- Departamento De Ciencias Básicas, Universidad Autónoma Metropolitana at Azcapotzalco, Azcapotzalco, Mexico
Stylized facts appear in electoral processes worldwide, from Brazil to India. Here, we update a statistics carried on in Mexican elections but considering the inhomogeneities in electoral districts through the Nominal List (NL) (the list of valid electors in a given decision process) for the last three presidential elections. We find that the NL distribution at polling station detail is composed of, at least, three windows with a step function structure. Next, we study the consequences of the windows structure for the statistical properties of the processes. We obtain that the asymmetric vote distribution by polling station recovers a Gaussian shape for two of the windows; meanwhile, the standardized distribution of votes follows a distorted Gaussian, near to a skew normal. The distribution of the turnout at each polling station or voters’ participation ratio is close to a skew normal one in the bulk and failing at the wings. The average of voters increases in a linear way with the Nominal List and depends on the window considered. The results do not depend on the municipality, political district or urban versus nonurban distinction, and the electoral process considered.
1 Introduction
Regularities and irregularities in electoral systems are a matter of interest for citizens, politicians, social and political scientists, physicists, and mathematicians. The few decades of digital records allowed the advancement of the so-called sociophysics (and econophysics) and the widespread use of the electoral forensics (see, for instance, Mebane et al. (2014) and Baltz et al. (2018) and references therein). Using the classification given in Schweitzer (2018), the major subareas in the interest of physicists are computational social science, complex networks, and data-driven models. Rewriting the classification, we have the area of theoretical models, the area of analysis of stylized facts, and the blurred interplay or interdisciplinary field. The present work focuses on the stylized facts’ area.
The statistical analysis of elections in Mexico using official data in order to demonstrate violations to the electoral laws starts with the conflictive election of 1988 with two works (Barberán et al., 1988; Auping-Birch, 1988). This historical event generated the creation and evolution of many more professional and impartial electoral authorities and the existence of available records of the electoral process. One of the duties of the new authorities was the conformation of a reliable electoral list of possible voters, the Padrón Electoral, and the Nominal List (NL), the list of allowed voters in a particular process. A recent analysis on the fraud fingerprints of that election was conducted by Cantú (2019), and a recent review on the Mexican presidential elections was performed by Ortega (2017).
With the evolution of technology, in Mexico and in many other countries, the existence of digital records allows the forensic of electoral data in an easy way. Hence, even nonexperts or outsiders can analyse the data. Such people are physicists and mathematicians; see the book of P. Ball for an introduction (Ball, 2004). However, the complexity of such a matter gives place to misunderstandings or extrapolations on the subject and conditioning the answers to the discovery of violations to the rules. Part of the confusion is trying to assume that the systems follow the same rules as the uncorrelated particles of simple physical models. In physics and in societal systems, correlations are important. Discovering true deviations is a hard task, and we need better tools, as those presented in, for example, reference (Klimek et al., 2012).
The existence of complex relations between the components of elections makes that an exhaustive analysis of the details in order to understand the source of regularities and irregularities. In the present work, we discuss the internal inhomogeneities in the Nominal List elections at polling station degrees of aggregation and their consequences in the statistics; in particular, we selected the voters’ participation and turnout distributions.
An aspect that is usual to analyze is the voters’ turnout, that is, the ratio of the participating voters to those that are allowed to participate. The overall statistics is a number usually reported in the media and in the electoral authorities’ official sites. However, such a statistics can be performed according to some degree of aggregation and, depending on the sample, follows a distribution, to say,
for the number of voters, where
As a motivation, we performed an exercise. In Figure 1, we plot some of the statistical quantities that shall be discussed in this work but for the 1988 presidential election. In the upper panel, we plot an approximation to the participation ratio at the electoral district level of aggregation for the presidential (black histogram) and deputies’ (blue histogram) cases. Even in this exercise, it is clear that there exist a larger number of districts with participation ratio near to one for president compared with deputies. Also, in both cases, the votes are not normally distributed. The normal fitting appears in broken lines, in black for deputies and in red for president. The number of votes and the number of allowed voters for deputies were taken from Centro de Estudios de la Democracia y Elecciones (1991) based on official sources. The total votes for president in each of the 300 districts were taken from Dictamen del Colegio Electoral (1994). Because in such a report the Nominal List was absent, we used the number appeared in the deputies’ database; hence, the results for the presidential case is, we hope, an approximation. This lack of reliable eligible voters or valid lists of voters for that election was part of the suspicion of fraud. In a study by Cantú (2019), an analysis on the official tallies was performed looking for the fraud fingerprints, but it was lacking a close analysis of the irregular data in the list of eligible voters. In the inferior panel of Figure 1, we plot the heat map of the “Nominal List” versus the total number of votes in each district for the 1988 presidential election. Neither the distribution of votes (histogram at the top) nor the distribution of this NL (histogram at the right) is a smooth function. Stricto sensu, the histogram of the NL, corresponds to the deputies’ election. We include this exercise in order to explore the results for a widely accepted fraudulent election with a nonnormal distribution for neither the participation ratio nor the distribution of votes. In an ulterior work, we shall present the distributions with the recorded and assumed fraudulent numbers in the official tallies using the data from Cantú (2019), since to the best of our knowledge, there is no digital record of them.
