- Department of Molecular Biology and Biochemistry, Guru Nanak Dev University, Amritsar, INDIA
ZP2, an important component of the zona matrix, surrounds mammalian oocytes and facilitates fertilization. Recently, some studies have documented the association of mutations in genes encoding the zona matrix with the infertile status of human females. Single nucleotide polymorphisms are the most common type of genetic variations observed in a population and as per the dbSNP database, around 5,152 SNPs are reported to exist in the human ZP2 (hZP2) gene. Although a wide range of computational tools are publicly available, yet no computational studies have been done to date to identify and analyze structural and functional effects of deleterious SNPs on hZP2. In this study, we conducted a comprehensive in silico analysis of all the SNPs found in hZP2. Six different computational tools including SIFT and PolyPhen-2 predicted 18 common nsSNPs as deleterious of which 12 were predicted to most likely affect the structure/functional properties. These were either present in the N-term region crucial for sperm-zona interaction or in the zona domain. 31 additional SNPs in both coding and non-coding regions were also identified. Interestingly, some of these SNPs have been found to be present in infertile females in some recent studies.
1 Introduction
Reproduction is a fundamental process, which ensures the continued existence of all life forms. Natural selection has preferred sexual reproduction over asexual one, even though it is lengthy and complicated. The reason being its great contribution to genetic diversity, which gives different life forms an upper hand in the race to the survival of the fittest. Sexual reproduction involves the unification of two gametes i.e. sperm and oocyte from the male and female parents respectively during fertilization. Mammalian oocytes are surrounded by an extracellular fibrous matrix called zona pellucida (ZP), which plays an important role in folliculogenesis, fertilization, block to polyspermy, and in the protection of embryo during pre-implantation (Zhao and Dean, 2002). In humans, ZP is composed of four distinct glycoproteins designated as ZP1, ZP2, ZP3, and ZP4 (Lefièvre et al., 2004). Among these constituent proteins, ZP2 plays a significant role in allowing sperm to bind to the unfertilized egg and eventually lead to post-fertilization block to polyspermy (Gahlay et al., 2010; Burkart et al., 2012). Human ZP2 protein (hZP2) is encoded by a single copy gene (hZP2) which is located on the 16th chromosome (band: p12.3-p12.2). It consists of 20 exons which encode for 5 mRNA splice variants. Among these, one variant (NM_003460) encodes for the 745 amino acid long protein product (NP_003451) forming the zona matrix. hZP2 is a glycoprotein having both N-linked (∼37% of its molecular weight) as well as O-linked glycosylation (∼8%) (Chiu et al., 2008). The nascent ZP2 protein has an N-terminal signal peptide sequence, a conserved ZP domain, a consensus furin cleavage site, and a C-terminal transmembrane domain (Gupta and Brunak, 2002).
Investigating the relationship between nucleotide variations at the DNA level and the subsequent changes in the structure and function of the associated proteins with the diseased condition is a major challenge for researchers. Single nucleotide polymorphisms (SNPs) are the most common type of genetic variation in humans. Among these, the non-synonymous SNPs (nsSNPs), which result in encoding for a different amino acid, can have drastic effects on protein structure, function, and the associated phenotype. Numerous studies have proven the role of SNPs in different diseased conditions like infectious diseases (Schröder and Schumann, 2005), Type 2 diabetes (Willer et al., 2007) breast cancer (Rajasekaran et al., 2007), polycystic ovary syndrome (PCOS) (Chen and Fang, 2018), male infertility (Zhang et al., 2014; Li et al., 2017), etc. With the availability of Next Generation genome sequencing and different databases like dbSNP, GWAS Central, SwissVar, etc. the presence of SNPs in different genes can be easily studied. Further, to assist genetic studies, several machine learning tools have also recently been developed to identify and predict the impact of variants of unknown significance and pathogenicity (Peterson et al., 2013; Niroula and Vihinen, 2016).
Although SNP analysis for several genes involved in different diseased conditions has been done, yet the role of SNPs in ZP genes in altering its protein’s structure and function and thus, their correlation with female infertility has not been widely studied. Association between SNP’s in ZP genes and fertilization failure in IVF (Männikkö et al., 2005), anomalies in ZP (Pökkylä et al., 2011; Zhou et al., 2019), familial infertility (Huang et al., 2014) have although been recently indicated in some studies. Of the various ZP proteins, ZP2 is critical in the first step of sperm-egg interaction facilitating the binding of sperm with an unfertilized oocyte (Gahlay et al., 2010). Sperm are unable to bind to an oocyte if the N-terminus region (51–149 aa) of ZP2 is absent (Avella et al., 2014). Post-fertilization, cleavage of ZP2 prevents the sperm to bind to the fertilized egg and is thus also involved in the post-fertilization block to polyspermy (Gahlay et al., 2010; Burkart et al., 2012). Considering this, it becomes imperative to study the effect of human ZP2 (hZP2) SNPs on fertility.
For this, the dbSNP database was analyzed and around 5,152 SNPs are reported to exist for our candidate gene hZP2 (retrieved as of Dec 2020). No computational study has been done so far to prioritize the deleterious SNPs in the hZP2 in terms of their disease causing potential. So, this study is aimed to explore the various bioinformatics tools, in order to identify and predict the most deleterious single nucleotide variations in hZP2 based on their predicted structural, functional, and regulatory effect(s) on the protein. Apart from increasing our existing knowledge in explaining the putative involvement of genetic background in deciding the reproductive fitness of the females, this knowledge can also be used to use these deleterious SNPs in the detection of idiopathic female infertility.
2 Materials and Methods
2.1 Datasets and SNP Retrieval
The hZP2 gene data was obtained from Entrez Gene on National Center for Biological Information (NCBI) website and Ensembl genome database. The SNP information for hZP2 (rsIDs, chromosomal position, residue change, and global minor allele frequency (MAF)) was retrieved from the NCBI dbSNP database. Among all the SNPs present in hZP2, the validated ones were cataloged into coding and non-coding. The coding SNPs were further categorized into non-synonymous, synonymous, nonsense, and frameshift. The non-synonymous SNPs were then subjected to a variety of in silico tools as shown in Figure 1.
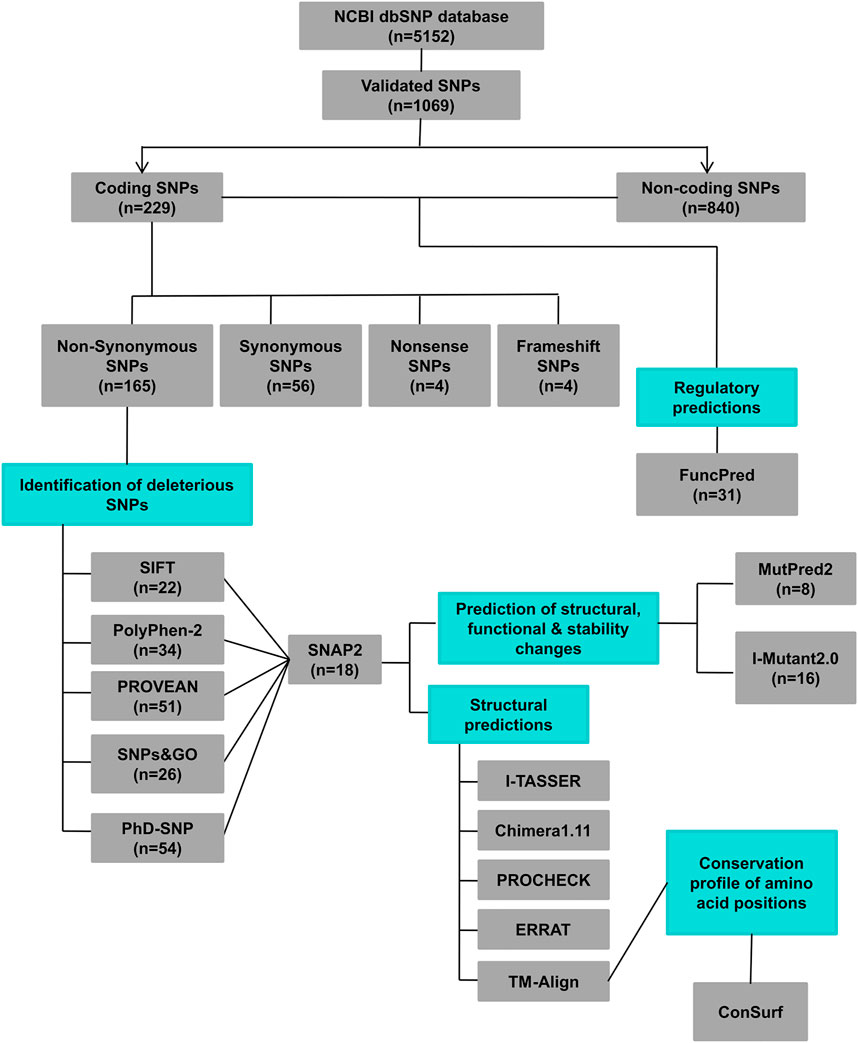
FIGURE 1. A schematic representation of different in silico tools used at different steps of this study (n = number of SNPs).
