A Pyroptosis-Related Gene Panel in Prognosis Prediction and Immune Microenvironment of Human Endometrial Cancer
- Department of Obstetrics and Gynecology, Shengjing Hospital of China Medical University, Shenyang, China
As the second common diagnosed cancer among gynecological tumors, endometrial cancer (EC) has heterogeneous pathogenesis and clinical manifestations. Therefore, prognosis prediction that considers gene expression value and clinical characteristics, is helpful to patients with EC. We downloaded RNA expression and clinical data from the TCGA database. We achieved 4 DEPRGs and constructed the PRG panel by univariate, lasso and multivariate Cox analysis. Based on the median value of the risk score, patients were divided into two groups. The Kaplan–Meier curve suggested that the patients with lower risk scores had better clinical outcomes of EC. AUC of ROC curves suggested the panel can be used as an independent predictor. Future analysis indicated the positive correlations between risk score and clinical characteristics. What’s more, we performed GO and KEGG functional analysis and immune environment exploration to get an understanding of the potential molecular mechanism and immunotherapeutic target. To future validate the panel, we found that the relapse-free and overall survival probability of 4 prognostic DEPRGs between high-expression group and low-expression group were different through the Kaplan–Meier plotter in UCEC. In addition, GEPIA database and RT-PCR experiment indicated GPX4 and GSDMD were highly expressed in UCEC compared to normal endometrial tissue, and TIRAP and ELANE were downregulated. This study identified a PRG panel to predict the prognosis immune microenvironment in human EC. Then, Kaplan–Meier analysis and AUC below the ROC curves was used to validate the panel. In addition, Chi-square was used to show the clinical significance. GO, KEGG and GSEA were used to show the functional differences. Different immune-related databases were used to analyze the immune characteristics. The Kaplan–Meier plotter website was used to assess the effect of genes on survival. GEPIA and RT-PCR were used to analyze the expression level. In summary, we identified 4 prognosis-associated pyroptosis-related genes (ELANE, GPX4, GSDMD, and TIRAP). The panel can also predict prognosis prediction and immune microenvironment in human endometrial cancer.
Introduction
There were 417267 new cases and 97370 new cancer deaths reported in 2020, endometrial cancer (EC) was ranked in the top 10 most common cancers in female patients across the world, and is the second most commonly diagnosed cancer among gynecological tumors (Sung et al., 2021). Most cases are diagnosed in the late stage of life, but there are increasing numbers being diagnosed at an early stage, meaning the onset of EC is younger (Amant et al., 2005; Moore and Brewer, 2017). Though the mortality is relatively lower than other gynecological tumors, the pathogenesis and clinical manifestations of EC are heterogeneous (Gupta, 2017). Therefore, prognosis prediction considering the gene expression value and clinical characteristics is helpful for patients with EC.
Pyroptosis mediated by gasdermin is a kind of programmed cell death, which is characterized by cell swelling until cell membrane rupture, leading to the release of cell contents and then activating a strong inflammatory response (Bergsbaken et al., 2009; He et al., 2015; Vande Walle and Lamkanfi, 2016; Kovacs and Miao, 2017; Man et al., 2017; Shi et al., 2017). For morphology, pyroptosis cells showed swelling under a light microscope, and there were many bubble-like protrusions. Compared with necrotic cells, the swelling degree of focal death is lower. Under the electron microscope, it can be seen that before the rupture of the membrane, the pyroptosis cells form a large number of vesicles, namely focal apoptotic bodies. The cell membrane then forms pores and breaks, meaning the content flows out. The biochemical characteristics of pyroptosis involve the formation of inflammatory bodies, the activation of caspase and gasdermin, and the release of a large number of inflammatory factors. Pyroptosis is also an important innate immune response and plays an important role in the fight against infection (Bergsbaken et al., 2009; Man et al., 2017; Robinson et al., 2019). Pyroptosis has been reported to be involved in different processes in different diseases, including cardiovascular diseases (Jia et al., 2019; Zhaolin et al., 2019), Parkinson’s disease (Wang S. et al., 2019), Alzheimer’s disease (Han et al., 2020), diabetic kidney disease (Wang Y. et al., 2019; Lin et al., 2020), inflammatory bowel disease (Chen et al., 2019), cancers (Pizato et al., 2018; Cui et al., 2019; Qiao et al., 2019; Yu et al., 2019; Zhang C. C. et al., 2019; Zhang T. et al., 2019; Teng et al., 2020), and so on.
Recently, many reports have outlined that pyroptosis-related genes and processes play an important role in cancers. For breast cancer, DRD2 which promotes macrophage M1 polarization, triggers gasdermin E (GSDME) and induces pyroptosis, which is then downregulated (Tan et al., 2021), and through increased levels of caspase-1, caspase-3, gasdermin D and E (GSDMD and GSDME), docosahexaenoic acid (DHA) and tetraarsenic hexoxide could promote pyroptosis and thus inhibit breast cancer progress (Pizato et al., 2018; An et al., 2021; Li, 2021). For colorectal cancer, lobaplatin (Yu et al., 2019), liver X receptors (LXPs) (Derangère et al., 2014), and arsenic trioxide (ATO) combined with ascorbic acid (AA) (Tian et al., 2020) could be used to better treat colorectal cancers through inducing pyroptosis. For hepatocellular carcinoma, Hage et al. (2019) reported that sorafenib could induce the pyroptosis of macrophages which were the key mediators of antitumor effect. Zhang X. et al. (2020) reported that miltirone, a phenanthraquinone derivative isolated from Radix Salviae Miltiorrhizae, could inhibit hepatocellular carcinoma cells proliferation and induce the proteolysis of GSDME and cleavage of caspase 3. Furthermore, gasdermin also attaches importance to gastric, lung, skin, and esophageal squamous cancer (Xia et al., 2019). Yang et al. (2020) have reported that NLRP3, caspase-1, and GSDMD were upregulated in EC, and activation in pyroptosis could lead to an anti-tumor effect. Since pyroptosis plays such an important role in cancers and to date there have been few studies exploring its role in EC, combined analysis of pyroptosis and EC is imminent.
This study used TCGA, a large-scale open database, for analyzing pyroptosis-related genes (PRGs) in EC and constructed a novel PRG panel to predict the overall survival and immune microenvironment in EC. This research confirmed that the panel can be used to predict the clinical outcomes of EC independently and could eventually instruct immunotherapy of EC in the future.
Materials and Methods
The process of data analysis is shown in the flow chart in Figure 1.
Data Acquisition
The RNA expression and clinical data of UCEC patients were downloaded from the TCGA website on April 2, 20211. After removing the cases with incomplete clinical information, 526 cases were remained, as shown in Table 1. The “limma” R package was used to normalize the RNA expression profiles. A total of 34 pyroptosis-related genes were achieved through literature mining and are provided in Table 2 (Man and Kanneganti, 2015; Wang and Yin, 2017; Karki and Kanneganti, 2019; Xia et al., 2019; Ye et al., 2021).
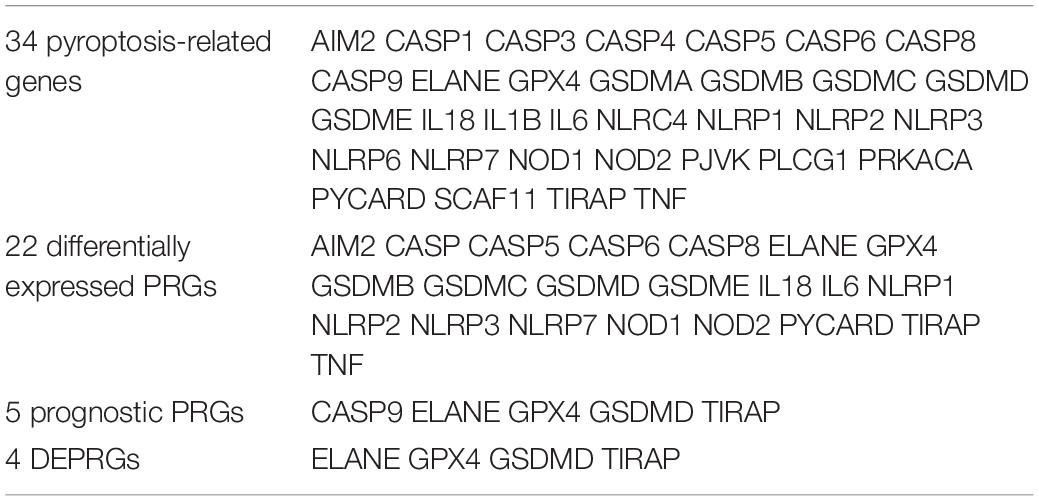
Table 2. 34 pyroptosis-related genes, 22 differentially expressed PRGs, 5 prognostic PRGs, and 4 DEPRGs.
Identification of Prognostic Differentially Expressed Pyroptosis-Related Genes
Differential expression genes (DEGs) were searched for with the “limma” R package by comparing tumor tissues with normal tissues. We screened DEGs by a Wilcoxon signed-rank test (false discovery rate, FDR < 0.05). The intersection of DEGs and pyroptosis-related genes (PRGs) was considered as significant differentially expressed PRGs (DEPRGs). We conducted a univariate Cox analysis of overall survival (OS) to screen prognostic DEPRGs. Benjamini and Hochberg (BH) correction was used to adjust p values. The expression condition of these prognostic DEPRGs were shown with the “pheatmap” R package (1.0.12).
Construction and Confirmation of a Prognostic Panel by Differentially Expressed Pyroptosis-Related Genes
The “glmnet” R package was used for constructing the DEPRGs panel by lasso regression analysis, and 10-fold cross confirmation and a P value of less than 0.05 were used to simplify and rectify the model (Liang et al., 2020). The gene expression levels should be corrected by demographics in multivariate regression models. At the same time, the risk score of patients was generated [risk score = Σ(expression value of each gene × and its coefficient)]. We used the median risk score to divide patients into two groups and visualized the results with the “Rtsne” R package. We then used the “stats” R package to conduct a principal components analysis (PCA) to study the different gene expression patterns of samples.
To validate this panel, we conducted a Kaplan-Meier analysis to show the clinical outcome differences between two groups with or without other clinical characteristics and visualized the survival curve using the “survive” and “survminer” R packages. The 1-, 2-, 3-, 4-, and 5-year ROC curves and the ROC curves compared with panels were drawn to predict the survival status with the “survivalROC” R packages. Univariate and multivariate Cox regression analyses then showed whether our panel could predict the prognosis of endometrial cancer independently.