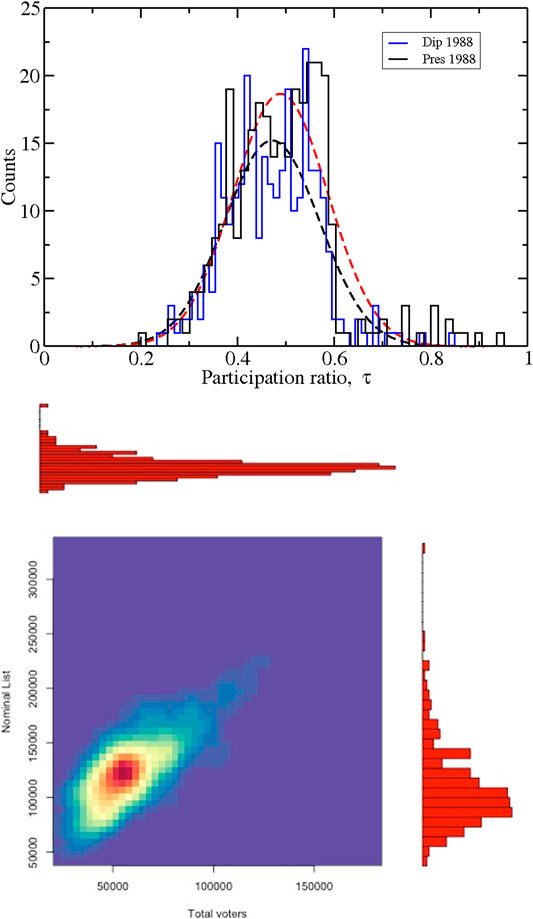
FIGURE 1. In the upper panel, we present the participation ratio, τ, for the deputies (blue histogram) and presidential case (black histogram) for the 1988 election for the 300 districts. The normal fitting appears for each case as the broken line. In the lower panel, as a heat map, the distribution of Nominal List and the total number of voters of the presidential election are presented. The corresponding histograms appear at the right and top. We use the official results published in Dictamen del Colegio Electoral (1994) for the number of votes and the data for deputies published in Centro de Estudios de la Democracia y Elecciones (1991) for the Nominal List. The later number was one of the reasons (among many others) that the 1988 election was considered rigged. So, the results for president are an approximation and for the presidential case is an exercise. Refer to text for details.
It is clear that there exist correlations between the sample size and the allowed participants, even following the rules. Hence, the goal of the present work is to show how “simple” correlations provoke deviation from the Gaussianity or the law of the large numbers. As we shall see, the correlations come from the geopolitical structure of the Nominal List. We use the dataset of federal electoral turnout of recent elections in Mexico. Such datasets are reported at the polling station aggregation level. Hence, we first describe the distribution of the sample size. For many years and through several works, such a probabilistic object was considered as a constant or, in the worst of the cases, as an asymmetric peak distribution, no matter if it is a Gaussian or Lorentzian, but we assumed a strongly peaked function around the maximum value accepted by the electoral authorities of 750. Such an assumption is false as we shall see in Section 3.1. The consequences of that are explored in the rest of the article. Previous to that, we describe the game field in the next section.