2.2 Identification of Deleterious or Disease-Associated nsSNPs
To filter out the deleterious non-synonymous SNPs (nsSNPs), six different bioinformatics tools were employed. These include SIFT (Sorting Intolerant From Tolerant; https://sift.bii.a-star.edu.sg/) (Ng and Henikoff, 2001; Kumar et al., 2009), PolyPhen-2 (Polymorphism phenotyping v2; http://genetics.bwh.harvard.edu/pph2/) (Adzhubei et al., 2010, 2013), PROVEAN (Protein Variation Effect Analyzer; http://provean.jcvi.org/index.php) (Choi et al., 2012; Choi and Chan, 2015), SNPs&GO (http://snps.biofold.org/snps-and-go/snps-and-go.html) (Calabrese et al., 2009; Capriotti et al., 2013), PhD-SNP (Predictor of human Deleterious Single Nucleotide Polymorphisms; http://snps.biofold.org/phd-snp/phd-snp.html) (Capriotti et al., 2006) and SNAP2 (Screening for Non-Acceptable Polymorphism; https://www.rostlab.org/services/snap/) (Hecht et al., 2015). All these algorithms use different approaches to classify a non-synonymous single nucleotide variation as deleterious or not. The inputs for these were given either in the form of rsIDs or amino acid substitutions (AAS) corresponding to all the nsSNPs in hZP2. The deleterious nsSNPs which were common in at least 3 or 4 algorithms were chosen for further characterization.
2.3 Prediction of Structural and Functional Alterations in hZP2 Protein Caused by Deleterious nsSNPs
MutPred2 (http://mutpred.mutdb.org) was used to predict the structural and functional alterations caused by the deleterious nsSNPs. MutPred2 quantifies the pathogenicity of amino acid substitutions and categorizes them as pathogenic or benign in humans and also predicts their impact on 50 different protein properties (Pejaver et al., 2017). The protein sequence in FASTA format along with the AAS corresponding to the selected nsSNPs was submitted as input in this web server. A p-value threshold of 0.05 and a prediction score ranging between 0 and 1 was used. A higher score reflects a higher probability of pathogenicity and the possible alterations in properties were represented as gain/loss of protein structure and/or function.
2.4 Predicting the Effect of nsSNPs on the Stability of hZP2 Protein
I-Mutant2.0 (http://folding.biofold.org/i-mutant/i-mutant2.0.html) predicts the change in stability of a protein, upon single point mutation. It is based on the dataset derived from the ProTherm database which is the most inclusive database of thermodynamic experimental data of free energy changes of protein stability upon mutation under different conditions (Capriotti et al., 2005). hZP2 protein sequence in FASTA format and individual AAS corresponding to selected deleterious nsSNPs were given as input and the corresponding change in stability (Reliability Index; RI) and free energy (Kcal/mol; represented as DDG) was obtained. The RI ranges from 0–10 with 10 being the highest in reliability.
2.5 3D Modeling of Protein Structure
The 3D model of hZP2 protein was obtained using I-TASSER (https://zhanglab.ccmb.med.umich.edu/I-TASSER/) which is the most advanced protein structure prediction server. It uses LOMETS, a multiple threading approach to identify structural templates, and generates a 3D atomic model by comparing it with structurally similar known proteins (Roy et al., 2010). The amino acid sequence of hZP2 protein in FASTA format was given as input. Out of the top five models generated in output, the one with the highest C-score was selected. The model thus selected was viewed in Chimera 1.11 (Pettersen et al., 2004) which allows interactive visualization and analysis of molecular structures and related data. For looking at the effect of SNPs, all the deleterious amino acid changes were substituted manually using its rotamer function, and any new network of contacts or clashes formed were assessed. In addition, 12 mutant models of hZP2 were also generated using I-TASSER by manually substituting the amino acids in FASTA sequence of hZP2 at positions corresponding to the 12 deleterious SNPs.
2.6 Quality Assessment of the 3D Model Generation
PROCHECK (https://servicesn.mbi.ucla.edu/PROCHECK/) was used to assess the quality of the 3D model that was generated above. This program gives an evaluation of the overall quality of the structure, based on various stereochemical properties (like phi-psi angles in most favored regions of Ramachandran plot, side-chain parameters, chi1-chi2 plots, etc.) by comparing them with the well-refined protein structures of the same resolution and also highlights the regions that may need further investigation (Laskowski et al., 1993). The selected model of hZP2 in PDB format was submitted as input. The 3D model was also verified by ERRAT (https://servicesn.mbi.ucla.edu/ERRAT/) (Colovos and Yeates, 1993) which compares the statistics of non-bonded interactions of different atoms of the submitted protein model with those of highly refined structures.
TM-Align (https://zhanglab.ccmb.med.umich.edu/TM-align/) was used to calculate TM-score and RMSD values of wild type and mutant models. TM-score tells about the topological similarity between wild type and mutant models and RMSD helps in measuring the average distance between alpha-carbon backbones of wild type and mutant models (Zhang and Skolnick, 2005). The TM-score varies between 0 and 1, with a value of one representing a perfect match between two structures. The RMSD value, on the other hand, represents the deviation of mutant structure from the wild type. A higher RMSD value means greater deviation.
2.7 Predicting the Conservation Score of Amino Acid Positions Corresponding to Deleterious nsSNPs
Since the evolutionarily conserved positions in a protein are considered important in terms of its structure and function, the conservation score of all the amino acid positions corresponding to deleterious nsSNPs was calculated using ConSurf (https://consurf.tau.ac.il/) (Ashkenazy et al., 2010). This bioinformatics tool uses PSI-BLAST, CSI-BLAST, or BLAST to find the homologous sequences for the given input sequence and performs multiple sequence alignment using different programs like MAFFT, PRANK, TCOFFEE, MUSCLE, or CLUSTALW and finally gives output in the form of a score that ranges from one to nine where nine represents most conserved and one represents highly variable amino acid position.
2.8 Predicting the Putative N and O Glycosylation Sites in hZP2
Since the glycosylation sites on native human zona are not known, we used prediction software to determine this. Potential N- and O-glycosylation sites in the full-length hZP2 (1–745 aa) were predicted using NetNGlyc-1.0 (http://www.cbs.dtu.dk/services/NetNGlyc/) and NetOGlyc-4.0 (http://www.cbs.dtu.dk/services/NetOGlyc/) respectively. NetNGlyc-1.0 predicts N-linked glycosylation sites in human proteins using artificial neural networks that examine the sequence context of Asn-Xaa-Ser/Thr sequons (Gupta R, 2002). NetOGlyc-4.0 predicts O-GalNAc (mucin type) glycosylation sites in mammalian proteins using neural network predictions (Steentoft et al., 2013). Boja et al., 2003 have characterized the N- and O-linked glycosylation sites in mouse ZP2 (mZP2) using mass spectrometry of native mouse zona proteins. Using this data, manual assertions were made for glycosylation in hZP2 by aligning hZP2 and mZP2 protein sequences using Clustal Omega (Madeira et al., 2019). These manual assertions were compared with those predicted by NetNGlyc-1.0 and NetOGlyc-4.0. In addition, to analyze any deviation in terms of loss or gain of N- or O-glycosylation sites caused by the shortlisted 12 deleterious nsSNPs, FASTA sequences corresponding to the polymorphisms were analyzed using NetNGlyc-1.0 and NetOGlyc 4.0 respectively. This was done to ascertain if a change in N- or O-glycosylations resulted in altered interaction with sperm or, modified the zona structure.
