Entrainment Dynamics Organised by Global Manifolds in a Circadian Pacemaker Model
- 1Department of Mathematics, University of Exeter, Exeter, United Kingdom
- 2Department of Mathematical Sciences, New Jersey Institute of Technology, Newark, NJ, United States
- 3Living Systems Institute, University of Exeter, Exeter, United Kingdom
- 4EPSRC Hub for Quantitative Modelling in Healthcare, University of Exeter, Exeter, United Kingdom
Circadian rhythms are established by the entrainment of our intrinsic body clock to periodic forcing signals provided by the external environment, primarily variation in light intensity across the day/night cycle. Loss of entrainment can cause a multitude of physiological difficulties associated with misalignment of circadian rhythms, including insomnia, excessive daytime sleepiness, gastrointestinal disturbances, and general malaise. This can occur after travel to different time zones, known as jet lag; when changing shift work patterns; or if the period of an individual’s body clock is too far from the 24 h period of environmental cycles. We consider the loss of entrainment and the dynamics of re-entrainment in a two-dimensional variant of the Forger-Jewett-Kronauer model of the human circadian pacemaker forced by a 24 h light/dark cycle. We explore the loss of entrainment by continuing bifurcations of one-to-one entrained orbits under variation of forcing parameters and the intrinsic clock period. We show that the severity of the loss of entrainment is dependent on the type of bifurcation inducing the change of stability of the entrained orbit, which is in turn dependent on the environmental light intensity. We further show that for certain perturbations, the model predicts counter-intuitive rapid re-entrainment if the light intensity is sufficiently high. We explain this phenomenon via computation of invariant manifolds of fixed points of a 24 h stroboscopic map and show how the manifolds organise re-entrainment times following transitions between day and night shift work.
1 Introduction
The function of the circadian timekeeping system is to align an organism’s physiology and behaviour with the daily environmental cycles conferred by Earth’s rotation. Alignment is achieved by endogenous circadian oscillators with periods close to, but not exactly, 24 h synchronising to external 24 h periodic signals, such as the light/dark cycle, in a process called entrainment. Properties of both the internal oscillator and the external forcing signal, such as intrinsic period and light intensity, combine to determine the stable phase of entrainment. For example, circadian clocks with long intrinsic periods can lead to delayed sleep phase syndrome (DSPS), a disorder where patients tend to be unable to fall asleep until late at night and have difficulty waking up in the morning. Bright light therapy can help to advance the entrained phase of DSPS patients so that their hormonal rhythms, sleep-wake patterns, and peak performance times are more in line with societal norms [1].
Failure to achieve entrainment can occur if the mismatch between the periods of the intrinsic oscillator and the external forcing is sufficiently large and the forcing signal is sufficiently weak. In non-24 h sleep-wake disorder (non-24), patients are unable to establish a stable 24 h sleep-wake rhythm [2–5]. Non-24 is common in blind people that do not have any light perception, leading to sleep-wake rhythms with the period of their intrinsic circadian clock. Since the intrinsic period of most people is greater than 24 h, this results in sleep-wake patterns where the phase of sleep onset progressively drifts later in the day from one day to the next. Non-24 is also experienced by sighted individuals where the combination of a short or long intrinsic period and a reduced sensitivity to light renders them unable to entrain to 24 h cycles.
Transient misalignment of circadian rhythms occurs when there is an abrupt shift in the phase of environmental cycles. After rapid travel across time zones, it can take several days for the circadian clock to establish a stable phase relationship with the light/dark cycle in the new time zone. During the re-entrainment process, travelers may experience insomnia, daytime sleepiness, gastrointestinal issues, and other symptoms collectively known as jet lag. Circadian misalignment and associated health problems can also be caused by a change in work patterns, for example, when a worker transitions from day shift to night shift or vice versa. In particular, rotating or permanent night-shift workers have increased incidence of cardiovascular disease and cancer compared to permanent day shift workers [6]. Furthermore, night shift workers that are not entrained to a permanent night shift work schedule exhibit decreased alertness and performance levels relative to entrained night shift workers [7].
Mathematical modeling and tools from dynamical systems theory can be used to gain insight into circadian rhythm sleep disorders and the re-entrainment process following shifts in the light/dark cycle [8, 9]. The circadian clock is a complex system consisting of cellular oscillators and network interactions within and across brain regions and tissues throughout the body. Mathematical models have been developed to describe the circadian system at various levels, including detailed models of the transcription/translation feedback loops underlying intracellular molecular clocks and models of the electrical activity of the suprachiasmatic nucleus—a network of ∼20,000 neurons in the hypothalamus that serves as the brain’s master circadian timekeeper. We refer the reader to [10, 11] for reviews of biochemical and electrophysiological circadian models. Other modelers have focused less on the cellular details and instead have tried to capture the behavior of the circadian system at the level of the whole organism (see [12] for a review). One such effort is the Forger–Jewett–Kronauer (FJK) model, a low-dimensional model of the human circadian system consisting of a central limit cycle oscillator that responds to light via processing by the retina [13]. This model is based on experiments measuring how the amplitude and phase of circadian rhythms in human subjects respond to light pulses. It has been extensively validated in laboratory and field conditions, making it an attractive choice for simulating jet lag and other perturbations to the circadian system [14]. Here, we use dynamical systems tools to analyse the entrainment dynamics of a reduction to the original FJK model.
The remainder of the manuscript is organised as follows. In Section 2, we consider the FJK model equations and then introduce the two-dimensional version of the model that we use in the present study. In Section 3, we characterise the bifurcations that lead to loss of entrainment when key parameters are varied and discuss these results in the context of non-24. In Section 4, we compute invariant manifolds of fixed points for a 24 h stroboscopic map constructed from the model. We show that these manifolds are able to explain the dynamics of a rapid re-entrainment phenomenon that has been previously observed under certain conditions in simulations and experiments. In Section 5, we illustrate how the manifolds organise the dynamics of re-entrainment following transitions between day and night shift work schedules. Finally, in Section 6, we discuss how our results relate to previous studies on the dynamics of circadian entrainment.
2 The Forger–Jewett–Kronauer Model
In 1990, Kronauer introduced a mathematical model of the human circadian pacemaker that reproduces many general features of how the circadian clock responds to light exposure in laboratory experiments [15]. Kronauer’s original model has subsequently been revised and extended to account for additional data on the effects of light observed in phase resetting experiments [16–18]. These models consist of a limit cycle oscillator, based on the Van der Pol equations, combined with a model of retinal light processing. Although these models are simplified descriptions of the human circadian system, they have become a widely-used tool for predicting circadian phase and have been carefully validated under a range of conditions [19–21]. Here we utilise the Forger–Jewett–Kronauer (FJK) version of the model [13]. This three-dimensional system of ordinary differential equations with external periodic forcing takes the following form:
where the dots indicate derivatives with respect to time. The variable C captures daily fluctuations in core body temperature, A is a phenomenological auxiliary variable, and
where N represents the number of hours of light in a 24 h interval.
