- 1Laboratory of Radiation Oncology and Radiobiology, Fujian Medical University Cancer Hospital and Fujian Cancer Hospital, Fuzhou, China
- 2Fujian Provincial Key Laboratory of Translational Cancer Medicine, Fuzhou, China
- 3Department of Biological Sciences, Columbia University, New York, NY, United States
- 4Department of Radiotherapy, Fujian Medical University Cancer Hospital and Fujian Cancer Hospital, Fuzhou, China
- 5Bio-Med Big Data Center, Shanghai Institute of Nutrition and Health, University of Chinese Academy of Sciences, Chinese Academy of Sciences, Shanghai, China
Non-coding RNAs have been shown to play important regulatory roles, notably in cancer development. In this study, we investigated the role of microRNAs and circular RNAs in Nasopharyngeal Carcinoma (NPC) by constructing a circRNA-miRNA-mRNA co-expression network and performing differential expression analysis on mRNAs, miRNAs, and circRNAs. Specifically, the Epstein-Barr virus (EBV) infection has been found to be an important risk factor for NPC, and potential pathological differences may exist for EBV+ and EBV- subtypes of NPC. By comparing the expression profile of non-cancerous immortalized nasopharyngeal epithelial cell line and NPC cell lines, we identified differentially expressed coding and non-coding RNAs across three groups of comparison: cancer vs. non-cancer, EBV+ vs. EBV- NPC, and metastatic vs. non-metastatic NPC. We constructed a ceRNA network composed of mRNAs, miRNAs, and circRNAs, leveraging co-expression and miRNA target prediction tools. Within the network, we identified the regulatory ceRNAs of CDKN1B, ZNF302, ZNF268, and RPGR. These differentially expressed axis, along with other miRNA-circRNA pairs we identified through our analysis, helps elucidate the genetic and epigenetic changes central to NPC progression, and the differences between EBV+ and EBV- NPC.
Introduction
Nasopharyngeal carcinoma (NPC) is an Epstein-Barr virus (EBV) associated malignancy with a characteristic geographical distribution. Globally, NPC is a rare condition, with less than one case per 100,000 people per year. However, the occurrence of NPC is much more common among the populations in Southern China and Southeast Asia, with up to 25–50 cases per 100,000 people per year (Jain et al., 2016). Ethnic Chinese born in North America develop NPC less frequently compared to those in Southern China, implying that both genetic susceptibility and environmental factors contribute to the development of NPC (Buell, 1974). Ample evidence shown that infection of EBV is a risk factor for NPC (Tsao et al., 2017; Chan et al., 2018). For instance, EBV genome and gene products are detected in virtually all tumors in NPC-endemic areas. Increased levels of IgA antibodies against EBV antigens, among other EBV-related biomarkers, have been used for early detection and screening for NPC in a few high-incidence areas (Shotelersuk et al., 2000; Li et al., 2018; Wu et al., 2018). Nevertheless, while many risk factors have been established, our understanding of the molecular regulatory mechanisms that lead to the development of NPC is limited.
The role of various non-coding RNAs in the regulation of many biological pathways and functions have been widely explored and verified in recent years. MicroRNAs (miRNAs) are non-coding RNAs around 22 nucleotides in length that play important regulatory roles, specifically by inhibiting gene expression through cleavage of mRNA or translational repression (Bartel, 2004). In addition to mRNAs, miRNAs interact with any target RNAs that contain complementary sites known as miRNA response elements (MREs). Since miRNAs can bind to multiple targets, the ceRNA hypothesis was proposed, stating that these target RNAs compete for a limited amount of miRNA (Salmena et al., 2011). In other words, the amount of ceRNAs can collectively impact the degree to which miRNAs regulate gene expression.
Circular RNAs (circRNAs) is a type of non-coding RNA structured as a covalently-bonded closed continuous loop, where the 5’-cap and 3’-poly-A tail are joined together. Studies have found circRNAs to play important regulatory roles in NPC growth and metastasis (Chen et al., 2019; Guo et al., 2019; Zhu et al., 2019; Yang et al., 2020). It is widely accepted that circRNAs inhibit target miRNA activity through a miRNA sponge mechanism, which in turn results in an upregulation of target gene expression (Panda, 2018; Zu et al., 2020). A study by Zhu et al. showed that highly expressed circ-ZNF609 absorbs microRNA-150-5p to upregulate Sp1 expression, which in turn promotes the proliferation and metastatic ability of NPC (Zhu et al., 2019). Another study by Yang et al. utilized a circRNA-miRNA-target gene network to reveal potential mechanism between circKITLG and miR-3198 (Yang et al., 2020). Furthermore, studies have shown the circRNA expression is tissue specific (Memczak et al., 2013; You et al., 2015). In short, the interactions between circRNAs and miRNAs have significant influence on key genes, and as a consequence affect the development and progression of cancer (Peng and Croce, 2016; Hirono et al., 2019; Hoey et al., 2019; Hong et al., 2019; Liu et al., 2020).
To analyze and illustrate this complicated collection of interactions, we constructed a circRNA-miRNA-mRNA network. We also performed differential expression (DE) analysis on three groups of comparison: cancerous vs. non-cancerous, EBV+ vs. EBV- cell lines, and metastatic vs. non-metastatic samples. Using these results, we identified axes of circRNA-miRNA-mRNA that are differentially expressed in each group of comparison. We identified 6 differentially expressed circRNA-miRNA-mRNA axes between EBV+ and EBV- cell lines, out of which we highlighted the hsa_circ_0008129/miR-221-3p/CDKN1B axis. Through this research, we identified several potential ceRNA axes that regulate NPC progression or EBV associated traits in NPC. These ceRNA pathways can help better understand the molecular landscape of NPC, and help guide therapeutic efforts.
Results
Overview of Computational Approach
We obtained the mRNA, miRNA, and circRNA expression profile for four cancer cell lines and four patient samples. We then performed differential expression (DE) analysis and constructed RNA interaction networks to isolate circRNA-miRNA-mRNA axes of interest. Simultaneously, we performed functional enrichment of RNAs of interest to reveal relevant pathways in the pathology of NPC. We provided a graphical outline of the computational workflow in Figure 1. Lastly, we cross-referenced our analysis results with two publicly available NPC RNA-seq datasets, GSE143797 (Yang et al., 2020) and GSE118721 (Lin et al., 2018), to highlight intersecting DE-RNAs.
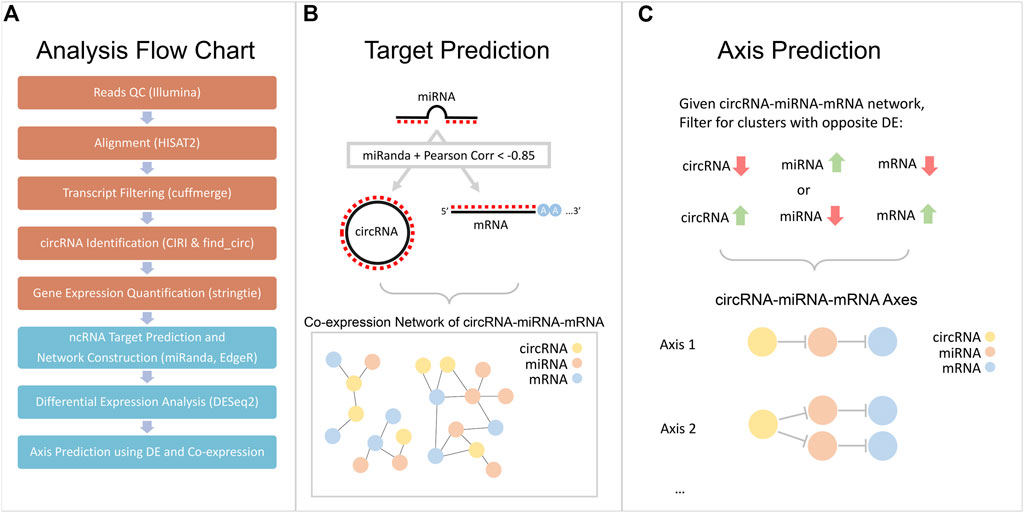
FIGURE 1. Flowchart of computational workflow. (A) Four cell lines and two patient samples were sequenced and processed to obtain an expression quantification for all three types of RNAs: circRNA, miRNA, and mRNA. (B) A graphical illustration of the construction of a co-expression network using Pearson correlation and miRanda target prediction. (C) A graphical illustration of axis prediction method, utilizing DE results and co-expression network.
Differential Expression Analysis and Functional Enrichment Analysis
DE analysis on mRNA, miRNA, and circRNA was performed across four cell lines, falling under three groups of comparison: cancer (C666, CNE-2, SUNE-1) vs. non-cancer (NP69); EBV+ (C666) vs. EBV- (CNE-2, SUNE-1); and metastatic vs. non-metastatic using patient samples. In the cancer vs. non-cancer and EBV+ vs EBV- DE comparisons, where more than one set of DE analysis was performed, we focused on the intersecting differentially expressed mRNAs, miRNAs, circRNAs (DE-mRNAs, DE-miRNAs, DE-circRNAs). We plotted the top 10 overexpressed and under-expressed DE-RNA by log2 fold change. The summary of the DE analysis results across cell lines and samples for mRNA, miRNA, and circRNA was shown respectively in Figure 2; Figure 3; Figure 4. The entire DE analysis results can be found in Supplementary Table S1; Supplementary Table S2; Supplementary Table S3 which contains all DE analysis results of cancer vs. non-cancer cell lines, EBV+ vs. EBV- cell lines, metastatic vs. non-metastatic patient samples, respectively.