A Chi-square test was then used to analyze the relationship between the panel and clinicopathological characteristics. These were shown in a strip chart and labeled as follows: p < 0.001 = ∗∗∗, p < 0.01 = ∗∗, and p < 0.05 = ∗ by “ComplexHeatmap” R package. Wilcoxon signed-rank test compared the risk scores among different groups of these clinicopathological characteristics. The scatter diagram showed the analysis results.
We then conducted operating characteristic curve (ROC) and decision curve analysis (DCA) to assess the sensitivity and specificity of the prognostic panel for UCEC in comparison to other clinicopathological factors (Vickers and Elkin, 2006). A nomogram was constructed, integrating the prognostic panel, for prediction of 1, 3, and 5-year OS of UCEC patients.
Gene Ontology, Kyoto Encyclopedia of Genes and Genomes Functional Enrichment, and Gene Set Enrichment Analysis Analysis Between High-Risk Versus Low-Risk Groups
Gene ontology (GO) and Kyoto Encyclopedia of Genes and Genomes (KEGG) pathway enrichment analyses predicted the potential functions of DEPRGs using the “clusterProfiler” R package. We then sorted and presented the top items of GO and KEGG pathways based on P-value < 0.05 through the statistical software R (version 4.0.2) and visualized the results in barplots using the “ggplot2” R package. Gene Set Enrichment Analysis (GSEA) software (Version 4.0.3)2 was also used to explore the potential biological function difference between the two groups. GSEA (version 3.0) was run for the “c2.cp.kegg.v.7.2.symbols.gmt” gene sets. The number of permutations was set to 1,000 and the phenotype labels were high-risk and low risk. FDR < 0.25 and NOM P < 0.05 indicated statistical significance.
Immune Characteristics Analysis
Using TIMER, CIBERSORT, CIBERSORT-ABS, QUANTISEQ, MCPcounter, XCELL, and EPIC, we analyzed the correlation between the riskScore and immune-cell characteristics in UCEC patients of TCGA database by Spearman correlation analysis, and the results were visualized in a diagram. A p value < 0.05 was considered as statistically significant.
The “gsva” R package compared normalized gene expression data with the gene sets that had common biological functions, chromosomal localization, and physiological regulation in ssGSEA (Subramanian et al., 2005). The infiltrating score of 16 types of immune cells and the activity of 13 immune-related pathways were concluded. To study the expression value of immune checkpoint-related genes in two groups, we performed a “ggstatsplot” R package and violin plot visualization.
Survival Analysis of Four Prognostic Differentially Expressed Pyroptosis-Related Genes
The Kaplan–Meier plotter3 could assess the effect of 54,675 genes on survival using 18,674 cancer samples (Győrffy et al., 2013). These cover 543 UCEC cancer patients with relapse-free and overall survival information (Nagy et al., 2021). Our study analyzed the relapse-free and overall survival of four prognostic DEPRGs through the Kaplan–Meier plotter in UCEC. Patients were classified into two groups according to the median of each prognostic DEPRG expression in the Kaplan–Meier plotter for relapse-free and overall survival. This classification method could show the survival probability differences between the high-expression group and the low-expression group.
Gene Expression Profiling Interactive Analysis Database Analysis of Four Prognostic Differentially Expressed Pyroptosis-Related Genes
GEPIA is an online database that facilitates the standardized analysis of a tremendous amount of RNA sequencing data in the TCGA and GTEx data sets(Tang et al., 2017)4. The expression level of 4 prognostic DEPRGs was validated in GEPIA (Gene Expression Profiling Interactive Analysis) based on TCGA data [num(T) = 174; num(N) = 13].
Ethics Statement
This study was carried out in accordance with the standards of the Helsinki Declaration of the World Medical Association and approved by the Ethics Committee of China Medical University. All clinical samples were collected from the Shengjing Hospital of China Medical University, with informed consent from all patients.
Tissue Collection
There were 56 clinical samples used in this study, including 33 cases of primary UCEC tissue and 23 cases of normal endometrial tissue. All samples were collected from the patients undergoing surgical excision at the Department of Obstetrics and Gynecology, Shengjing Hospital of China Medical University. No patient received radiotherapy, chemotherapy, or hormone therapy before surgery. The histopathological diagnosis was obtained from the Pathology Department according to the criteria of the World Health Organization.
Total RNA Extraction and Quantitative Real-Time RT-PCR
RNA isolation of endometrial tissue samples was conducted through TRIzol Reagent (Invitrogen, United States). The synthesis of complementary DNA (cDNA) was conducted using PrimeScriptTM RT reagent Kit with gDNA Eraser (Takara), through reverse transcription reaction. Quantitative polymerase chain reactions for TIRAP, ELANE, GPX4, GSDMD, and GAPDH were conducted in a volume of 20 μL using SYBR Premix Ex Taq (Takara) in the ABI 7500 Fast (Life Technologies, Carlsbad, CA, United States). GAPDH was selected as the internal reference gene. The primer sequences were as follows: TIRAP forward 5- CAGGAGGCATTGCTGATGAT-3; TIRAP reverse 5-GGGTAGTGGGCTGTCCTGTGAG-3; ELANE forward 5-GGAGCCCATAACCTCTCGC-3; ELANE reverse 5- GAGCAAGTTTACGGGGTCGT-3; GPX4 forward 5-GAGG CAAGACCGAAGTAAACTAC-3; GPX4 reverse 5-CCGAACT GGTTACACGGGAA-3; GSDMD forward 5- GTGTGTCAA CCTGTCTATCAAGG-3; GSDMD reverse 5-CATGGCATC GTAGAAGTGGAAG-3; GAPDH forward 5- CAGGAGGCA TTGCTGATGAT-3; GAPDH reverse 5-GAAGGCTG GGGCTCATTT-3. The relative levels of gene expression were evaluated by the 2−ΔΔCT method using GAPDH as the control.
Statistical Analysis
Statistical analysis was conducted using the R package and Graphpad Prism 8. Variance homogeneous and normal distributed continuous variables were analyzed using the unpaired student’s t-test, otherwise, the Mann-Whitney U-test or Kruskal-Wallis H-test was used. DEGs were screened by a Wilcoxon signed-rank test (false discovery rate, FDR < 0.05), and Benjamini and Hochberg (BH) corrections were used to adjust p values. The relationship between DEPRGS and clinicopathological manifestations were evaluated using logistic regression analyses and a heatmap graph. The survival analysis of UCEC patients based on the DEPRG panel was assessed using Kaplan–Meier survival analysis. A P-value of less than 0.05 was considered to be statistically significant (p < 0.001 = ∗∗∗, p < 0.01 = ∗∗, and p < 0.05 = ∗).
Results
Identification of Prognostic Differentially Expressed Pyroptosis-Related Genes of TCGA Database
In total, 5 of the 34 pyroptosis-related genes were related to prognosis and four of them (ELANE, GPX4, GSDMD, and TIRAP) had different expression values between tumor and normal tissues, which were, thus, chosen as prognostic DEPRGs (Figure 2A). The heatmap in endometrial cancer showed that ELANE and GSDMD were upregulated and GPX4 and TIRAP were downregulated (Figure 2B). The univariate Cox analysis of OS of the prognostic pyroptosis-related DEGs indicated that the four genes were low-risk factors (HR < 1) (Figure 2C). Therefore, these four genes were constituted into the optimal prognostic risk model of DEPRGs. The details of the genes are shown in Table 2.
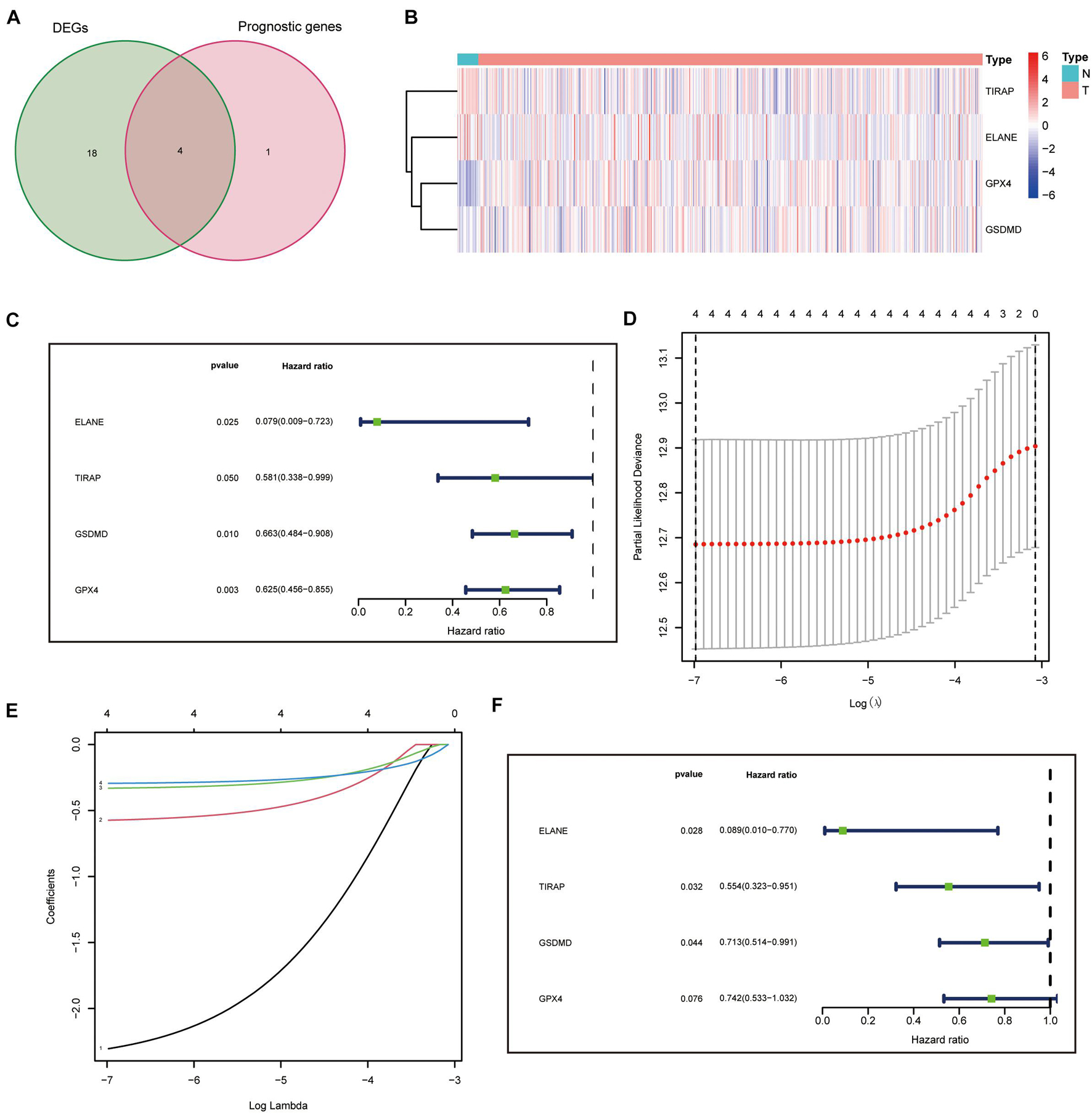
Figure 2. Identification and analysis of prognostic DEPRGs. (A) 4 identified prognostic DEPRGs, and their expression condition (B). Univariate (C), lasso Cox (D,E), and multivariate (F) analysis of prognostic DEPRGs.
