- 1State Key Laboratory of Food Science and Technology, Jiangnan University, Wuxi, China
- 2School of Food Science and Technology, Jiangnan University, Wuxi, China
- 3National Engineering Research Center for Functional Food, Jiangnan University, Wuxi, China
- 4Beijing Innovation Center of Food Nutrition and Human Health, Beijing Technology and Business University (BTBU), Beijing, China
Lactobacillus plantarum, a widely used probiotic in the food industry, exists in diverse habitats, which has led to its niche-specific genetic evolution. However, the relationship between this type of genetic evolution and the bacterial phenotype remains unclear. Here, six L. plantarum strains derived from paocai and human feces were analyzed at the genomic and phenotypic levels to investigate the features of adaptive evolution in different habitats. A comparative genomic analysis showed that 93 metabolism-related genes underwent structural variations (SVs) during adaptive evolution, including genes responsible for carbohydrate, lipid, amino acid, inorganic ion and coenzyme transport and metabolism, and energy production and conversion. Notably, seven virulence factor-related genes in strains from both habitats showed SVs — similar to the pattern found in the orthologous virulence genes of pathogenic bacteria shared similar niches, suggesting the possibility of horizontal gene transfer. These genomic variations further influenced the metabolic abilities of strains and their interactions with the commensal microbiota in the host intestine. Compared with the strains from feces, those from paocai exhibited a shorter stagnation period and a higher growth rate in a diluted paocai solution because of variations in functional genes. In addition, opposite correlations were identified between the relative abundances of L. plantarum strains and the genus Bifidobacterium in two media inoculated with strains from the two habitats. Overall, our findings revealed that the niche-specific genetic evolution of L. plantarum strains is associated with their fermentation abilities and physiological functions in host gut health. This knowledge can help guiding the exploration and application of probiotics from the specific niches-based probiotic exploitation.
Introduction
Lactobacillus is a genus of gram-positive acid-tolerant bacteria that occupy a wide variety of niches. It is one of the most widely used probiotic species in the food industry because of its well-known health benefits to the host (Seddik et al., 2017). L. plantarum is a nomadic species that exists in a variety of habitats, including plants, the gastro-intestinal tracts of animals (including humans), and food materials (Martino et al., 2016).
Several studies have shown that the habitat diversity of probiotics leads to their niche-specific genetic evolution through single nucleotide polymorphisms (SNPs) and genomic structural variations (SVs) (Zhang, 2008; Barrick et al., 2009). The latter can be further classified into copy number variations caused by deletions, insertions or duplications; changes in orientation caused by inversions; and changes in chromosomal location caused by translocations and fusions (Wellenreuther et al., 2019). A previous study of a Western population identified 16 point mutations in the genome of the gut commensal microbe Bacteroides fragilis due to changes in the intestinal environment caused by dietary variations (Zhao et al., 2019). A comparative genomic study on 21 L. salivarius strains isolated from different habitats identified 15 genes that were involved in adaptation to the host, most of which encoded extracellular proteins and orthologs related to exopolysaccharide production (Lee et al., 2017). Another study on L. mucosae LM1 identified potential niche-specific genes for involved in the adaptation to various digestive tracts, such as glycogen metabolism- and folate biosynthesis- related genes. (Valeriano et al., 2019).
It should be noted that the characteristics of niche-specific evolution in L. plantarum genomes are controversial. A previous research based on phylogenomic and functional divergence analyses revealed that genomic variations in L. plantarum were uncoupled from their environmental origin (Martino et al., 2016) (Martino et al., 2016). Another study, which compared the genomes of 108 L. plantarum strains, showed that strain-specific genomic profiles were not associated with their original habitats (Sukjung et al., 2018). However, other studies reported that L. plantarum could undergo genomic variations because of ecological constraints, as indicated by the tendency of strains from the same habitats possess similar functional genomic profiles (Papizadeh et al., 2017; Cen et al., 2020). Also, a clinical study revealed that L. plantarum P-8 underwent genomic variations under the adaptive pressure of the host intestinal environment (Song et al., 2018). The differences between these experimental results were probably caused by diverse experimental and analytical methods and variations in the number of strains analyzed. Therefore, more studies are warranted to clarify the characteristics of niche-specific L. plantarum evolution.
Variations in bacterial genes, especially functional protein-encoding genes, are known to influence the bacterial phenotype. One study showed that different abundances of sugar-converting genes in three L. paracasei strains led to strain-level variations in carbohydrate utilization (Stefanovic and McAuliffe, 2018). Another study on the vaginal probiotic strain L. rhamnosus identified between-strain differences in genes related to the carbohydrate metabolic pathway and confirmed the phenotypic variations in a sugar utilization assay (Petrova et al., 2018).
Because of the wide usage of Lactobacillus species in food products, clarification of the relationship between the niche-specific adaptive evolution and phenotype of Lactobacillus spp. will be helpful in the development of potential probiotics. However, the majority of published studies in this area only have focused only on genome level changes, without further validation at the phenotypic level (Martino et al., 2016; Kelleher et al., 2017; Valeriano et al., 2019). Our previous research uncovered genomic variations in 140 L. plantarum strains (Cen et al., 2020). Nevertheless, the relationships between the genomic differences, original habitats, and phenotypes of L. plantarum strains remain unclear.
Therefore, in this study, a comparative genomic analysis was performed to identify niche-specific genomic variations in L. plantarum strains under adaptive pressure and associated these with various phenotypes. A fermentation assay was performed to verify the influences of adaptive evolution on the metabolism of these strains. In addition, 16S rRNA gene sequencing was performed to identify the correlation between the genomic variations in strains and the interaction of these strains with the host gut flora.
Materials and Methods
Bacterial Strains and Incubation Conditions
According to our previous research, six L. plantarum strains with different phylogeny were used in this study (Cen et al., 2020). Information about the six L. plantarum strains is listed in Table 1. The strains that had been preserved at - 80 °C in glycerol stock were grown overnight in de Man-Rogosa-Sharpe (MRS) broth (Sangon Biotech, Shanghai, China) at 37°C in an anaerobic workstation [the gas supply system was described in a previous article (Cen et al., 2020)], followed by 2% (v/v) subculture in fresh MRS.
Comparative Genomic Analysis
The whole-genome sequencing protocol and assembly and prediction methods were performed as described in a previous study (Zhai et al., 2019).
