- 1INM-11, Forschungszentrum, Jülich, Germany
- 2Key Laboratory for Multiscale Simulations of Complex Systems, VNU University of Science, Vietnam National University, Hanoi, Vietnam
- 3IAS-5/INM-9, Forschungszentrum, Jülich, Germany
- 4Faculty of Mathematics, Computer Science and Natural Sciences, RWTH Aachen University, Aachen, Germany
- 5Department of Applied Science and Technology (DISAT), Politecnico di Torino, Torino, Italy
- 6College of Chemistry and Molecular Engineering, Institute of Theoretical and Computational Chemistry, Peking University, Beijing, China
- 7The Alexander Silberman Institute of Life Science, The Hebrew University of Jerusalem, Edmond J. Safra Campus at Givat Ram, Jerusalem, Israel
- 8Department of Physics, RWTH Aachen University, Aachen, Germany
- 9Department of Neurology, University Hospital Aachen (UKA), RWTH Aachen University, Aachen, Germany
- 10Jülich Supercomputing Centre (JSC), Forschungszentrum, Jülich, Germany
Human NEET proteins, such as NAF-1 and mitoNEET, are homodimeric, redox iron-sulfur proteins characterized by triple cysteine and one histidine-coordinated [2Fe-2S] cluster. They exist in an oxidized and reduced state. Abnormal release of the cluster is implicated in a variety of diseases, including cancer and neurodegeneration. The computer-aided and structure-based design of ligands affecting cluster release is of paramount importance from a pharmaceutical perspective. Unfortunately, experimental structural information so far is limited to only one ligand/protein complex. This is the X-ray structure of furosemide bound to oxidized mitoNEET. Here we employ an enhanced sampling approach, Localized Volume-based Metadynamics, developed by some of us, to identify binding poses of furosemide to human mitoNEET protein in solution. The binding modes show a high variability within the same shallow binding pocket on the protein surface identified in the X-ray structure. Among the different binding conformations, one of them is in agreement with the crystal structure’s one. This conformation might have been overstabilized in the latter because of the presence of crystal packing interactions, absent in solution. The calculated binding affinity is compatible with experimental data. Our protocol can be used in a straightforward manner in drug design campaigns targeting this pharmaceutically important family of proteins.
Introduction
The human NEET [2Fe-2S] homodimeric proteins (such as mitoNEET (Colca et al., 2004; Paddock et al., 2007) and NAF-1 (Conlan et al., 2009)) have emerged as important targets for pharmaceutical intervention, from cancer and diabetes, to metabolic and neurodegenerative diseases (Nechushtai et al., 2020). These proteins are located on the outer membrane of mitochondria and mitochondria associated membranes, and, in the case of NAF-1, also on the endoplasmic reticulum’s membrane. Each subunit features a 3Cys:1His coordinated [2Fe-2S] cluster (Figure 1), either in a reduced (Fe(III)-Fe(II)) or oxidized (Fe(III)-Fe(III)) state. In the reduced state, the ferrous ion is located close to the protein surface and bound to the histidine (Dicus et al., 2010) (Figure 1). The clusters are reduced and inert in physiological conditions. Oxidation under oxidative stress leads to a cluster-labile oxidized state: the cluster can then be released or transferred to apo-acceptors (Landry and Ding, 2014). Cancer cells may express more human NEET proteins than healthy ones to support their required high level of mitochondrial iron and reactive oxygen species (Darash-Yahana et al., 2016). In contrast, cells undergoing neurodegenerative or metabolic disease express less or no human NEET proteins (Kusminski et al., 2016; Nechushtai et al., 2020). Thus, drugs regulating the [2Fe-2S] cluster stability of human NEET proteins might be able to counteract cell derangement associated with many diseases.
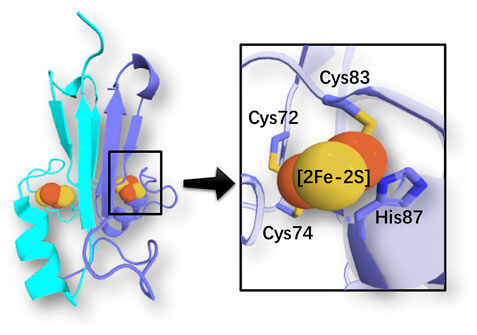
FIGURE 1. Coordination of an iron-sulfur cluster in a member of the NEET protein family (PDB ID: 2QH7 (Paddock et al., 2007)). Cartoon representation of chain A (light blue) and B (cyan). Sulfur and iron atoms are represented by yellow and orange spheres, respectively.
So far, a few ligands targeting mitoNEET (Colca et al., 2004; Paddock et al., 2007) and human NAF-1 (Conlan et al., 2009) have been identified. They have been shown to affect cluster release in vitro, and to bind in their cluster binding domain (Geldenhuys et al., 2019; Marjault et al., 2021). Efficient computational protocols predicting poses and affinities of ligands would be of paramount importance to improve the potency of such drug leads. They allow for artificial intelligence-based screening of new compounds, with optimal solubility and selectivity (Adeshina et al., 2020; Gao et al., 2020). In addition, they provide an estimation of ligands affinities for the oxidized human NEET proteins, which is very useful as accurate in vitro measurements of such affinities may at times be challenging because of the high liability of the cluster at acidic pH (Zuo et al., 2021).