2 Materials and Methods
The datasets we consider are those published by the electoral authorities, the Instituto Nacional Electoral (INE) or its previous version, the Instituto Federal Electoral (IFE), in the official web pages (INE, 2019; IFE, 2014) consulted recently or in previous years. For a standardized version, we downloaded data from the atlas on electoral results (ATLAS, I. N. E., 2016) for the final results, called conteo distrital or districtal counting. These data correspond to the reviewed results with the political parties and include revision of anomalies reported to the electoral authorities. We considered only federal elections and no local ones; hence, our results concern about national events and with a major interest between the electorates. Datasets from 2006 federal election were compared with old files downloaded by the author during the year 2006.
The recent versions of datasets are in comma-separated values format (csv); hence, it is easy to use R or awk and sed software to clean and submit them to analysis. Older versions could be in Excel (XML) format or worse. For any dataset, a clean process is required and a record of the changes performed is considered.
The electoral distribution has several levels of aggregation, the larger level corresponds to the 300 districts corresponding to the representatives at the low chamber. They must be distributed in electoral sections, each of them with a prescribed amount of electors. The polling stations are splitted up in subsidiary ones, distributed according to the first family name; hence, the main station contains the register of those whose name starts with A up to I, for instance. The “contigua 1” or C1 admits to vote those with family name starting with H–M and so on and so forth. Each station has its list and its cabin but shares the same location, a schoolyard to say. In the dataset, each line corresponds to a poll, no matter if it is the main or the subsidiary. This alphabetical order is of procedural nature, and it does not correlate with sociodemographic variables. Hence, it is expected that considering the precinct does not change the statistics.
Each entry in the recent dataset contains the number of allowed voters in each electoral cabin; it is called the Lista Nominal or Nominal List (NL). Here, we refer to it as the number of allowed voters for the particular election. Such a number was not reported in dataset elections before year 2006. That is the reason why we considered only the last three presidential cases. Additionally, the record includes the special cabins, devoted to valid electors in transit, since they do not have a list and do not know in advance who will vote there. In the dataset, the entry devoted to the NL entry is empty. In all the data files, the field appears empty, and the R selection with the na.omit(y2) option is used. However, for Deputies 2015 (Diputado MR 2015), President 2006 (Presidente 2006), and 2012 (Presidente 2012), the field appears with a value of zero. Anyway, they are not considered for the participation ratio. The statistical analysis is performed using R and Fortran code.
3 Results
The existence of stylized facts in Mexican elections is well established (Borghesi et al., 2012; Hernández-Saldaña, 2013). The origins of them are not well understood, and many of them represent deviations from the large number theorem and are part of the richness in a complex process. Some considerations can help to understand them and cast some light on how we count and how we consider the errors. In the current work, we analyze the voter participation and voter turnout or participation ratio and how they are influenced by the geopolitical configurations of the maximum number of allowed voters in each polling station, named the Nominal List (NL). Hence, we present in the next subsection the distribution of this quantity. In Section 3.2, we shall discuss how the group conformation in the electoral process distorts the voters’ distribution, and in Section 3.3, we shall discuss the participation ratio distribution.
3.1 The Zones in the Nominal List
As mentioned before, the Nominal List (NL) or Lista Nominal for its Spanish denomination is the official record of all the citizens allowed to vote in a particular election, and a particular polling station is assigned to the voter according to its residence place. The electoral map is divided into sections with 100–3,000 electors in accordance with the law (LEGIPE, 2019), and they must fit the 300 low chamber sits. However, a uniform distribution in a heterogeneous country such as Mexico is a difficult task. The last redistricting process (2006 and 2012) was performed using a simulated annealing algorithm in order to optimize the number of possible voters in each precinct. In addition to the simulated annealing, a bee swarm strategy was applied for the 2018 election (Gutiérrez-Andrade et al., 2019). As we shall see below, the result represents an improvement in the uniformity of polling stations in the country. The main goal with this process is to avoid gerrymandering and other political skews. In this section, we show how the zones appear in the district count files for several elections; next, we analyze the effect of it on two statistics: the distribution of vote and the participation ratio or voters’ turnout, both per cabin.
Previous analysis (Hernández-Saldaña, 2009; Hernández-Saldaña, 2013) considered that the Nominal List in each polling station is conformed for around 750 registered voters. Some small deviations could be expected with an asymmetrical Gaussian or a one-sided Lorentzian distribution, for instance. However, this is not the case and we correct this assumption in this section. We focus on the last three presidential elections, but the same happens with both chambers’ elections, since the Nominal List is the same. For the local elections, the administration is different and requires a special analysis in each state.