Orthologs in the six strains were defined using OrthoMCL (https://orthomcl.org/orthomcl/) with its default parameters (Fischer et al., 2011). Next, the chosen core-gene sequences were aligned using MAFFT-7.427 (https://mafft.cbrc.jp/alignment/software) (Katoh and Standley, 2013), and the phylogeny was inferred by the neighbor-joining method (Saitou and Nei, 1987). The phylogenetic tree was visualized using Evolview v2 online (www.evolgenius.info/evolview).
Orthologous proteins, carbohydrate-active enzymes, virulence factors, and antibiotic factors were annotated against the COG (Tatusov et al., 2000), CAZyme (Cantarel et al., 2009), VFDB (Chen et al., 2005) and CARD (Jia et al., 2017) databases, respectively, using BLAST (https://github.com/mosuka/blast) with its default parameters (Altschul et al., 1990).
Growth of Lactobacillus Strains in a Paocai Diluent and Simulated Intestinal Juice
After sub-culturing twice, a 5% (v/v) inoculum of each of the six strains was inoculated into a paocai solution diluted with five parts of water (hereafter, the paocai diluent) and simulated intestinal juice, which was prepared according to a published method with minor modification (Minekus et al., 1999). Briefly, the fermentation medium was prepared as described in a previous study (Lau et al., 2016). Feces were washed twice with PBS and were dissolved with PBS to prepare the feces suspension in the ratio of 1:1 by weight. The feces suspension and the medium were mixed in the ratio of 10:1 by volume. The mixtures were incubated at 37°C in an anaerobic workstation.
Colony-forming units (CFUs) of all of the six strains grown in a paocai diluent were counted every 4 h during a 36-h period by the plate count method. The growth profiles of the strains in the simulated intestinal juice were measured by RT-PCR at 0, 6, 12, 24, and 36 h after inoculation. The design of the primer sequences and calculation of relative quantification were performed as described previously (Klocke et al., 2006). All experiments were conducted in triplicate.
DNA Extraction and 16S rRNA Gene Sequencing
Cultures grown in the simulated intestinal juice were collected at the same time points as indicated for the RT-PCR assay. Metagenomic DNA was extracted using the FastDNA Spin Kit for Soil (MP Biomedicals, Shanghai, China), according to the manufacturer’s instructions. The V3-V4 regions of the bacterial 16S rRNA gene were amplified via PCR. The PCR primers and reaction program were as described previously (Fang et al., 2020). PCR products were gel-purified using GeneClean Turbo (MP Biomedicals, Shanghai, China). The DNA concentrations were measured using a dsDNA assay with NanoDrop spectrophotometers (Thermo, Shanghai, China). Subsequently, 16S rRNA sequencing was performed as described previously (Zhai et al., 2019).
Sequence Processing and Bioinformatics Analysis
After sequencing, the 16S rRNA sequence data were analyzed using the QIIME2 (https://github.com/qiime2/) pipeline according to a previously published method (Zhai et al., 2019). Briefly, the raw sequences were aligned to exclude low-quality and short-length sequences. High-quality sequences with similarities > 97% were clustered into the same OTUs and representative sequences of each cluster were used to identify bacterial taxa. Beta diversity metrics were computed according to the Manhattan method in R-4.0.2 (https://cran.r-project.org/bin/windows/base/) using genus-level data.
Statistical Analysis
All statistical analyses were performed using R-4.0.2. The pheatmap package was used to perform a cluster analysis of different functional genes between the two niche groups and to visualize. A principal component analysis (PCA) was performed using the prcomp function in the basic package, and the result was visualized using the ggbiplot package. The pairwise.t.test function was used to perform pairwise comparisons between the groups, and the wilcox.test function was used to perform two-sample Wilcoxon tests. A Pearson’s correlation analysis and a false discovery rate (FDR) were conducted using the psych package, and the correlation matrix was visualized using the corrplot package. A linear multiple regression model was established using the lm function in R, and its accuracy was tested using Spearman’s correlation (Johnson et al., 2019). The model was as follows: rn,i = f (Rn−1, Gj)where rn,i represents the relative abundance of the genus i at n hours after the strain was inoculated into the medium, Rn-1 represents the projection of the relative abundance of the microbiome on the PC1 and PC2 of PCA, and Gj represents the projection of the inoculated strain’s functional gene numbers on the PC1 and PC2 of PCA.
Results
Functional Genomic SVs in L. plantarum Strains from Different Habitats
The six L. plantarum strains were divided into two groups based on their initial habitats (paocai-derived strains and feces-derived strains). Next, the core genes that differed between the two groups were selected and annotated using four databases to identify their functions.
On average, 238 unique genes were identified in the human feces-derived strains, whereas only 33 unique genes were identified in the paocai-derived strains (Figure 1A). No significant differences (p > 0.05) were observed in the GC% and genome size between the two groups of strains (Figure 1B), indicating that natural selection in different niches led to SVs in only a few of the core genes. In particular, the mutation rates were 5.29% and 2.40% in the paocai-derived strains and feces-derived strains, respectively.