2.9 Functional Predictions of Both Coding and Non-Coding SNPs
To predict the functional effect of both coding and non-coding SNPs, FuncPred (https://snpinfo.niehs.nih.gov/snpinfo/snpfunc.html) was used. This web-based server selects the SNPs from Genome Wide Association Studies (GWAS) and uses GWAS-SNP p-value data to predict the effect of SNPs on functional characteristics like splice sites, Transcription factor binding sites (TFBS), microRNA binding sites, etc. (Xu and Taylor, 2009). The rsIDs of all the validated nsSNPs (coding and non-coding) in the hZP2 gene were used as input and predictions were obtained.
3 Results
3.1 Retrieval of SNP Dataset From dbSNP Database
According to the dbSNP database, a total of 5,152 SNPs were reported in the human ZP2 gene (transcript ID: NM_003460 and protein ID: NP_003451). Out of these 5,152, only 1,069 were found to be validated. Further, among the validated, 229 SNPs were in the coding region and the remaining 840 were in the non-coding region (3’ & 5′ near gene region, 3’ & 5’ UTRs, and introns). Among the 229 coding SNPs, 165 were non-synonymous SNPs (missense; nsSNPs), 56 were synonymous (same-sense; sSNPs), four were nonsense, and four were frameshift (insertions and deletions).
3.2 18 nsSNPs in the hZP2 Gene Were Predicted to Be Deleterious
nsSNPs produce amino acid allelic variants of the gene which may affect the structure and function of the protein. Hence, the 165 nsSNPs were selected for further investigations. Of those 22 were predicted to be deleterious by the SIFT web server, with a SIFT score of ≤0.05 (Supplementary Table S1). Two of the nsSNPs, rs374388107 and rs267604453, had a score of 0 which is considered the most damaging score. Another program, PolyPhen-2 predicted 64 nsSNPs as probably/possibly damaging. Out of these 64, 30 were marked as “probably damaging” (PolyPhen score between 0.950 and 1). The remaining 34 nsSNPs were designated as “possibly damaging” (PolyPhen score between 0.850 and 0.950) (Supplementary Table S2). PROVEAN web server predicted a total of 51 out of 165 nsSNPs as deleterious, with a PROVEAN score of less than the cut-off value (-2.5) (Supplementary Table S3). According to the predictions by another algorithm SNPs&GO, only 26 nsSNPs are found to be disease-associated as they had a probability value of >0.50 which predicts disease association (Supplementary Table S4). PhD-SNP prediction classified 54 nsSNPs as disease-associated (Supplementary Table S5).
To effectively select the most deleterious nsSNPs and reduce the rate of false-positive predictions, we shortlisted 18 nsSNPs which were commonly classified as deleterious in at least 3 or 4 out of the above mentioned five algorithmic tools by manual concordance (Table 1). These were classified as deleterious nsSNPs. These deleterious nsSNPs were cross-validated for having an effect or being a neutral variant using another web-server called SNAP2, which predicted the functional effects caused by these nsSNPs. All the 18 nsSNPs were predicted as “effect variants” with highly expected accuracy, making these good candidates for further investigation (Supplementary Table S6).
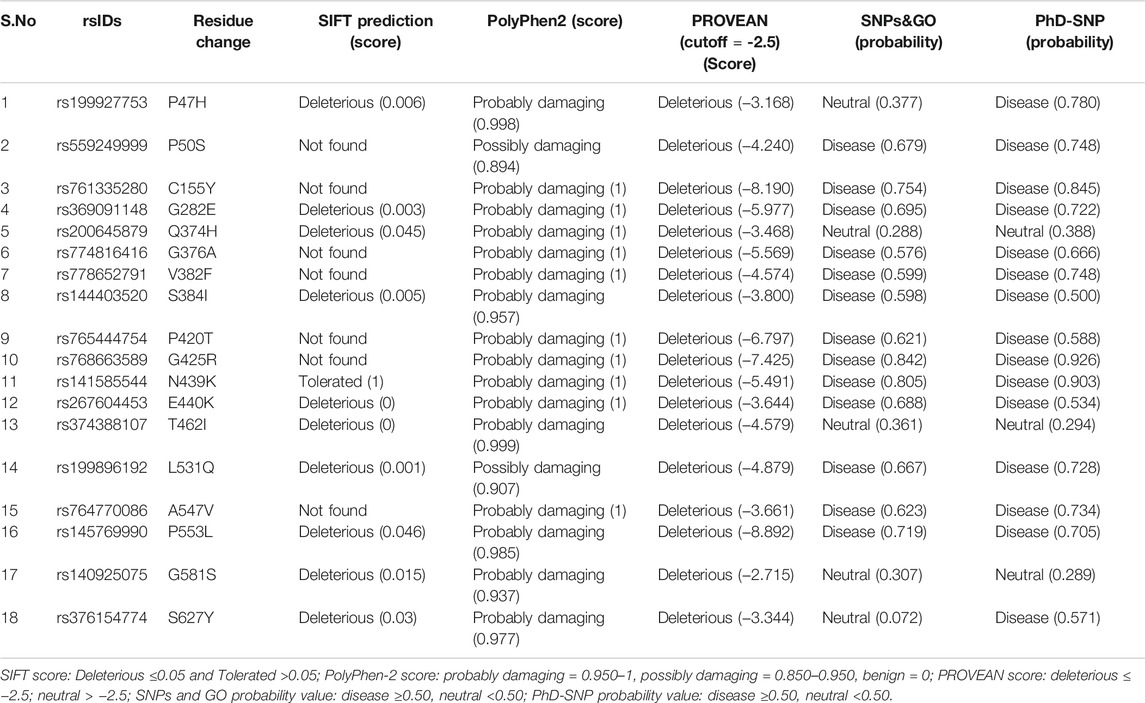
TABLE 1. List of 18 deleterious nsSNPs in hZP2 gene, as identified by five different in silico tools. The score or probability for each is mentioned within brackets.
3.3 Prediction of Functional and Structural Modifications of Deleterious nsSNPs on hZP2 Protein
Of the 18 deleterious nsSNPs submitted to MutPred2 web-server, only eight were found to score more than 0.50 and thus, were predicted to result in structural and functional alterations like change in stability of protein, gain or loss of relative solvent accessibility, loss of disulfide linkage, loss of DNA binding sites, loss of strand, an altered transmembrane protein, altered metal-binding site, etc. (Table 2). Apart from MutPred2, I-Mutant predicted the effect of these mutations on the stability of hZP2 protein. A decrease in stability was observed for 16 out of the 18 nsSNPs. The resultant free energy change (kcal/mol) and the reliability index for each of the substitutions are shown in Table 3.
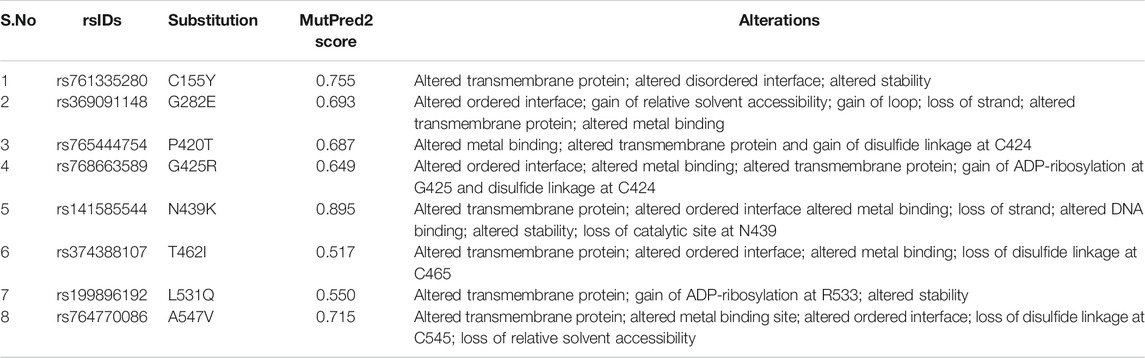
TABLE 2. List of eight deleterious nsSNPs and the resulting structural and functional alterations in hZP2 protein, as predicted by MutPred2.