In Figure 2A, we show the distributions of the number of registered voters in the Lista Nominal or Nominal List (NL), in each polling station from the registers in presidential elections from 2006 to 2018. The figure is drawn with lines. The black lines represent the presidential election of 2006, the blue lines represent the corresponding list to 2012, and the red lines represent the last case, 2018. The graph corresponds to a point for each value in the Nominal List; hence, we plot how many cabins have 750 registered voters, how many have 749, and so on and so forth. The first thing to notice is the existence of three windows instead of a highly concentrated asymmetric distribution before and in 750 voters. We label them as
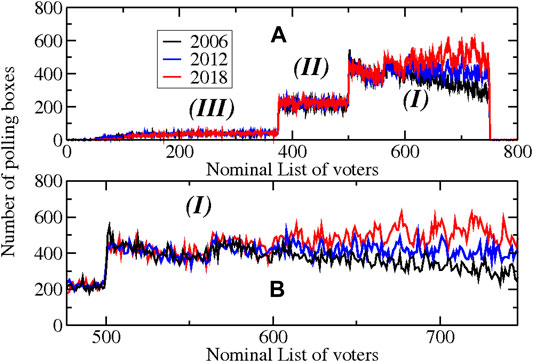
FIGURE 2. (A) Number of polling boxes with a certain number of voters in the Nominal List (NL) for the presidential elections 2006 (in black lines), 2012 (in blue), and 2018 (in red). As can be seen, they present at least three zones with jumps at 375 and 500. We named
The region
We notice two other features in Figure 2B: for the region
It is important to notice that the goal of the redistricting process is to put in a homogeneous situation all the geoeconomical regions, regardless of the urban or nonurban classification and the political party that is preponderant. The exception is the communities with a majority of Native Americans (in the continental sense, not the United States meaning).
3.2 The Distribution of Total Number of Votes
As discussed before, we shall explore the consequences of the NL stratification. Hence, we proceed to analyze the distribution of voters in each polling box, that is, the total number of voters that participate in an election. In Figure 3, we present the histogram of the distributions for the three presidential elections, normalized to the total number of participants, that is, the total sum of votes is different in each case. In black, we present the distribution for all the polling boxes. As can be seen in all the cases, the Gaussianity is not fulfilled. A shoulder appears in the left side of the distributions. However, if we separate the results according to the allowed amount of voters in each box, that is, the Nominal List, an explanation emerges. The left shoulder is composed of the polling stations with few registered voters. That is what we call zone
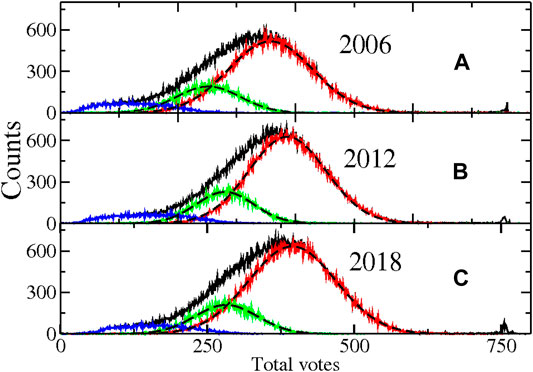
FIGURE 3. Distribution of total votes for the presidential turnout of 2006 (A), 2012 (B), and 2018 (C) normalized to the total number of valid votes in each process. In black line, in all the panels, the distribution corresponds to the whole election. In red, the corresponding distribution is plotted if we consider votes that come from polling stations with a Nominal List between 501 and 750, named
Since we explained the shoulder origin, we focus on the properties of the distribution for the zones
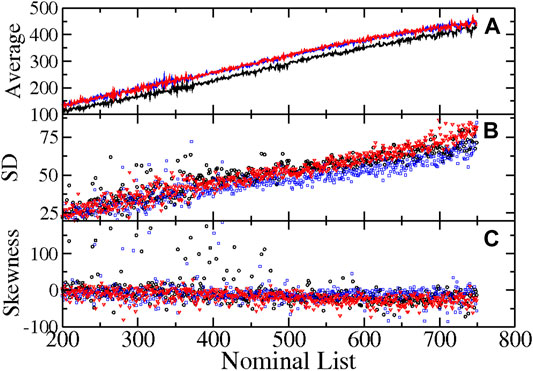
FIGURE 4. In (A), we plot the average number of voters participating in each polling station as a function of the Nominal List for the presidential elections for 2006 (black), 2012 (blue), and 2018 (red). The relation is linear in all the described zones, but the slope is smaller for zone
Additionally, in Figure 3, we show the result for the special polling boxes for the overall case; they appear as the distribution fluctuations for values around 750 votes (the small peaks around that value). In the registers, these polling stations appear with zero allowed voters since they are devoted to persons in transit. Notice that if the number of persons allowed to vote in these cabins is set to 750 or so, they will appear as an extramaximum in the participation ratio around unity. Such suspicious distributions have been assumed as a signature of manipulations. Two examples with a considerable deviation are the Mexican elections in 1988 (Barberán et al., 1988; Auping-Birch, 1988) and the Duma elections in Russia in 2011 (Neretin, 2012), where large peaks appeared. Hence, a clear identification of those special cases in the database is important. For a more recent and available reference for the Mexican scenario, see López-Gallardo (2018), where some of the graphs from Barberán et al. (1988) are reprinted. The votes from registered citizens in foreign countries are considered neither in these analyses nor in the current work.