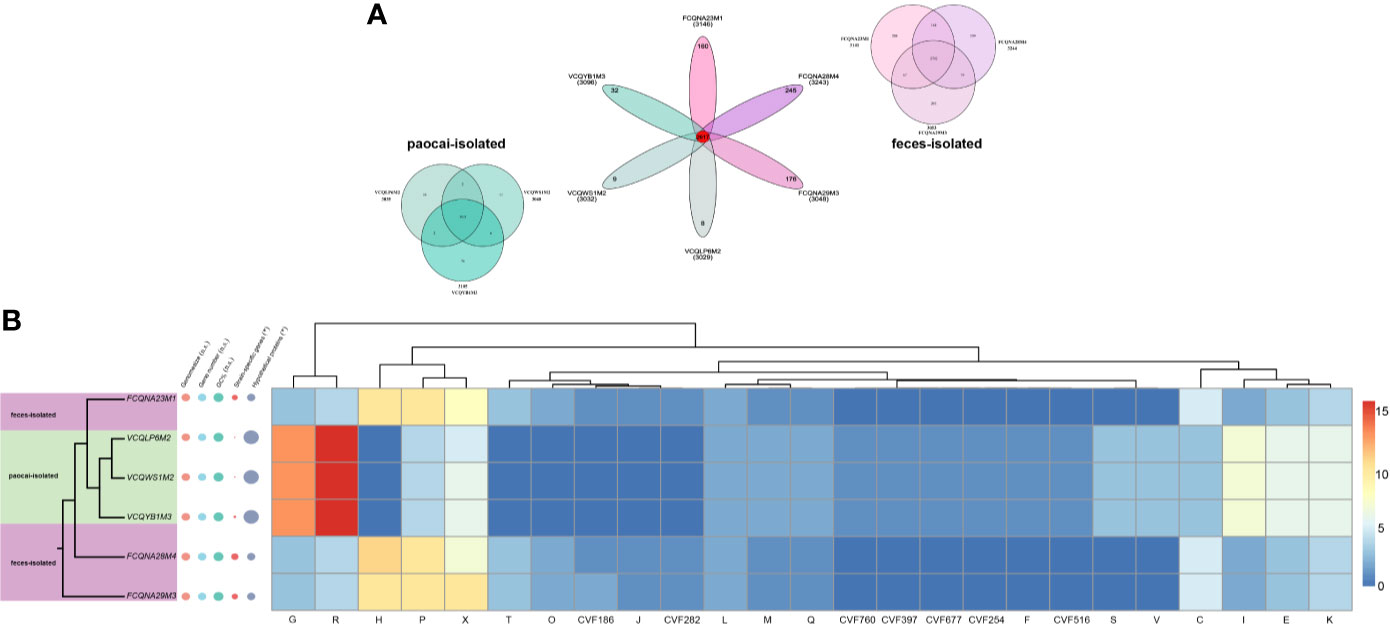
Figure 1 Comparative genomic and phylogenetic analyses of six L. plantarum strains. (A) Venn diagrams based on the pan genes of six L. plantarum strains isolated from paocai and human feces. (B) Phylogenetic tree of six L. plantarum strains constructed using the neighbor-joining method (left), and heatmap of the numbers of different core genes present in the strains’ genomes (the genes were annotated using four databases) (right). The basic information of strains’ genes, including genome sizes, gene numbers, GC%, and hypothetical protein numbers, is plotted beside the tree. The size of the circle represents the size of the value. n.s. represents p > 0.1, *represents p ≤ 0.1.
Functional annotations revealed the presence of SVs in 93 metabolism-related genes, including those involved in carbohydrate (G), lipid (I), amino acid (E), inorganic ion (P) and coenzyme (H) transport and metabolism, and energy production and conversion (C) (Figure 1B). Furthermore, 11 genes in the two groups classified into ‘mobilome, prophages, transposons’ category (X) were determined to have been acquired by horizontal gene transfer (HGT) (Figure 1B).
Notably, the annotation of virulence factors revealed that several virulence genes in the paocai-derived strains were orthologous to those of bacteria widely distributed in the soil and water (CVF254, CVF760, CVF677, and CVF197), whereas the virulence genes annotated in the human feces-derived strains were homologous to those of animal pathogenic bacteria (CVF282 and CVF516) (Figure 1B).
Differences in the Growth Profiles of the Six L. plantarum Strains Between the Paocai Diluent and Simulated Intestinal Juice Media
To verify how genomic changes influence strains’ phenotypes, fermentation assays were conducted in a diluted paocai solution and simulated intestinal juice, which mimicked the initial habitats of the strains. A correlation analysis was then performed to associate the phenotypic variations with the genomic differences.
Figure 2A shows the growth profiles of the six strains in the paocai diluent. The paocai-derived strains had a shorter stagnation period and a higher growth rate, indicating that they could adapt to the fermentation broth environment more rapidly than the human feces-derived strains (p < 0.05). A further correlation analysis showed the maximum CFUs during growth were correlated with genes related to nucleotide (F) and carbohydrate transport/metabolism (G), secondary metabolites biosynthesis/transport/catabolism (Q), transcription (K) and cell wall/membrane/envelope biogenesis (M) (Figure 2C).
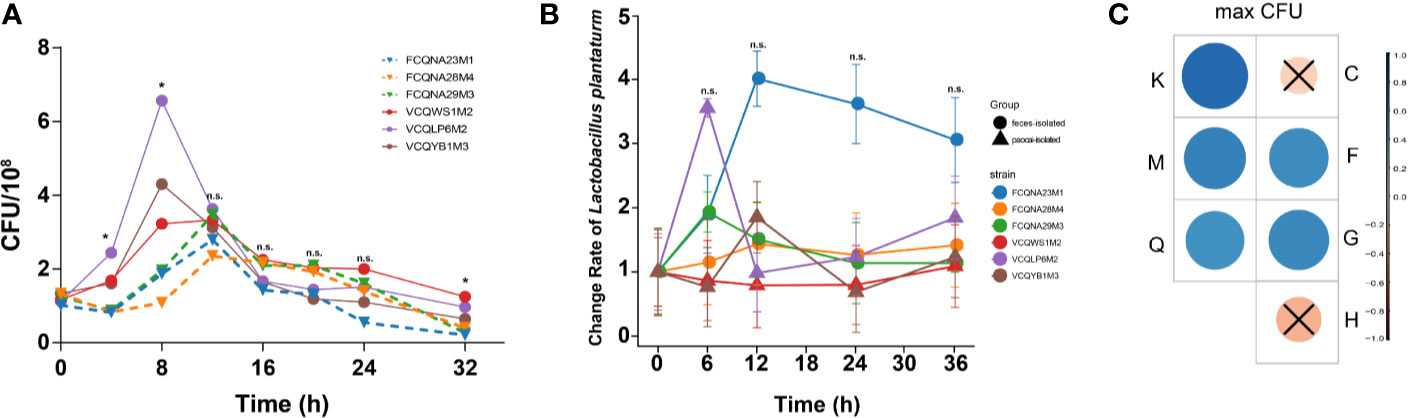
Figure 2 Growth profiles of L. plantarum strains in two media and their associations with genes. (A) Growth profiles of six L. plantarum strains in a paocai diluent. (B) Change rate of the relative abundance of six L. plantarum strains in the simulated intestinal juice. (C) Correlation grid plot for the correlation between maximum CFUs of L. plantarum strains in the simulated intestinal juice and functional genes. Red represents positive correlation; blue represents negative correlation. *represents p < 0.05; n.s. represents p > 0.05; × represents p > 0.05.