In the absence of light (i.e., with
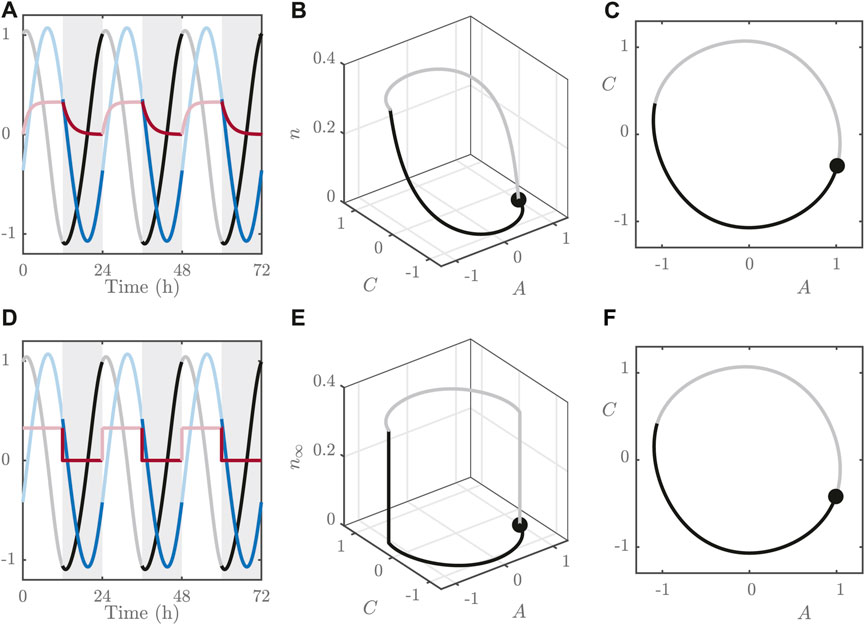
FIGURE 1. (A–C): Simulations of the FJK model Eqs 1–5 for
The dynamics for n are significantly faster than those for A and C, as can be seen in the time series in Figure 1. This means that n rapidly adopts its steady state value:
This observation allows us to approximate the full, three variable system with a 2-dimensional version by replacing Eq. 4 with
where
The effect of changing shift work patterns, or of the jet lag induced by travelling across time zones (assuming variation in longitude only), can be modelled by changing
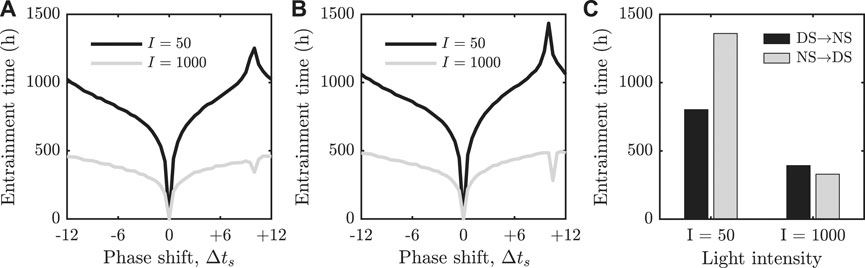
FIGURE 2. (A) + (B): Re-entrainment times as a function of the shift in the phase of the external forcing. The FJK model is shown in panel (A) and our two dimensional reduction is shown in panel (B). For
Similarly to results reported in [23–26], we observe that there is a pronounced peak in the re-entrainment for
3 Bifurcation Analysis
The behaviour of dynamical systems in general is organised by invariant sets in phase space and their associated manifolds. For periodically forced systems, these invariant sets typically take the form of periodic orbits, where we note that there may be many such objects in the full phase space. With this in mind, we aim to find periodic orbits of the two variable FJK model (hereby referred to as the 2D model) for the default parameter values; see Table 1. In particular, we seek to identify bifurcations in the number of periodic orbits since these transitions are relevant to understanding the dynamics of the entrainment process. Hence, we proceed to probe the bifurcation structure of the 2D model, using the methodology detailed in the Supplementary Material S1.
3.1 Variation of Light Intensity
We first perform bifurcation analysis in the light intensity parameter I and display the results in Figure 3. The bifurcation diagram in Figure 3A shows the branches of solutions under variation of I and we identify two fold bifurcations (around
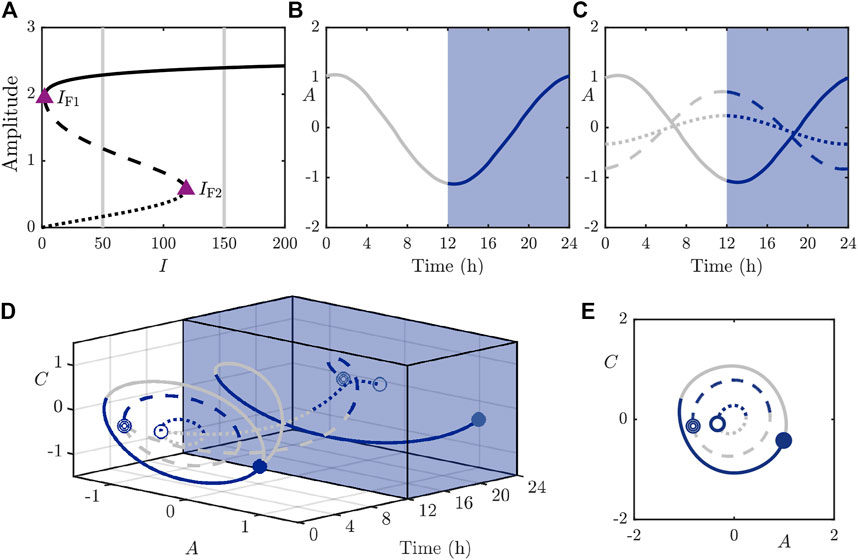
FIGURE 3. The stable (solid), saddle (dashed), and fully unstable (dotted) periodic orbits in the 2D model. (A): Bifurcation diagram of the periodic orbits under variation of I with other parameters as in Table 1. Purple triangles mark folds of periodic orbits at approximately
3.2 Variation of Day Length
In addition to light intensity, the day length, expressed by the parameter N, also influences the number and type of periodic orbits supported by the system, as illustrated in Figures 4A–C. For
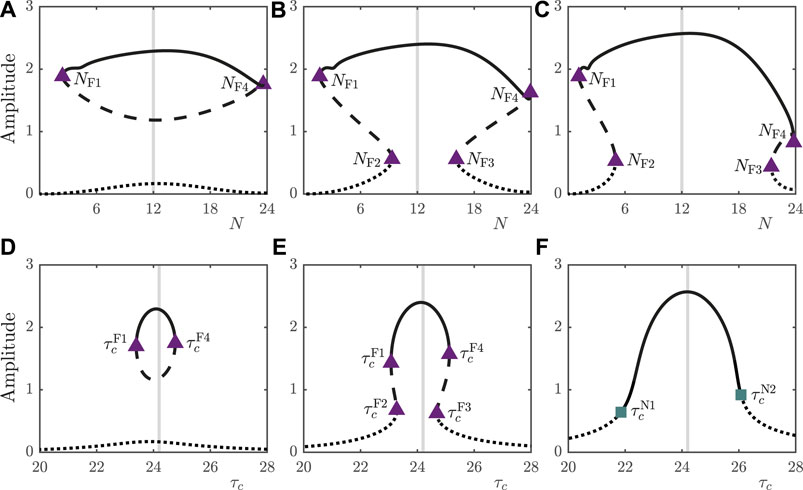
FIGURE 4. (A–C): Bifurcation diagrams of the 2D model under variation in N for
3.3 Variation of Natural Period
As well as considering properties of the external environment such as light intensity and day length, it is pertinent to consider the influence of intrinsic properties of the circadian oscillator on entrainment. The natural period,
3.4 Behaviour Near the Bifurcations
In Figures 4D–F, we observe that the type of bifurcation leading to loss of stability of the entrained orbit under variation of
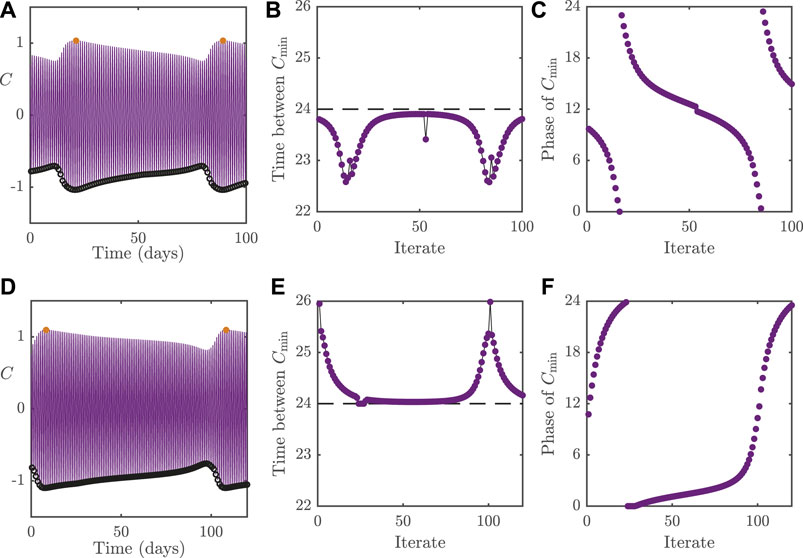
FIGURE 5. Dynamics of the 2D model with
To demonstrate the effect that the loss of entrainment has on the circadian rhythm, we plot in Figures 5B,E, the cycle-to-cycle variation of the period, as measured by the interval between successive nadirs in C; denoted
