- 1School of Stomatology, Dalian Medical University, Dalian, China
- 2School of Life Sciences and Technology, Harbin Institute of Technology, Harbin, China
Osteoporosis is the most common bone metabolic disease, characterized by bone mass loss and bone microstructure changes due to unbalanced bone conversion, which increases bone fragility and fracture risk. Glucocorticoids are clinically used to treat a variety of diseases, including inflammation, cancer and autoimmune diseases. However, excess glucocorticoids can cause osteoporosis. Herein we performed an integrated analysis of two glucocorticoid-related microarray datasets. The WGCNA analysis identified 3 and 4 glucocorticoid-related gene modules, respectively. Differential expression analysis revealed 1047 and 844 differentially expressed genes in the two datasets. After integrating differentially expressed glucocorticoid-related genes, we found that most of the robust differentially expressed genes were up-regulated. Through protein-protein interaction analysis, we obtained 158 glucocorticoid-related candidate genes. Enrichment analysis showed that these genes are significantly enriched in the osteoporosis related pathways. Our results provided new insights into glucocorticoid-induced osteoporosis and potential candidate markers of osteoporosis.
Introduction
Osteoporosis is the most common bone disease in the world, which is characterized by low bone mass, microstructure degeneration of bone tissue and decreased bone strength (Coughlan and Dockery, 2014). Osteoporosis is mainly divided into two categories, primary and secondary (Glaser and Kaplan, 1997). Primary osteoporosis further divided into three subtypes, postmenopausal osteoporosis, age-related osteoporosis and idiopathic osteoporosis. Secondary osteoporosis is a metabolic bone disease caused by disease or drugs, including glucocorticoid-induced osteoporosis. Excessive glucocorticoids can affect the differentiation and maturation of osteoblasts, leading to a decrease in their number and function, and can also promote the apoptosis of osteoblasts, thus further reduce bone formation (Whittier and Saag, 2016). Since the successful application of microarray technology, it has been widely used for expression profiling analysis in almost all fields of biological research (Sun et al., 2018). Due to its high-throughput characteristics, microarray technology has greatly advanced many areas of biological research by transforming the study of biology from a single gene level to the whole transcriptome-wide level (Canales et al., 2006; Cheng et al., 2019). Based on genome-wide microarray expression data, Xiao et al. (2008) studied osteoporosis-related B cells and emphasized the role of B cells in the pathogenesis of osteoporosis. Liu et al. (2005) compared gene expression in circulating monocytes from high and low bone mineral density samples based on the microarray data, revealing the role of monocytes in the pathophysiological mechanism of osteoporosis. In addition, some studies have focused on glucocorticoids and explored the mechanism by which they induce bone cell apoptosis (Lu et al., 2007; Jewell et al., 2012). However, these studies were only conducted in a limited number of single datasets one by one, and there was heterogeneity between different datasets. Therefore, more robust results will be obtained by integrative analysis of the multiple datasets.
In this study, we performed integrative analysis of two glucocorticoid-related osteosarcomas microarray datasets. Glucocorticoid-related gene modules were identified firstly. Then differential expression analysis was performed to obtain glucocorticoid-related differentially expressed genes in each dataset. In order to obtain robust results, the differentially expressed glucocorticoid-related genes of two datasets were intersected. Network analysis revealed that there were 158 robust glucocorticoid-related differentially expressed genes with interacting protein partners in protein-protein interaction network. Finally, gene function enrichment analysis showed that differentially expressed genes under glucocorticoid conditions were enriched in pathways associated with osteoporosis.
Materials and Methods
GEO Datasets
The glucocorticoid-related osteosarcoma U-2 OS bone cells microarray datasets of GSE6711 and GSE26857 were downloaded from Gene Expression Omnibus (GEO) database1 (Edgar et al., 2002), which included a total of 66 samples (48 glucocorticoid-treated and 18 untreated).
Identification of Glucocorticoid-Related Gene Modules
Glucocorticoid-related gene modules of two datasets were identified by R package WGCNA, respectively (Langfelder and Horvath, 2008). The soft power applied for gene modules identification was 7 (GSE6711) and 24 (GSE26857). Correlation coefficients between the module Eigengenes and traits were calculated using Pearson’s method. Glucocorticoid-related gene modules were defined as those with correlation coefficients greater than 0.5 (Cheng et al., 2018).
Identification of Differentially Expressed Genes (DEGs)
The DEGs of glucocorticoid-related gene modules were identified using the R package limma with a threshold of |log2FoldChange| >1 and P < 0.05. Then the genes up-regulated/down-regulated in both two datasets were considered as the robust up-regulated/down-regulated glucocorticoid-related DEGs.
The Construction of Protein-Protein Interaction (PPI) Network
For the final up- or down-regulated glucocorticoid-related DEGs, we constructed PPI network by STRING database2, respectively (von Mering et al., 2003). Then Cytoscape (V.3.7.2) was used for network visualization (Shannon et al., 2003).
Pathway Enrichment Analysis
We performed pathway enrichment analysis of DEGs included in PPI network using Enrichr3, an online enrichment analysis tool (Chen et al., 2013; Kuleshov et al., 2016). The significance threshold is P < 0.05.
