Prognosis and Immunotherapy Response With a Novel Golgi Apparatus Signature-Based Formula in Lung Adenocarcinoma
- 1Guangdong Provincial Key Laboratory of Malignant Tumor Epigenetics and Gene Regulation, Department of Medical Oncology, Center of Phase I Clinical Trial, Center of Breast Tumor, Sun Yat-Sen Memorial Hospital, Sun Yat-Sen University, Guangzhou, China
- 2Department of Hematology, Xiangya Hospital, Central South University, Changsha Hunan, China
- 3Artificial Intelligence & Digital Media Concentration Program, Beijing Normal University-Hong Kong Baptist University United International College, Zhuhai, China
The Golgi apparatus (GA) is a cellular organelle that participates in the packaging, modification, and transport of proteins and lipids from the endoplasmic reticulum to be further fabricated before being presented to other cellular components. Recent studies have demonstrated that GA facilitates numerous cellular processes in cancer development. Therefore, this study aimed to establish a novel lung adenocarcinoma (LUAD) risk evaluation model based on GA gene signatures. In this study, we used TCGA-LUAD (n = 500) as the training cohort and GSE50081 (n = 127), GSE68465 (442), and GSE72094 (398) as the validation cohorts. Two immunotherapy datasets (GSE135222 and GSE126044) were also obtained from a previous study. Based on machine algorithms and bioinformatics methods, a GA gene-related risk score (GARS) was established. We found that the GARS independently predicted the prognosis of LUAD patients and remained effective across stages IA to IIIA. Then, we identified that the GARS was highly correlated with mutations in P53 and TTN. Further, this study identified that GARS is related to multiple immune microenvironmental characteristics. Furthermore, we investigated GSE135222 and GSE126044 and found that a lower GARS may be indicative of an improved therapeutic effect of PD-1/PD-L1 therapy. We also found that high GARS may lead to a better response to multiple anticancer drugs. Finally, we established a nomogram to better guide clinical application. To our knowledge, this is the first study to demonstrate a novel GA signature-based risk score formula to predict clinical prognosis and guide the treatment of LUAD patients.
Introduction
Lung adenocarcinoma (LUAD) is the predominant subtype of non-small cell lung cancer (NSCLC) (Sung et al., 2021). With the application of novel targeted drugs and immune therapy, the mortality of LUAD has slightly declined; however, the prognosis of LUAD remains unsatisfactory (Siegel et al., 2018; Cui et al., 2021). Moreover, because of tumor heterogeneity, establishing a systematic and precise method to stratify the risk of LUAD is critical.
The Golgi apparatus (GA) is a cellular organelle that participates in the packaging, modification, and transport of proteins and lipids from the endoplasmic reticulum to be further fabricated before being presented to other cellular components (Liu et al., 2021a). In addition to its well-known roles, recent studies have demonstrated that the GA facilitates numerous cellular processes in cancer development, including innate immune reactions, angiogenesis, tumor migration, and invasion (Li et al., 2019; Tao et al., 2020). Hence, some researchers have achieved satisfactory results in the treatment of tumors using manipulated Golgi-targeted nano drug delivery systems (Zhang et al., 2022). Moreover, the membranous nets that link the GA with the endoplasmic reticulum, mitochondria, autophagosomes, and endosomes provide feasible access to cellular signal transmission and subsequent effector responses (Li et al., 2019; Tao et al., 2020). With the in-depth study of cellular organelles, scientists have gradually focused on the role of the GA in the development and progression of cancer. So far, there has been no relevant research on the prognostic significance of GA-related gene set-based formulas in cancer. Thus, investigating GA-related gene sets in LUAD may provide valuable therapeutic guidance in analyzing the most relevant function of the GA related to tumor prognosis.
In the present study, we aimed to construct a GA signature-based LUAD prognosis forecasting system using bioinformatics to guide further clinical treatment.
Materials and Methods
Data Acquisition
Gene expression data, gene mutation information, and clinical survival data of LUAD patients were acquired from The Cancer Genome Atlas (TCGA) database (TCGA-LUAD) (Schabath et al., 2016) and the Gene Expression Omnibus (GEO) database (GSE50081, GSE68465, GSE72094) (Zhang et al., 2021; Zhou et al., 2021). TCGA-LUAD was used as the training cohort, while GSE50081, GSE68465, and GSE72094 were used as the validation cohorts. The “sva” package of the R software was adopted to rectify the batch effect between different datasets using the “combat” algorithm. GA-related genes were extracted from the hallmark gene set in the Molecular Signatures Database v7.0, which includes 1,608 GA-related genes. This study was approved by the Ethics and Research Committees of Sun Yat-Sen Memorial Hospital, Sun Yat-Sen University.
Screening of Differentially Expressed Golgi Apparatus-Related Genes
Information on the expression of 1,608 GA-related genes was acquired from TCGA-LUAD database. Differentially expressed genes (DEGs) between normal lung tissue and LUAD were then identified through the Wilcoxon test according to Log2Fc <−1 or >1 and p < 0.05, with Log2Fc > 1 and Log2Fc < −1 indicating upregulated and downregulated genes, respectively. Heatmaps were generated to express differential genes.
Functional Exploration of Differentially Expressed Genes
R software package (clusterprofiler, version 3.12) was used to perform Gene Ontology (GO) and Kyoto Encyclopedia of Genes and Genomes (KEGG) pathway enrichment analysis. With the use of Fisher’s exact test, those with false discovery rate (FDR)-corrected p-values of less than 0.05 were regarded as marked indicators.
Construction and Verification of the Golgi Apparatus Gene-Related Risk Score Formula
First, RNA expression in TCGA-LUAD, GSE50081, GSE68465, and GSE72094 datasets was cross-checked to identify co-expressed and differentially expressed GA-related genes. Consequently, univariate Cox analysis of overall survival (OS) was applied to screen for GA-related genes with prognostic value. Subsequently, least absolute shrinkage and selection operator (LASSO) regression with 10-fold cross-validation was performed, 1,000 cycles of the “glmnet” package were run using the R software, and 1,000 random stimulations were set. Based on the best lambda value, the optimal possible gene was selected to construct the model, and a GA gene-related risk score (GARS) was constructed.
The GARS was calculated based on the expression of each gene and its corresponding regression coefficients based on the following equation: risk score = ∑ genes Cox coefficient × gene expression. The patients were then categorized into the high and low GARS groups according to the best cutoff value, using the R package of “surv_cutpoint” in “survminer.” The predictive sensitivity of the GARS was painted via the R Package “survivalROC” for estimation. The model effectiveness was evaluated in the validation set using the same coefficient and cutoff values that were used in the training set.
Given the lack of information on immune therapy in TCGA-LUAD cohort, the immune therapy predictive capability of the risk score formula was evaluated using the two lung cancer cohorts GSE135222 and GSE126044 (Cho et al., 2020; J. Y.; Kim et al., 2020).
Establishment of the Nomogram
This study used the Cox regression model along with the R package “rms” to build an OS prediction nomogram that set 1-, 3-, and 5-year OS as the endpoints. The C-index was used to estimate the discriminative ability of the nomogram. Calibration plots were used to visualize the consistency between the predicted and factual 1-, 3-, and 5-year OS.
Differential Analysis of Immune Cell Infiltration, Immune Function, Immune Checkpoint, and Validation of Immunotherapy Response
Immune cell infiltration was identified using timer 2.0 (cistrome.shinyapps.io/timer/) via the MCPCOUNTER, CIBERSORT, QUANTISEQ, Timer, CIBERSORT-ABS, EPIC, and XCELL algorithms. The “gsva” R package was applied to study the single-sample gene set enrichment analysis (ssGSEA) to count the activities of 13 immune pathways. Immune checkpoint-related genes were retrieved as previously described (Dib et al., 2017). The ESTIMATE algorithm was used to calculate the stromal and immune scores, as well as the ESTIMATE score of TCGA-LUAD samples.
Predicting Drug Therapeutic Response
To predict the immunotherapy sensitivity, the Immunophenoscore (IPS) was calculated using the Cancer Immunome Atlas (https://tcia.at/). The IC50 of common chemotherapeutic drugs was counted in the training group via the administered “pRRophetic” package to evaluate the predictive capability of the GARS for drug therapeutic responses. The Wilcoxon rank test was then used to compare the difference in IC50 between the low and high GARS groups. Finally, we applied the “ggplot” R package to paint the result in the histogram.
Statistical Analysis
DEGs were screened using the Wilcoxon test and compared using Fisher’s exact test. Univariate Cox analysis of OS was performed to identify relevant genes and their prognostic value. Kaplan–Meier survival curves were generated and compared between the two groups using the log-rank test. The association between the prognostic model risk score and immune score was assessed using Spearman’s correlation analysis. All statistical analyses were performed using R version 4.0.0 (R-project.org) and its adequate packages. Statistical significance was set at p ≤ 0.05.
Result
A total of 500 LUAD patients from the training sets and 967 LUAD patients from the validation sets were selected. The detailed clinical features of these patients are summarized in Table 1. A flowchart of the study is presented in Figure 1.
Differentially Expressed and Golgi Apparatus-Related Genes
In the training set, by applying the Wilcoxon test according to Log2Fc < −1 or >1 and p < 0.05, we identified 353/1,608 GA-related DEGs between LUAD and adjacent normal tissues. With Log2Fc > 1 and Log2Fc < −1 indicating upregulated and downregulated genes, respectively, we found 212 upregulated and 141 downregulated GA-related genes (Figure 2A).
