- 1Institute for Epidemiology and Pathogen Diagnostics, Julius Kühn Institute (JKI) – Federal Research Centre for Cultivated Plants, Braunschweig, Germany
- 2Estación Experimental INIA Las Brujas, Instituto Nacional de Investigación Agropecuaria (INIA), Rincón del Colorado, Uruguay
Introduction: Understanding how pasture-crop rotation design influences long-term soil health and microbial dynamics is crucial for sustainable agroecosystems. Pasture-crop rotations may alleviate soil degradation, but their long-term effects or legacy on soil and rhizosphere microbiomes, including potential recruitment of plant-beneficial microorganisms, and their link to crop yield need to be better understood. This study examines how land use intensity and grazed pasture legacy influence soil and rhizosphere prokaryotic diversity, composition, functionality, and crop productivity.
Methods: A gradient of land use intensities ranging from continuous cropping (CCG), short (SR) and long (LR) pasture-crop rotations, permanent improved pasture (PIP), and natural grassland (NGL) were sampled in a long-term field experiment established in 1995 in the Uruguayan Pampa. Moreover, two stages of the rotation, one year after pasture sown with sorghum (SRS, LRS) and two years after pasture with soybean (SRG, LRG), were studied to assess the persistence of pasture-derived legacies. Soil physicochemical and biological properties were measured to evaluate soil health along with crop productivity. Bulk soil, soybean, and sorghum rhizosphere prokaryotic communities were analyzed across land use intensities using 16S rRNA gene amplicon sequencing and functional assays on rhizosphere bacterial isolates.
Results: Results showed a partial retention of soil health assessed through selected soil properties, i.e. soil organic C, total N, and soil extractable protein content were higher in LRG compared to CCG, while an intermediate response was observed in SRG. LR preserved the pasture legacy, maintaining bulk soil prokaryotic community composition similar to PIP and distinct from CCG, while SR converged to CCG and diverged from PIP. Soybean rhizosphere prokaryotic diversity and composition was strongly shaped by crop type and by soybean inoculation with Bradyrhizobium elkanii, overriding the effects of intensification and pasture legacy. Key soil taxa (Streptomyces, Solibacillus, Sphingomonas and Bradyrhizobium) were linked with improved soil functionality. Linking 16S rRNA gene sequencing data of rhizosphere taxa with rhizobacterial isolates showed that Pseudomonas, Bacillus, and Microbacterium, all exhibiting multiple plant-beneficial activities in vitro, were enriched in pasture rotations.
Discussion: This study highlights that pasture-crop rotation design, particularly pasture duration and plant composition, influences prokaryotic services and soil health, contributing towards the development of resilient agroecosystems.
1 Introduction
Soil provides essential ecosystem services such as nutrient cycling, carbon (C) sequestration, water regulation, and biodiversity conservation (Karlen et al., 2019; Lehmann et al., 2020). Soil health, defined as the capacity of soil to function as a living ecosystem, integrates biological, chemical and physical properties that are crucial for sustainable agriculture and food security (Lehmann et al., 2020; Banerjee and van der Heijden, 2023). Traditional physicochemical assessments are insufficient to capture soil health, making microbial parameters, such as community structure, function, abundance, and diversity, important bioindicators due to the role of microorganisms in nutrient cycling, organic matter decomposition, and disease suppression (Lehman et al., 2015; Verdenelli et al., 2019; Hermans et al., 2020; Lehmann et al., 2020; Norris et al., 2020; Fierer et al., 2021; Banerjee and van der Heijden, 2023). Intensive agricultural practices, characterized by short-term economic benefits and simplified management supported by an intensive use of synthetic inputs (fuel, fertilizers, pesticides, herbicides), are widespread. However, these practices pose negative impacts on soil health by increasing soil compaction, decreasing soil organic matter, accumulating agrochemicals, and consequently disrupting soil microbial communities (Sadras et al., 2005; Carbonetto et al., 2014; Pittelkow et al., 2015; Tsiafouli et al., 2015; Norris and Congreves, 2018; Lehmann et al., 2020; Cerecetto et al., 2021a). In contrast, conservation practices like reduced tillage, cover cropping, organic amendments and crop rotations have been shown to enhance soil microbial activity and diversity, and soil fertility and structure (Chaparro et al., 2012; Bender et al., 2016; Williams et al., 2023; Hartmann and Six, 2023). However, despite their proven benefits, conservation practices can result in variable yields and unbalanced plant nutrition under certain conditions, particularly with no-tillage (Pittelkow et al., 2015). Therefore, further research is needed to understand how rhizosphere biological processes influence plant performance under these conservation practices. A key concept emerging in this context is soil legacy, or soil memory, which refers to the long-term effects of past agricultural practices on soil properties and microbial communities (Lapsansky et al., 2016; Bakker et al., 2018). These legacy effects may modulate rhizosphere processes and, consequently, plant responses to current management. Understanding soil legacy is critical for designing sustainable management strategies.
The Pampa biome, spanning Uruguay, central-eastern Argentina, and southern Brazil, is one of the world’s largest and most diverse grassland ecosystems (Scottá and da Fonseca, 2015). Historically managed through extensive cattle grazing on native grasslands, this biome has undergone rapid agricultural intensification, primarily through no-tillage and glyphosate-resistant soybean (Glycine max L.) expansion, leading to soil degradation, biodiversity loss, and reductions in ecosystem services (de Faccio Carvalho et al., 2021a; Macedo et al., 2022). To reverse these trends, the integration of crop and livestock systems through pasture-crop rotations has been proposed as a sustainable alternative to continuous cropping, improving soil structure, C sequestration, nutrient cycling, and microbial diversity (McDaniel et al., 2014; Garrett et al., 2017; Pravia et al., 2019; Macedo et al., 2022; Pereyra-Goday et al., 2022, 2024).
The positive effects of pasture-crop rotations on soil physicochemical properties have been well documented across various agroecosystems (Franzluebbers et al., 2014; Garrett et al., 2017; Ernst et al., 2018; King and Blesh, 2018; Jaurena et al., 2021; Sekaran et al., 2021; Macedo et al., 2022; Rubio et al., 2022; Santos Silva et al., 2022). Moreover, impacts of pasture-crop rotations on soil microbial community composition and diversity have been demonstrated in different regions (Walkup et al., 2020; Sekaran et al., 2021; Williams et al., 2023; dos Santos-Goulart et al., 2024). However, microbial responses are highly context-dependent, varying with soil type, climate, land use, and management practices (French et al., 2021). Therefore, results from other regions are not necessarily transferable to the Pampa biome, which features distinct environmental and management conditions. For instance, dos Santos-Goulart et al. (2024) demonstrated that soil bacteria did not respond to pasture-crop rotation when compared to a native forest in the Brazilian Cerrado biome, whereas the fungal community exhibited shifts in response to the rotation scheme. In contrast, Cerecetto et al. (2024) found that soil prokaryotic communities shifted when pasture-crop rotations were compared to a native grassland in the Uruguayan Pampa biome, and such shifts were observed only in a few of the studied pasture-crop rotations for fungal communities. These differences underscore the need for site-specific research to develop precision microbiome management tailored to local conditions with customized agricultural practices aimed at enhancing crop health and agroecosystem sustainability (Berg et al., 2017; Lehmann et al., 2020; French et al., 2021). Although a few studies have explored how pasture-crop rotations affect soil prokaryotic communities in the Pampa biome (Frene et al., 2022; Barbero et al., 2025), they lack the grazing effects, a central component of mixed crop-livestock systems, or overlook the legacy of long-term pastures. Moreover, there is limited knowledge on how pasture duration influences the microbiome. Furthermore, few studies have investigated how grazed pastures impact rhizosphere microbial communities (Cerecetto et al., 2024; Martins Costa et al., 2024) and have linked these changes to plant-beneficial traits and their potential functional implications on crop productivity.
To address these knowledge gaps, this study expands upon earlier findings from one of the longest-running long-term field experiments (LTEs) in the Pampa region, established in 1995 in Uruguay (Cerecetto et al., 2024). Previous work in this LTE demonstrated that incorporating grazed pastures into crop rotations positively influenced subsequent grain crop cycles through physicochemical, bulk soil and rhizosphere microbial changes, preserving soil structure and nutrient availability while supporting crop performance (Cerecetto et al., 2024). However, it remained unclear whether this pasture legacy persisted over time, varied with pasture duration, or promoted plant-beneficial prokaryote recruitment in crop rhizospheres. To investigate this, the same LTE was re-sampled in a subsequent growing season to evaluate soil health indicators across five land use intensities: continuous cropping (CC), short (SR) and long (LR) pasture-crop rotations, permanent improved pasture (PIP), and natural grassland (NGL). Additionally, two rotation stages, one (SRS, LRS) and two years (SRG, LRG) after pasture, were assessed to determine the persistence of pasture-derived legacies. Moreover, SR and LR differ in pasture duration and in pasture plant species composition, which may further influence the persistence of pasture-derived legacies. Soil health was determined using physicochemical properties (bulk density, soil nutrients), biological proxies (heterotrophic soil respiration, soil potentially oxidizable C, soil extractable protein content), and microbial indicators (diversity and composition of bulk soil and rhizosphere prokaryotic communities via 16S rRNA gene amplicon sequencing). Potential rhizosphere functions were inferred by linking sequencing data to 16S rRNA gene sequences of rhizobacterial isolates with plant-beneficial traits. Crop performance was evaluated through biomass and nutrient content, serving as an indirect proxy for soil health and enabling the exploration of connections between soil properties, prokaryotic communities, and plant growth. It was hypothesized that introducing grazed pastures into crop rotations in the Uruguayan Pampa biome preserves soil health by maintaining soil properties, shaping soil and rhizosphere prokaryotic communities, and recruiting plant-beneficial prokaryotes, which collectively enhance crop performance. Furthermore, it was hypothesized that the legacy effects of grazed pastures persist longer in LR than in SR, due to extended exposure to and stabilization of soil-microbiome feedback mechanisms. Differences in pasture plant species composition between LR and SR may contribute to these effects, although this was not directly assessed in the present study.
2 Materials and methods
2.1 Field experiment
This study was carried out in a long-term field experiment (LTE) at the National Institute of Agricultural Research (INIA) Palo a Pique Research Unit (33°15’54.4” S 54°29’28.1” W, elevation 60 m), Treinta y Tres, Uruguay. The LTE was installed in 1995 in a slightly degraded area after a short history of soybean cropping with conventional tillage followed by a sown pasture (Pravia et al., 2019). The dominant soil type is Typic Argiudol with a clay loam texture, and high to moderate erosion risk due to the gently sloping hills of modest altitude dominating the landscape. The region has a temperate sub-humid climate (Rovira et al., 2020).
Five land use intensities were evaluated under no-tillage. From highest to lowest land use intensity, the treatments are continuous grain crop rotation without pasture (CC), short pasture-crop rotation (SR, two years of sown pasture, two years of grain crops), long pasture-crop rotation (LR, four years of sown pasture, two years of grain crops), permanent improved pasture (PIP, permanent sown pasture) and natural grassland (NGL; Table 1). Pasture plant species composition in SR and LR differs, in SR, the pasture is composed of red clover (Trifolium pratense L.) and wheat (Triticum aestivum L.), and in LR of tall fescue (Festuca arundinacea L.), white clover (Trifolium repens L.) and bird’s-foot trefoil (Lotus corniculatus L.) (Rovira et al., 2020; Table 1). Cattle of the same age and body weight graze the pastures in SR, LR, PIP and NGL, mimicking a commercial farm. Grazing was excluded from SR and LR plots at the grain cropping phase. NGL was chosen as a reference for soil with minimum disturbance since only grazing cattle was permitted, while neither fertilization nor herbicides have been used.
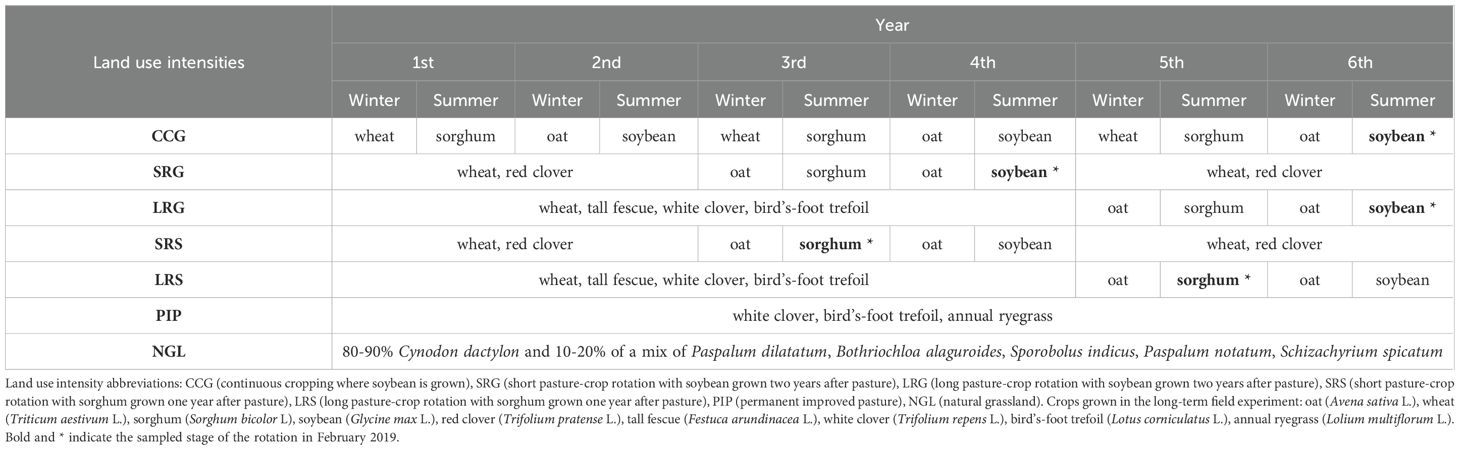
Table 1. Crop sequences of the studied land use intensities in the long-term field experiment established in 1995.
Sorghum (Sorghum bicolor L. cv ACA 558) was sampled one year after pasture, after the first winter crop [oat (Avena sativa L.)], while soybean (cv DM 60i62 IPRO) was sampled two years after pasture, after the second winter crop (oat; Table 1). This cropping sequence reflects different pasture legacy durations, specifically one and two years after pasture, respectively. Soybean was sampled in CC, SR, and LR plots further on referred to CCG, SRG, and LRG (with “G” indicating Glycine max), and sorghum was sampled additionally in SR and LR plots further on referred to SRS and LRS (Table 1).
Management practices, including machinery operations, fertilization, and agrochemical applications were similar among CC, SR, and LR, following standard commercial farm practices (Terra et al., 2006). Soybean seeds sown in CCG, SRG, and LRG were inoculated with two commercially recommended strains of Bradyrhizobium elkanii, U1301 (syn. SEMIA 587) and U1302 (syn. SEMIA 5019), in accordance with national agricultural recommendations (Cerecetto et al., 2021b). The inoculant was applied at a rate of 300 mL per 50 kg of seeds, resulting in a concentration of 1.2x1010 to 6x109 colony-forming units (CFU) per kg of seeds, following manufacturer’s instructions. Details on fertilization and herbicide applications are provided in the Supplementary Material (Supplementary Table S1). Further information on the LTE and grazing management can be found in Terra et al. (2006), Rovira et al. (2020), Pereyra-Goday et al. (2022), and Cerecetto et al. (2024).
2.2 Soil and plant sampling
The sampling was carried out in the summer of 2019 (February). Soil and plant samples were collected when plants were mostly at their full flowering growth stage, with plants ranging from beginning to end of flowering (BBCH stages 61-69; Meier, 2018). Six independent samples (replicates) assigned along a slope gradient were sampled within a 3-ha plot for each land use intensity (CCG, SRG, SRS, LRG, LRS and PIP) as well as within the NGL located next to the LTE (Table 1).
Bulk soil samples were taken from CCG, SRG, LRG, SRS, LRS, PIP and NGL. A composite sample of 15–20 soil cores per replicate was randomly collected between plants with a 2 cm soil core auger at 0–15 cm depth, and mixed and homogenized by sieving with a 2 mm mesh size to remove roots and macrofauna. A fraction of these bulk soil samples were kept at –20°C until DNA extraction. Two samples per replicate were taken from the 0–5 cm soil layer to determine bulk density (BD) using a soil core sampler (5 cm diameter x 5 cm depth).
Shoot biomass and rhizosphere samples were taken from CCG, SRG, and LRG (all soybean) as well as from SRS and LRS (both sorghum). In detail, plants growing within one linear meter were collected per replicate to estimate shoot biomass and nutrient content. Soybean and sorghum grains were harvested with a commercial harvester at harvesting time to estimate grain yield and grain nutrient content. The root system of three plants was sampled per replicate, briefly washed with distilled water to remove loosely adhering soil and pooled into a composite sample. The rhizosphere, defined as soil closely attached to roots, was retrieved from 5 g of roots resuspended in a total of 45 mL of 0.3% (w/v) saline solution, applied in three successive 15 mL extractions. Each extraction was followed by 1 min of Stomacher treatment according to Schreiter et al. (2014). One ml of the supernatant was used for serial dilutions to isolate rhizobacteria (see section 2.8), and the remaining supernatant was centrifuged (Schreiter et al. 2014) to harvest rhizosphere pellets, which were kept at –20°C until DNA extraction.