Results
Identification of Glucocorticoid-Related Gene Modules
Glucocorticoid-induced osteoporosis is the most common form of secondary osteoporosis (Whittier and Saag, 2016; Coskun Benlidayi, 2018). To explore the correlation between glucocorticoid-induced gene expression disorder and osteoporosis, we collected two microarray datasets of glucocorticoid-related osteosarcoma cell lines from GEO database. The two datasets contained 66 samples, 48 of which were treated with glucocorticoids. According to the result of sample hierarchical clustering, we removed two outliers in GSE6711 (Figure 1).
In order to obtain glucocorticoid-related genes, R package WGCNA was utilized. For all gene expression profiles, a total of 29 and 16 gene modules were identified in GSE6711 (Figure 2A) and GSE26857 (Figure 2B), respectively. The correlation between gene modules and traits suggested that three and four gene modules were correlated with glucocorticoids (R > 0.5, Figures 2C,D). Of these, brown was the most correlated module, followed by magenta module (GSE6711) and red module (GSE26857). In total, 3318 (GSE6711) and 5880 (GSE26857) genes were identified as glucocorticoid-related genes.
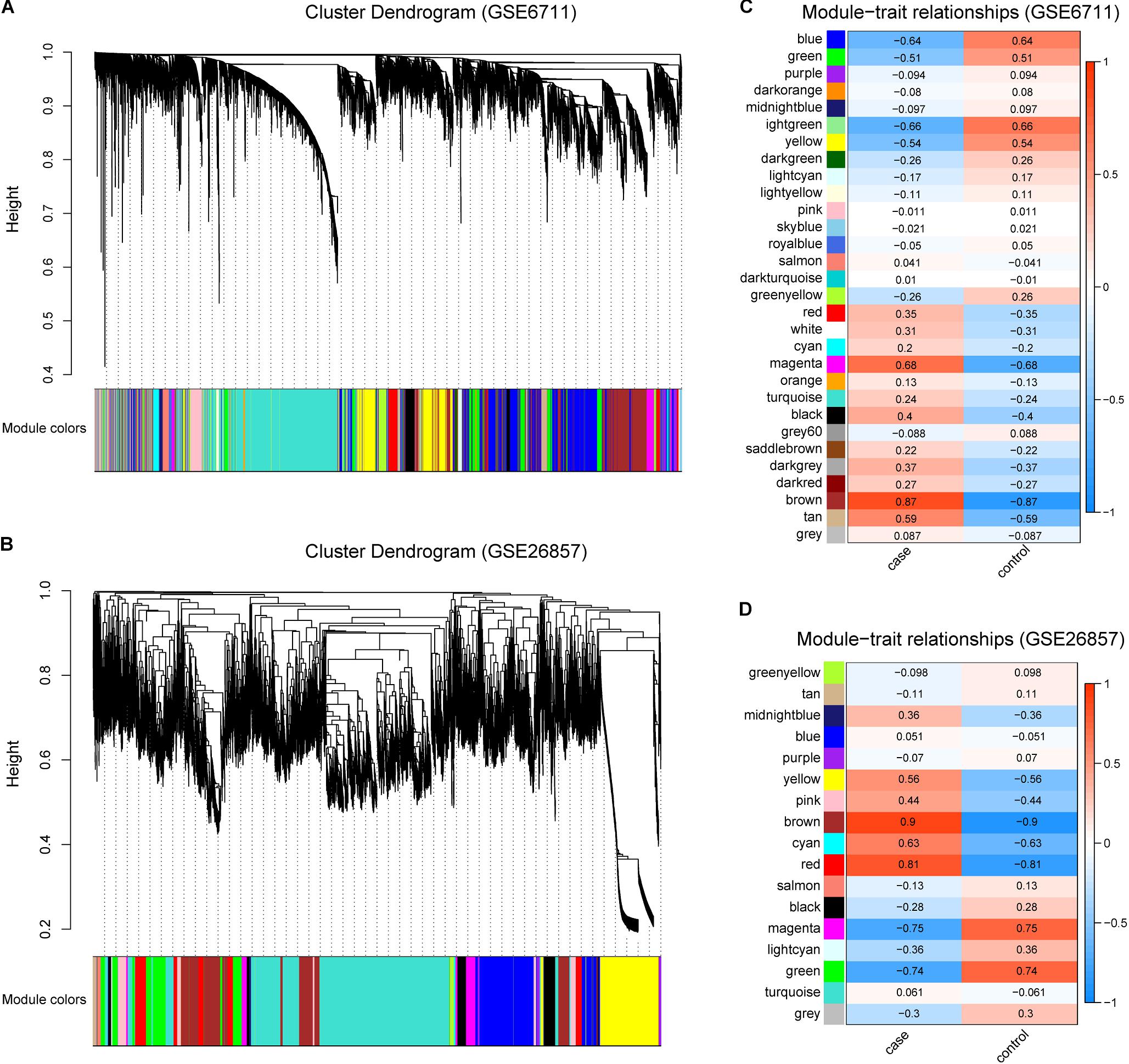
Figure 2. Identification of Glucocorticoid-related Gene Modules. (A,B) Gene modules identified by WGCNA in GSE6711 (A) and GSE26857 (B). (C,D) The correlation between gene modules and trait in GSE6711 (C) and GSE26857 (D), case represented glucocorticoid-treated and control represented untreated.