However, paocai- and human feces- derived L. plantarum strains did not exhibit differences in their rates of change in the relative abundance at any time point during growth in the simulated intestinal juice (p > 0.05, Figure 2B).
Diverse Interactions Between L. plantarum Strains from Diverse Niches and the Host Commensal Bacteria
16S rRNA gene sequencing was performed to reveal the changes in the fecal microbiota structure in the simulated intestinal juice. The result showed that the strains from the two habitats had different regulatory effects on the intestinal flora over time (Figure 3A).
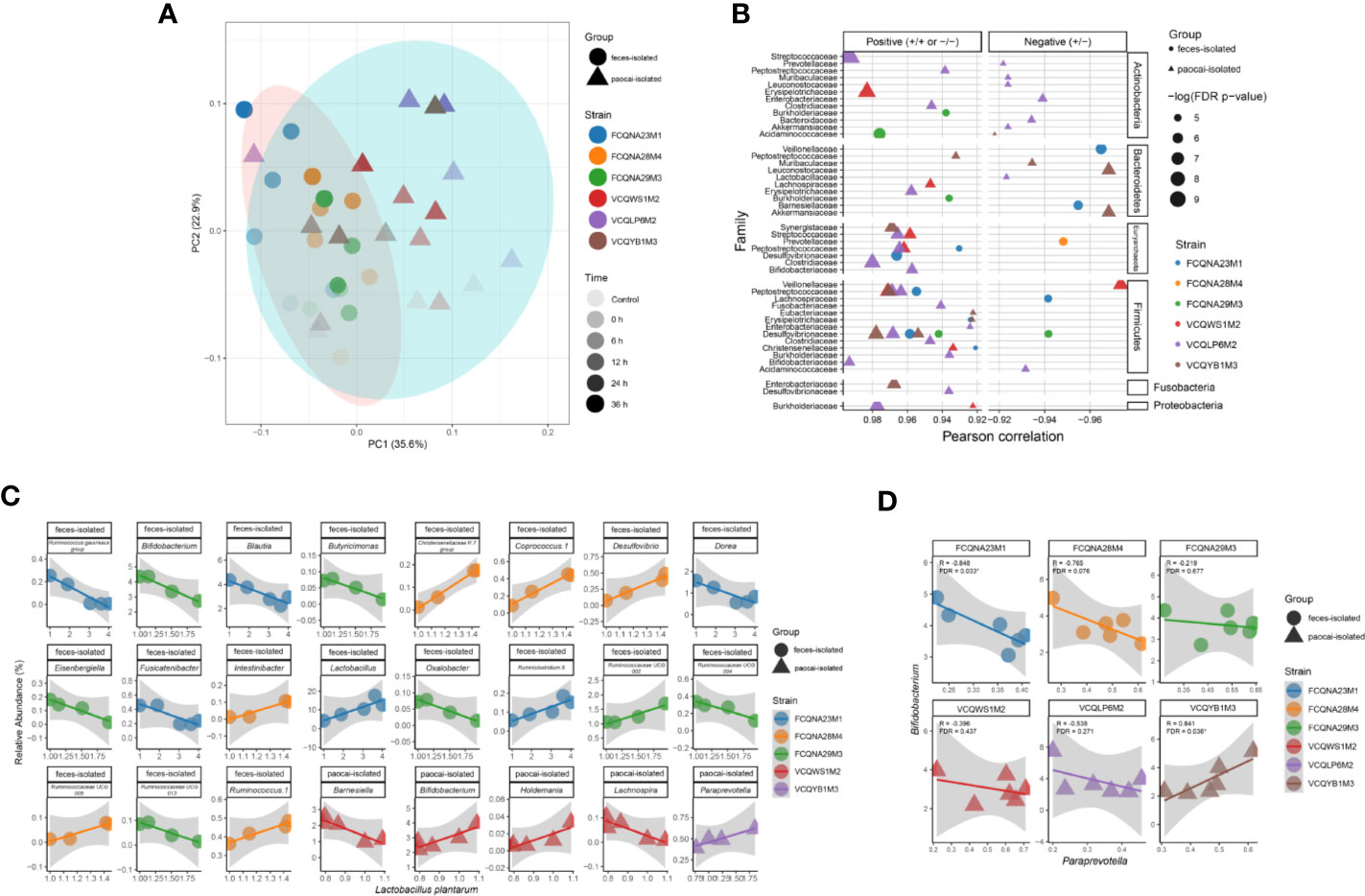
Figure 3 Diversity and correlation analyses between L. plantarum strains and microbiome in the simulated intestinal juice. (A) Variation of β diversity over time in the simulated intestinal juice. Correlation analysis between the relative abundance of each bacterial family (B) and between the genera Bifidobacterium and Paraprevotella (D) with the inoculation of different L. plantarum strains in the simulated intestinal juice. X and Y axes represent the relative abundances of the labelled genera. (C) Correlation analysis between L. plantarum strains and other genera in the simulated intestinal juice.
The Pearson’s correlation analysis of the relative abundances of gut microbiota at the family level showed that 44 couples of families were associated with bacterial family from different phyla in the medium inoculated with paocai-isolated strains, whereas in the medium inoculated with feces-isolated strains, only 13 families were demonstrated correlation with other families (FDR < 0.05) (Figure 3B). In particular, the medium inoculated with L. plantarum FCQNA28M4 (isolated from human feces), showed only one negative correlation between the Prevotellaceae family and one of the families in the Euryarchaeota phylum (R < 0, FDR < 0.05). In the medium inoculated with L. plantarum VCQLP6M2 (isolated from paocai), 26 couples of families were correlated with bacterial families belonging to different phyla (R > 0, FDR < 0.05).
Regarding the associations between L. plantarum and intestinal flora at the genus level, paocai- and feces- derived L. plantarum strains were correlated with five and 19 genera of the intestinal flora, respectively (FDR < 0.05). Among the 19 genera, 11 were negatively correlated with L. plantarum strains. In particular, L. plantarum VCQLP6M2 showed no significant association with any other bacterial genus (Figure 3C). Notably, the correlation between the relative abundances of L. plantarum FCQNA29M3 and Bifidobacterium was negative (R < 0, FDR < 0.05), whereas the correlation between the relative abundances of L. plantarum VCQWS1M2 and Bifidobacterium was positive (R > 0, FDR < 0.05) (Figure 3C).