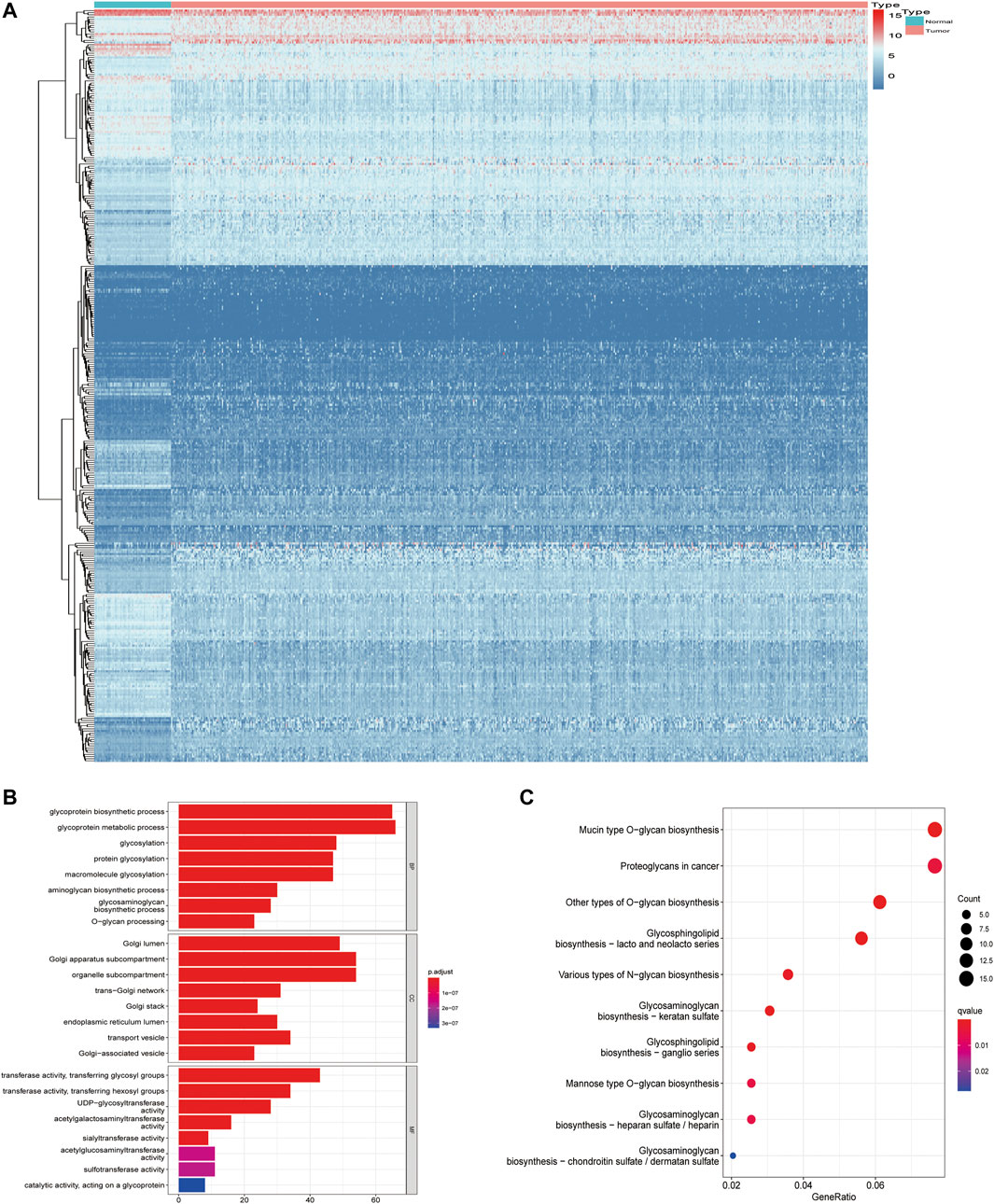
FIGURE 2. Screening of differentially expressed Golgi apparatus-related genes. (A) Heatmaps of differentially expressed Golgi apparatus-related mRNAs. (B) GO enrichment of Golgi apparatus-related DEGs. (C) KEGG pathways of Golgi apparatus-related DEGs. GO, Gene Ontology; DEGs, differentially expressed genes; KEGG, Kyoto Encyclopedia of Genes and Genomes.
Functional Analysis of Golgi Apparatus-Related Differentially Expressed Genes
Through GO enrichment analysis, we identified the most obvious GO category with significant enrichment of GA-related genes. The most significantly altered GA-related genes were involved in glycoprotein metabolic processes (Figure 2B; Supplementary Table S1A). Subsequently, we performed KEGG analysis and identified the top KEGG category with significant enrichment of GA-related genes. The altered GA-related genes were mostly associated with mucin-type O-glycan biosynthesis (Figure 2C; Supplementary Table S1B).
Predictive Capability of the Golgi Apparatus Gene-Related Risk Score
A total of 11,047 genes were co-expressed, among which 242 of 353 GA-related DEGs were selected. These 242 genes were then used in univariate Cox regression analysis. A total of 86 prognostic genes were identified. To avoid overfitting the prognostic model, LASSO regression analysis was performed (Figures 3A,B). Finally, 14 genes were selected and included in the risk score formula: GARS = RGS20 × 0.181 − B4GALT4 × 0.033 + PDGFB × 0.179 + NTSR1 × 0.117 + ATP8B3 × 0.044 + AHSG × 0.082 − CTLA4 × 0.142 + KIF20A × 0.159 + BCAN × 0.133 − 0.321 × CAV3 − CBFA2T3 × 0.109 + OPRM1 × 0.328 + FURIN × 0.102 + F2RL1 × 0.120. Based on the optimistic cutoff, 151 and 349 patients were categorized into the high GARS and low GARS groups, respectively (Figures 3C–E). Kaplan–Meier survival analysis showed a lower OS in the high GARS group than in the low GARS group (hazard ratio (HR) = 3.07; 95% CI 2.29–4.12; p < 0.0001). The area under the curve (AUC) for predicting 1-, 3-, and 5-year OS was 0.720, 0.727, and 0.726, respectively (Figures 3F,G). These results showed that the risk model based on the 14 genes had high accuracy in predicting the OS of patients with LUAD.
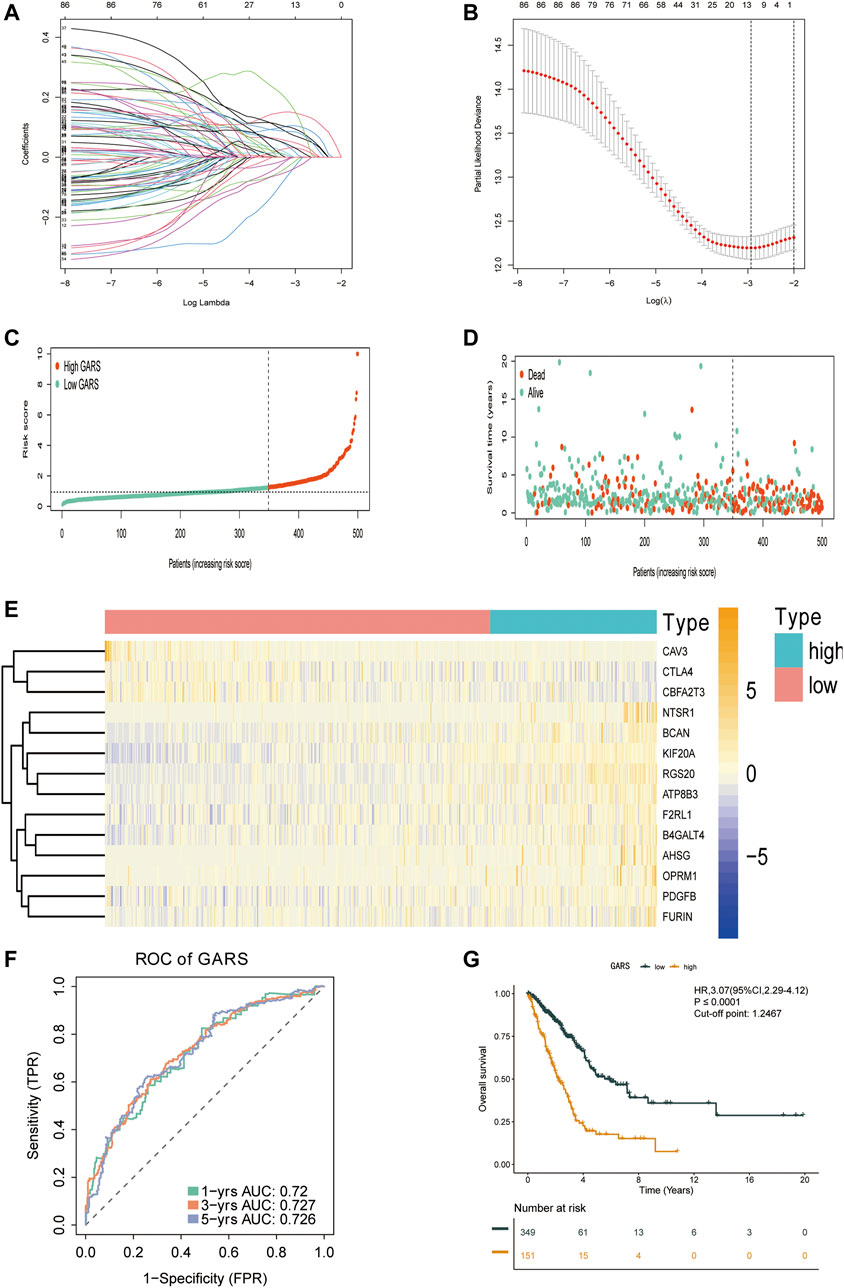
FIGURE 3. Construction of the GARS formula. (A) LASSO coefficient profile plots of the 86 prognostic-related genes showing that the variations in the size of the coefficients of parameters shrink with an increasing value of the k penalty. (B) Penalty plot for the LASSO model for the 86 prognostic genes with error bars denoting the standard errors. (C) Distribution of GARS for the training set. (D) Patterns of the survival time and survival status between the high GARS and low GARS groups for the training set. (E) Heatmaps of the 14 prognostic genes for each patient in the training set. (F) Time-related ROC analysis exhibited the prognostic value of the GARS in the training set. (G) Kaplan–Meier survival curve of the patients in the high GARS and low GARS groups for OS in the training set. GARS, Golgi apparatus gene-related risk score; LASSO, least absolute shrinkage and selection operator; ROC, receiver operating characteristic; OS, overall survival.