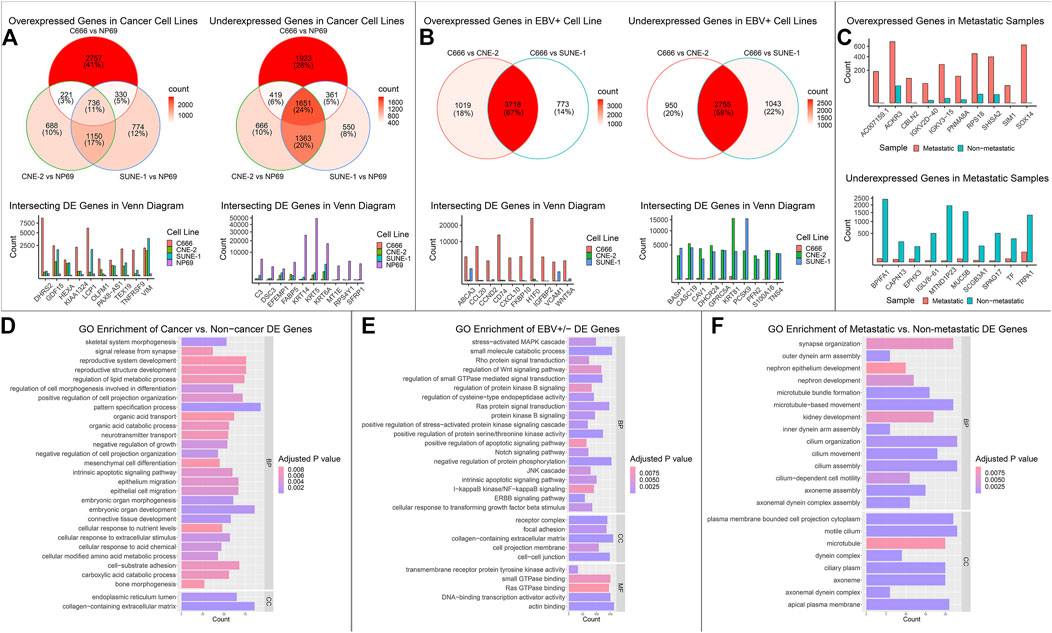
FIGURE 2. Overview of mRNA landscape across comparisons. The differential expression (DE) results for mRNAs across the three groups of comparison: cancer vs. non-cancer, EBV + vs. EBV-, and metastatic vs non-metastatic, were integrated into one figure. (A) The cancer vs. non-cancer group consisted of three sets of DE analysis: NP69 (non-cancer) vs. C666, CNE-2, and SUNE-1 (cancer). The expression of top DE genes that intersected all three sets of DE analysis was plotted. (B) The EBV+ vs. EBV- group consisted of two sets of DE analysis: C666 (EBV+) vs. CNE-2, SUNE-1 (EBV-). The expression of intersecting DE genes was plotted. (C) Since there was only one set of DE analysis, the expression of top DE genes was plotted. (D–F) The intersecting DE genes in each comparison were functionally enriched using the GO database, and top enriched pathways were plotted. The x-axis value represented the gene count in the corresponding pathway, and the color of the bar represented the adjusted p value of the over representation test.
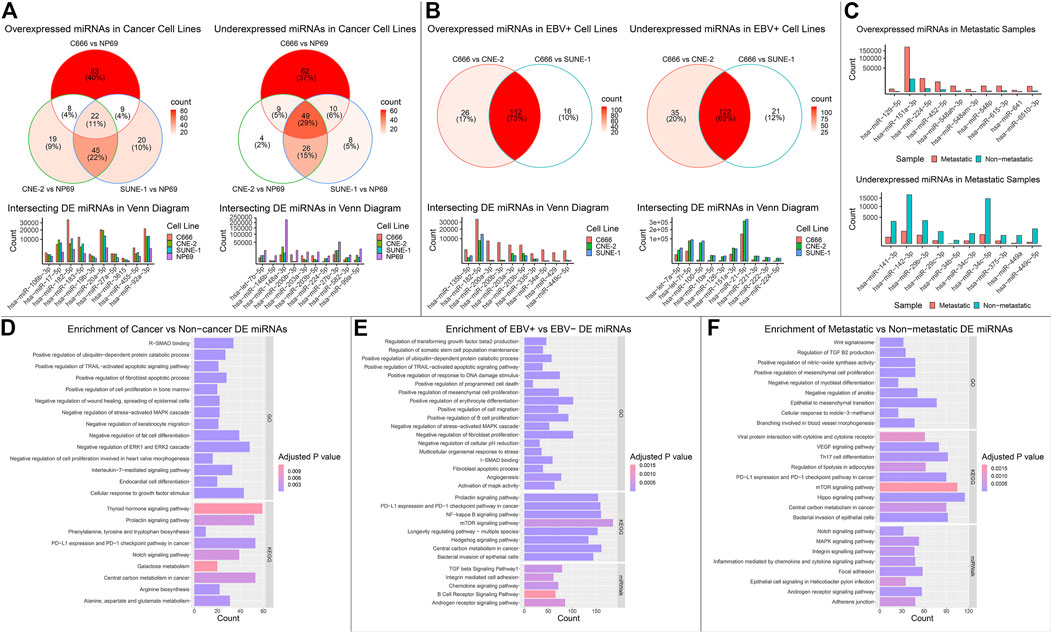
FIGURE 3. Overview of miRNA landscape across comparisons. The differential expression (DE) results for miRNAs across the three groups of comparison were integrated into one figure. (A–C) The expression of top intersecting DE-miRNA in each comparison was plotted. (D–F) The intersecting DE-miRNAs in each comparison were functionally enriched using the miEAA website, surveying across GO, KEGG, and miRWalk databases. The top enriched pathways were plotted. The x-axis value represented the gene count in the corresponding pathway, and the color of the bar represented the adjusted p value of the over representation test.
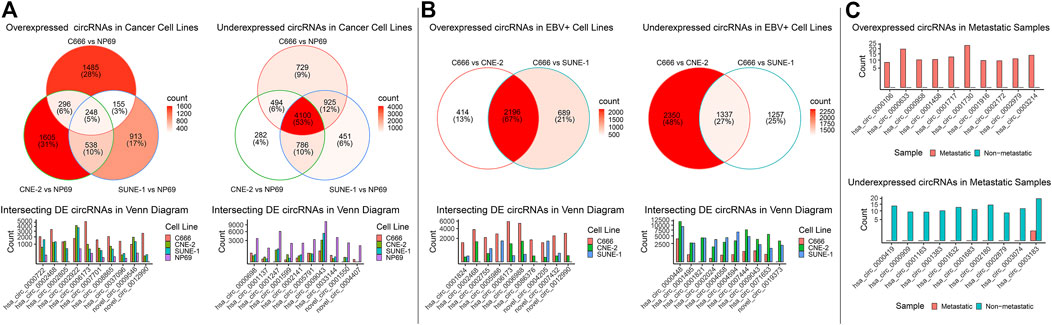
FIGURE 4. Overview of circRNA landscape across comparisons. The differential expression (DE) results for circRNAs across the three groups of comparison were integrated into one figure. (A, C) The expression of top intersecting DE-circRNA in each comparison was plotted. Functional enrichment was not performed as there were no commonly recognized tools for circRNA enrichment.
We then performed functional enrichment analysis on the intersecting set of DE-mRNA across each of the three groups of analysis (cancer, EBV, metastatic). We searched the GO Biological Pathways (BP), Molecular Functions (MF), and Cellular Component (CC) database using an over-representation test in the clusterProfiler R package (Wu et al., 2021). We highlighted functionally relevant and statistically significant pathways (Figures 2D–F). Similarly, we performed functional enrichment analysis on the intersecting set of DE-miRNA under each category of comparison. We used the miEAA website (Kern et al., 2020), searching across the GO, KEGG, and miRWalk (Sticht et al., 2018) databases. We highlighted functionally relevant and statistically significant pathways in Figures 3D–F. We then performed miRNA target gene enrichment using the miRTarBase (Huang et al., 2020) database, under the miEAA. The target enrichment results can be found in Supplementary Table S4.
Enrichment of miRNA Target Genes and circRNA Source Genes
We performed target gene enrichment on the set of DE-miRNAs under each group of DE comparison. We utilized the miEAA (Kern et al., 2020) to perform Over-Representation Analysis (ORA), separately for the overexpressed and under-expressed DE-miRNAs to capture the directionality of mRNA regulation. Supplementary Figure S1 highlighted interesting functional enrichment results of DE-miRNA, and the full enrichment result can be found in Supplementary Table S4. In theory, the target genes for the set of overexpressed DE-miRNA would be down-regulated, and vice versa. Studies have shown that circRNAs regulate the expression of its source gene, such as circSEP3 and circSMARCA5 (Conn et al., 2017; Xu et al., 2020). To evaluate circRNA’s role as source gene regulators in NPC, we performed ORA using the KEGG database on the source genes of DE-circRNAs under each DE group (Supplementary Table S5).