Construction of a Prognostic Panel by Differentially Expressed Pyroptosis-Related Genes
A prognostic panel was constructed using the four DEPRGs by lasso regression analysis (Figures 2D,E) and multivariate Cox analysis (Figure 2F). The risk score of each patient was calculated according to the formula: risk score = expression value of ELANE × (−2.41387833412429) + expression value of GPX4 × (−0.298331831704336) + expression value of GSDMD × (−0.337701574941294) + expression value of TIRAP × (−0.58979182292574).
Prognostic Confirmation of the Prognostic Panel by Differentially Expressed Pyroptosis-Related Genes
The median risk score was taken for dividing patients into two groups (high- and low-risk group) and visualizing the results, as seen in Figure 3A. The survival status of each group was also visualized, as seen in Figure 3B. Patients in the two groups were distributed in different directions according to the PCA and t-SNE analysis (Figures 3C,D). Then, KM analysis showed that the patients with lower risk scores had higher survival potential with a p value < 0.01 (Figure 3E). The 1-, 2-, 3-, 4-, and 5-year ROC curves showed all areas under the curve (AUC) were over 0.6, and AUC under 5-year ROC curve was 0.692 (Figure 3F).
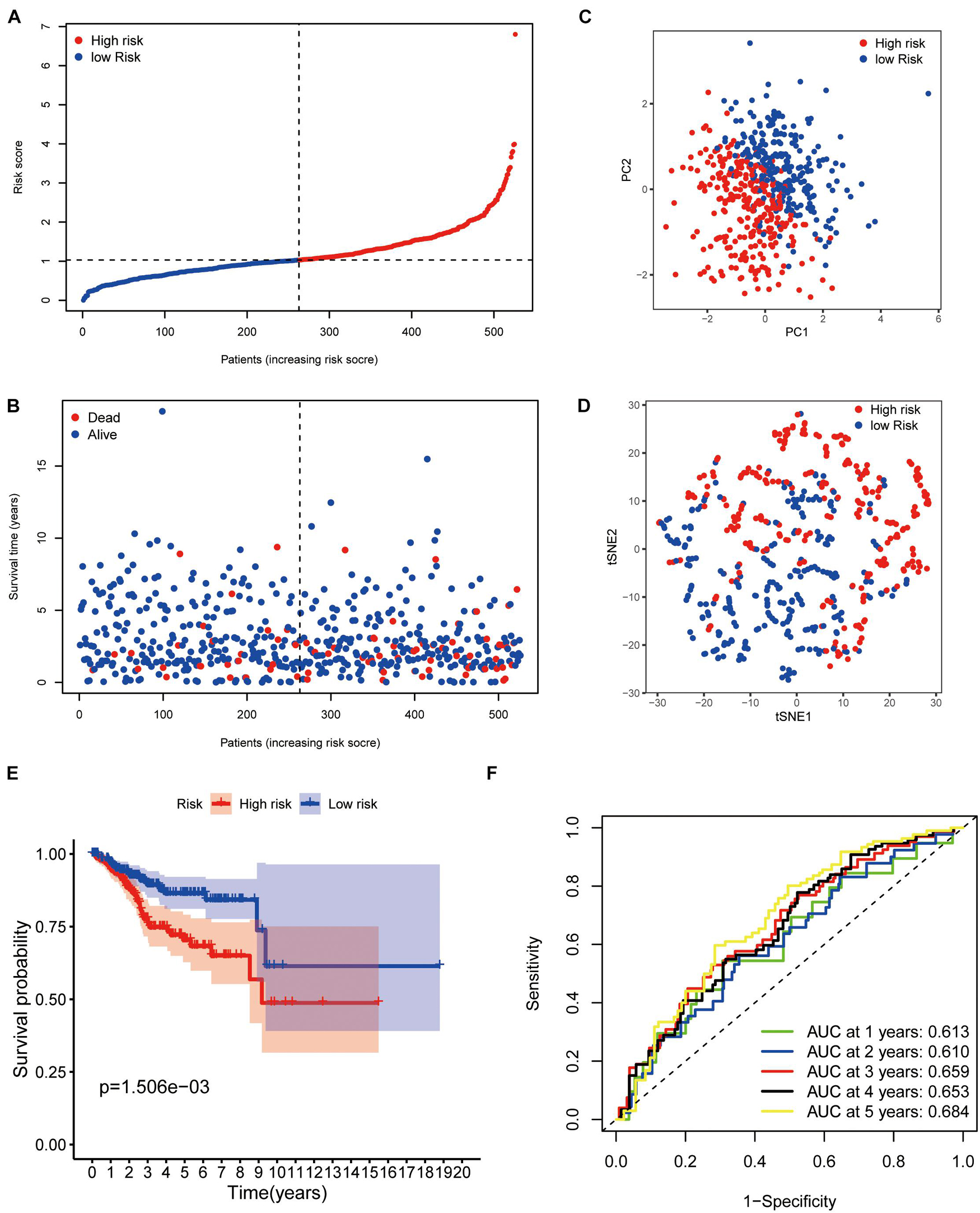
Figure 3. Prognostic confirmation of the panel by DEPRGs. (A) Risk scores and (B) survival status of EC patients. (C) PCA, (D) t-SNE studying the different gene expression patterns of samples. (E) Kaplan-Meier plot showing patients in the low-risk group survived longer than patients in the high-risk group. (F) The 1-, 2-, 3-, 4-, and 5-year ROC curve to predict the survival status.
Independence Confirmation and Clinical Evaluation of the Panel by Differentially Expressed Pyroptosis-Related Genes
We carried univariate Cox analyses among the common clinical features first, and multivariate Cox analysis next, which showed our panel can predict the prognosis of endometrial cancer independently (Figures 4A,B).
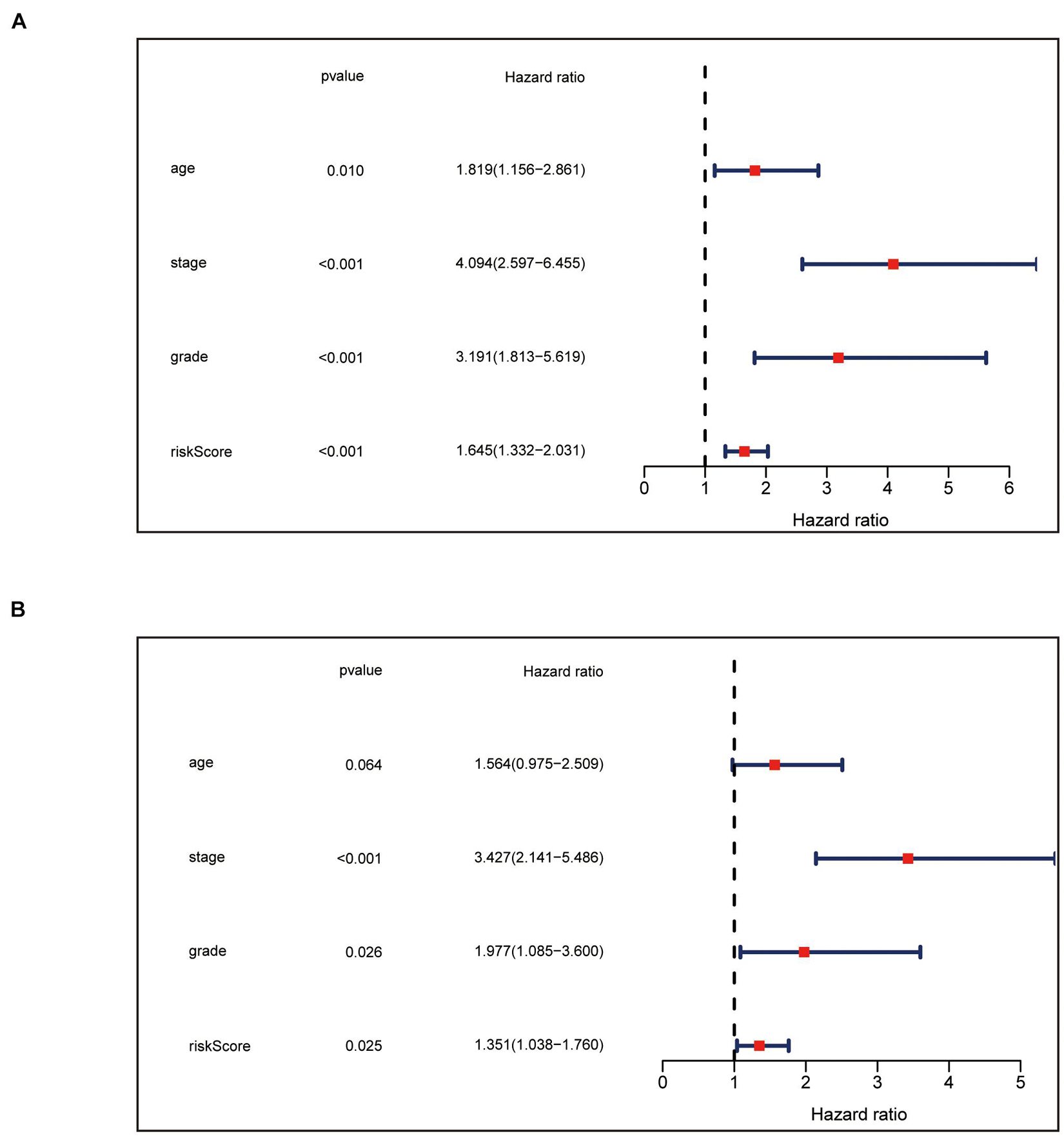
Figure 4. Independence confirmation of the panel by DEPRGs. (A) Univariate and (B) Multivariate Cox regression analysis of the panel and other clinical characteristics associated with OS.