2.3 Soil properties
Bulk soil samples were first dried at room temperature and sent to INIA Soil and Plant Laboratory to determine soil chemical properties. pH was measured by potentiometric determination in water (Beretta et al., 2014). Soil total nitrogen (N) was analyzed by combustion at 900°C and subsequent N2 thermal conductivity detection. Plant available phosphorus (P) was measured by the Bray-I method (Bray and Kurtz, 1945). Plant-available potassium (K) was evaluated by ammonium acetate (pH 7) extraction followed by atomic emission (Jackson, 1964). Soil organic C (SOC) was quantified by 900°C combustion and subsequent CO2 infrared detection (Wright and Bailey, 2001).
Heterotrophic soil respiration was measured as an indicator of microbial activity using an incubation-alkaline absorption method (Moebius-Clune et al., 2016). The CO2 trapped in a 0.5 M KOH solution incubated together with 20 g of air-dried soil was used to estimate heterotrophic respiration as mg CO2 g-1 dry soil day-1. Potentially oxidizable C (PoxC), an indicator of easily available C for soil heterotrophic community, was assessed by oxidation of a solution of 0.2 M KMnO4 in 1 M CaCl2 (pH 7.2) (Weil et al., 2003). The soil autoclaved citrate extractable (ACE) protein content, an indicator of N-bound of soil organic matter that can be mineralized by microorganisms, was determined according to Moebius-Clune et al. (2016). Briefly, proteins were extracted from air-dried soil with sodium citrate buffer (20 mM, pH 7.0), autoclaved, and determined using the bicinchoninic acid (BCA) assay.
BD was calculated after drying the soil at 105°C for 24 hours (BD = soil core dry weight/soil core volume; Lienhard et al., 2013).
2.4 Plant properties
Shoot biomass was oven-dried at 55°C and weighed afterwards to determine the dry weight. All grains harvested in each plot were weighed at 13% humidity to obtain grain yield. Shoot and grain dry samples were sent to INIA Soil and Plant Laboratory to determine shoot nutrients content. Shoot organic C was determined by 900°C combustion and subsequent CO2 infrared detection technique. Shoot and grain N were analyzed by combustion at 900°C and subsequent N2 thermal conductivity detection. Shoot and grain P were measured by sulfuric digestion and vanadomolybdate colorimetry. Shoot and grain K were evaluated by dry digestion and atomic emission.
2.5 DNA extraction and sequencing
DNA was extracted from 0.5 g of frozen bulk soil or frozen rhizosphere pellet (wet weight) using a FastPrep-24 bead-beating system and the FastDNA Spin Kit for Soil (MP Biomedicals, USA), following the manufacturer’s protocol. DNA quality was checked by agarose gel electrophoresis and stored at -20°C.
Four out of six replicates per land use intensity were randomly selected for sequencing to achieve sufficient sequencing depth per sample within budget. Bulk soil and rhizosphere prokaryotic communities were characterized by sequencing the V3-V4 region of the 16S rRNA gene, amplified with primers 341F (5’-CCTAYGGGRBGCASCAG-3’) and 806R (5’-GGACTACNNGGGTATCTAAT-3’; Sundberg et al., 2013), targeting both bacterial and archaeal domains. Library construction and high-throughput amplicon sequencing were performed by Novogene (UK) on an Illumina NovaSeq PE250 platform (Illumina, USA).
2.6 Processing of amplicon sequencing reads
Cutadapt version 2.3 (Martin, 2011) was used to remove primer sequences of the first PCR. Primer-trimmed sequence reads were error-corrected and merged using a minimum overlap of five base pairs. Amplicon sequence variants (ASVs, = 100% sequence similarity) were identified using DADA2 version 1.10.0 (Callahan et al., 2016) plugin for QIIME2 (Bolyen et al., 2018). Each ASV sequence was given a taxonomic annotation (80% confidence threshold) using a q2-feature-classifier classify-sklearn module trained with the SILVA v. 138 database (Quast et al., 2013). Sequences affiliated to chloroplasts, mitochondria, or unclassified at the domain level and ASVs with less than ten reads were discarded. Furthermore, spurious ASVs with a length < 380 bp or > 420 bp were also discarded based on the expected amplicon length range for the 341F/806R primers. Raw sequences are available at NCBI Sequence Read Archive (SRA; https://www.ncbi.nlm.nih.gov/sra) under the accession number PRJNA1051053.
Sequencing of 16S rRNA gene amplicons generated 5,919,482 high-quality sequences (between 55,576 and 113,187 reads per sample) and 18,002 ASVs. Rarefaction curves reached saturation (Supplementary Figure S1).
2.7 Amplicon sequencing data analyses
Amplicon sequencing data analyses were done with R v. 4.2.2 (https://www.r-project.org/) using packages phyloseq v. 1.42.0 (McMurdie and Holmes, 2013), vegan v. 2.6-2 (Oksanen et al., 2022) and ANCOM-BC v. 2.0.1 (Lin and Peddada, 2020; Lin et al., 2022). Graphics were prepared with the R packages ggplot2 v. 3.4.0 (Wickham, 2016), pheatmap v. 1.0.12 (Kolde, 2019) and corrplot v. 0.92 (Wei and Simko, 2021).
Data analyses were performed separately for each soil compartment. Repeated rarefaction was performed for alpha-diversity analysis by subsampling the ASV table 999 times to a standardized sequencing depth equal to the minimum library size per compartment after quality filtering, in order to retain all samples (53,833 reads for bulk soil and 68,293 reads for rhizosphere samples). Alpha-diversity indices, including richness, Shannon diversity and Pielou’s evenness, were calculated and averaged across these iterations to obtain robust diversity estimates and minimize biases introduced by differences in sequencing depth. For beta-diversity, repeated rarefaction was applied using the avgdist function from the vegan package. The ASV table was subsampled 999 times at the same sequencing depth as used for alpha-diversity (53,833 for bulk soil and 68,293 for rhizosphere samples). An averaged Bray-Curtis distance matrix was then generated to reduce the impact of sequencing depth variation, ensuring a stable estimation of community dissimilarities for downstream multivariate analyses. A rank-based approach of land use intensity was used to perform a non-parametric multivariate analysis of variance (PERMANOVA; Anderson, 2001) based on Bray-Curtis dissimilarities to test the effect of land use intensity on the prokaryotic communities. NGL was ranked as no intensity (0), PIP as lowest intensity (1), LR and SR as medium intensity (2 and 3, respectively, based on the proportion of time with sown pasture within the rotation), and CC as highest intensity (4). Afterwards, pairwise PERMANOVA analyses were performed using a Benjamini-Hochberg correction. Non-metric multidimensional scaling (NMDS) analyses based on the Bray-Curtis distance were used to visualize community dissimilarities. The heteroscedasticity of community assemblages using Bray–Curtis distance was tested using permutational analysis of multivariate dispersions (PERMDISP; Anderson, 2006).
Distance-based redundancy analyses (db-RDA) based on Bray-Curtis dissimilarities were performed to assess the contribution of land use intensity and soil properties to the variation in bulk soil prokaryotic communities. Relevant soil parameters were selected using backward and forward selection (ordiR2step function from the vegan package).
The relative abundances of the 25 most abundant prokaryotic genera in bulk soil and rhizosphere were visualized in heatmaps to compare their abundance distributions. Partial 16S rRNA gene sequences of the commercial soybean inoculant strains (B. elkanii U1301, B. elkanii U1302) were mapped to ASV sequences obtained from 16S rRNA gene amplicon sequences to assess the presence of the inoculant in the sequencing data. The mapping was performed using the NCBI BLAST+ blastn megablast tool (Camacho et al., 2009; Cock et al., 2015) on the local Galaxy server of the Julius Kühn Institute (Germany).
An analysis of the composition of microbiomes with bias correction (ANCOM-BC; Lin and Peddada, 2020; Lin et al., 2022) was performed at the genus level for bulk soil samples to identify genera whose relative abundances consistently varied among land use intensities. Afterwards, logistic regression models were applied to each discriminant genus to assess its association to each land use intensity. Only models with adjusted p-values < 0.05, after applying the Benjamini-Hochberg correction, were considered. Only the taxa that were significantly and positively associated with a specific land use intensity were considered in this study and termed unique responders, as previously applied in Cerecetto et al. (2024). Additional ANCOM-BC were performed at the ASV level for rhizosphere samples to identify differentially abundant ASVs among CCG, SRG, and LRG and between SRS and LRS.
Spearman correlations were performed between the relative abundance of the most abundant prokaryotic genera in the bulk soil and soil properties, and between bulk soil unique responders and soil properties to explore the relationship between the prokaryotic bulk soil community and soil properties.
2.8 Cultivation-dependent isolation of soybean and sorghum rhizobacteria
Dilution series up to 10–8 of each supernatant were prepared and plated on R2A supplemented with 100 µg mL-1 cycloheximide to reduce fungal growth. The plates were incubated at 28°C, and CFU were determined after 24 h, 48 h, and seven days. In total, one hundred bacterial colonies were picked randomly and further cultivated on R2A to obtain purified isolates.
2.9 Identification of soybean and sorghum rhizobacterial isolates
Genomic DNA of bacterial isolates was extracted using the Genomic DNA Extraction Kit (Qiagen, Germany) and the Silica Bead DNA Gel Extraction Kit (Thermo Fisher Scientific, USA) following the manufacturer’s instructions. Isolates partial 16S rRNA gene sequences were amplified according to Heuer et al. (2009) and sequenced in Macrogen Europe (The Netherlands). The obtained forward and reverse sequences were trimmed and assembled using CLC MainWorkbench v. 20.0.3 (Qiagen, Denmark). The resulting consensus sequences were blasted to the RDP database.
Isolates’ 16S rRNA gene consensus sequences were mapped to ASV sequences, obtained by 16S rRNA gene amplicon sequencing, on the local Galaxy server of the Julius Kühn Institute, employing NCBI BLAST + blastn megablast tool (Camacho et al., 2009; Cock et al., 2015) and using a 98% of sequence similarity as threshold, commonly used to approximate species-level delineation for partial 16S rRNA gene sequences while accounting for sequencing and PCR errors (Nishioka and Tamaki, 2022).
A maximum likelihood phylogenetic tree was constructed using MEGA v. 11.0.13. This tree was based on the 16S rRNA gene sequences of ASVs that were found to be differentially abundant in sorghum and soybean rhizospheres (as identified by ANCOM-BC), as well as isolates that shared more than 98% similarity with these ASVs, and relevant NCBI reference strains.
2.10 Screening of rhizobacterial isolates for potential plant-beneficial traits
Protease, β-1,3-glucanase, and cellulase activity were tested according to Weinert et al. (2010), and chitinolytic activity was determined after Berg et al. (2001) to characterize biocontrol potential of isolates. These enzymes degrade proteins and polysaccharides present e.g. in fungal cell walls, thereby suppressing fungal pathogens (Elsayed et al., 2021). Phosphate solubilization and secretion of siderophores were assessed according to Nautiyal (1999) and Schwyn and Neilands (1987), respectively. Phosphate solubilization enhances phosphate availability for plant growth and development (Alori et al., 2017), while siderophores chelate iron, facilitating its uptake by plants and restricting its access to pathogens (Ansari et al., 2017). The production of phytohormone indole-3-acetic acid (IAA) and the 1-aminocyclopropane-1-carboxylic acid (ACC) deaminase activity were measured based on Koo et al. (2010). IAA promotes root elongation and branching, enhancing water and nutrient uptake (Lebrazi et al., 2020), while ACC deaminase alleviates plant stress by lowering ethylene levels under salinity or drought (Orozco-Mosqueda et al., 2018). The production of N-Acyl homoserine lactones (AHLs) was detected in a cross-streak assay using Chromobacterium violaceum cv026 for short-chain detection of C4-C8 AHLs and C. violaceum VIr07 for long-chain detection of C10-C16 AHLs (Durán et al., 2016). The ability to produce AHLs indicates quorum sensing activity, which influences bacterial communication, biofilm formation, and plant responses, with short-chain AHLs promoting growth and long-chain AHLs enhancing resistance (Shrestha and Schikora, 2020).
2.11 Univariate statistical analyses
Univariate statistical analyses and graphics were done with R v. 4.2.2 using packages agricolae v. 1.3-5 (de Mendiburu, 2020) and ggplot2 v. 3.4.0 (Wickham, 2016).
Plant and soil properties, and prokaryotic alpha-diversity indices were evaluated as follows: if normality or homogeneity of variance were violated, overall differences were assessed with Kruskal-Wallis tests and pairwise differences with Wilcoxon rank sum tests with Benjamini-Hochberg corrections. If assumptions were not violated, overall differences were evaluated with one-way ANOVAs and pairwise differences with Tukey’s HSD tests. All statistical tests were performed separately for each soil compartment.
3 Results
3.1 Land use intensification negatively affected soil properties
The measured soil nutrients were affected by land use intensity at 0–15 cm depth. Differences among land use intensities were assessed based on ANOVA-Tukey´s HSD or Kruskal-Wallis-pairwise Wilcoxon tests (p < 0.05) when normality or homogeneity of variance assumptions were violated. Soil organic C (SOC) and total N were lowest in continuous cropping with soybean (CCG), with the highest values of SOC in long pasture-crop rotation with soybean grown two years after pasture (LRG, p = 0.022) and total N in LRG, short pasture-crop rotation with soybean grown two years after pasture (SRG) and permanent improved pasture (PIP, p = 0.041, Figures 1a, b). PIP showed the highest levels of potentially oxidizable C (PoxC), and CCG and natural grassland (NGL) the lowest (p = 0.002, Figure 1c). Available P was highest in CCG and lowest in NGL (p = 0.030, Figure 1d). Contrary to available P, K levels were higher in NGL compared to the rest of the land use intensities, except for SRG and PIP (p = 0.016, Figure 1e). The pH was also significantly different among land use intensities at 0–15 cm, and was higher in PIP than in pasture-crop rotations (p = 0.026, Figure 1f).
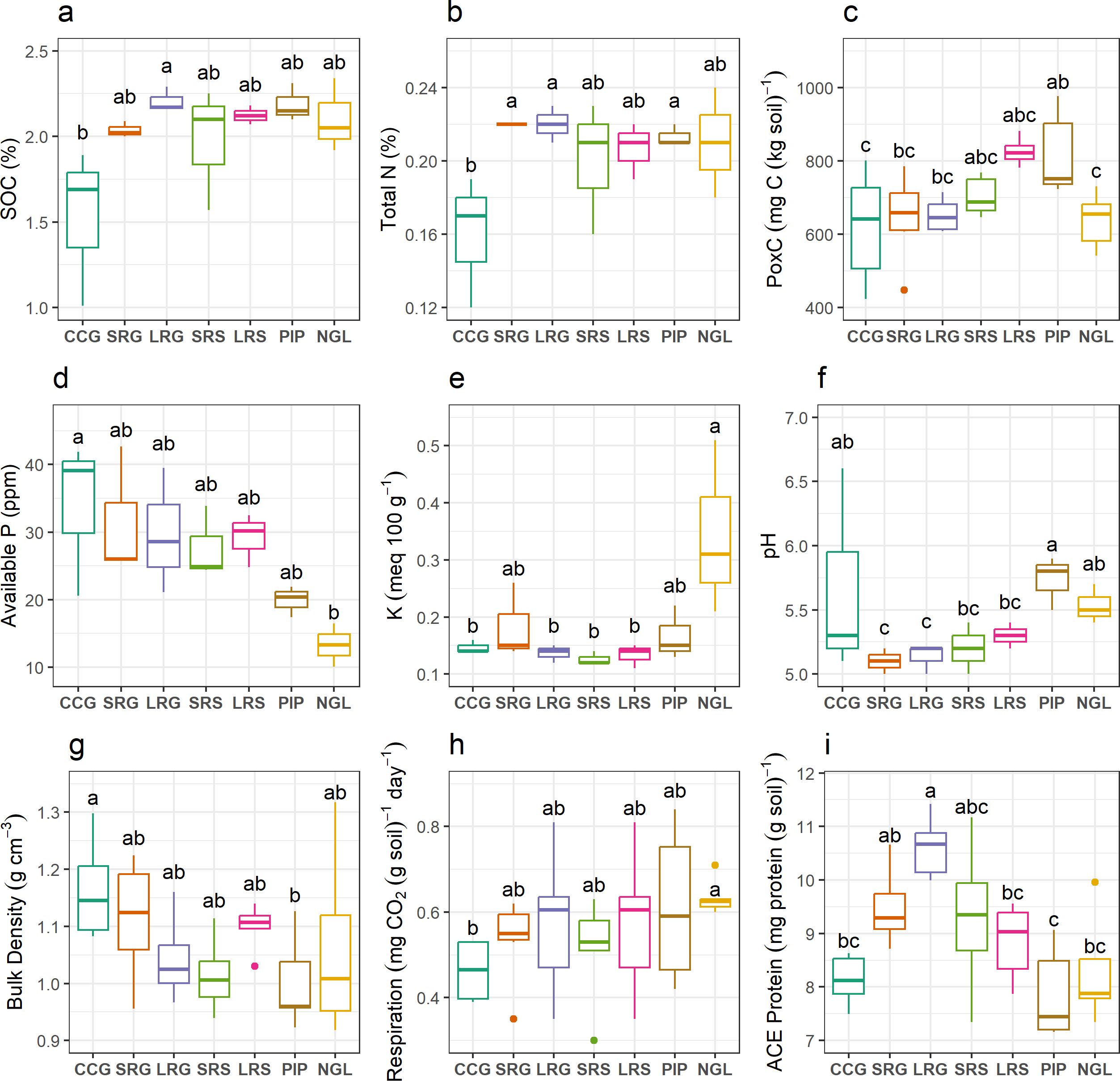
Figure 1. Measured soil physicochemical properties and biological proxies: (a) Soil organic C (SOC); (b) Total N; (c) Potentially oxidizable C (PoxC); (d) Available P; (e) K; (f) pH; (g) Bulk density; (h) heterotrophic soil respiration; (i) Soil autoclaved citrate extractable (ACE) protein content. Data represents mean and standard deviation of six replicates. Significant differences among land use intensities are indicated by different letters, according to ANOVA-Tukey´s HSD (p < 0.05). Land use intensity abbreviations: CCG (continuous cropping where soybean is grown), SRG (short pasture-crop rotation with soybean grown two years after pasture), LRG (long pasture-crop rotation with soybean grown two years after pasture), SRS (short pasture-crop rotation with sorghum grown one year after pasture), LRS (long pasture-crop rotation with sorghum grown one year after pasture), PIP (permanent improved pasture), NGL (natural grassland).