Docking approaches, currently used in the design of ligands targeting enzymes and receptors binding sites, may encounter difficulties here. Indeed, they do not accurately estimate all the possible interaction and desolvation contributions of ligands targeting proteins which lack well-defined binding pockets (Deng et al., 2015). Thus, docking of small molecules on the flat/shallow binding sites of these proteins may lead to false-positives (Li et al., 2014; Guterres and Im, 2020). This problem can be even more exacerbated in transition metal-based systems (Chen et al., 2007), like the NEET proteins.1
Both problems were addressed in the past by some of us by 1) developing molecular simulation docking protocols on proteins lacking specific pocket definitions (Kranjc et al., 2009); and 2) by parameterizing both oxidized and reduced NEET [2Fe-2S] clusters for molecular simulations (Pesce et al., 2017; Zuo et al., 2021). Here, by capitalizing on this work, we use a variant of well-tempered metadynamics (WT-MetaD) enhanced sampling simulations (Barducci et al., 2008) to predict the pose and the potency of the ligand targeting mitoNEET. WT-MetaD is an exact method to calculate the free energy of binding as a function of collective variables (CVs) (Barducci et al., 2008). This variant is the so-called Localized Volume-based (LV) MetaD. This approach has already been successfully applied to study ligand binding to proteins with very high computational efficiency (Zhao et al., 2021).
We focus on the furosemide (4-Chloro-2-[(furan-2-ylmethyl)amino]-5-sulfamoylbenzoic acid) molecule (Chart 1), which slows down cluster release in vitro, and its binding to mitoNEET in the oxidized state (Geldenhuys et al., 2019). This is the only ligand/human NEET protein complex deposited on the protein data bank so far (Geldenhuys et al., 2019). Affinity measurements by radioligand displacement (Geldenhuys et al., 2016) are also available. The X-ray structure shows that the ligand binds in a shallow binding pocket located at the interface between the cluster and the upper part of the monomer (Figure 2A). Specifically, the ligand’s carboxyl group forms hydrogen bonds (H-bonds) with the iron bound histidine residue (H87) of one subunit and a lysine (K55) from the other (Figure 2B). The benzene ring forms hydrophobic interactions with V57, P100, I102, while the furan ring with V70. The NH group forms an intramolecular H-bond with the carboxyl group of the ligand. Finally, the sulfonamide group also forms an H-bond with the protein from the adjacent asymmetric unit (Figure 2C).
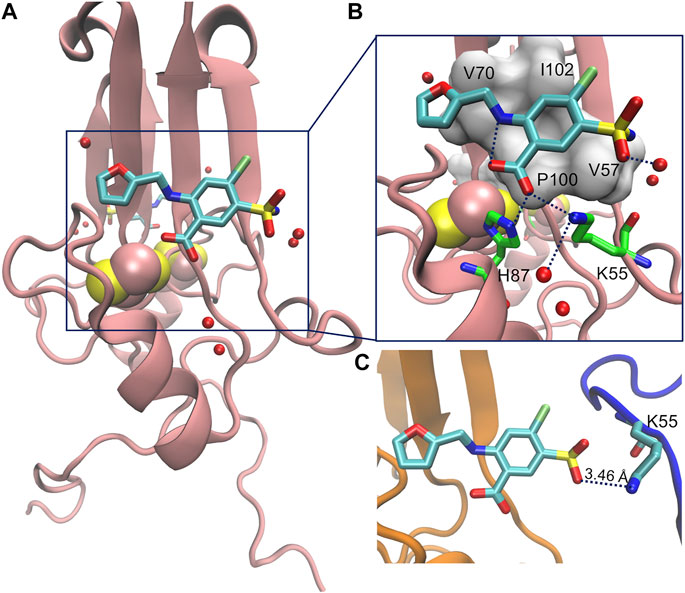
FIGURE 2. (A) Crystal structure of ligand furosemide binding to mitoNEET protein at pH 7.0. (B) Close up showing furosemide-protein H-bonds/salt bridges interactions. (C) Interactions of the ligand with the protein image (in blue color) in the crystal.
Our simulations provide a quantitative estimation of the affinity of binding, which is not too dissimilar from experiment. Most importantly, we suggest, based on our calculations, that furosemide can actually bind in several binding poses around the same surface pocket, including the one observed in the crystal structure. The latter may be stabilized by crystal packing interactions in the solid state (Figure 2C), as observed before (Marelli et al., 2014). These interactions are absent in water solution (Kranjc et al., 2009; Arif et al., 2011; Geldenhuys et al., 2019).
Materials and Methods
System Preparation
The crystal structure of furosemide binding to mitoNEET protein was downloaded from the Protein Data Bank (PDB ID: 6DE9) (Geldenhuys et al., 2019). Maestro (Sastry et al., 2013) (VERSION 2017-2) and GROMACS/2019.4 (Lindahl et al., 2001; Abraham et al., 2019) patched with Plumed 2.5 (Jakalian et al., 2002; Bonomi et al., 2019) were used to perform preparation steps. For protein, water, ions and [2Fe-2S] clusters, we used the AMBER ff99SB-ILDN-DEP (Lindorff-Larsen et al., 2010), TIP3P (Jorgensen et al., 1983), the Åqvist potential (Ȧqvist, 1990) and force field parameters calculated in our previous work (Pesce et al., 2017), respectively. The ligand was parameterized using the General AMBER Force Field (Wang et al., 2004) obtaining the single-point charges using the semi-empirical AM1-BCC method (Jakalian et al., 2002) generated by the acpype utility script (Sousa da Silva and Vranken, 2012) (Supplementary Figure SI1). The system with protein and ligand was solvated in a periodic octahedron box with 28,008 TIP3P (Jorgensen et al., 1983) water molecules. Finally, counterions Na+ (80) and Cl− (87) were added to neutralize the system and mimic the physiological salt concentration at 150 mM. The distance from the protein to the edge of the box turned out to be 20 Å or more during the simulations, avoiding self-interaction artifacts.