The chi-square test, analyzing the relationship between the panel and clinicopathological characteristics, suggested that age, clinical stage, and tumor grade were significantly associated with the risk Score (p < 0.001) (Figure 5A). Wilcoxon signed-rank test comparing the risk score differences among different groups of these clinicopathological characteristics indicated age clinical stage, and tumor grade showed a positive relationship to risk scores (Figures 5B–D). The AUC of the panel, traditional clinicopathological characteristics, and DCA are shown in Figures 6A,B. There was also a nomogram incorporating the panel and clinicopathological characteristics in Figure 6C.
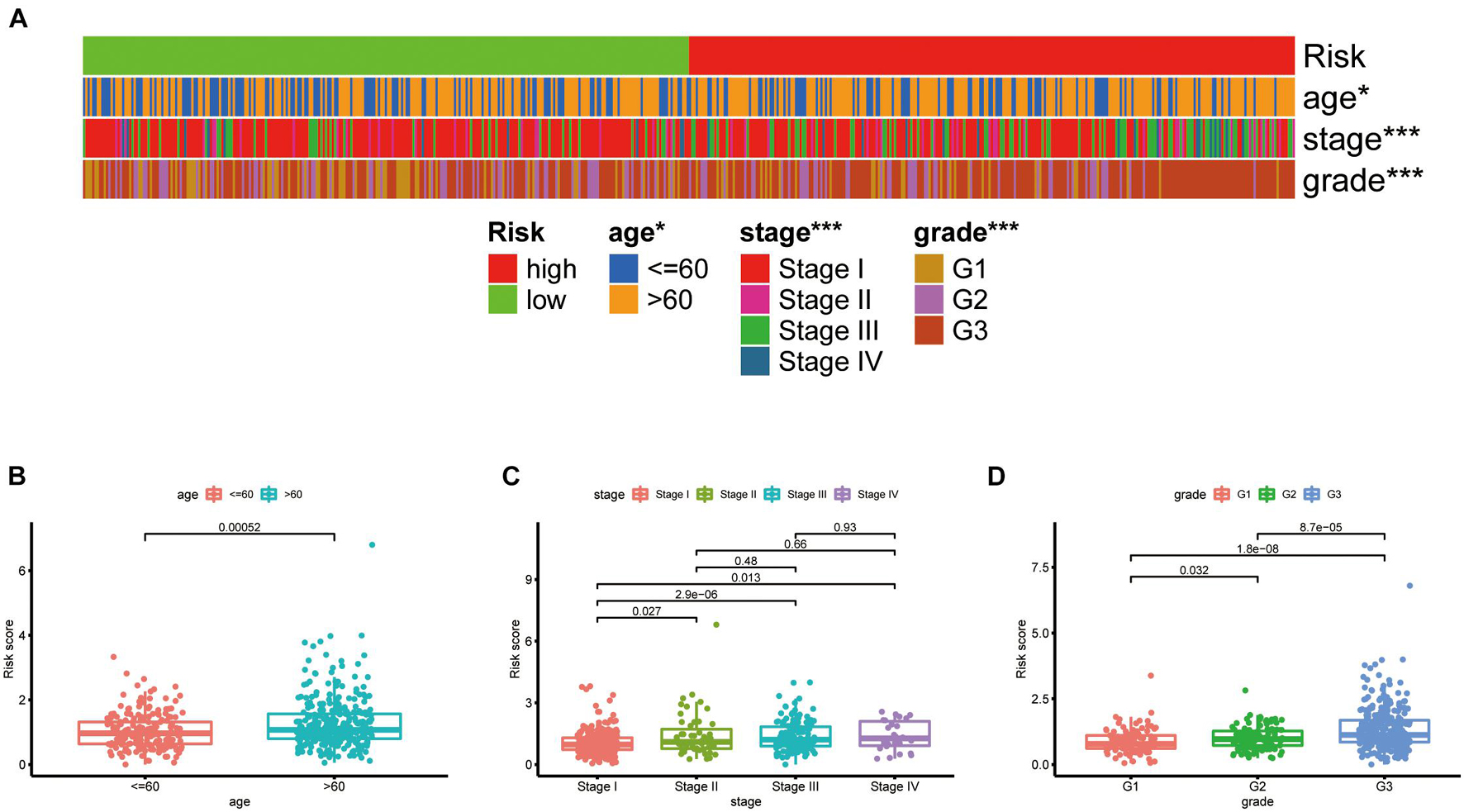
Figure 5. Clinical evaluation of the panel by DEPRGs. A band chart (A) and the scatter diagram indicating that (B) age, (C) clinical stage, and (D) tumor grade were significantly associated with the risk score.
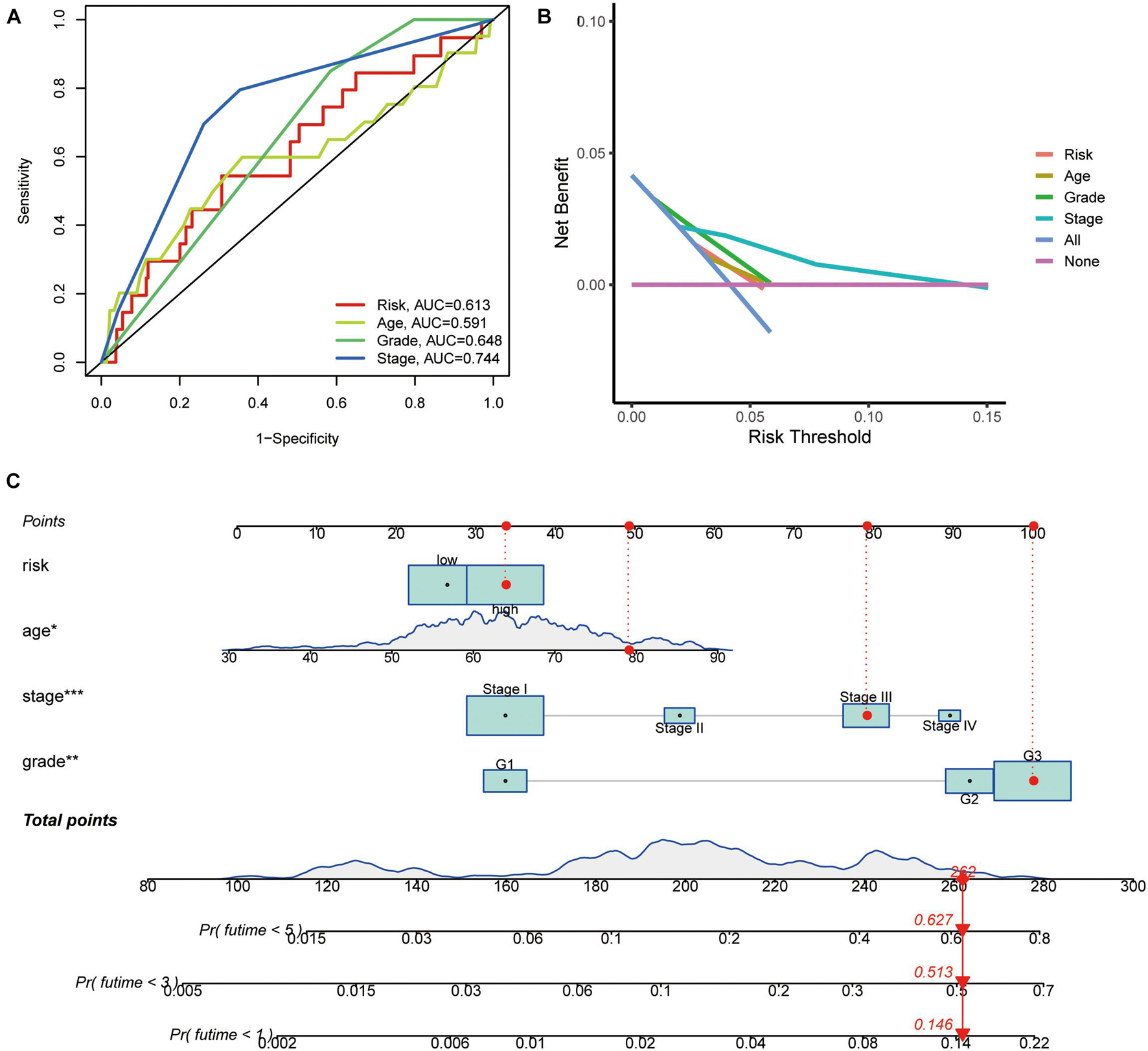
Figure 6. Indicators of the panel diagnosis by DEPRGs. (A) The clinical ROC curve, (B) decision curve analysis, and (C) c-index of the nomogram of the panel.
Gene Ontology, Kyoto Encyclopedia of Genes and Genomes Functional Enrichment, and Gene Set Enrichment Analysis Analysis Between High-Risk Versus Low-Risk Groups
To understand the molecular mechanism related to the risk score, the DEGs between two groups were used for GO and KEGG pathway analysis (Figures 7A,B). GO functional enrichment indicated that processes related to immunoglobin, immune response, and phagocytosis were enriched (Figure 7A). KEGG pathways analysis also indicated DEGs are enriched in some classic pathways including Wnt and cAMP signaling pathways and some necessary substance metabolism (Figure 7B). The details are shown in Tables 3, 4. The pathways enriched in the high-risk group based on GSEA are shown in Figures 7C–N.