Stability of the Golgi Apparatus Gene-Related Risk Score Formula
To check the stability of the GARS developed from the training set, patients in the validation sets (GSE50081, GSE68465, and GSE72094) were also divided into the high and low GARS groups according to the same cutoff value and risk formula as those in TCGA cohort. The results showed a markedly lower OS in the high GARS group than in the low GARS group (Figures 4B,D,F). In the GSE50081, the AUCs for predicting the 1-, 3-, and 5-year OS were 0.736, 0.714, and 0.733, respectively (HR = 3.27; 95% CI = 1.84–5.79; p < 0.0001) (Figure 4A). In the GSE68465 set, the AUCs for predicting the 1-, 3-, and 5-year OS were 0.669, 0.663, and 0.62, respectively (HR = 1.60; 95% CI = 1.23–2.10; p < 0.001) (Figure 4C). In the GSE72094 cohort, the corresponding AUCs were 0.728, 0.655, and 0.637, respectively (HR = 2.74; 95% CI = 1.89–3.97; p < 0.0001) (Figure 4E).
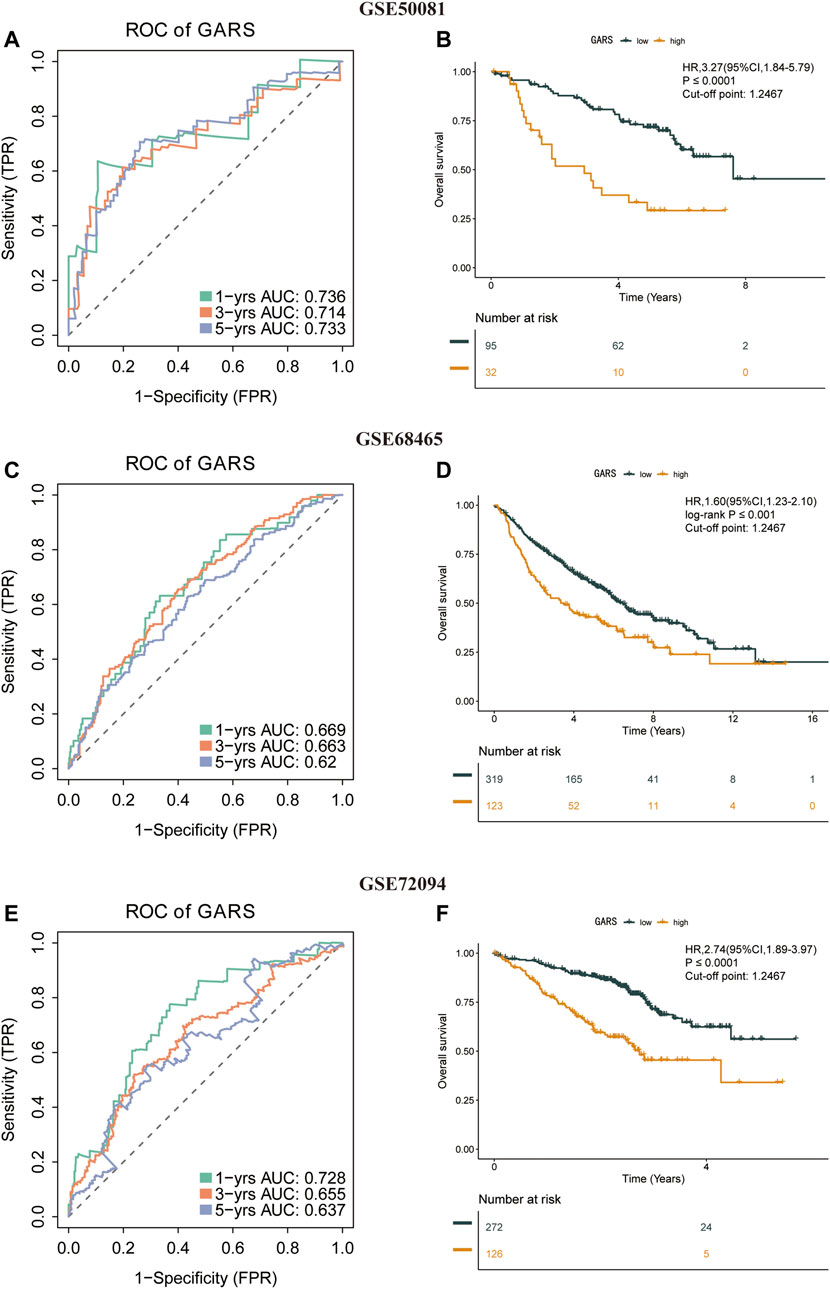
FIGURE 4. Stability of the GARS formula in the validation sets. (A) Time-related ROC analysis proved the prognostic value of the GARS in the GSE50081. (B) Kaplan–Meier survival curve of the patients in the high GARS and low GARS groups for OS in the GSE50081. (C) Time-related ROC analysis proved the prognostic value of the GARS in the GSE68465. (D) Kaplan–Meier survival curve of the patients in the high GARS and low GARS groups for OS in the GSE68465. (E) Time-related ROC analysis proved the prognostic value of the risk score in the GSE72094. (F) Kaplan–Meier survival curve of the patients in the high GARS and low GARS groups for OS in the GSE72094. GARS, Golgi apparatus gene-related risk score; ROC, receiver operating characteristic; OS, overall survival.
Subgroup Analysis of Different Stages With the Golgi Apparatus Gene-Related Risk Score Formula
At present, the treatment of LUAD is usually based on the clinical stage (Bang et al., 2020). Particularly, patients with IA are not recommended for any further anticancer therapy after the removal of the tumor (Chou et al., 2021). However, the prognosis of patients at the same stage was divergent. Thus, we analyzed the association between the different stages and GARS in TCGA-LUAD database. The GARS accurately identified stage IA LUAD patients with different prognoses (HR = 2.93; 95% CI 1.28–6.71; p < 0.01) (Figure 5A). Further, the GARS remained effective across stages IB to IIIA (Figures 5B–E). However, it did not distinguish between the prognosis of patients with stage IIIB and stage IV LUAD (Figures 5F,G). This may be due to the low population of these groups. Besides, the result above is also illustrated in a forest plot (Figure 5H).
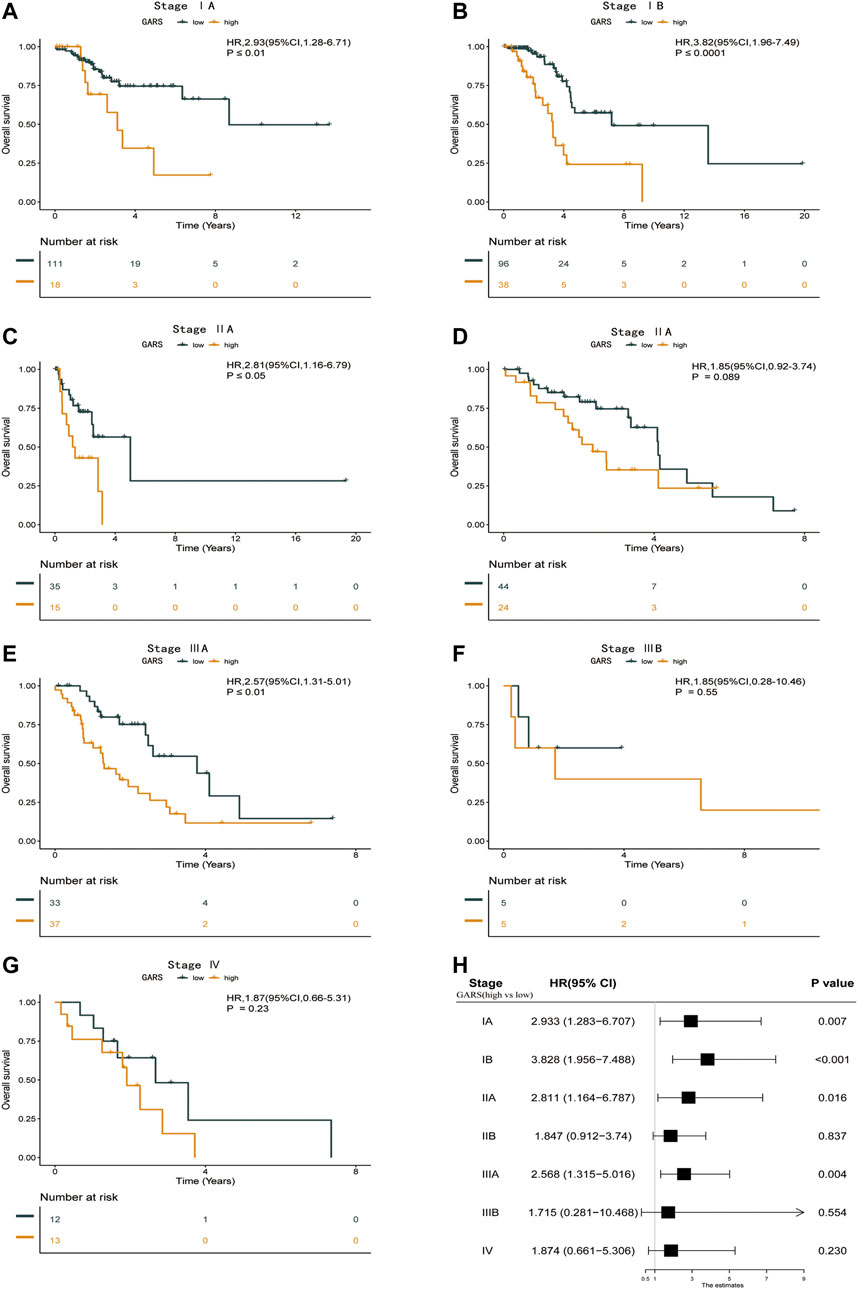
FIGURE 5. Subgroup analysis of different stages with the GARS formula. (A) Kaplan–Meier survival curve of the patients in the high GARS and low GARS groups for OS in stage IA LUAD patients. (B) Kaplan–Meier survival curve of the patients in the high GARS and low GARS groups for OS in stage IB LUAD patients. (C) Kaplan–Meier survival curve of the patients in the high GARS and low GARS groups for OS in stage IIA LUAD patients. (D) Kaplan–Meier survival curve of the patients in the high GARS and low GARS groups for OS in stage II LUAD patients. (E) Kaplan–Meier survival curve of the patients in the high GARS and low GARS groups for OS in stage IIIA LUAD patients. (F) Kaplan–Meier survival curve of the patients in the high GARS and low GARS groups for OS in stage IIIB LUAD patients. (G) Kaplan–Meier survival curve of the patients in the high GARS and low GARS groups for OS in stage Ⅳ LUAD patients. (H) Forest plot illustrating the survival of the patients in the high GARS and low GARS groups in different stages. GARS, Golgi apparatus gene-related risk score; OS, overall survival; LUAD, lung adenocarcinoma.