The bonds were constrained using the LINCS algorithm (Hess et al., 1997). The smooth Particle Mesh Ewald method (Essmann et al., 1995) was used to treat the long-range electrostatic interactions, with a grid spacing value of 1.2 Å. The cutoff for short-range electrostatic interactions and van der Waals was set to 14 Å. The temperature and pressure of system (T = 298 K, p = 1 bar) were controlled using the Nose-Hoover thermostat (coupling the system every 0.2 ps with a chain length of 10) (Evans and Holian, 1985) and isotropic Parrinello−Rahman barostat (coupling the system every 0.5 ps with a compressibility of 4.5.10–5 bar−1) (Parrinello and Rahman, 1981), respectively. The integration step was set to 2 fs.
Molecular Simulations
We performed energy minimization to the system with the steepest descent algorithm, setting the converge criteria to 2.4 kcal mol−1 nm−1 of the maximum force (Haug et al., 1976). Then, we gradually heated the system in 40 points to 298 K in 1 ns of annealing (Supplementary Figure SI2). The system underwent the first 5 ns NVT Molecular Dynamics (MD) at 298 K with a harmonic restraint of 240 kcal mol−1 nm−2 on both furosemide and protein to maintain the initial experimental conformation. All the bonds were constrained with the LINCS algorithm (Hess et al., 1997). Then, 75 ns NPT MD were performed. Next, the free energy landscape associated with furosemide binding to the protein was investigated by Localized Volume-based Metadynamics (LV-MetaD).
LV-MetaD is a WT-MetaD (Barducci et al., 2008) protocol where a history-dependent potential (called bias) is deposited on three apt collective variables (CVs), i.e., a convenient representation of the reciprocal position of the furosemide with respect to the host protein. To minimize convergence time, the furosemide is constrained in a limited (localized) volume close to the binding pose observed in crystal structure via the imposition of a restraining potential. The coordinate system used to represent the furosemide position in the reference frame of the host protein depends on the shape of the restraining potential. Here, we used a parabolic solid volume restraining as in the original implementation of the method (Zhao et al., 2021). The collective variables were: ρ, defined as the distance between the center of mass of the furosemide and the protein, τ, the parameter that defines the parabolic-solid shape of the volume (Zhao et al., 2021), θ, defined as the azimuthal angle of its orthogonal projection on the x-y plane (Supplementary Figure SI3). To guarantee a correct sampling for both the bound and the unbound state, we limited the restraining volume (and thus the CVs ranges) to include 1) the binding pose observed in crystal structure, 2) the neighboring regions, and 3) enough volume to observe the ligand being completely solvated (Supplementary Figure SI4). The protein-furosemide axis was aligned to the x-axis in our system. To avoid artifacts associated with periodic boundary conditions, we applied a restraining bias that kept the protein’s center of mass to 10 Å or less from the simulation box center. To avoid unfolding problems due to volume bias, the protein backbone atoms, which are not inside the volume, were restrained to their initial positions so that the overall RMSD was smaller than 3 Å.
We applied the bias potential on the system along the defined CVs, setting the initial height of Gaussian hills to 0.287 kcal/mol and deposited every 1 ps. The Gaussian widths are 1 Å, 0.04, and pi/8 for ρ, τ, θ, respectively. The bias factor was chosen to be 20. LV-MetaD for 650 ns. The last 100 ns trajectory was used for the reweighting procedure using the Tiwary-Parrinello estimator (Tiwary and Parrinello, 2015). The reweighting procedure allows us to compute the projection of the free energy landscape as a function of apt order parameters that define clearly the bound and unbound states. From this last free energy surface, it is possible to obtain furosemide’s binding free energy. In our case, we choose to consider two variables: the distance between the protein and the furosemide centers of mass, and the number of H-bonds between the furosemide and the residues inside the volume, defined using the following switching function:
Here we have n = 8 and m = 12, d0 was set to 0 and r0 = 2.5 Å. The number of H-bonds is ∑sij. To evaluate the errors of the free energy, we used a block average analysis (Supplementary Figure SI5). In the lowest energy basin, each pose was equilibrated by 30ns of unbiased MD. MD and LV-MetaD simulations were carried out by GROMACS/2019.4 (Lindahl et al., 2001; Abraham et al., 2019) patched with PLUMED-2.5.2 (Tribello et al., 2014; Bonomi et al., 2019).
Results and Discussion
The identification of ligand poses on NEET proteins may require approaches that go beyond straightforward molecular docking, as the ligand binds on the protein surface (and not to a binding site), close to a multinuclear iron site. Here we have used enhanced sampling methods to predict poses and affinity of furosemide (Chart 1) to the mitoNEET protein, similarly to what done by some of us in the case of a ligand binding to the surface of the prion protein, where the accuracy of our prediction was established by a comparison with NMR data (Kranjc et al., 2009). Our computational protocol profits also from an apt parametrization of the metal cluster recently developed by some of us (Pesce et al., 2017; Zuo et al., 2021).
Our protocol has involved 75 ns of molecular dynamics (MD, Supplementary Figure SI6) starting from the X-ray structure of oxidized mitoNEET in complex with furosemide (Figure 2A). After a short simulated annealing procedure, the system was brought to the same conditions as the in vitro assays. The MD calculations are followed up by Localized Volume-based Metadynamics (Zhao et al., 2021) enhanced sampling method. These predict the free energy of furosemide unbinding in the canonical ensemble as a function of three apt collective variables (Supplementary Figure SI3 and Methods for details). The simulations converged after 600 ns (see Supplementary Figures SI7, SI8). With the reweighting procedure (Tiwary and Parrinello, 2015), we find it convenient to plot the free energy as a function of the distance d of the centers of mass of the furosemide and of the [2Fe-2S] cluster, as well as the number N of furosemide/protein H-bonds and salt bridges.
