the production of talk for an overhearing audience. In: Van Dijk T, editors. Handbook of Discourse Analysis. London: Academic Press (1985).
47. Heritage J, Greatbatch D. On the institutional character of talk: the case of the news interview. In: Boden D, Zimmerman DH, editors. Talk and Social Structure. Cambridge: Polity Press (1991).
48. Baker R, Angus D, Smith-Conway ER, Baker KS, Gallois C, Smith A, et al. Visualising conversations between care home staff and residents with dementia. Ageing Soc. (2015) 35:270–97. doi: 10.1017/S0144686X13000640
49. Gallois C, Markel N. Turn taking: social personality and conversational style. J Pers Soc Psychol. (1975) 31:1134. doi: 10.1037/h0076949
50. Levinson SC, Torreira F. Timing in turn-taking and its implications for processing models of language. Front Psychol. (2015) 6:731. doi: 10.3389/fpsyg.2015.00731
51. Angus D, Yu Y, Vrbik P, Back A, Wiles J. PauseCode: computational conversation timing analysis. In: Proceedings of the 4th International Workshop on Multimodal Analyses Enabling Artificial Agents in Human-Machine Interaction. Boulder, CO: ACM (2018). p. 43–7. doi: 10.1145/3279972.3279975
53. Argyle M. Non-Verbal Communication in Human Social Interaction. Oxford, UK: Cambridge University Press (1972).
54. Bargh JA. Social Psychology and the Unconscious: The Automaticity of Higher Mental Processes. New York, NY: Psychology Press (2013). doi: 10.4324/9780203783016
55. Hassin RR, Uleman JS, Bargh JA. The New Unconscious. Oxford Series in Social Cognition and Social Neuroscience. New York, NY: Oxford University Press (2005). doi: 10.1093/acprof:oso/9780195307696.001.0001
56. Choudhury T, Pentland A. Modeling Face-to-Face Communication Using the Sociometer. Technical Report, MIT Media Lab (2003). Retrieved from: https://www.media.mit.edu/publications/modeling-face-to-face-communication-using-the-sociometer-2/
Keywords: recurrence, conversation, visualization, language, discourse
Citation: Angus D (2019) Recurrence Methods for Communication Data, Reflecting on 20 Years of Progress. Front. Appl. Math. Stat. 5:54. doi: 10.3389/fams.2019.00054
Received: 31 May 2019; Accepted: 16 October 2019;
Published: 05 November 2019.
Edited by:
Charles L. Webber Jr., Loyola University Chicago, United StatesReviewed by:
Meysam Hashemi, INSERM U1106 Institut de Neurosciences des Systèmes, FranceM. Sambath, Periyar University, India
Massimo Rizzi, Istituto Di Ricerche Farmacologiche Mario Negri, Italy
Copyright © 2019 Angus. This is an open-access article distributed under the terms of the Creative Commons Attribution License (CC BY). The use, distribution or reproduction in other forums is permitted, provided the original author(s) and the copyright owner(s) are credited and that the original publication in this journal is cited, in accordance with accepted academic practice. No use, distribution or reproduction is permitted which does not comply with these terms.
*Correspondence: Daniel Angus, ZGFuaWVsLmFuZ3VzQHF1dC5lZHUuYXU=