Functional Analysis Between the High Golgi Apparatus Gene-Related Risk Score Group and the Low Golgi Apparatus Gene-Related Risk Score Group
Further analysis of the differences in GO enrichment pathways between the low and high GARS groups showed that the most different pathways were the humoral immune response, external side of the plasma membrane, and antigen binding (Figure 6A; Supplementary Table S2A). We also analyzed the differences in KEGG enrichment pathways between the low and high GARS groups, indicating that complement and coagulation cascades are the most significant pathways (Figure 6B; Supplementary Table S2B). This may be a potential reason for the lower OS in the high GARS group.
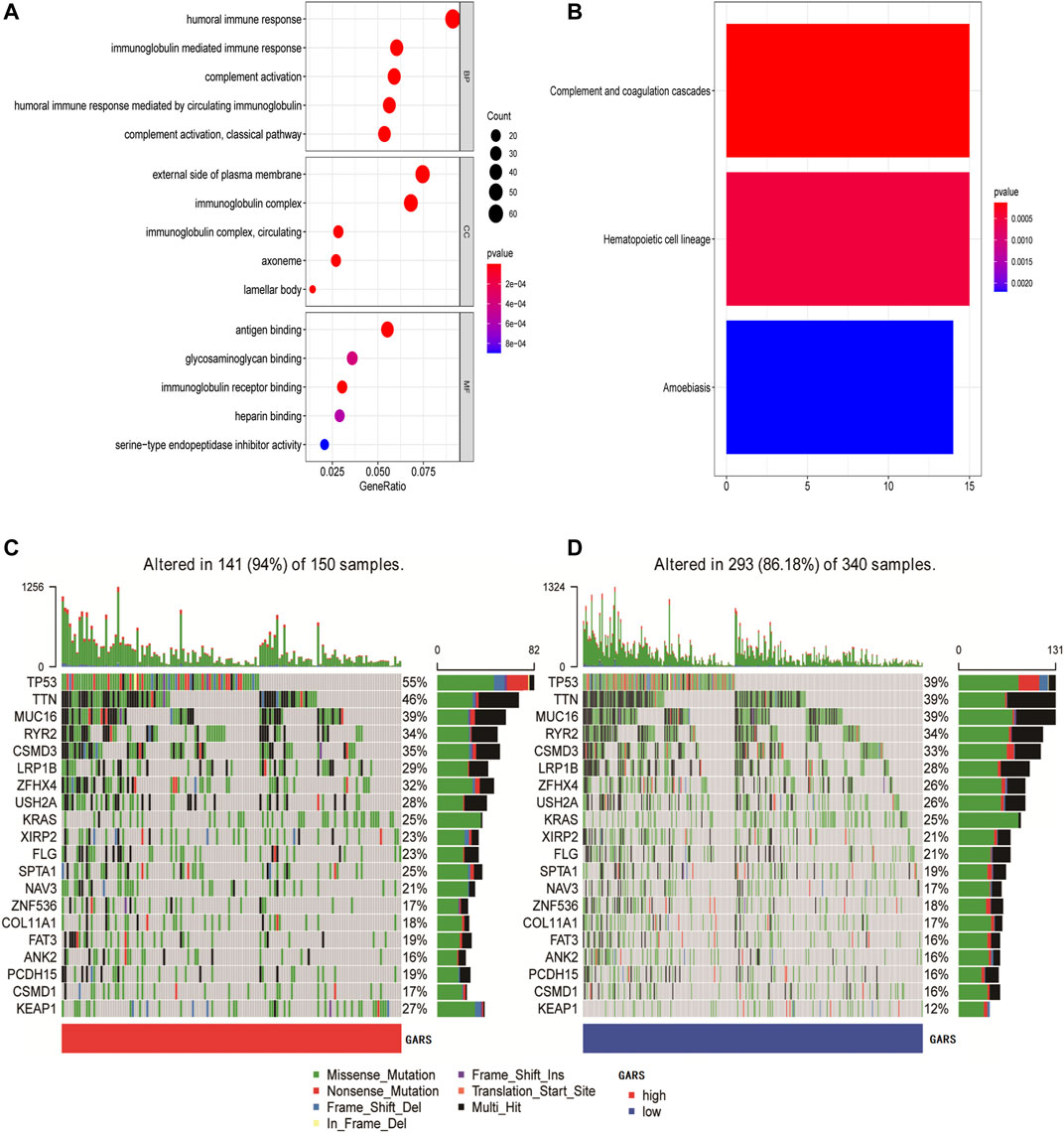
FIGURE 6. Functional analysis and identification of mutation landscape. (A) GO enrichment between the high GARS patients and low GARS patients in TCGA-LUAD cohort. (B) KEGG pathways between the high GARS patients and low GARS patients in TCGA-LUAD cohort. (C, D) Comparison of the mutation landscape between groups with high and low GARS. GO, Gene Ontology; GARS, Golgi apparatus gene-related risk score; KEGG, Kyoto Encyclopedia of Genes and Genomes.
Identification of the Golgi Apparatus Gene-Related Risk Score-Associated Mutation Landscape
The mutation landscapes in the high and low GARS groups were compared. The results showed that more mutation events occurred in samples with higher GA scores, with TP53 and TTN mutations being the predominant alterations in these samples (Figures 6C,D).
Immune Status Analysis
The relationship between the GARS and immune status of the patients in TCGA cohort was investigated. There were significant alterations in immune cells (Figure 7A). The immune-related pathway also significantly differed, including CCR, HLA, T CELL co-inhibition, T-cell co-stimulation, and type II IFN response. Subsequently, we compared the expression of immune checkpoint-related genes between the high GARS and low GARS groups. In this study, we found that ICOS, ADORA2A, CD27 IDO2, LGALS9, CD80, BTNL2, BD86, CD160, TNFRSF25, CTLA4, TNFRSF14, CD28, CD48, BTLA, TNFRSF4, CD200R1, CD40LG, TIGHT, TNFSF15, TNFSF18, KIR3DL1, TNFSF14, and CD244 were significantly lower in the high GARS group (Figure 7C). The tumor immune microenvironment was also assessed using the immune score, ESTIMATE score, and stromal score, which also illustrated the negative correlation between GARS and immune reaction (Figure 7D). These results indicated stronger tumor immune activities in the low GARS group than in the high GARS group.