3.4 Analysis of the Effect of Deleterious nsSNPs on the Structure and Function of hZP2 Protein
For predicting structural alterations caused due to nsSNPs, first, the 3D model of wild type hZP2 was generated using I-TASSER. Out of the five models generated, the model with the highest C-score of -1.76, an estimated TM-score of 0.50 ± 0.15, and an estimated RMSD of 12.5 ± 4.3Å was used for further analysis (Figure 2A). The stereo-chemical quality of the protein model was checked using the PROCHECK program based on various factors like overall G-factor, phi-psi angles, chi1-chi2 plots, side-chain parameters, etc. which were found to be within limits and thus the structure was found acceptable and worth investigating further (Figure 2B). Ramachandran plot showed 58.0% residues in the core region, 32.7% in the allowed region, 6.0% in the generously allowed region, and 3.4% in the disallowed region (Figure 2C). ERRAT2 program which verifies the quality of the protein model based on non-bonded interactions predicted the overall quality factor to be 70.21. The generally accepted range for a high-quality model is > 50.
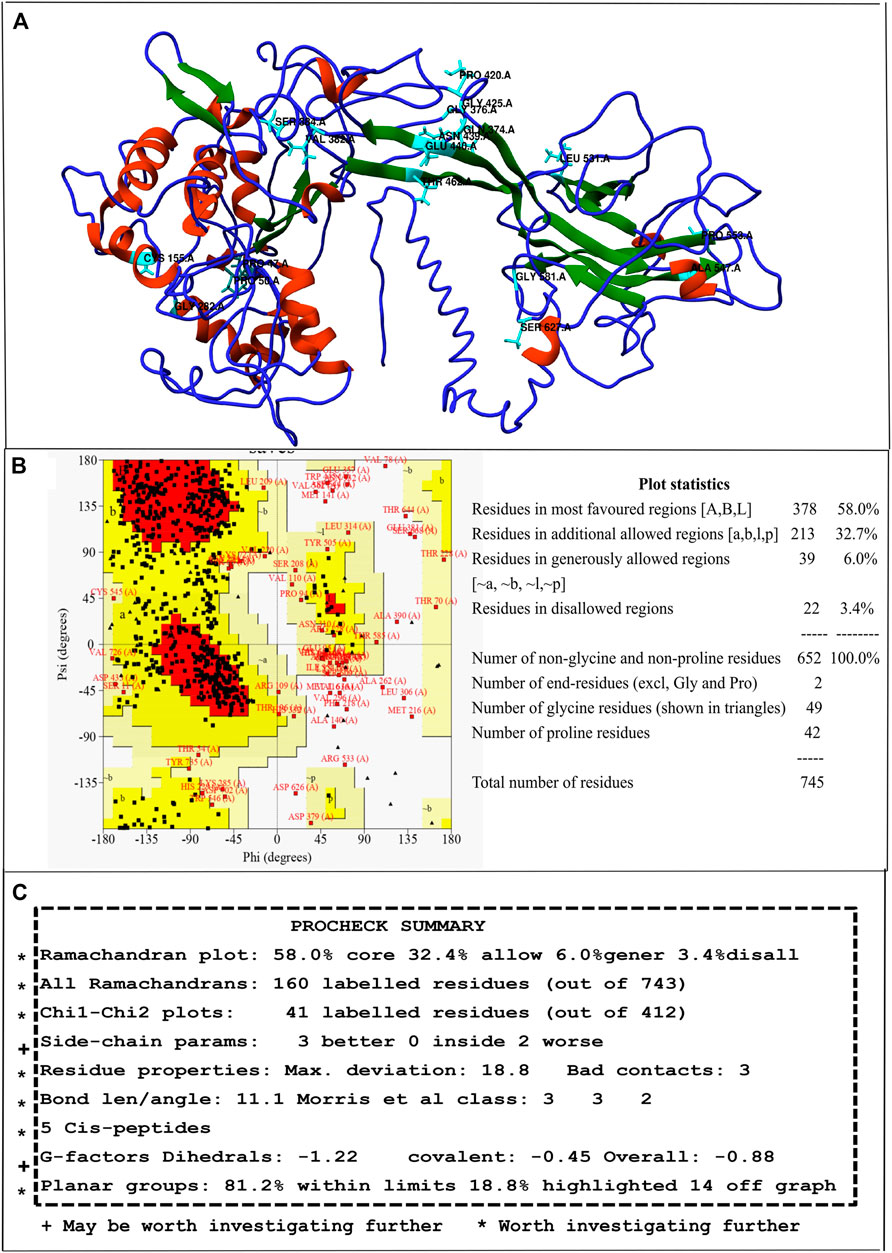
FIGURE 2. Full-length 3D model of hZP2 generated by I-TASSER. (A) Helix regions are shown in red, strand in blue, and coil in green. The positions of 18 deleterious nsSNPs are highlighted in cyan. (B) Ramachandran plot for the 3D model of hZP2 (C) Screenshot showing the summary of PROCHECK results.
The model generated above was used to study the effect of the mutations on the protein’s 3D structure using Chimera 1.11. Each of the 18 nsSNPs shortlisted above was checked to identify the formation of any new network of contacts and/clashes (Table 4). Out of the 18 nsSNPs, 12 were found to form new networks of clashes and contacts and these were used for further analysis. An example of this is shown in Figure 3 in which, Alanine547 formed no network of clashes/contacts in wild type hZP2. However, when it was replaced by Valine, five new pseudo bonds with Val611, Met595, and Trp546 were formed.
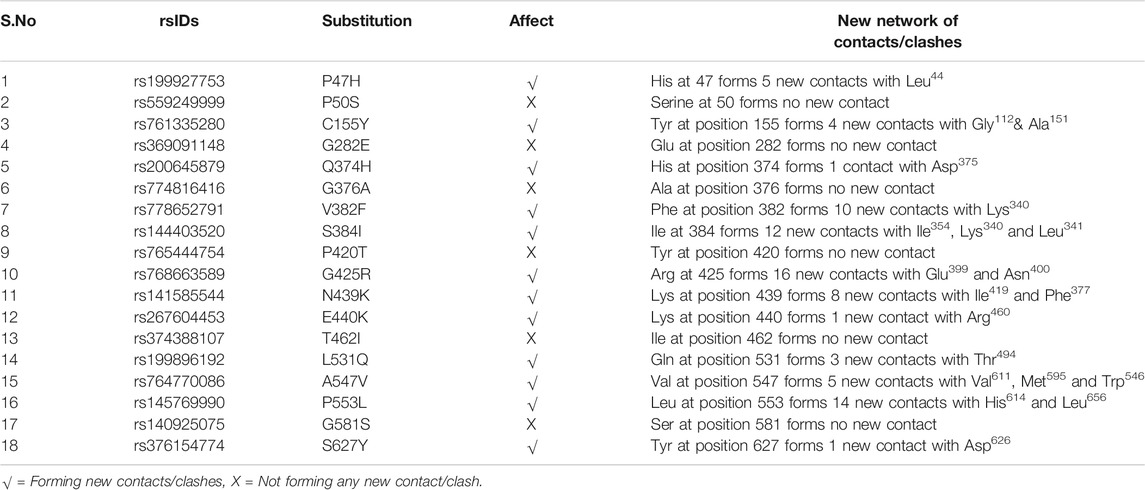
TABLE 4. List of 18 deleterious SNPs along with the possible new network of clashes/contacts formed by the substituted amino acids at the corresponding positions as predicted by Chimera 1.11.
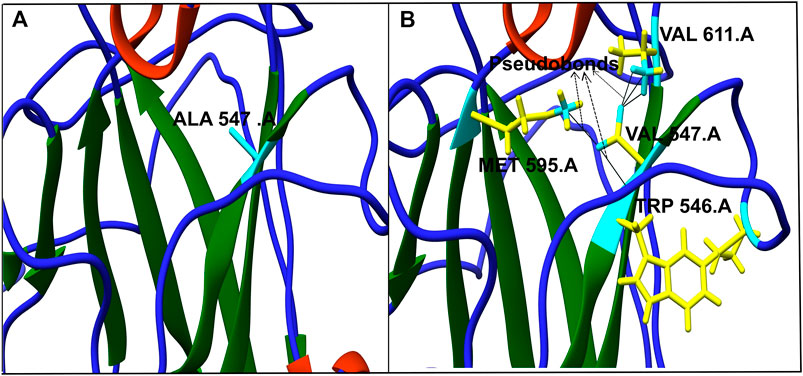
FIGURE 3. Effect of single nucleotide variation rs764770086 (A547V) on protein’s 3D structure. Alanine at position 547 (cyan) in wild protein forms no contacts in native form (A) whereas Valine at the same position (yellow and cyan) forms five new contacts with Val611, Met595, and Trp546 (B). The networks of clashes or new pseudobonds are shown as black lines.