Intensity affected soil bulk density (BD), with the highest values observed in CCG and the lowest in PIP (p = 0.019, Figure 1g). Pasture-crop rotations showed BD values comparable to PIP and to CCG (Figure 1g).
Furthermore, heterotrophic soil respiration and soil autoclaved citrate extractable (ACE) protein content were affected by land use intensity (Figures 1h, i). Heterotrophic soil respiration was significantly lower only in CCG compared to NGL (p = 0.024), while all pasture-crop rotations and PIP did not differ from either CCG or NGL (Figure 1h). Moreover, soil ACE protein content was higher in LRG compared to CCG, long pasture-crop rotation with sorghum grown one year after pasture (LRS), PIP and NGL (p < 0.001, Figure 1i).
3.2 Bulk soil prokaryotic communities shaped by land use intensity
Shannon diversity indices of bulk soil communities were highest in LRG, SRG and short pasture-crop rotation with sorghum grown one year after pasture (SRS) and lowest in NGL (p = 0.011, Figure 2a). Species richness followed the same pattern, with the highest values observed in SRG, LRG, and PIP, and the lowest in NGL (p < 0.001, Supplementary Figure S2a). However, Pielou’s evenness index did not show significant differences among land use intensities (p = 0.070, Supplementary Figure S2b).
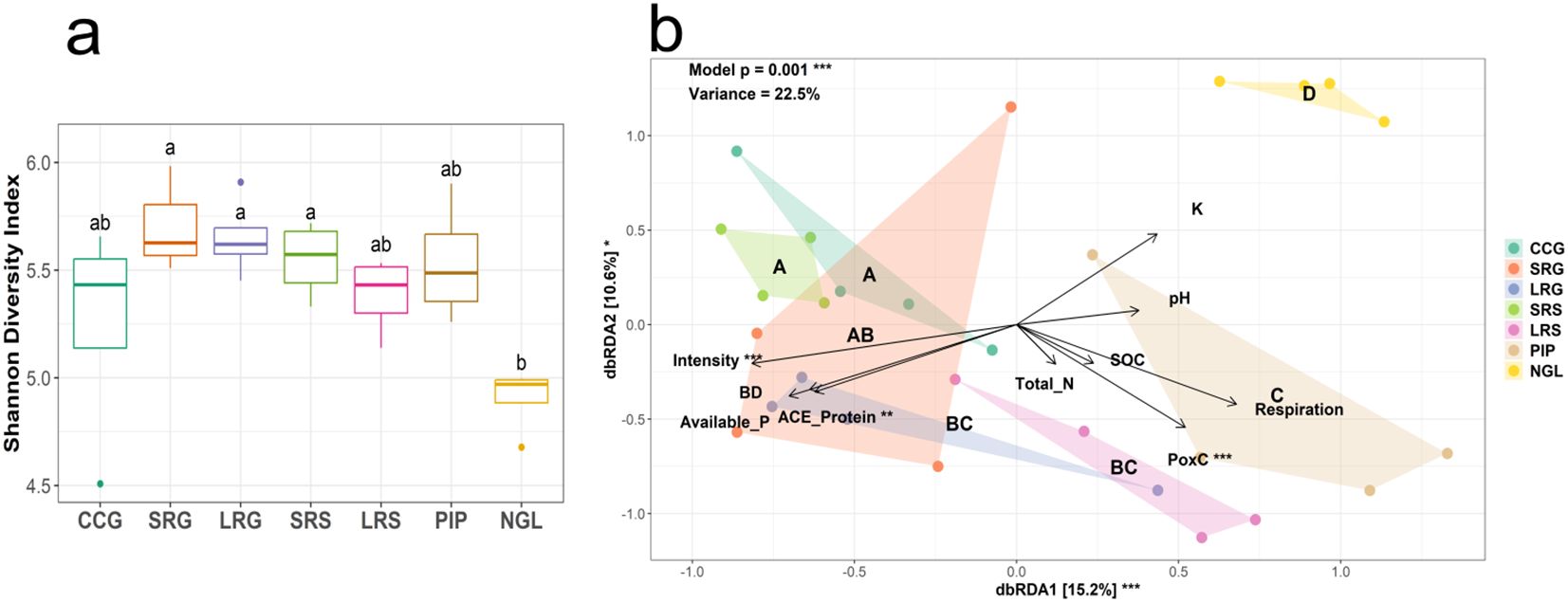
Figure 2. (a) Bulk soil prokaryotic Shannon diversity indices. Significant differences among land use intensities are indicated by different letters, according to ANOVA-Tukey´s HSD (p < 0.05). (b) Prokaryotic bulk soil community distance-based redundancy analysis (db-RDA) based on Bray-Curtis distance. Significance of model, axis and factors were determined by ANOVA. Points represent individual samples, colored by land use intensity. Convex hulls group samples by land use intensity. Different letters indicate statistical differences in prokaryotic composition between land use intensities based on pairwise non-parametric multivariate analysis of variance (PERMANOVA; p < 0.05), with land use intensities sharing the same letter not being significantly different. Land use intensity abbreviations: CCG (continuous cropping where soybean is grown), SRG (short pasture-crop rotation with soybean grown two years after pasture), LRG (long pasture-crop rotation with soybean grown two years after pasture), SRS (short pasture-crop rotation with sorghum grown one year after pasture), LRS (long pasture-crop rotation with sorghum grown one year after pasture), PIP (permanent improved pasture), NGL (natural grassland). Soil property and biological proxy abbreviations: BD (bulk density), SOC (soil organic C), PoxC (potentially oxidizable C), ACE Protein (soil autoclaved citrate extractable protein content). *(p < 0.05), **(p < 0.01), ***(p ≤ 0.001).
PERMANOVA, NMDS, and db-RDA revealed that bulk soil prokaryotic community composition was significantly affected by land use intensity (p = 0.001, Table 2, Figure 2b; Supplementary Figure S3). Pairwise PERMANOVA tests showed that LRG and LRS communities were significantly different from those in CCG (p = 0.004, p = 0.048) but similar to those in PIP (p = 0.060, p = 0.095), whereas SRG and SRS communities were similar to CCG (p = 0.500, p = 0.054) and significantly different from PIP (p = 0.020, p = 0.029, Figure 2b; Supplementary Table S2). Notably, all bulk soil land use intensities communities differed significantly from NGL (p < 0.05, Figure 2b; Supplementary Table S2). Additionally, PERMDISP tests were non-significant (p > 0.05), indicating that community differences were driven by land use intensity rather than dispersion effects (Supplementary Tables S3, S4).

Table 2. Land use intensity effect on bulk soil and soybean and sorghum rhizosphere prokaryotic communities tested by non-parametric multivariate analysis of variance (PERMANOVA).
Moreover, a db-RDA was conducted to assess the contribution of land use intensity, soil physicochemical properties, and biological proxies to the differentiation of bulk soil prokaryotic communities. The results indicated that intensity, along with PoxC and soil ACE protein content, were the primary drivers of bulk soil prokaryotic communities (Figure 2b). Land use intensity and soil ACE protein content were positively correlated with bulk soil prokaryotic communities in CCG, SRG, LRG, and SRS and negatively with LRS, PIP, and NGL, contributing to their separation along axis 1 (Figure 2b). Contrarily, PoxC was positively correlated with LRS and PIP and negatively with CCG, SRG, LRG, SRS, and NGL, contributing to their separation along axes 1 and 2 (Figure 2b).
3.3 Key taxa as indicators for soil health
Spearman’s correlations were employed to explore the correlation of the 25 most abundant bulk soil prokaryotic genera with measured soil physicochemical properties and biological proxies (Figures 3a, b). Bacillus (Firmicutes), unclassified Nitrososphaeraceae (Thaumarchaeota) and Acidobacteriales (Acidobacteria) members, Candidatus Udaeobacter (Verrucomicrobia), unclassified Gaiellales (Actinobacteria) members, Candidatus Nitrocosmicus (Thaumarchaeota) and Acidothermus (Actinobacteria) were among the dominant genera (Figure 3a). For a further description of the taxonomic composition of prokaryotic bulk soil community, please see the Supplementary Material (Supplementary Figure S4a).
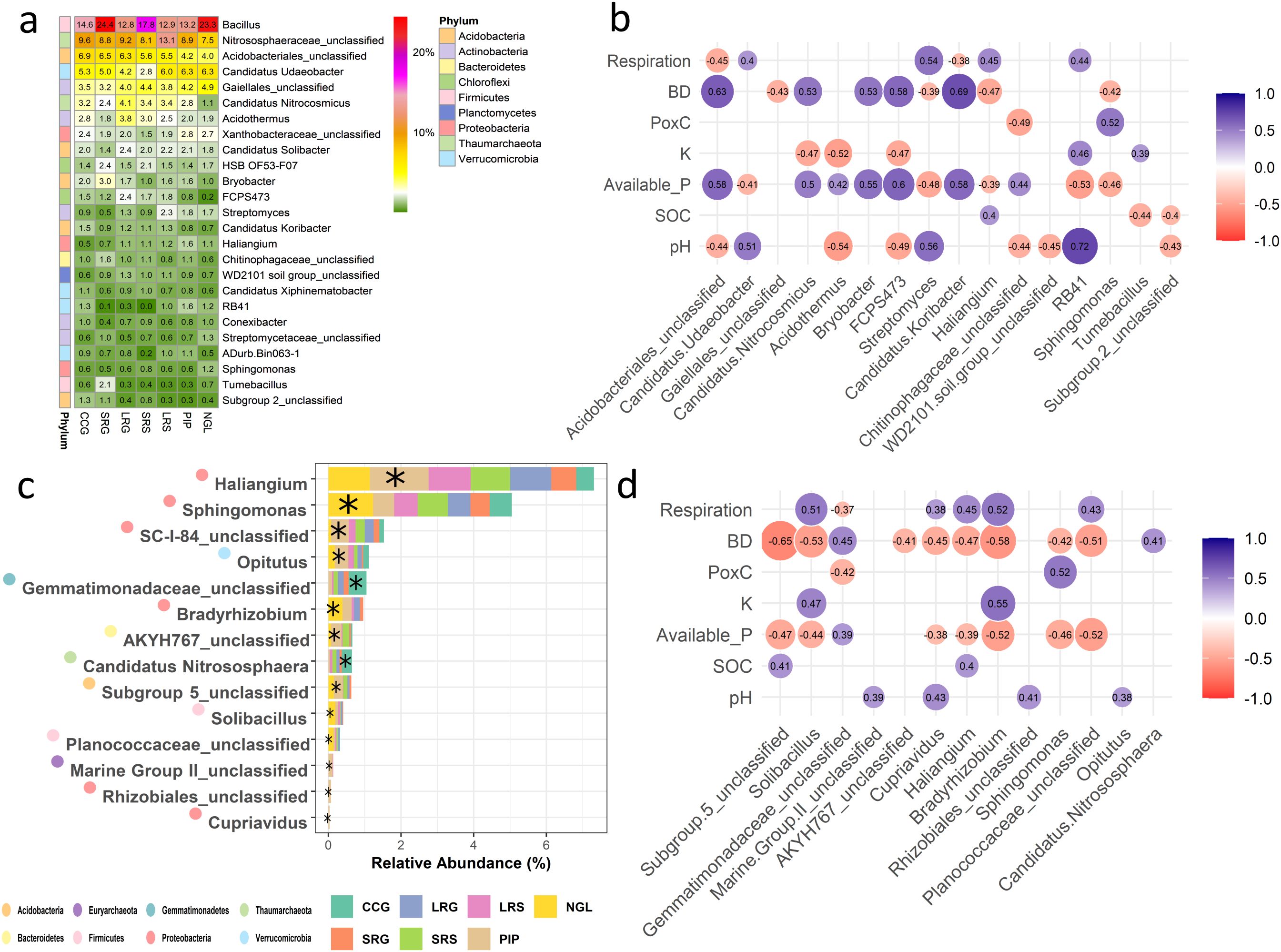
Figure 3. (a) Relative abundance of the 25 most abundant prokaryotic taxa in bulk soil, based on ASVs assigned to the genus level. Colors and numbers indicate mean relative abundance per taxon (n = 4). (b) Spearman correlations between the relative abundance of the 25 most abundant prokaryotic genera in bulk soil and measured soil physicochemical properties and biological proxies. (c) Prokaryotic unique responders to each land use intensity in bulk soil determined by analysis of the composition of microbiomes with bias correction (ANCOM-BC) at the genus level and logistic regression models applied to each discriminant genus. Data represents the mean relative abundance of each taxon (n = 4) in each land use intensity. * Indicates the land use intensity for which the taxa are unique responders. (d) Spearman correlations between the relative abundance of the bulk soil prokaryotic unique responders to land use intensities and measured soil physicochemical properties and biological proxies. Only significant correlations are shown (p < 0.05). Colors and numbers indicate correlation coefficients. Land use intensity abbreviations: CCG (continuous cropping where soybean is grown), SRG (short pasture-crop rotation with soybean grown two years after pasture), LRG (long pasture-crop rotation with soybean grown two years after pasture), SRS (short pasture-crop rotation with sorghum grown one year after pasture), LRS (long pasture-crop rotation with sorghum grown one year after pasture), PIP (permanent improved pasture), NGL (natural grassland). Soil property and biological proxy abbreviations: BD (bulk density), SOC (soil organic C), PoxC (potentially oxidizable C).
This initial correlation analysis of the top 25 most abundant genera revealed significant relationships between prokaryotic composition and soil properties. Sixteen genera showed significant correlations (p < 0.05) with heterotrophic soil respiration, BD, PoxC, K, available P, SOC, and pH. Among them, 11 had correlation coefficients greater than 0.5 (|r| > 0.5) (Figure 3b). The genera that positively correlated with BD [i.e. Acidobacteriales, Candidatus Nitrocosmicus, Bryobacter (Acidobacteria), FCPS473 (Chloroflexi), Candidatus Koribacter (Acidobacteria)] were also positively correlated with available P and exhibited highest relative abundances in CCG, SRG, and LRG (Figures 3a, b). Contrarily, the genera that positively correlated with PoxC, heterotrophic soil respiration and pH [Sphingomonas (Proteobacteria) with PoxC, Streptomyces (Actinobacteria) with heterotrophic soil respiration and pH, and Candidatus Udaeobacter and RB41 (Acidobacteria) with pH] exhibited highest relative abundances in LRS, PIP, and NGL (Figures 3a, b).
Using ANCOM-BC and multiple logistic regressions we identified unique responders to land use intensities (Figure 3c), however, none of them belonged to the dominant genera (Figure 3a), with the exception of Sphingomonas, which was a unique responder in NGL (Figure 3c). A Spearman’s correlation analysis was conducted to explore the relationships between unique responders and soil physicochemical properties and biological proxies. Bradyrhizobium (Proteobacteria) showed significant positive correlations with heterotrophic soil respiration and K, and significant negative correlations with BD and available P, while Solibacillus (Firmicutes) was significantly positively correlated with heterotrophic soil respiration and significantly negatively with BD (Figure 3d). Additionally, unclassified members of Planococcaceae (Firmicutes) were significantly negatively correlated with BD and available P, and members of Subgroup 5 (Acidobacteria) were significantly negatively correlated with BD (Figure 3d). Moreover, Sphingomonas, Bradyrhizobium, Solibacillus, and unclassified members of Planococcaceae were identified as unique responders in NGL, while Subgroup 5 was a unique responder in PIP (Figure 3c). Furthermore, it is worth noting that at the genus level, pasture-crop rotations did not have any unique responders as identified by ANCOM-BC and multiple logistic regressions. Instead, the main compositional differences in terms of differentially abundant genera were observed among the intensity extremes, i.e., CCG, PIP, and NGL (Figure 3c).
3.4 Rhizosphere prokaryotic communities mainly shaped by crop type and, to a lesser extent, by land use intensity
Shannon diversity and Pielou’s evenness indices significantly differed between crops and were higher in sorghum than in soybean (p < 0.001). Within each crop type, no differences were observed among land use intensities (p > 0.05, Figure 4a; Supplementary Figure S2b). Moreover, species richness did not significantly differ among crops and/or intensities (p > 0.05, Supplementary Figure S2a).