Basin I is the absolute minimum, lower than about 2 kcal/mol than the local minima II and III. In I, the ligand features three poses with diverse orientations (Ia-c). In each pose the ligand is rather close to the cluster (0.77 nm < d < 0.95 nm) and exhibits extensive intramolecular interactions (5 < N < 9, Figure 3). This includes the salt bridge between the ligand and Nζ@K55 and the H-bond with Nε@H87 (Supplementary Table SI2) (Figure 4), present also in the X-ray structure (Geldenhuys et al., 2019). However, in Ia-b, the salt bridge involves both oxygen atoms and not only one atom as in the X-ray structure (Figure 2), and in Ic, the furosemide’s carboxyl group forms a H-bond with T88 side chain. In all the minima shown here, the carboxy-NH intramolecular H-bond is maintained.
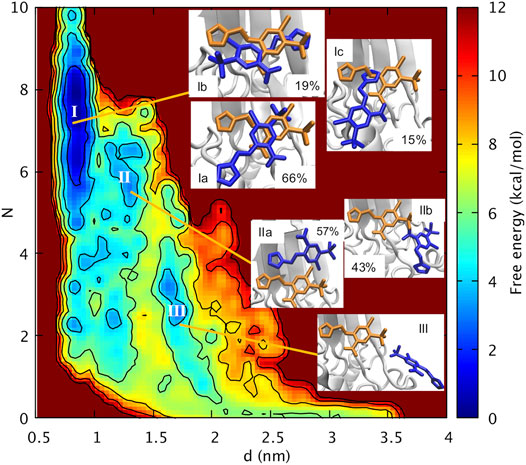
FIGURE 3. Free energy of furosemide unbinding as a function of the distance between the centers of mass of the furosemide and of the Fe-S cluster (d) and the number of H-bonds/salt bridges (N).
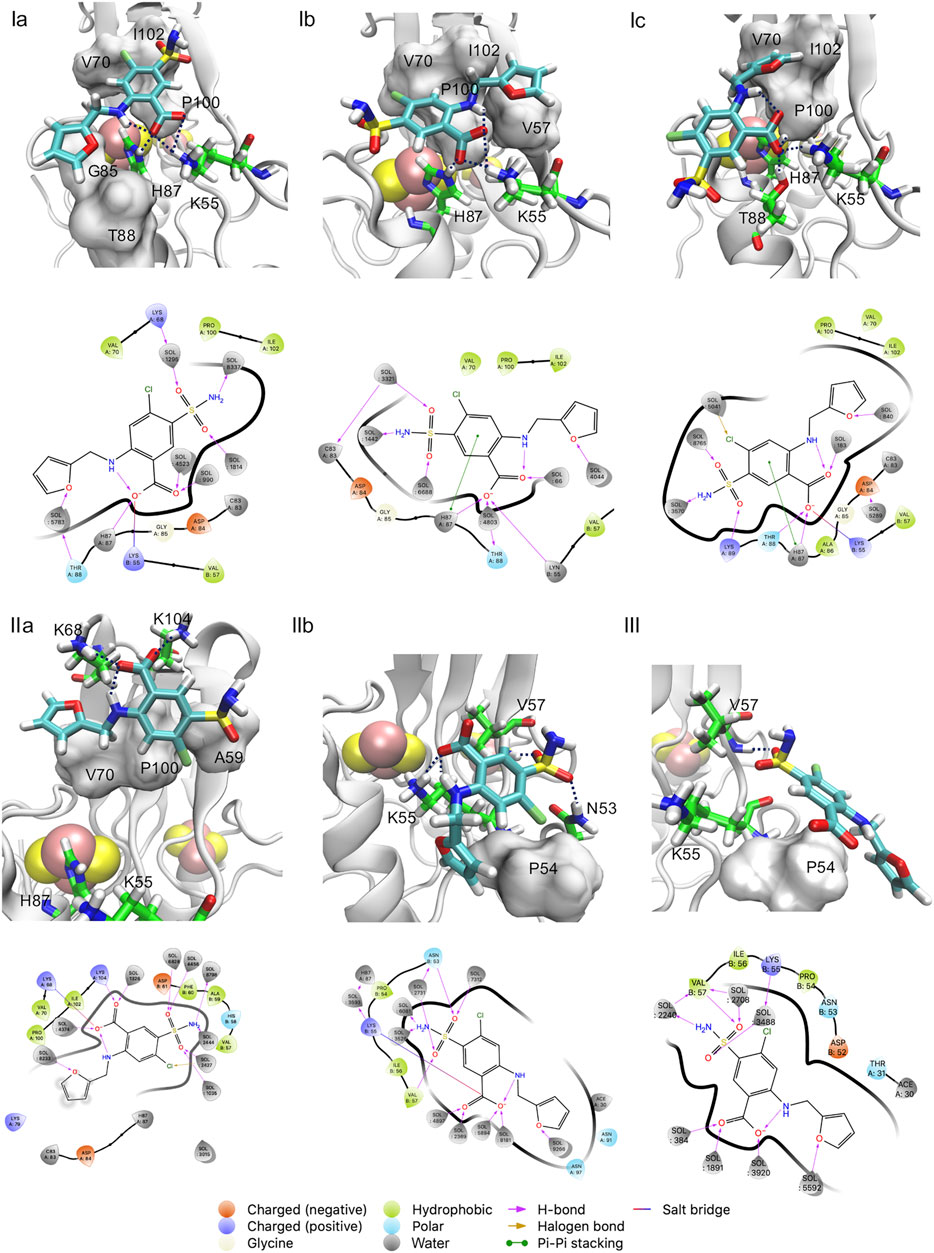
FIGURE 4. (Ia–c, IIa,b, III) poses of Figure 2. Both the 3D structure and the ligand-protein interaction diagram are shown. H-bonds/salt bridges are drawn as dashed lines in the 3D structures.
