- 1Anaesthesia Department, China-Japan Union Hospital, JiLin University, Changchun, China
- 2School of Life Sciences, Shanghai University, Shanghai, China
- 3Department of Otorhinolaryngology Head and Neck Surgery, China-Japan Union Hospital of Jilin University, Changchun, China
Hearing loss is a total or partial inability to hear. Approximately 5% of people worldwide experience this condition. Hearing capacity is closely related to language, social, and basic emotional development; hearing loss is particularly serious in children. The pathogenesis of childhood hearing loss remains poorly understood. Here, we sought to identify new genes potentially associated with two types of hearing loss in children: congenital deafness and otitis media. We used a network-based method incorporating a random walk with restart algorithm, as well as a protein-protein interaction framework, to identify genes potentially associated with either pathogenesis. A following screening procedure was performed and 18 and 87 genes were identified, which potentially involved in the development of congenital deafness or otitis media, respectively. These findings provide novel biomarkers for clinical screening of childhood deafness; they contribute to a genetic understanding of the pathogenetic mechanisms involved.
Introduction
Deafness refers to a total or partial inability to hear, also known as hearing impairment or hearing loss (Olusanya et al., 2019). According to the World Health Organization, approximately 5% of people worldwide exhibit deafness or various extents of hearing impairment (Murray et al., 2019; Olusanya et al., 2019); approximately 10% of these people (34 million) are children (Murray et al., 2019). Although this number does not fully reflect the non-negligible threat imposed by hearing loss on human health, an independent report from the National Institute on Deafness and Other Communication Disorders of the United States revealed that the fight against deafness was urgent (Wass et al., 2019). In the USA, over 15% of all people currently exhibit hearing loss or have previously exhibited hearing loss (Moeller, 2000). Hearing loss is often age-associated; individuals over 60 years of age tend to have hearing impairments (Uchida et al., 2019). However, deafness or hearing loss is even more serious in children, because hearing is closely related to language-learning, social behavior, and basic emotional development (Trudeau et al., 2021). Therefore, an exploration of the pathological factors associated with childhood deafness is critical for child health and of considerable interest to researchers. The clinical pathogenesis of hearing loss in children is either congenital (Korver et al., 2017) or acquired (Pichichero, 2018). Congenital causes have been associated with genetic factors and family histories (Korver et al., 2017). X-linked hearing loss is the most typical form of congenital hearing loss, passed from mothers to their sons (O’brien et al., 2021). Genes PRPS1, POU3F4, SMPX, AIFM1, and COL4A6 have all been associated with X-linked hearing loss (Song et al., 2012). However, otitis media and ototoxicity also trigger childhood hearing loss (Vanneste and Page, 2019). Otitis media is a complex process that involves multiple infections and specific genetic susceptibilities (Vanneste and Page, 2019). Acute otitis media (the most common form of the condition) has been associated with infections by various bacteria including Streptococcus pneumoniae, Hemophilus influenzae, Moraxella catarrhalis, and Staphylococcus aureus (Deniz et al., 2018). Additionally, acute otitis media susceptibility and recurrence have been associated with genetic factors. In 2011, researchers in Helsinki University Central Hospital reported that genetic factors contributed to childhood recurrent acute otitis media in 38.5% of affected patients and chronic otitis media in 22.1% of affected patients, highlighting the substantial contributions of genetic traits to these conditions (Hafrén et al., 2012). Furthermore, genome-wide association studies have shown that particular genes, including FNDC1, are associated with otitis media (Van Ingen et al., 2016), validating the essential roles of genetics in otitis media-induced hearing loss. Notably, drug ototoxicity was not significantly associated with the genetic background (Lanvers-Kaminsky et al., 2017). In summary, both congenital deafness and environmental otitis media (i.e., the two major pathogeneses of childhood hearing loss) feature strong genetic predispositions.
Although major efforts have been made to describe the pathogenesis of childhood hearing loss, the underlying mechanism remains unclear; only a few genes are known or suspected to be associated with the disease. Here, we focused on congenital hearing loss and otitis media-related hearing loss; both are associated with clear genetic predispositions. We used DisGeNet (https://www.disgenet.org/) to generate a list of genes associated with hearing loss (Piñero et al., 2017); we then employed a network-based method to identify novel latent biomarkers and genetic traits predisposing to congenital and otitis media-associated hearing loss. We used a random walk with restart (RWR) algorithm (Kohler et al., 2008; Macropol et al., 2009) by setting genes associated with otitis media or congenital deafness as the seed nodes to a STRING [19] protein-protein interaction (PPI) network to discover new candidate genes. A following screening procedure was conducted to select essential candidates. Eighteen latent congenital genes and 87 otitis media-associated genes were identified; some were associated with either pathogenesis. These may serve as novel biomarkers for clinical deafness screening in children; they will help to identify the pathogenetic mechanisms involved.
Materials and Methods
Genes Associated with Hearing Loss in Children
We focused on genes associated with hearing loss in children. The American Speech-Language-Hearing Association (Alsarraf et al., 1998; Dhooge, 2003) defines such hearing loss in children as either acquired or associated with otitis media or congenital deafness. We downloaded the relevant genes from DisGeNet (Piñero et al., 2017) (https://www.disgenet.org/, version 7.0, accessed in April 2021). In total, 175 genes were associated with otitis media, while 72 were associated with congenital deafness and 2 were associated with acquired hearing loss; thus, we did not study acquired hearing loss. The genes associated with congenital deafness and otitis media are listed in Supplementary Tables S1, S2, respectively. We used a network-based method to identify novel candidate genes associated with either pathogenesis.
Network-Based Identification of Novel Genes
PPIs are widely used to explore protein or gene-related problems. Several studies have reported that compared with non-interacting proteins, interacting proteins are more likely to have similar functions (Ng et al., 2010; Hu et al., 2011; Chen et al., 2016a; Cai et al., 2017; Zhao et al., 2019; Gao et al., 2021). Such interactions can be used to identify novel genes that are associated with known disease-related genes. We used the STRING database (https://www.string-db.org/, version 10.0) (Szklarczyk et al., 2015) to construct a PPI network; we then applied the powerful, network RWR algorithm (Kohler et al., 2008; Macropol et al., 2009) to discover novel candidate genes associated with otitis media or congenital deafness. Human PPI information collected in STRING is contained in “9606.protein.links.v10.txt.gz”. Each PPI features two proteins identified by their Ensembl IDs, as well as a confidence score indicating the PPI strength. Each score ranges from 1 to 999 and is derived by considering several types of PPIs. In fact, PPIs in STRING can not only indicate the interactions between proteins but also reflect functional associations of proteins. Thus, they can widely measure protein associations. We used the PPIs to build a network in which all 19,247 proteins served as nodes. Two nodes were considered adjacent if and only if they formed a PPI; thus, each edge was a PPI. We assigned a weight to each edge for indicating the strengths of the PPI, which was defined as the confidence score of the corresponding PPI. The network was termed N.