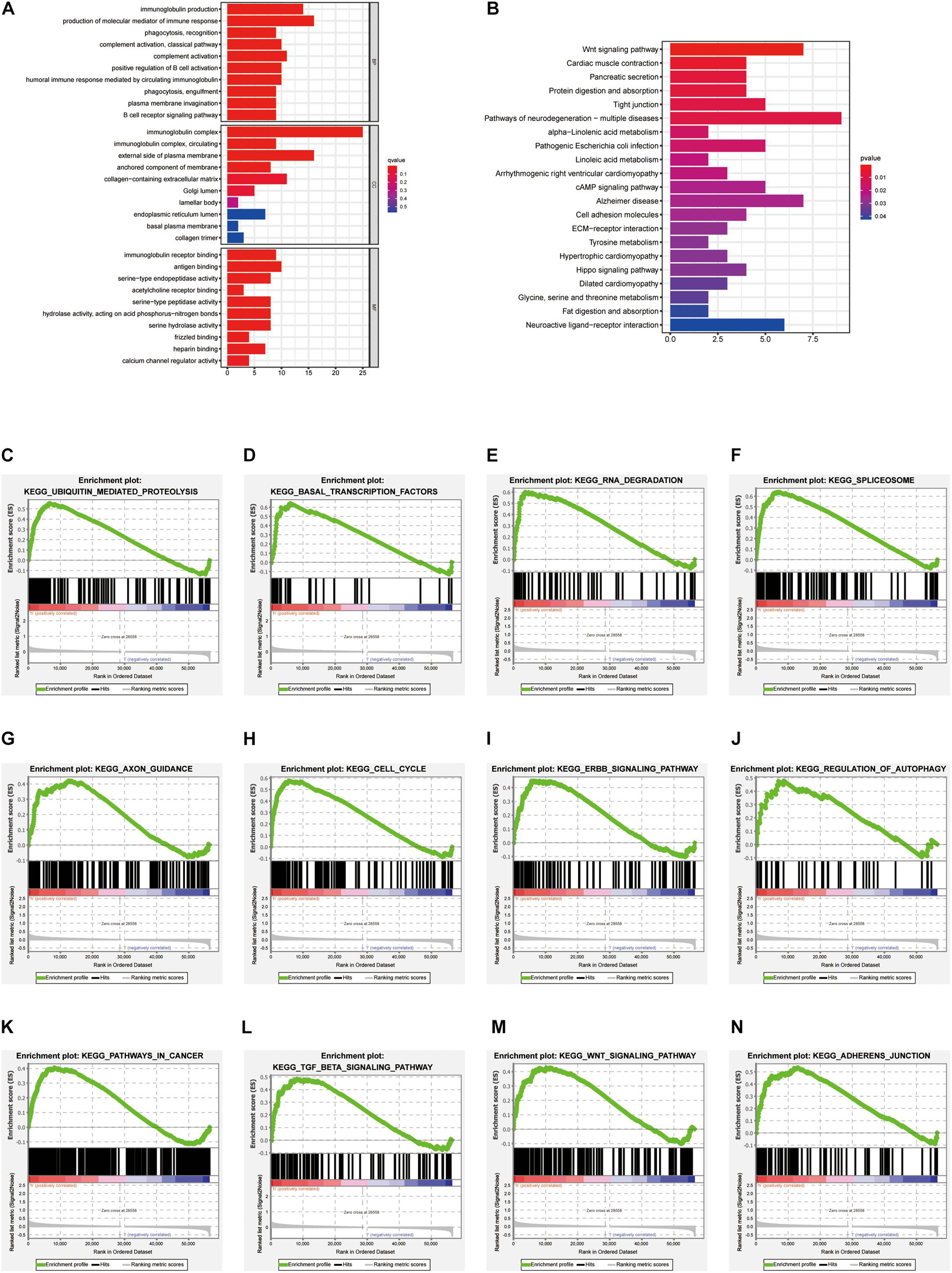
Figure 7. GO, KEGG functional enrichment, and GSEA analysis between high-risk versus low-risk groups. Top 10 results of (A) GO functional and (B) KEGG pathway enrichment of DEGs between the two groups. (C–N) The pathway enriched in the high-risk group based on GSEA.
Immune Characteristics Analysis
Through TIMER, CIBERSORT, CIBERSORT-ABS, QUANTISEQ, MCPcounter, XCELL, and EPIC conjoint analysis, we indicated that patients with high riskScore accumulated tumor-infiltrating immune cells such as macrophage, NK cells, B cells, and T cells (Figure 8A). In addition, we quantified the scores of different immune cell subsets, related functions, or pathways with ssGSEA for analyzing the relationship between risk scores and immune status. Interestingly, the immune function of CCR, check point, cytolytic activity, T cell co inhibition, T cell co stimulation, type I IFN response, type II IFN response and immune cell proportion of CD8+ T cells, DCs, neutrophils, pDCs, T helper cells, Th1 cells, and TIL were significantly different among the two groups (Figures 8B,C). Furthermore, patients with high-risk scores had low expression of immune checkpoint related genes (Figure 8D).
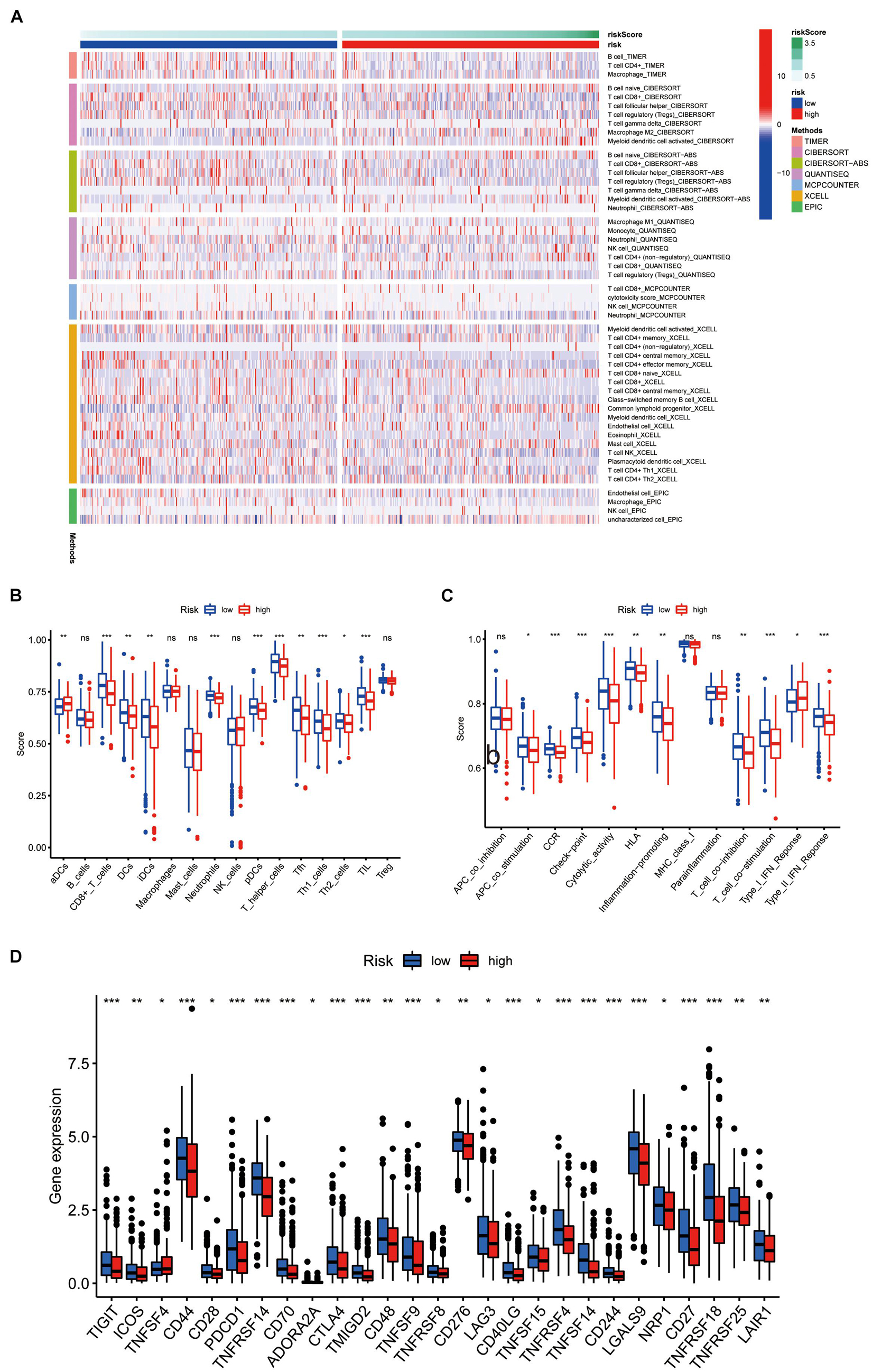
Figure 8. Immune characteristics analysis. (A) Heatmap for immune responses based on TIMER, CIBERSORT, CIBERSORT-ABS, QUANTISEQ, MCPcounter, XCELL, and EPIC algorithms among high and the low risk group. The differences of (B) immune cells, (C) related pathways between two groups based on ssGSEA. (D) Expression difference of immune checkpoint related genes between the two groups.
External Validation of the Four Prognostic Differentially Expressed Pyroptosis-Related Gene Panel
We first analyzed the relapse-free and overall survival of four prognostic DEPRGs through the Kaplan–Meier plotter in UCEC. The results showed that the survival probability differences between the high-expression group and the low-expression group were consistent with the former analysis (Figures 9A–H). We then used the GEPIA database and RT-PCR experiment to analyze the expression levels of the four prognostic DEPRGs, which indicated that GPX4 and GSDMD were highly expressed in UCEC compared to normal endometrial tissue, and TIRAP and ELANE were downregulated (Figures 10A–H).