Differential Expression Analysis of Glucocorticoid-Related Genes
The occurrence of diseases is often accompanied by gene expression disorders. We further analyzed the differential expression of glucocorticoid-related genes (Figures 3A,B). In GSE6711 dataset, 31.6% (1047/3318) genes were differentially expressed, including 751 up-regulated and 296 down-regulated (Figure 3C). In GSE26857 dataset, 14.4% (844/5880) genes were differentially expressed, including 756 up-regulated and 88 down-regulated (Figure 3C). It can be found that most of glucocorticoid-related DEGs were differentially up-regulated. Furthermore, the heatmap showed that DEGs were able to group the samples by sample types, which is glucocorticoid-treated (case) and untreated (control) (Figures 3D,E).
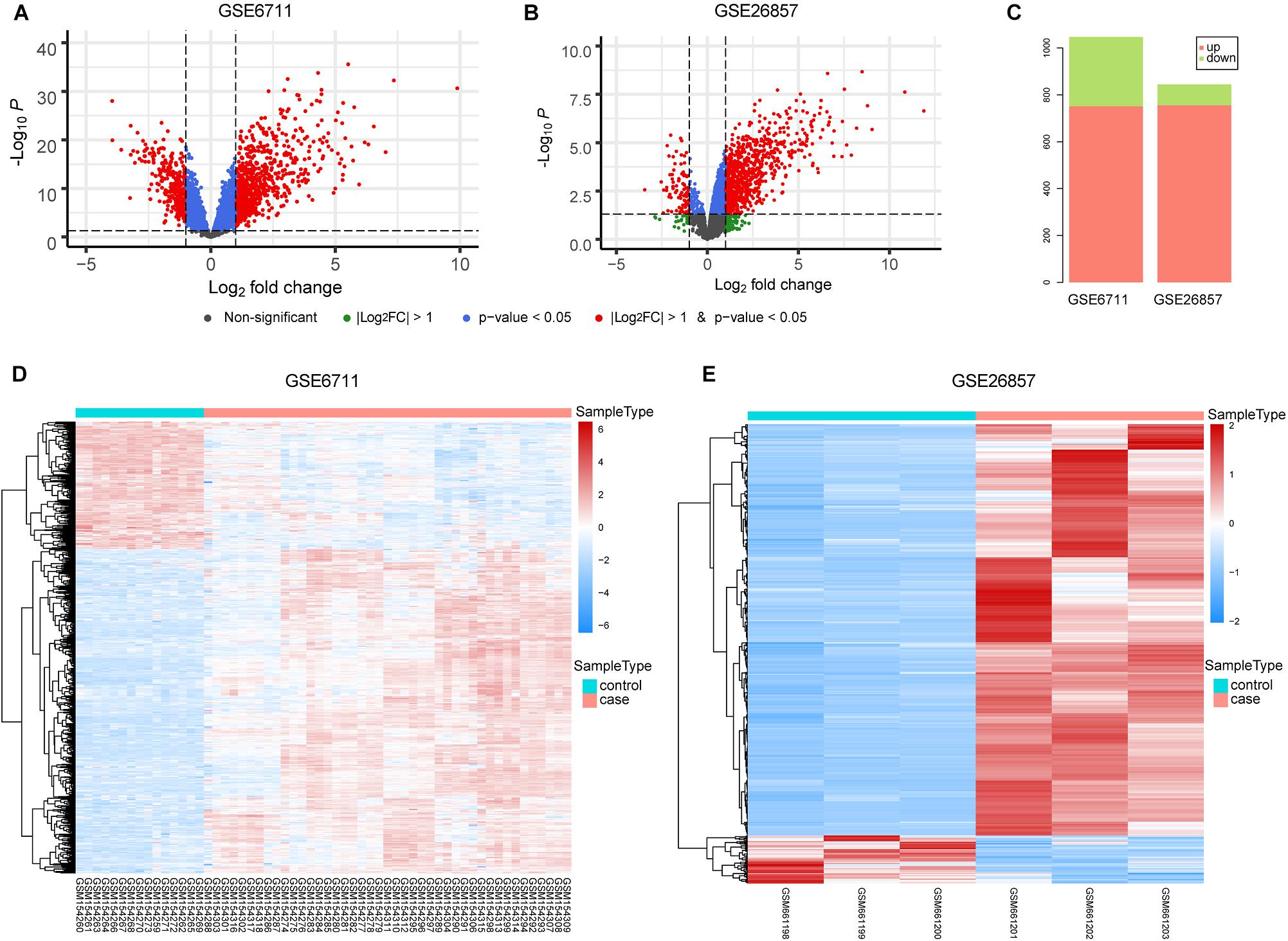
Figure 3. Differential Expression Analysis of Glucocorticoid-related Genes. (A,B) The volcano plot of glucocorticoid-related genes in GSE6711 (A) and GSE26857 (B). (C) Barplot of differentially expressed glucocorticoid-related genes. (D,E) Heatmap of differentially expressed glucocorticoid-related gene expression profile.
Integration of Glucocorticoid-Related DEGs
Due to the data heterogeneity, there are some differences in the analysis results of different datasets. Therefore, integrative analysis of different datasets can get more robust results. We integrated the common glucocorticoid-related DEGs of two datasets. A total of 243 robust glucocorticoid-related DEGs were obtained, which accounted for 23.2 and 28.8% of glucocorticoid-related DEGs in two datasets (Figure 4A). Of the robust glucocorticoid-related DEGs, 242 genes were consistent in their deregulation directions between two datasets, including 227 up-regulated and 15 down-regulated genes (Figure 4B).