To compare the differences between dynamic behaviour associated with the different bifurcation types, we plot in Figure 6 the system behaviour following Neimark–Sacker instabilities. The panels in the figure follow the same layout as in Figure 5. Whilst we would conclude mathematically that the circadian oscillator is no longer entrained following such a bifurcation as in the case for dynamics near a fold, from a physiological perspective, the oscillator may still be regarded as entrained if the oscillatory dynamics around the now unstable periodic solution are small in amplitude (relative to the original limit cycle). In a similar vein to Figure 5, the top row in Figure 6 displays dynamics for
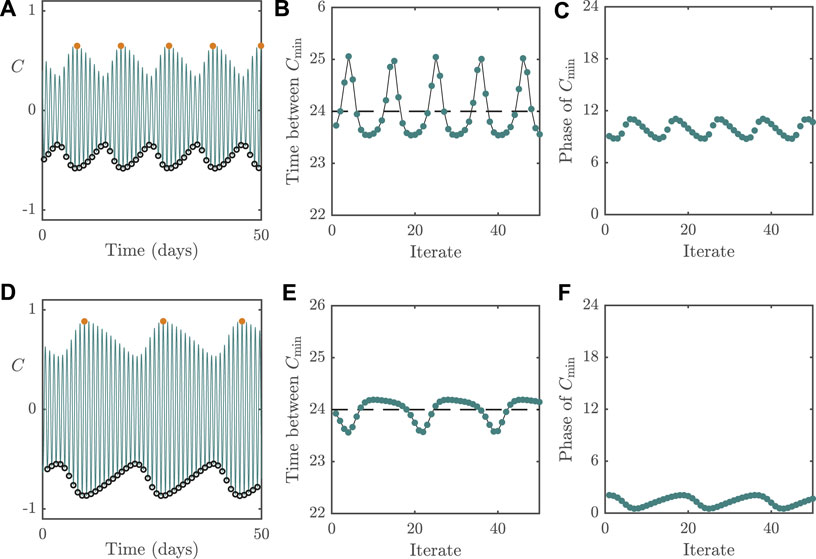
FIGURE 6. Dynamics of the 2D model with
For parameter values near the Neimark–Sacker instabilities, we see that the cycle-to-cycle period of C now varies around 24 h, as can be seen in Figures 6B,E. The consequence of this is that the phase of C relative to the external forcing, although varying from cycle-to-cycle, is constrained to an interval around the correct phase (i.e., the phase of the underlying limit cycle), as shown in Figures 6C,F. This means that even though people with such dynamics will not be entrained to a 24 h period, their phase variation relative to the external forcing will be of small amplitude, meaning that they may not experience significant circadian rhythm disorders. Overall, this highlights the importance of high light intensities (or, equivalently, in boosting sensitivity to light) for combating non-24 h sleep/wake disorders.
3.5 Summary of Bifurcation Analysis
The observations of the one-parameter continuations may be summarised via the three-parameter bifurcation diagram as shown in Figure 7A, with two-dimensional slices of the full diagram shown in Figures 7B–D. Figure 7A shows the surfaces of fold bifurcations (purple) together with the surfaces of Neimark–Sacker bifurcations (teal) that bound parameter regimes (indicated in Figures 7B–D) with different entrainment behavior. Figure 7B and Figure 7C showcase the so-called Arnol’d tongues that are commonly observed in forced oscillator systems. In the central, light orange shaded, region in Figure 7B, bounded by the Neimark–Sacker (teal) and fold (purple) bifurcation lines, only the stable periodic orbit exists and so the system entrains easily. In the lower, darker orange shaded part of the central region, the stable, unstable and saddle periodic orbits coexist. In this region, the system ultimately converges to the stable periodic orbit, since it is the only attracting set, but the pathway involved in this convergence may be heavily shaped by the other orbits, as will be investigated in Section 4. In the white region to the left and right of the shaded regions, no stable 24 h period orbit exists and so we conclude that the circadian oscillator does not entrain to a 24 h rhythm. Similarly, the light orange shaded region in Figure 7C supports only a single, stable periodic orbit, whilst the region bounded between the two fold curves possesses all three types of periodic orbit. To the left and right of the outer fold curve, only the unstable orbit exists, and hence there is no possibility to entrain to a 24 h rhythm. Figure 7D shows the so-called Arnol’d onion [25, 28], where we now identify a central portion in which only the stable periodic orbit exists. This is surrounded by a region supporting all three orbit types, which itself is surrounded by the white region with only the unstable orbit.