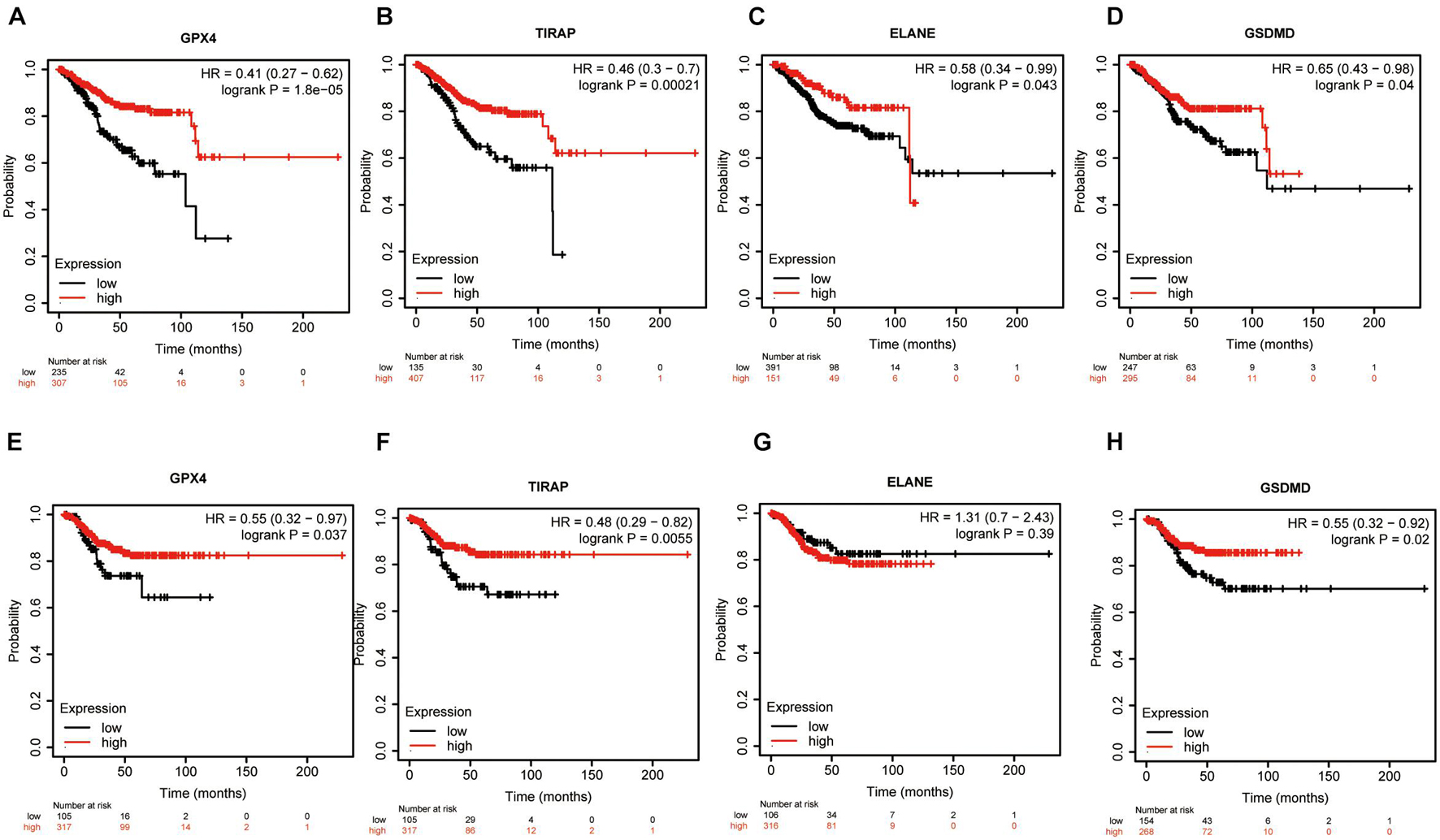
Figure 9. Survival Analysis of four prognostic DEPRGs. The OS (A–D) and RFS (E–H) difference in groups with high or low expression of prognostic DEPRGs.
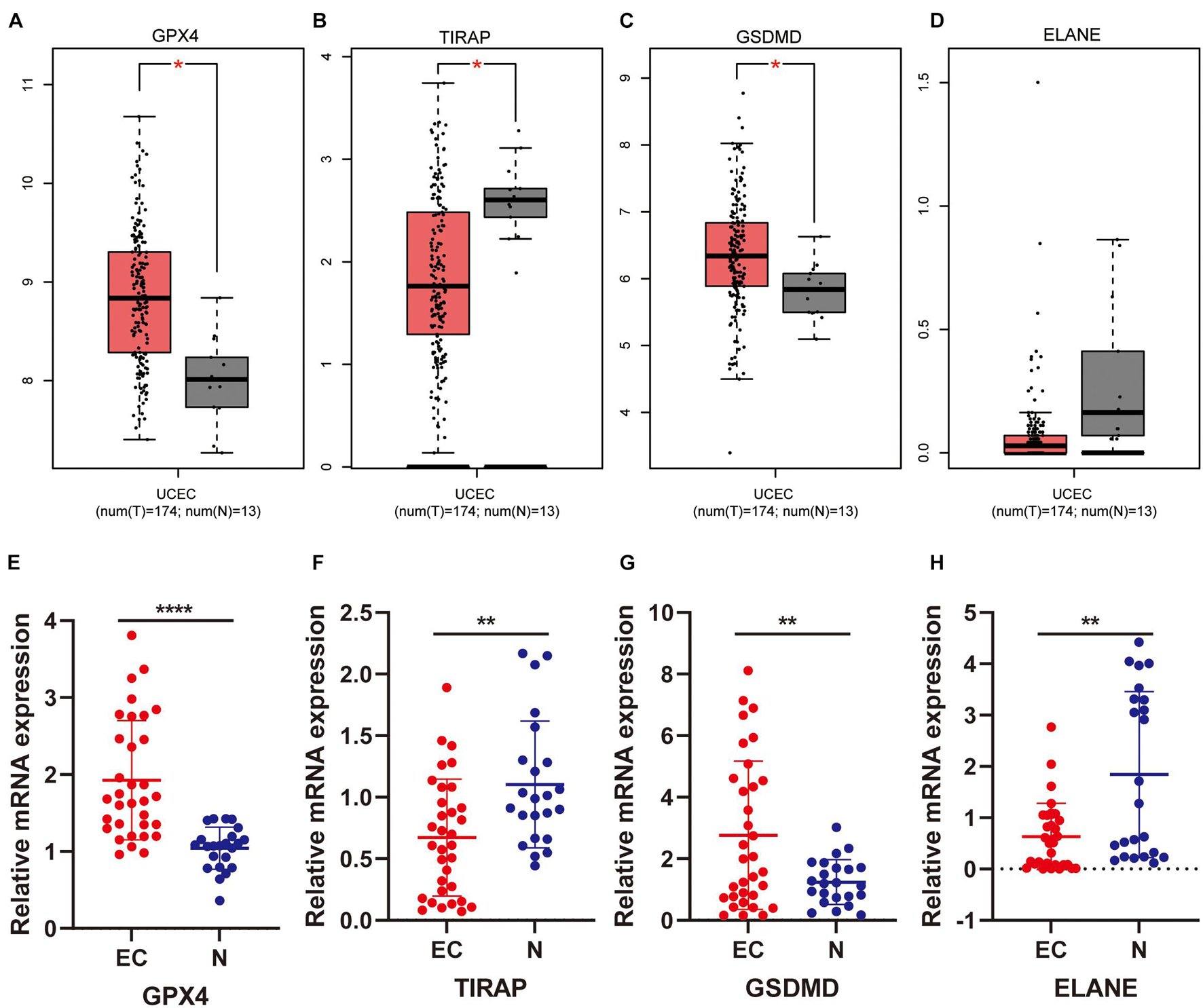
Figure 10. Expression validation of four prognostic DEPRGs. The expression difference between EC and normal endometrial tissues based on the GEPIA database (A–D) and RT-PCR (E–H).
Discussion
Recently, pyroptosis related genes, proteins, and related molecular processes in carcinogenesis, cancer novel therapy have attracted much attention in the research. There is a correlation with EC, as reported in another previous study (Yang et al., 2020). There is also another PRG panel that can help predict the overall survival and immune microenvironment in gastric and ovarian cancer (Shao et al., 2021; Ye et al., 2021). However, a PRG panel in EC has never been reported.
We used the TAGA database and 34 PRGs were analyzed in cases of UCEC and healthy people. We found four PRGs (ELANE, GPX4, GSDMD, and TIRAP) were significantly differentially expressed and related to overall survival in EC. As a member of the gasdermin family, GSDMD cleaved by caspase-1 and caspase-11/4/5 can trigger pyroptosis (Kayagaki et al., 2015; Shi et al., 2015, 2017). The role of GSDMD has been reported in EC (Yang et al., 2020). As a member of serine protease, ELANE can hydrolyze many proteins besides elastin. As Kambara et al. (2018) have reported, ELANE could mediate GSDMD cleavage and activation in a caspase-independent manner in neutrophils, and could in the future be used to promote macrophage pyroptosis. GPX4, an enzyme that can effectively reduce lipid peroxidation, regulates lipid peroxidation-dependent caspase-11 activation and GSDMD cleavage (Kang et al., 2018). TIRAP regulates the expression of caspase-11, a caspase-1-related protease, which is crucial for the activation of inflammatory bodies (Gurung et al., 2012). All in all, these 4 PRGs are included in pyroptosis and affect cancer cell progression, but whether it takes action in EC needs more exploration due to a few being reported in EC.