Interestingly, L. plantarum strains in the two groups exhibited opposite influences on the associations between the Bifidobacterium and Paraprevotella genera (Figure 3D), such that the correlation between the relative abundances of these two genera was negative under the influence of the feces-isolated strain FCQNA23M1 (R < 0, FDR < 0.05), but was positive under the influence of the paocai-isolated strain VCQYB1M3 (R > 0, FDR < 0.05) (Figure 3D). These findings suggest that the adaptative evolution of strains in different niches plays a significant role in their interactions with the host gut flora.
Relationship Between the Functional Genes of L. plantarum and the Commensal Gut Microbiota
To explore whether the diverse effects of L. plantarum strains on the intestinal flora are related to their genomes, linear multiple regression models with different functional genes as variables were established in this study.
The model in which the relative abundances of the microbiome were set as variables exhibited a low accuracy (Figure 4A). The introduction of functional gene annotations (from the four databases) as variables significantly improved the prediction accuracy of the model (p < 0.05, Figure 4A). This result suggests that the influences of the introduced strains on the intestinal flora were significantly related to the functional genes of the strains.
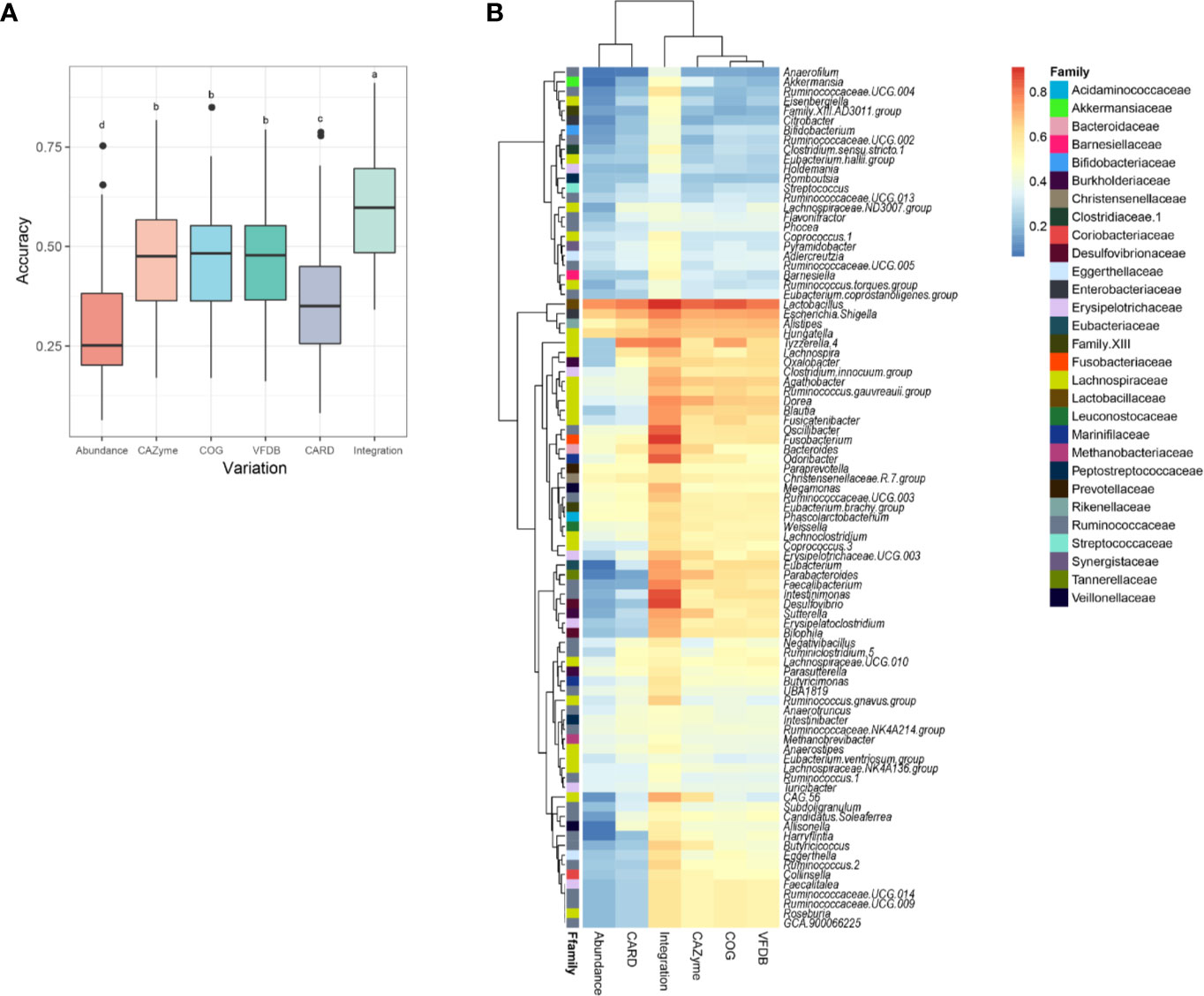
Figure 4 Correlation analysis between L. plantarum functional genomes and their influences on intestinal flora. (A) Boxplot for the accuracy scores of predicting the variation in microbiome using different functional genes. (B) Heatmap for the accuracy scores of predicting the variation in each genus using different functional genes. Labels of a, b, c, and d in the boxplot represent significant differences at p < 0.05.
The prediction accuracy of the model for each genus in the gut community is presented in a heatmap (Figure 4B). Different correlation coefficients exist between various genera of the host gut microbiome and functional genes of L. plantarum. The majority of bacteria belonging to the Lachnospiraceae family exhibited strong correlations with the L. plantarum genome (average R > 0.5). However, several genera, including Akkermansia (R = 0.486), Adlercreutzia (R = 0.475), and Holdemania (R = 0.458), were only weakly associated with the functional genes of L. plantarum. These results indicate that a supplement of L. plantarum can interact with the intestinal bacteria via multiple pathways due to the adaptive evolution of its diverse functional genes.