The orientations of the aromatic rings and the interactions of the sulfonamide group with the protein differ from those of the X-ray structure.
In Ia, by far the most populated conformer2, the sulfonamide group forms a water mediated H-bond with Nζ@K68 (Supplementary Table SI2), while, as discussed above, it interacts with the protein from the adjacent asymmetric unit in the X-ray structure. The furan ring replaces its hydrophobic interactions with V70, present in the X-ray structure, with those with G85 and T88 (Supplementary Table SI2); the benzene ring, while keeping its hydrophobic interactions with P100, I102, replaces the interactions with V57 with those with V70 (Supplementary Table SI2). In Ib, the furan ring interacts with V57 and I102, while the benzene ring interacts with V70 and P100 and it also forms a π-π stacking interaction with H87 (Supplementary Table SI2). The sulfonamide and the carboxyl groups form water-mediated H-bonds with the C83 backbone unit3 and the T88 side chain, respectively (Supplementary Table SI2). In Ic, the furan moiety forms hydrophobic contacts I102, V70, P100, the benzene ring is solvent-exposed.
30 ns MD starting from Ia-c shows that 1) binding poses Ib-c are transient and can interconvert into each other within a few ns (Supplementary Figure SI9). 2) Ia samples other orientations including the one found in the crystallographic pose (Supplementary Figure SI10), and this binding pose reproduces also the experimental electronic density (Supplementary Figure SI11E).4 This variability results from the very shallow binding site as found in the mitoNEET and is already hinted at by challenges in resolving the electron density around the ligand’s furan moiety (Supplementary Figure SI11A)5. The discrepancy between the presence of a unique binding pose and an ensemble of poses (including the X-ray one) in the simulations is attributed here to a packing effect in the crystal. Indeed, in the periodic system (crystal structure), the ligand features a H-bond with K55 of an image protein and this interaction obviously does not present in water solution. We can expect therefore that this interaction stabilizes a specific conformation, following the conformational selection hypothesis (Nussinov et al., 2014), while in water solution an ensemble of conformations may be present.
The free energy of binding/unbinding (7.7 ± 0.8 kcal/mol), from basin I to the fully solvated ligand is not too dissimilar from the experimental free energy of binding at the same temperature (5.8 kcal/mol) (Supplementary Table SI3).
Basin II is located a bit farther from the cluster than I (1.1 nm < d < 1.4 nm, Figure 3). It forms a smaller number of polar intermolecular interactions (5.2 < N < 6.7). It features two similarly populated poses (IIa,b, Figure 4). The H-bond between the carboxyl group and H87 is replaced by a salt bridge with K68 (in IIa) or by an H-bond with the solvent (in IIb). The salt bridge with K55 is maintained only in IIb. In IIa, it involves K104. The sulfonamide group forms H-bonds with V57 backbone and N53 in IIb. The furan ring forms hydrophobic interactions with V70 (IIa) and P54 (IIb), while the benzene ring with A59, I102 (IIa), V57 side chain (IIb). The aromatic rings are more solvent exposed than those in I. The higher solvation of the furosemide may account, at least in part, for the higher free energy of this minimum.
Basin III is located farther from the cluster than II (1.5 nm < d < 1.8 nm). It has lost all the intermolecular interactions in I-II (2.3 < N < 3.5, Figure 3). The carboxyl group is fully hydrated, while the sulfonamide forms direct and water-mediated H-bonds with V57 as well as a water-mediated H-bond with K55 (Figure 4). The aromatic rings have hydrophobic contacts with only P54 and are more solvent-exposed than basin I and II.
In conclusion, our simulations reproduce the pose of the X-ray structure (Supplementary Figure SI9B) and the experimental electronic density (Supplementary Figure SI11E), suggesting that this is only one among an ensemble of structures in water solution. The binding free energy values are quantitatively close to the experimental data. Thus, our paper is consistent with the available experimental data.
Conclusion
Here, we have investigated furosemide binding to mitoNEET in the oxidized state with the following goals in mind: 1) the comparison with the X-ray structure, which is in the oxidized state (Geldenhuys et al., 2019) and 2) to present an advanced computational approach able to investigate, for the first time to the best of our knowledge, quantitatively ligand binding to human NEET proteins, a highly important pharmacological target. Our study suggests that the ligand binds to several isoenergetic poses in water solution, including the one emerging from the X-ray structure. The latter pose is likely to have been selected because of crystal packing interactions. The calculations provide an estimate of the affinity which is fully compatible with that experimentally determined. Driven by the computational findings here, NMR and/or site-directed mutagenesis experiments in the binding regions, such as in those in (Kranjc et al., 2009) and (Zhou et al., 2010) for other ligands bound to protein surfaces, would be additional validations of our calculations.
Our protocol is very general and it emerges as a useful tool to predict binding affinity and multiple poses of ligands targeting human NEET proteins.
Data Availability Statement
The raw data supporting the conclusion of this article will be made available by the authors, without undue reservation.
Author Contributions
LH and JG performed the calculations and prepared figures and tables as well as helped in writing the manuscript. RC, PC, TN, ZS, KZ, JS, and GR wrote the paper with input from all authors. JS and PC supervised the project and gave valuable corrections.
Funding
PC, GR, and JS acknowledge the Deutsche Forschungsgemeinschaft (DFG) via the Research Training Group RTG2416 MultiSenses-MultiScales (368482240/GRK2416). KZ is supported by the Marie Skłodowska-Curie Grant agreement no. 765048. TN and LH are supported partially by the Vietnam National University–Hanoi grant number TXTCN.21.28.