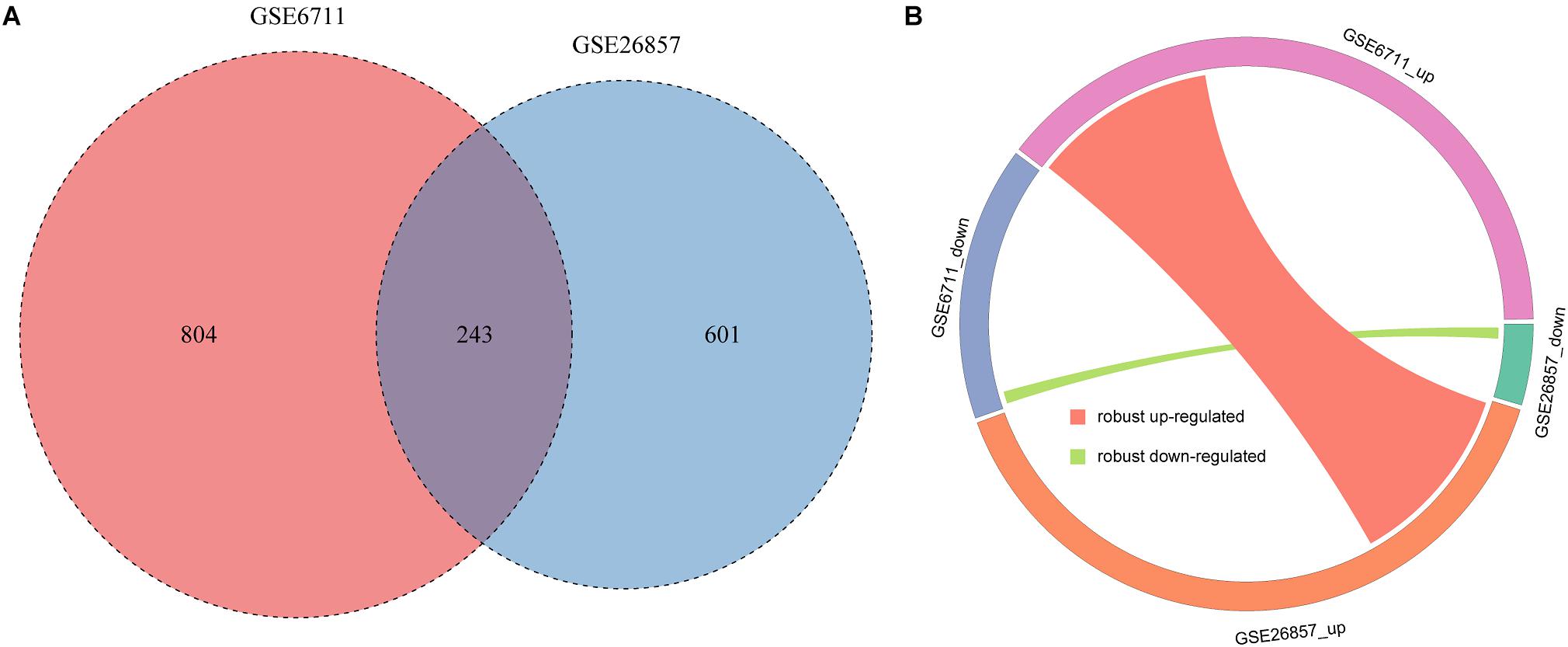
Figure 4. Integration of glucocorticoid-related DEGs. (A) The intersection of glucocorticoid-related DEGs in two datasets. (B) Circos plot of robust glucocorticoid-related DEGs.
Protein-Protein Interaction Network of Robust Glucocorticoid-Related DEGs
The robust up-regulated and down-regulated DEGs were used to construct PPI networks, respectively. The results suggested that there are 148 up-regulated and 10 down-regulated DEGs have PPI relationship (Figure 5). The degree distribution of up-regulated DEGs PPI network ranges from 1 to 10 and the top 16 hub genes which degree greater than 5 can be found in Supplementary Figure S1.