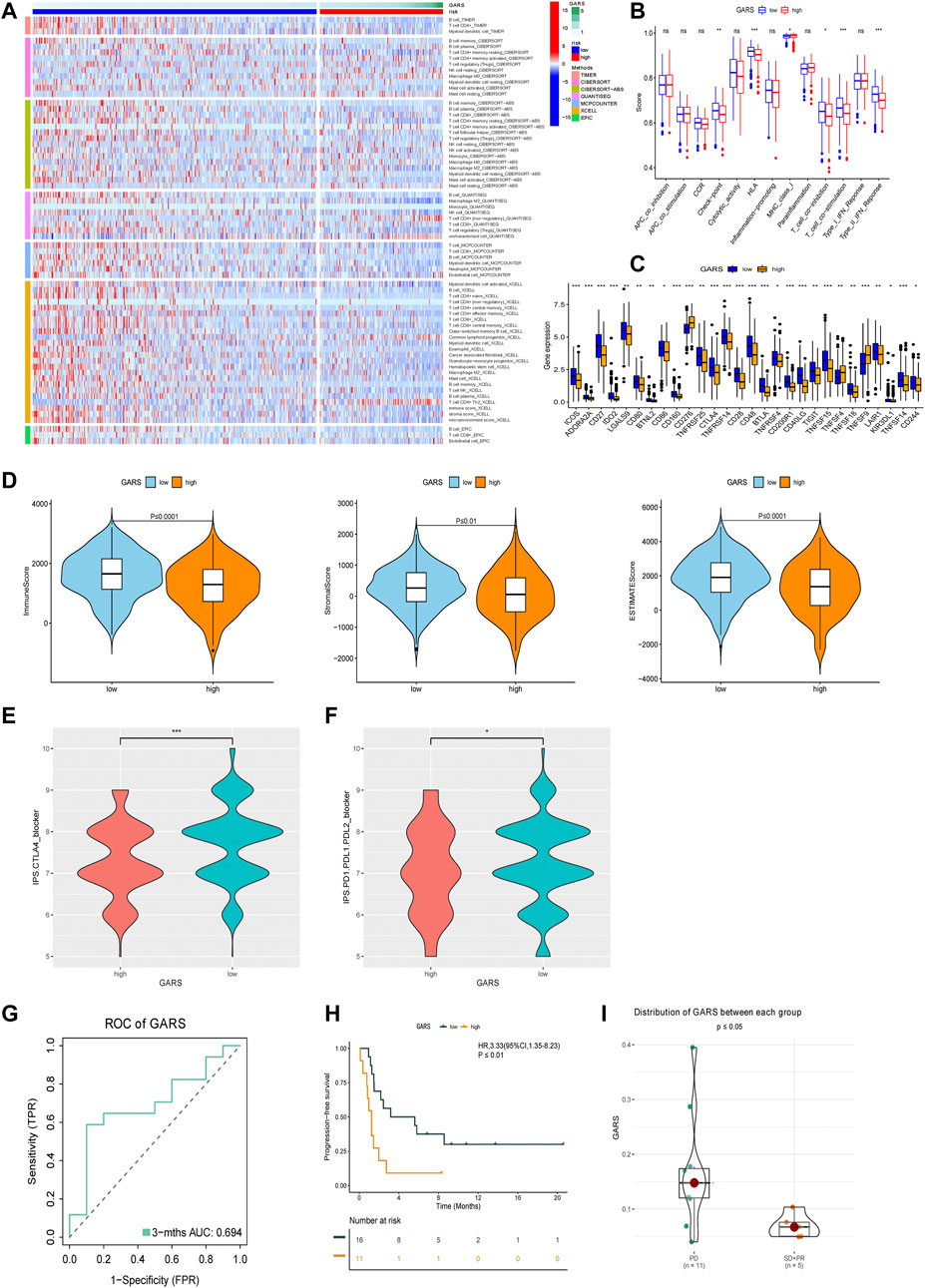
FIGURE 7. Analysis of GARS-related immune status and validation of immunotherapy response. (A) Heatmap for immune responses based on MCPCOUNTER, CIBERSORT, QUANTISEQ, Timer, CIBERSORT-ABS, EPIC, and XCELL algorithms among high GARS group and low GARS group. (B) Immune pathway analysis. (C) The expression of immune-related checkpoints among high and low GARS groups. (D) The relationship between GARS, Immune score, Stromal score, and ESTIMATE score. (E, F) IPS score between high GARS group and low GARS group. (G) ROC analysis proved the prognostic value of the GARS in the PD-1/PD-L1 treatment cohort GSE135222. (H) Kaplan–Meier survival curve of the patients in the high GARS and low GARS groups for OS in the PD-1/PD-L1 treatment cohort GSE135222. (I) Analysis of the immunotherapy response between high and low GARS group in the PD-1/PD-L1 treatment cohort GSE126044. GARS, Golgi apparatus gene-related risk score; IPS, Immunophenoscore; ROC, receiver operating characteristic; OS, overall survival.
Immune Treatment Analysis
Because of the high immune connection between GARS and immune status, we further estimated the prognostic predictive value of GARS with immune therapy. Surprisingly, GARS not only correlated with the IPS score of PD-1 and CTLA4 but also correlated with the prognosis of lung cancer patients who received PD-1/PD-L1 therapy (Figures 7E–I).
Comparison of the Sensitivity to Anti-Lung Cancer Drugs Between Patients With Different Golgi Apparatus Gene-Related Risk Score
Different molecular subtypes of LUAD should guide clinical decisions. Therefore, the sensitivity to nine common anti-LUAD drugs was compared between the high and low GARS groups to determine potential treatment modalities. The results demonstrated that the IC50s of cisplatin, docetaxel, erlotinib, etoposide, gemcitabine, paclitaxel, rapamycin, sorafenib, and vinorelbine were significantly higher in patients with higher GARS (Figures 8A–I). These drugs include almost all the common drugs used to treat LUAD patients according to the National Comprehensive Cancer Network (NCCN; https://www.nccn.org) guidelines for NSCLC. This fully shows the value of the GARS.
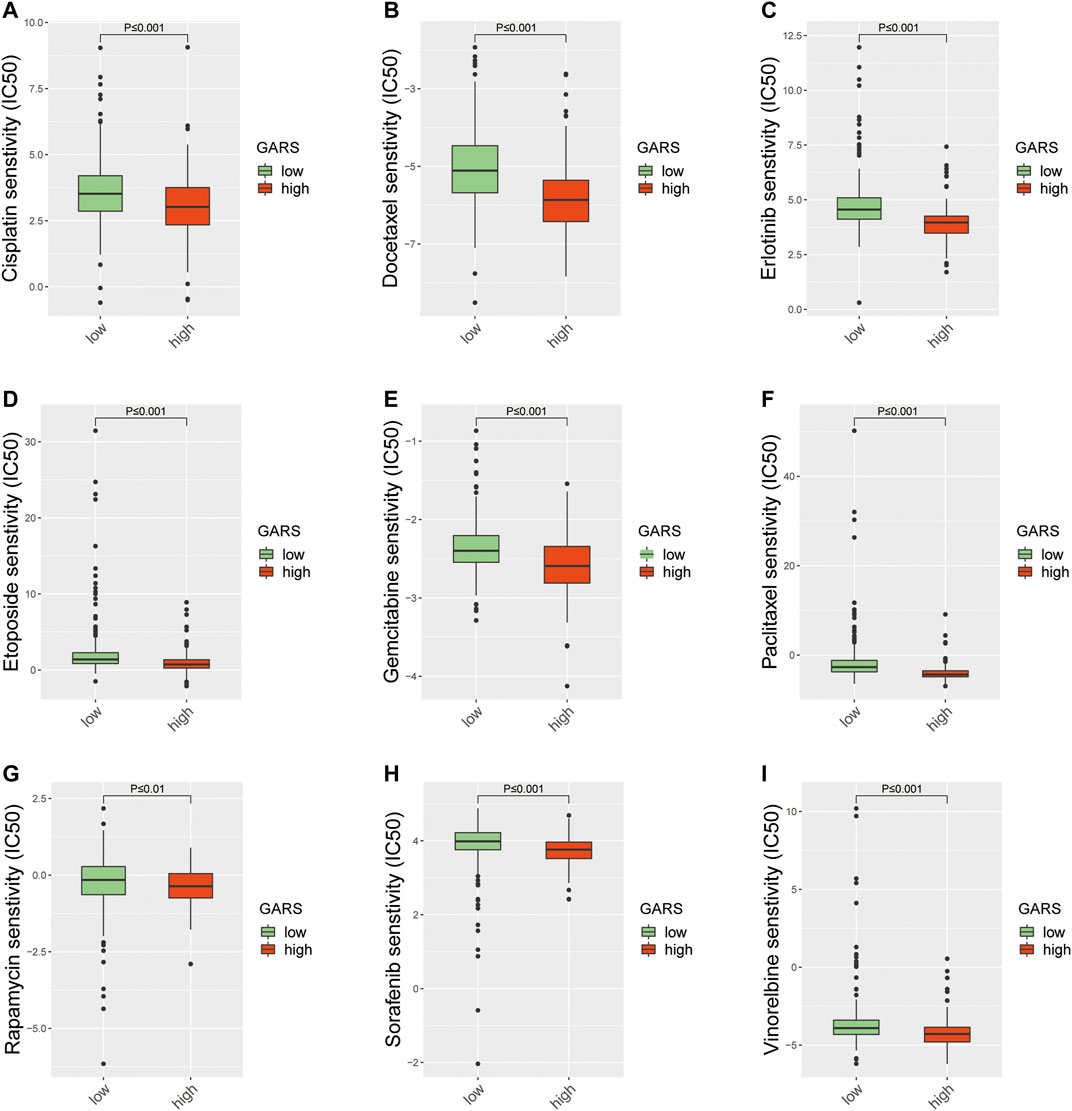
FIGURE 8. The difference of anticancer drug sensitivity between the high GARS group and low GARS group. (A) The IC50 of cisplatin among groups. (B) The IC50 of docetaxel among groups. (C) The IC50 of erlotinib among groups. (D) The IC50 of etoposide among groups. (E) The IC50 of gemcitabine among groups. (F) The IC50 of paclitaxel among groups. (G) The IC50 of rapamycin among groups. (H) The IC50 of sorafenib among groups. (I) The IC50 of vinorelbine among groups. GARS, Golgi apparatus gene-related risk score.
Golgi Apparatus Gene-Related Risk Score Independently Predict the Overall Survival of Lung Adenocarcinoma
In univariate analysis according to OS, GARS and stage were identified as significant prognostic factors in predicting OS (Supplementary Figure S1A). After other potential confounding factors were controlled, GARS and stage were found to be independent prognostic factors (Supplementary Figure S1B). These results also indicate that GARS may help to further stratify the prognosis of patients at different stages.
The Construction and Validation of the Golgi Apparatus Gene-Related Risk Score-Related Nomogram
A nomogram was established based on the noted parameters, including age, stage, sex, and GARS. As shown in the nomogram, each risk factor was associated with a specific score, and the calculated scores could accurately predict the prognosis of 1-, 3-, and 5-year survival rates (Figures 9A–D). This also provides a suitable clinical application scenario for GARS.