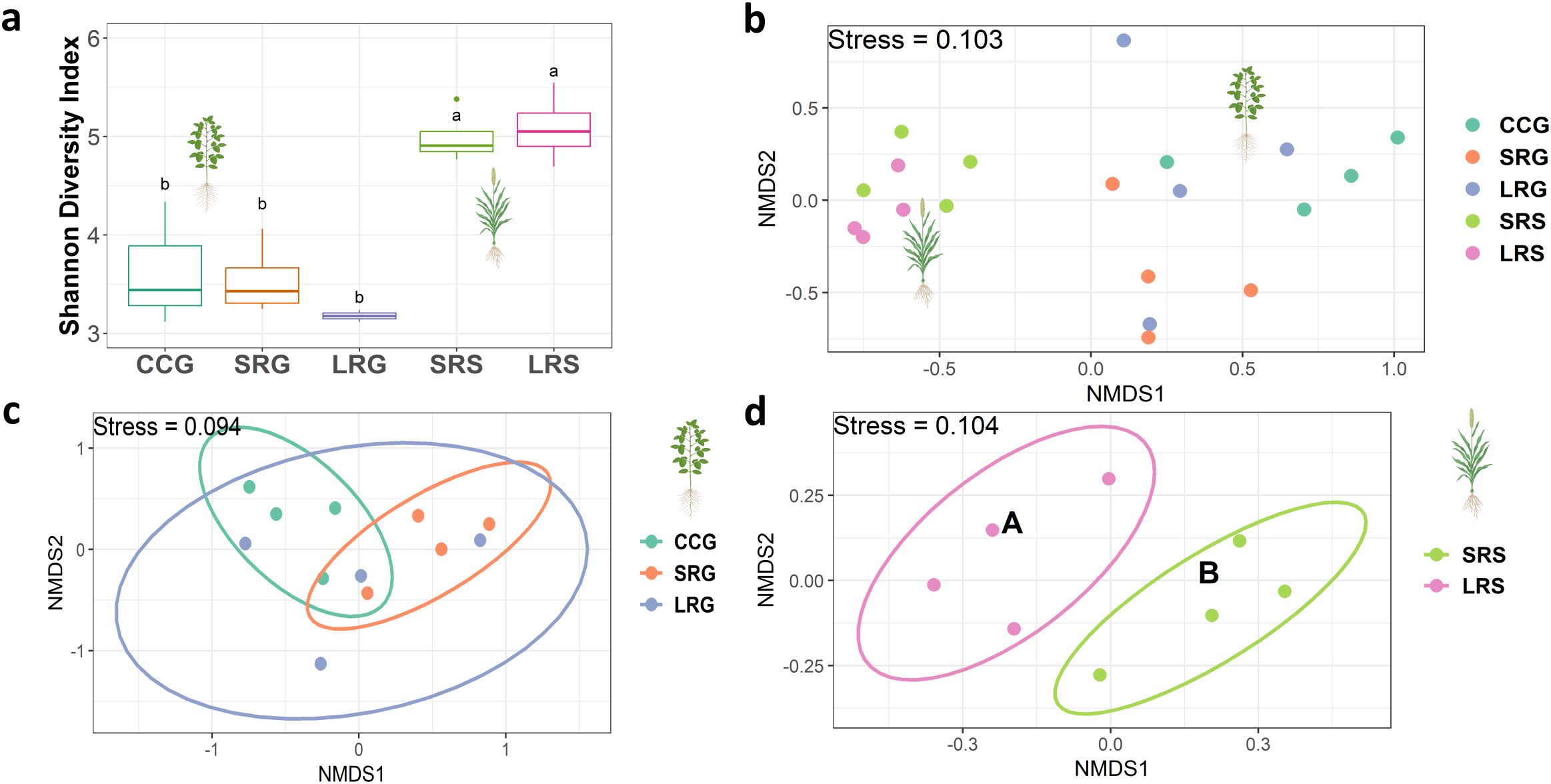
Figure 4. (a) Soybean and sorghum rhizosphere prokaryotic Shannon diversity indices. Significant differences between crop type are indicated by different letters, according to ANOVA-Tukey´s HSD (p < 0.05). No significant differences among land use intensities were found (p > 0.05). (b) Soybean and sorghum rhizosphere prokaryotic communities non-metric multi-dimensional scaling (NMDS) based on Bray-Curtis distance. Each point represents an individual sample, colored by land use intensity. (c) Soybean rhizosphere prokaryotic community NMDS based on Bray-Curtis distance. Each point represents an individual sample, colored by land use intensity. Ellipses represent 95% confidence intervals around the group centroids, assuming a multivariate normal distribution. Missing letters indicate no significant differences in prokaryotic composition among land use intensities based on pairwise non-parametric multivariate analysis of variance (PERMANOVA; p > 0.05). (d) Sorghum rhizosphere prokaryotic community NMDS based on Bray-Curtis distance. Each point represents an individual sample, colored by land use intensity. Ellipses represent 95% confidence intervals around the group centroids, assuming a multivariate normal distribution. Different letters indicate statistical differences in prokaryotic composition between land use intensities based on pairwise PERMANOVA (p < 0.05). Land use intensity abbreviations: CCG (continuous cropping where soybean is grown), SRG (short pasture-crop rotation with soybean grown two years after pasture), LRG (long pasture-crop rotation with soybean grown two years after pasture), SRS (short pasture-crop rotation with sorghum grown one year after pasture), LRS (long pasture-crop rotation with sorghum grown one year after pasture). Plant figures created with BioRender.
PERMANOVA tests and NMDS indicated that the effects of land use intensity were detected only in the sorghum rhizosphere (p = 0.033), not in the soybean rhizosphere (p = 0.075, Table 2, Figures 4b–d; Supplementary Table S2). Prokaryotic community composition differed significantly between short and long pasture-crop rotations with sorghum (SRS and LRS), but not among CCG, short, and long pasture-crop rotations with soybean (SRG, and LRG, Figures 4c, d, Table 2; Supplementary Table S2). Moreover, pairwise PERMANOVA tests comparing SRS vs. SRG (p = 0.027) and LRS vs. LRG (p = 0.033) showed significant differences (Supplementary Table S2). Additionally, PERMDISP tests were non-significant (p > 0.05), indicating that community differences were driven by land use intensity, crop type or pasture legacy duration rather than dispersion effects (Supplementary Tables S3, S4).
3.5 Rhizosphere isolates linked with ASVs increased in pasture-crop rotations expressed a diverse set of plant-beneficial traits
When examining the top 25 most abundant genera in the soybean rhizosphere, Bradyrhizobium (Proteobacteria) and unclassified members of Xanthobacteraceae (Proteobacteria) were dominant across CCG, SRG, and LRG (Figure 5a). Additionally, a total of 100 ASVs, obtained from amplicon sequencing and assigned to these two taxa, matched the 16S rRNA gene sequences of the commercial soybean inoculant strains (B. elkanii U1301, B. elkanii U1302) with > 99.74% sequence identity. However, one ASV matched both strains with 100% sequence identity, but could only be reliably classified within the Xanthobacteraceae family, likely due to the limited resolution of the 16S rRNA gene V3-V4 region V3-V4 region for differentiating closely related genera within this family (Supplementary Table S5). Moreover, the relative abundance of all ASVs assigned to unclassified members of Xanthobacteraceae, that matched the inoculated strains, was significantly higher than that of Bradyrhizobium, with the highest values observed in SRG and LRG (Supplementary Table S6).
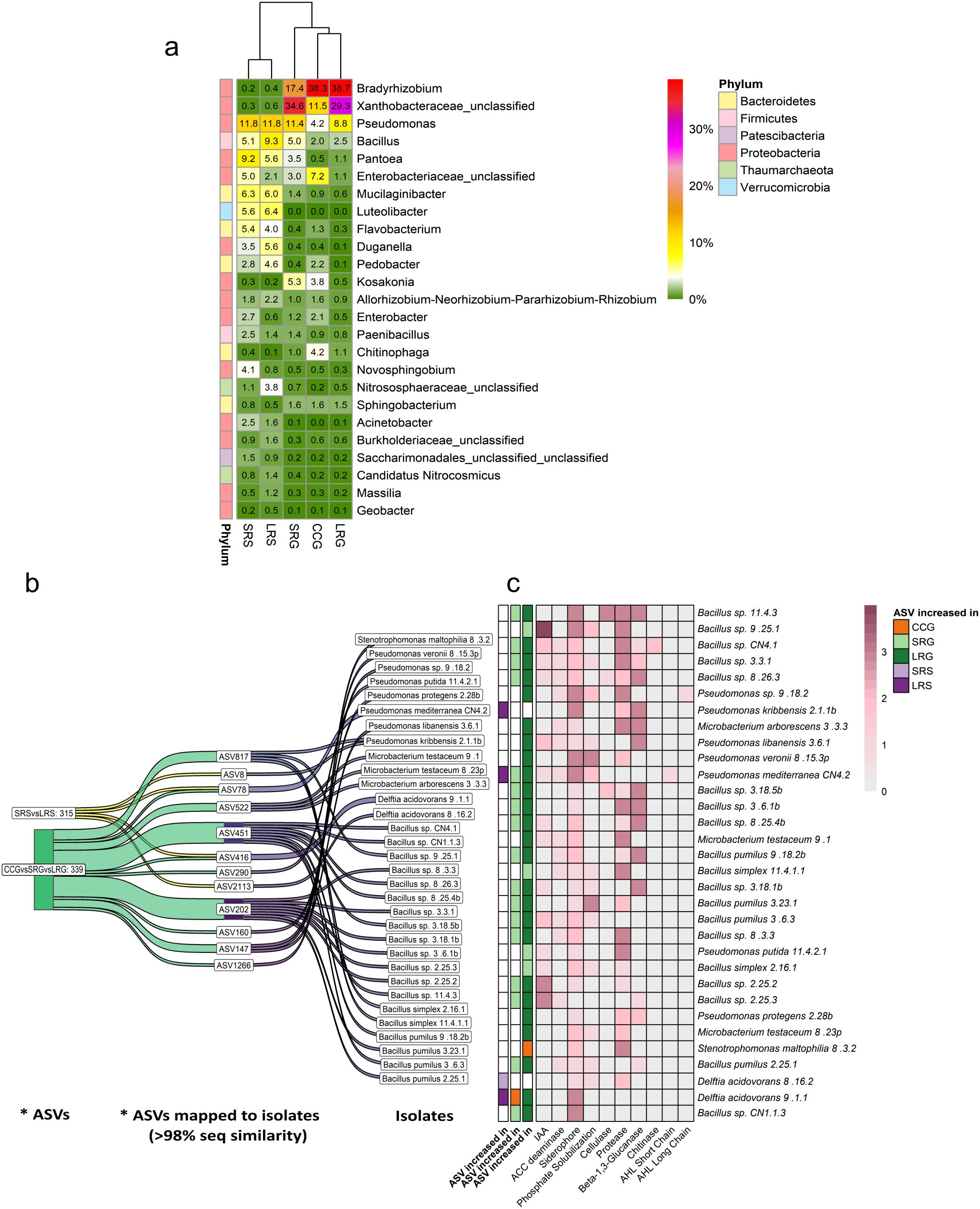
Figure 5. (a) Relative abundance of the 25 most abundant prokaryotic taxa in soybean and sorghum rhizospheres, based on ASVs assigned to the genus level. Colors and numbers indicate mean relative abundance of each taxon (n = 4). (b) Isolates that shared > 98% of sequence similarity with ASVs that were found differentially abundant after performing an analysis of the composition of microbiomes with bias correction (ANCOM-BC) in sorghum (SRS vs. LRS) and soybean (CCG vs. SRG vs. LRG) rhizospheres, separately. * ASVs (differentially abundant ASVs, p < 0.05 in ANCOM-BC). (c) Plant-beneficial traits of the isolates mapped to the differentially abundant ASVs and the land use intensities where these ASVs are increased. The results of ANCOM-BC are shown in the first three columns: comparison SRS vs. LRS (first column) and CCG vs. SRG vs. LRG (second and third column; results are split into two columns since some ASVs were increased in two of the three land use intensities). White indicates no significant increase in any of the compared land use intensities. Land use intensity abbreviations: CCG (continuous cropping where soybean is grown), SRG (short pasture-crop rotation with soybean grown two years after pasture), LRG (long pasture-crop rotation with soybean grown two years after pasture), SRS (short pasture-crop rotation with sorghum grown one year after pasture), LRS (long pasture-crop rotation with sorghum grown one year after pasture). Plant-beneficial trait abbreviations: ACC deaminase (1-aminocyclopropane-1-carboxylate deaminase), AHLs (acyl-homoserine lactones), IAA (indole-3-acetic acid).
An ANCOM-BC was conducted to assess whether specific ASVs differed in abundance among CCG, SRG, and LRG. This analysis revealed that a total of 339 ASVs were differentially abundant among CCG, SRG, and LRG (p < 0.05, Figure 5b). Furthermore, an additional ANCOM-BC was conducted to detect significant differences between SRS and LRS at ASV level, revealing a total of 315 differentially abundant ASVs (p < 0.05, Figure 5b). Across both comparisons, twelve of these differentially abundant ASVs shared more than 98% sequence similarity with 32 of the 100 bacterial rhizosphere isolates obtained in this study (Figure 5b). Most of these isolates belonged to the genera Bacillus (Firmicutes) and Pseudomonas (Proteobacteria), and a few to Microbacterium (Actinobacteria), Delftia (Proteobacteria) and Stenotrophomonas (Proteobacteria) (Figures 5b, c, Supplementary Table S7, Supplementary Figure S5). Additionally, most isolates showed a wide range of plant-beneficial traits according to in vitro tests and were mapped to ASVs significantly increased in pasture-crop rotations compared to CCG (Figure 5c). Stenotrophomonas maltophilia 8.3.2 and Delftia acidovorans 9.1.1 were the only two isolates which were linked to ASVs increased in CCG and showed the least plant-beneficial traits. S. maltophilia 8.3.2 was only able to produce siderophores and protease, and D. acidovarans 9.1.1 only produced siderophores (Figure 5c). It is worth noting that the ASV mapped to D. acidovorans 9.1.1 (ASV416) was also increased in LRG, apart from CCG, when compared to SRG, and in LRS when compared to SRS. Yet the ASV mapped to S. maltophilia 8.3.2 (ASV290) was only increased in CCG when compared to SRG and LRG (Figure 5c).
Moreover, it should be noted that Pseudomonas and Bacillus were among the top 25 genera in the sorghum rhizosphere, while in the soybean rhizosphere, they were the most abundant after Bradyrhizobium and unclassified members of Xanthobacteraceae. Although, Microbacterium, Delftia, and Stenotrophomonas were not among the most abundant genera (Figure 5a), they were successfully isolated. For a further description of the taxonomic composition of sorghum and soybean rhizosphere prokaryotic communities, please see the Supplementary Material (Supplementary Figure S4b).
3.6 Pasture legacy in commercial grain yield
Soybean shoot biomass did not differ significantly between plants cultivated two years after pasture in SRG and LRG, and those cultivated in CCG (p = 0.083); however, a trend towards higher shoot biomass in LRG and SRG than CCG was observed (Table 3). This pattern was also reflected in commercial grain yield, where LRG and SRG produced twice the grain yield of CCG (Table 3). It is worth noting that these commercial grain yields were obtained for the whole plot without replicates. Additionally, the majority of measured shoot and grain nutrient contents did not differ significantly among CCG, SRG, and LRG (p > 0.05), except for shoot K, which was highest in CCG and LRG (p = 0.002, Supplementary Tables S8, S9).
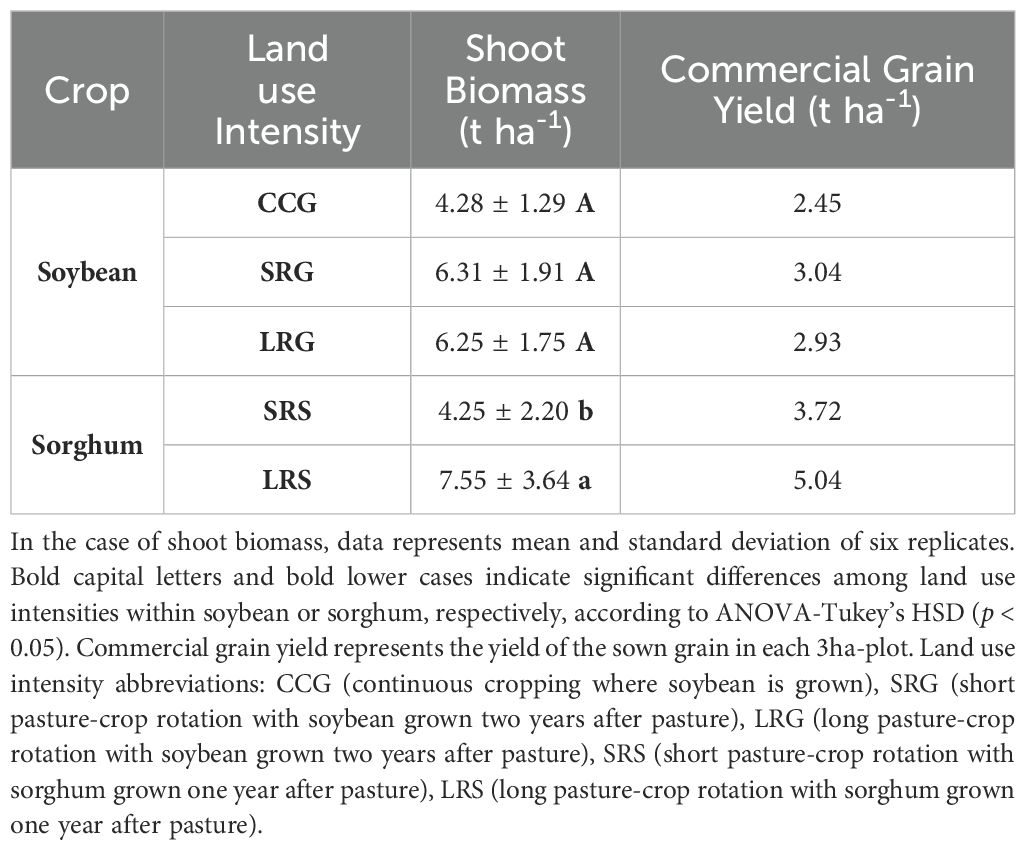
Table 3. Soybean and sorghum shoot biomass and commercial grain yield, expressed as dry weight of shoot and grain per hectare, respectively.