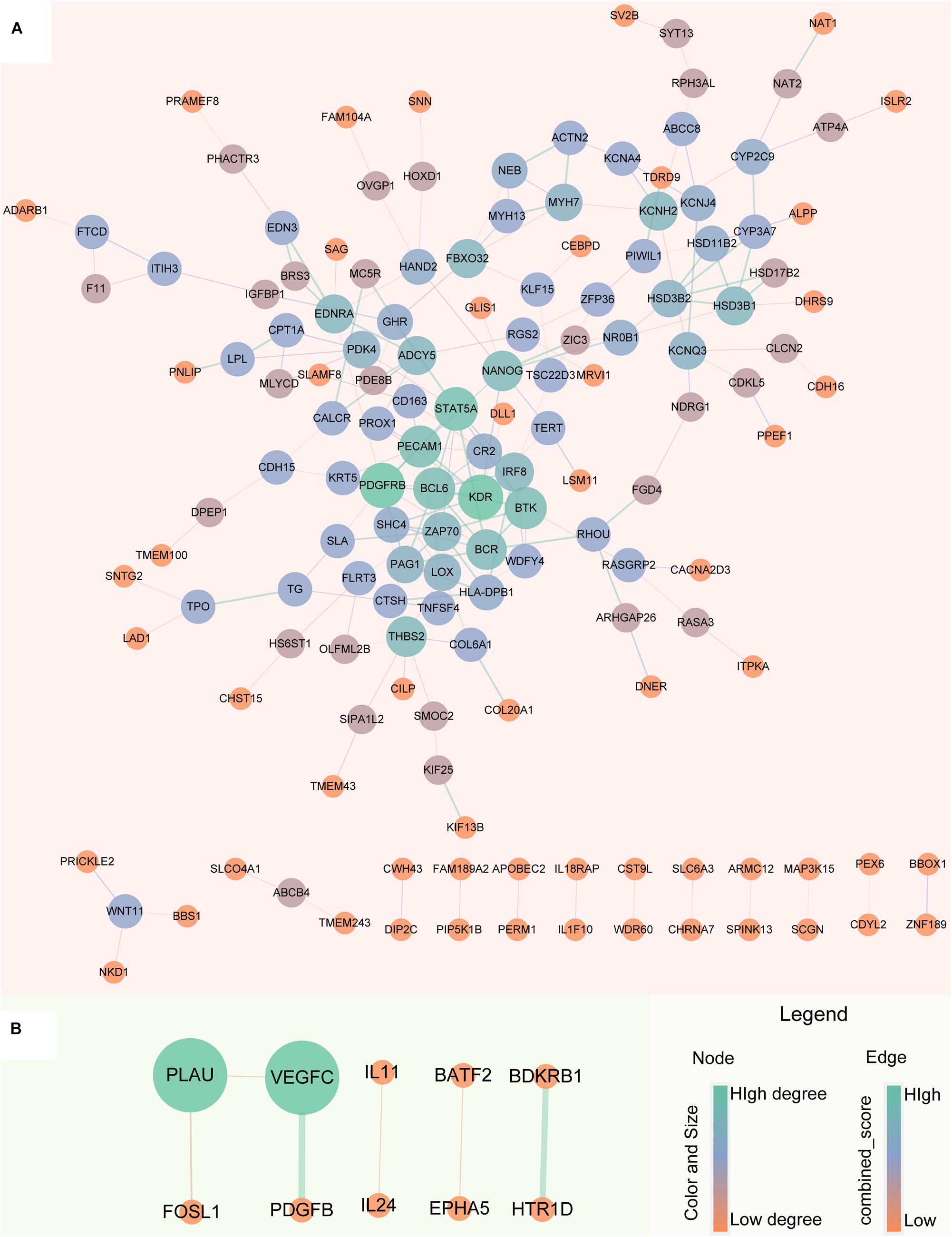
Figure 5. Protein-Protein Interaction network of robust glucocorticoid-related DEGs. (A) PPI network of robust up-regulated glucocorticoid-related DEGs. (B) PPI network of robust down-regulated glucocorticoid-related DEGs. The size and color of the node depends on the degree, the larger the degree, the larger the node. The width of the edge is determined by the combined score of STRING, the larger the score, the wider the edge.
To better understand the biological characteristics of robust glucocorticoid-related DEGs, we performed pathway enrichment analysis using up or down-regulated genes included in PPI networks by Enrichr, an online enrichment analysis tool. The up-regulated genes were significantly enriched in 30 KEGG pathways, including cushing syndrome, cortisol synthesis and secretion, Th1 and Th2 cell differentiation and Th17 cell differentiation (Figure 6A). Cushing’s syndrome is an endocrine disorder characterized by excessive cortisol secretion (Jiang et al., 2014; Pivonello et al., 2016). Many studies have shown that increased cortisol levels are associated with decreased bone mineral density (van Schoor et al., 2007; Osella et al., 2012; Toth and Grossman, 2013). The evidence for a relationship between the immune and the skeletal systems has long been recognized (Takayanagi, 2010; Yuan et al., 2010, 2011). Activated Th cells are important source of osteoclasts under inflammatory conditions (Rifas and Weitzmann, 2009). It is well known that osteoclasts are responsible for bone resorption and play an important role in osteoporosis (Detsch and Boccaccini, 2015; Kylmaoja et al., 2016; Zhang et al., 2020).