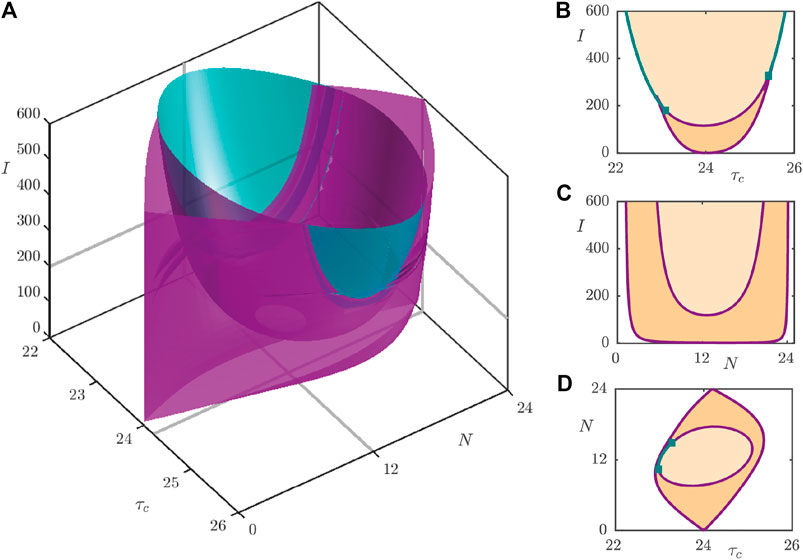
FIGURE 7. (A): Three-parameter bifurcation diagram of the 2D model under variation of
4 Entrainment Times
The bifurcation diagrams in Section 3 indicate where in parameter space the circadian oscillator can be entrained to a 24 h cycle under our choice of forcing. Even in these regions of parameter space, however, the dynamics involved with the entrainment can be markedly different depending on initial conditions. To demonstrate this point, we compute the time taken for a trajectory starting from an arbitrary initial condition to converge to the stable limit cycle; we refer to such times as entrainment times. Formally, we define the entrainment time,
where ε is a tolerance which we hereon set to
where
In Figures 2A,B, we recapitulated results from [25] by plotting the entrainment times for initial conditions along the stable limit cycle at different relative phases (note that the definition of entrainment times between the two studies are different). This section is dedicated to understanding this phenomenon. To this end, we consider a stroboscopic map
The above may equivalently be thought of as a Poincaré return map, with a section chosen at the zero phase of the forcing cycle. In Figure 8, we show sequences of map iterates of Eq. 11 for
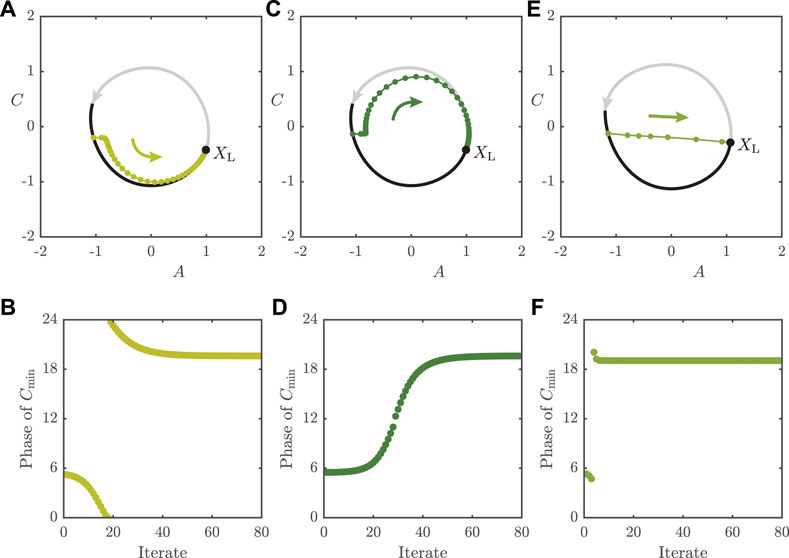
FIGURE 8. Long re-entrainment times for
In Figure 8E, we plot iterates for the map with
4.1 Manifolds as Organisers of Entrainment Times
We compute manifolds following the steps outlined in Supplementary Material S2. Figure 9 shows the projection of the periodic orbits in the
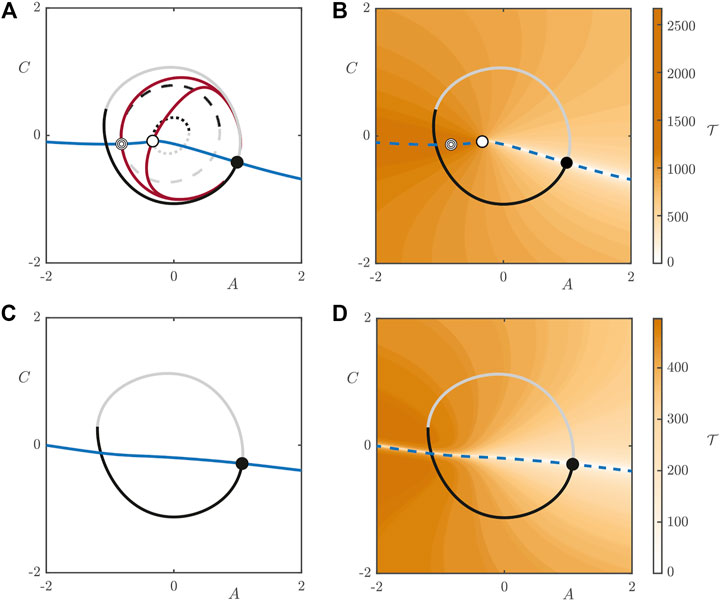
FIGURE 9. Intersections of the manifolds of the periodic orbits of the 2D model with the plane of zero phase for
If we increase the light intensity to
This plot highlights the existence of a “river” of points in the
Thus far, we have set
noting that
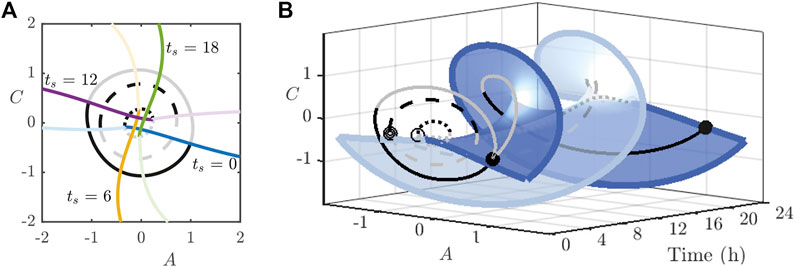
FIGURE 10. (A): Intersection of the strong stable manifolds (dark coloured) of the stable periodic orbit and stable manifolds (light coloured) of the saddle periodic orbit for the indicated values of
5 Application to Shift Work
In developed economies, millions of people either permanently work the night shift or rotate in and out of night shift work. When a worker rotates from day shift to night shift, or vice versa, the abrupt change in the pattern of their exposure to light can cause circadian misalignment and jet lag-like symptoms until they entrain to the light/dark cycle of the new shift. Here we study the dynamics of re-entrainment following shift work rotations and use the manifolds of the stable and saddle limit cycles discussed in Section 4.1 to explain the simulation results shown in Figure 2. In particular, we focus on the following two questions. Firstly, at low light intensity (
We assume that a worker on the day shift works from 7 AM to 3 PM and sleeps from 11 PM to 6 AM, whereas someone on the night shift works from 11 PM to 7 AM and sleeps from 8 AM to 3 PM. In either case, they are exposed to
5.1 Rotating From Day Shift to Night Shift at Low Light Intensity
To simulate a worker rotating from day shift to night shift, we assume that they remain entrained to the DS light/dark cycle until 11 PM, which is the
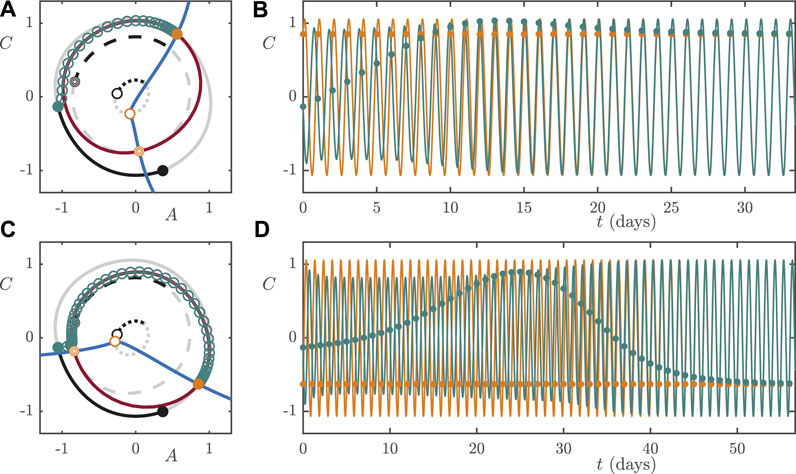
FIGURE 11. Low lux (
5.2 Rotating From Night Shift to Day Shift at Low Light Intensity
Next we consider a worker rotating from night shift to day shift. We assume that they remain entrained to the NS light/dark cycle until 8 AM, which is at the
5.3 Rotating Between Shifts at High Light Intensity
We assume the same shift work schedules and rotation protocols as in Section 5.1 and 5.2. With
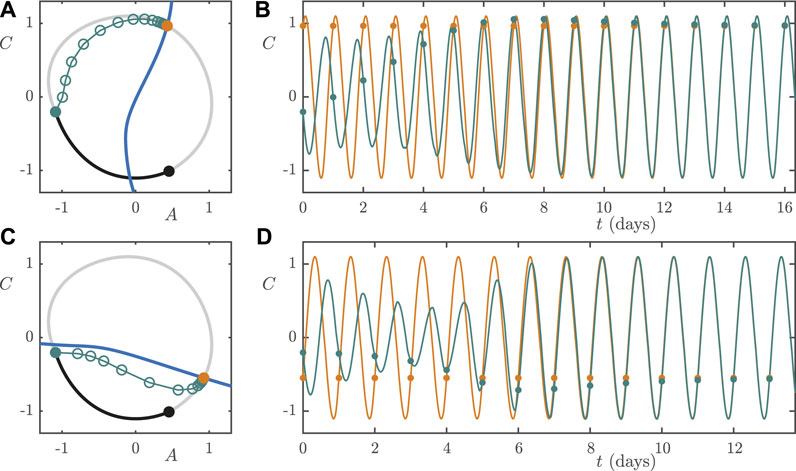
FIGURE 12. High lux (
6 Discussion
The first key result of our paper is the identification of the boundaries of the parameter regimes in which the circadian oscillator can entrain to external forcing. To this end, we perform one-parameter continuation of the periodic orbits in each of light intensity I, day length N and natural period
Interestingly, Schmal et al. [28] identify higher order “strings” of onions for lower
The second key result of our paper is the computation of the geometric structures in phase space that organise previously observed re-entrainment dynamics; namely, the invariant manifolds of fixed points for a 24 h stroboscopic map constructed from the model. Computation of these manifolds allows us to find and fully characterise a separatrix between phase advancing and phase delaying re-entrainment trajectories. This phase space separatrix was conjectured to exist following studies of the one-dimensional iterative map reduction of the FJK model [25]. The separatrix is also responsible for the rapid re-entrainment phenomenon that has been observed under certain conditions in simulations and experiments [24, 25, 30–32]. If we interpret Figure 2B as representing entrainment times following international travel, we see that for low light intensity, the longest entrainment times occur for journeys approximately 10 h East (
Invariant manifolds of saddle orbits as organizing centres of entrainment times have recently been identified in a forced Kuramoto model of pacemaker cell dynamics [24] and a hierarchical system of planar Novak–Tyson models [26]. Moreover, the shortcut traced out by the strong stable manifold has recently been experimentally identified from wearable device data and exploited to develop jet lag strategies that align with it [32]. Here, jet lag is measured by self-reported mood scores that show that people who appear to hit the shortcut feel “better” according to a simple positive-negative scale. The authors propose an algorithm based on simulations of the FJK model [13, 17] to create a schedule for optimum re-entrainment that consists of varying periods of light and dark each day. Their strategies rely on knowing the location in phase space of the “optimal solution”, i.e., the strong stable manifold of the entrainment orbit. Their first approach is to identify the optimal solution for phase space corresponding to the situation immediately after arrival. They propose a light/dark schedule to move initial conditions to this optimal solution, but find that the trajectories never reach the optimum and, for some initial conditions, re-entrainment is not achieved. With respect to our formulation, changing the light/dark schedule corresponds to varying the parameter N, which leads to a shift in the position of relevant manifold(s). The authors’ second approach is to update the schedule to fit the optimum for the system at the next step (N value). They show this is a superior strategy in that it leads to faster re-entrainment for all initial conditions. In both cases, the approach to finding the “optimal solution” is a brute force simulation method over the phase space. Here, we directly compute the optimal solution for high light intensity values as the strong stable manifold of the stable fixed point of the stroboscopic map defined by Eq. 11.