Target Prediction and Network Construction
We constructed a circRNA-miRNA-mRNA co-expression network to investigate the role of ceRNA regulation in NPC. The Pearson correlation between all possible circRNA-miRNA and miRNA-mRNA pairs were calculated, and pairs with a correlation coefficient < −0.85 were considered to be significant. In efforts to reduce false positives, we used miRanda and its default parameters to determine whether the circRNA-miRNA and miRNA-mRNA pairs were valid targets, and removed pairs that were not deemed target pairs (Supplementary Table S6). Lastly, we filtered for miRNAs that were paired to at least one mRNA and circRNA to isolate complete ceRNA axes. The interaction network analysis yielded 428 miRNA-mRNA pairs, and 131 miRNA-circRNA pairs. We then extracted a subset of the network that contained only DE-miRNAs and their associated miRNAs and circRNAs, and visualized it using Cytoscape (Figure 5).
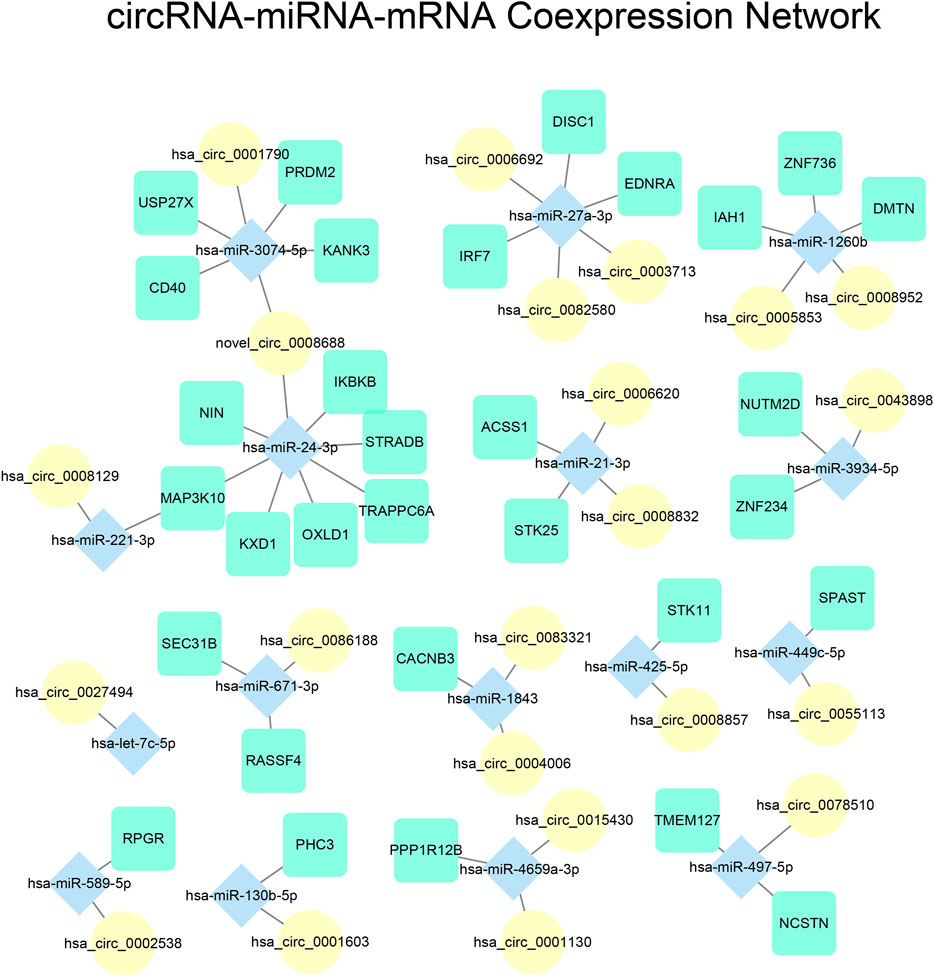
FIGURE 5. Visualization of circRNA-miRNA-mRNA network. Edges represent RNA pairs that have Pearson correlation coefficient < −0.85 and verified target interaction calculated by miRanda. mRNAs, miRNAs, and circRNAs were illustrated using different shapes and colors.
Differentially Expressed circRNA-miRNA-mRNA Axes
The previous network analysis allowed us to gain an overview of the ceRNA landscape in NPC. However, not all elements in the network are implicated in NPC, therefore we leveraged DE analysis results to reveal the functionally relevant axes. From the previously constructed co-expression network, we extracted axes that contain at least one DE-circRNA and one DE-miRNA, and with opposite directions of differential expression between circRNA-miRNA and mRNA-miRNA pairs. In this process, we implicitly removed circRNAs that did not function as miRNA sponges, but instead regulate gene expression through other plausible routes such as binding RNA polymerases (Zhang et al., 2013), inducing methylation (Chen N. et al., 2018), or alternative splicing (Conn et al., 2017). These circRNAs are false positives, and will not show opposite differential expression to its predicted target miRNAs. In efforts to reduce false negatives, we included high scoring (TargetScore≥97) miRNA-mRNA pairs from the miRDB database (Chen and Wang, 2020). The result across three groups of DE comparisons: cancer (C666, CNE-2, SUNE-1) vs. non-cancer (NP69), EBV+ (C666) vs. EBV- (CNE-2, SUNE-1), and metastatic vs. non-metastatic patient samples, are shown in Table 1; Table 2; Table 3, respectively.
In Table 1, the differentially expressed circRNA-miRNA-mRNA axes in cancerous (C666, SUNE-1, CNE-2) vs. non-cancer (NP69) cell lines were listed. They must show significant differential expression between cancer and noncancerous cell lines (|log2 fold|≥ 1.0 and adjusted p value ≦ 0.05) and the miRNA must show negative correlation (correlation coefficient < −0.85) with predicted target by miRanda or miRDB. Adjusted p values and insignificant DE-mRNAs associations were omitted for visual clarity. The hsa_circ_103,862 is verified to bind miR-493-5p (Wang et al., 2020).
Similarly, in Table 2, the differentially expressed circRNA-miRNA -mRNA axis in EBV+ (C666) vs EBV- (CNE-2, SUNE-1) cell lines were listed. A positive value indicated higher expression in the C666 cell line and vice versa. They must show significant differential expression between EBV+ and EBV- cell lines (|log2 fold|≥ 1.0 and adjusted p value ≦ 0.05) and the miRNA must show negative correlation (correlation coefficient < −0.85) with predicted target by miRanda or miRDB. Insignificant DE-mRNAs associations and adjusted p values were omitted for visual clarity. CDKN1B, Ensemble ID ENSG00000111276, is verified to be a target of miR-221-3p via luciferase reporter assay (Fornari et al., 2008; Yin et al., 2019).
In Table 3, differentially expressed miRNAs in two metastatic vs. two non-metastatic patient samples were shown. A positive value indicated higher expression in the metastatic group and vice versa. Only miRNAs showing significant differential expression (|log2 fold| ≥ 1.0 and adjusted p value ≦ 0.05) were included.
From the list of circRNA-miRNA-mRNA axes, we identified the hsa_circ_0008129/miR-221-3p/CDKN1B axis, which was significantly differentially expressed in EBV+ (C666) vs. EBV- (CNE-2, SUNE-1) cell lines. We also highlighted the ceRNA axis behind ZNF302, ZNF268, TAB3, SAR1B, BRMS1L, ZIC2, and MBNL2.
Differential Expression Analysis in Public Data
To verify our computational findings and highlight promising DE-RNAs, we utilized two additional public datasets: GSE143797 for circRNA expression in NPC and normal tissue (Yang et al., 2020), GSE118721 for miRNA and mRNA expression in NPC and normal tissue (Lin et al., 2018). We performed a Two-way Student T test to test for differentially expressed RNAs in tumor verses normal tissue, and reported genes with adjusted p value ≦ 0.05 and whose direction of differentiation aligns with our data (Supplementary Table S7). We plotted the most highly differentially expressed mRNA, miRNA, and circRNA, and their respective fold changes in data from this study and prior ones (Figure 6).