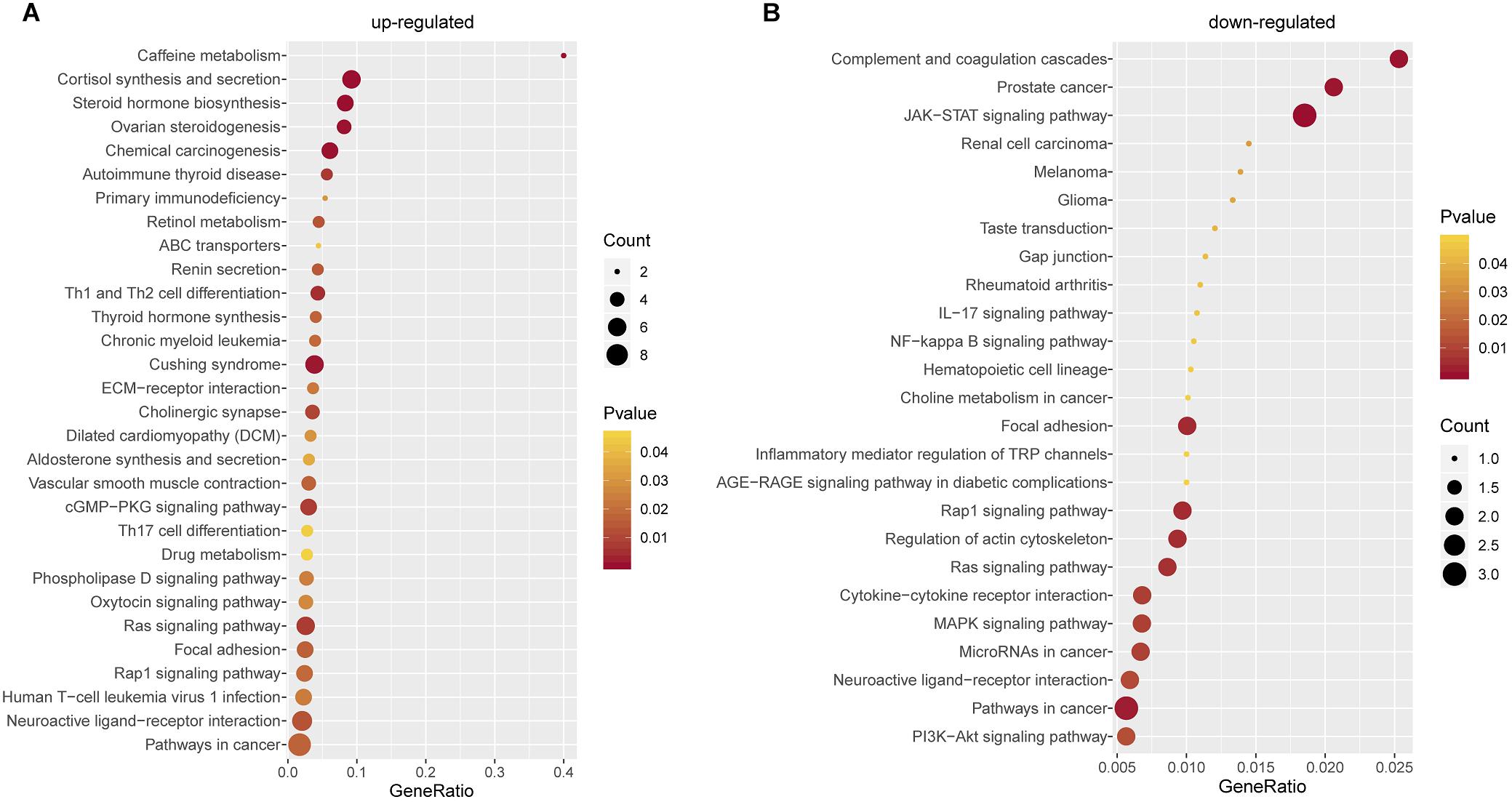
Figure 6. Pathway Enrichment Analysis. (A) Pathway enrichment analysis of up-regulated glucocorticoid-related DEGs in PPI network. (B) Pathway enrichment analysis of down-regulated glucocorticoid-related DEGs in PPI network.
There were 25 pathways significantly enriched in down-regulated glucocorticoid-related DEGs (Figure 6B). Akt is the key element in osteoblast differentiation (Sugatani and Hruska, 2005). PI3K-Akt signaling pathway is one of the significant pathways, which can inhibit osteoporosis by promoting proliferation, differentiation and osteogenesis of osteoblasts (Xi et al., 2015). In addition, down-regulated genes were also enriched in multiple cancer-related pathways, it has been found that glucocorticoids play a role in the treatment of cancer (Pufall, 2015; Hu and Chen, 2017; McNamara et al., 2018).
Discussion
In this study, we performed an integrative analysis of glucocorticoid-related microarray datasets. The WGCNA method enables the identification of glucocorticoid-related genes. Then differential expression analysis screened out the glucocorticoid-related dysregulated genes. By further integration, the robust dysregulated genes were obtained. Through protein-protein interaction network analysis of robust dysregulated genes, 158 candidate genes were obtained. And they significantly enriched the osteoporosis-related pathway.
In summary, we used microarray data to identify glucocorticoid-related dysregulated genes, which are associated with osteoporosis-related pathway. Our findings elucidate the expression mechanism of glucocorticoid-related genes and provide new guides for the diagnosis and treatment of glucocorticoid-induced osteoporosis.
Data Availability Statement
Publicly available datasets were analyzed in this study. This data can be found here: The glucocorticoid-related osteosarcoma U-2 OS bone cells microarray datasets of GSE6711 and GSE26857 were downloaded from Gene Expression Omnibus (GEO) database (https://www.ncbi.nlm.nih.gov/geo/).
Author Contributions
HL and GM designed the experiments. XJ obtained data from GEO. XY, XJ, PW, YH, HZ, XR, SC, WF, PZ, CC, and GM analyzed the data. XJ and HL wrote the manuscript. All authors read and approved the manuscript.
Funding
This work was supported by the National Natural Science Foundation of China (Grant Nos. 61871068 and 81671827).
Conflict of Interest
The authors declare that the research was conducted in the absence of any commercial or financial relationships that could be construed as a potential conflict of interest.
Supplementary Material
The Supplementary Material for this article can be found online at: https://www.frontiersin.org/articles/10.3389/fcell.2020.00252/full#supplementary-material
FIGURE S1 | The degree distribution of up-regulated DEGs PPI network.