3.3 The Participation Ratio
In the literature, the participation ratio is an important quantity to be analyzed. It is defined in Eq. 1 as
is not Gaussian. In Eq. 2,
In Figure 5, we plot the distribution of
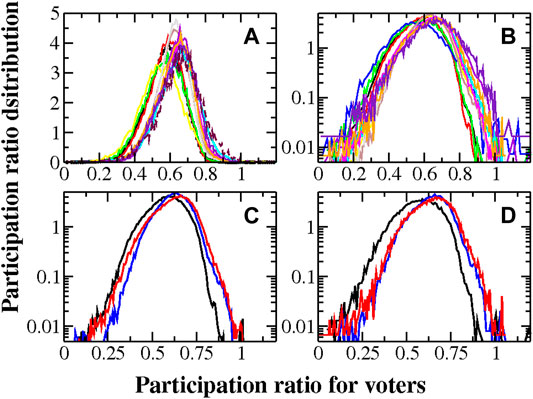
FIGURE 5. Distributions of the participation ratio of voters, τ, for the presidential elections are plotted. In (A), all the cases are presented, considering all the valid voters and regular cabins and the ratio of the zones
In an homogeneous scenario, where (almost) all polling states have a unique number of allowed voters, the Gaussianity in the distribution of voters is reflected in the Gaussianity in the participation ratio, since
However, as explained in Section 3.2, the distribution of allowed voters is not so simple. Even more, from Figure 2, the Nominal List is distributed within windows with fluctuations around an average, from around 400 in zone
In Figure 6, the heat map is plotted for the election of 2018; it represents the total vote as function of the Nominal List. It shows that the number of voters in the tally is not larger than the registered ones. The function seems to be linear but with large fluctuations and scarce of data for small values, there are votes in all polling stations. This behavior is seen in Figure 4, with near to linear standard deviation and negative skewness, as explained in the previous section. In the last section in the heat map, where the NL is near to 750, the fluctuations are larger and the standard deviation is no longer linear. The behavior is similar for all the considered cases, including the controversial election of year 2006.
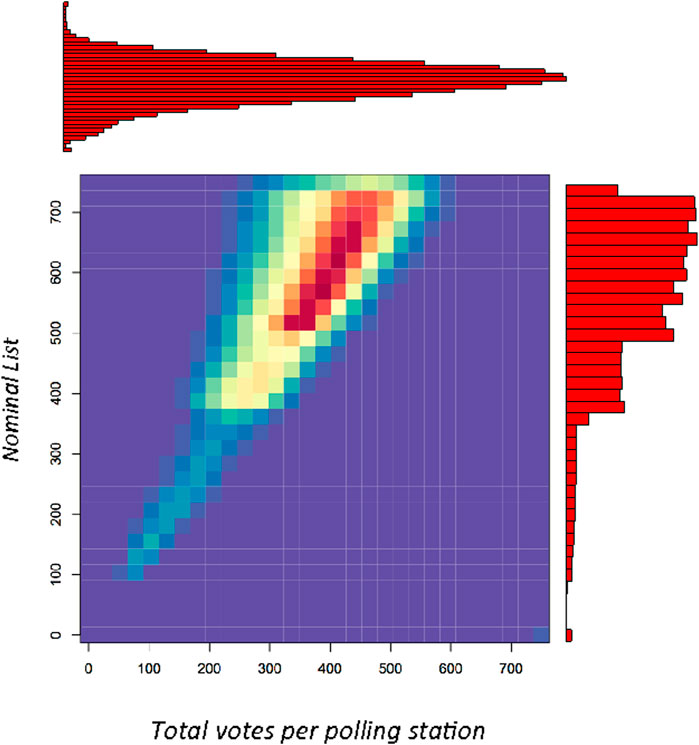
FIGURE 6. Heat map of the total number of votes per polling station and Nominal List for the 2018 presidential election: the color code starts in blue for zero values and ends in red for increasing values. In the upper part, distribution of total votes which appears in Figure 3C as black line is presented. At the right, the Nominal List histogram is given, a detailed view appears in Figure 2.