Conflict of Interest
The authors declare that the research was conducted in the absence of any commercial or financial relationships that could be construed as a potential conflict of interest.
Publisher’s Note
All claims expressed in this article are solely those of the authors and do not necessarily represent those of their affiliated organizations or those of the publisher, the editors, and the reviewers. Any product that may be evaluated in this article, or claim that may be made by its manufacturer, is not guaranteed or endorsed by the publisher.
Acknowledgments
The authors acknowledge many fruitful discussions with Rachel Nechushtai. GR and PC acknowledge the European Union’s Horizon 2020 Framework Programme for Research and Innovation under the Specific Grant Agreement No. 945539 (Human Brain Project SGA3).
Supplementary Material
The Supplementary Material for this article can be found online at: https://www.frontiersin.org/articles/10.3389/fcell.2022.886568/full#supplementary-material
Footnotes
1In spite of these limitations, successful applications of simplified docking approaches such as MAD-28 to mitoNEET/NAF-1 have been reported (Bai et al., 2015). These applications are reported in the SI for furosemide binding to mitoNEET, allowing for a comparison with the free energy calculations performed in this work (Supplementary Table SI1).
2The populations for Ia-c are 66%, 19%, 15%, respectively.
3This cysteine is bound to the iron atom close to the solvent.
4For a direct comparison between X-ray and basins poses see Supplementary Figure SI12.
5The populations for IIa and IIb are 57% and 43%, respectively
References
Abraham, M. J. V. d. S. D., Lindahl, E., and Hess, B. (2019). GROMACS User Manual Version 2019.4 [Online]. Available at: http://www.gromacs.org, Accessed 2022).
Adeshina, Y. O., Deeds, E. J., and Karanicolas, J. (2020). Machine Learning Classification Can Reduce False Positives in Structure-Based Virtual Screening. Proc. Natl. Acad. Sci. U.S.A. 117 (31), 18477–18488. doi:10.1073/pnas.2000585117
Ȧqvist, J. (1990). Ion-water Interaction Potentials Derived from Free Energy Perturbation Simulations. J. Phys. Chem. 94 (21), 8021–8024. doi:10.1021/j100384a009
Arif, W., Xu, S., Isailovic, D., Geldenhuys, W. J., Carroll, R. T., and Funk, M. O. (2011). Complexes of the Outer Mitochondrial Membrane Protein MitoNEET with Resveratrol-3-Sulfate. Biochemistry 50 (25), 5806–5811. doi:10.1021/bi200546s
Bai, F., Morcos, F., Sohn, Y.-S., Darash-Yahana, M., Rezende, C. O., Lipper, C. H., et al. (2015). The Fe-S Cluster-Containing NEET Proteins mitoNEET and NAF-1 as Chemotherapeutic Targets in Breast Cancer. Proc. Natl. Acad. Sci. U.S.A. 112(12), 3698–3703. doi:10.1073/pnas.1502960112
Barducci, A., Bussi, G., and Parrinello, M. (2008). Well-Tempered Metadynamics: A Smoothly Converging and Tunable Free-Energy Method. Phys. Rev. Lett. 100 (2), 020603. doi:10.1103/PhysRevLett.100.020603
Bonomi, M., Bussi, G., Camilloni, C., Tribello, G. A., Banáš, P., Barducci, A., et al. (2019). Promoting Transparency and Reproducibility in Enhanced Molecular Simulations. Nat. Methods 16 (8), 670–673. doi:10.1038/s41592-019-0506-8
Capelli, R., Carloni, P., and Parrinello, M. (2019). Exhaustive Search of Ligand Binding Pathways via Volume-Based Metadynamics. J. Phys. Chem. Lett. 10 (12), 3495–3499. doi:10.1021/acs.jpclett.9b01183
Chen, D., Menche, G., Power, T. D., Sower, L., Peterson, J. W., and Schein, C. H. (2007). Accounting for Ligand-Bound Metal Ions in Docking Small Molecules on Adenylyl Cyclase Toxins. Proteins 67 (3), 593–605. doi:10.1002/prot.21249
Colca, J. R., McDonald, W. G., Waldon, D. J., Leone, J. W., Lull, J. M., Bannow, C. A., et al. (2004). Identification of a Novel Mitochondrial Protein ("mitoNEET") Cross-Linked Specifically by a Thiazolidinedione Photoprobe. Am. J. Physiology-Endocrinology Metab. 286 (2), E252–E260. doi:10.1152/ajpendo.00424.2003
Conlan, A. R., Axelrod, H. L., Cohen, A. E., Abresch, E. C., Zuris, J., Yee, D., et al. (2009). Crystal Structure of Miner1: The Redox-Active 2Fe-2S Protein Causative in Wolfram Syndrome 2. J. Mol. Biol. 392 (1), 143–153. doi:10.1016/j.jmb.2009.06.079
Darash-Yahana, M., Pozniak, Y., Lu, M., Sohn, Y.-S., Karmi, O., Tamir, S., et al. (2016). Breast Cancer Tumorigenicity Is Dependent on High Expression Levels of NAF-1 and the Lability of its Fe-S Clusters. Proc. Natl. Acad. Sci. U.S.A. 113 (39), 10890–10895. doi:10.1073/pnas.1612736113