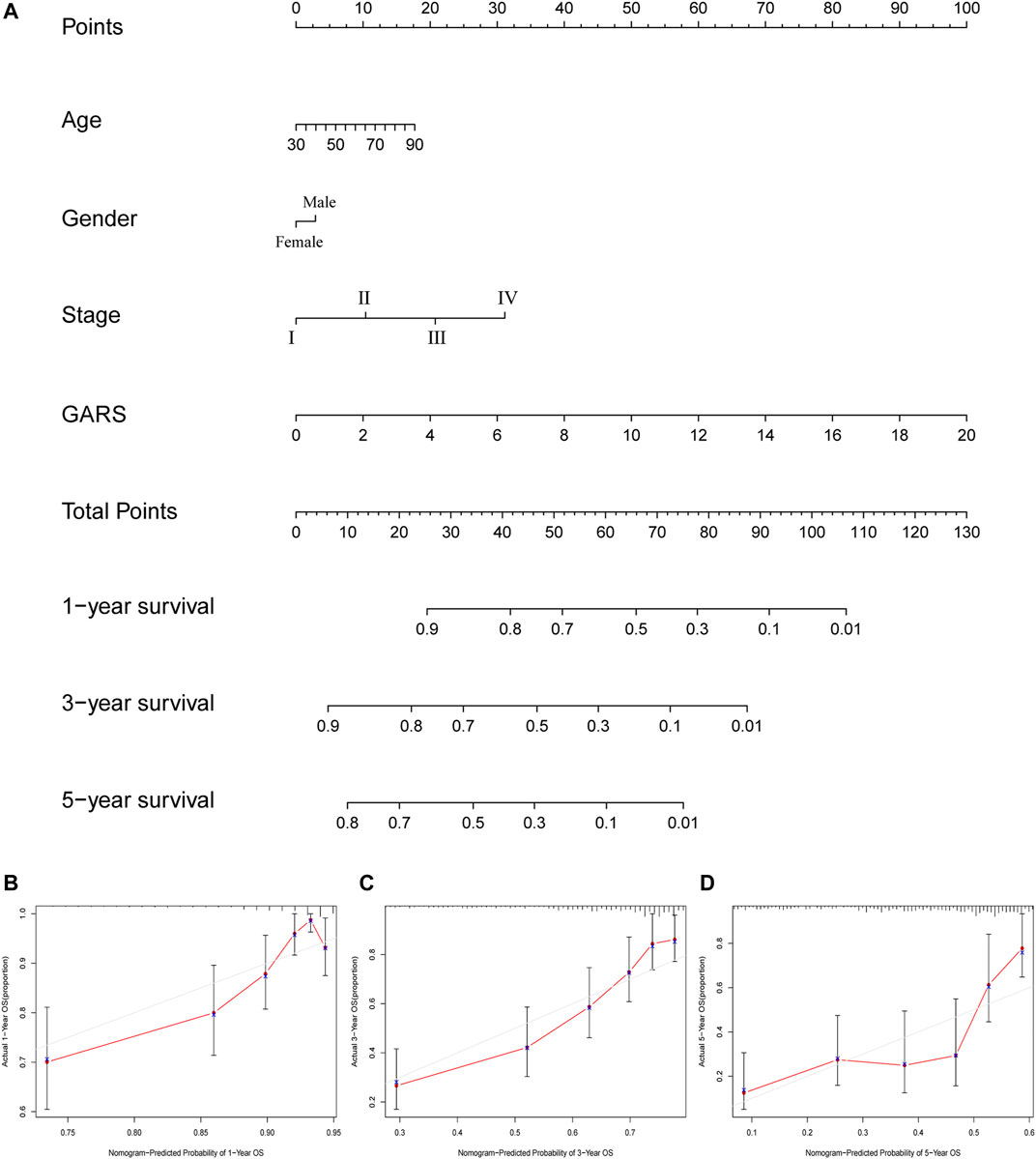
FIGURE 9. The calculation of GARS-related prognostic nomogram. (A) The prognostic nomogram to predict the 1-, 3-, and 5-year OS of LUAD patients. For each patient, we calculated the points of the clinical–pathological features and summed up the points to obtain the total points. The predicted 1-, 3-, and 5-year OS can be estimated based on the total points of each patient. (B) The calibration curves for predicting patient survival at 1-year OS. (C) The calibration curves for predicting patient survival at 3-year OS. (D) The calibration curves for predicting patient survival at 5-year OS. GARS, Golgi apparatus gene-related risk score; OS, overall survival; LUAD, lung adenocarcinoma.
Discussion
LUAD is a major type of lung cancer with a high incidence rate (Li Z. et al., 2021; Wang et al., 2021). The prognosis of LUAD remains devastating because of its obscure pathogenesis (Peng et al., 2021). Therefore, individual therapies for patients with LUAD are needed. In the past decade, the study of genomic and transcriptional factors has rapidly pushed the individualized treatment of tumors. However, most of these studies were based on cellular function, cellular structure-based investigations, which are rare. This study, to our best knowledge, is the first to use the Golgi apparatus gene set to carry out this kind of research. In this study, a novel formula, GARS, which independently forecasts the prognosis of LUAD patients, was developed and validated. This may provide a precise method to evaluate prognosis and guide treatment in LUAD patients.
The Golgi complex is a membrane-bound organelle that lies in a key position of multiple cellular pathways (Lujan and Campelo, 2021). The main functions of the Golgi apparatus are to maintain cellular protein and lipid homeostasis and to mediate protein sorting and export (Lujan and Campelo, 2021). Accumulating evidence has shown that a proper Golgi structure is essential for its suitable functions, especially glycosylation (X. Zhang, 2021). Notably, altered glycosylation of cell surface proteins and lipids is a well-accepted cancer hallmark (Martins et al., 2021; Zhang, 2021).
Because the promising potential of GA genes and cellular structure is the foundation of cellular function, this study first used the GA signature, which was extracted from the Molecular Signatures Database (MSigDB), to predict the prognosis of LUAD patients. In summary, 14 genes were included in the GARS formula for LUAD prognosis. By manipulating this formula, we classified LUAD patients into the high and low GARS groups. The formula had AUCs of 0.720, 0.727, and 0.726 for predicting 1-, 3-, and 5-year OS, respectively, indicating its high reliability and accuracy. Furthermore, the high GARS group had a significantly lower OS than the low GARS group. The risk score also showed high predictive capability in the GSE72094, GSE68465, and GSE50081 validation sets. Subsequently, to better guide clinical prognosis prediction, we applied GARS at different clinical stages. Surprisingly, we found that the risk score remained significantly effective across stages IA to IIIA. However, the risk score did not distinguish between the risk in stage IIIB and stage IV. It should be noted that stage IA lung cancer is usually treated only by surgery (Chang et al., 2021), indicating that patients with high GARS IA LUAD need further treatment.
Furthermore, among the 14 genes, TP53 and TTN were found to be mutating more in the high GARS group. Recently, mutations in these two genes were found to be correlated with the response to immunotherapy (Z. Liu et al., 2021b; Muñoz-Fontela et al., 2016). Mutation or loss of p53 in cancers can influence the activity and recruitment of T cells, which can induce immune evasion (Blagih et al., 2020). The gain-of-function p53 mutation type can induce the accumulation of neutrophils, which leads to resistance to immunotherapy (Siolas et al., 2021). Hence, we explored the correlation of GARS with the immune microenvironment and immunotherapy response.
Immunotherapy is a novel treatment for LUAD. Identifying a novel method that can classify patients who might benefit from immunotherapy remains a tremendous challenge (Camidge et al., 2019; Yu et al., 2019; Yu et al., 2020; Wu et al., 2021). Recent studies have demonstrated numerous differences between the GA and the immune microenvironment; the GA can participate in the activation of the NLRP3 inflammasome, sensing nucleic acids, and influencing interferon (IFN)-inducible GTPases (Vey et al., 1994; Gonugunta et al., 2017; Chen and Chen, 2018), highlighting its potential in predicting immune-related anticancer therapy. In this study, we found that the immune response was significantly higher in the low GARS group, including lower immune cell infiltration (such as CD4+ T cells, CD8+ T cells, NK cells, monocytes, M0 macrophages, M2 macrophages, and B cells), immune pathway response, gene expression of immune checkpoints, immune score, stromal score, and ESTIMATE score. These results illustrate that lower immune activities were revealed in the high GARS group. At present, researchers investigating LUAD patients who might respond to immunotherapy mainly relied on IPS (Guo et al., 2021); however, few studies have been validated using a direct lung cancer cohort. To further explore whether GARS can predict the efficacy of immunotherapy, this study not only administered IPS score to forecast but also used GSE126044 and GSE13522 to direct confirmation. These results strongly verified the potential of GARS in predicting the efficacy of LUAD immunotherapy. In addition, the most significant GO and KEGG pathways between high and low GARS are the humoral immune response, external side plasma membrane, antigen binding, and complement and coagulation cascades. This indicates that the most important LUAD prognosis-related function of GA is its intimate connection with immune response. Taken together, these results suggest that patients in the low GARS group may respond better to immunotherapy.