The RWR algorithm is powerful. It simulates a walker that commences at a node set and then randomly moves in the network. The start nodes are termed seed nodes. The walker delivers probabilities of seed nodes to all other nodes in the network. Given a network and k seed nodes, each seed node is assigned a probability of 1/k; the other nodes are assigned probabilities of zero. These probabilities form a vector termed P0. The vector is repeatedly updated as follows:
where A is the column-wise, normalized adjacency matrix of the network and r is the restarting probability, which was set to 0.8 in this study. Updating stops when
We used the RWR program established by Li and Patra (Li and Patra, 2010). Genes associated with congenital deafness or otitis media were fed into the program, which ran on the PPI network N. Nodes with probabilities higher than 10−5 served as raw candidate genes for congenital deafness or otitis media.
Screening Procedure
Some raw candidate genes associated with congenital deafness or otitis media can be identified using a network-based method. However, several false-positives may be included in the results. To eliminate such genes and select only valid candidates, we used a screening procedure that featured three sequential tests.
Permutation Test
The RWR algorithm was executed on the PPI network N to discover raw candidate genes. The structure of N may influence the outcome. Some nodes are readily assigned high probabilities because of their special locations in the network. However, they may have low or no associations with congenital deafness or otitis media. Thus, there is a need to test the statistical significance of the probability that each raw candidate gene is valid. Accordingly, we randomly generated 1,000 gene sets, each of which had the same number of genes associated with congenital deafness or otitis media. For each gene set, such genes were set as the seed nodes of the RWR algorithm. Thus, each candidate gene was assigned a probability in each random gene set. When all 1,000 sets had been tested, each candidate gene had been assigned 1,000 probabilities. By comparing the probability on actual seed nodes to the probabilities on randomly generated sets, the statistical significance of each probability was revealed. We used the Z-score to evaluate significance as follows:
where g is a raw candidate gene identified by the network-based method,
Association Test
The second test directly evaluated the associations between candidate genes and congenital deafness or otitis media. For each candidate gene, such associations can be measured by associations between that gene and other genes associated with either condition. Proteins that interact in STRING always exhibit strong associations that can be quantified using confidence scores. For proteins p and q, the confidence score is denoted as
Genes with high MAS values are strongly associated with at least one gene linked to congenital deafness or otitis media. Thus, such genes may also be highly related to either condition. We set the threshold for selection of essential candidate genes to 900; this is the cutoff of the highest STRING confidence score.
Function Test
The last test further filtered candidate genes according to the similarities between their functional terms and the functional terms of genes associated with congenital deafness or otitis media. If the functional terms of a candidate gene are similar to the functional terms of a gene that is validly associated with either condition, that gene may also be linked to one of the conditions. We first used enrichment theory (Carmona-Saez et al., 2007; Huang et al., 2011; Huang et al., 2012; Chen et al., 2016b; Chen et al., 2019) to evaluate the associations between genes and functional terms (GO terms and KEGG pathway terms). Given one gene and one functional term, the gene set containing that gene and genes with which it interacted (in the PPI network of STRING) was constructed; another gene set containing genes annotated by the functional term was built. The associations between the gene and the functional term were calculated as the −log10 of the hypergeometric test p-value of the gene sets constructed above. For any gene g, its associations with all functional terms were computed and collected in a vector denoted
In a manner similar to MAS calculation, for each candidate gene g, the maximum function score (MFS) was computed as follows:
Essential genes can be selected by choosing an appropriate MAS threshold.
Functional Enrichment Analyses on Identified Genes
To explore biological functions associated with identified genes, we applied gene ontology (GO) enrichment analyses using R package topGO (https://bioconductor.org/packages/release/bioc/html/topGO.html, v.2.42.0). The threshold of p-value was set to 0.001 for selecting enriched GO terms in three subclasses: biological processes (BP), cellular components (CC) and molecular functions (MF).
Results
We sought genes associated with pediatric congenital deafness or otitis media. We used a network-based method to identify such genes. The entire procedure is illustrated in Figure 1. The numbers of genes remaining after each filtration step are listed in Table 1.
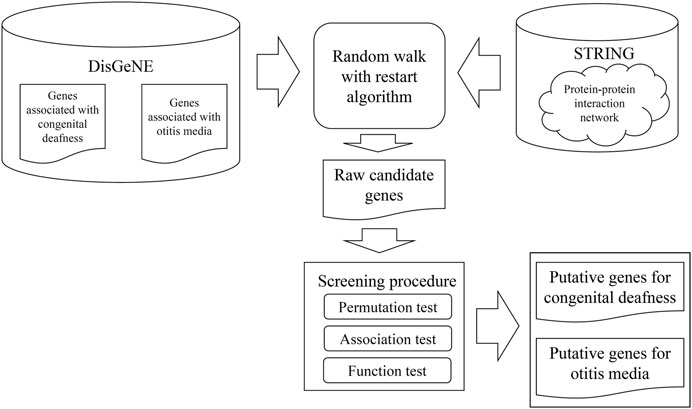
FIGURE 1. Procedures used to identify new genes that might have roles in the development of childhood congenital deafness or otitis media-mediated hearing loss. Genes associated with either pathogenesis were retrieved from DisGeNE and the STRING protein-protein interaction networks were explored. The genes and networks were fed into a random walk with restart algorithm; we sought to discover new candidate genes. These genes were screened using three tests to select putative genes.
Congenital Deafness
Genes associated with congenital deafness were fed into the RWR algorithm, which ran on PPI network N. Each node in the network was assigned a probability. The selection threshold for raw candidate genes was set to 10−5; this yielded 5,426 genes (Supplementary Table S3). We then engaged in screening (i.e., filtration) to identify essential genes. First, we used the permutation test to evaluate the statistical significance of probability that each raw candidate gene was essential; the Z-scores for all genes are listed in Supplementary Table S3. In total, 367 candidate genes were assigned Z-scores greater than 1.96. These were fed into the association test, which assigned an MAS to each gene (Supplementary Table S3). At a threshold of 900, 117 genes were selected; these were finally evaluated using the function test. The MFS values are listed in Supplementary Table S3. At an MFS threshold of 0.9, 18 genes were chosen. These “putative genes” were considered to be closely associated with congenital deafness; they are listed in Supplementary Table S4.