The analytical form of the joint distribution probability distribution is hard to achieve, mainly, since the distribution in the denominator of t is not a step function. Hence, in order to obtain some insight, we proceed to consider an ensemble of distributions for each value in the Nominal List
The skewness for the vast majority of the cases is negative and concentrated around a value. Hence, a graph of all the
where
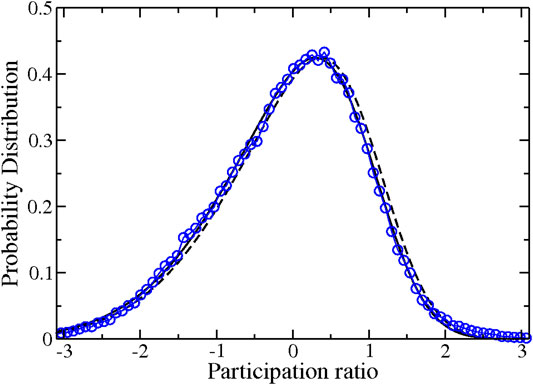
FIGURE 7. Distribution of the normalized participation ratio,
Another aspect to consider is the form of the individual distributions for each NL value. Even if they are skew normal functions, the sum of them is neither Gaussian nor skew normal. In a closed form is the sum of Gaussian and a finite sum of functions that involve the Kampé de Fériet function (Nadarajah and Li, 1985).
4 Discussion
The return of physicists to analyze the complexity of social and economical behavior came with the use of simple models. However, simple models not always mimic the nature of the systems. Two common places describe such a point of view: 1) the Markovian elector and 2) the large number law for elections. It is a fact that electors have memory; if the people forgot something, the parties are there to remember it. The violation of the second is sometimes the reason to call for a violation of the electoral system. However, as the present work pretend to show, correlated variables give place to apparent violations of the law. For instance, in a recent study (Peters, 2019), the author focused on a problem of assuming ergodicity in economics; meanwhile, economics is a time-dependent system by excellence. Hence, the author claimed that a reconsideration of the use of ergodicity in economics could explain some puzzles. Thus, complex systems present complex behavior, no matter if we understand the cause or mechanism. The existence of stylized facts in electoral systems is the evidence that no particular phenomena occur in the system and their interrelationships. Hence, a careful analysis of the database and the conditions is required.
Statistical analysis assumes that in a random process, the proportion of positive events to the total number of events is a random variable with Gaussian distributions if we consider a large number of events. For a not-so-large number, it could be deviations or approximations, for instance, a binomial distribution that approaches a Gaussian for large number of experiments. However, Mexican electors, as the vast majority of the electors, are not balls in the Bernoulli experiment. As shown in Figure 2, the sampling could be tricky, even when the electors have a Gaussian distribution.