3.5 Comparative Modeling of Wild Type and Mutant hZP2 Protein
Structural models for the 12 nsSNPs which formed new network of clashes and contacts were also generated using I-TASSER. Out of five models obtained in output for each of the 12 mutant proteins, models with the highest C-score were selected for further analysis. Finally, the wild-type and mutant models were compared using TM-align. The TM-Score and RMSD values for each mutant model are shown in Table 5. Mutant models for C155Y (rs761335280) and S384I (rs768663589) were found to have the lowest TM-score i.e. 0.22707 and 0.20968 and a high RMSD value i.e. 8.27 and 8.44 respectively, thus showing a greater deviation from wild type protein. When the conservation score of 12 amino acid positions (i.e. corresponding to 12 deleterious nsSNPs) on the protein was calculated using ConSurf, it was found that six out of 12 nsSNPs (C155Y, G425R, N439K, E440K, A547V, and S627Y) are in highly conserved positions, thus, showing their functional significance (Table 5).
3.6 Effect of SNPs on the Glycosylation Status of hZP2
NetNGlyc predicted 6 amino acid positions in the wild-type hZP2 protein (N87, N105, N122, N223, N269, and N400). When these were compared with the N glycosylation sites characterized in native mZP2, four of these (N87, N223, N269, and N400) were present in mouse too. The other two positions (N105 and N122) were specific to hZP2 (Figure 4). Out of the 12 deleterious nsSNPs, none of them was present at a predicted N-glycosylation site or caused any change in the N-glycosylation pattern due to this polymorphism.
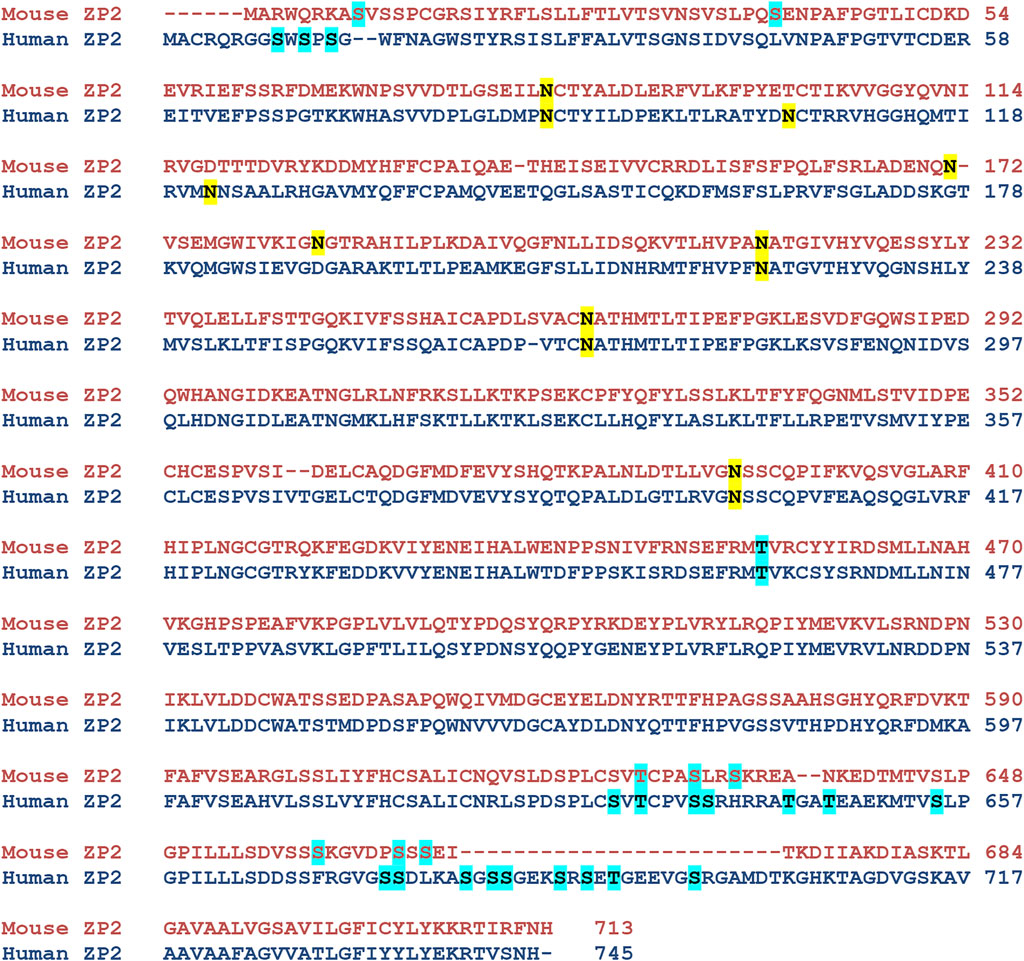
FIGURE 4. Prediction of N- and O-linked glycosylation sites in hZP2 protein. NetNGlyc-1.0 and NetOGlyc-4.0 were used to predict N-linked and O-linked glycosylation sites in hZP2 respectively. An additional O-glycosylation site at T462, based on the data from native mouse ZP2 mass spectrophotometric studies, was also assigned manually. The N-linked and O-linked glycosylation sites in mouse and human ZP2 are highlighted in yellow and cyan respectively.
NetOGlyc predicted 19 potential O-glycosylation sites (S9, S11, S13, S631, T633, S637, S638, T644, T647, S655, S674, S675, S680, S682, S683, S687, S689, T691, and S697). In addition, based on manual assertion after comparing with mouse mass spectrophotometric data, Thr462 was also assigned to be potentially glycosylated in hZP2 (Figure 4). O-linked glycosylation occurs by transferring oligosaccharides to serine and threonine residues. Among the 12 nsSNPs, only two SNPs (S384I and S627Y) translated into the amino acid substitutions where serine was being replaced. However, none including the above two were present at the predicted O-glycosylation sites. When the corresponding mutant sequence for these SNPs was analyzed by NetOGlyc-4.0, loss of glycosylation sites was observed only for C155Y (S655), V382F (S674, S691), N439K (S655), E440K (S691), L531Q (S655), A547V (S674, S682), P553L (S655, S674) and S627Y (S631, S674, S691). No loss/gain of O-glycosylation was observed for P47H, Q374H, S384I, and G425R.
3.7 31 SNPs Are Predicted to Affect hZP2 Gene Regulation
The 1,069 validated SNPs from both coding and non-coding region were also submitted to FuncPred to detect their role in the regulation of gene expression. Only 31 of these were found to have an effect. Five coding SNPs (rs16971234, rs2075520, rs2075526, rs34159042, and rs35162028) were found to affect splicing [exonic splicing enhancers (ESE) and exonic splicing silencers (ESS)] and the remaining 26 SNPs from the non-coding region were found to affect transcription factor binding sites (TFBS). Also, no SNP in the 3′UTR region was found to create or abolish the miRNA binding site. The detailed results are shown in Table 6. It is interesting to find that most of these regulatory SNPs have high global MAF values (Supplementary Figure S1).
4 Discussion
ZP2 is an important constituent of the zona matrix as ZP2 null female mice produce zona deficient oocytes and are infertile (Rankin et al., 2001). Using transgenic studies, it has been shown that the cleavage status of ZP2 determines if the egg will be recognized by sperm or not and thereafter prevent polyspermy (Gahlay et al., 2010). Any change in the amino acid sequence of ZP2 protein may affect its structural and functional properties and can thereby affect the ability of the egg to fuse with sperm and/or prevent polyspermy. This can impact the reproductive fitness of mammalian females. These changes in the amino acid sequence of ZP2 may exist naturally in any population in the form of SNPs at the genomic level and result in either a gain of function, loss of function, or no change to the protein.
Female infertility is a major issue that is usually associated with hormonal or physiological issues. However, loss of function in any of the zona proteins due to SNPs may be another contributing factor (Pökkylä et al., 2011; Huang et al., 2014; Liu et al., 2017). The availability of a vast amount of genomic data and various bioinformatics algorithms makes it possible to shortlist the SNP’s which may affect the protein structure and function and hence female fertility. Using in silico analysis, we identified 12 deleterious nsSNPs, out of a total of 1,069 SNPs, which cause structural and/or functional changes. Among these 12, two are located within the N-terminal domain of hZP2 and the remaining 10 are located within the highly conserved zona domain of the protein (Figure 5). Previous studies have demonstrated that the N-terminal region of ZP2 protein (39–154 aa) is crucial for the initial zona-sperm interaction and the zona domain (372–631 aa) participates in the structural integrity of the zona matrix by regulating the polymerization of ZP proteins (Jovine et al., 2005; Baibakov et al., 2012; Avella et al., 2014).