For the obtained putative genes, their associations with validated genes were investigated. We extracted all PPIs between putative and validated genes. The confidence scores of these PPIs are illustrated in a heat map, as shown in Figure 2. It can be observed that each putative gene had some interacting genes with confidence scores no less than 900, suggesting strong associations with validated genes. This can be further inferred that putative genes had special relationships with congenital deafness.
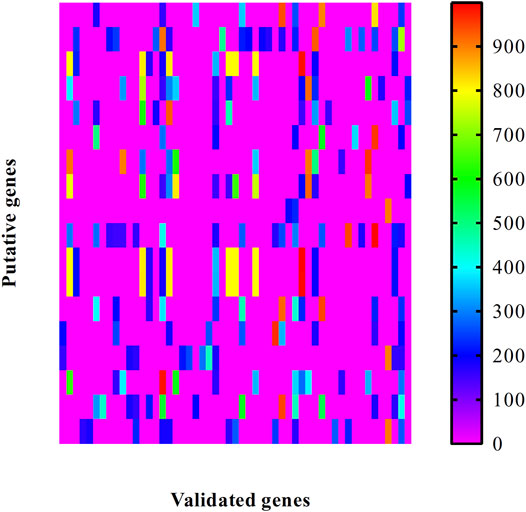
FIGURE 2. Heat map to illustrate the associations between putative genes and validated ones associated with congenital deafness. Row represents putative genes and column indicates validated genes.
Otitis Media
We used the method described above to identify putative otitis media-associated genes. The RWR algorithm with genes associated with otitis media as seed nodes was performed on the PPI network N. The probabilities of all nodes were obtained. We selected nodes with probabilities over 10−5; this yielded 5,631 genes (Supplementary Table S5). These genes were filtered as described above. The Z-scores, MAS values, and MFS values are listed in Supplementary Table S5. Use of thresholds of 1.96 for the Z-score, 900 for the MAS, and 0.96 for the MFS yielded 87 “putative genes” (Supplementary Table S6).
Likewise, the PPIs between putative and validated genes were investigated. A heat map was plotted to indicate the strength of these PPIs, as shown in Figure 3. Also, each putative genes had one or more interacting genes with highest confidence (confidence score ≥900). It is suggested that these putative genes may have special associations with otitis media.
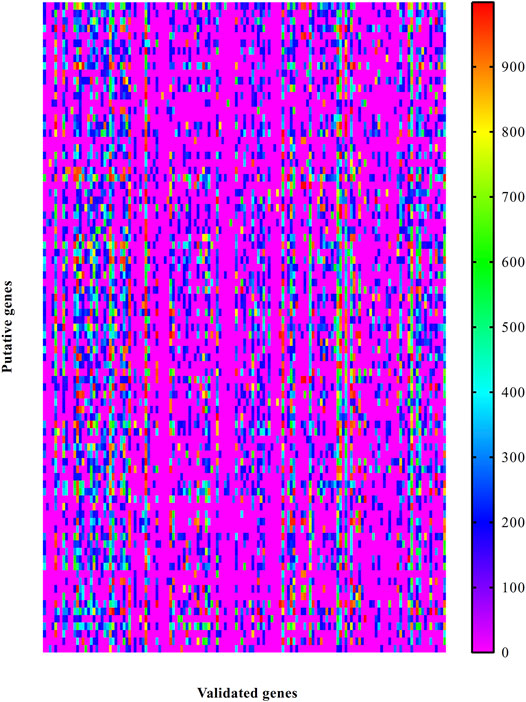
FIGURE 3. Heat map to illustrate the associations between putative genes and validated ones associated with otitis media. Row represents putative genes and column indicates validated genes.
GO Enrichment Analyses on Putative Genes
GO Enrichment Analyses on Congenital Deafness Associated Putative Genes
For congenital deafness, 18 putative genes were obtained. These genes were set as gene of interest and all available genes were set as background for topGO. 18 enriched GO terms were obtained, which are provided in Supplementary Table S7. These terms and their p-values are also illustrated in Figure 4. Among these GO terms, eight were BP GO terms, six were CC GO terms and four were MF GO terms.
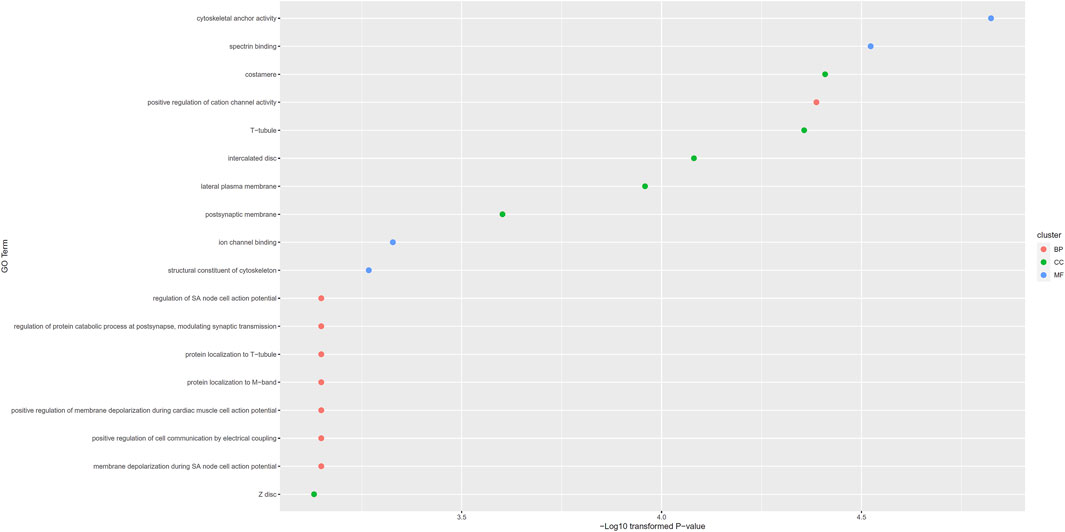
FIGURE 4. Gene ontology (GO) enrichment results for putative genes associated with congenital deafness. GO terms with p-value less than 0.001 are selected and ranked by their p-values.
GO Enrichment Analyses on Otitis Media Associated Putative Genes
For 87 putative genes associated with otitis media, we did the same enrichment analysis. Results are available in Supplementary Table S8. We obtained 65 enriched GO terms. These GO terms and their p-values are shown in Figure 5. Of these 65 GO terms, fifty-two belonged to BP, five belonged to CC and eight belonged to MF.