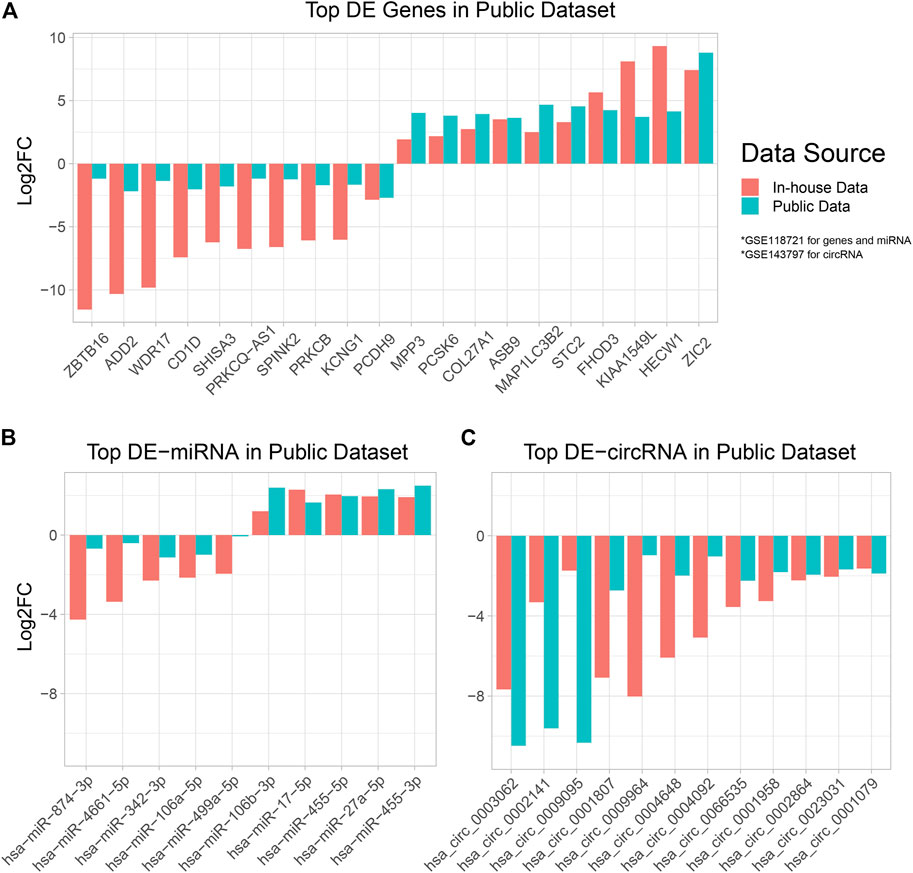
FIGURE 6. Bar plot of DE-RNAs in cross-referenced public datasets. The differentially expressed mRNA, miRNA, and circRNAs across two public datasets GSE118721 (Lin et al., 2018) and GSE143797 (Yang et al., 2020) were established and plotted alongside in-house data. A positive Log2FC denotes overexpression in tumor tissue, and a negative Log2FC denotes under-expression in tumor tissue. (A,B) DE performed using data from this study and GSE118721 (Lin et al., 2018). (C) DE performed using data from this study and GSE143797 (Yang et al., 2020).
Discussion
The role of ceRNAs in inducing the epigenetics changes necessary for the development of tumors is a topic of great research interest. The ceRNA network method is an efficient method to capture the complexity of interactions between a diverse pool of ceRNAs, and different studies have demonstrated its effectiveness in discovering important epigenetic changes in cancer. Specifically, this method allows the emerging role of circRNAs as both miRNA sponges and direct transcription regulators to be integrated into the omics landscape of cancer.
In this study, we profiled the expression of mRNAs, miRNAs, and circRNAs in NPC cell lines and non-cancerous human immortalized nasopharyngeal epithelial cell line. We utilized the differential expression of mRNAs, miRNAs, circRNAs, and the construction of a co-expression network to identify differentially expressed circRNA-miRNA-mRNA axes in NPC. Many of the axes we found contain well-established oncogenic miRNAs, and potentially point to post-transcriptional regulatory events in NPC. We found convincing evidence for the existence of a hsa_circ_0002538/miR-589-5p/RPGR axis dysregulated in NPC, and a hsa_circ_0008129/miR-221-3p/CDKN1B axis, which is differentially regulated in EBV+ vs. EBV- cell lines. Again, the final collection of axes underwent a stringent set of filters, namely, the RNA components have to: negatively co-expressed with correlation coefficient < −0.85, exhibit significant DE in its respective group, and predicted to interact by the miRanda software or miRDB database. It is nevertheless important to keep in mind that cell culture often drift from its ancestral expression profiles, therefore our findings warrant further validation using ideally fresh tissue samples.
Cancer vs. Noncancer Cell Lines: Differentially Expressed circRNA -miRNA-mRNA Axes
We have found evidence in Axis 1 (Table 1) for a potential hsa_circ_0002538↑miR-589-5p↓RPGR↑ axis. miR-589-5p is a well-established cancer-associated miRNA, found to inhibit MAP3K8 in hepatocellular carcinoma (Zhang et al., 2016), regulate tumor growth in HCC by targeting MIG-6 (Xu et al., 2018), and act as tumor suppressor in prostate cancer (Ji et al., 2019). Alongside the under-expressed miR-589-5p, we observe an overexpression of hsa_circ_0002538, predicted to bind to miR-589-5p. We postulate that dysregulation of hsa_circ_0002538 is the source of downstream abnormalities in expression. RPGR encodes the retinitis pigmentosa GTPase regulator, whose function in NPC and cancer is unknown. Given that other various GTPases are well known in their regulatory roles in cancer, it is plausible that RPGR is associated with NPC progression (Fernández-Medarde and Santos, 2011; Prieto-Dominguez et al., 2019; Boudhraa et al., 2020; Clayton and Ridley, 2020). It is also possible that RPGR is simply a passenger event in the axis.
Furthermore, we identified three miRNA-mRNA axes, for which no DE-circRNA component was found in our analysis. In Axis 2 (Table 1), miR-27b-3p↓TAB3↑, over-expression of TAB3 has been found to promote tumor progression in NSCLC (Chen et al., 2016), colorectal cancer (Luo et al., 2017), and triple-negative breast cancer (Tao et al., 2016). Under-expression of miR-27b-3p has been shown to induce drug resistance in breast cancer (Chen D. et al., 2018). In Axis 3 (Table 1), miR-20a-5p↑ SAR1B↓ BRMS1L↓, reduced BRMS1L in breast cancer tissues was shown to be associated with metastasis and poor patient survival (Gong et al., 2014). Specifically, Gong et. Al. found that BRMS1L inhibited epithelial-mesenchymal transition, and thus inhibiting breast cancer metastasis. In Axis 4 (Table 1), miR-493-5p↓ ZIC2↑ MBNL2↑, MBNL2 is abnormally expressed in lung and breast cancer (Zhang J. et al., 2019), as well as hepatocellular carcinoma (Lee et al., 2016). Interestingly, despite overexpressed in these cancer types, both studies showed that MBNL2 suppresses tumor progression. ZIC2 was shown to be highly overexpressed in NPC both in the data used in this study, as well as in a study by Lv et al. (Lv et al., 2021) and Lin et al. (Lin et al., 2018).
EBV+ vs. EBV- Cell Lines: Differentially Expressed circRNA-miRNA -mRNA Axes
In comparison to few complete axes in the cancer vs non-cancer comparison, the EBV+ (C666) vs. EBV- (CNE-2, SUNE-1) comparisons revealed several axes of circRNA-miRNA exhibiting differential expression. Surveying through the DE results of target miRNA-circRNA pairs, we observed that the directions of differential expression are almost uniformly opposite, which confirms the theory that circRNAs serve as miRNA sponges.
In Axis 3 (Table 2) we observed hsa_circ_0008129↑miR-221-3p↓CDKN1B↑RIMS3↑ in EBV+ (C666) cell line. Prior studies confirmed that miR-221-3p targets the cell cycle regulator CDKN1B, also known as p27, through luciferase reporter assay (Fornari et al., 2008; Yin et al., 2019). CDKN1B encodes the cyclin dependent kinase inhibitor 1B protein, belonging to the Cip/Kip protein family. CDKN1B functions as a cell cycle check point at G1-S by repressing cyclin-dependent kinases that are necessary for progression from G1 to S phase (Sherr and Roberts, 1999). Overall, CDKN1B is mainly dysregulated at the post-transcriptional level in human cancer, which supports our finding of a significantly differentially expressed ceRNA axis. Further, CDKN1B is described as haplo-insufficient tumor suppressor gene, where animals lacking one copy of CDKN1B already displayed tumor-prone phenotypes (Fero et al., 1998). RIMS3 is not reported to be associated with cancer to the best of our knowledge, and was likely a passenger event.
The role of the miRNA component of this axis, miR-221-3p, has been studied extensively across cancer types. A study by Wang et al. showed that miR-221-3p serve as potential prognostic predictors for hepatocellular carcinoma (Wang et al., 2019). Other studies have shown miR-221-3p to be involved in drug resistance in glioma cells (Milani et al., 2019) and breast cancer (Chen et al., 2020) by increasing antiapoptotic abilities. There have been no studies done on hsa_circ_0008129 to the best of our knowledge. Our results show evidence for a hsa_circ_0008129/miR-221-3p/CDKN1B axis, which potentially plays a role in explaining the pathological differences between EBV+ and EBV- NPC.