For sorghum, grown one year after pasture in SRS and LRS, shoot biomass differed significantly (p < 0.001), with LRS exhibiting a higher shoot biomass and commercial grain yield (Table 3). Nevertheless, no significant differences were found in their shoot and grain nutrient contents (p > 0.05, Supplementary Tables S8, S9).
4 Discussion
Understanding the role of grazed pastures in shaping bulk soil and rhizosphere microbiomes is essential for designing sustainable rotation strategies that maintain soil health and enhance crop performance. Therefore, in a LTE in the Uruguayan Pampa, the effects of land use intensification and pasture legacy on soil properties, bulk soil and rhizosphere prokaryotic communities and crop performance were evaluated. The first hypothesis was partially supported: while introducing grazed pastures into crop rotations preserved certain soil health indicators in comparison to continuous cropping (CC), the enhancement was limited to certain soil properties (e.g., SOC, total N, soil ACE protein content) (Figure 1). In addition, shifts in bulk soil prokaryotic community composition were associated with increased values of key soil health indicators, such as PoxC, heterotrophic soil respiration, and soil ACE protein content, and with decreased values of bulk density (Figures 2, 3). Moreover, pasture-crop rotations enriched rhizosphere prokaryotic communities with potentially plant-beneficial bacteria, and overall, they led to improved grain yield (Figure 5, Table 3). The second hypothesis was partially supported: while the pasture legacy persisted in the long pasture-crop rotation (LR), shaping prokaryotic communities and influencing sorghum performance (Figures 2–5, Table 3), the lack of significant differences between LR and short pasture-crop rotation (SR) in most soil physicochemical properties and biological proxies (Figure 1) suggests that pasture duration and plant species composition had only a minor effect on these conventional indicators of soil health. However, differences in prokaryotic composition and sorghum performance between LR and SR (Figures 2–5, Table 3) point to the biological relevance of more subtle shifts in soil conditions, emphasizing the role of prokaryotic dynamics in mediating long-term management effects.
4.1 Impact of intensification and pasture legacy on soil properties
The intensification of agricultural practices significantly influenced soil physicochemical properties and biological proxies. Less intensive systems, such as pasture-crop rotation, permanent improved pasture (PIP) and natural grassland (NGL), significantly improved soil properties in seven out of nine comparisons relative to continuous cropping with soybean (CCG), highlighting the degradative effect of CCG. Particularly, pasture-crop rotations showed significant differences from CCG in only a subset of parameters, even two years after pasture. Nonetheless, the pasture legacy effect persisted, with higher SOC, total N, and soil ACE protein content observed relative to CCG. For the remaining soil properties, pasture-crop rotations occupied an intermediate position between CCG and the less intensive systems (PIP and NGL), as they did not show significant differences with either CCG, PIP, or NGL, which suggests a gradual but incomplete decline in soil health benefits. This reflects a residual pasture legacy effect, although less pronounced than that reported by Cerecetto et al. (2024), where measurements conducted immediately after the pasture phase showed stronger benefits for soil health.
The persistence of elevated SOC in long pasture-crop rotations two years after pasture (LRG) compared to CCG, along with similar levels to NGL and PIP, supports findings by Cerecetto et al. (2024), who observed higher SOC under LR and NGL at 0–15 cm depth. Furthermore, long-term monitoring at this LTE corroborates the observed trends, with CC leading to a 17% reduction in SOC compared to systems with a high proportion of pastures (Rovira et al., 2020). These benefits are attributed to increased organic matter inputs through plant residues and cattle manure (Souza et al., 2008; de Moraes et al., 2014). Moreover, among SOC fractions, PoxC, a proxy of readily available C for soil microorganisms (Moebius-Clune et al., 2016), was highest under PIP and pasture-crop rotations one year after the pasture (SRS and LRS), while NGL and CCG exhibited the lowest levels. The observed lower PoxC levels in NGL and CCG may reflect limited inputs of fresh and diverse organic matter (Wooliver et al., 2022), though for different underlying reasons. In NGL, despite having high SOC, the absence of recent inputs from plant residues or amendments, typical of more intensively managed systems like pasture-crop rotations, may result in a greater proportion of recalcitrant C and a lower labile fraction (Cotrufo et al., 2013). In contrast, CCG showed the lowest SOC values, likely due to continuous biomass removal, low stubble levels, reduced crop diversity, and long-term soil degradation, all of which limit the overall buildup of SOC, including the labile pool measured by PoxC (Lal, 2004). This, in turn, negatively affected heterotrophic soil respiration, a proxy for microbial activity (Błońska et al., 2017). Unexpectedly, while NGL had low PoxC, it exhibited the highest heterotrophic soil respiration rates, suggesting an active microbial community capable of efficiently metabolizing recalcitrant organic matter. Similar findings have been reported in other studies, suggesting that agricultural practices can decrease total microbial activity (Kumar et al., 2023). In a study performed in the Argentinian Pampas, the highest microbial activity values were recorded in a pristine environment, while the lowest were observed under crop monoculture (Barbero et al., 2025). Moreover, Rubio et al. (2022) reported SOC and PoxC depletion and diminished microbial activity under CC in comparable LTEs in the Uruguayan Pampa, consistent with findings from the present study.
Soil compaction, estimated by bulk density, followed similar trends, with CCG exhibiting the highest bulk density, while PIP the lowest. Intermediate bulk density levels under pasture-crop rotations, especially SRS and LRG, suggest partial maintenance of soil porosity probably due to root effect. Yet, unlike Cerecetto et al. (2024), where significantly lower bulk density was maintained in the same LTE under pasture-crop rotations compared to CC, our findings suggest the structural benefits of pasture diminished with time, possibly due to increasing machinery traffic and decreased plant-cover time (Alvarez et al., 2014). This time-dependent decay of pasture-derived structural benefits was similarly reported by Franzluebbers et al. (2014) and Ernst et al. (2018).
Nutrient availability, particularly N, also differed among land use intensities. As reported in Cerecetto et al. (2024), total N remained higher in pasture-crop rotations and PIP than in CCG even two years after pasture, indicating long-term fertility benefits. This persistence can be attributed to N-fixing symbioses of legumes, cattle manure inputs, and root turnover (Ramirez et al., 2012; McDaniel et al., 2014; Pereyra-Goday et al., 2022). Microbial biomass and necromass can also act as reservoirs of slowly mineralizable N, contributing to long-term nutrient retention (Liang et al., 2017). Furthermore, microbial transformation of plant-derived N into microbial products may promote its stabilization within soil aggregates (Cotrufo et al., 2013), especially under systems with regular organic matter additions, such as grazed pasture-crop rotations. Elevated soil ACE protein content, a proxy of organic N available for microbial mineralization, in short and long pasture-crop rotations two years after the pasture (SRG and LRG) further supports the role of grazed pasture in enhancing N storage over the long term. However, this improvement in soil N was not reflected in soybean shoot N content, likely due to effective symbiotic N fixation (SNF) across all land uses with soybean. This can be attributed to the routine inoculation of soybean crops with Bradyrhizobium elkanii N fixing strains (Cerecetto et al., 2021b) that ensures effective SNF regardless of land use. Similar findings have been reported in other studies in South America, where inoculation consistently sustains SNF and minimizes the influence of background soil fertility on shoot N content (review by Hungria et al., 2005).
Overall, the lack of significant differences between LR and SR for most soil physicochemical properties and biological proxies suggests that pasture duration and differences in pasture plant species composition have a minor effect on these type of soil health indicators. However, when prokaryotic communities and crop performance were considered, LR and SR differed, emphasizing the impact of long-term management practices on prokaryotic assemblages and their interactions with soil properties. Although these properties did not differ significantly on their own, the observed shifts in prokaryotic communities and sorghum performance suggest that subtle changes in soil conditions may be biologically meaningful. This indicates that soil health cannot be fully captured by physicochemical and biological proxies alone, as prokaryotic dynamics, shaped by legacy effects of management, may mediate key processes that ultimately influence plant performance.
4.2 Effects of intensification and pasture legacy on bulk soil prokaryotic community diversity and composition
Land use intensification significantly shaped bulk soil prokaryotic diversity and composition, consistent with previous reports in the literature (Walkup et al., 2020; Sekaran et al., 2021; Frene et al., 2022; Williams et al., 2023; dos Santos-Goulart et al., 2024; Barbero et al., 2025). Interestingly, alpha-diversity was lowest in NGL and highest in CCG, with pasture-crop rotations in between. The dominance of the invasive plant species Cynodon dactylon in NGL led to more homogeneous vegetation, limiting root exudate diversity and reducing microbial habitat heterogeneity, hence likely resulting in a reduced prokaryotic alpha-diversity. This pattern reflects the Intermediate Disturbance Hypothesis, which posits that moderate disturbance, such as no-tillage CCG, can increase microbial alpha-diversity, while more stable conditions, such as NGL, may support lower alpha-diversity levels (Mendes et al., 2015). Despite no-tillage, CCG is the most intensive system due to high agrochemical use and machinery traffic over time. Similar trends were observed by Mendes et al. (2015) and Tomazelli et al. (2023), who found increased microbial diversity in managed systems compared to undisturbed soils. However, as noted by Bansal et al. (2024) and Barbero et al. (2025), greater microbial diversity does not necessarily reflect improved soil functionality. In low-disturbance systems, microbial diversity and functions may even be decoupled (Bansal et al., 2024; Barbero et al., 2025). Likewise, a review by Wooliver et al. (2022) highlighted that SOC responses to crop diversification are more closely linked to changes in microbial community composition than to shifts in alpha-diversity. This supports the idea that community composition and the presence of key functional groups may be more relevant indicators of soil health than alpha-diversity alone (Bender et al., 2016). Therefore, although alpha-diversity has been widely proposed as an indicator of soil health (Bender et al., 2016), the results of this study suggest that in relatively stable no-tillage systems, low alpha-diversity indices do not necessarily indicate loss of soil functions and health. In fact, NGL, PIP, and pasture-crop rotations, despite showing lower alpha-diversity than CCG, often exhibited parameters that indicated better soil health. This underscores that alpha-diversity alone is not a reliable indicator of soil health in this context and should be interpreted along with functional and physicochemical properties.
Shifts in community composition from NGL to CCG were observed, with land use intensity as one of the main drivers of bulk soil prokaryotic community structure, underscoring the profound impact of long-term management practices, including the legacy effects of grazed pasture, as reported in similar studies in the Cerrado and Pampa biomes (Frene et al., 2022; Cerecetto et al., 2024; dos Santos-Goulart et al., 2024). These shifts are related to cumulative effects of grazed pasture legacy, mediated through root exudates, rhizodepositions, plant residues, and cattle manure, that regulate microbial biomass, activity and shape microbial assemblages (Acosta-Martínez et al., 2010; Schmidt et al., 2019; Frene et al., 2022). As reviewed by Wooliver et al. (2022), crop rotation influences beta-diversity more than alpha-diversity, by driving shifts in the relative abundance of microbial taxa in response to variations in organic matter quality, root exudates, and nutrient availability due to different plant-derived inputs and management practices. These shifts often involve functionally important microbial groups, especially those involved in C cycling, without necessarily affecting overall richness. This further supports the idea that changes in prokaryotic community composition, rather than alpha-diversity alone, are more informative indicators of soil health, particularly in systems under reduced disturbance, such as no-tillage. Therefore, assessing beta-diversity may offer a more reliable perspective on prokaryotic responses to management, as it captures shifts in community structure that are closely linked to soil functions and health, beyond what alpha-diversity indices can reveal.
Furthermore, it was observed that prokaryotic communities in LR differed from CCG and closely resembled those in PIP, while SR was indistinguishable from CCG, suggesting that pasture legacy persists in LR but is lost in SR. This partially aligns with the findings of Cerecetto et al. (2024), who reported that the pasture legacy effect was strongest in SR immediately after the pasture phase but disappeared after one year. Moreover, Giongo et al. (2024) and Kaloterakis et al. (2024) demonstrated that the rotational position of crops significantly shapes microbial community composition driven by the distinct soil legacy effects of the preceding crop. In SR, the shorter pasture duration and simpler plant composition of the pasture may explain the rapid loss of legacy, while in LR, the more complex plant composition and extended pasture duration likely contributed to support more stable prokaryotic assemblages. Garland et al. (2021) and Wooliver et al. (2022) emphasize that time under cover crops and plant diversity are key drivers of microbial structure and soil functions. Therefore, strategies that promote lasting pasture phases with grazing cattle, as in LR, have a stronger impact on prokaryotic community composition.
4.3 Impacts of prokaryotic community shifts on soil health
Besides being closely linked to the intensification of the agricultural system, shifts in bulk soil prokaryotic community composition were also associated with key soil properties, such as PoxC and soil ACE protein content, as revealed by the db-RDA analysis, highlighting their functional relevance. Less intensive systems, LRS and PIP, were closely associated with higher PoxC levels, supporting the use of PoxC as a sensitive indicator of soil health in pasture-crop rotations. Also, SRG and LRG were associated with increased soil ACE protein content, likely due to the effect of legume-based pastures and strengthened by soybean inoculation with N-fixing bacteria. Recent evidence showed that legume rhizodeposition, including the release of specific metabolites such as flavonoids and coumarins, can enhance biological N fixation by stimulating both symbiotic and free-living N-fixing bacteria in diversified cropping systems (Qiao et al., 2024). In summary, the interaction between plant inputs, plant functional types (e.g., legumes vs. grasses), and the soil microbiome plays a key role in C and nutrient cycling and can drive both compositional shifts and functional changes in microbial communities (Villarino et al., 2021; Sokol et al., 2022). Overall, these results highlight the dynamic nature of bulk soil prokaryotic communities in response to land use intensity and reinforce the role of pasture in maintaining soil health, even under increasing cropping intensity. Moreover, they support the notion that prokaryotic community composition, rather than alpha-diversity alone, offers a more sensitive indicator of soil health, especially under low-disturbance systems. This distinction is crucial for interpreting prokaryotic indicators in systems where disturbance is minimized and supports a shift toward composition-based assessments of soil health.
Further insights were provided by ANCOM-BC and multiple logistic regressions, which identified unique responders associated with healthier soils in pasture-based land use intensities, such as NGL and PIP. Unclassified members of Planococcaceae (NGL), Solibacillus (NGL), Bradyrhizobium (NGL), Sphingomonas (NGL), and Subgroup 5 (PIP) showed significant positive correlations with heterotrophic soil respiration and PoxC and significant negative correlations with bulk density and available P, suggesting their potential as positive bioindicators of soil health. These key taxa are known for key functional roles, including exopolysaccharide (EPS) production, phosphate solubilization, and organic matter decomposition that contributes to the pool of labile C (PoxC) and the cycling of C and other nutrients, thereby supporting microbial biomass and respiration (Shivaji et al., 2014; Cerecetto et al., 2021b; Chaddad et al., 2024; Sorouri et al., 2024). EPS and microbial activity promote soil aggregation, enhancing soil structure and reducing compaction, thereby improving aeration, water infiltration, and root penetration (Zethof et al., 2020; Bettermann et al., 2021; de Faccio Carvalho et al., 2021b; O’Callaghan et al., 2022).
Interestingly, no specific unique responders, were detected for pasture-crop rotations, which may reflect functional redundancy, where soil health is maintained through a broader network of taxa rather than a few dominant indicators (Delgado-Baquerizo et al., 2016), or subtle differences undetected across broader comparisons. Although not identified as a unique responder based on ANCOM-BC and multiple logistic regressions, the genus Streptomyces was significantly positively correlated with heterotrophic soil respiration and had a higher relative abundance in the less intensive land uses, i.e. LR, PIP and NGL. This genus is known for degrading complex organic compounds that release C and N substrates, which can be utilized by other microorganisms (Moreno-Espíndola et al., 2018), further supporting its potential as a positive functional bio-indicator. Conversely, Candidatus Nitrososphaera was identified as a unique responder in the most intensive land use, CCG, and was associated with higher bulk density, supporting the idea that taxonomic indicators are more likely to emerge under intensity-driven microbial selection (Shade et al., 2012; Banerjee et al., 2019). It is important to note that while CCG exhibited higher alpha-diversity, this does not contradict the identification of key taxa adapted to agricultural intensification in this system. Alpha-diversity metrics do not necessarily reflect the community composition and abundance distribution patterns or functional dynamics of microbial communities. In CCG, elevated diversity may coexist with the enrichment of a few disturbance-tolerant taxa, such as Candidatus Nitrososphaera, which are favored under intensive management. These findings underscore the need to consider broader ecological interactions when evaluating soil prokaryotic communities. Land use intensification, plant diversity, and prokaryotic communities are tightly interconnected and co-regulate key soil processes (Frene et al., 2022; Cerecetto et al., 2024). Therefore, it is relevant to consider both community composition and potential functionality along with alpha-diversity metrics and soil properties when assessing soil health through soil prokaryotes.