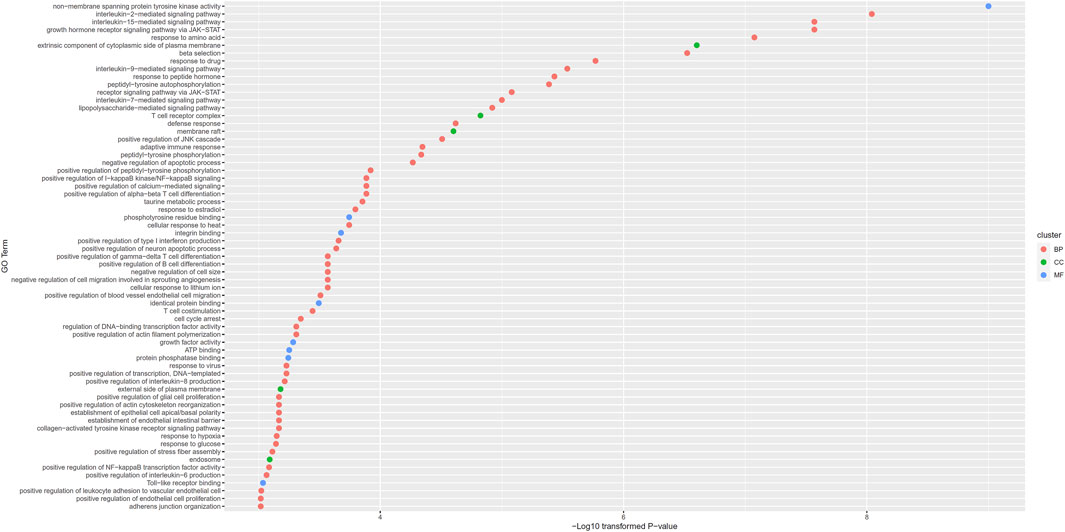
FIGURE 5. Gene ontology (GO) enrichment results for putative genes associated with otitis media. GO terms with p-value less than 0.001 are selected and ranked by their p-values.
Discussion
We used a network-based method to identify putative genes associated with congenital deafness or otitis media. Below, we discuss some genes.
Putative Genes Associated with Congenital Deafness
We identified 18 putative genes, of which 5 were chosen for detailed analysis (Table 2). The first is PRKACB (ENSP00000359719), which encodes a catalytic subunit of cAMP-dependent protein kinase. The enzyme is expressed in hearing-associated organs in utero. In 2017, researchers from Southeast University showed that mouse PRKACB regulated the development of Lgr5+ hair cells (inner ear progenitor cells) (Cheng et al., 2017). Therefore, PRKACB is functionally associated with cochlear development; the cochlea is a sensorineural hearing organ. Cochlear impairment and abnormalities are reportedly associated with congenital hearing loss in children (O’malley et al., 1995; Korver et al., 2017; Van Wieringen et al., 2019). It is thus reasonable to expect that a regulator of cochlear development, such as PRKACB, would be associated with congenial pediatric deafness. We identified another putative gene with a similar biological function. PRKACG (ENSP00000366488) encodes another protein of the same complex. In 2016, researchers from the University of Bristol confirmed that the gain-of-function variant DIAPH1 caused macrothrombocytopenia and hearing loss (Stritt et al., 2016). PRKACG acts downstream of DIAPH1, thus participating in DIAPH1-related biological effects. PRKACG may also be functionally connected to pediatric hearing loss.
The next putative gene is PAX2 (ENSP00000396259), which is regarded as a key transcription factor that regulates the development of multiple systems, including the central nervous system (Ziman et al., 2001) and the eyes (Adam et al., 1993). In 2006, researchers from the McLaughlin Research Institute for Biomedical Sciences reported that PAX2 interacted with EYA1 to regulate the development of sensory regions in the inner ear (Zou et al., 2006). Developmental abnormalities of these regions are directly associated with congenital hearing loss (Kimura et al., 2018), implying that PAX2 is a relevant putative gene involved in congenital pediatric deafness.
PRKX (ENSP00000262848) is also associated with congenital pediatric hearing loss. A 2019 review concerning chromosomal aberrations associated with endocrine abnormalities in children confirmed that PRKX regulated the development of hearing (Haltrich, 2019). PRKX is located on the X chromosome; it is functionally connected to X-linked congenital hearing loss (Song et al., 2012).
The next putative gene is MATK (ENSP00000378485); this regulates signal transduction in hematopoietic cells (Grgurevich et al., 1997; Lee et al., 2006). In 2017, a clinical case report in JAMA Otolaryngology—Head and Neck Surgery stated that MATK was associated with unilateral hearing loss and otorrhea (Costello et al., 2017). Acute megakaryoblastic leukemia has been functionally connected to unilateral, congenital hearing loss; the pathogenetic backgrounds are related (Costello et al., 2017). MATK encodes megakaryocyte-associated tyrosine kinase, which is structurally similar to C-terminal Src kinase; notably, megakaryocyte-associated tyrosine kinase is associated with acute megakaryoblastic leukemia (Jhun et al., 1995). Therefore, MATK might be involved in the development of ear tumors that cause adaptive hearing loss.
Putative Genes Associated with Otitis Media
We identified 87 genes putatively associated with otitis media (Supplementary Table S6); we subjected 5 of these genes to detailed analysis (Table 3). The first such gene is RAC3 (ENSP00000304283). Although there is insufficient direct evidence that RAC3 is involved in otitis media, a clinical genomic database (ClinVar Miner) (Henrie et al., 2018) indicates that the Talkowski Laboratory of Massachusetts General Hospital has demonstrated associations of RAC3 variants with otitis media. The next gene is HCK (ENSP00000365012); this member of the Src tyrosine kinase family regulates the innate immune response (Ernst et al., 2002). HCK was previously reported to be specifically associated with chronic otitis media and its major chronic complications in children with hearing loss (Suri et al., 2016), validating our findings. The next putative gene is ITK (ENSP00000398655), which encodes an IL2-and T cell-associated kinase. In 2008, the gene was reported to potentially mediate the inflammation of otitis media (Juhn et al., 2008). Furthermore, a report concerning early diagnosis of PI3Kδ syndrome in a 2-year-old girl revealed an association between ITK deficiency and recurrent otitis media (Saettini et al., 2017).