In Axis 2 (Table 2), we observed an overexpression of miR-1260b in all EBV- cell lines. MiR-1260b is a well-studied oncogenic miRNA, and has been found to regulate proliferation in non-small cell lung carcinoma (NSCLC) (Xu et al., 2015; Xia et al., 2019) and prostate cancer (Hirata et al., 2014). ZNF268 is predicted to be a high affinity target of miR-1260b, with a target score of 100 (the maximum of target score) in the miRDB database (Chen and Wang, 2020), and is found to be overexpressed in cervical cancer (Wang et al., 2012) and ovarian carcinomas (Hu et al., 2013). Specifically, Wang et al. found that knockdown of ZNF268 in cervical cancer cells caused cell cycle arrest at the G0/G1 phase (Wang et al., 2012). Similarly, ZNF302 is predicted to be a miR-1260b target gene (score = 98), and high expression of ZNF302 is associated with poor survival in Endometrial Carcinoma in TCGA-UCEC (Supplementary Figure S2). We found both ZNF268 and ZNF302 to be overexpressed in EBV + cell line C666, which may explain why there is higher malignancy in the EBV + subtype of NPC. Alongside miR-1260b and zinc finger proteins, we observed up-regulation of hsa_circ_005853 and hsa_circ_0008952 in the EBV+ subtype of NPC., which were predicted to bind miR-1260b. These circRNAs have not been studied to the best of our knowledge. The above results suggest that the Axis 2 (Table 2) plays a key role in the progression of NPC and warrants further studies.
In Axis 1, 4, 5, 6 (Table 2), we observed abnormal expression of various circRNA-miRNA pairs, some of which were previously found to be associated with cancer. In Axis 1 (Table 2), has_circ_0027494↑ let-7c-5p↓, let-7c-5p is found to be a tumor suppressor across many cancer types, including breast cancer (Fu et al., 2017), mucosal melanoma (Tang et al., 2019), acute erythroleukemia (Mortazavi and Sharifi, 2018), among others (Wagner et al., 2014; Liu et al., 2018; Chirshev et al., 2019). We postulate that hsa_circ_0027494 has inhibitory effects on let-7c-5p (Panda, 2018; Zhang P.-F. et al., 2019; Zhang X. et al., 2019), leading to downstream overexpression of let-7c-5p targeted genes, which could explain tumorigenic activities that stands differently in EBV + vs EBV- NPC. Similarly, for Axis 4, 5, 6 (Table 2), it is plausible that they regulate certain target genes that underlies traits of EBV+ NPC.
In sum, our method combining DE analysis and co-expression network analysis yielded 6 circRNA-miRNA pairs that show significant DE and co-expression. Out of these results, we were able to establish the hsa_circ_0008129↑ miR-221-3p↓ CDKN1B↑ axis, where overexpression of hsa_circ_0008129 led to the sponging of miR-221-3p and upregulation of CDKN1B.
Metastatic vs. Non-metastatic Patient Samples: Differentially Expressed mRNAs
No significant mRNA or circRNA differential expression was detected in the differential expression analysis of metastatic vs. non-metastatic samples. However, we did find 4 miRNAs that were differentially expressed in the metastatic samples than the non-metastatic samples (Table 3).
miR-130b-5p was overexpressed in the metastatic samples. This miRNA is likely not involved in pan-cancer metastatic mechanisms, as different cancer types exhibit different expression patterns with contradicting effects. Namely, miR-130b-5p has been shown to promote proliferation and migration in gastric cancer via targeting RASAL1 (Chen H. et al., 2018), as well as in osteosarcoma via binding to TIMP2 (Cheng et al., 2019). On the other hand, miR-130b-5p exhibits anti-tumor effects in pancreatic ductal adenocarcinoma (Fukuhisa et al., 2019) and prostate cancer (Chen et al., 2015). Little literature exists on the functions of miR-449c-5p (Table 3), yet given its significant downregulation in the metastatic group (Log2 = -6.9275, p = 1.0903E-19), we postulated that miR-449c-5p could target a metastasis-associated mRNA.
Materials and Methods
Data Source
We used four cell lines, including three nasopharyngeal carcinoma (NPC) cell line: SUNE-1, C666 (EBV+), CNE-2, and one non-cancerous human immortalized nasopharyngeal epithelial cell line NP69. Furthermore, we collected samples from four patients diagnosed with NPC in Fujian Cancer Hospital. Two of the four NPC patients were grouped into the metastatic group, and the remaining two patients were grouped into the non-metastatic group.
Statistics
All adjusted p values were reported at false discovery rate of 0.05 unless otherwise specified. All measures of correlation referred to Pearson correlation unless otherwise specified.
Sequencing and Preprocessing
We used Hisat2 to align the RNA reads to the reference genome (Kim et al., 2019). We used StringTie (https://github.com/gpertea/stringtie) (Pertea et al., 2015) to perform RNA transcript assembly. After RNA transcript assembly, we used cuffmerge in the Cufflinks software (Trapnell et al., 2010) to filter the transcript, namely by removing transcripts smaller than 200 nt, or have less than 2 exons, or transcripts with unidentified directions. Then we referenced the remaining transcript assembly using cuffcompare to select for known circRNA, mRNA, and miRNAs. We used StringTie to profile the expression of different RNAs given the filtered transcript data. Information related to QC and mapping can be found in Supplementary Table S8.
Identification of circRNAs
We used two software to identify the circRNAs within the transcript assembly: find_circ (Memczak et al., 2013) and CIRI2 (Gao et al., 2015; Gao et al., 2017). Each software produced a set of identified circRNAs, and we took the intersecting set between both to minimize false positive rates. We profiled the expression of circRNAs using CIRI2. We obtained the expression of 15,036 circRNAs, out of which 7,029 were novel circRNAs found in this study. The complete list of all circRNAs identified in this study can be found in Supplementary Table S9.
Differential Expression Analysis
We performed DE analysis for miRNA, circRNA, and mRNA separately among different pairs of cell lines using DESeq2 (Love et al., 2014). Namely, we performed DE analysis on each category of comparison: cancer (C666, CNE-2, SUNE-1) vs. non-cancer (NP69); EBV+ (C666) vs. EBV- (CNE-2, SUNE-1); and an additional set of DE analysis using two metastatic vs. two non-metastatic patient samples. The threshold for qualifying as differential expression was set to be edgeR adjusted p value ≦ 0.05 and |log2FoldChange| ≥ 1.0.
Network Construction
To investigate the role of ceRNA regulation in NPC, we constructed a co-expression network representing the interactions between miRNA-circRNA pairs and miRNA-mRNA pairs. We first calculated Pearson correlation between all possible miRNA-circRNA and miRNA-mRNA pairs, and keep only pairs with correlation coefficient < −0.85. The specific cutoff was chosen to capture the biological mechanism where circRNA inhibits miRNA and miRNA inhibits mRNA. Further, we used the miRanda software (Betel et al., 2010) to predict miRNA-circRNA and miRNA-mRNA target interactions, and we kept only pairs that were calculated to be valid targets by the default parameters of miRanda. Due to the limited number of cell lines for co-expression calculation, and potential flaws in the miRanda software, we further curate the list of miRNA-mRNA pairs by adding those with target score≥97 in the miRDB database (Chen and Wang, 2020). The target score are assigned by the target prediction algorithm in miRDB and the higher the target score is, the more confidence the prediction has (Chen and Wang, 2020). It ranged from 50 to 100. The target scores of miRNA-mRNA pairs in Table 1; Table 2 were given in Supplementary Table S10.
Identifying Significant circRNA-miRNA-mRNA Axes
In order to identify functionally relevant axes of regulation between circRNA-miRNA-mRNA, we applied additional filters specifying negative correlations. Namely, we selected circRNA-miRNA-mRNA axes in the network satisfying: 1) contained at least 2 differentially expressed components; 2) circRNA-miRNA and miRNA-mRNA pairs within the axis exhibited opposite differential expression. The motivation behind such criteria was that given a functionally relevant gene with abnormal expression, the upstream circRNAs and miRNAs, if indeed responsible for the abnormal expression, should have opposing directions of DE. Namely, miRNAs inhibit expression of target gene using Dicer and Drosha protein complexes, and circRNAs inhibit target miRNA through the miRNA sponge mechanism. Therefore, within a relevant axis, miRNAs should be negatively correlated to circRNAs and mRNAs.
DE of Public Datasets
GSE143797 profiled the expression of four NPC tissue and matched healthy tissue, identifying 93 upregulated circRNAs and 77 downregulated circRNAs (Yang et al., 2020). GSE118721 profiled the mRNA and miRNA expression of six EBV-positive NPC biopsy specimens and normal nasopharyngeal samples (Lin et al., 2018). We performed a Two-way Student T test to identify differentially expressed coding and non-coding RNAs both datasets, and calculated Log2 Fold Changes.
Kaplan-Meier Plots of Genes of Interest
The GEPIA portal (Tang et al., 2017) (gepia.cancer-pku.cn) was used to plot Kaplan-Meier curves for genes of interest. Appropriate TCGA datasets are used, and the cutoff for high and low expression is the top and bottom 25th percentile. Overall survival was plotted alongside Hazard Ratio and the 95% confidence interval.
Conclusion
Our study compared the expression profile between NPC vs. non-cancerous human immortalized nasopharyngeal epithelial cell lines, EBV+ vs. EBV- NPC cell lines, and metastatic vs. non-metastatic patient samples. Specifically, we constructed a circRNA-miRNA-mRNA co-expression network, and performed differential expression analysis. This allowed us to filter for highly correlated non-coding RNA axis that show significant differential expression, suggesting that they are both biologically linked and significant in the genetic landscape of NPC. We found multiple circRNA-miRNA-mRNA axes relevant in both NPC and more specifically in EBV+ NPC. We highlighted the regulatory ceRNA axes behind key differentially expressed genes including CDKN1B, ZNF302, ZNF268, TAB3, SAR1B, BRMS1L, ZIC2, and MBNL2. We believe our in-silico findings illustrated the regulatory role that ceRNAs play in NPC, and these results should be further studied using experimental techniques.