Our bifurcation analysis, as presented in Figure 7, shows the boundaries of the entrainment region in the three-parameter space consist of both fold and Neimark–Sacker bifurcations. In particular, Figure 7B suggest that loss of entrainment due to a Neimark–Sacker bifurcation in the
Examining the relationships between parameters for light sensitivity G, light intensity scaling factor
Our approach to the set-up of the continuation problem is to establish a multi-segment two-point boundary value problem in Auto [34, 35] to find and follow the periodic orbits of the two-dimensional FJK system. This approach was facilitated by our choice, for simplicity, of a square wave function Eq. 6 for the light/dark forcing giving instantaneous transitions at dawn/dusk. This multi-segment approach is similar in spirit to that taken in [36, 37]. This approach can be readily modified to include, for example, more realistic light protocols, such as variations in the quality and intensity of light similar to those studies in the green unicellular alga Ostreococcus tauri [38, 39]. Here we choose a two-state model that switches from complete darkness to full light, this could readily be extended in future studies to incorporate multiple light levels such as indoor and outdoor light regimes as well as darkness, as used in [40]. Including additional light levels as different “steps” would correspond to having additional segments in our multi-segment BVP with similar matching conditions at the transition times. This multi-segment approach has limitations and an alternative approach would be to convert the 2D autonomous system into a 3D non-autonomous one for implementation in Auto. This alternative approach would allow us to relax the condition on the light/dark forcing and use, for example, a sinusoidal signal reflecting a more gradual change, closer to the real light/dark cycle. Moreover, our choice of multi-segment encoding of the model means that the time shift parameter
The framework we propose here can in principle be extended to the analysis of arbitrary perturbations by computing the invariant manifolds and periodic orbits that constitute organising structures of the system. In Figures 11, 12 we compare two such example perturbations in the context of shift work, the change from night to day shift and vice versa. This approach could be used to identify non-pharmacological strategies for shift workers thereby minimising the risk factors for a range of chronic diseases including cancer, metabolic syndrome and stroke [41]. Another interesting problem to consider is to use our approach to design “compromise” schedules for permanent night shift workers that avoid circadian misalignment during the work week yet also enable them to be awake during daylight hours on weekends thereby improving quality of life [42, 43].
Our study centres around a two dimensional reduction of the FJK model. The reduction of the original system to a planar one facilitates the construction of the full invariant manifolds of the system, as depicted in Figure 10B. Such construction was achieved by first computing one dimensional manifolds of an appropriately chosen stroboscopic map and then by taking integrating these manifolds around the entire forcing cycle. Advances in numerical continuation algorithms, such as those included in the recent Matlab-based CoCo package [44], provide functionality to compute two-dimensional manifolds directly, so that our approach could then be used to compute manifolds for the original three-variable FJK model (though we remark that visualising manifolds of systems with more than two dimensions is difficult). Given the significant similarity between the original FJK model and our 2D variant, we expect that similar conclusions about the role of invariant manifolds in organising re-entrainment dynamics would be observed in the full FJK model.
In addition to the FJK model, there exist a number of other models that describe different aspects of circadian rhythms, such as variability in the expression of clock proteins [45–47], or describe the dynamics of the suprachiasmatic nucleus in a more physiological manner [48]. It remains an open question as to how our results might relate to such models. In this regard, it is worth noting that dynamics in general systems can be at least partially understood via analysis of their invariant sets and associated manifolds. As such, whilst the specific observations we make may not directly carry over to these different models, the general approach is likely to still be valid. Regarding our specific choice of system, we remark the original FJK model was constructed using empirical data from human participants [13, 17]. We believe that this fact coupled with the focus on simple descriptions of core macroscopic variables makes it the ideal model for our study. One important macroscopic variable not included in the FJK model is that of sleep duration. Even in the absence of variation in circadian phase, the dynamics of the sleep/wake cycle are intricate [49]. Given the tight bidirectional coupling between circadian rhythms and sleep/wake cycles, incorporation of sleep as a macroscopic variable promises to be an interesting direction for future investigation [50, 51].
It is well known that seasonality of day length affects the circadian rhythms of humans, animals, and plants [28, 52–54]. More recently, switching between different seasonal day lengths (such as travelling from winter to summer) has been studied by considering the phase response curves of a heuristic phase-amplitude model [55]. Our modelling framework presented here could readily be adapted to identify the organising structures, in particular, the invariant manifolds that organise re-entrainment after sudden seasonal switches. A further model extension, that to our knowledge has not been modelled before, is to incorporate forcing at multiple time scales the encompass both day length and seasonality. Additionally, on even longer timescales, there is evidence from evolutionary biology that human core body temperature is decreasing [56] and it is not clear what effect this will have on circadian rhythms.