FGR (ENSP00000363115; also known as SRC2), another member of the Src tyrosine kinase family, is also associated with otitis media. This gene has roles in immune responses against pathogens in multiple organs, including ears (Kim et al., 2008). Additionally, the gene has been widely reported to participate in Epstein–Barr virus-associated malignancies (Klein et al., 1988). In 2017, Epstein–Barr virus infection was confirmed as a major etiological and pathological factor for secretory otitis media in children (Vogelnik and Matos, 2017), validating the link between FGR and otitis media.
CDC42 (ENSP00000314458) is an immune system-associated gene; we found that it was closely associated with otitis media. In 2021, CDC42 deficiency was shown to be associated with recurrent pneumonia, otitis media, and bacteremia (Kashani et al., 2021). CDC42 interacts with another effector gene, RAC1 (Hoppe and Swanson, 2004); a homolog of RAC1 (i.e., RAC3, discussed above) was shown to be associated with hearing loss, confirming that CDC42 is linked to otitis media.
In summary, several putative genes are associated with the two types of pediatric deafness. Their identification may provide insights concerning the pathogeneses involved.
Functional Enrichment Analyses on Putative Genes
For GO functional enrichment analyses on putative genes yielded by our computational method, multiple significant GO terms were identified. The detailed analyses on the top three enriched GO terms ranking by p-values for congenital deafness and otitis media were presented below.
For congenital deafness, the first enriched GO term is cytoskeletal anchor activity (GO:0008093). According to recent publications, mutations in cytoskeletal encoding proteins have been shown to be associated with congenital deafness (Riazuddin et al., 2006), reflecting the potential associations between congenital deafness and cytoskeletal anchor activity. The second enriched term is spectrin binding (GO:0030507). In 2017, a recessive mutation on spectrin associated gene has been shown to be associated with congenital central deafness (Knierim et al., 2017), validating this result. Furthermore, costamere (GO:0043034) is the third enriched GO term (in CC) associated with congenital deafness. According to recent next-generation sequencing analyses (Schraders et al., 2011), costameres has been shown to be associated with progressive hearing impairment.
More GO terms were enriched by putative genes associated with otitis media, including non−membrane spanning protein tyrosine kinase activity (GO:0004715) and interleukin mediated signaling pathway (GO:0038100, GO0035723). Non-membrane spanning protein tyrosine kinase has been shown to be associated with specific inflammatory effects and pathogen infections (Gu et al., 2009; Rocha-Sanchez et al., 2013). Considering that otitis media is associated with infection and inflammatory effects around middle ears, it is reasonable for otitis media associated genes to enrich in inflammatory effects. As for interleukin mediated signaling pathways, middle ear inflammation has been shown to be associated with interleukin related signaling pathways, validating this result (Kerschner et al., 2006; Shi et al., 2014).
Shared Putative Genes Associated with Both Congenital Deafness and Otitis Media
By comparing the putative genes associated with congenital deafness and otitis media, only one shared gene CDH1 (ENSG00000039068) was identified. The pathogenesis of congenital deafness and otitis media are totally different according to recent studies. Congenital deafness means the hearing loss is present at birth linking the pathogenesis to genetic factors or stimulations during pregnancy. However, as for otitis media, generally, otitis media is caused by infections and happens after birth. CDH1 has been widely reported to be associated with hearing loss (Friedman and Avraham, 2009; Kanavy et al., 2019). Specifically, CDH1 has been reported to be associated with congenital deafness due to the pathogenic alteration of inner ear but not middle ear (Friedman and Avraham, 2009), which has totally different pathogenic regions comparing with otitis media. As for otitis media, CDH1 has been shown to participate in the pathogenesis of otitis media via regulation on the inflammatory proliferative responses against infections (Kurabi et al., 2013).Therefore, although both subtypes of hearing loss have been shown to be associated with gene CDH1, the contribution and regulatory role of CDH1 on them are totally different, reflecting the complex regulatory mechanisms for childhood hearing loss.
In summary, CHD1 is associated with two types of pediatric deafness. Its dentification may provide insights concerning the pathogeneses involved.
Conclusion
We used a network-based method to identify new candidate genes involved in childhood hearing loss caused by congenital deafness and otitis media. The genes included PRKACB, PAX2, PRKX, PRKACG, MATK, RAC3, HCK, ITK, FGR, and CDC42. They may be involved in the pathogenesis of childhood hearing loss.
Data Availability Statement
Publicly available datasets were analyzed in this study. This data can be found here: https://www.disgenet.org/.
Author Contributions
LL designed the study. FL, XF and SJD performed the experiments. FL and XF analyzed the results. FL and XF wrote the manuscript. All authors contributed to the research and reviewed the manuscript.
Funding
This study was granted by the Finance Department of Jilin Province, China (Grant 2020scz49).
Conflict of Interest
The authors declare that the research was conducted in the absence of any commercial or financial relationships that could be construed as a potential conflict of interest.
Publisher’s Note
All claims expressed in this article are solely those of the authors and do not necessarily represent those of their affiliated organizations, or those of the publisher, the editors and the reviewers. Any product that may be evaluated in this article, or claim that may be made by its manufacturer, is not guaranteed or endorsed by the publisher.
Supplementary Material
The Supplementary Material for this article can be found online at: https://www.frontiersin.org/articles/10.3389/fcell.2021.783500/full#supplementary-material
References
Adam, M. P., Ardinger, H. H., Pagon, R. A., Wallace, S. E., Bean, L. J. H., Mirzaa, G., et al. (1993). GeneReviews. Seattle (WA): University of Washington.