4.4 Effects of intensification and pasture legacy on rhizosphere prokaryotic community diversity and composition
In contrast to bulk soil, crop type and inoculation with N-fixing bacteria emerged as dominant factors shaping the diversity and composition of rhizosphere prokaryotic communities. The observed differences between sorghum and soybean were primarily attributed to plant species, particularly through their influence on root exudates, other rhizodeposits and root morphology (Berg and Smalla, 2009). The impact of land use intensification was significant only in the rhizosphere of sorghum under SRS and LRS, similar to previous studies (Banerjee et al., 2019; Zhou et al., 2023; Cerecetto et al., 2024; Domeignoz-Horta et al., 2024; Martins Costa et al., 2024). The contrasting responses between SR and LR may result from their differences in pasture duration and plant species composition, which likely shaped distinct legacy effects and influenced rhizosphere microbial sensitivity to subsequent intensification. However, the pasture legacy previously detected in this LTE in the oat rhizosphere communities (Cerecetto et al., 2024) was no longer observed for soybean, likely due to the overriding effect of soybean inoculation with Bradyrhizobium elkanii. Bradyrhizobium was the dominant genus across all soybean-based land use intensities, and BLAST analysis confirmed the presence of both inoculated strains (B. elkanii U1301, U1302) in the soybean rhizosphere. Similar observations have been made in other cropping systems, where inoculation significantly shaped rhizosphere microbial communities regardless of agricultural management (Deng et al., 2019; Behr et al., 2023). Interestingly, Martins Costa et al. (2024) reported a significant pasture legacy effect on plant-growth-promoting bacteria in the soybean rhizosphere in a mixed crop-livestock system where no inoculation was applied, allowing the pasture legacy to become evident. These findings highlight that beyond plant species, both anthropogenic factors, such as inoculation, and biological factors, such as plant diversity, play central roles in structuring rhizosphere microbial communities (Zhou et al., 2023; Domeignoz-Horta et al., 2024).
4.5 The role of pasture legacy on shoot biomass and grain yield
The effect of inoculation also appeared to override the influence of both land use intensity and pasture legacy on soybean shoot biomass and shoot nutrient content. This suggests that inoculation of soybean with B. elkanii may buffer or standardize plant nutrient uptake and growth across different land use intensities. In contrast, in the absence of inoculation, as in sorghum, biomass differences reflected the underlying effects of land use intensity and pasture legacy persistence, reinforcing the idea that inoculation can modulate or mask soil-driven variability in plant responses. Global meta-analyses have shown that longer cover crop durations (Peng et al., 2024) and legume-based mixtures (Chahal and Van Eerd, 2023) enhance subsequent crop yields. These findings suggest that the longer and more legume-rich pasture phase in LR may have contributed to improved productivity through soil legacy effects. In line with this, long-term field experiments and modeling studies in Uruguay have shown that increasing the frequency and duration of grass–legume pastures in rotations improves subsequent crop yields under no-tillage (Baethgen et al., 2021).
Despite the masking effect of inoculation on shoot biomass and shoot nutrient content, pasture-based rotations still resulted in 20% higher commercial grain yields compared to CCG. This outcome may be attributed to two key factors. First, the pasture legacy improved soil physicochemical properties and functionality through the soil microbiome, enhancing conditions for crop establishment and growth. Second, it enriched beneficial rhizobacteria, potentially supporting crop performance beyond soil properties alone. The presence of bacterial taxa with multiple plant-beneficial traits under pasture-crop rotations supports this interpretation, highlighting the role of microbial legacy in sustaining crop productivity. In the present study, several isolates belonging to genera such as Bacillus, Pseudomonas, Microbacterium, and Delftia were also enriched in pasture-crop rotations according to sequencing data, and exhibited diverse plant-beneficial traits, including IAA production, ACC deaminase activity, siderophore production, phosphate solubilization, protease, and glucanase activity. The enrichment of these taxa in the rhizosphere suggests that pasture-crop rotations promote rhizosphere prokaryotic communities with high functional potential. Furthermore, by promoting root development, nutrient availability, and stress tolerance, these traits not only support plant growth but also create positive feedback loops that improve soil health through increased organic inputs and microbial activity (Rubio et al., 2022). In contrast, the Stenotrophomonas maltophilia strain linked to ASVs enriched in CCG exhibited fewer beneficial traits, indicating a less supportive prokaryotic environment for crop productivity in CCG. These patterns further reinforce the importance of legacy effects in shaping rhizosphere microbiome functionality.
As in the present study, Martins Costa et al. (2024) reported an enrichment of Pseudomonas and other taxa in the soybean rhizosphere under integrated crop-livestock systems compared to CC. Moreover, another study found that Pseudomonas enhances soybean’s resilience to abiotic stress and promotes plant growth (Zheng et al., 2024). Furthermore, Martins Costa et al. (2024) identified Bacillus as a keystone taxon in both CC and mixed crop-livestock systems. Chang et al. (2022) also found a high abundance and diversity of Bacillus species in the soybean rhizosphere under intercropping systems, suggesting its potential role in plant growth promotion. It is well documented that many Bacillus members contribute to pathogen suppression in the soybean rhizosphere (Liu et al., 2021) and to N fixation (Ding et al., 2005; Senger et al., 2022). Additionally, Microbacterium emerged as another rhizosphere key taxon associated with pasture legacy. This genus is known to exhibit a wide range of plant growth-promoting traits, such as phosphate solubilization, siderophore and IAA production, ACC deaminase activity, N fixation, biocontrol potential, and to enhance crop performance across various plant species (Barnett et al., 2006; Pereira et al., 2007; Sheng et al., 2009; Madhaiyan et al., 2010; Bhattacharyya and Jha, 2012; Lin et al., 2012; Rashid et al., 2012; Zhang et al., 2017; Freitas et al., 2019; Tsavkelova et al., 2024). In a previous study in the same LTE, Microbacterium was also identified as a key taxon linked to increased oat biomass under pasture legacy effects (Cerecetto et al., 2024). Although, in the present study, Microbacterium was not among the 25 most abundant genera in the soybean and sorghum rhizosphere based on sequencing data, it was still successfully isolated on R2A, underscoring the persistence of the pasture legacy. This highlights the value of integrating both approaches to gain a more comprehensive understanding of prokaryotic function and legacy persistence, providing insights into the role of microbiomes in sustaining soil health and crop productivity. Moreover, different strains of the same species with identical 16S rRNA gene sequences varied in the presence or expression of traits in vitro, underscoring the necessity of complementary genotypic and phenotypic analyses. The functional potential cannot be captured by 16S rRNA gene sequencing data alone, and gene presence does not guarantee expression under all conditions. Identifying key microbial taxa associated with improved crop performance is essential for developing microbiome-based agricultural strategies (Bender et al., 2016; Bano et al., 2021). In this context, Microbacterium emerges as a promising candidate due to its functional diversity and persistence across multiple crop species (oat, sorghum, soybean) and rotation phases.
5 Conclusions
Pasture-crop rotations improved certain soil properties, such as SOC, total N, and soil ACE protein content, compared to continuous cropping (CC), while for other properties, an intermediate response was observed, suggesting a partial and residual pasture legacy effect. Bulk soil prokaryotic communities were shifted by land use intensity and pasture legacy, with composition rather than diversity more strongly associated with soil functionality. Although the inoculation with B. elkanii masked the effect of land use intensity and pasture legacy on soybean rhizosphere prokaryotic communities, pasture-crop rotations still supported taxa with potential plant-beneficial traits, which may have contributed to the higher grain yields. Finally, when considering prokaryotic community composition and sorghum performance, the pasture legacy effect on soil health persisted in long pasture-crop rotation (LR), while it seemed to fade within a year in short pasture-crop rotation (SR), likely due to its shorter pasture duration and differences in plant species composition inherent to the experimental design. Together, the findings highlight that both pasture duration and plant species composition may play a role in sustaining prokaryote-mediated ecosystem functions, and reinforce the value of pasture-based rotations, especially those with longer, legume-rich pastures, in maintaining soil functionality and crop productivity under no-tillage conditions. Future studies should further disentangle the functional contributions of microbial communities to crop performance in grazed pasture-crop rotations. In particular, the recurrent detection of Microbacterium across different rotation phases highlights its potential as a key taxon for plant–bacteria interactions in this particular LTE in the Uruguayan Pampa biome. The isolated strains could provide valuable insights into their functional role in pasture-based agroecosystems.
Data availability statement
The datasets presented in this study can be found in online repositories. The names of the repository/repositories and accession number(s) can be found below: https://www.ncbi.nlm.nih.gov/, PRJNA1051053.
Author contributions
VC: Conceptualization, Data curation, Formal analysis, Investigation, Methodology, Visualization, Writing – original draft, Writing – review & editing. KS: Conceptualization, Funding acquisition, Investigation, Project administration, Supervision, Validation, Writing – review & editing. DB: Conceptualization, Investigation, Validation, Writing – review & editing. CL: Conceptualization, Funding acquisition, Investigation, Project administration, Supervision, Validation, Writing – review & editing.
Funding
The author(s) declare that financial support was received for the research and/or publication of this article. This work was supported by Instituto Nacional de Investigación Agropecuaria (INIA), Uruguay (Project INIA SA35 -Effect of agricultural management on soil microbiome-implication for plant growth and health), and by Julius Kühn Institute (JKI), Germany. The work of Victoria Cerecetto was supported by INIA, JKI, the Uruguayan Innovation and Research National Agency (ANII)-Sistema Nacional de Investigadores and by the Gisela und Hermann Stegemann-Stiftung, Braunschweig, Germany.
Acknowledgments
We acknowledge Pablo Rovira, José Terra and Alexander Bordagorry for maintenance of Palo a Pique LTE and providing information regarding the LTE management. We thank Gonzalo Vazquez, Gastón Tejera, Jorge Secco, Jonathan Machi, Peter Schlenzak, Alfredo Fernández, and Mariana Silvera for assistance during soil sampling and processing. We also thank Sabrina Butz for her technical assistance in the genomic DNA extraction of the isolates. Our sincere thanks to Claudia Barlocco for kindly providing us with the partial 16S rRNA gene sequence of the Bradyrhizobium elkanii strains (U1301, U1302) used for soybean inoculation.
Conflict of interest
The authors declare that the research was conducted in the absence of any commercial or financial relationships that could be construed as a potential conflict of interest.
Generative AI statement
The author(s) declare that no Generative AI was used in the creation of this manuscript.
Publisher’s note
All claims expressed in this article are solely those of the authors and do not necessarily represent those of their affiliated organizations, or those of the publisher, the editors and the reviewers. Any product that may be evaluated in this article, or claim that may be made by its manufacturer, is not guaranteed or endorsed by the publisher.
Supplementary material
The Supplementary Material for this article can be found online at: https://www.frontiersin.org/articles/10.3389/fbrio.2025.1582787/full#supplementary-material
References
Acosta-Martínez V., Bell C. W., Morris B. E. L., Zak J., and Allen V. G. (2010). Long-term soil microbial community and enzyme activity responses to an integrated cropping-livestock system in a semi-arid region. Agric. Ecosyst. Environ. 137, 231–240. doi: 10.1016/j.agee.2010.02.008
Alori E. T., Glick B. R., and Babalola O. O. (2017). Microbial phosphorus solubilization and its potential for use in sustainable agriculture. Front. Microbiol. 8. doi: 10.3389/fmicb.2017.00971
Alvarez C. R., Taboada M. A., Perelman S., and Morrás H. J. M. (2014). Topsoil structure in no-tilled soils in the Rolling Pampa, Argentina. Soil Res. 52, 533–542. doi: 10.1071/SR13281
Anderson M. J. (2001). A new method for non-parametric multivariate analysis of variance. Austral Ecol. 26, 32–46. doi: 10.1111/j.1442-9993.2001.01070.pp.x
Anderson M. J. (2006). Distance-based tests for homogeneity of multivariate dispersions. Biometrics 62, 245–253. doi: 10.1111/j.1541-0420.2005.00440.x
Ansari R. A., Mahmood I., Rizvi R., and Sumbul A. (2017). “Siderophores: augmentation of soil health and crop productivity,” in Probiotics in agroecosystem. Eds. Kumar V., Kumar M., Sharma S., and Prasad R. (Springer, Singapure), 291–312.