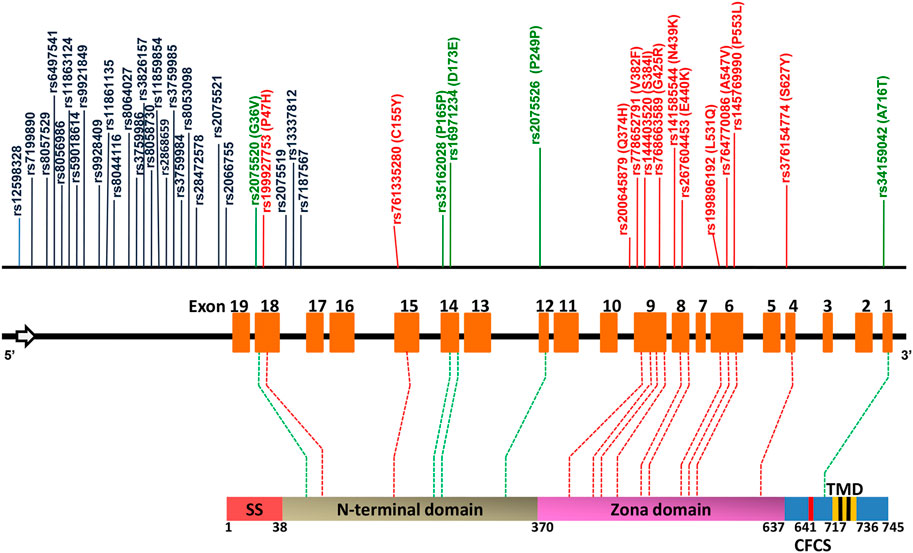
FIGURE 5. A map of hZP2 gene and hZP2 protein, highlighting the positions of the various deleterious structural, functional and regulatory SNPs predicted by in silico analysis. Regulatory SNPs in the non-coding region are marked in black while those in the coding region are marked in green. Deleterious nsSNPs are marked in red. The sperm binding domain (aa 51–149) is present within the N-terminal domain. CFCS = consensus furin cleavage site; SS = signal sequence; TMD = transmembrane domain.
The two nsSNPs present in the N-terminal region associated with zona-sperm interaction are rs199927753 and rs761335280. In rs199927753 (P47H) the small, non-reactive amino acid Pro is altered to His, a polar amino acid that can transfer protons on and off with ease, and in rs761335280 (C155Y), a Cys is substituted with a hydrophobic Tyr whose reactive hydroxyl group now makes it more likely for it to be involved in interactions with non-carbon atoms which was not earlier possible with Cys. These changes are predicted to affect the zona-sperm interaction (Table 7). It is important to note that the C155Y position is highly conserved suggesting its importance in this process.
The remaining 10 nsSNPs are present in the zona domain of the protein and are probably affecting the structural integrity of the ZP matrix by altering the zona domain’s polymerization. This may be one of the background factors for causing various types of zona anomalies. The predicted structural changes due to amino acid substitutions in rs200645879 (Q374H), rs778652791 (V382F), rs144403520 (S384I), rs267604453 (E440K), rs768663589 (G425R), rs141585544 (N439K), rs199896192 (L531Q), rs764770086 (A547V), rs145769990 (P553L), and rs376154774 (S627Y) have been discussed in Table 7. Interestingly, the substitution in the SNP rs764770086 (A547V) is predicted to cause loss of disulfide linkage in the neighboring Cys (Cys545). The substitution in rs768663589 (G425R), on the other hand, predicts a gain of disulfide linkage at Cys424. ZP2 is rich in disulfide linkages and the gain or loss of these can cause structural changes affecting sperm-egg interaction.
Amongst these nsSNPs, the most deleterious SIFT and PolyPhen-2 score was observed with rs267604453 (E440K). Similarly, rs145769990 (P553L) seems to be an important SNP as it was predicted to be deleterious or disease linked by all the five algorithmic tools (SIFT, PolyPhen-2, PROVEAN, SNPs&GO, and PhD-SNP) that were used in the study (Table 1). Six of these (C155Y, G425R, N439K, E440K, A547V, and S627Y) are present in highly conserved positions.
ZP proteins are differentially glycosylated with Asn (N-) and Ser/Thr (O-) linked glycosylation. Several studies have implicated these glycans in either sperm-ZP interaction or in imparting structural characteristics to zona which makes the ZP available to the sperm receptors to bind to, or in imparting species specificity to this process (Yonezawa et al., 2007; Pang et al., 2011; Chiu et al., 2014; Clark, 2014). Based on these results, it can be hypothesized that the absence of glycans can result in changes that either affect the interaction between the egg and sperm, or its structure. In our predictions, we observed a changed glycosylation pattern for only O-glycans and except for S631, all other O-glycosylation sites which were lost were present downstream of aa 640. A propeptide corresponding to 641–745 aa is removed in mature hZP2. Hence, loss of these glycosylation sites will have no major effect either on sperm interaction or on the structure of the zona. Only S631 may be involved but that needs to be confirmed especially in the light of the fact that even though NetOGlyc predicted eight O-glycosylation sites for mouse ZP2 (S9, S40, T626, S630, S633, S660, S666, S668), mass spectrophotometric analysis on native mouse zona found only a single O-glycosylated site (T455) which was otherwise absent in the prediction (Boja et al., 2003).
In addition to these 12 deleterious nsSNPs, we also identified 31 regulatory SNPs that may affect the expression of the hZP2 gene at the transcription or translation level. A total of 26 regulatory SNPs (out of 31) are present in the non-coding region of the gene and are predicted to affect the transcription factor binding sites (TFBS) (Table 6). These SNPs were found to have a high global MAF value which signifies their occurrence in the population at a high frequency. Five SNPs (rs16971234, rs2075520, rs2075526, rs34159042, and rs35162028) from the coding region were predicted to affect splicing by acting either as exonic splicing enhancers (ESE) or exonic splicing silencers (ESS). These splicing regulatory elements (ESE or ESS) function by enrolling trans-acting splicing elements which can enhance or suppress the splice-site recognition and/or spliceosome assembly by various mechanisms resulting in a different mRNA transcript (Matlin et al., 2005). Most of these SNPs are located in the 5′ near gene region or in introns close to the 5′end of the gene.
It was interesting to find that among the five coding regulatory SNPs, one with rsID rs16971234 encodes for the substitution of Asp at position 173 with Glu. This nsSNP has a global MAF value of 0.2831 as per 1,000 genome project validation which points towards the high occurrence of this polymorphism in the population. The SNP is present in the N-terminal region which has been recognized as the cleavage site for the metalloprotease Ovastacin (Burkart et al., 2012). Altered splicing due to this SNP can result in a change in the cleavage site because of which Ovastacin cannot act. Transgenic mice studies in which the cleavage site was altered resulted in eggs where the ZP2 could not be cleaved post-fertilization and sperm continued to bind (Gahlay et al., 2010). These females were also found to have very low fertility rates. Also, this is a highly conserved position among mammals (Burkart et al., 2012). It will be interesting to study if this polymorphism in females also results in infertility. Two of the other predicted regulatory SNPs rs2075521 and rs2075526 have also been identified in a study conducted on three women with recurrent oocyte lysis during their IVF attempts (Ferré et al., 2014). Another regulatory SNP rs2075520 has been found in another study on patients with zona anomalies (Pökkylä et al., 2011). Thus, these studies support our results regarding the association of predicted deleterious SNPs with the reproductive fitness of females.
It has been hypothesized that multiple low-affinity binding sites may be involved in oocyte-sperm interaction, as this may be the natural way of preventing complete fertilization failure by numerous de novo generated nucleotide changes (Castle, 2002). Thus, it is possible that at the population level, several SNPs individually or in combination with others may affect fertility. However, further, experimental investigations are necessary to confirm the deleterious status of these SNPs at the population level. The identification and characterization of these will help in explaining the etiology behind various types of zona anomalies and unexplained infertility in human females and could potentiate their use in the diagnosis of female infertility.