Footnotes
References
Canales, R. D., Luo, Y., Willey, J. C., Austermiller, B., Barbacioru, C. C., Boysen, C., et al. (2006). Evaluation of DNA microarray results with quantitative gene expression platforms. Nat. Biotechnol. 24, 1115–1122. doi: 10.1038/nbt1236
Chen, E. Y., Tan, C. M., Kou, Y., Duan, Q., Wang, Z., Meirelles, G. V., et al. (2013). Enrichr: interactive and collaborative HTML5 gene list enrichment analysis tool. BMC Bioinformatics 14:128. doi: 10.1186/1471-2105-14-128
Cheng, L., Hu, Y., Sun, J., Zhou, M., and Jiang, Q. (2018). DincRNA: a comprehensive web-based bioinformatics toolkit for exploring disease associations and ncRNA function. Bioinformatics 34, 1953–1956. doi: 10.1093/bioinformatics/bty002
Cheng, L., Wang, P., Tian, R., Wang, S., Guo, Q., Luo, M., et al. (2019). LncRNA2Target v2.0: a comprehensive database for target genes of lncRNAs in human and mouse. Nucleic Acids Res. 47, D140–D144. doi: 10.1093/nar/gky1051
Coskun Benlidayi, I. (2018). Denosumab in the treatment of glucocorticoid-induced osteoporosis. Rheumatol. Int. 38, 1975–1984. doi: 10.1007/s00296-018-4106-1
Coughlan, T., and Dockery, F. (2014). Osteoporosis and fracture risk in older people. Clin. Med. 14, 187–191. doi: 10.7861/clinmedicine.14-2-187
Detsch, R., and Boccaccini, A. R. (2015). The role of osteoclasts in bone tissue engineering. J. Tissue Eng. Regen. Med. 9, 1133–1149. doi: 10.1002/term.1851
Edgar, R., Domrachev, M., and Lash, A. E. (2002). Gene expression omnibus: NCBI gene expression and hybridization array data repository. Nucleic Acids Res. 30, 207–210. doi: 10.1093/nar/30.1.207
Glaser, D. L., and Kaplan, F. S. (1997). Osteoporosis. definition and clinical presentation. Spine 22(24 Suppl.), 12S–16S. doi: 10.1097/00007632-199712151-00003
Hu, J., and Chen, Q. (2017). The role of glucocorticoid receptor in prostate cancer progression: from bench to bedside. Int. Urol. Nephrol. 49, 369–380. doi: 10.1007/s11255-016-1476-8
Jewell, C. M., Scoltock, A. B., Hamel, B. L., Yudt, M. R., and Cidlowski, J. A. (2012). Complex human glucocorticoid receptor dim mutations define glucocorticoid induced apoptotic resistance in bone cells. Mol. Endocrinol. 26, 244–256. doi: 10.1210/me.2011-1116
Jiang, Q., Wang, J., Wang, Y., Ma, R., Wu, X., and Li, Y. (2014). TF2LncRNA: identifying common transcription factors for a list of lncRNA genes from ChIP-Seq data. Biomed Res. Int. 2014:317642. doi: 10.1155/2014/317642
Kuleshov, M. V., Jones, M. R., Rouillard, A. D., Fernandez, N. F., Duan, Q., Wang, Z., et al. (2016). Enrichr: a comprehensive gene set enrichment analysis web server 2016 update. Nucleic Acids Res. 44, W90–W97. doi: 10.1093/nar/gkw377
Kylmaoja, E., Nakamura, M., and Tuukkanen, J. (2016). Osteoclasts and remodeling based bone formation. Curr. Stem Cell Res. Ther. 11, 626–633. doi: 10.2174/1574888x10666151019115724
Langfelder, P., and Horvath, S. (2008). WGCNA: an R package for weighted correlation network analysis. BMC Bioinformatics 9:559. doi: 10.1186/1471-2105-9-559
Liu, Y. Z., Dvornyk, V., Lu, Y., Shen, H., Lappe, J. M., Recker, R. R., et al. (2005). A novel pathophysiological mechanism for osteoporosis suggested by an in vivo gene expression study of circulating monocytes. J. Biol. Chem. 280, 29011–29016. doi: 10.1074/jbc.M501164200
Lu, N. Z., Collins, J. B., Grissom, S. F., and Cidlowski, J. A. (2007). Selective regulation of bone cell apoptosis by translational isoforms of the glucocorticoid receptor. Mol. Cell Biol. 27, 7143–7160. doi: 10.1128/MCB.00253-07
McNamara, K. M., Kannai, A., and Sasano, H. (2018). Possible roles for glucocorticoid signalling in breast cancer. Mol. Cell Endocrinol. 466, 38–50. doi: 10.1016/j.mce.2017.07.004
Osella, G., Ventura, M., Ardito, A., Allasino, B., Termine, A., Saba, L., et al. (2012). Cortisol secretion, bone health, and bone loss: a cross-sectional and prospective study in normal non-osteoporotic women in the early postmenopausal period. Eur. J. Endocrinol. 166, 855–860. doi: 10.1530/EJE-11-0957
Pivonello, R., Isidori, A. M., De Martino, M. C., Newell-Price, J., Biller, B. M., and Colao, A. (2016). Complications of Cushing’s syndrome: state of the art. Lancet Diabetes Endocrinol. 4, 611–629. doi: 10.1016/S2213-8587(16)00086-3