7 Conclusion
In this paper, we study the loss of entrainment in a two-dimensional reduction of the forced FJK model for human circadian rhythms. We use bifurcation theory to identify parameter regimes in which the circadian oscillator is unable to entrain to a 24 h cycle and identify the instabilities leading to this phenomenon under variation of key system parameters. For parameter regimes where entrainment is attainable, we show how re-entrainment times are organised by the invariant manifolds of the periodic orbits of the system. Finally, we study re-entrainment following phase shifts of the light/dark cycle and discuss these results in the context of shift work transitions.
Code Availability
AUTO, Python, CUDA, and Matlab code needed to reproduce all results in this manuscript may be downloaded via Git from https://github.com/kyle-wedgwood/EntrainmentTimesManifolds. For help with installing software or running code, please contact k.c.a.wedgwood@exeter.ac.uk.
Data Availability Statement
Publicly available datasets were analyzed in this study. This data can be found here: https://github.com/kyle-wedgwood/EntrainmentTimesManifolds.
Author Contributions
JC, CD, and KW contributed to conception and design of the study. JC performed the continuation in AUTO. CD and KW performed simulations of entrainment times. JC, CD, and KW wrote sections of the manuscript. All authors contributed to manuscript revision, read, and approved the submitted version.
Funding Statement
JC gratefully acknowledges funding from MRC Skills Development Fellowship MR/S019499/1. CD gratefully acknowledges the financial support of the US-UK Fulbright Commission, the EPSRC via grant EP/N014391/1, and the National Science Foundation via grant DMS 1555237. KW gratefully acknowledges the financial support of the EPSRC via grant EP/T017856/1 and from MRC via the Skills Development Fellowship MR/P01478X/1.
Conflict of Interest
The authors declare that the research was conducted in the absence of any commercial or financial relationships that could be construed as a potential conflict of interest.
Publisher’s Note
All claims expressed in this article are solely those of the authors and do not necessarily represent those of their affiliated organizations, or those of the publisher, the editors and the reviewers. Any product that may be evaluated in this article, or claim that may be made by its manufacturer, is not guaranteed or endorsed by the publisher.
Acknowledgments
We would like to thank Peter Ashwin and Amitabha Bose for insightful comments and discussions regarding this project.
Supplementary Material
The Supplementary Material for this article can be found online at: https://www.frontiersin.org/articles/10.3389/fams.2021.703359/full#supplementary-material
Supplementary Video 1 | Movie of the entraining trajectories following rotation from day shift to night shift at (DS→NS) low light intensity I = 50; accompanies Figure 11 A,B. Left panel shows the projections of the periodic orbits, and their points of zero phase, for the day shift in black and grey. The points of zero phase corresponding to the periodic orbits for the night shift (which has a different phase of entrainment than the day shift) are shown in orange. The unstable and (strong) stable manifolds of the periodic orbits at the phases indicated by the orange markers are shown in red and blue, respectively. The worker transitions from day shift to night shift when f(t) = 1 → f(t) = 0, with their instantaneous f(t) = 1 → f(t) = 0, values at this time indicated by the first solid teal dot (to the left of the panel). Subsequent teal markers indicate the f(t) = 1 → f(t) = 0, values of the trajectory starting from this point evaluated at successive 24 h intervals. The trajectory ultimately converges to the entrained phase indicated by the solid orange marker. The right panel shows the C coordinate of the time course of the entraining trajectory (teal) as it converges to the stable entrained periodic orbit of the night shift (orange). Solid markers on each trajectory indicate the C value of the trajectory evaluated at successive 24 h intervals (corresponding to the teal markers in the projection on the left).
Supplementary Video 2 | Movie of the entraining trajectories following rotation from night shift to day shift (NS→DS) at low light intensity I=50; accompanies Figure 11 C,D. Left panel shows the projections of the periodic orbits, and their points of zero phase, for the night shift in black and grey. The points of zero phase corresponding to the periodic orbits for the day shift (which has a different phase of entrainment than the night shift) are shown in orange. The unstable and (strong) stable manifolds of the periodic orbits at the phases indicated by the orange markers are shown in red and blue, respectively. The worker transitions from night shift to day shift when f(t) = 1 → f(t) = 0, with their instantaneous (A,C) values at this time indicated by the first solid teal dot (to the left of the panel). Subsequent teal markers indicate the (A,C) values of the trajectory starting from this point evaluated at successive 24 h intervals. The trajectory ultimately converges to the entrained phase indicated by the solid orange marker. The right panel shows the C coordinate of the time course of the entraining trajectory (teal) as it converges to the stable entrained periodic orbit of the day shift (orange). Solid markers on each trajectory indicate the C value of the trajectory evaluated at successive 24 h intervals (corresponding to the teal markers in the projection on the left).
Supplementary Video 3 | Movie of the entraining trajectories following rotation from day shift to night shift (DS→NS) at high light intensity I=1000; accompanies Figure 12 A,B. Left panel shows the projections of the periodic orbits, and their points of zero phase, for the day shift in black and grey. The points of zero phase corresponding to the periodic orbits for the night shift (which has a different phase of entrainment than the day shift) are shown in orange. The stable manifold of the periodic orbit at the phase indicated by the orange marker is shown in blue. The worker transitions from day shift to night shift when f(t) = 1 → f(t) = 0, with their instantaneous (A,C) values at this time indicated by the first solid teal dot (to the left of the panel). Subsequent teal markers indicate the (A,C) values of the trajectory starting from this point evaluated at successive 24 h intervals. The trajectory ultimately converges to the entrained phase indicated by the solid orange marker. The right panel shows the C coordinate of the time course of the entraining trajectory (teal) as it converges to the stable entrained periodic orbit of the night shift (orange). Solid markers on each trajectory indicate the C value of the trajectory evaluated at successive 24 h intervals (corresponding to the teal markers in the projection on the left).
Supplementary Video 4 | Movie of the entraining trajectories following rotation from night shift to day shift (NS→DS) at high light intensity I=1000; accompanies Figure 12 C,D. Left panel shows the projections of the periodic orbits, and their points of zero phase, for the night shift in black and grey. The points of zero phase corresponding to the periodic orbits for the day shift (which has a different phase of entrainment than the night shift) are shown in orange. The stable manifold of the periodic orbit at the phase indicated by the orange marker is shown in blue, respectively. The worker transitions from night shift to day shift when f(t) = 1 → f(t) = 0, with their instantaneous (A,C) values at this time indicated by the first solid teal dot (to the left of the panel). Subsequent teal markers indicate the (A,C) values of the trajectory starting from this point evaluated at successive 24 h intervals. The trajectory ultimately converges to the entrained phase indicated by the solid orange marker. The right panel shows the C coordinate of the time course of the entraining trajectory (teal) as it converges to the stable entrained periodic orbit of the day shift (orange). Solid markers on each trajectory indicate the C value of the trajectory evaluated at successive 24 h intervals (corresponding to the teal markers in the projection on the left).