Discussion
Evolution involves sorting and variation. The former process involves two mechanisms, namely genetic drift and natural selection (Arber, 2000; Barrick et al., 2009; Gibson and Eyre-Walker, 2019). Natural selection can be further divided into positive selection, which promotes the spread of beneficial alleles, and negative selection, which hinders the spread of deleterious alleles (Barrick et al., 2009). These selections have strong influences on the phenotypes of an organism and should be taken into consideration when exploring and applying probiotics from various habitats.
In our previous study, 20 L. plantarum strains were isolated from different habitats, and their genomic variations in response to ecological constraints were identified by comparative genomics (Cen et al., 2020). However, the relationship between these genomic variations and the phenotypes remained unclear. Hence, in this study, six L. plantarum strains isolated from human feces and paocai were analyzed at the genomic and phenotypic levels to investigate the features of adaptive evolution in different habitats.
Previous studies have shown that only a few bacterial genes undergo SVs due to adaptive evolution, suggesting that evolution at the strain level is dominated by genetic drift, which occurs at a relatively slow rate (Gibson and Eyre-Walker, 2019; Vatanen et al., 2019). This pattern is consistent with our finding that strains isolated from the two different habitats had similar genome sizes and GC% (Figure 1B). Previous studies have also reported that differences in the abundances of functional genes can have significant effects on the phenotypes (Maldonado-Gómez et al., 2016; Hughes et al., 2020), and this is supported by our results (Figure 1B, Figures 2A, C). As the original habitats seem to play an important role in the variations of metabolism-related genes, this factor should be considered when exploring commercial probiotic strains.
Notably, the analysis of virulence factors in the two groups showed that several virulence genes in the paocai-derived strains were orthologous to those of bacteria widely found in soil and water, including katA of Pseudomonas aeruginosa (Chung et al., 2016), Staphylococcus aureus (Reiß et al., 2012) and Yersinia enterocolitica (Reuter et al., 2014), gtcA of Listeria monocytogenes (Promadej et al., 1999), fbpC of Escherichia coli (Blum et al., 2012) and Vibrio antiquaries (Hasan et al., 2015), and agrA of Staphylococcus aureus (Srivastava et al., 2014). However, virulence genes in the feces-derived strains were homologous to those of animal pathogenic bacteria, such as rmlC of Salmonella enterica (Graninger et al., 1999), Bordetella bronchiseptica (Park et al., 2012), Streptococcus pneumoniae (Ko et al., 2013), Mycobacterium tuberculosis (Norman et al., 2019) and Shigella boydii (Liu et al., 2008), and fagC of Corynebacterium pseudotuberculosis (Billington et al., 2002), Corynebacterium ulcerans (Trost et al., 2011), Corynebacterium kutscheri (Turnbull et al., 2019) and Corynebacterium cystitidis (Turnbull et al., 2019) (Figure 2B). This suggests that strains can obtain the virulence genes from the surrounding micro-environments through HGT, similar to the process by which bacteria acquire antibiotic-resistant genes (San Millan et al., 2016). Further investigation is warranted to uncover the mechanism by which virulence factors are transferred between organisms and the rate at which this transfer occurs. Considering the potential HGT of virulence factors, it is necessary to re-evaluate the safety of commonly recognized probiotics (Saarela, 2019; Sotoudegan et al., 2019).
The traditional Chinese pickled vegetable paocai is produced by fermentation without sterilization. The high salinity and acidity (average pH is 3.86) of paocai result in a relatively simple ecological environment dominated by the bacterial genera Lactobacillus, Pediococcus, Serratia, Stenotrophomonas, and Weissella (Liu et al., 2019). As a result, bacterial growth in the relatively low-diversity environment was strongly influenced by the chemical environment rather than the interaction between bacteria (Cao et al., 2017). Therefore, the strains adaptive to this kind of chemical environment had growth advantage when incubated in the medium with similar environment. The result in our study was consistent with this pattern (Figure 2A). This result could be attributable to the differences in metabolic-functional genes between the two groups of L. plantarum strains, especially those involved in carbohydrate (G) and coenzyme (H) transport/metabolism (Figure 1B, Figure 2C).
However, our in-vitro fermentation assays did not demonstrate significant associations between strains’ growth abilities and the adaptive evolution-triggered gene variations of the species (Figure 2B). Compared with paocai, the human colon is a sophisticated microsystem involving various types of bacteria-host cross-talks, and a weakly alkaline chemical environment containing trillions of microorganisms and diverse immune niches (James et al., 2020). In such a complex environment, bacterial growth is governed not only by bacterial metabolic genes, but also by the complex interactions between probiotics and the intestinal flora. Numerous mechanisms such as bacterial competition and mutualism govern these complex interactions (Bauer et al., 2018). Thus, our finding suggests that when developing new probiotic products, both the survival rates of the probiotic strains throughout the digestive tract and their growth profiles in the gut environment should be considered (Pham and Mohajeri, 2018).
The 16S rRNA gene sequencing data showed the complex relationships among the endogenous host gut bacteria under the influence of exogenous probiotics (Figures 3B–D). The comparative genomic analysis and phenotypic evaluation indicated that these relationships were possibly attributable to the different amounts of metabolites produced by strains from diverse initial habitats. For example, a previous study revealed that 5 μg/ml of exopolysaccharides (EPSs) isolated from Bifidobacterium longum suppressed lipopolysaccharide-induced cell growth inhibition, whereas 80 μg/ml of EPSs inhibited the growth of seven species of foodborne pathogenic bacteria (Wu et al., 2010). Furthermore, the data showed that only a few genera were affected by the probiotics (Figure 3C), and that the relative abundances of the other genera changed possibly in response to the metabolic cascades of the gut microbiota, which were strongly affected by the intestinal microenvironment (Coyte and Rakoff-Nahoum, 2019; Zmora et al., 2019). Similar to our findings, a previous study indicated that supplementation with L. plantarum ZLP001 increased the abundance of butyrate-producing bacteria, which in turn improved the butyrate content in the intestinal environment, strengthened the epithelial defense functions, and modulated the gut microbiota. Whether gene variations due to niche-specific evolution affect gut microbial cross-talks directly or change the metabolic cascades of the gut microbiota by regulating the intestinal chemical environment remains unclear. Further studies are needed to identify the relationships between genomic variations due to adaptive evolution and their effects on gut microbial genera, as this information can assist the design of probiotic preparations for the modulation of specific gut microbial species.