Alsarraf, R., Jung, C. J., Crowley, C., Perkins, J., and Gates, G. A. (1998). Otitis media Health Status Evaluation: a Pilot Study for the Investigation of Cost-Effective Outcomes of Recurrent Acute Otitis media Treatment. Ann. Otol Rhinol Laryngol. 107, 120–128. doi:10.1177/000348949810700207
Cai, Y.-D., Zhang, Q., Zhang, Y.-H., Chen, L., and Huang, T. (2017). Identification of Genes Associated with Breast Cancer Metastasis to Bone on a Protein-Protein Interaction Network with a Shortest Path Algorithm. J. Proteome Res. 16, 1027–1038. doi:10.1021/acs.jproteome.6b00950
Carmona-Saez, P., Chagoyen, M., Tirado, F., Carazo, J. M., and Pascual-Montano, A. (2007). GENECODIS: a Web-Based Tool for Finding Significant Concurrent Annotations in Gene Lists. Genome Biol. 8, R3. doi:10.1186/gb-2007-8-1-r3
Chen, L., Pan, X., Zhang, Y.-H., Liu, M., Huang, T., and Cai, Y.-D. (2019). Classification of Widely and Rarely Expressed Genes with Recurrent Neural Network. Comput. Struct. Biotechnol. J. 17, 49–60. doi:10.1016/j.csbj.2018.12.002
Chen, L., Xing, Z., Huang, T., Shu, Y., Huang, G., and Li, H.-P. (2016a). Application of the Shortest Path Algorithm for the Discovery of Breast Cancer-Related Genes. Cbio 11, 51–58. doi:10.2174/1574893611666151119220024
Chen, L., Zhang, Y.-H., Zheng, M., Huang, T., and Cai, Y.-D. (2016b). Identification of Compound-Protein Interactions through the Analysis of Gene Ontology, KEGG Enrichment for Proteins and Molecular Fragments of Compounds. Mol. Genet. Genomics 291, 2065–2079. doi:10.1007/s00438-016-1240-x
Cheng, C., Guo, L., Lu, L., Xu, X., Zhang, S., Gao, J., et al. (2017). Characterization of the Transcriptomes of Lgr5+ Hair Cell Progenitors and Lgr5- Supporting Cells in the Mouse Cochlea. Front. Mol. Neurosci. 10, 122. doi:10.3389/fnmol.2017.00122
Costello, M. S., Stevens, S., and Samy, R. N. (2017). Unilateral Hearing Loss and Otorrhea. JAMA Otolaryngol. Head Neck Surg. 143, 727–728. doi:10.1001/jamaoto.2016.3824
Deniz, Y., Van Uum, R. T., De Hoog, M. L. A., Schilder, A. G. M., Damoiseaux, R. A. M. J., and Venekamp, R. P. (2018). Impact of Acute Otitis media Clinical Practice Guidelines on Antibiotic and Analgesic Prescriptions: a Systematic Review. Arch. Dis. Child. 103, 597–602. doi:10.1136/archdischild-2017-314103
Dhooge, I. J. M. (2003). Risk Factors for the Development of Otitis media. Curr. Allergy Asthma Rep. 3, 321–325. doi:10.1007/s11882-003-0092-8
Ernst, M., Inglese, M., Scholz, G. M., Harder, K. W., Clay, F. J., Bozinovski, S., et al. (2002). Constitutive Activation of the SRC Family Kinase Hck Results in Spontaneous Pulmonary Inflammation and an Enhanced Innate Immune Response. J. Exp. Med. 196, 589–604. doi:10.1084/jem.20020873
Friedman, L. M., and Avraham, K. B. (2009). MicroRNAs and Epigenetic Regulation in the Mammalian Inner Ear: Implications for Deafness. Mamm. Genome 20, 581–603. doi:10.1007/s00335-009-9230-5
Gao, J., Hu, B., and Chen, L. (2021). A Path-Based Method for Identification of Protein Phenotypic Annotations. Cbio 16, 1214–1222. doi:10.2174/1574893616666210531100035
Grgurevich, S., Linnekin, D., Musso, T., Zhang, X., Modi, W., Varesio, L., et al. (1997). The Csk-like proteins Lsk, Hyl, and Matk represent the same Csk homologous kinase (Chk) and are regulated by stem cell factor in the megakaryoblastic cell line MO7e. Growth Factors 14, 103–115. doi:10.3109/08977199709021514
Gu, D., Sater, A. K., Ji, H., Cho, K., Clark, M., Stratton, S. A., et al. (2009). Xenopus δ-catenin Is Essential in Early Embryogenesis and Is Functionally Linked to Cadherins and Small GTPases. J. Cel. Sci. 122, 4049–4061. doi:10.1242/jcs.031948
Hafrén, L., Kentala, E., Järvinen, T. M., Leinonen, E., Onkamo, P., Kere, J., et al. (2012). Genetic Background and the Risk of Otitis media. Int. J. Pediatr. Otorhinolaryngol. 76, 41–44. doi:10.1016/j.ijporl.2011.09.026
Haltrich, I. (2019). Chromosomal Aberrations with Endocrine Relevance (Turner Syndrome, Klinefelter Syndrome, Prader-Willi Syndrome). Exp. Suppl. 111, 443–473. doi:10.1007/978-3-030-25905-1_20
Henrie, A., Hemphill, S. E., Ruiz-Schultz, N., Cushman, B., Distefano, M. T., Azzariti, D., et al. (2018). ClinVar Miner: Demonstrating Utility of a Web-Based Tool for Viewing and Filtering ClinVar Data. Hum. Mutat. 39, 1051–1060. doi:10.1002/humu.23555
Hoppe, A. D., and Swanson, J. A. (2004). Cdc42, Rac1, and Rac2 Display Distinct Patterns of Activation during Phagocytosis. MBoC 15, 3509–3519. doi:10.1091/mbc.e03-11-0847
Hu, L., Huang, T., Shi, X., Lu, W.-C., Cai, Y.-D., and Chou, K.-C. (2011). Predicting Functions of Proteins in Mouse Based on Weighted Protein-Protein Interaction Network and Protein Hybrid Properties. PloS one 6, e14556. doi:10.1371/journal.pone.0014556
Huang, T., Chen, L., Cai, Y.-D., and Chou, K.-C. (2011). Classification and Analysis of Regulatory Pathways Using Graph Property, Biochemical and Physicochemical Property, and Functional Property. PLoS ONE 6, e25297. doi:10.1371/journal.pone.0025297
Huang, T., Zhang, J., Xu, Z.-P., Hu, L.-L., Chen, L., Shao, J.-L., et al. (2012). Deciphering the Effects of Gene Deletion on Yeast Longevity Using Network and Machine Learning Approaches. Biochimie 94, 1017–1025. doi:10.1016/j.biochi.2011.12.024