Data Availability Statement
The datasets presented in this study can be found in online repositories. The names of the repository/repositories and accession number(s) can be found in the article/Supplementary Material.
Ethics Statement
The studies involving human participants were reviewed and approved by the Ethics Committee of the Fujian Medical University Cancer Hospital and Fujian Cancer Hospital (Fuzhou, China). The patients/participants provided their written informed consent to participate in this study.
Author Contributions
XL, YS, TH designed the experiments, KL, JZ, QZ performed the experiments, SW did the data analysis, XL, SW, and TH wrote the manuscript.
Funding
This study was supported by the Natural Science Foundation of Fujian Province (Nos. 2019J01196, 2020J011109), Medical Innovation Program of Fujian Province (No. 2019-CX-5) and National Natural Science Foundation Project of China (No. 81972717), Fujian Provincial Clinical Research Center for Cancer Radiotherapy and Immunotherapy(No. 2020Y2012), Strategic Priority Research Program of Chinese Academy of Sciences (XDB38050200, XDA26040304), National Key R&D Program of China (2018YFC0910403, 2017YFC1201200) and Shanghai Municipal Science and Technology Major Project (2017SHZDZX01).
Conflict of Interest
The authors declare that the research was conducted in the absence of any commercial or financial relationships that could be construed as a potential conflict of interest.
Publisher’s Note
All claims expressed in this article are solely those of the authors and do not necessarily represent those of their affiliated organizations, or those of the publisher, the editors and the reviewers. Any product that may be evaluated in this article, orclaim that may be made by its manufacturer, is not guaranteed or endorsed by the publisher.
Supplementary Material
The Supplementary Material for this article can be found online at: https://www.frontiersin.org/articles/10.3389/fcell.2021.782473/full#supplementary-material
References
Betel, D., Koppal, A., Agius, P., Sander, C., and Leslie, C. (2010). Comprehensive Modeling of microRNA Targets Predicts Functional Non-Conserved and Non-Canonical Sites. Genome Biol. 11 (8), R90. doi:10.1186/gb-2010-11-8-r90
Boudhraa, Z., Carmona, E., Provencher, D., and Mes-Masson, A.-M. (2020). Ran GTPase: A Key Player in Tumor Progression and Metastasis. Front. Cel Dev. Biol. 8, 345. doi:10.3389/fcell.2020.00345
Buell, P. (1974). The Effect of Migration on the Risk of Nasopharyngeal Cancer Among Chinese. Cancer Res. 34 (5), 1189–1191.
Chan, A. T. C., Hui, E. P., Ngan, R. K. C., Tung, S. Y., Cheng, A. C. K., Ng, W. T., et al. (2018). Analysis of Plasma Epstein-Barr Virus DNA in Nasopharyngeal Cancer after Chemoradiation to Identify High-Risk Patients for Adjuvant Chemotherapy: A Randomized Controlled Trial. J. Clin. Oncol. 36 (31), 3091–3100. doi:10.1200/JCO.2018.77.7847
Chen, Y., and Wang, X. (2020). miRDB: an Online Database for Prediction of Functional microRNA Targets. Nucleic Acids Res. 48 (D1), D127–D131. doi:10.1093/nar/gkz757
Chen, Q., Zhao, X., Zhang, H., Yuan, H., Zhu, M., Sun, Q., et al. (2015). MiR-130b Suppresses Prostate Cancer Metastasis through Down-Regulation of MMP2. Mol. Carcinog. 54 (11), 1292–1300. doi:10.1002/mc.22204
Chen, J., Gu, J., Feng, J., Liu, Y., Xue, Q., Ni, T., et al. (2016). TAB3 Overexpression Promotes Cell Proliferation in Non-Small Cell Lung Cancer and Mediates Chemoresistance to CDDP in A549 Cells via the NF-Κb Pathway. Tumor Biol. 37 (3), 3851–3861. doi:10.1007/s13277-015-3896-y
Chen, D., Si, W., Shen, J., Du, C., Lou, W., Bao, C., et al. (2018a). miR-27b-3p Inhibits Proliferation and Potentially Reverses Multi-Chemoresistance by Targeting CBLB/GRB2 in Breast Cancer Cells. Cell Death Dis 9 (2), 188. doi:10.1038/s41419-017-0211-4
Chen, H., Yang, Y., Wang, J., Shen, D., Zhao, J., and Yu, Q. (2018b). miR-130b-5p Promotes Proliferation, Migration and Invasion of Gastric Cancer Cells via Targeting RASAL1. Oncol. Lett. 15 (5), 6361–6367. doi:10.3892/ol.2018.8174
Chen, N., Zhao, G., Yan, X., Lv, Z., Yin, H., Zhang, S., et al. (2018c). A Novel FLI1 Exonic Circular RNA Promotes Metastasis in Breast Cancer by Coordinately Regulating TET1 and DNMT1. Genome Biol. 19 (1), 218. doi:10.1186/s13059-018-1594-y
Chen, L., Zhou, H., and Guan, Z. (2019). CircRNA_000543 Knockdown Sensitizes Nasopharyngeal Carcinoma to Irradiation by Targeting miR-9/platelet-Derived Growth Factor Receptor B Axis. Biochem. Biophysical Res. Commun. 512 (4), 786–792. doi:10.1016/j.bbrc.2019.03.126
Chen, Z., Pan, T., Jiang, D., Jin, L., Geng, Y., Feng, X., et al. (2020). The lncRNA-GAS5/miR-221-3p/DKK2 Axis Modulates ABCB1-Mediated Adriamycin Resistance of Breast Cancer via the Wnt/β-Catenin Signaling Pathway. Mol. Ther. - Nucleic Acids 19, 1434–1448. doi:10.1016/j.omtn.2020.01.030
Cheng, Z. H., Luo, C., and Guo, Z. L. (2019). MicroRNA-130b-5p Accelerates the Migration and Invasion of Osteosarcoma via Binding to TIMP2. Eur. Rev. Med. Pharmacol. Sci. 23 (21), 9267–9276. doi:10.26355/eurrev_201911_19419
Chirshev, E., Oberg, K. C., Ioffe, Y. J., and Unternaehrer, J. J. (2019). Let‐7as Biomarker, Prognostic Indicator, and Therapy for Precision Medicine in Cancer. Clin. Translational Med. 8 (1), 24. doi:10.1186/s40169-019-0240-y
Clayton, N. S., and Ridley, A. J. (2020). Targeting Rho GTPase Signaling Networks in Cancer. Front. Cel Dev. Biol. 8, 222. doi:10.3389/fcell.2020.00222
Conn, V. M., Hugouvieux, V., Nayak, A., Conos, S. A., Capovilla, G., Cildir, G., et al. (2017). A circRNA from SEPALLATA3 Regulates Splicing of its Cognate mRNA through R-Loop Formation. Nat. Plants 3 (5), 17053. doi:10.1038/nplants.2017.53
Fernández-Medarde, A., and Santos, E. (2011). Ras in Cancer and Developmental Diseases. Genes Cancer 2 (3), 344–358. doi:10.1177/1947601911411084
Fero, M. L., Randel, E., Gurley, K. E., Roberts, J. M., and Kemp, C. J. (1998). The Murine Gene p27Kip1 Is Haplo-Insufficient for Tumour Suppression. Nature 396 (6707), 177–180. doi:10.1038/24179
Fornari, F., Gramantieri, L., Ferracin, M., Veronese, A., Sabbioni, S., Calin, G. A., et al. (2008). MiR-221 Controls CDKN1C/p57 and CDKN1B/p27 Expression in Human Hepatocellular Carcinoma. Oncogene 27 (43), 5651–5661. doi:10.1038/onc.2008.178
Fu, X., Mao, X., Wang, Y., Ding, X., and Li, Y. (2017). Let-7c-5p Inhibits Cell Proliferation and Induces Cell Apoptosis by Targeting ERCC6 in Breast Cancer. Oncol. Rep. 38 (3), 1851–1856. doi:10.3892/or.2017.5839
Fukuhisa, H., Seki, N., Idichi, T., Kurahara, H., Yamada, Y., Toda, H., et al. (2019). Gene Regulation by Antitumor miR-130b-5p in Pancreatic Ductal Adenocarcinoma: the Clinical Significance of Oncogenic EPS8. J. Hum. Genet. 64 (6), 521–534. doi:10.1038/s10038-019-0584-6
Gao, Y., Wang, J., and Zhao, F. (2015). CIRI: An Efficient and Unbiased Algorithm for De Novo Circular RNA Identification. Genome Biol. 16 (1), 4. doi:10.1186/s13059-014-0571-3