References
1. Figueiro, M. Delayed Sleep Phase Disorder: Clinical Perspective with a Focus on Light Therapy. Nat Sci Sleep (2016) 8:91–106. doi:10.2147/nss.s85849
2. Emens, JS, and Eastman, CI. Diagnosis and Treatment of Non-24-h Sleep-Wake Disorder in the Blind. Drugs (2017) 77(6):637–50. doi:10.1007/s40265-017-0707-3
3. McArthur, AJ, Lewy, AJ, and Sack, RL. Non-24-hour Sleep-Wake Syndrome in a Sighted Man: Circadian Rhythm Studies and Efficacy of Melatonin Treatment. Sleep (1996) 19(7):544–53. doi:10.1093/sleep/19.7.544
4. Watanabe, T, Kajimura, N, Kato, M, Sekimoto, M, Hori, T, and Takahashi, K. Case of a Non‐24 H Sleep-Wake Syndrome Patient Improved by Phototherapy. Psychiatry Clin Neurosciences (2000) 54(3):369–70. doi:10.1046/j.1440-1819.2000.00719.x
5. Burgess, HJ, and Emens, JS. Circadian-Based Therapies for Circadian Rhythm Sleep-Wake Disorders. Curr Sleep Med Rep (2016) 2(3):158–65. doi:10.1007/s40675-016-0052-1
6. Smith, MR, and Eastman, CI. Shift Work: Health, Performance and Safety Problems, Traditional Countermeasures, and Innovative Management Strategies to Reduce Circadian Misalignment. Nat Sci Sleep (2012) 4:111–32. doi:10.2147/NSS.S10372
7. Smith, MR, Fogg, LF, and Eastman, CI. A Compromise Circadian Phase Position for Permanent Night Work Improves Mood, Fatigue, and Performance. Sleep (2009) 32(11):1481–9. doi:10.1093/sleep/32.11.1481
8. Gonze, D. Modeling Circadian Clocks: From Equations to Oscillations. Cent Eur J Biol (2011) 6(5):699–711. doi:10.2478/s11535-011-0061-5
9. Goldbeter, A, and Leloup, J-C. From Circadian Clock Mechanism to Sleep Disorders and Jet Lag: Insights from a Computational Approach. Biochem Pharmacol (2021)(December 2020) 114482. doi:10.1016/j.bcp.2021.114482
10. Henson, MA. Multicellular Models of Intercellular Synchronization in Circadian Neural Networks. Chaos, Solitons & Fractals (2013) 50:48–64. doi:10.1016/j.chaos.2012.11.008
11. Belle, MDC, and Diekman, CO. Neuronal Oscillations on an Ultra‐slow Timescale: Daily Rhythms in Electrical Activity and Gene Expression in the Mammalian Master Circadian Clockwork. Eur J Neurosci (2018) 48:2696–717. doi:10.1111/ejn.13856
12. Asgari-Targhi, A, and Klerman, EB. Mathematical Modeling of Circadian Rhythms. Wiley Interdiscip Rev Syst Biol Med (2019) 11(2):e1439–34. doi:10.1002/wsbm.1439
13. Forger, DB, Jewett, ME, and Kronauer, RE. A Simpler Model of the Human Circadian Pacemaker. J Biol Rhythms (1999) 14(6):533–8. doi:10.1177/074873099129000867
14. Stone, JE, Postnova, S, Sletten, TL, Rajaratnam, SMW, and Phillips, AJK. Computational Approaches for Individual Circadian Phase Prediction in Field Settings. Curr Opin Syst Biol (2020) 22(August):39–51. doi:10.1016/j.coisb.2020.07.011
15. Kronauer, RE. A Quantitative Model for the Effects of Light on the Amplitude and Phase of the Deep Circadian Pacemaker, Based on Human Data. In: J Horne, editor. Sleep ’90, Proceedings of the Tenth European Congress on Sleep Research. Bochum: Pontenagel Press (1990). p. 306–9.
16. Jewett, ME, and Kronauer, RE. Refinement of Limit Cycle Oscillator Model of the Effects of Light on the Human Circadian Pacemaker. J Theor Biol (1998) 192(4):455–65. doi:10.1006/jtbi.1998.0667
17. Jewett, ME, Forger, DB, and Kronauer, RE. Revised Limit Cycle Oscillator Model of Human Circadian Pacemaker. J Biol Rhythms (1999) 14(6):493–500. doi:10.1177/074873049901400608
18. Kronauer, RE, Forger, DB, and Jewett, ME. Quantifying Human Circadian Pacemaker Response to Brief, Extended, and Repeated Light Stimuli over the Phototopic Range. J Biol Rhythms (1999) 14(6):500–15. doi:10.1177/074873099129001073
19. Indic, P, Forger, DB, Hilaire, MAS, Dean, DA, Brown, EN, Kronauer, RE, et al. Comparison of Amplitude Recovery Dynamics of Two Limit Cycle Oscillator Models of the Human Circadian Pacemaker. Chronobiology Int (2005) 22(4):613–29. doi:10.1080/07420520500180371 Available from: http://www.pubmedcentral.nih.gov/articlerender.fcgi?artid=3797655{\&}tool=pmcentrez{\&}rendertype=abstract.
20. St. Hilaire, MA, Klerman, EB, Khalsa, SBS, Wright, KP, Czeisler, CA, and Kronauer, RE. Addition of a Non-photic Component to a Light-Based Mathematical Model of the Human Circadian Pacemaker. J Theor Biol (2007) 247(4):583–99. doi:10.1016/j.jtbi.2007.04.001
21. Klerman, EB, and Hilaire, MS. Review: On Mathematical Modeling of Circadian Rhythms, Performance, and Alertness. J Biol Rhythms (2007) 22(2):91–102. doi:10.1177/0748730407299200
22. Stone, JE, McGlashan, EM, Quin, N, Skinner, K, Stephenson, JJ, Cain, SW, et al. The Role of Light Sensitivity and Intrinsic Circadian Period in Predicting Individual Circadian Timing. J Biol Rhythms (2020) 35(6):628–40. doi:10.1177/0748730420962598
23. Leloup, J-C, and Goldbeter, A. Critical Phase Shifts Slow Down Circadian Clock Recovery: Implications for Jet Lag. J Theor Biol (2013) 333:47–57. doi:10.1016/j.jtbi.2013.04.039
24. Lu, Z, Klein-Cardeña, K, Lee, S, Antonsen, TM, Girvan, M, and Ott, E. Resynchronization of Circadian Oscillators and the East-West Asymmetry of Jet-Lag. Chaos (2016) 26(9):094811. doi:10.1063/1.4954275
25. Diekman, CO, and Bose, A. Reentrainment of the Circadian Pacemaker during Jet Lag: East-West Asymmetry and the Effects of north-south Travel. J Theor Biol (2018) 437:261–85. doi:10.1016/j.jtbi.2017.10.002
26. Liao, G, Diekman, C, and Bose, A. Entrainment Dynamics of Forced Hierarchical Circadian Systems Revealed by 2-dimensional Maps. SIAM J Appl Dyn Syst (2020) 19(3):2135–61. doi:10.1137/19m1307676
27. Bordyugov, G, Abraham, U, Granada, A, Rose, P, Imkeller, K, Kramer, A, et al. Tuning the Phase of Circadian Entrainment. J R Soc Interf (2015) 12(108):20150282. doi:10.1098/rsif.2015.0282
28. Schmal, C, Myung, J, Herzel, H, and Bordyugov, G. A Theoretical Study on Seasonality. Front Neurol (2015) 6(MAY):94–11. doi:10.3389/fneur.2015.00094
29. Glass, L, Guevara, MR, Shrier, A, and Perez, R. Bifurcation and Chaos in a Periodically Stimulated Cardiac Oscillator. Physica D: Nonlinear Phenomena (1983) 7:89–101. doi:10.1016/0167-2789(83)90119-7
31. Serkh, K, and Forger, DB. Optimal Schedules of Light Exposure for Rapidly Correcting Circadian Misalignment. Plos Comput Biol (2014) 10(4):e1003523. doi:10.1371/journal.pcbi.1003523
32. Christensen, S, Huang, Y, Walch, OJ, and Forger, DB. Optimal Adjustment of the Human Circadian Clock in the Real World. Plos Comput Biol (2020) 16(12):e1008445. doi:10.1371/journal.pcbi.1008445
33. Taylor, SR. Delays Are Self-Enhancing: An Explanation of the East-West Asymmetry in Recovery from Jetlag. J Biol Rhythms (2021) 36:127–36. doi:10.1177/0748730421990482
34. Doedel, EJ. AUTO: A Program for the Automatic Bifurcation Analysis of Autonomous Systems. Congr Numer (1981) 30(265-284):25–93.