Jhun, B. H., Rivnay, B., Price, D., and Avraham, H. (1995). The MATK Tyrosine Kinase Interacts in a Specific and SH2-dependent Manner with C-Kit. J. Biol. Chem. 270, 9661–9666. doi:10.1074/jbc.270.16.9661
Juhn, S. K., Jung, M.-K., Hoffman, M. D., Drew, B. R., Preciado, D. A., Sausen, N. J., et al. (2008). The Role of Inflammatory Mediators in the Pathogenesis of Otitis media and Sequelae. Clin. Exp. Otorhinolaryngol. 1, 117–138. doi:10.3342/ceo.2008.1.3.117
Kanavy, D. M., Mcnulty, S. M., Jairath, M. K., Brnich, S. E., Bizon, C., Powell, B. C., et al. (2019). Comparative Analysis of Functional Assay Evidence Use by ClinGen Variant Curation Expert Panels. Genome Med. 11, 77–19. doi:10.1186/s13073-019-0683-1
Kashani, P., Marwaha, A., Feanny, S., Kim, V. H.-D., Atkinson, A. R., Leon-Ponte, M., et al. (2021). Progressive Decline of T and B Cell Numbers and Function in a Patient with CDC42 Deficiency. Immunol. Res. 69, 53–58. doi:10.1007/s12026-020-09168-y
Kerschner, J. E., Yang, C., Burrows, A., and Cioffi, J. A. (2006). Signaling Pathways in Interleukin-1??-Mediated Middle Ear Mucin Secretion. The Laryngoscope 116, 207–211. doi:10.1097/01.mlg.0000191467.63650.9e
Kim, Y.-C., Kim, S.-Y., Choi, D., Ryu, C.-M., and Park, J. M. (2008). Molecular Characterization of a Pepper C2 Domain-Containing SRC2 Protein Implicated in Resistance against Host and Non-host Pathogens and Abiotic Stresses. Planta 227, 1169–1179. doi:10.1007/s00425-007-0680-2
Kimura, Y., Masuda, T., and Kaga, K. (2018). Vestibular Function and Gross Motor Development in 195 Children with Congenital Hearing Loss-Assessment of Inner Ear Malformations. Otol Neurotol 39, 196–205. doi:10.1097/mao.0000000000001685
Klein, C., Busson, P., Tursz, T., Young, L. S., and Raab-Traub, N. (1988). Expression of Thec-Fgr Related Transcripts in Epstein-Barr Virus-Associated Malignancies. Int. J. Cancer 42, 29–35. doi:10.1002/ijc.2910420107
Knierim, E., Gill, E., Seifert, F., Morales-Gonzalez, S., Unudurthi, S. D., Hund, T. J., et al. (2017). A Recessive Mutation in Beta-IV-Spectrin (SPTBN4) Associates with Congenital Myopathy, Neuropathy, and central Deafness. Hum. Genet. 136, 903–910. doi:10.1007/s00439-017-1814-7
Köhler, S., Bauer, S., Horn, D., and Robinson, P. N. (2008). Walking the Interactome for Prioritization of Candidate Disease Genes. Am. J. Hum. Genet. 82, 949–958. doi:10.1016/j.ajhg.2008.02.013
Korver, A. M. H., Smith, R. J. H., Van Camp, G., Schleiss, M. R., Bitner-Glindzicz, M. A. K., Lustig, L. R., et al. (2017). Congenital Hearing Loss. Nat. Rev. Dis. Primers 3, 16094. doi:10.1038/nrdp.2016.94
Kurabi, A., Pak, K., Dang, X., Coimbra, R., Eliceiri, B. P., Ryan, A. F., et al. (2013). Ecrg4 Attenuates the Inflammatory Proliferative Response of Mucosal Epithelial Cells to Infection. PloS one 8, e61394. doi:10.1371/journal.pone.0061394
Lanvers-Kaminsky, C., Zehnhoff-Dinnesen, A. a., Parfitt, R., and Ciarimboli, G. (2017). Drug-induced Ototoxicity: Mechanisms, Pharmacogenetics, and Protective Strategies. Clin. Pharmacol. Ther. 101, 491–500. doi:10.1002/cpt.603
Lee, B.-C., Avraham, S., Imamoto, A., and Avraham, H. K. (2006). Identification of the Nonreceptor Tyrosine Kinase MATK/CHK as an Essential Regulator of Immune Cells Using Matk/CHK-Deficient Mice. Blood 108, 904–907. doi:10.1182/blood-2005-12-4885
Li, Y., and Patra, J. C. (2010). Genome-wide Inferring Gene-Phenotype Relationship by Walking on the Heterogeneous Network. Bioinformatics 26, 1219–1224. doi:10.1093/bioinformatics/btq108
Macropol, K., Can, T., and Singh, A. K. (2009). RRW: Repeated Random Walks on Genome-Scale Protein Networks for Local Cluster Discovery. BMC bioinformatics 10, 283. doi:10.1186/1471-2105-10-283
Moeller, M. P. (2000). Early Intervention and Language Development in Children Who Are Deaf and Hard of Hearing. Pediatrics 106, E43. doi:10.1542/peds.106.3.e43
Murray, J. J., Hall, W. C., and Snoddon, K. (2019). Education and Health of Children with Hearing Loss: the Necessity of Signed Languages. Bull. World Health Organ. 97, 711–716. doi:10.2471/blt.19.229427
Ng, K.-L., Ciou, J.-S., and Huang, C.-H. (2010). Prediction of Protein Functions Based on Function-Function Correlation Relations. Comput. Biol. Med. 40, 300–305. doi:10.1016/j.compbiomed.2010.01.001
O'brien, A., Aw, W. Y., Tee, H. Y., Naegeli, K. M., Bademci, G., Tekin, M., et al. (2021). Confirmation of COL4A6 Variants in X-Linked Nonsyndromic Hearing Loss and its Clinical Implications. Eur. J. Hum. Genet. doi:10.1038/s41431-021-00881-2
O'malley, B. W., Li, D., and Turner, D. S. (1995). Hearing Loss and Cochlear Abnormalities in the Congenital Hypothyroid (Hyt/hyt) Mouse. Hearing Res. 88, 181–189. doi:10.1016/0378-5955(95)00111-g
Olusanya, O. B., Davis, A. C., and Hoffman, H. J. (2019). Hearing Loss: Rising Prevalence and Impact. Bull. World Health Organ. 97, 646–646A. doi:10.2471/BLT.19.224683
Pichichero, M. E. (2018). Helping Children with Hearing Loss from Otitis media with Effusion. The Lancet 392, 533–534. doi:10.1016/s0140-6736(18)31862-2
Piñero, J., Bravo, A., Queralt-Rosinach, N., Gutiérrez-Sacristán, A., Deu-Pons, J., Centeno, E., et al. (2017). DisGeNET: a Comprehensive Platform Integrating Information on Human Disease-Associated Genes and Variants. Nucleic Acids Res. 45, D833–D839. doi:10.1093/nar/gkw943