Gao, Y., Zhang, J., and Zhao, F. (2017). Circular RNA Identification Based on Multiple Seed Matching. Brief. Bioinform. 19 (5), 803–810. doi:10.1093/bib/bbx014
Gong, C., Qu, S., Lv, X.-B., Liu, B., Tan, W., Nie, Y., et al. (2014). BRMS1L Suppresses Breast Cancer Metastasis by Inducing Epigenetic Silence of FZD10. Nat. Commun. 5 (1), 5406. doi:10.1038/ncomms6406
Guo, Y., Yang, J., Huang, Q., Hsueh, C., Zheng, J., Wu, C., et al. (2019). Circular RNAs and Their Roles in Head and Neck Cancers. Mol. Cancer 18 (1), 44. doi:10.1186/s12943-019-1003-5
Hirata, H., Hinoda, Y., Shahryari, V., Deng, G., Tanaka, Y., Tabatabai, Z. L., et al. (2014). Genistein Downregulates Onco-miR-1260b and Upregulates sFRP1 and Smad4 via Demethylation and Histone Modification in Prostate Cancer Cells. Br. J. Cancer 110 (6), 1645–1654. doi:10.1038/bjc.2014.48
Hirono, T., Jingushi, K., Nagata, T., Sato, M., Minami, K., Aoki, M., et al. (2019). MicroRNA-130b Functions as an oncomiRNA in Non-small Cell Lung Cancer by Targeting Tissue Inhibitor of Metalloproteinase-2. Sci. Rep. 9 (1), 6956. doi:10.1038/s41598-019-43355-8
Hoey, C., Ahmed, M., Fotouhi Ghiam, A., Vesprini, D., Huang, X., Commisso, K., et al. (2019). Circulating miRNAs as Non-invasive Biomarkers to Predict Aggressive Prostate Cancer after Radical Prostatectomy. J. Transl Med. 17 (1), 173. doi:10.1186/s12967-019-1920-5
Hong, B. S., Ryu, H. S., Kim, N., Kim, J., Lee, E., Moon, H., et al. (2019). Tumor Suppressor miRNA-204-5p Regulates Growth, Metastasis, and Immune Microenvironment Remodeling in Breast Cancer. Cancer Res. 79 (7), 1520–1534. doi:10.1158/0008-5472.CAN-18-0891
Hu, L., Wang, W., Cai, J., Luo, J., Huang, Y., Xiong, S., et al. (2013). Aberrant Expression of ZNF268 Alters the Growth and Migration of Ovarian Cancer Cells. Oncol. Lett. 6 (1), 49–54. doi:10.3892/ol.2013.1318
Huang, H.-Y., Lin, Y.-C. -D., Li, J., Huang, K.-Y., Shrestha, S., Hong, H.-C., et al. (2020). miRTarBase 2020: Updates to the Experimentally Validated microRNA-Target Interaction Database. Nucleic Acids Res. 48 (D1), D148–D154. doi:10.1093/nar/gkz896
Jain, A., Chia, W. K., and Toh, H. C. (20162016). Immunotherapy for Nasopharyngeal Cancer—A Review. Chin. Clin. Oncol. 5 (2), 10. doi:10.21037/cco.2016.03.08
Ji, L., Jiang, X., Mao, F., Tang, Z., and Zhong, B. (2019). miR-589-5p Is Downregulated in Prostate Cancer and Regulates Tumor Cell Viability and Metastasis by Targeting CCL-5. Mol. Med. Rep. 20 (2), 1373–1382. doi:10.3892/mmr.2019.10334
Kern, F., Fehlmann, T., Solomon, J., Schwed, L., Grammes, N., Backes, C., et al. (2020). miEAA 2.0: Integrating Multi-Species microRNA Enrichment Analysis and Workflow Management Systems. Nucleic Acids Res. 48 (W1), W521–W528. doi:10.1093/nar/gkaa309
Kim, D., Paggi, J. M., Park, C., Bennett, C., and Salzberg, S. L. (2019). Graph-Based Genome Alignment and Genotyping with HISAT2 and HISAT-Genotype. Nat. Biotechnol. 37 (8), 907–915. doi:10.1038/s41587-019-0201-4
Lee, Y.-H., Jhuang, Y.-L., Chen, Y.-L., Jeng, Y.-M., and Yuan, R.-H. (2016). Paradoxical Overexpression of MBNL2 in Hepatocellular Carcinoma Inhibits Tumor Growth and Invasion. Oncotarget 7 (40), 65589–65601. doi:10.18632/oncotarget.11577
Li, Y. Q., Khin, N. S., and Chua, M. L. K. (2018). The Evolution of Epstein-Barr Virus Detection in Nasopharyngeal Carcinoma. Cancer Biol. Med. 15 (1), 1–5. doi:10.20892/j.issn.2095-3941.2017.0176
Lin, C., Zong, J., Lin, W., Wang, M., Xu, Y., Zhou, R., et al. (2018). EBV-miR-BART8-3p Induces Epithelial-Mesenchymal Transition and Promotes Metastasis of Nasopharyngeal Carcinoma Cells through Activating NF-Κb and Erk1/2 Pathways. J. Exp. Clin. Cancer Res. 37 (1), 283. doi:10.1186/s13046-018-0953-6
Liu, G.-X., Ma, S., Li, Y., Yu, Y., Zhou, Y.-X., Lu, Y.-D., et al. (2018). Hsa-let-7c Controls the Committed Differentiation of IGF-1-Treated Mesenchymal Stem Cells Derived from Dental Pulps by Targeting IGF-1R via the MAPK Pathways. Exp. Mol. Med. 50 (4), 1–14. doi:10.1038/s12276-018-0048-7
Liu, Q., Zhang, W., Wu, Z., Liu, H., Hu, H., Shi, H., et al. (2020). Construction of a Circular RNA‐microRNA‐MessengerRNA Regulatory Network in Stomach Adenocarcinoma. J. Cel Biochem 121 (2), 1317–1331. doi:10.1002/jcb.29368
Love, M. I., Huber, W., and Anders, S. (2014). Moderated Estimation of Fold Change and Dispersion for RNA-Seq Data with DESeq2. Genome Biol. 15 (12), 550. doi:10.1186/s13059-014-0550-8
Luo, C., Yuan, R., Chen, L., Zhou, W., Shen, W., Qiu, Y., et al. (2017). TAB3 Upregulates Survivin Expression to Promote Colorectal Cancer Invasion and Metastasis by Binding to the TAK1-TRAF6 Complex. Oncotarget 8 (63), 106565–106576. doi:10.18632/oncotarget.22497
Lv, B., Li, F., Liu, X., and Lin, L. (2021). The Tumor-Suppressive Role of microRNA-873 in Nasopharyngeal Carcinoma Correlates with Downregulation of ZIC2 and Inhibition of AKT Signaling Pathway. Cancer Gene Ther. 28 (1), 74–88. doi:10.1038/s41417-020-0185-8
Memczak, S., Jens, M., Elefsinioti, A., Torti, F., Krueger, J., Rybak, A., et al. (2013). Circular RNAs Are a Large Class of Animal RNAs with Regulatory Potency. Nature 495 (7441), 333–338. doi:10.1038/nature11928
Milani, R., Brognara, E., Fabbri, E., Manicardi, A., Corradini, R., Finotti, A., et al. (2019). Targeting miR-155-5p and miR-221-3p by Peptide Nucleic Acids Induces Caspase-3 Activation and Apoptosis in Temozolomide-Resistant T98G Glioma Cells. Int. J. Oncol. 55 (1), 59–68. doi:10.3892/ijo.2019.4810
Mortazavi, D., and Sharifi, M. (2018). Antiproliferative Effect of Upregulation of Hsa-Let-7c-5p in Human Acute Erythroleukemia Cells. Cytotechnology 70 (6), 1509–1518. doi:10.1007/s10616-018-0241-5
Panda, A. C. (2018). Circular RNAs Act as miRNA Sponges. Adv. Exp. Med. Biol. 1087, 67–79. doi:10.1007/978-981-13-1426-1_6
Peng, Y., and Croce, C. M. (2016). The Role of MicroRNAs in Human Cancer. Sig Transduct Target. Ther. 1 (1), 15004. doi:10.1038/sigtrans.2015.4
Pertea, M., Pertea, G. M., Antonescu, C. M., Chang, T.-C., Mendell, J. T., and Salzberg, S. L. (2015). StringTie Enables Improved Reconstruction of a Transcriptome from RNA-Seq Reads. Nat. Biotechnol. 33 (3), 290–295. doi:10.1038/nbt.3122
Prieto-Dominguez, N., Parnell, C., and Teng, Y. (2019). Drugging the Small GTPase Pathways in Cancer Treatment: Promises and Challenges. Cells 8 (3), 255. doi:10.3390/cells8030255
Salmena, L., Poliseno, L., Tay, Y., Kats, L., and Pandolfi, P. P. (2011). A ceRNA Hypothesis: The Rosetta Stone of a Hidden RNA Language? Cell 146 (3), 353–358. doi:10.1016/j.cell.2011.07.014
Sherr, C. J., and Roberts, J. M. (1999). CDK Inhibitors: Positive and Negative Regulators of G1-phase Progression. Genes Develop. 13 (12), 1501–1512. doi:10.1101/gad.13.12.1501
Shotelersuk, K., Khorprasert, C., Sakdikul, S., Pornthanakasem, W., Voravud, N., and Mutirangura, A. (2000). Epstein-Barr Virus DNA in Serum/Plasma as a Tumor Marker for Nasopharyngeal Cancer. Clin. Cancer Res. 6 (3), 1046–1051.