35. Doedel, EJ, Champneys, AR, Dercole, F, Fairgrieve, TF, Kuznetsov, YA, Oldeman, B, et al. AUTO-07P: Continuation and Bifurcation Software for Ordinary Differential Equations (2007). Available from: http://cmvl.cs.concordia.␣ca/auto/.
36. Creaser, Jennifer L., Krauskopf, B, and Osinga, HM. Finding First Foliation Tangencies in the Lorenz System. SIAM J Appl Dyn Syst (2017) 16(4):2127–64. doi:10.1137/17m1112716
37. Langfield, P, Krauskopf, B, and Osinga, HM. Solving Winfree's Puzzle: The Isochrons in the FitzHugh-Nagumo Model. Chaos (2014) 24(1):013131. doi:10.1063/1.4867877
38. Thommen, Q, Pfeuty, B, Morant, PE, Corellou, F, Bouget, FY, and Lefranc, M. Robustness of Circadian Clocks to Daylight Fluctuations: Hints from the Picoeucaryote Ostreococcus Tauri. Plos Comput Biol (2010) 6(11):e1000990. doi:10.1371/journal.pcbi.1000990
39. Thommen, Q, Pfeuty, B, Schatt, P, Bijoux, A, Bouget, FY, and Lefranc, M. Probing Entrainment of Ostreococcus Tauri Circadian Clock by Blue and green Light through a Mathematical Modeling Approach. Front Genet (2015) 5(FEB):1–13. doi:10.3389/fgene.2015.00065
40. Skeldon, AC, Phillips, AJ, and Dijk, DJ. The Effects of Self-Selected Light-Dark Cycles and Social Constraints on Human Sleep and Circadian Timing: a Modeling Approach. Sci Rep (2017) 7(February):45158. doi:10.1038/srep45158 Available from: http://www.nature.com/articles/srep45158.
41. Crowther, ME, Ferguson, SA, Vincent, GE, and Reynolds, AC. Non-Pharmacological Interventions to Improve Chronic Disease Risk Factors and Sleep in Shift Workers: A Systematic Review and Meta-Analysis. Clocks & Sleep (2021) 3(1):132–78. doi:10.3390/clockssleep3010009
42. Lee, C, Smith, MR, and Eastman, CI. A Compromise Phase Position for Permanent Night Shift Workers: Circadian Phase after Two Night Shifts with Scheduled Sleep and Light/dark Exposure. Chronobiology Int (2006) 23(4):859–75. doi:10.1080/07420520600827160
43. Brum, MCB, Dantas Filho, FF, Schnorr, CC, Bertoletti, OA, Bottega, GB, and da Costa Rodrigues, T. Night Shift Work, Short Sleep and Obesity. Diabetol Metab Syndr (2020) 12(1):13. doi:10.1186/s13098-020-0524-9
45. Ruoff, P, Vinsjevik, M, Monnerjahn, C, and Rensing, L. The Goodwin Oscillator: On the Importance of Degradation Reactions in the Circadian Clock. J Biol Rhythms (1999) 14(6):469–79. doi:10.1177/074873099129001037
46. Vasalou, C, Herzog, ED, and Henson, MA. Small-World Network Models of Intercellular Coupling Predict Enhanced Synchronization in the Suprachiasmatic Nucleus. J Biol Rhythms (2009) 24(1):243–54. doi:10.1177/0748730409333220 Available from: https://www.ncbi.nlm.nih.gov/pmc/articles/PMC3624763/pdf/nihms412728.pdf.
47. François, P, Despierre, N, and Siggia, ED. Adaptive Temperature Compensation in Circadian Oscillations. Plos Comput Biol (2012) 8(7):e1002585–12. doi:10.1371/journal.pcbi.1002585
48. Diekman, CO, and Forger, DB. Clustering Predicted by an Electrophysiological Model of the Suprachiasmatic Nucleus. J Biol Rhythms (2009) 24(4):322–33. doi:10.1177/0748730409337601
49. Skeldon, AC, Dijk, DJ, and Derks, G. Mathematical Models for Sleep-Wake Dynamics: Comparison of the Two-Process Model and a Mutual Inhibition Neuronal Model. PLoS ONE (2014) 9(8):e103877. doi:10.1371/journal.pone.0103877
50. Watson, LA, McGlashan, EM, Hosken, IT, Anderson, C, Phillips, AJK, and Cain, SW. Sleep and Circadian Instability in Delayed Sleep-Wake Phase Disorder. J Clin Sleep Med (2020) 16(9):1431–6. doi:10.5664/jcsm.8516
51. Murray, JM, Magee, M, Sletten, TL, Gordon, C, Lovato, N, Ambani, K, et al. Light-based Methods for Predicting Circadian Phase in Delayed Sleep-Wake Phase Disorder. Sci Rep (2021) 11(1):1–12. doi:10.1038/s41598-021-89924-8
52. Pittendrigh, CS, and Daan, S. A Functional Analysis of Circadian Pacemakers in Nocturnal Rodents. J Comp Physiol (1976) 106(3):291–331. doi:10.1007/bf01417859
53. Hazlerigg, DG, and Wagner, GC. Seasonal Photoperiodism in Vertebrates: from Coincidence to Amplitude. Trends Endocrinol Metab (2006) 17(3):83–91. doi:10.1016/j.tem.2006.02.004
54. De Caluwé, J, de Melo, JRF, Tosenberger, A, Hermans, C, Verbruggen, N, Leloup, J-C, et al. Modeling the Photoperiodic Entrainment of the Plant Circadian Clock. J Theor Biol (2017) 420(January):220–31. doi:10.1016/j.jtbi.2017.03.005
55. Tokuda, IT, Schmal, C, Ananthasubramaniam, B, and Herzel, H. Conceptual Models of Entrainment, Jet Lag, and Seasonality. Front Physiol (2020) 11:334. doi:10.3389/fphys.2020.00334
56. Protsiv, M, Ley, C, Lankester, J, Hastie, T, and Parsonnet, J. Decreasing Human Body Temperature in the United States since the Industrial Revolution. eLife (2020) 9:e49555. doi:10.7554/eLife.49555
57. Krauskopf, B, and Osinga, HM. Computing Invariant Manifolds via the Continuation of Orbit Segments. In: Numerical Continuation Methods for Dynamical Systems. Springer (2007). p. 117–54. doi:10.1007/978-1-4020-6356-5_4
Keywords: entrainment, circadian, rhythms, bifurcation analysis, continuation, manifolds
Citation: Creaser JL, Diekman CO and Wedgwood KCA (2021) Entrainment Dynamics Organised by Global Manifolds in a Circadian Pacemaker Model. Front. Appl. Math. Stat. 7:703359. doi: 10.3389/fams.2021.703359
Received: 30 April 2021; Accepted: 06 July 2021;
Published: 29 July 2021.
Edited by:
Víctor F. Breña-Medina, Instituto Tecnológico Autónomo de México, MexicoReviewed by:
Quentin Thommen, Université de Lille, FranceAlexandre P. Rodrigues, University of Porto, Portugal
Copyright © 2021 Creaser, Diekman and Wedgwood. This is an open-access article distributed under the terms of the Creative Commons Attribution License (CC BY). The use, distribution or reproduction in other forums is permitted, provided the original author(s) and the copyright owner(s) are credited and that the original publication in this journal is cited, in accordance with accepted academic practice. No use, distribution or reproduction is permitted which does not comply with these terms.
*Correspondence: Kyle C. A. Wedgwood, K.C.A.Wedgwood@exeter.ac.uk