Overall, our study identified niche-specific adaptive evolution in the L. plantarum genome and provided an insight into how the SVs in functional genes affected the fermentation abilities of L. plantarum strains and their interactions with commensal microbes. This finding provides a promising outlook regarding the mining of new probiotic niches.
Data Availability Statement
The datasets presented in this study can be found in online repositories. The names of the repository/repositories and accession number(s) can be found here: https://www.ncbi.nlm.nih.gov/, SRR12559883; https://www.ncbi.nlm.nih.gov/, SRR12559881; https://www.ncbi.nlm.nih.gov/, SRR12559880; https://www.ncbi.nlm.nih.gov/, SRR12559736; https://www.ncbi.nlm.nih.gov/, SRR12559737; https://www.ncbi.nlm.nih.gov/, SRR12559735.
Author Contributions
QP: Methodology, software, formal analysis, visualization, writing—original draft. SC: Methodology, software, formal analysis, visualization. LY, FT, JZ, HZ: Validation, investigation. QZ: Conceptualization, writing—review and editing, supervision, funding acquisition. WC: Project administration, funding acquisition. All authors contributed to the article and approved the submitted version.
Funding
This work was supported by the National Natural Science Foundation of China Program [No. 31871773 and No. 31820103010]; Projects of Innovation and Development Pillar Program for Key Industries in Southern Xinjiang of Xinjiang Production and Construction Corps [2018DB002]; National First-Class Discipline Program of Food Science and Technology [JUFSTR20180102]; the BBSRC Newton Fund Joint Centre Award; and Collaborative Innovation Center of Food Safety and Quality Control in Jiangsu Province.
Conflict of Interest
The authors declare that the research was conducted in the absence of any commercial or financial relationships that could be construed as a potential conflict of interest.
References
Altschul S. F., Gish W., Miller W., Myers E. W., Lipman D. J. (1990). Basic local alignment search tool. J. Mol. Biol. 215 (3), 403–410. doi: 10.1016/S0022-2836(05)80360-2
Arber W. (2000). Genetic variation: molecular mechanisms and impact on microbial evolution. FEMS Microbiol Rev. 24 (1), 1–7. doi: 10.1016/S0168-6445(99)00032-7
Barrick J. E., Yu D. S., Yoon S. H., Jeong H., Oh T. K., Schneider D., et al. (2009). Genome evolution and adaptation in a long-term experiment with Escherichia coli. Nature 461 (7268), 1243–1247. doi: 10.1038/nature08480
Bauer M. A., Kainz K., Carmona-Gutierrez D., Madeo F. (2018). Microbial wars: Competition in ecological niches and within the microbiome. Microb Cell 5 (5), 215–219. doi: 10.15698/mic2018.05.628
Billington S. J., Esmay P. A., Songer J. G., Jost B. H. (2002). Identification and role in virulence of putative iron acquisition genes from Corynebacterium pseudotuberculosis. FEMS Microbiol Lett. 208 (1), 41–45. doi: 10.1111/j.1574-6968.2002.tb11058.x
Blum S., Sela N., Heller E. D., Sela S., Leitner G. (2012). Genome analysis of bovine-mastitis-associated Escherichia coli O32:H37 strain P4. J. Bacteriol 194 (14), 3732–3732. doi: 10.1128/JB.00535-12
Cantarel B. L., Coutinho P. M., Rancurel C., Bernard T., Lombard V., Henrissat B. (2009). The Carbohydrate-Active EnZymes database (CAZy): an expert resource for Glycogenomics. Nucleic Acids Res. 37 (suppl_1), D233–D238. doi: 10.1093/nar/gkn663
Cao J., Yang J., Hou Q., Xu H., Zheng Y., Zhang H., et al. (2017). Assessment of bacterial profiles in aged, home-made Sichuan paocai brine with varying titratable acidity by PacBio SMRT sequencing technology. Food Control 78, 14–23. doi: 10.1016/j.foodcont.2017.02.006
Cen S., Yin R., Mao B., Zhao J., Zhang H., Zhai Q., et al. (2020). Comparative genomics shows niche-specific variations of Lactobacillus plantarum strains isolated from human, Drosophila melanogaster, vegetable and dairy sources. Food Biosci. 35, 100581. doi: 10.1016/j.fbio.2020.100581
Chen L., Yang J., Yu J., Yao Z., Sun L., Shen Y., et al. (2005). VFDB: a reference database for bacterial virulence factors. Nucleic Acids Res. 33 (suppl_1), D325–D328. doi: 10.1093/nar/gki008
Chung I.-Y., Kim B.-o., Jang H.-J., Cho Y.-H. (2016). Dual promoters of the major catalase (KatA) govern distinct survival strategies of Pseudomonas aeruginosa. Sci. Rep. 6 (1), 31185. doi: 10.1038/srep31185
Coyte K. Z., Rakoff-Nahoum S. (2019). Understanding Competition and cooperation within the mammalian gut microbiome. Curr. Biol. 29 (11), R538–R544. doi: 10.1016/j.cub.2019.04.017
Fang S., Zhang Y., Shi X., Zheng H., Li S., Zhang Y., et al. (2020). Identification of male-specific SNP markers and development of PCR-based genetic sex identification technique in crucifix crab (Charybdis feriatus) with implication of an XX/XY sex determination system. Genomics 112 (1), 404–411. doi: 10.1016/j.ygeno.2019.03.003
Fischer S., Brunk B. P., Chen F., Gao X., Harb O. S., Iodice J. B., et al. (2011). Using OrthoMCL to assign proteins to OrthoMCL-DB groups or to cluster proteomes into new ortholog groups. Curr. Protoc. Bioinf. 35 (1), 6.12.11–16.12.19. doi: 10.1002/0471250953.bi0612s35
Gibson B., Eyre-Walker A. (2019). Investigating Evolutionary Rate Variation in Bacteria. Mol. Biol. Evol. 87 (9), 317–326. doi: 10.1007/s00239-019-09912-5