These four PRGs were used to construct the prediction panel. In univariate Cox analysis, there may be some false correlation or indirect correlation between the independent variable and the dependent variable. For example, factor A has no impact on the outcome event, while factor B is an influencing factor for the outcome event. However, since factor A is only simple and has a strong correlation with factor B, there is collinearity between them, so in univariate Cox analysis, there may be significant differences in factor A, which leads to factor a being mistaken as an influencing factor and included in the multi-factor analysis. In multivariate analysis, by adjusting the influence of factor B, the “false correlation” between factor A and the dependent variable disappears. At this time, it can be considered that factor A is not an influencing factor for the outcome event. Therefore, we conducted univariate, lasso, and multivariate Cox analyses to create the panel and avoid bias. KM plotter analysis, AUC of ROC curves, and univariate and multivariate cox analysis suggest that it can be used to predict the overall survival of EC patients independently. In addition, the risk score calculated by this panel in each patient was positively related to clinical characteristics, such as age, stage, and grade. The DEGs between two groups then conducted functional enrichment in GO, KEGG, GSEA, and immune characteristics analysis. In GO analysis, “phagocytosis,” “immunoglobin,” and “immune response” had the most frequent occurrences among the top 10 results, which suggested that the immune microenvironment may play a part in EC progression. In KEGG analysis, popular pathways, such as the Wnt signaling pathway, and cAMP signaling pathway, and some necessary substant metabolism, stressed the roles of PRGs in endometrial cancer. In GSEA analysis, pathways related to carcinogeneses such as ubiquitin-mediated proteolysis, basal transcription factors, cell cycle, regulation of autophagy, ERBB, TGFβ, Wnt pathways, and pathways in cancer were enriched. We next undertook immune function and immune cell distribution among the two groups. The results indicated that antigen-presenting cells, including DCs, pDCs, and T helper cells can help present pyroptosis cells to the T cells, and costimulate T cells and para secretion interferon to trigger a subsequent reaction. As reported, gasdermin-mediated cell pyroptosis was related to T cells, dendritic cells, neutrophils, and NK cells. Natural killer cells and cytolytic T cells release granzyme A or B mediating caspase activation and gasdermin family protein cleavage in macrophages, which increases pore-forming activity and formation of pore-forming protein, and thus cell pyroptosis (Wang et al., 2013; Jorgensen et al., 2016; Kambara et al., 2018; Xi et al., 2019; Liu et al., 2020; Zhang Z. et al., 2020; Zhou et al., 2020). However, how the pyroptosis-related immune system plays a role in EC needs more exploration and confirmation in vitro and in vivo.
Finally, we used an external database and low-throughput experiments to validate the four prognostic DEPRGs in EC. The results of the KM-plotter showed that low expression of these four prognostic DEPRGs had a longer survival time, which is consistent with the former analysis. Furthermore, the results of the GEPIA and RT-PCR experiment showed the expression level difference in EC and normal endometrial tissues.
In summary, we identified fpur prognosis-associated pyroptosis-related genes (ELANE, GPX4, GSDMD, and TIRAP). The model can also predict prognosis prediction and microenvironment in human endometrial cancer. However, to date, there have been few studies in the molecular mechanisms in pyroptosis related pathways and immunotherapy, and future large-scale clinical cohorts need to be undertaken to correct this panel.
Data Availability Statement
The original contributions presented in the study are included in the article/Supplementary Material, further inquiries can be directed to the corresponding author.
Ethics Statement
The studies involving human participants were reviewed and approved by This study was carried out in accordance with the standards of the Helsinki Declaration of the World Medical Association, and approved by the Ethics Committee of China Medical University. The patients/participants provided their written informed consent to participate in this study.
Author Contributions
XZ downloaded the dataset, analyzed the data, and was a major contributor in writing the manuscript. QY reviewed the manuscript. Both authors read and approved the final manuscript.
Funding
This work was supported by the National Natural Science Foundation of China (No. 81872125) and the Outstanding Scientific Fund of Shengjing Hospital (No. 201704).
Conflict of Interest
The authors declare that the research was conducted in the absence of any commercial or financial relationships that could be construed as a potential conflict of interest.
Publisher’s Note
All claims expressed in this article are solely those of the authors and do not necessarily represent those of their affiliated organizations, or those of the publisher, the editors and the reviewers. Any product that may be evaluated in this article, or claim that may be made by its manufacturer, is not guaranteed or endorsed by the publisher.
Supplementary Material
The Supplementary Material for this article can be found online at: https://www.frontiersin.org/articles/10.3389/fcell.2021.705828/full#supplementary-material
Abbreviations
EC, endometrial cancer; UCEC, uterine Corpus Endometrial Carcinoma; GSDMD, gasdermin D; GSDME, gasdermin E; DHA, docosahexaenoic acid; LXPs, liver X receptors; ATO, arsenic trioxide; AA, ascorbic acid; NLRP3, NACHT Domain-, Leucine-Rich Repeat-, and PYD-Containing Protein 3; PRGs, pyroptosis-related genes; DEGs, Differential expression genes; DEPRGs, differentially expressed PRGs; OS, overall survival; RFS, relapse-free survival; BH, Benjamini and Hochberg; PCA, principal components analysis; ROC, operating characteristic curve; DCA, decision curve analysis; GO, Gene ontology; KEGG, Kyoto Encyclopedia of Genes and Genomes; GSEA, Gene Set Enrichment Analysis; GEPIA, Gene Expression Profiling Interactive Analysis; T, tumor; N, normal; FDR, false discovery rate.
Footnotes
- ^ https://portal.gdc.cancer.gov/repository
- ^ http://www.broad.mit.edu/gsea
- ^ http://kmplot.com/analysis/
- ^ http://gepia.cancer-pku.cn/
References
Amant, F., Moerman, P., Neven, P., Timmerman, D., Van Limbergen, E., and Vergote, I. (2005). Endometrial cancer. Lancet 366, 491–505.
An, H., Heo, J. S., Kim, P., Lian, Z., Lee, S., Park, J., et al. (2021). Tetraarsenic hexoxide enhances generation of mitochondrial ROS to promote pyroptosis by inducing the activation of caspase-3/GSDME in triple-negative breast cancer cells. Cell Death Dis 12:159.
Bergsbaken, T., Fink, S. L., and Cookson, B. T. (2009). Pyroptosis: host cell death and inflammation. Nat. Rev. Microbiol. 7, 99–109. doi: 10.1038/nrmicro2070
Chen, X., Liu, G., Liu, Y., Wu, G., Wang, S., and Yuan, L. (2019). NEK7 interacts with NLRP3 to modulate the pyroptosis in inflammatory bowel disease via NF-κB signaling. Cell Death Dis. 10:906.
Cui, J., Zhou, Z., Yang, H., Jiao, F., Li, N., Gao, Y., et al. (2019). MST1 suppresses pancreatic cancer progression via ros-induced pyroptosis. Mol. Cancer Res. 17, 1316–1325. doi: 10.1158/1541-7786.mcr-18-0910
Derangère, V., Chevriaux, A., Courtaut, F., Bruchard, M., Berger, H., Chalmin, F., et al. (2014). Liver X receptor β activation induces pyroptosis of human and murine colon cancer cells. Cell Death Differ. 21, 1914–1924. doi: 10.1038/cdd.2014.117
Gupta, D. (2017). Clinical behavior and treatment of endometrial cancer. Adv. Exp. Med. Biol. 943, 47–74. doi: 10.1007/978-3-319-43139-0_2
Gurung, P., Malireddi, R. K., Anand, P. K., Demon, D., Vande Walle, L., Liu, Z., et al. (2012). Toll or interleukin-1 receptor (TIR) domain-containing adaptor inducing interferon-β (TRIF)-mediated caspase-11 protease production integrates Toll-like receptor 4 (TLR4) protein- and Nlrp3 inflammasome-mediated host defense against enteropathogens. J. Biol. Chem. 287, 34474–34483. doi: 10.1074/jbc.m112.401406
Győrffy, B., Surowiak, P., Budczies, J., and Lánczky, A. (2013). Online survival analysis software to assess the prognostic value of biomarkers using transcriptomic data in non-small-cell lung cancer. PLoS One 8:e82241. doi: 10.1371/journal.pone.0082241
Hage, C., Hoves, S., Strauss, L., Bissinger, S., Prinz, Y., Pöschinger, T., et al. (2019). Sorafenib induces pyroptosis in macrophages and triggers natural killer cell-mediated cytotoxicity against hepatocellular carcinoma. Hepatology 70, 1280–1297. doi: 10.1002/hep.30666
Han, C., Yang, Y., Guan, Q., Zhang, X., Shen, H., and Sheng, Y. (2020). New mechanism of nerve injury in Alzheimer’s disease: β-amyloid-induced neuronal pyroptosis. J. Cell Mol. Med. 24, 8078–8090. doi: 10.1111/jcmm.15439
He, W. T., Wan, H., Hu, L., Chen, P., Wang, X., Huang, Z., et al. (2015). Gasdermin D is an executor of pyroptosis and required for interleukin-1β secretion. Cell Res. 25, 1285–1298. doi: 10.1038/cr.2015.139
Jia, C., Chen, H., Zhang, J., Zhou, K., Zhuge, Y., Niu, C., et al. (2019). Role of pyroptosis in cardiovascular diseases. Int. Immunopharmacol. 67, 311–318. doi: 10.1016/s0939-4753(03)80058-3
Jorgensen, I., Lopez, J. P., Laufer, S. A., and Miao, E. A. (2016). IL-1β, IL-18, and eicosanoids promote neutrophil recruitment to pore-induced intracellular traps following pyroptosis. Eur. J. Immunol. 46, 2761–2766. doi: 10.1002/eji.201646647
Kambara, H., Liu, F., Zhang, X., Liu, P., Bajrami, B., Teng, Y., et al. (2018). Gasdermin D exerts anti-inflammatory effects by promoting neutrophil death. Cell Rep. 22, 2924–2936. doi: 10.1016/j.celrep.2018.02.067
Kang, R., Zeng, L., Zhu, S., Xie, Y., Liu, J., Wen, Q., et al. (2018). Lipid peroxidation drives gasdermin D-mediated pyroptosis in lethal polymicrobial sepsis. Cell Host Microbe 24, 97–108.e4.