As shown in Figure 3, disentangling the NL by zones explains the shoulder in the distribution of voters. Only the zone
The distributions of the several cases analyzed here show that the distortion is not simply a cutoff of the sample size. In Figure 7, we fitted a skewed Gaussian to the standardized participation ratio, η, where the mode is larger than the mean. Hence, instead of having a Gaussian distribution with a truncated tail, we have a distorted Gaussian due to the existence of a constraint. A way to explain the skewed distribution for τ and η is considering a truncation process. In Azzalini and Valle (1996), a mechanism of hidden truncation is used to obtain a skew normal function (see Arnold and Beaver (2002) and references therein). They consider a process where the accepted values must be above a certain limit. The argument is presented as a proposition in there, and it is as follows: consider a couple of random variables
where the second line comes from the fact that Y is standardized. Without lack of generality, the relation of Y and Z can be written as
for Z and W i.i.d. variables. Since
In the current case, we consider the requirement that
if the variables are related as in Eq. 6. Under the conditions considered by Azzalini and Valle (1996), η has a skew normal distribution. Since the only free parameter is
5 Conclusion
In this work, we deal with the distribution of the official list of allowed participants in a given election, named Nominal List (NL) or Lista Nominal in Spanish. The number of voters is previously designed (see Gutiérrez-Andrade et al. (2019)) with considerations to avoid gerrymandering and other influences from the political parties. Here we found that the number of voters is distributed according to zones resembling step functions (see Figure 2). The three zones were explicitly marked, with zone
However, it is clear that there exist correlations between the distribution of voters and the NL. The average of the number of voters participated in each station grows linearly with NL, as shown in Figure 4. This conditioned the behavior of the participation ratio distribution, considered as the turnout distribution at a polling station degree of aggregation. For this quantity and the standardized variable, we obtain a near to skew normal function, with deviations at the wings, as shown in Figure 7. The analysis was performed for the most populated zones.
Even the disentangled zones are able to explain the asymmetry in the distribution of the standardized participation ratio. Such a distribution could be compatible with a skewed Gaussian function, as showed in Figure 7. The complicated constraint of the Nominal List (NL), shown in Eq. (2), gives a similar distribution for all the cases analyzed.
Beyond the actual distribution followed by the participation ratio of voters, the skewed Gaussian appears consistently in all the cases, regardless of the geopolitical or economical situation, neither its urban nor nonurban condition. So, the standardized participation ratio of voters could have a generic distribution, like the skewed Gaussian. A way to introduce the relation of the Nominal List (NL) and the number of voters, v, is through the constraint
A final remark is that no priori assumptions must be taken when handling truly complex systems, such as the electoral processes.
Data Availability Statement
The datasets generated for this study are available on request to the corresponding author.
Author Contributions
HH-S conceived the paper, cleaned the data, coded the programs, and wrote the paper.
Funding
PRODEP-SEP support through Programa de Apoyo para el Fortalecimiento de los Cuerpos Académcos. The author is a member of the Cuerpo Académico Física Teórica y Materia Condensada. Divisional project CBI, CB008-13, Universidad Autónoma Metropolitana at Azcapotzalco, gave support.
Conflict of Interest
The author declares that the research was conducted in the absence of any commercial, financial or political relationships that could be construed as a potential conflict of interest.
Acknowledgments
The author thank H. Várguez and E. Várguez for their kindness during the time this work was in progress.
References
Arnold,, B. C., Beaver, R. J., Bhaumik, A., Dey, D. K., Cuadras, C. M., Sarabia, J. M., et al. (2002). Multivariate models related to hidden truncation and/or selective reporting. Test 11, 7–54. doi:10.1007/bf02595728
ATLAS, I. N. E. (2016). Sistema de consulta de la estadística de las elecciones federales 2014-2015/atlas de resultados de las elecciones federales 1991-2015. Mexico: Instituto Federal Electoral.
Auping-Birch,, J. (1988). Elecciones federales de México: julio de 1988: interpretación de los resultados oficiales mediante el análisis matemático. Mexico: IEES.
Azzalini,, A. (1985). A class of distributions which includes the normal ones. Scand J. Statist. 12. 171–178. doi:10.6092/issn.1973-2201/711
Azzalini,, A., and Valle, A. D. (1996). The multivariate skew-normal distribution. Biometrika 83, 715–26. doi:10.1093/biomet/83.4.715
Baltz,, S., McAlister, K., Pineda, A., Vasselai, F., and Mebane, W. (2018). “Agent-based models of strategic electoral behavior in election forensics,” in Annual Meeting of the Midwest political science association, Chicago, IL, November 22.
Barberán,, J., Cárdenas, C., Mojardín, A. L., and Zavala, J. (1988). Radiografía del fraude: análisis de los resultados oficiales del 6 de julio. Mexico: nuestro Tiempo.