Sticht, C., De La Torre, C., Parveen, A., and Gretz, N. (2018). miRWalk: An Online Resource for Prediction of microRNA Binding Sites. PLOS ONE 13 (10), e0206239. doi:10.1371/journal.pone.0206239
Tang, Z., Li, C., Kang, B., Gao, G., Li, C., and Zhang, Z. (2017). GEPIA: A Web Server for Cancer and normal Gene Expression Profiling and Interactive Analyses. Nucleic Acids Res. 45 (W1), W98–w102. doi:10.1093/nar/gkx247
Tang, H., Ma, M., Dai, J., Cui, C., Si, L., Sheng, X., et al. (2019). miR-let-7b and miR-Let-7c Suppress Tumourigenesis of Human Mucosal Melanoma and Enhance the Sensitivity to Chemotherapy. J. Exp. Clin. Cancer Res. 38 (1), 212. doi:10.1186/s13046-019-1190-3
Tao, T., He, Z., Shao, Z., and Lu, H. (2016). TAB3 O-GlcNAcylation Promotes Metastasis of Triple Negative Breast Cancer. Oncotarget 7 (16), 22807–22818. doi:10.18632/oncotarget.8182
Trapnell, C., Williams, B. A., Pertea, G., Mortazavi, A., Kwan, G., van Baren, M. J., et al. (2010). Transcript Assembly and Quantification by RNA-Seq Reveals Unannotated Transcripts and Isoform Switching during Cell Differentiation. Nat. Biotechnol. 28 (5), 511–515. doi:10.1038/nbt.1621
Tsao, S. W., Tsang, C. M., and Lo, K. W. (2017). Epstein-Barr Virus Infection and Nasopharyngeal Carcinoma. Phil. Trans. R. Soc. B 372 (1732), 20160270. doi:10.1098/rstb.2016.0270
Wagner, S., Ngezahayo, A., Murua Escobar, H., and Nolte, I. (2014). Role of miRNALet-7and its Major Targets in Prostate Cancer. Biomed. Res. Int. 2014, 376326. doi:10.1155/2014/376326
Wang, W., Guo, M., Hu, L., Cai, J., Zeng, Y., Luo, J., et al. (2012). The Zinc Finger Protein ZNF268 Is Overexpressed in Human Cervical Cancer and Contributes to Tumorigenesis via Enhancing NF-Κb Signaling. J. Biol. Chem. 287 (51), 42856–42866. doi:10.1074/jbc.M112.399923
Wang, X., Liao, X., Huang, K., Zeng, X., Liu, Z., Zhou, X., et al. (2019). Clustered microRNAs Hsa-miR-221-3p/hsa-miR-222-3p and Their Targeted Genes Might Be Prognostic Predictors for Hepatocellular Carcinoma. J. Cancer 10 (11), 2520–2533. doi:10.7150/jca.29207
Wang, X., Wu, T., Wang, P., Yang, L., Li, Q., Wang, J., et al. (2020). Circular RNA 103862 Promotes Proliferation and Invasion of Laryngeal Squamous Cell Carcinoma Cells through the miR-493-5p/GOLM1 Axis. Front. Oncol. 10, 1064. doi:10.3389/fonc.2020.01064
Wu, L., Li, C., and Pan, L. (2018). Nasopharyngeal Carcinoma: A Review of Current Updates. Exp. Ther. Med. 15 (4), 3687–3692. doi:10.3892/etm.2018.5878
Wu, T., Hu, E., Xu, S., Chen, M., Guo, P., Dai, Z., et al. (2021). ClusterProfiler 4.0: A Universal Enrichment Tool for Interpreting Omics Data. The Innovation 2 (3), 100141. doi:10.1016/j.xinn.2021.100141
Xia, Y., Wei, K., Yang, F.-M., Hu, L.-Q., Pan, C.-F., Pan, X.-L., et al. (2019). miR-1260b, Mediated by YY1, Activates KIT Signaling by Targeting SOCS6 to Regulate Cell Proliferation and Apoptosis in NSCLC. Cel Death Dis 10 (2), 112. doi:10.1038/s41419-019-1390-y
Xu, L., Li, L., Li, J., Li, H., Shen, Q., Ping, J., et al. (2015). Overexpression of miR-1260b in Non-Small Cell Lung Cancer Is Associated with Lymph Node Metastasis. Aging Dis. 6 (6), 478–485. doi:10.14336/ad.2015.0620
Xu, M., Wang, Y., He, H. T., and Yang, Q. (2018). MiR-589-5p Is a Potential Prognostic Marker of Hepatocellular Carcinoma and Regulates Tumor Cell Growth by Targeting MIG-6. Neoplasma 65 (5), 753–761. doi:10.4149/neo_2018_171125N762
Xu, X., Zhang, J., Tian, Y., Gao, Y., Dong, X., Chen, W., et al. (2020). CircRNA Inhibits DNA Damage Repair by Interacting with Host Gene. Mol. Cancer 19 (1), 128. doi:10.1186/s12943-020-01246-x
Yang, J., Gong, Y., Jiang, Q., Liu, L., Li, S., Zhou, Q., et al. (2020). Circular RNA Expression Profiles in Nasopharyngeal Carcinoma by Sequence Analysis. Front. Oncol. 10, 601. doi:10.3389/fonc.2020.00601
Yin, G., Zhang, B., and Li, J. (2019). miR-221-3p Promotes the Cell Growth of Non-Small Cell Lung Cancer by Targeting P-27. Mol. Med. Rep. 20 (1), 604–612. doi:10.3892/mmr.2019.10291
You, X., Vlatkovic, I., Babic, A., Will, T., Epstein, I., Tushev, G., et al. (2015). Neural Circular RNAs Are Derived from Synaptic Genes and Regulated by Development and Plasticity. Nat. Neurosci. 18 (4), 603–610. doi:10.1038/nn.3975
Zhang, Y., Zhang, X.-O., Chen, T., Xiang, J.-F., Yin, Q.-F., Xing, Y.-H., et al. (2013). Circular Intronic Long Noncoding RNAs. Mol. Cel 51 (6), 792–806. doi:10.1016/j.molcel.2013.08.017
Zhang, X., Jiang, P., Shuai, L., Chen, K., Li, Z., Zhang, Y., et al. (2016). miR-589-5p Inhibits MAP3K8 and Suppresses CD90+ Cancer Stem Cells in Hepatocellular Carcinoma. J. Exp. Clin. Cancer Res. 35 (1), 176. doi:10.1186/s13046-016-0452-6
Zhang, J., Zheng, Z., Wu, M., Zhang, L., Wang, J., Fu, W., et al. (2019a). The Natural Compound Neobractatin Inhibits Tumor Metastasis by Upregulating the RNA-Binding-Protein MBNL2. Cel Death Dis 10 (8), 554. doi:10.1038/s41419-019-1789-5
Zhang, P.-F., Wei, C.-Y., Huang, X.-Y., Peng, R., Yang, X., Lu, J.-C., et al. (2019b). Circular RNA circTRIM33-12 Acts as the Sponge of MicroRNA-191 to Suppress Hepatocellular Carcinoma Progression. Mol. Cancer 18 (1), 105. doi:10.1186/s12943-019-1031-1
Zhang, X., Wang, S., Wang, H., Cao, J., Huang, X., Chen, Z., et al. (2019c). Circular RNA circNRIP1 Acts as a microRNA-149-5p Sponge to Promote Gastric Cancer Progression via the AKT1/mTOR Pathway. Mol. Cancer 18 (1), 20. doi:10.1186/s12943-018-0935-5
Zhu, L., Liu, Y., Yang, Y., Mao, X. M., and Yin, Z. D. (2019). CircRNA ZNF609 Promotes Growth and Metastasis of Nasopharyngeal Carcinoma by Competing with microRNA-150-5p. Eur. Rev. Med. Pharmacol. Sci. 23 (7), 2817–2826. doi:10.26355/eurrev_201904_17558
Keywords: nasopharyngeal carcinoma (NCP), epstein-barr virus (EBV), circRNA, miRNA, network
Citation: Lin X, Wang S, Lin K, Zong J, Zheng Q, Su Y and Huang T (2021) Competitive Endogenous RNA Landscape in Epstein-Barr Virus Associated Nasopharyngeal Carcinoma. Front. Cell Dev. Biol. 9:782473. doi: 10.3389/fcell.2021.782473
Received: 24 September 2021; Accepted: 20 October 2021;
Published: 04 November 2021.
Edited by:
Liang Cheng, Harbin Medical University, ChinaReviewed by:
Jie Wang, Guangzhou Institutes of Biomedicine and Health (CAS), ChinaYungang Xu, Xi'an Jiaotong University, China
Copyright © 2021 Lin, Wang, Lin, Zong, Zheng, Su and Huang. This is an open-access article distributed under the terms of the Creative Commons Attribution License (CC BY). The use, distribution or reproduction in other forums is permitted, provided the original author(s) and the copyright owner(s) are credited and that the original publication in this journal is cited, in accordance with accepted academic practice. No use, distribution or reproduction is permitted which does not comply with these terms.
*Correspondence: Ying Su, empzdXlpbmdAMTI2LmNvbQ==; Tao Huang, dG9odWFuZ3Rhb0AxMjYuY29t
†These authors share first authorship