Karki, R., and Kanneganti, T. D. (2019). Diverging inflammasome signals in tumorigenesis and potential targeting. Nat. Rev. Cancer 19, 197–214. doi: 10.1038/s41568-019-0123-y
Kayagaki, N., Stowe, I. B., Lee, B. L., O’Rourke, K., Anderson, K., Warming, S., et al. (2015). Caspase-11 cleaves gasdermin D for non-canonical inflammasome signalling. Nature 526, 666–671. doi: 10.1038/nature15541
Kovacs, S. B., and Miao, E. A. (2017). Gasdermins: effectors of pyroptosis. Trends Cell Biol. 27, 673–684. doi: 10.1016/j.tcb.2017.05.005
Li, Y. (2021). Dihydroartemisinin induces pyroptosis by promoting the AIM2/caspase-3/DFNA5 axis in breast cancer cells. Chem. Biol. Interact. 340:109434. doi: 10.1016/j.cbi.2021.109434
Liang, J. Y., Wang, D. S., Lin, H. C., Chen, X. X., Yang, H., Zheng, Y., et al. (2020). A novel ferroptosis-related gene signature for overall survival prediction in patients with hepatocellular carcinoma. Int. J. Biol. Sci. 16, 2430–2441. doi: 10.7150/ijbs.45050
Lin, J., Cheng, A., Cheng, K., Deng, Q., Zhang, S., Lan, Z., et al. (2020). New insights into the mechanisms of pyroptosis and implications for diabetic kidney disease. Int. J. Mol. Sci. 21:7057. doi: 10.3390/ijms21197057
Liu, Y., Fang, Y., Chen, X., Wang, Z., Liang, X., Zhang, T., et al. (2020). Gasdermin E-mediated target cell pyroptosis by CAR T cells triggers cytokine release syndrome. Sci. Immunol. 5:eaax7969. doi: 10.1126/sciimmunol.aax7969
Man, S. M., and Kanneganti, T. D. (2015). Regulation of inflammasome activation. Immunol. Rev. 265, 6–21. doi: 10.1111/imr.12296
Man, S. M., Karki, R., and Kanneganti, T. D. (2017). Molecular mechanisms and functions of pyroptosis, inflammatory caspases and inflammasomes in infectious diseases. Immunol. Rev. 277, 61–75. doi: 10.1111/imr.12534
Moore, K., and Brewer, M. A. (2017). Endometrial cancer: is this a new disease? Am. Soc. Clin. Oncol. Educ. Book 37, 435–442. doi: 10.14694/edbk_175666
Nagy, Á, Munkácsy, G., and Gyõrffy, B. (2021). Pancancer survival analysis of cancer hallmark genes. Sci. Rep. 11:6047.
Pizato, N., Luzete, B. C., Kiffer, L. F. M. V., Corrêa, L. H., de Oliveira Santos, I., Assumpção, J. A. F., et al. (2018). Omega-3 docosahexaenoic acid induces pyroptosis cell death in triple-negative breast cancer cells. Sci. Rep. 8:1952.
Qiao, L., Wu, X., Zhang, J., Liu, L., Sui, X., Zhang, R., et al. (2019). α-NETA induces pyroptosis of epithelial ovarian cancer cells through the GSDMD/caspase-4 pathway. FASEB J. 33, 12760–12767. doi: 10.1096/fj.201900483rr
Robinson, N., Ganesan, R., Hegedûs, C., Kovács, K., Kufer, T. A., and Virág, L. (2019). Programmed necrotic cell death of macrophages: focus on pyroptosis, necroptosis, and parthanatos. Redox Biol. 26:101239. doi: 10.1016/j.redox.2019.101239
Shao, W., Yang, Z., Fu, Y., Zheng, L., Liu, F., Chai, L., et al. (2021). The pyroptosis-related signature predicts prognosis and indicates immune microenvironment infiltration in gastric cancer. Front. Cell Dev. Biol. 9:676485.
Shi, J., Gao, W., and Shao, F. (2017). Pyroptosis: gasdermin-mediated programmed necrotic cell death. Trends Biochem. Sci. 42, 245–254. doi: 10.1016/j.tibs.2016.10.004
Shi, J., Zhao, Y., Wang, K., Shi, X., Wang, Y., Huang, H., et al. (2015). Cleavage of GSDMD by inflammatory caspases determines pyroptotic cell death. Nature 526, 660–665. doi: 10.1038/nature15514
Subramanian, A., Tamayo, P., Mootha, V. K., Mukherjee, S., Ebert, B. L., Gillette, M. A., et al. (2005). Gene set enrichment analysis: a knowledge-based approach for interpreting genome-wide expression profiles. Proc. Natl. Acad. Sci. U.S.A. 102, 15545–15550. doi: 10.1073/pnas.0506580102
Sung, H., Ferlay, J., Siegel, R. L., Laversanne, M., Soerjomataram, I., Jemal, A., et al. (2021). Global cancer statistics 2020: GLOBOCAN estimates of incidence and mortality worldwide for 36 cancers in 185 countries. CA Cancer J. Clin. 71, 209–249. doi: 10.3322/caac.21660
Tan, Y., Sun, R., Liu, L., Yang, D., Xiang, Q., Li, L., et al. (2021). Tumor suppressor DRD2 facilitates M1 macrophages and restricts NF-κB signaling to trigger pyroptosis in breast cancer. Theranostics 11, 5214–5231. doi: 10.7150/thno.58322
Tang, Z., Li, C., Kang, B., Gao, G., Li, C., and Zhang, Z. (2017). GEPIA: a web server for cancer and normal gene expression profiling and interactive analyses. Nucleic Acids Res. 45, W98–W102.
Teng, J. F., Mei, Q. B., Zhou, X. G., Tang, Y., Xiong, R., Qiu, W. Q., et al. (2020). Polyphyllin VI Induces caspase-1-mediated pyroptosis via the induction of ROS/NF-κB/NLRP3/GSDMD signal axis in non-small cell lung cancer. Cancers (Basel) 12:193. doi: 10.3390/cancers12010193
Tian, W., Wang, Z., Tang, N. N., Li, J. T., Liu, Y., Chu, W. F., et al. (2020). Ascorbic acid sensitizes colorectal carcinoma to the cytotoxicity of arsenic trioxide via promoting reactive oxygen species-dependent apoptosis and pyroptosis. Front. Pharmacol. 11:123.
Vickers, A. J., and Elkin, E. B. (2006). Decision curve analysis: a novel method for evaluating prediction models. Med. Decis Making 26, 565–574. doi: 10.1177/0272989x06295361
Wang, B., and Yin, Q. (2017). AIM2 inflammasome activation and regulation: a structural perspective. J Struct. Biol. 200, 279–282. doi: 10.1016/j.jsb.2017.08.001
Wang, Q., Imamura, R., Motani, K., Kushiyama, H., Nagata, S., and Suda, T. (2013). Pyroptotic cells externalize eat-me and release find-me signals and are efficiently engulfed by macrophages. Int. Immunol. 25, 363–372. doi: 10.1093/intimm/dxs161
Wang, S., Yuan, Y. H., Chen, N. H., and Wang, H. B. (2019). The mechanisms of NLRP3 inflammasome/pyroptosis activation and their role in Parkinson’s disease. Int. Immunopharmacol. 67, 458–464. doi: 10.1016/j.intimp.2018.12.019
Wang, Y., Zhu, X., Yuan, S., Wen, S., Liu, X., Wang, C., et al. (2019). TLR4/NF-κB signaling induces gsdmd-related pyroptosis in tubular cells in diabetic kidney disease. Front. Endocrinol. (Lausanne) 10:603.
Xi, G., Gao, J., Wan, B., Zhan, P., Xu, W., Lv, T., et al. (2019). GSDMD is required for effector CD8(+) T cell responses to lung cancer cells. Int. Immunopharmacol. 74, 105713. doi: 10.1016/j.intimp.2019.105713
Xia, X., Wang, X., Cheng, Z., Qin, W., Lei, L., Jiang, J., et al. (2019). The role of pyroptosis in cancer: pro-cancer or pro-“host”? Cell Death Dis. 10:650.
Yang, Y., Liu, P. Y., Bao, W., Chen, S. J., Wu, F. S., and Zhu, P. Y. (2020). Hydrogen inhibits endometrial cancer growth via a ROS/NLRP3/caspase-1/GSDMD-mediated pyroptotic pathway. BMC Cancer 20:28.
Ye, Y., Dai, Q., and Qi, H. (2021). A novel defined pyroptosis-related gene signature for predicting the prognosis of ovarian cancer. Cell Death Discov. 7:71.
Yu, J., Li, S., Qi, J., Chen, Z., Wu, Y., Guo, J., et al. (2019). Cleavage of GSDME by caspase-3 determines lobaplatin-induced pyroptosis in colon cancer cells. Cell Death Dis. 10:193.
Zhang, C. C., Li, C. G., Wang, Y. F., Xu, L. H., He, X. H., Zeng, Q. Z., et al. (2019). Chemotherapeutic paclitaxel and cisplatin differentially induce pyroptosis in A549 lung cancer cells via caspase-3/GSDME activation. Apoptosis 24, 312–325. doi: 10.1007/s10495-019-01515-1
Zhang, T., Li, Y., Zhu, R., Song, P., Wei, Y., Liang, T., et al. (2019). Transcription factor p53 suppresses tumor growth by prompting pyroptosis in non-small-cell lung cancer. Oxid. Med. Cell. Longev. 2019:8746895.
Zhang, X., Zhang, P., An, L., Sun, N., Peng, L., Tang, W., et al. (2020). Miltirone induces cell death in hepatocellular carcinoma cell through GSDME-dependent pyroptosis. Acta Pharm. Sin. B 10, 1397–1413. doi: 10.1016/j.apsb.2020.06.015
Zhang, Z., Zhang, Y., Xia, S., Kong, Q., Li, S., Liu, X., et al. (2020). Gasdermin E suppresses tumour growth by activating anti-tumour immunity. Nature 579, 415–420. doi: 10.1038/s41586-020-2071-9
Zhaolin, Z., Guohua, L., Shiyuan, W., and Zuo, W. (2019). Role of pyroptosis in cardiovascular disease. Cell Prolif. 52, e12563. doi: 10.1111/cpr.12563
Keywords: endometrial cancer, pyroptosis-related genes, overall survival, panel, tumor immune environment
Citation: Zhang X and Yang Q (2021) A Pyroptosis-Related Gene Panel in Prognosis Prediction and Immune Microenvironment of Human Endometrial Cancer. Front. Cell Dev. Biol. 9:705828. doi: 10.3389/fcell.2021.705828
Received: 06 May 2021; Accepted: 22 September 2021;
Published: 14 October 2021.
Edited by:
Tugba Bagci-Onder, Koç University, TurkeyReviewed by:
Ivarne L. S. Tersariol, Federal University of São Paulo, BrazilTriona Ni Chonghaile, Royal College of Surgeons in Ireland, Ireland
Zongqiang Huang, First Affiliated Hospital of Zhengzhou University, China
Runju Zhang, Zhejiang University, China
Copyright © 2021 Zhang and Yang. This is an open-access article distributed under the terms of the Creative Commons Attribution License (CC BY). The use, distribution or reproduction in other forums is permitted, provided the original author(s) and the copyright owner(s) are credited and that the original publication in this journal is cited, in accordance with accepted academic practice. No use, distribution or reproduction is permitted which does not comply with these terms.
*Correspondence: Qing Yang, yangqing_sj@126.com