Borghesi,, C., and Bouchaud, J. P. (2010). Spatial correlations in vote statistics: a diffusive field model for decision-making. Eur. Phys. J. B 75, 395–404. doi:10.1063/PT.3.384510.1140/epjb/e2010-00151-1
Borghesi,, C., Raynal, J. C., and Bouchaud, J. P. (2012). Election turnout statistics in many countries: similarities, differences, and a diffusive field model for decision-making. PLoS One. 7, e36289. doi:10.1371/journal.pone.0036289
Cantú,, F. (2019). The fingerprints of fraud: evidence from Mexico's 1988 presidential election. Am Polit Sci Rev. 113, 710–26. doi:10.1017/s0003055419000285
Centro de Estudios de la Democracia y Elecciones (1991). Cede laboratorio de análisis político y políticas públicas, base de datos. Mexico City: UAM Iztapalapa.
Dictamen del Colegio Electoral (1994). “Declaratoria de Presidente Electo de los Estados Unidos Mexicanos al Licenciado Carlos Salinas de Gortari para el periodo 1988-1994 emitida por la Cámara de Diputados de LIV Legislatura, erigida en Colegio Electoral,” in Elecciones a Debate 1988: las actas electorales perdidas. EditorsA. S. Gutiérrez (Mexico: Diana).
Gutiérrez-Andrade,, M. Á., Rincón-García, E. A., de-los-Cobos-Silva, S. G., Lara-Velázquez, P., Mora-Gutiérrez, R. A., and Ponsich, A. (2019). Simulated annealing and artificial bee colony for the redistricting process in Mexico. Inform. J. Appl. Analytics. 49, 189–200. doi:10.1287/inte.2019.0992
Hernández-Saldaña,, H. (2009). On the corporate votes and their relation with daisy models. Physica A Stat. Mech. Appl. 388, 2699–704. doi:10.1016/j.physa.2009.03.016
Hernández-Saldaña,, H. (2013). Result on three predictions on july 2012 federal elections in Mexico based on past regularities. Plos ONE. 8, e82584. doi:10.1371/journal.pone.0082584
Klimek,, P., Yegorov, Y., Hanel, R., and Thurner, S. (2012). Statistical detection of systematic election irregularities. Proc. Natl. Acad. Sci. U.S.A. 109, 6469–16473. doi:10.1073/pnas.1210722109
LEGIPE, (2012). Compendio de Legislación nacional electoral. Tomo II. Mexico: Instituto Nacional Electoral.
Mebane,, W., and Jackson, J. E. (2014). “Preference heterogeneities in models of electoral behavior,” in Annual Meeting of the Midwest political science association, Chicago, United States, April 3–April 6, 2014.
Nadarajah,, S., and Li, R. (1985). The exact density of the sum of independent skew normal random variables. Scand. J. Statist. 12:171–178. doi:10.1016/j.cam.2016.06.032
Neretin,, Y. (2012). On statistical researches of parliament elections in Russian federation. NY: Cornell University arxiv.
O’Hagan,, A., and Leonard, T. (1976). Bayes estimation subject to uncertainty about parameter constraints. Biometrika 63, 201–203. doi:10.1093/biomet/63.1.201
Ortega,, R. Y. (2017). Presidential elections in Mexico: from Hegemony to Pluralism. Cham (Switzerland): Springer International Publishing.
Peters,, O. (2019). The ergodicity problem in economics. Nat. Phys. 15, 1216–1221. doi:10.1038/s41567-019-0732-0
Keywords: electoral systems, opinion formation, zone design, stylized facts, sociophysics, skewed Gaussian model, electoral forensics, electoral abstentionism
Citation: Hernández-Saldaña H (2021) Geopolitical Inhomogeneities in the Registered Voters’ Distribution and Their Influence in the Voters’ Participation Ratio Distribution: The Mexican Case. Front. Appl. Math. Stat. 7:518371. doi: 10.3389/fams.2021.518371
Received: 07 December 2019; Accepted: 15 February 2021;
Published: 28 April 2021.
Edited by:
Raluca Eftimie, University of Franche-Comté, FranceReviewed by:
Miguel Pineda, University College London, United KingdomFrancisco Cantu, University of Houston, United States
Copyright © 2021 Hernández-Saldaña. This is an open-access article distributed under the terms of the Creative Commons Attribution License (CC BY). The use, distribution or reproduction in other forums is permitted, provided the original author(s) and the copyright owner(s) are credited and that the original publication in this journal is cited, in accordance with accepted academic practice. No use, distribution or reproduction is permitted which does not comply with these terms.
*Correspondence: H. Hernández-Saldaña, aGhzQGF6Yy51YW0ubXg=