Deng, N., Forli, S., He, P., Perryman, A., Wickstrom, L., Vijayan, R. S. K., et al. (2015). Distinguishing Binders from False Positives by Free Energy Calculations: Fragment Screening against the Flap Site of HIV Protease. J. Phys. Chem. B 119 (3), 976–988. doi:10.1021/jp506376z
Dicus, M. M., Conlan, A., Nechushtai, R., Jennings, P. A., Paddock, M. L., Britt, R. D., et al. (2010). Binding of Histidine in the (Cys)3(His)1-Coordinated [2Fe−2S] Cluster of Human mitoNEET. J. Am. Chem. Soc. 132 (6), 2037–2049. doi:10.1021/ja909359g
Essmann, U., Perera, L., Berkowitz, M. L., Darden, T., Lee, H., and Pedersen, L. G. (1995). A Smooth Particle Mesh Ewald Method. J. Chem. Phys. 103 (19), 8577–8593. doi:10.1063/1.470117
Evans, D. J., and Holian, B. L. (1985). The Nose-Hoover Thermostat. J. Chem. Phys. 83 (8), 4069–4074. doi:10.1063/1.449071
Gao, D., Chen, Q., Zeng, Y., Jiang, M., and Zhang, Y. (2020). Applications of Machine Learning in Drug Target Discovery. Cdm 21 (10), 790–803. doi:10.2174/1567201817999200728142023
Geldenhuys, W. J., Yonutas, H. M., Morris, D. L., Sullivan, P. G., Darvesh, A. S., and Leeper, T. C. (2016). Identification of Small Molecules that Bind to the Mitochondrial Protein mitoNEET. Bioorg. Med. Chem. Lett. 26 (21), 5350–5353. doi:10.1016/j.bmcl.2016.09.009
Geldenhuys, W. J., Long, T. E., Saralkar, P., Iwasaki, T., Nuñez, R. A. A., Nair, R. R., et al. (2019). Crystal Structure of the Mitochondrial Protein mitoNEET Bound to a Benze-Sulfonide Ligand. Commun. Chem. 2 (1), 77. doi:10.1038/s42004-019-0172-x
Guterres, H., and Im, W. (2020). Improving Protein-Ligand Docking Results with High-Throughput Molecular Dynamics Simulations. J. Chem. Inf. Model. 60 (4), 2189–2198. doi:10.1021/acs.jcim.0c00057
Haug, E. J., Arora, J. S., and Matsui, K. (1976). A Steepest-Descent Method for Optimization of Mechanical Systems. J. Optim. Theor. Appl. 19 (3), 401–424. doi:10.1007/bf00941484
Hess, B., Bekker, H., Berendsen, H. J. C., and Fraaije, J. G. E. M. (1997). LINCS: A Linear Constraint Solver for Molecular Simulations. J. Comput. Chem. 18 (12), 1463–1472. doi:10.1002/(sici)1096-987x(199709)18:12<1463::aid-jcc4>3.0.co;2-h
Jakalian, A., Jack, D. B., and Bayly, C. I. (2002). Fast, Efficient Generation of High-Quality Atomic Charges. AM1-BCC Model: II. Parameterization and Validation. J. Comput. Chem. 23 (16), 1623–1641. doi:10.1002/jcc.10128
Jorgensen, W. L., Chandrasekhar, J., Madura, J. D., Impey, R. W., and Klein, M. L. (1983). Comparison of Simple Potential Functions for Simulating Liquid Water. J. Chem. Phys. 79 (2), 926–935. doi:10.1063/1.445869
Kranjc, A., Bongarzone, S., Rossetti, G., Biarnés, X., Cavalli, A., Bolognesi, M. L., et al. (2009). Docking Ligands on Protein Surfaces: The Case Study of Prion Protein. J. Chem. Theor. Comput. 5 (9), 2565–2573. doi:10.1021/ct900257t
Kusminski, C. M., Chen, S., Ye, R., Sun, K., Wang, Q. A., Spurgin, S. B., et al. (2016). MitoNEET-Parkin Effects in Pancreatic α- and β-Cells, Cellular Survival, and Intrainsular Cross Talk. Diabetes 65 (6), 1534–1555. doi:10.2337/db15-1323
Landry, A. P., and Ding, H. (2014). Redox Control of Human Mitochondrial Outer Membrane Protein MitoNEET [2Fe-2S] Clusters by Biological Thiols and Hydrogen Peroxide. J. Biol. Chem. 289 (7), 4307–4315. doi:10.1074/jbc.M113.542050
Li, Y., Han, L., Liu, Z., and Wang, R. (2014). Comparative Assessment of Scoring Functions on an Updated Benchmark: 2. Evaluation Methods and General Results. J. Chem. Inf. Model. 54 (6), 1717–1736. doi:10.1021/ci500081m
Limongelli, V., Bonomi, M., and Parrinello, M. (2013). Funnel Metadynamics as Accurate Binding Free-Energy Method. Proc. Natl. Acad. Sci. U.S.A. 110 (16), 6358–6363. doi:10.1073/pnas.1303186110
Lindahl, E., Hess, B., and van der Spoel, D. (2001). GROMACS 3.0: a Package for Molecular Simulation and Trajectory Analysis. J. Mol. Model. 7 (8), 306–317. doi:10.1007/s008940100045
Lindorff-Larsen, K., Piana, S., Palmo, K., Maragakis, P., Klepeis, J. L., Dror, R. O., et al. (2010). Improved Side-Chain Torsion Potentials for the Amber ff99SB Protein Force Field. Proteins 78 (8), 1950–1958. doi:10.1002/prot.22711
Madhavi Sastry, G., Adzhigirey, M., Day, T., Annabhimoju, R., and Sherman, W. (2013). Protein and Ligand Preparation: Parameters, Protocols, and Influence on Virtual Screening Enrichments. J. Comput. Aided. Mol. Des. 27 (3), 221–234. doi:10.1007/s10822-013-9644-8