Baethgen W. E., Parton W. J., Rubio V., Kelly R. H., and Lutz S. M. (2021). Ecosystem dynamics of crop–pasture rotations in a fifty-year field experiment in southern South America: Century model and field results. Soil Sci. Soc Am. J. 85, 423–437. doi: 10.1002/saj2.20204
Bakker P. A. H. M., Pieterse C. M. J., de Jonge R., and Berendsen R. L. (2018). The soil-borne legacy. Cell 172, 1178–1180. doi: 10.1016/j.cell.2018.02.024
Banerjee S. and van der Heijden M. G. A. (2023). Soil microbiomes and one health. Nat. Rev. Microbiol. 21, 6–20. doi: 10.1038/s41579-022-00779-w
Banerjee S., Walder F., Büchi L., Meyer M., Held A. Y., Gattinger A., et al. (2019). Agricultural intensification reduces microbial network complexity and the abundance of keystone taxa in roots. ISME J. 13, 1722–1736. doi: 10.1038/s41396-019-0383-2
Bano S., Wu X., and Zhang X. (2021). Towards sustainable agriculture: rhizosphere microbiome engineering. Appl. Microbiol. Biotechnol. 105, 7141–7160. doi: 10.1007/s00253-021-11555-w
Bansal S., Gonzalez-Maldonado N., Yao E., Wong C. T. F., Adamo I., Acin-Albiac M., et al. (2024). Regenerative soil management practices no-till and sheep grazing induce significant but contrasting short-term changes in the vineyard soil microbiome. Plants People Planet. 7, 176–193. doi: 10.1002/ppp3.10575
Barbero F. M., Dominchin M. F., Verdenelli R. A., Frasier I., Restovich S. B., Campilongo Mancilla E. J., et al. (2025). Impact of land use changes on soil chemical properties, enzyme activities and microbial communities in two contrasting localities of the Argentinian Pampas. Appl. Soil Ecol. 206, 105836. doi: 10.1016/j.apsoil.2024.105836
Barnett S. J., Roget D. K., and Ryder M. H. (2006). Suppression of Rhizoctonia solani AG-8 induced disease on wheat by the interaction between Pantoea, Exiguobacterium, and Microbacteria. Soil Res. 44, 331–342. doi: 10.1071/SR05113
Behr J. H., Kampouris I. D., Babin D., Sommermann L., Francioli D., Kuhl-Nagel T., et al. (2023). Beneficial microbial consortium improves winter rye performance by modulating bacterial communities in the rhizosphere and enhancing plant nutrient acquisition. Front. Plant Sci. 14. doi: 10.3389/fpls.2023.1232288
Bender S. F., Wagg C., and van der Heijden M. G. A. (2016). An underground revolution: Biodiversity and soil ecological engineering for agricultural sustainability. Trends Ecol. Evol. 31, 440–452. doi: 10.1016/j.tree.2016.02.016
Beretta A., Bassahun D., and Musselli R. (2014). Medir el pH del suelo en reposo o agitando la mezcla suelo:agua? Agrocienc. Urug. 182, 90–94. doi: 10.31285/AGRO.18.469
Berg G., Fritze A., Roskot N., and Smalla K. (2001). Evaluation of potential biocontrol rhizobacteria from different host plants of Verticillium dahliae Kleb. J. Appl. Microbiol. 91, 963–971. doi: 10.1046/j.1365-2672.2001.01462.x
Berg G., Koberl M., Rybakova D., Müller H., Grosch R., and Smalla K. (2017). Plant microbial diversity is suggested as the key to future biocontrol and health trends. FEMS Microbiol. Ecol. 93, fix050. doi: 10.1093/femsec/fix050
Berg G. and Smalla K. (2009). Plant species and soil type cooperatively shape the structure and function of microbial communities in the rhizosphere. FEMS Microbiol. Ecol. 68, 1–13. doi: 10.1111/j.1574-6941.2009.00654.x
Bettermann A., Zethof J. H. T., Babin D., Cammeraat E. L. H., Solé-Benet A., Lázaro R., et al. (2021). Importance of microbial communities at the root-soil interface for extracellular polymeric substances and soil aggregation in semiarid grasslands. Soil Biol. Biochem. 159, 108301. doi: 10.1016/j.soilbio.2021.108301
Bhattacharyya P. N. and Jha D. K. (2012). Plant growth-promoting rhizobacteria (PGPR): emergence in agriculture. World J. Microbiol. Biotechnol. 28, 1327–1350. doi: 10.1007/s11274-011-0979-9
Błońska E., Lasota J., and Zwydak M. (2017). The relationship between soil properties, enzyme activity and land use. For. Res. Pap. 78, 39–44. doi: 10.1515/frp-2017-0004
Bolyen E., Rideout J. R., Dillon M. R., Bokulich N. A., Abnet C. C., Al-Ghalith G. A., et al. (2018). QIIME 2: reproducible, interactive, scalable, and extensible microbiome data science. Peer J. Prepr 6, e27295v2. doi: 10.7287/peerj.preprints.27295v2
Bray R. H. and Kurtz L. T. (1945). Determination of total, organic and available forms of phosphorus in soils. Soil Sci. 59, 39–45. doi: 10.1097/00010694-194501000-00006
Callahan B. J., McMurdie P. J., Rosen M. J., Han A. W., Johnson A. J. A., and Holmes S. P. (2016). DADA2: high-resolution sample inference from Illumina amplicon data. Nat. Methods 13, 581–583. doi: 10.1038/nmeth.3869
Camacho C., Coulouris G., Avagyan V., Ma N., Papadopoulos J., Bealer K., et al. (2009). BLAST+: architecture and applications. BMC Bioinform. 10, 421. doi: 10.1186/1471-2105-10-421
Carbonetto B., Rascovan N., Álvarez R., Mentaberry A., and Vázquez M. P. (2014). Structure, composition and metagenomic profile of soil microbiomes associated to agricultural land use and tillage systems in Argentine Pampas. PloS One 9, e99949. doi: 10.1371/journal.pone.0099949
Cerecetto V., Beyhaut E., Amenc L., Trives C., Altier N., and Drevon J. J. (2021b). Contrasting expression of rhizobial phytase in nodules of two soybean cultivars grown under low phosphorus availability. Front. Sustain. Food Syst. 4. doi: 10.3389/fsufs.2020.607678
Cerecetto V., Leoni C., Jurburg S. D., Kampouris I. D., Smalla K., and Babin D. (2024). Pasture-crop rotations modulate the soil and rhizosphere microbiota and preserve soil structure supporting oat cultivation in the Pampa biome. Soil Biol. Biochem. 195, 109451. doi: 10.1016/j.soilbio.2024.109451
Cerecetto V., Smalla K., Nesme J., Garaycochea S., Fresia P., Sørensen S. J., et al. (2021a). Reduced tillage, cover crops and organic amendments affect soil microbiota and improve soil health in Uruguayan vegetable farming systems. FEMS Microbiol. Ecol. 97, fiab023. doi: 10.1093/femsec/fiab023
Chaddad Z., Lamrabet M., Bennis M., Kaddouri K., Alami S., Bouhnik O., et al. (2024). “Nitrogen-fixing Bradyrhizobium spp. as plant growth-promoting bacteria to Improve soil quality and plant tolerance to biotic and abiotic stresses,” in Soil bacteria. Eds. Dheeman S., Islam M. T., Egamberdieva D., and Siddiqui M. N. (Springer, Singapore). doi: 10.1007/978-981-97-3473-3_3
Chahal I. and Van Eerd L. L. (2023). Do cover crops increase subsequent crop yield in temperate climates? A meta-analysis. Sustainability 15, 6517. doi: 10.3390/su15086517
Chang X., Wei D., Zeng Y., Zhao X., Hu Y., Wu X., et al. (2022). Maize-soybean relay strip intercropping reshapes the rhizosphere bacterial community and recruits beneficial bacteria to suppress Fusarium root rot of soybean. Front. Microbiol. 13. doi: 10.3389/fmicb.2022.1009689
Chaparro J. M., Sheflin A. M., Manter D. K., and Vivanco J. M. (2012). Manipulating the soil microbiome to increase soil health and plant fertility. Biol. Fertil. Soils. 48, 489–499. doi: 10.1007/s00374-012-0691-4
Cock P. J. A., Chilton J. M., Grüning B., Johnson J. E., and Soranzo N. (2015). NCBI BLAST+integrated into galaxy. Gigascience 4, 39. doi: 10.1186/s13742-015-0080-7
Cotrufo M. F., Wallenstein M. D., Boot C. M., Denef K., and Paul E. (2013). The Microbial Efficiency-Matrix Stabilization (MEMS) framework integrates plant litter decomposition with soil organic matter stabilization: do labile plant inputs form stable soil organic matter? Glob. Change Biol. 19, 988–995. doi: 10.1111/gcb.12113
de Faccio Carvalho P. C., de Albuquerque Nunes P. A., Pontes-Prates A., Szymczak L. S., de Souza W. F., Moojen Gomes F., et al. (2021b). Reconnecting grazing livestock to crop landscapes: reversing specialization trends to restore landscape multifunctionality. Front. Sustain. Food Syst. 5, 750765. doi: 10.3389/fsufs.2021.750765
de Faccio Carvalho P. C., Savian J. V., della Chiesa T., de Souza W. F., Terra J. A., Pinto P., et al. (2021a). Land-use intensification trends in the Rio de la Plata region of South America: towards specialization or recoupling crop and livestock production. Front. Agr. Sci. Eng. 8, 97–110. doi: 10.15302/J-FASE-2020380
Delgado-Baquerizo M., Maestre F., Reich P., Jeffries T. C., Gaitan J. J., Encinar D., et al. (2016). Microbial diversity drives multifunctionality in terrestrial ecosystems. Nat. Commun. 7, 10541. doi: 10.1038/ncomms10541
de Mendiburu F. (2020). Agricolae: statistical procedures for agricultural research. R package version 1. Available online at: https://CRAN.R-project.org/package=agricolae. (Accessed October 10, 2024).
de Moraes A., de Faccio Carvalho P. C., Anghinoni I., Campos Lustosa S. B., de Andrade Costa S. E. V. G., and Kunrath T. R. (2014). Integrated crop–livestock systems in the Brazilian subtropics. Europ. J. Agro. 57, 4–9. doi: 10.1016/j.eja.2013.10.004
Deng S., Wipf H. M. L., Pierroz G., Raab T. K., Khanna R., and Coleman-Derr D. (2019). A plant growth-promoting microbial soil amendment dynamically alters the strawberry root bacterial microbiome. Sci. Rep. 9, 17677. doi: 10.1038/s41598-019-53623-2
Ding Y., Wang J., Liu Y., and Chen S. (2005). Isolation and identification of nitrogen-fixing bacilli from plant rhizospheres in Beijing region. J. Appl. Microbiol. 99, 1271–1281. doi: 10.1111/j.1365-2672.2005.02738.x
Domeignoz-Horta L. A., Cappelli S. L., Shrestha R., Gerin S., Lohila A. K., Heinonsalo J., et al. (2024). Plant diversity drives positive microbial associations in the rhizosphere enhancing carbon use efficiency in agricultural soils. Nat. Commun. 15, 8065. doi: 10.1038/s41467-024-52449-5
dos Santos-Goulart P. F., Schulman P., Macedo R., Mendes L. W., Costa-Coelho G. R., and Rodrigues L. A. (2024). Biodiversity and community structures of fungi and bacteria in soils under a crop-livestock integration system. Appl. Soil Ecol. 202, 105565. doi: 10.1016/j.apsoil.2024.105565
Durán N., Justo G. Z., Durán M., Brocchi M., Cordi L., Tasic L., et al. (2016). Advances in Chromobacterium violaceum and properties of violacein: its main secondary metabolite: a review. Biotechnol. Adv. 34, 1030–1045. doi: 10.1016/j.bioteChadv.2016.06.003
Elsayed T. R., Grosch R., and Smalla K. (2021). Potato plant spheres and to a lesser extent the soil type influence the proportion and diversity of bacterial isolates with in vitro antagonistic activity towards Ralstonia solanacearum. FEMS Microbiol. Ecol. 97, fiab038. doi: 10.1093/femsec/fiab038
Ernst O. R., Dogliotti S., Cadenazzi M., and Kemanian A. R. (2018). Shifting crop-pasture rotations to no-till annual cropping reduces soil quality and wheat yield. Field Crops Res. 217, 180–187. doi: 10.1016/j.fcr.2017.11.014
Fierer N., Wood S. A., Bueno, and de Mesquita C. P. (2021). How microbes can, and cannot, be used to assess soil health. Soil Biol. Biochem. 153, 108111. doi: 10.1016/j.soilbio.2020.108111
Franzluebbers A. J., Sawchik J., and Taboada M. A. (2014). Agronomic and environmental impacts of pasture-crop rotations in temperate North and South America. Agric. Ecosyst. Environ. 190, 18–26. doi: 10.1016/j.agee.2013.09.017
Freitas M. A., Medeiros F. H. V., Melo I. S., Pereira P. F., Peñaflor M. F. G. V., Bento J. M. S., et al. (2019). Stem inoculation with bacterial strains Bacillus amyloliquefaciens (GB03) and Microbacterium imperiale (MAIIF2a) mitigates Fusarium root rot in cassava. Phytoparasitica 47, 135–142. doi: 10.1007/s12600-018-0706-2
French E., Kaplan I., Iyer-Pascuzzi A., Nakatsu C. H., and Enders L. (2021). Emerging strategies for precision microbiome management in diverse agroecosystems. Nature. Plants. 7, 256–267. doi: 10.1038/s41477-020-00830-9
Frene J. P., Figuerola E., Gabbarini L. A., Erijman L., and Wall L. G. (2022). Impact of diversification and intensification of crop rotation (DICR) in soil bacterial microbiota in on-farm study after four and seven years. Appl. Soil Ecol. 179, 104592. doi: 10.1016/j.apsoil.2022.104592
Garland G., Edlinger A., Banerjee S., Degrune F., García-Palacios P., Pescador D. S., et al. (2021). Crop cover is more important than rotational diversity for soil multifunctionality and cereal yields in European cropping systems. Nat. Food. 2, 28–37. doi: 10.1038/s43016-020-00210-8
Garrett R. D., Niles M. T., Gil J. D. B., Gaudin A., Chaplin-Kramer R., Assmann A., et al. (2017). Social and ecological analysis of commercial integrated crop livestock systems: current knowledge and remaining uncertainty. Agric. Syst. 155, 136–146. doi: 10.1016/j.agsy.2017.05.003
Giongo A., Arnhold J., Grunwald D., Smalla K., and Braun-Kiewnick A. (2024). Soil depths and microhabitats shape soil and root-associated bacterial and archaeal communities more than crop rotation in wheat. Front. Microb. 3. doi: 10.3389/frmbi.2024.1335791
Hartmann M. and Six J. (2023). Soil structure and microbiome functions in agroecosystems. Nat. Rev. Earth. Environ. 4, 4–18. doi: 10.1038/s43017-022-00366-w
Hermans S. M., Buckley H. L., Case B. S., Curran-Cournane F., Taylor M., and Lear G. (2020). Using soil bacterial communities to predict physico-chemical variables and soil quality. Microbiome 8, 79. doi: 10.1186/s40168-020-00858-1
Heuer H., Kopmann C., Binh C. T. T., Top E. M., and Smalla K. (2009). Spreading antibiotic resistance through spread manure: characteristics of a novel plasmid type with low %G+C content. Environ. Microbiol. 11, 937–949. doi: 10.1111/j.1462-2920.2008.01819.x
Hungria M., Franchini J. C., Campo R. J., and Graham P. H. (2005). “The importance of nitrogen fixation to soybean cropping in South America,” in Nitrogen fixation in agriculture, forestry, ecology, and the environment. Nitrogen fixation: Origins, applications, and research Progress, vol. 4 . Eds. Werner D. and Newton W. E. (Springer, Dordrecht). doi: 10.1007/1-4020-3544-6_3
Jaurena M., Durante M., Devincenzi T., Savian J. V., Bendersky D., Moojen F. G., et al. (2021). Native grasslands at the core: A new paradigm of intensification for the campos of southern South America to increase economic and environmental sustainability. Front. Sustain. Food Syst. 5. doi: 10.3389/fsufs.2021.547834
Kaloterakis N., Rashtbari M., Razavi B. S., Braun-Kiewnick A., Giongo A., Smalla K., et al. (2024). Preceding crop legacy modulates the early growth of winter wheat by influencing root growth dynamics, rhizosphere processes, and microbial interactions. Soil Biol. Biochem. 191, 109343. doi: 10.1016/j.soilbio.2024.109343
Karlen D. L., Veum K. S., Sudduth K. A., Obrycki J. F., and Nunes M. R. (2019). Soil health assessment: Past accomplishments, current activities, and future opportunities. Soil Tillage Res. 195, 104365. doi: 10.1016/j.still.2019.104365
King A. E. and Blesh J. (2018). Crop rotations for increased soil carbon: Perenniality as a guiding principle. Ecol. Appl. 28, 249–261. doi: 10.1002/eap.1648
Kolde R. (2019). pheatmap: pretty Heatmaps. R package version 1.0.12. Available online at: https://CRAN.R-project.org/package=pheatmap. (Accessed October 10, 2024).
Koo S. Y., Hong S. H., Ryu H. W., and Cho K. (2010). Plant growth-promoting trait of rhizobacteria isolated from soil contaminated with petroleum and heavy metals. J. Microbiol. Biotechnol. 20, 587–593. doi: 10.4014/jmb.0907.07017
Kumar K. A., Singh R. D., and Sahu S. K. (2023). Influence of land use on soil microbial communities of sub-Himalayas of India: insights from phospholipid fatty acid profiles, ribosomal intergenic spacer profiles, soil enzymes, and carbon pools. Agrofor. Syst. 97, 1571–1585. doi: 10.1007/s10457-023-00879-3
Lal R. (2004). Soil carbon sequestration impacts on global climate change and food security. Science 304, 1623–1627. doi: 10.1126/science.1097396
Lapsansky E. R., Milroy A. M., Andales M. J., and Vivanco J. M. (2016). Soil memory as a potential mechanism for encouraging sustainable plant health and productivity. Curr. Opin. Biotechnol. 38, 137–142. doi: 10.1016/j.copbio.2016.01.014
Lebrazi S., Niehaus K., Bednarz H., Fadil M., Chraibi M., and Fikri-Benbrahim K. (2020). Screening and optimization of indole-3-acetic acid production and phosphate solubilization by rhizobacterial strains isolated from Acacia cyanophylla root nodules and their effects on its plant growth. J. Genet. Eng. Biotechnol. 8, 71. doi: 10.1186/s43141-020-00090-2
Lehman R. M., Cambardella C. A., Stott D. E., Acosta-Martinez V., Manter D. K., Buyer J. S., et al. (2015). Understanding and enhancing soil biological health: the solution for reversing soil degradation. Sustainability 7, 988–1027. doi: 10.3390/su7010988
Lehmann J., Bossio D. A., Kögel-Knabner I., and Rillig M. C. (2020). The concept and future prospects of soil health. Nat. Rev. Earth Environ. 1, 544–553. doi: 10.1038/s43017-020-0080-8
Liang C., Schimel J., and Jastrow J. (2017). The importance of anabolism in microbial control over soil carbon storage. Nat. Microbiol. 2, 17105. doi: 10.1038/nmicrobiol.2017.105
Lienhard P., Tivet F., Chabanne A., Dequiedt S., Lelièvre M., Sayphoummie S., et al. (2013). No-till and cover crops shift soil microbial abundance and diversity in Laos tropical grasslands. Agron. Sustain. Dev. 33, 375–384. doi: 10.1007/s13593-012-0099-4
Lin H., Eggesbo M., and Peddada S. D. (2022). Linear and nonlinear correlation estimators unveil undescribed taxa interactions in microbiome data. Nat. Commun. 13, 1–16. doi: 10.1038/s41467-022-32243-x
Lin L., Guo W., Xing Y., Zhang X., Li Z., Hu C., et al. (2012). The actinobacterium Microbacterium sp. 16SH accepts pBBR1-based pPROBE vectors, forms biofilms, invades roots, and fixes N2 associated with micropropagated sugarcane plants. Appl. Microbiol. Biotechnol. 93, 1185–1195. doi: 10.1007/s00253-011-3618-3
Lin H. and Peddada S. D. (2020). Analysis of compositions of microbiomes with bias correction. Nat. Commun. 11, 3514. doi: 10.1038/s41467-020-17041-7
Liu H., Li J., Carvalhais L. C., Percy C. D., Prakash Verma J., Schenk P. M., et al. (2021). Evidence for the plant recruitment of beneficial microbes to suppress soilborne pathogens. New Phytol. 229, 2873–2885. doi: 10.1111/nph.17057
Macedo I., Roel A., Velazco J. I., Bordagorri A., Terra J. A., and Pittelkow C. M. (2022). Intensification of rice-pasture rotations with annual crops reduces the stability of sustainability across productivity, economic, and environmental indicators. Agric. Syst. 202, 103488. doi: 10.1016/j.agsy.2022.103488
Madhaiyan M., Poonguzhali S., Lee J. S., Lee K. C., Saravanan V. S., and Santhanakrishnan P. (2010). Microbacterium azadirachtae sp. nov., a plant-growth-promoting actinobacterium isolated from the rhizoplane of neem seedlings. Int. J. Syst. Evol. Microbiol. 60, 1687–1692. doi: 10.1099/ijs.0.015800-0
Martin M. (2011). Cutadapt removes adapter sequences from high-throughput sequencing reads. EMBnet J. 17, 10–12. doi: 10.14806/ej.17.1.200
Martins Costa R., Barbosa Rocha S. M., Hermelindo Ventura S., Oliveira de Aviz R., Lima Leite M. R., Antunes de Souza H., et al. (2024). Differential recruitment of plant growth-promoting bacteria community by soybean rhizosphere in no-tillage and integrated crop-livestock. Rhizosphere 31, 100948. doi: 10.1016/j.rhisph.2024.100948
McDaniel M. D., Grandy A. S., Tiemann L. K., and Weintraub M. N. (2014). Crop rotation complexity regulates the decomposition of high and low quality residues. Soil Biol. Biochem. 78, 243–254. doi: 10.1016/j.soilbio.2014.07.027
McMurdie P. J. and Holmes S. (2013). Phyloseq: an R package for reproducible interactive analysis and graphics of microbiome census data. PloS One 8, e61217. doi: 10.1371/journal.pone.0061217
Meier U. (2018). Growth Stages of Mono- and Dicotyledonous Plants. BBCH Monograph (Quedlinburg: Julius Kühn Institut). doi: 10.5073/20180906-074619
Mendes L. W., de Lima Brossi M. J., Kuramae E. E., and Tsai S. M. (2015). Land-use system shapes soil bacterial communities in Southeastern Amazon region. Appl. Soil Ecol. 95, 151–160. doi: 10.1016/j.apsoil.2015.06.005
Moebius-Clune B. N., Moebius-Clune D. J., Gugino B. K., Idowu O. J., Schindelbeck R. R., and Ristow A. J. (2016). Comprehensive Assessment of Soil Health – The Cornell Framework. Edition 3.2 (New York: Cornell University).