Data Availability Statement
The datasets presented in this study can be found in online repositories. The names of the repository/repositories and accession number(s) can be found in the article/Supplementary Material.
Author Contributions
Author Contributions: Conceptualization, GG; methodology, NR and GG; formal analysis, NR; investigation, NR; writing—original draft preparation, NR; writing—review and editing, GG; supervision, GG; project administration, GG; funding acquisition, GG. All authors have read and agreed to the published version of the manuscript.
Funding
This research received no external funding. However, funding from the DBT-BioCARe grant (BT-BioCARe/01/9770/2013-14) and grant allocated under the RUSA 2.0 Scheme of UGC was available to support the authors.
Conflict of Interest
The authors declare that the research was conducted in the absence of any commercial or financial relationships that could be construed as a potential conflict of interest.
Publisher’s Note
All claims expressed in this article are solely those of the authors and do not necessarily represent those of their affiliated organizations, or those of the publisher, the editors and the reviewers. Any product that may be evaluated in this article, or claim that may be made by its manufacturer, is not guaranteed or endorsed by the publisher.
Supplementary Material
The Supplementary Material for this article can be found online at: https://www.frontiersin.org/articles/10.3389/fcell.2021.763166/full#supplementary-material
Supplementary Figure 1 | A graphical representation of global MAF values of 31 SNPs predicted to effect the regulation of hZP2 by FuncPred. These 31 SNPs include 5 coding (green) and 26 non-coding SNPs (blue).
References
Adzhubei, I. A., Schmidt, S., Peshkin, L., Ramensky, V. E., Gerasimova, A., Bork, P., et al. (2010). A Method and Server for Predicting Damaging Missense Mutations. Nat. Methods 7, 248–249. doi:10.1038/nmeth0410-248
Adzhubei, I., Jordan, D. M., and Sunyaev, S. R. (2013). Predicting Functional Effect of Human Missense Mutations Using PolyPhen‐2. Curr. Protoc. Hum. Genet. 76. doi:10.1002/0471142905.hg0720s76
Ashkenazy, H., Erez, E., Martz, E., Pupko, T., and Ben-Tal, N. (2010). ConSurf 2010: Calculating Evolutionary Conservation in Sequence and Structure of Proteins and Nucleic Acids. Nucleic Acids Res. 38, W529–W533. doi:10.1093/nar/gkq399
Avella, M. A., Baibakov, B., and Dean, J. (2014). A Single Domain of the ZP2 Zona Pellucida Protein Mediates Gamete Recognition in Mice and Humans. J. Cel. Biol. 205, 801–809. doi:10.1083/jcb.201404025
Baibakov, B., Boggs, N. A., Yauger, B., Baibakov, G., and Dean, J. (2012). Human Sperm Bind to the N-Terminal Domain of ZP2 in Humanized Zonae Pellucidae in Transgenic Mice. J. Cel. Biol. 197, 897–905. doi:10.1083/jcb.201203062
Boja, E. S., Hoodbhoy, T., Fales, H. M., and Dean, J. (2003). Structural Characterization of Native Mouse Zona Pellucida Proteins Using Mass Spectrometry. J. Biol. Chem. 278, 34189–34202. doi:10.1074/jbc.M304026200
Burkart, A. D., Xiong, B., Baibakov, B., Jiménez-Movilla, M., and Dean, J. (2012). Ovastacin, a Cortical Granule Protease, Cleaves ZP2 in the Zona Pellucida to Prevent Polyspermy. J. Cel. Biol. 197, 37–44. doi:10.1083/jcb.201112094
Calabrese, R., Capriotti, E., Fariselli, P., Martelli, P. L., and Casadio, R. (2009). Functional Annotations Improve the Predictive Score of Human Disease-Related Mutations in Proteins. Hum. Mutat. 30, 1237–1244. doi:10.1002/humu.21047
Capriotti, E., Calabrese, R., and Casadio, R. (2006). Predicting the Insurgence of Human Genetic Diseases Associated to Single point Protein Mutations with Support Vector Machines and Evolutionary Information. Bioinformatics 22, 2729–2734. doi:10.1093/bioinformatics/btl423
Capriotti, E., Calabrese, R., Fariselli, P., Martelli, P., Altman, R. B., and Casadio, R. (2013). WS-SNPs&GO: a Web Server for Predicting the Deleterious Effect of Human Protein Variants Using Functional Annotation. BMC Genomics 14, S6. doi:10.1186/1471-2164-14-s3-s6
Capriotti, E., Fariselli, P., and Casadio, R. (2005). I-Mutant2.0: Predicting Stability Changes upon Mutation from the Protein Sequence or Structure. Nucleic Acids Res. 33, W306–W310. doi:10.1093/nar/gki375
Castle, P. (2002). Could Multiple Low-Affinity Bonds Mediate Primary Sperm-Zona Pellucida Binding? Reproduction 124, 29–32. doi:10.1530/rep.0.1240029
Chen, Y., and Fang, S.-y. (2018). Potential Genetic Polymorphisms Predicting Polycystic Ovary Syndrome. Endocr. Connect. 7, R187–R195. doi:10.1530/EC-18-0121
Chiu, P. C. N., Lam, K. K. W., Wong, R. C. W., and Yeung, W. S. B. (2014). The Identity of Zona Pellucida Receptor on Spermatozoa: an Unresolved Issue in Developmental Biology. Semin. Cel. Dev. Biol. 30, 86–95. doi:10.1016/J.SEMCDB.2014.04.016
Chiu, P. C. N., Wong, B. S. T., Lee, C. L., Pang, R. T. K., Lee, K.-F., Sumitro, S. B., et al. (2008). Native Human Zona Pellucida Glycoproteins: Purification and Binding Properties. Hum. Reprod. 23, 1385–1393. doi:10.1093/humrep/den047
Choi, Y., and Chan, A. P. (2015). PROVEAN Web Server: A Tool to Predict the Functional Effect of Amino Acid Substitutions and Indels. Bioinformatics 31, 2745–2747. doi:10.1093/bioinformatics/btv195
Choi, Y., Sims, G. E., Murphy, S., Miller, J. R., and Chan, A. P. (2012). Predicting the Functional Effect of Amino Acid Substitutions and Indels. PLoS One 7, e46688. doi:10.1371/journal.pone.0046688
Clark, G. F. (2014). A Role for Carbohydrate Recognition in Mammalian Sperm-Egg Binding. Biochem. Biophysical Res. Commun. 450, 1195–1203. doi:10.1016/J.BBRC.2014.06.051
Colovos, C., and Yeates, T. O. (1993). Verification of Protein Structures: Patterns of Nonbonded Atomic Interactions. Protein Sci. 2, 1511–1519. doi:10.1002/pro.5560020916
Ferré, M., Amati-Bonneau, P., Morinière, C., Ferré-L’Hôtellier, V., Lemerle, S., Przyrowski, D., et al. (2014). Are Zona Pellucida Genes Involved in Recurrent Oocyte Lysis Observed during In Vitro Fertilization? J. Assist. Reprod. Genet. 31, 221–227. doi:10.1007/s10815-013-0141-8
Gahlay, G., Gauthier, L., Baibakov, B., Epifano, O., and Dean, J. (2010). Gamete Recognition in Mice Depends on the Cleavage Status of an Egg's Zona Pellucida Protein. Science 329, 216–219. doi:10.1126/science.1188178
Gupta, R., and Brunak, S. (2002). Prediction of Glycosylation across the Human Proteome and the Correlation to Protein Function. Pac. Symp. Biocomput, 310–322.