Riazuddin, S., Khan, S. N., Ahmed, Z. M., Ghosh, M., Caution, K., Nazli, S., et al. (2006). Mutations in TRIOBP, Which Encodes a Putative Cytoskeletal-Organizing Protein, Are Associated with Nonsyndromic Recessive Deafness. Am. J. Hum. Genet. 78, 137–143. doi:10.1086/499164
Rocha-Sanchez, S. M., Scheetz, L. R., Siddiqi, S., Weston, M. W., Smith, L. M., Dempsey, K., et al. (2013). Lack of Rbl1/p107 Effects on Cell Proliferation and Maturation in the Inner Ear. Jbbs 03, 534–555. doi:10.4236/jbbs.2013.37056
Saettini, F., Pelagatti, M. A., Sala, D., Moratto, D., Giliani, S., Badolato, R., et al. (2017). Early Diagnosis of PI3Kδ Syndrome in a 2 Years Old Girl with Recurrent Otitis and Enlarged Spleen. Immunol. Lett. 190, 279–281. doi:10.1016/j.imlet.2017.08.021
Schraders, M., Haas, S. A., Weegerink, N. J. D., Oostrik, J., Hu, H., Hoefsloot, L. H., et al. (2011). Next-generation Sequencing Identifies Mutations of SMPX, Which Encodes the Small Muscle Protein, X-Linked, as a Cause of Progressive Hearing Impairment. Am. J. Hum. Genet. 88, 628–634. doi:10.1016/j.ajhg.2011.04.012
Shi, J., Li, J., Guan, H., Cai, W., Bai, X., Fang, X., et al. (2014). Anti-fibrotic Actions of Interleukin-10 against Hypertrophic Scarring by Activation of PI3K/AKT and STAT3 Signaling Pathways in Scar-Forming Fibroblasts. PloS one 9, e98228. doi:10.1371/journal.pone.0098228
Song, M. H., Lee, K. Y., Choi, J. Y., Bok, J., and Kim, U. K. (2012). Nonsyndromic X-Linked Hearing Loss. Front. Biosci. (Elite Ed. 4, 924–933. doi:10.2741/430
Stritt, S., Nurden, P., Turro, E., Greene, D., Jansen, S. B., Westbury, S. K., et al. (2016). A Gain-Of-Function Variant in DIAPH1 Causes Dominant Macrothrombocytopenia and Hearing Loss. Blood 127, 2903–2914. doi:10.1182/blood-2015-10-675629
Suri, D., Rawat, A., and Singh, S. (2016). X-linked Agammaglobulinemia. Indian J. Pediatr. 83, 331–337. doi:10.1007/s12098-015-2024-8
Szklarczyk, D., Franceschini, A., Wyder, S., Forslund, K., Heller, D., Huerta-Cepas, J., et al. (2015). STRING V10: Protein-Protein Interaction Networks, Integrated over the Tree of Life. Nucleic Acids Res. 43, D447–D452. doi:10.1093/nar/gku1003
Trudeau, S., Anne, S., Otteson, T., Hopkins, B., Georgopoulos, R., and Wentland, C. (2021). Diagnosis and Patterns of Hearing Loss in Children with Severe Developmental Delay. Am. J. Otolaryngol. 42, 102923. doi:10.1016/j.amjoto.2021.102923
Uchida, Y., Sugiura, S., Nishita, Y., Saji, N., Sone, M., and Ueda, H. (2019). Age-related Hearing Loss and Cognitive Decline - the Potential Mechanisms Linking the Two. Auris Nasus Larynx 46, 1–9. doi:10.1016/j.anl.2018.08.010
Van Ingen, G., Li, J., Goedegebure, A., Pandey, R., Li, Y. R., March, M. E., et al. (2016). Genome-wide Association Study for Acute Otitis media in Children Identifies FNDC1 as Disease Contributing Gene. Nat. Commun. 7, 12792. doi:10.1038/ncomms12792
Van Wieringen, A., Boudewyns, A., Sangen, A., Wouters, J., and Desloovere, C. (2019). Unilateral Congenital Hearing Loss in Children: Challenges and Potentials. Hearing Res. 372, 29–41. doi:10.1016/j.heares.2018.01.010
Vanneste, P., and Page, C. (2019). Otitis media with Effusion in Children: Pathophysiology, Diagnosis, and Treatment. A Review. J. Otology 14, 33–39. doi:10.1016/j.joto.2019.01.005
Vogelnik, K., and Matos, A. (2017). Facial Nerve Palsy Secondary to Epstein-Barr Virus Infection of the Middle Ear in Pediatric Population May Be More Common Than We Think. Wien Klin Wochenschr 129, 844–847. doi:10.1007/s00508-017-1259-y
Wass, M., Ching, T. Y. C., Cupples, L., Wang, H.-C., Lyxell, B., Martin, L., et al. (2019). Orthographic Learning in Children Who Are Deaf or Hard of Hearing. Lshss 50, 99–112. doi:10.1044/2018_lshss-17-0146
Zhao, R., Chen, L., Zhou, B., Guo, Z.-H., Wang, S., and Aorigele, (2019). Recognizing Novel Tumor Suppressor Genes Using a Network Machine Learning Strategy. IEEE Access 7, 155002–155013. doi:10.1109/access.2019.2949415
Ziman, M. R., Rodger, J., Chen, P., Papadimitriou, J. M., Dunlop, S. A., and Beazley, L. D. (2001). Pax Genes in Development and Maturation of the Vertebrate Visual System: Implications for Optic Nerve Regeneration. Histol. Histopathol 16, 239–249. doi:10.14670/HH-16.239
Keywords: hearing loss, children, random walk with restart, protein-protein interaction, biomarker
Citation: Liang F, Fu X, Ding S and Li L (2021) Use of a Network-Based Method to Identify Latent Genes Associated with Hearing Loss in Children. Front. Cell Dev. Biol. 9:783500. doi: 10.3389/fcell.2021.783500
Received: 26 September 2021; Accepted: 15 November 2021;
Published: 29 November 2021.
Edited by:
Liang Cheng, Harbin Medical University, ChinaReviewed by:
Xuefeng Gu, Shanghai University of Medicine and Health Sciences, ChinaWei Kong, Shanghai Maritime University, China
Copyright © 2021 Liang, Fu, Ding and Li. This is an open-access article distributed under the terms of the Creative Commons Attribution License (CC BY). The use, distribution or reproduction in other forums is permitted, provided the original author(s) and the copyright owner(s) are credited and that the original publication in this journal is cited, in accordance with accepted academic practice. No use, distribution or reproduction is permitted which does not comply with these terms.
*Correspondence: Lin Li, bGlsaW4wMUBqbHUuZWR1LmNu
†These authors have contributed equally to this work