The GARS constructed in this study may also instruct clinicians to select other anticancer drugs for the treatment of LUAD. In this study, a high GARS was significantly correlated with high drug sensitivity, including cisplatin, docetaxel, erlotinib, etoposide, gemcitabine, paclitaxel, rapamycin, sorafenib, and vinorelbine. Among them, cisplatin, docetaxel, etoposide, gemcitabine, paclitaxel, and vinorelbine are traditional chemotherapeutic drugs that have been proven to be beneficial in LUAD (I. Kim et al., 2019; Z. Li Y. et al., 2021; Perdrizet et al., 2021; Provencio et al., 2021; Yamaguchi et al., 2021). Erlotinib, the first approved target drug for NSCLC, is a reversible tyrosine kinase inhibitor that targets the epidermal growth factor receptor and can significantly improve the survival of LUAD patients (Faehling et al., 2018). In addition, rapamycin (also called sirolimus) and sorafenib also have the potential for treating LUAD patients (Moran et al., 2017; Lim et al., 2016). Hence, the GARS we constructed may also instruct clinicians to select appropriate drugs for the treatment of LUAD.
In addition, to better guide clinical application, a nomogram was established to predict the prognosis of LUAD patients. This table also indicates the importance of GARS in predicting the survival of LUAD patients.
This study had some limitations. Because the proportion of late-stage patients is lower, the prediction ability of this GARS formula for late-stage LUAD is ambiguous. Although the study can stratify patients with stage IA LUAD, stage IA patients usually only received surgical therapy. Therefore, GARS may not be able to precisely predict further treatment in stage IA LUAD patients based on this risk stratification.
Conclusion
In conclusion, the present study is the first to administer the GA gene signature to construct a GARS that exhibited high diagnostic accuracy in predicting prognosis in patients with LUAD. Functionally, the GARS was related to the TP53 mutation, TTN mutation, activation of immune microenvironment, the effect of immunotherapy, and multiple anticancer drugs in LUAD patients. We also constructed an accessible nomogram for clinical applications. In addition, this study may also provide direction for scientific research on GA and tumor development.
Data Availability Statement
Publicly available datasets were analyzed in this study. This data can be found here: TCGA-LUAD from TCGA database (https://portal.gdc.cancer.gov/); GSE68465, GSE72094, GSE50081, GSE135222 and GSE126044 cohort from GEO database (https://www.ncbi.nlm.nih.gov/geo/).
Ethics Statement
The studies involving human participants were reviewed and approved by the ethics committee of Sun Yat-sen memorial hospital. The patients/participants provided their written informed consent to participate in this study.
Author Contributions
Conceptualization: YJ, YY, and HY. Formal analysis: WO and YJ. Funding acquisition: HY. Investigation: WO and YJ. Project administration: WO and YJ. Supervision: YY and HY. Validation: YY and HY. Original draft writing: YJ. Writing—review and editing: YJ, WO, CZ, and YY. All authors have read and agreed to the published version of the manuscript.
Funding
This study was supported by grants 2017A030313822 and 2017A030313828 from the Natural Science Foundation of Guangdong Province, grant 2020ZX09201021 from the National Science and Technology Major Project, grant YXRGZN201902 from the Medical Artificial Intelligence Project of Sun Yat-Sen Memorial Hospital; Grant No.2021M703771 from China Postdoctoral Science Foundation Funded Project; Grants 81572596, 81972471 and 82073408 from the National Natural Science Foundation of China, grant 201704020131 from the Guangzhou Science and Technology Major Program, grant 2017B030314026 from the Guangdong Science and Technology Department, grant 2018007 from the Sun Yat-Sen University Clinical Research 5010 Program, grant SYS-C-201801 from the Sun Yat-Sen Clinical Research Cultivating Program, grant A2020558 from the Guangdong Medical Science and Technology Program, grant SYSU-81000-20200311-0001 and SYSU-05160-20200506-0001 from Tencent Charity Foundation.
Conflict of Interest
The authors declare that the research was conducted in the absence of any commercial or financial relationships that could be construed as a potential conflict of interest.
Publisher’s Note
All claims expressed in this article are solely those of the authors and do not necessarily represent those of their affiliated organizations, or those of the publisher, the editors, and the reviewers. Any product that may be evaluated in this article, or claim that may be made by its manufacturer, is not guaranteed or endorsed by the publisher.
Supplementary Material
The Supplementary Material for this article can be found online at: https://www.frontiersin.org/articles/10.3389/fcell.2021.817085/full#supplementary-material
References
Bang, Y.-J., Golan, T., Dahan, L., Fu, S., Moreno, V., Park, K., et al. (2020). Ramucirumab and Durvalumab for Previously Treated, Advanced Non-small-cell Lung Cancer, Gastric/gastro-Oesophageal junction Adenocarcinoma, or Hepatocellular Carcinoma: An Open-Label, Phase Ia/b Study (JVDJ). Eur. J. Cancer 137, 272–284. doi:10.1016/j.ejca.2020.06.007
Blagih, J., Buck, M. D., and Vousden, K. H. (2020). p53, Cancer and the Immune Response. J. Cel. Sci. 133 (5), jcs237453. doi:10.1242/jcs.237453
Camidge, D. R., Doebele, R. C., and Kerr, K. M. (2019). Comparing and Contrasting Predictive Biomarkers for Immunotherapy and Targeted Therapy of NSCLC. Nat. Rev. Clin. Oncolclinical Oncology 16 (6), 341–355. doi:10.1038/s41571-019-0173-9
Chang, J., Mehran, R., Feng, L., Verma, V., Liao, Z., Welsh, J., et al. (2021). Stereotactic Ablative Radiotherapy for Operable Stage I Non-small-cell Lung Cancer (Revised STARS): Long-Term Results of a Single-Arm, Prospective Trial with Prespecified Comparison to Surgery. Lancet Oncol. 22, 1448–1457. doi:10.1016/s1470-2045(21)00401-0
Chen, J., and Chen, Z. J. (2018). PtdIns4P on Dispersed Trans-golgi Network Mediates NLRP3 Inflammasome Activation. Nature 564 (7734), 71–76. doi:10.1038/s41586-018-0761-3
Cho, J.-W., Hong, M. H., Ha, S.-J., Kim, Y.-J., Cho, B. C., Lee, I., et al. (2020). Genome-wide Identification of Differentially Methylated Promoters and Enhancers Associated with Response to Anti-PD-1 Therapy in Non-small Cell Lung Cancer. Exp. Mol. Med. 52 (9), 1550–1563. doi:10.1038/s12276-020-00493-8
Chou, H.-P., Lin, K.-H., Huang, H.-K., Lin, L.-F., Chen, Y.-Y., Wu, T.-H., et al. (2021). Prognostic Value of Positron Emission Tomography in Resected Stage IA Non-small Cell Lung Cancer. Eur. Radiol. 31, 8021–8029. doi:10.1007/s00330-021-07801-4
Cui, C., Liu, Y., Gerloff, D., Rohde, C., Pauli, C., Köhn, M., et al. (2021). NOP10 Predicts Lung Cancer Prognosis and its Associated Small Nucleolar RNAs Drive Proliferation and Migration. Oncogene 40 (5), 909–921. doi:10.1038/s41388-020-01570-y
Dib, L., San-Jose, L., Ducrest, A.-L., Salamin, N., and Roulin, A. (2017). Selection on the Major Color Gene Melanocortin-1-Receptor Shaped the Evolution of the Melanocortin System Genes. Int. J. Mol. Sci. 18 (12), 2618. doi:10.3390/ijms18122618
Faehling, M., Achenbach, J., Staib, P., Steffen, U., Tessen, H. W., Gaillard, V. E., et al. (2018). Erlotinib in Routine Clinical Practice for First-Line Maintenance Therapy in Patients with Advanced Non-small Cell Lung Cancer (NSCLC). J. Cancer Res. Clin. Oncol. 144 (7), 1375–1383. doi:10.1007/s00432-018-2649-x
Gonugunta, V. K., Sakai, T., Pokatayev, V., Yang, K., Wu, J., Dobbs, N., et al. (2017). Trafficking-Mediated STING Degradation Requires Sorting to Acidified Endolysosomes and Can Be Targeted to Enhance Anti-tumor Response. Cel Rep. 21 (11), 3234–3242. doi:10.1016/j.celrep.2017.11.061
Guo, J.-N., Chen, D., Deng, S.-H., Huang, J.-R., Song, J.-X., Li, X.-Y., et al. (2021). Identification and Quantification of Immune Infiltration Landscape on Therapy and Prognosis in Left- and Right-Sided colon Cancer. Cancer Immunol. Immunother.. doi:10.1007/s00262-021-03076-2
Kim, I.-K., McCutcheon, J. N., Rao, G., Liu, S. V., Pommier, Y., Skrzypski, M., et al. (2019). Acquired SETD2 Mutation and Impaired CREB1 Activation Confer Cisplatin Resistance in Metastatic Non-small Cell Lung Cancer. Oncogene 38 (2), 180–193. doi:10.1038/s41388-018-0429-3