Hecht, M., Bromberg, Y., and Rost, B. (2015). Better Prediction of Functional Effects for Sequence Variants. BMC Genomics 16, S1. doi:10.1186/1471-2164-16-S8-S1
Huang, H.-L., Lv, C., Zhao, Y.-C., Li, W., He, X.-M., Li, P., et al. (2014). Mutant ZP1 in Familial Infertility. N. Engl. J. Med. 370, 1220–1226. doi:10.1056/nejmoa1308851
Jovine, L., Darie, C. C., Litscher, E. S., and Wassarman, P. M. (2005). Zona Pellucida Domain Proteins. Annu. Rev. Biochem. 74, 83–114. doi:10.1146/annurev.biochem.74.082803.133039
Kumar, P., Henikoff, S., and Ng, P. C. (2009). Predicting the Effects of Coding Non-synonymous Variants on Protein Function Using the SIFT Algorithm. Nat. Protoc. 4, 1073–1081. doi:10.1038/nprot.2009.86
Laskowski, R. A., MacArthur, M. W., Moss, D. S., and Thornton, J. M. (1993). PROCHECK: a Program to Check the Stereochemical Quality of Protein Structures. J. Appl. Cryst. 26, 283–291. doi:10.1107/s0021889892009944
Lefièvre, L., Conner, S. J., Salpekar, A., Olufowobi, O., Ashton, P., Pavlovic, B., et al. (2004). Four Zona Pellucida Glycoproteins Are Expressed in the Human. Hum. Reprod. 19, 1580–1586. doi:10.1093/humrep/deh301
Li, L., Sha, Y., Wang, X., Li, P., Wang, J., Kee, K., et al. (2017). Whole-exome Sequencing Identified a Homozygous BRDT Mutation in a Patient with Acephalic Spermatozoa. Oncotarget 8, 19914–19922. doi:10.18632/oncotarget.15251
Liu, W., Li, K., Bai, D., Yin, J., Tang, Y., Chi, F., et al. (2017). Dosage Effects of ZP2 and ZP3 Heterozygous Mutations Cause Human Infertility. Hum. Genet. 136, 975–985. doi:10.1007/s00439-017-1822-7
Madeira, F., Park, Y. m., Lee, J., Buso, N., Gur, T., Madhusoodanan, N., et al. (2019). The EMBL-EBI Search and Sequence Analysis Tools APIs in 2019. Nucleic Acids Res. 47, W636–W641. doi:10.1093/NAR/GKZ268
Männikkö, M., Törmälä, R.-M., Tuuri, T., Haltia, A., Martikainen, H., Ala-Kokko, L., et al. (2005). Association between Sequence Variations in Genes Encoding Human Zona Pellucida Glycoproteins and Fertilization Failure in IVF. Hum. Reprod. 20, 1578–1585. doi:10.1093/humrep/deh837
Matlin, A. J., Clark, F., and Smith, C. W. J. (2005). Understanding Alternative Splicing: Towards a Cellular Code. Nat. Rev. Mol. Cel. Biol. 6, 386–398. doi:10.1038/nrm1645
Ng, P. C., and Henikoff, S. (2001). Predicting Deleterious Amino Acid Substitutions. Genome Res. 11, 863–874. doi:10.1101/gr.176601
Niroula, A., and Vihinen, M. (2016). Variation Interpretation Predictors: Principles, Types, Performance, and Choice. Hum. Mutat. 37, 579–597. doi:10.1002/humu.22987
Pang, P.-C., Chiu, P. C. N., Lee, C.-L., Chang, L.-Y., Panico, M., Morris, H. R., et al. (2011). Human Sperm Binding Is Mediated by the Sialyl-Lewis X Oligosaccharide on the Zona Pellucida. Science 333, 1761–1764. doi:10.1126/SCIENCE.1207438
Pejaver, V., Urresti, J., Lugo-Martinez, J., Pagel, K. A., Lin, G. N., Nam, H. J., et al. (2020). Inferring the molecular and phenotypic impact of amino acid variants with MutPred2. Nat. Commun. 11, 5918. doi:10.1038/s41467-020-19669-x
Peterson, T. A., Doughty, E., and Kann, M. G. (2013). Towards Precision Medicine: Advances in Computational Approaches for the Analysis of Human Variants. J. Mol. Biol. 425, 4047–4063. doi:10.1016/j.jmb.2013.08.008
Pettersen, E. F., Goddard, T. D., Huang, C. C., Couch, G. S., Greenblatt, D. M., Meng, E. C., et al. (2004). UCSF Chimera? A Visualization System for Exploratory Research and Analysis. J. Comput. Chem. 25, 1605–1612. doi:10.1002/jcc.20084
Pökkylä, R.-M., Lakkakorpi, J. T., Nuojua-Huttunen, S. H., and Tapanainen, J. S. (2011). Sequence Variations in Human ZP Genes as Potential Modifiers of Zona Pellucida Architecture. Fertil. Sterility 95, 2669–2672. doi:10.1016/j.fertnstert.2011.01.168
Rajasekaran, R., Sudandiradoss, C., Doss, C. G. P., and Sethumadhavan, R. (2007). Identification and In Silico Analysis of Functional SNPs of the BRCA1 Gene. Genomics 90, 447–452. doi:10.1016/j.ygeno.2007.07.004
Rankin, T. L., O'Brien, M., Lee, E., Wigglesworth, K., Eppig, J., and Dean, J. (2001). Defective Zonae Pellucidae in Zp2-Null Mice Disrupt Folliculogenesis, Fertility and Development. Development 128, 1119–1126. doi:10.1242/dev.128.7.1119
Roy, A., Kucukural, A., and Zhang, Y. (2010). I-TASSER: A Unified Platform for Automated Protein Structure and Function Prediction. Nat. Protoc. 5, 725–738. doi:10.1038/nprot.2010.5
Schröder, N., and Schumann, R. (2005). Single Nucleotide Polymorphisms of Toll-like Receptors and Susceptibility to Infectious Disease. Lancet Infect. Dis. 5, 156–164. doi:10.1016/S1473-3099(05)01308-310.1016/s1473-3099(05)70023-2
Steentoft, C., Vakhrushev, S. Y., Joshi, H. J., Kong, Y., Vester-Christensen, M. B., Schjoldager, K. T.-B. G., et al. (2013). Precision Mapping of the Human O-GalNAc Glycoproteome through SimpleCell Technology. EMBO J. 32, 1478–1488. doi:10.1038/EMBOJ.2013.79
Willer, C. J., Bonnycastle, L. L., Conneely, K. N., Duren, W. L., Jackson, A. U., Scott, L. J., et al. (2007). Screening of 134 Single Nucleotide Polymorphisms (SNPs) Previously Associated with Type 2 Diabetes Replicates Association with 12 SNPs in Nine Genes. Diabetes 56, 256–264. doi:10.2337/db06-0461
Xu, Z., and Taylor, J. A. (2009). SNPinfo: Integrating GWAS and Candidate Gene Information into Functional SNP Selection for Genetic Association Studies. Nucleic Acids Res. 37, W600–W605. doi:10.1093/nar/gkp290
Yonezawa, N., Kanai, S., and Nakano, M. (2007). Structural Significance of N-Glycans of the Zona Pellucida on Species-Selective Recognition of Spermatozoa between Pig and Cattle. Soc. Reprod. Fertil. Suppl. 63, 217–228.
Zhang, S., Tang, Q., Wu, W., Yuan, B., Lu, C., Xia, Y., et al. (2014). Association between DAZL Polymorphisms and Susceptibility to Male Infertility: Systematic Review with Meta-Analysis and Trial Sequential Analysis. Sci. Rep. 4. doi:10.1038/srep04642
Zhang, Y., and Skolnick, J. (2005). TM-align: A Protein Structure Alignment Algorithm Based on the TM-Score. Nucleic Acids Res. 33, 2302–2309. doi:10.1093/nar/gki524
Zhao, M., and Dean, J. (2002). The Zona Pellucida in Folliculogenesis, Fertilization and Early Development. Rev. Endocr. Metab. Disord. 3, 19–26. doi:10.1023/A:1012744617241
Keywords: human ZP2, fertilization, SNP, in silico study, female infertility
Citation: Rajput N and Gahlay GK (2021) Identification and in silico Characterization of Deleterious Single Nucleotide Variations in Human ZP2 Gene. Front. Cell Dev. Biol. 9:763166. doi: 10.3389/fcell.2021.763166
Received: 23 August 2021; Accepted: 25 October 2021;
Published: 17 November 2021.
Edited by:
Matteo Avella, University of Tulsa, United StatesReviewed by:
Bart Gadella, Utrecht University, NetherlandsHitoshi Nishimura, Setsunan University, Japan
Copyright © 2021 Rajput and Gahlay. This is an open-access article distributed under the terms of the Creative Commons Attribution License (CC BY). The use, distribution or reproduction in other forums is permitted, provided the original author(s) and the copyright owner(s) are credited and that the original publication in this journal is cited, in accordance with accepted academic practice. No use, distribution or reproduction is permitted which does not comply with these terms.
*Correspondence: Gagandeep Kaur Gahlay, Z2FnYW5kZWVwZ2FobGF5QGduZHUuYWMuaW4=