Pufall, M. A. (2015). Glucocorticoids and Cancer. Adv. Exp. Med. Biol. 872, 315–333. doi: 10.1007/978-1-4939-2895-8_14
Rifas, L., and Weitzmann, M. N. (2009). A novel T cell cytokine, secreted osteoclastogenic factor of activated T cells, induces osteoclast formation in a RANKL-independent manner. Arthritis Rheum 60, 3324–3335. doi: 10.1002/art.24877
Shannon, P., Markiel, A., Ozier, O., Baliga, N. S., Wang, J. T., Ramage, D., et al. (2003). Cytoscape: a software environment for integrated models of biomolecular interaction networks. Genome Res. 13, 2498–2504. doi: 10.1101/gr.1239303
Sugatani, T., and Hruska, K. A. (2005). Akt1/Akt2 and mammalian target of rapamycin/Bim play critical roles in osteoclast differentiation and survival, respectively, whereas Akt is dispensable for cell survival in isolated osteoclast precursors. J. Biol. Chem. 280, 3583–3589. doi: 10.1074/jbc.M410480200
Sun, M. A., Shao, X., and Wang, Y. (2018). Microarray data analysis for transcriptome profiling. Methods Mol. Biol. 1751, 17–33. doi: 10.1007/978-1-4939-7710-9_2
Takayanagi, H. (2010). New immune connections in osteoclast formation. Ann. N.Y. Acad. Sci. 1192, 117–123. doi: 10.1111/j.1749-6632.2009.05303.x
Toth, M., and Grossman, A. (2013). Glucocorticoid-induced osteoporosis: lessons from Cushing’s syndrome. Clin. Endocrinol. 79, 1–11. doi: 10.1111/cen.12189
van Schoor, N. M., Dennison, E., Lips, P., Uitterlinden, A. G., and Cooper, C. (2007). Serum fasting cortisol in relation to bone, and the role of genetic variations in the glucocorticoid receptor. Clin. Endocrinol. 67, 871–878. doi: 10.1111/j.1365-2265.2007.02978.x
von Mering, C., Huynen, M., Jaeggi, D., Schmidt, S., Bork, P., and Snel, B. (2003). STRING: a database of predicted functional associations between proteins. Nucleic Acids Res. 31, 258–261. doi: 10.1093/nar/gkg034
Whittier, X., and Saag, K. G. (2016). Glucocorticoid-induced osteoporosis. Rheum Dis. Clin. North Am. 42, 177–189. doi: 10.1016/j.rdc.2015.08.005
Xi, J. C., Zang, H. Y., Guo, L. X., Xue, H. B., Liu, X. D., Bai, Y. B., et al. (2015). The PI3K/AKT cell signaling pathway is involved in regulation of osteoporosis. J. Recept. Signal Transduct. Res. 35, 640–645. doi: 10.3109/10799893.2015.1041647
Xiao, P., Chen, Y., Jiang, H., Liu, Y. Z., Pan, F., Yang, T. L., et al. (2008). In vivo genome-wide expression study on human circulating B cells suggests a novel ESR1 and MAPK3 network for postmenopausal osteoporosis. J. Bone Miner. Res. 23, 644–654. doi: 10.1359/jbmr.080105
Yuan, F. L., Hu, W., Lu, W. G., Li, X., Li, J. P., Xu, R. S., et al. (2011). Targeting interleukin-21 in rheumatoid arthritis. Mol. Biol. Rep. 38, 1717–1721. doi: 10.1007/s11033-010-0285-x
Yuan, F. L., Li, X., Lu, W. G., Xu, R. S., Zhao, Y. Q., Li, C. W., et al. (2010). Regulatory T cells as a potent target for controlling bone loss. Biochem. Biophys. Res. Commun. 402, 173–176. doi: 10.1016/j.bbrc.2010.09.120
Keywords: osteoporosis, glucocorticoid, microarray, protein-protein interaction, enrichment analysis
Citation: Ying X, Jin X, Wang P, He Y, Zhang H, Ren X, Chai S, Fu W, Zhao P, Chen C, Ma G and Liu H (2020) Integrative Analysis for Elucidating Transcriptomics Landscapes of Glucocorticoid-Induced Osteoporosis. Front. Cell Dev. Biol. 8:252. doi: 10.3389/fcell.2020.00252
Received: 07 February 2020; Accepted: 25 March 2020;
Published: 16 April 2020.
Edited by:
Yongchun Zuo, Inner Mongolia University, ChinaReviewed by:
Liang Yu, Xidian University, ChinaYanshuo Chu, The University of Texas MD Anderson Cancer Center, United States
Copyright © 2020 Ying, Jin, Wang, He, Zhang, Ren, Chai, Fu, Zhao, Chen, Ma and Liu. This is an open-access article distributed under the terms of the Creative Commons Attribution License (CC BY). The use, distribution or reproduction in other forums is permitted, provided the original author(s) and the copyright owner(s) are credited and that the original publication in this journal is cited, in accordance with accepted academic practice. No use, distribution or reproduction is permitted which does not comply with these terms.
*Correspondence: Guowu Ma, TWd3NjQwMjRAMTYzLmNvbQ==; Huiying Liu, bGh5MDQ1MTIwMDBAZG11LmVkdS5jbg==
†These authors have contributed equally to this work