Marelli, U. K., Frank, A. O., Wahl, B., La Pietra, V., Novellino, E., Marinelli, L., et al. (2014). Receptor-Bound Conformation of Cilengitide Better Represented by its Solution-State Structure Than the Solid-State Structure. Chem. Eur. J. 20 (44), 14201–14206. doi:10.1002/chem.201403839
Marjault, H.-B., Zuo, K., Mittler, R., Carloni, P., and Nechushtai, R. (2021). “Chapter 21 - NEET Proteins as Novel Drug Targets for Mitochondrial Dysfunction,” in Clinical Bioenergetics. Editor S. Ostojic (Academic Press), 477–488. doi:10.1016/b978-0-12-819621-2.00021-8
Nechushtai, R., Karmi, O., Zuo, K., Marjault, H.-B., Darash-Yahana, M., Sohn, Y.-S., et al. (2020). The Balancing Act of NEET Proteins: Iron, ROS, Calcium and Metabolism. Biochim. Biophys. Acta (Bba) - Mol. Cel Res. 1867 (11), 118805. doi:10.1016/j.bbamcr.2020.118805
Nussinov, R., Ma, B., and Tsai, C.-J. (2014). Multiple Conformational Selection and Induced Fit Events Take Place in Allosteric Propagation. Biophysical Chem. 186, 22–30. doi:10.1016/j.bpc.2013.10.002
Paddock, M. L., Wiley, S. E., Axelrod, H. L., Cohen, A. E., Roy, M., Abresch, E. C., et al. (2007). MitoNEET Is a Uniquely Folded 2Fe-2S Outer Mitochondrial Membrane Protein Stabilized by Pioglitazone. Proc. Natl. Acad. Sci. U.S.A. 104 (36), 14342–14347. doi:10.1073/pnas.0707189104
Parrinello, M., and Rahman, A. (1981). Polymorphic Transitions in Single Crystals: A New Molecular Dynamics Method. J. Appl. Phys. 52 (12), 7182–7190. doi:10.1063/1.328693
Pesce, L., Calandrini, V., Marjault, H.-b., Lipper, C. H., Rossetti, G., Mittler, R., et al. (2017). Molecular Dynamics Simulations of the [2Fe-2S] Cluster-Binding Domain of NEET Proteins Reveal Key Molecular Determinants that Induce Their Cluster Transfer/Release. J. Phys. Chem. B 121 (47), 10648–10656. doi:10.1021/acs.jpcb.7b10584
Sousa da Silva, A. W., and Vranken, W. F. (2012). ACPYPE - AnteChamber PYthon Parser interfacE. BMC Res. Notes 5 (1), 367. doi:10.1186/1756-0500-5-367
Tiwary, P., and Parrinello, M. (2015). A Time-independent Free Energy Estimator for Metadynamics. J. Phys. Chem. B 119 (3), 736–742. doi:10.1021/jp504920s
Tribello, G. A., Bonomi, M., Branduardi, D., Camilloni, C., and Bussi, G. (2014). PLUMED 2: New Feathers for an Old Bird. Comp. Phys. Commun. 185 (2), 604–613. doi:10.1016/j.cpc.2013.09.018
Wang, J., Wolf, R. M., Caldwell, J. W., Kollman, P. A., and Case, D. A. (2004). Development and Testing of a General Amber Force Field. J. Comput. Chem. 25 (9), 1157–1174. doi:10.1002/jcc.20035
Zhao, Q., Capelli, R., Carloni, P., Lüscher, B., Li, J., and Rossetti, G. (2021). Enhanced Sampling Approach to the Induced-Fit Docking Problem in Protein-Ligand Binding: The Case of Mono-ADP-Ribosylation Hydrolase Inhibitors. J. Chem. Theor. Comput. 17 (12), 7899–7911. doi:10.1021/acs.jctc.1c00649
Zhou, T., Lin, J., Feng, Y., and Wang, J. (2010). Binding of Reduced Nicotinamide Adenine Dinucleotide Phosphate Destabilizes the Iron−Sulfur Clusters of Human MitoNEET. Biochemistry 49 (44), 9604–9612. doi:10.1021/bi101168c
Keywords: NEET proteins, rational drug design, localized volume-based metadynamics, furosemide binding pose and affinity, furosemide, molecular dynamics, [2Fe-2S] cluster
Citation: Hoang LG, Goßen J, Capelli R, Nguyen TT, Sun Z, Zuo K, Schulz JB, Rossetti G and Carloni P (2022) Multiple Poses and Thermodynamics of Ligands Targeting Protein Surfaces: The Case of Furosemide Binding to mitoNEET in Aqueous Solution. Front. Cell Dev. Biol. 10:886568. doi: 10.3389/fcell.2022.886568
Received: 28 February 2022; Accepted: 04 April 2022;
Published: 26 April 2022.
Edited by:
Kourosh Honarmand Ebrahimi, King’s College London, United KingdomReviewed by:
Sergey Samsonov, University of Gdansk, PolandHuangen Ding, Louisiana State University, United States
Copyright © 2022 Hoang, Goßen, Capelli, Nguyen, Sun, Zuo, Schulz, Rossetti and Carloni. This is an open-access article distributed under the terms of the Creative Commons Attribution License (CC BY). The use, distribution or reproduction in other forums is permitted, provided the original author(s) and the copyright owner(s) are credited and that the original publication in this journal is cited, in accordance with accepted academic practice. No use, distribution or reproduction is permitted which does not comply with these terms.
*Correspondence: Riccardo Capelli, cmljY2FyZG8uY2FwZWxsaUBwb2xpdG8uaXQ=; Giulia Rossetti, Zy5yb3NzZXR0aUBmei1qdWVsaWNoLmRl
†These authors have contributed equally to this work and share first authorship