Moreno-Espíndola I. P., Ferrara-Guerrero M. J., Luna-Guido M. L., Ramírez-Villanueva D., De León-Lorenzana A., Gómez-Acata S., et al. (2018). The bacterial community structure and microbial activity in a traditional organic milpa farming system under different soil moisture conditions. Front. Microbiol. 9. doi: 10.3389/fmicb.2018.02737
Nautiyal C. S. (1999). An efficient microbiological growth medium for screening phosphate solubilizing microorganisms. FEMS Microbiol. Lett. 170, 265–270. doi: 10.1111/j.1574-6968.1999.tb13383.x
Nishioka T. and Tamaki H. (2022). Improved cultivation and isolation of diverse endophytic bacteria inhabiting Dendrobium roots by using simply modified agar media. Microbiol. Spectr. 10, e02238–e02222. doi: 10.1128/spectrum.02238-22
Norris C. E., Bean G. M., Cappellazzi S. B., Cope M., Greub K. L. H., Liptzin D., et al. (2020). Introducing the North American project to evaluate soil health measurements. Agron. J. 112, 3195–3215. doi: 10.1002/agj2.20234
Norris C. E. and Congreves K. A. (2018). Alternative management practices improve soil health indices in intensive vegetable cropping systems: A review. Front. Environ. Sci. 6. doi: 10.3389/fenvs.2018.00050
O’Callaghan M., Ballard R. A., and Wright D. (2022). Soil microbial inoculants for sustainable agriculture: limitations and opportunities. Soil Use Manage. 38, 1340–1369. doi: 10.1111/sum.12811
Oksanen J., Simpson G. L., Blanchet F. G., Kindt R., Legendre P., Minchin P. R., et al. (2022). Vegan: community ecology package. R package version 2. Available online at: https://CRAN.R-project.org/package=vegan. (Accessed October 10, 2024).
Orozco-Mosqueda M. C., Rocha-Granados M. C., Glick B. R., and Santoyo G. (2018). Microbiome engineering to improve biocontrol and plant growth-promoting mechanisms. Microbiol. Res. 208, 25–31. doi: 10.1016/j.micres.2018.01.005
Peng Y., Wang L., Jacinthe P. A., and Ren W. (2024). Global synthesis of cover crop impacts on main crop yield. Field Crops Res. 310, 109343. doi: 10.1016/j.fcr.2024.109343
Pereira P., Nesci A., and Etcheverry M. (2007). Effects of biocontrol agents on Fusarium verticillioides count and fumonisin content in the maize agroecosystem: impact on rhizospheric bacterial and fungal groups. Biol. Control. 42, 281–287. doi: 10.1016/j.biocontrol.2007.05.015
Pereyra-Goday F., Jebari A., Takahashi T., Rovira P., Ayala W., Lee M. R. F., et al. (2024). Carbon footprint of mixed farming crop-livestock rotational-based grazing beef systems using long term experimental data. Agron. Sustain. Dev. 44, 41. doi: 10.1007/s13593-024-00977-1
Pereyra-Goday F., Rovira P., Ayala W., and Rivero M. J. (2022). Management and productivity of key integrated crop–livestock systems in Uruguay: the Palo a Pique long-term experiment’s third phase. Agronomy 12, 3023. doi: 10.3390/agronomy12123023
Pittelkow C., Liang X., Linquist B., van Groenigen K. J., Lee J., Lundy M. E., et al. (2015). Productivity limits and potentials of the principles of conservation agriculture. Nature 517, 365–368. doi: 10.1038/nature13809
Pravia M. V., Kemanian A. R., Terra J. A., Shi Y., Macedo I., and Goslee S. (2019). Soil carbon saturation, productivity, and carbon and nitrogen cycling in crop-pasture rotations. Agric. Syst. 171, 13–22. doi: 10.1016/j.agsy.2018.11.001
Qiao M., Sun R., Wang Z., Dumack K., Xie X., Dai C., et al. (2024). Legume rhizodeposition promotes nitrogen fixation by soil microbiota under crop diversification. Nat. Commun. 15, 2924. doi: 10.1038/s41467-024-47159-x
Quast C., Pruesse E., Yilmaz P., Gerken J., Schweer T., Yarza P., et al. (2013). The SILVA ribosomal RNA gene database project: improved data processing and web-based tools. Nucleic Acids Res. 41, D590–D596. doi: 10.1093/nar/gks1219
Ramirez K. S., Craine J. M., and Fierer N. (2012). Consistent effects of nitrogen amendments on soil microbial communities and processes across biomes. Glob. Change Biol. 18, 1918–1927. doi: 10.1111/j.1365-2486.2012.02639.x
Rashid S., Charles T. C., and Glick B. R. (2012). Isolation and characterization of new plant growth-promoting bacterial endophytes. Appl. Soil Ecol. 61, 217–224. doi: 10.1016/j.apsoil.2011.09.011
Rovira P., Ayala W., Terra J., García-Préchac F., Harris P., Lee M. R. F., et al. (2020). The ‘Palo a Pique’ long-term research platform: first 25 years of a crop–livestock experiment in Uruguay. Agronomy 10, 441. doi: 10.3390/agronomy10030441
Rubio V., Sawchik J., and van Es H. (2022). Soil health benefits from sequence intensification, fertilization, and no-tillage in annual cropping systems. Soil Secur. 9, 100074. doi: 10.1016/j.soisec.2022.100074
Sadras V. O., O’Leary G. J., and Roget D. K. (2005). Crop responses to compacted soil: capture and efficiency in the use of water and radiation. Field Crops Res. 91, 131–148. doi: 10.1016/j.fcr.2004.06.011
Santos Silva L., dos Santos Laroca J. V., Prates Coelho A., Custódio Gonçalves E., Pimenta Gomes R., Pereira Pacheco L., et al. (2022). Does grass-legume intercropping change soil quality and grain yield in integrated crop-livestock systems. Appl. Soil Ecol. 170, 104257. doi: 10.1016/j.apsoil.2021.104257
Schmidt J. E., Vannette R. L., Igwe A., Blundell R., Casteel C. L., and Gaudin A. C. M. (2019). Effects of agricultural management on rhizosphere microbial structure and function in processing tomato plants. Appl. Environ. Microbiol. 85, e01064–e01019. doi: 10.1128/AEM.01064-19
Schreiter S., Ding G. C., Heuer H., Neumann G., Sandmann M., Grosch R., et al. (2014). Effect of the soil type on the microbiome in the rhizosphere of field-grown lettuce. Front. Microbiol. 5. doi: 10.3389/fmicb.2014.00144
Schwyn B. and Neilands J. B. (1987). Universal chemical assay for the detection and determination of siderophores. Anal. Biochem. 160, 47–56. doi: 10.1016/0003-2697(87)90612-9
Scottá F. and da Fonseca E. L. (2015). Multiscale trend analysis for pampa grasslands using ground data and vegetation sensor imagery. Sensors 15, 1766–17692. doi: 10.3390/s150717666
Sekaran U., Kumar S., and Gonzalez-Hernandez J. L. (2021). Integration of crop and livestock enhanced soil biochemical properties and microbial community structure. Geoderma 381, 114686. doi: 10.1016/j.geoderma.2020.114686
Senger M., Moresco E., Dalbosco M., Santin R., Inderbitzin P., and Barrocas E. N. (2022). Methods to quantify Bacillus simplex-based inoculant and its effect as a seed treatment on field-grown corn and soybean in Brazil. J. Seed Sci. 44, e202244041. doi: 10.1590/2317-1545v44263329
Shade A., Peter H., Allison S. D., Baho D., Berga M., Buergmann H., et al. (2012). Fundamentals of microbial community resistance and resilience. Front. Microbiol. 3. doi: 10.3389/fmicb.2012.00417
Sheng X. F., He L. Y., Zhou L., and Shen Y. Y. (2009). Characterization of Microbacterium sp. F10a and its role in polycyclic aromatic hydrocarbon removal in low-temperature soil. Can. J. Microbiol. 55, 529–535. doi: 10.1139/W09-005
Shivaji S., Srinivas T. N. R., and Reddy G. S. N. (2014). “The Family Planococcaceae,” in The Prokaryotes. Eds. Rosenberg E., DeLong E. F., Lory S., Stackebrandt E., and Thompson F. (Springer, Berlin, Heidelberg). doi: 10.1007/978-3-642-30120-9_351
Shrestha A. and Schikora A. (2020). AHL-priming for enhanced resistance as a tool in sustainable agriculture. FEMS Microbiol. Ecol. 96, fiaa226. doi: 10.1093/femsec/fiaa226
Sokol N. W., Slessarev E., Marschmann G. L., Nicolas A., Blazewicz S. J., Brodie E. L., et al. (2022). Life and death in the soil microbiome: how ecological processes influence biogeochemistry. Nat. Rev. Microbiol. 20, 415–430. doi: 10.1038/s41579-022-00695-z
Sorouri B., Scales N. C., Gaut B. S., and Allison S. D. (2024). Sphingomonas clade and functional distribution with simulated climate change. Microbiol. Spectr. 12, e00236–e00224. doi: 10.1128/spectrum.00236-24
Souza E. D., Costa S.E.V.G.A., Lima C. V. S., Anghinoni I., Meurer E. J., and Carvalho P. C. F. (2008). Carbono orgânico e fósforo microbiano em sistemas de integrac¸ ão agricultura-pecuária submetidos a intensidades de pastejo em plantio direto. Rev. Bras. Ciênc. Solo. 32, 1273–1282. doi: 10.1590/S0100-06832008000300035
Sundberg C., Al-Soud W. A., Larsson M., Alm E., Yekta S. S., Svensson B. H., et al. (2013). 454 pyrosequencing analyses of bacterial and archaeal richness in 21 full-scale biogas digesters. FEMS Microbiol. Ecol. 85, 612–626. doi: 10.1111/1574-6941.12148
Terra J., García-Préchac F., Salvo L., and Hernández J. (2006). Soil use intensity impacts on total and particulate soil organic matter in no-till pasture-crop rotations under direct grazing. Adv. Geoecol. 38, 233–241.
Tomazelli D., Klauberg-Filho O., Camargo Mendes S. D., Baldissera T. C., Cervo Garagorry F., Tsai S. M., et al. (2023). Pasture management intensification shifts the soil microbiome composition and ecosystem functions. Agric. Ecosyst. Environ. 346, 108355. doi: 10.1016/j.agee.2023.108355
Tsavkelova E. A., Volynchikova E. A., Potekhina N. V., Lavrov K. V., and Avtukh A. N. (2024). Auxin production and plant growth promotion by Microbacterium albopurpureum sp. nov. from the rhizoplane of leafless Chiloschista parishii Seidenf. orchid. Front. Plant Sci. 15. doi: 10.3389/fpls.2024.1360828
Tsiafouli M. A., Thebault E., Sgardelis S. P., de Ruiter P. C., van der Putten W. H., Birkhofer K., et al. (2015). Intensive agriculture reduces soil biodiversity across Europe. Glob. Change Biol. 21, 973–985. doi: 10.1111/gcb.12752
Verdenelli R. A., Dominchin M. F., Pérez-Brandan C., Rovea A., Vargas-Gil S., and Meriles J. M. (2019). Effect of long-term mineral fertilisation on soil microbial abundance, community structure and diversity in a Typic hapludoll under intensive farming systems. Ann. Appl. Biol. 175, 363–375. doi: 10.1111/aab.12546
Villarino S. H., Pinto P., Jackson R. B., and Piñeiro G. (2021). Plant rhizodeposition: a key factor for soil organic matter formation in stable fractions. Sci. Adv. 7, eabd3176. doi: 10.1126/sciadv.abd3176
Walkup J., Freedman Z., Kotcon J., and Morrissey E. M. (2020). Pasture in crop rotations influences microbial biodiversity and function reducing the potential for nitrogen loss from compost. Agric. Ecosyst. Environ. 304, 107122. doi: 10.1016/j.agee.2020.107122
Wei T. and Simko V. (2021). R package ‘corrplot’: visualization of a correlation matrix (version 0.92). Available online at: https://github.com/taiyun/corrplot. (Accessed October 10, 2024).
Weil R. R., Islam K. R., Stine M. A., Gruver J. B., and Samson-Liebig S. E. (2003). Estimating active carbon for soil quality assessment: a simplified method for laboratory and field use. Am. J. Alt. Ag. 18, 3–17. doi: 10.1079/AJAA200228
Weinert N., Meincke R., Gottwald C., Heuer H., Schloter M., Berg G., et al. (2010). Bacterial diversity on the surface of potato tubers in soil and the influence of the plant genotype. FEMS Microbiol. Ecol. 74, 114–123. doi: 10.1111/j.1574-6941.2010.00936.x
Williams A., Birt H. W. G., Raghavendra A., and Dennis P. G. (2023). Cropping system diversification influences soil microbial diversity in subtropical dryland farming systems. Microb. Ecol. 85, 1473–1484. doi: 10.1007/s00248-022-02074-w
Wooliver R., Kivlin S. N., and Jagadamma S. (2022). Links among crop diversification, microbial diversity, and soil organic carbon: mini review and case studies. Front. Microbiol. 13. doi: 10.3389/fmicb.2022.854247
Wright A. F. and Bailey J. S. (2001). Organic carbon, total carbon, and total nitrogen determinations in soils of variable calcium carbonate contents using a Leco CN-2000 dry combustion analyzer. Commun. Soil Sci. Plant Anal. 32, 3243–3258. doi: 10.1081/CSS-120001118
Zethof J. H. T., Bettermann A., Vogel C., Babin D., Cammeraat E. L. H., Solé-Benet A., et al. (2020). Prokaryotic community composition and extracellular polymeric substances affect soil microaggregation in carbonate containing semiarid grasslands. Fron. Environ. Sci. 8. doi: 10.3389/fenvs.2020.00051
Zhang B. H., Salam N., Cheng J., Li H. Q., Yang J. Y., Zha D. M., et al. (2017). Microbacterium lacusdiani sp. nov., a phosphate-solubilizing novel actinobacterium isolated from mucilaginous sheath of Microcystis. J. Antibiot. 70, 147–151. doi: 10.1038/ja.2016.125
Zheng Y., Cao X., Zhou Y., Ma S., Wang Y., Li Z., et al. (2024). Purines enrich root associated Pseudomonas and improve wild soybean growth under salt stress. Nat. Commun. 15, 3520. doi: 10.1038/s41467-024-47773-9
Keywords: soil microbiota, rhizosphere microbiota, land use intensification, pasture legacy, microbial diversity
Citation: Cerecetto V, Smalla K, Babin D and Leoni C (2025) Plant-beneficial bacteria are promoted in pasture-crop rotations in the Uruguayan Pampa, contributing to soil health and crop performance. Front. Bacteriol. 4:1582787. doi: 10.3389/fbrio.2025.1582787
Received: 24 February 2025; Accepted: 16 April 2025;
Published: 21 May 2025.
Edited by:
Anil K. H. Raghavendra, New South Wales Department of Primary Industries, AustraliaReviewed by:
Juan Frene, New Mexico State University, United StatesHenry Birt, The University of Manchester, United Kingdom
Copyright © 2025 Cerecetto, Smalla, Babin and Leoni. This is an open-access article distributed under the terms of the Creative Commons Attribution License (CC BY). The use, distribution or reproduction in other forums is permitted, provided the original author(s) and the copyright owner(s) are credited and that the original publication in this journal is cited, in accordance with accepted academic practice. No use, distribution or reproduction is permitted which does not comply with these terms.
*Correspondence: Carolina Leoni, Y2xlb25pQGluaWEub3JnLnV5; Victoria Cerecetto, dmNlcmVjZXR0b0BpbmlhLm9yZy51eQ==