Kim, J. Y., Choi, J. K., and Jung, H. (2020). Genome-wide Methylation Patterns Predict Clinical Benefit of Immunotherapy in Lung Cancer. Clin. Epigenet 12 (1), 119. doi:10.1186/s13148-020-00907-4
Li, H., Zhang, P., Luo, J., Hu, D., Huang, Y., Zhang, Z.-R., et al. (2019). Chondroitin Sulfate-Linked Prodrug Nanoparticles Target the Golgi Apparatus for Cancer Metastasis Treatment. ACS nano 13 (8), 9386–9396. doi:10.1021/acsnano.9b04166
Li, Y., Sun, R., Li, R., Chen, Y., and Du, H. (2021a). Prognostic Nomogram Based on Circular RNA-Associated Competing Endogenous RNA Network for Patients with Lung Adenocarcinoma. Oxidative Med. Cell. longevity 2021, 1–13. doi:10.1155/2021/9978206
Li, Z., Liu, Z., Wu, Y., Li, H., Sun, Z., Han, C., et al. (2021b). Efficacy and Safety of Apatinib Alone or Apatinib Plus Paclitaxel/docetaxel versus Paclitaxel/docetaxel in the Treatment of Advanced Non-small Cell Lung Cancer: A Meta-Analysis. Thorac. Cancer 12, 2838–2848. doi:10.1111/1759-7714.14131
Liu, J., Huang, Y., Li, T., Jiang, Z., Zeng, L., and Hu, Z. (2021a). The Role of the Golgi Apparatus in Disease (Review). Int. J. Mol. Med. 47 (4), 38. doi:10.3892/ijmm.2021.4871
Liu, Z., Wang, L., Guo, C., Liu, L., Jiao, D., Sun, Z., et al. (2021b). TTN/OBSCN 'Double-Hit' Predicts Favourable Prognosis, 'immune-Hot' Subtype and Potentially Better Immunotherapeutic Efficacy in Colorectal Cancer. J. Cel Mol Med 25 (7), 3239–3251. doi:10.1111/jcmm.16393
Lujan, P., and Campelo, F. (2021). Should I Stay or Should I Go? Golgi Membrane Spatial Organization for Protein Sorting and Retention. Arch. Biochem. Biophys. 707, 108921. doi:10.1016/j.abb.2021.108921
Martins, Á. M., Ramos, C. C., Freitas, D., and Reis, C. A. (2021). Glycosylation of Cancer Extracellular Vesicles: Capture Strategies, Functional Roles and Potential Clinical Applications. Cells 10 (1), 109. doi:10.3390/cells10010109
Moran, T., Palmero, R., Provencio, M., Insa, A., Majem, M., Reguart, N., et al. (2017). A Phase Ib Trial of Continuous Once-Daily Oral Afatinib Plus Sirolimus in Patients with Epidermal Growth Factor Receptor Mutation-Positive Non-small Cell Lung Cancer And/or Disease Progression Following Prior Erlotinib or Gefitinib. Lung Cancer 108, 154–160. doi:10.1016/j.lungcan.2017.03.009
Lim, S. M., Cho, B. C., Kim, S.-W., Kang, S. Y., Heo, D. S., and Kim, H. T. (2016). A multicenter phase II study of sorafenib in combination with erlotinib in patients with advanced non-small cell lung cancer (KCSG-0806). Lung Cancer 93 (1), 9–352. doi:10.1016/j.lungcan.2015.12.005
Muñoz-Fontela, C., Mandinova, A., Aaronson, S. A., and Lee, S. W. (2016). Emerging Roles of P53 and Other Tumour-Suppressor Genes in Immune Regulation. Nat. Rev. Immunol. 16 (12), 741–750. doi:10.1038/nri.2016.99
Peng, B., Li, H., Na, R., Lu, T., Li, Y., Zhao, J., et al. (2021). Identification of a Novel Prognostic Signature of Genome Instability-Related LncRNAs in Early Stage Lung Adenocarcinoma. Front. Cel Dev. Biol. 9, 706454. doi:10.3389/fcell.2021.706454
Perdrizet, K., Sutradhar, R., Li, Q., Liu, N., Earle, C. C., and Leighl, N. B. (2021). Second and Later-Line Erlotinib Use in Non-small Cell Lung Cancer: Real World Outcomes and Practice Patterns Overtime in Canada. J. Thorac. Dis. 13 (9), 5419–5429. doi:10.21037/jtd-21-804
Provencio, M., Majem, M., Guirado, M., Massuti, B., de las Peñas, R., Ortega, A. L., et al. (2021). Phase II Clinical Trial with Metronomic Oral Vinorelbine and Tri-weekly Cisplatin as Induction Therapy, Subsequently Concomitant with Radiotherapy (RT) in Patients with Locally Advanced, Unresectable, Non-small Cell Lung Cancer (NSCLC). Analysis of Survival and Value of ctDNA for Patient Selection. Lung Cancer 153, 25–34. doi:10.1016/j.lungcan.2021.01.005
Schabath, M. B., Welsh, E. A., Fulp, W. J., Chen, L., Teer, J. K., Thompson, Z. J., et al. (2016). Differential Association of STK11 and TP53 with KRAS Mutation-Associated Gene Expression, Proliferation and Immune Surveillance in Lung Adenocarcinoma. Oncogene 35 (24), 3209–3216. doi:10.1038/onc.2015.375
Siegel, R. L., Miller, K. D., and Jemal, A. (2018). Cancer Statistics, 2018. CA: A Cancer J. Clinicians 68 (1), 7–30. doi:10.3322/caac.21442
Siolas, D., Vucic, E., Kurz, E., Hajdu, C., and Bar-Sagi, D. (2021). Gain-of-function p53R172H Mutation Drives Accumulation of Neutrophils in Pancreatic Tumors, Promoting Resistance to Immunotherapy. Cel Rep. 36 (8), 109578. doi:10.1016/j.celrep.2021.109578
Sung, H., Ferlay, J., Siegel, R. L., Laversanne, M., Soerjomataram, I., Jemal, A., et al. (2021). Global Cancer Statistics 2020: GLOBOCAN Estimates of Incidence and Mortality Worldwide for 36 Cancers in 185 Countries. CA A. Cancer J. Clin. 71 (3), 209–249. doi:10.3322/caac.21660
Tao, Y., Yang, Y., Zhou, R., and Gong, T. (2020). Golgi Apparatus: An Emerging Platform for Innate Immunity. Trends Cell Biology 30 (6), 467–477. doi:10.1016/j.tcb.2020.02.008
Vey, M., Schäfer, W., Berghöfer, S., Klenk, H. D., and Garten, W. (1994). Maturation of the Trans-golgi Network Protease Furin: Compartmentalization of Propeptide Removal, Substrate Cleavage, and COOH-Terminal Truncation. J. Cel. Biol. 127, 1829–1842. doi:10.1083/jcb.127.6.1829
Wang, S., Ma, H., Li, H., Liu, Q., Huang, S., Huang, L., et al. (2021). Alternatively Expressed Transcripts Analysis of Non-small Cell Lung Cancer Cells under Different Hypoxic Microenvironment. J. Oncol. 2021, 1–9. doi:10.1155/2021/5558304
Wu, J., Li, L., Zhang, H., Zhao, Y., Zhang, H., Wu, S., et al. (2021). A Risk Model Developed Based on Tumor Microenvironment Predicts Overall Survival and Associates with Tumor Immunity of Patients with Lung Adenocarcinoma. Oncogene 40 (26), 4413–4424. doi:10.1038/s41388-021-01853-y
Yamaguchi, M., Tada, H., Mitsudomi, T., Seto, T., Yokoi, K., Katakami, N., et al. (2021). Phase III Study of Adjuvant Gemcitabine Compared with Adjuvant Uracil-Tegafur in Patients with Completely Resected Pathological Stage IB-IIIA Non-small Cell Lung Cancer (WJTOG0101). Int. J. Clin. Oncol. 26, 2216–2223. doi:10.1007/s10147-021-02012-9
Yu, Y., Zeng, D., Ou, Q., Liu, S., Li, A., Chen, Y., et al. (2019). Association of Survival and Immune-Related Biomarkers with Immunotherapy in Patients with Non-small Cell Lung Cancer. JAMA Netw. Open 2 (7), e196879. doi:10.1001/jamanetworkopen.2019.6879
Yu, Y., Zhang, W., Li, A., Chen, Y., Ou, Q., He, Z., et al. (2020). Association of Long Noncoding RNA Biomarkers with Clinical Immune Subtype and Prediction of Immunotherapy Response in Patients with Cancer. JAMA Netw. Open 3 (4), e202149. doi:10.1001/jamanetworkopen.2020.2149
Zhang, A., Yang, J., Ma, C., Li, F., and Luo, H. (2021). Development and Validation of a Robust Ferroptosis-Related Prognostic Signature in Lung Adenocarcinoma. Front. Cel Dev. Biol. 9, 616271. doi:10.3389/fcell.2021.616271
Zhang, M., Xu, N., Xu, W., Ling, G., and Zhang, P. (2022). Potential Therapies and Diagnosis Based on Golgi-Targeted Nano Drug Delivery Systems. Pharmacol. Res. 175, 105861. doi:10.1016/j.phrs.2021.105861
Zhang, X. (2021). Alterations of Golgi Structural Proteins and Glycosylation Defects in Cancer. Front. Cel Dev. Biol. 9, 665289. doi:10.3389/fcell.2021.665289
Keywords: Golgi apparatus, gene signature, lung adenocarcinoma, machine algorithm, immunotherapy, anticancer drugs
Citation: Jiang Y, Ouyang W, Zhang C, Yu Y and Yao H (2022) Prognosis and Immunotherapy Response With a Novel Golgi Apparatus Signature-Based Formula in Lung Adenocarcinoma. Front. Cell Dev. Biol. 9:817085. doi: 10.3389/fcell.2021.817085
Received: 17 November 2021; Accepted: 20 December 2021;
Published: 20 January 2022.
Edited by:
Ming Yi, Huazhong University of Science and Technology, ChinaReviewed by:
Wangjun Liao, Southern Medical University, ChinaLinghui Zhou, Zhejiang University, China
Shiyu Li, Huazhong University of Science and Technology, China
Copyright © 2022 Jiang, Ouyang, Zhang, Yu and Yao. This is an open-access article distributed under the terms of the Creative Commons Attribution License (CC BY). The use, distribution or reproduction in other forums is permitted, provided the original author(s) and the copyright owner(s) are credited and that the original publication in this journal is cited, in accordance with accepted academic practice. No use, distribution or reproduction is permitted which does not comply with these terms.
*Correspondence: Yunfang Yu, yuyf9@mail.sysu.edu.cn; Herui Yao, yaoherui@mail.sysu.edu.cn