Graninger M., Nidetzky B., Heinrichs D. E., Whitfield C., Messner P. (1999). Characterization of dTDP-4-dehydrorhamnose 3,5-epimerase and dTDP-4-dehydrorhamnose reductase, required for dTDP-L-rhamnose biosynthesis in Salmonella enterica serovar Typhimurium LT2. J. Biol. Chem. 274 (35), 25069–25077. doi: 10.1074/jbc.274.35.25069
Hasan N. A., Grim C. J., Lipp E. K., Rivera I. N. G., Chun J., Haley B. J., et al. (2015). Deep-sea hydrothermal vent bacteria related to human pathogenic Vibrio species. Proc. Natl. Acad. Sci. U. S. A. 112 (21), E2813. doi: 10.1073/pnas.1503928112
Hughes D. A., Bacigalupe R., Wang J., Rühlemann M. C., Tito R. Y., Falony G., et al. (2020). Genome-wide associations of human gut microbiome variation and implications for causal inference analyses. Nat. Microbiol. 5 (9), 1079–1087. doi: 10.1038/s41564-020-0743-8
James K. R., Gomes T., Elmentaite R., Kumar N., Gulliver E. L., King H. W., et al. (2020). Distinct microbial and immune niches of the human colon. Nat. Immunol. 21 (3), 343–353. doi: 10.1038/s41590-020-0602-z
Jia B., Raphenya A. R., Alcock B., Waglechner N., Guo P., Tsang K. K., et al. (2017). CARD 2017: expansion and model-centric curation of the comprehensive antibiotic resistance database. Nucleic Acids Res. 45 (D1), D566–D573. doi: 10.1093/nar/gkw1004
Johnson A. J., Vangay P., Al-Ghalith G. A., Hillmann B. M., Ward T. L., Shields-Cutler R. R., et al. (2019). Daily sampling reveals personalized diet-microbiome associations in humans. Cell Host Microbe 25 (6), 789–802.e785. doi: 10.1016/j.chom.2019.05.005
Katoh K., Standley D. M. (2013). MAFFT multiple sequence alignment software Version 7: Improvements in performance and usability. Mol. Biol. Evol. 30 (4), 772–780. doi: 10.1093/molbev/mst010
Kelleher P., Bottacini F., Mahony J., Kilcawley K. N., van Sinderen D. (2017). Comparative and functional genomics of the Lactococcus lactis taxon; insights into evolution and niche adaptation. BMC Genom. 18, 267. doi: 10.1186/s12864-017-3650-5
Klocke M., Mundt K., Idler C., McEniry J., O’Kiely P., Barth S. (2006). Monitoring Lactobacillus plantarum in grass silages with the aid of 16S rDNA-based quantitative real-time PCR assays. Syst. Appl. Microbiol. 29 (1), 49–58. doi: 10.1016/j.syapm.2005.06.001
Ko K. S., Baek J. Y., Song J.-H. (2013). Capsular Gene Sequences and Genotypes of “Serotype 6E” Streptococcus pneumoniae Isolates. J. Clin. Microbiol. 51 (10), 3395–3399. doi: 10.1128/JCM.01645-13
Lau J. T., Whelan F. J., Herath I., Lee C. H., Collins S. M., Bercik P., et al. (2016). Capturing the diversity of the human gut microbiota through culture-enriched molecular profiling. Genome Med. 8 (1), 72. doi: 10.1186/s13073-016-0327-7
Lee J. -Y., Han G. G., Kim E. B., Choi Y. -J. (2017). Comparative genomics of Lactobacillus salivarius strains focusing on their host adaptation. Microbiol Res 205, 48–58. doi: 10.1016/j.micres.2017.08.008
Liu B., Knirel Y. A., Feng L., Perepelov A. V., Senchenkova S., Wang Q., et al. (2008). Structure and genetics of Shigella O antigens. FEMS Microbiol Rev. 32 (4), 627–653. doi: 10.1111/j.1574-6976.2008.00114.x
Liu Z., Peng Z., Huang T., Xiao Y., Li J., Xie M., et al. (2019). Comparison of bacterial diversity in traditionally homemade paocai and Chinese spicy cabbage. Food Microbiol 83, 141–149. doi: 10.1016/j.fm.2019.02.012
Maldonado-Gómez M. X., Martínez I., Bottacini F., O’Callaghan A., Ventura M., van Sinderen D., et al. (2016). Stable engraftment of Bifidobacterium longum AH1206 in the human gut depends on individualized features of the resident microbiome. Cell Host Microbe 20 (4), 515–526. doi: 10.1016/j.chom.2016.09.001
Martino M. E., Bayjanov J. R., Caffrey B. E., Wels M., Joncour P., Hughes S., et al. (2016). Nomadic lifestyle of Lactobacillus plantarum revealed by comparative genomics of 54 strains isolated from different habitats. Environ. Microbiol. 18 (12), 4974–4989. doi: 10.1111/1462-2920.13455
Minekus M., Smeets-Peeters M., Bernalier A., Marol-Bonnin S., Havenaar R., Marteau P., et al. (1999). A computer-controlled system to simulate conditions of the large intestine with peristaltic mixing, water absorption and absorption of fermentation products. Appl. Microbiol Biotechnol. 53 (1), 108–114. doi: 10.1007/s002530051622
Norman A., Folkvardsen D. B., Overballe-Petersen S., Lillebaek T. (2019). Complete Genome Sequence of Mycobacterium tuberculosis DKC2, the Predominant Danish Outbreak Strain. Microbiol. Resour. Announc. 8 (4), e01554–e01518. doi: 10.1128/MRA.01554-18
Papizadeh M., Rohani M., Nahrevanian H., Javadi A., Pourshafie M. R. (2017). Probiotic characters of Bifidobacterium and Lactobacillus are a result of the ongoing gene acquisition and genome minimization evolutionary trends. Microb. Pathog. 111, 118–131. doi: 10.1016/j.micpath.2017.08.021
Park J., Zhang Y., Buboltz A. M., Zhang X., Schuster S. C., Ahuja U., et al. (2012). Comparative genomics of the classical Bordetella subspecies: the evolution and exchange of virulence-associated diversity amongst closely related pathogens. BMC Genom. 13 (1), 545. doi: 10.1186/1471-2164-13-545
Petrova M. I., Macklaim J. M., Wuyts S., Verhoeven T., Vanderleyden J., Gloor G. B., et al. (2018). Comparative genomic and phenotypic analysis of the vaginal probiotic Lactobacillus rhamnosus GR-1. Front. Microbiol. 9, 1278. doi: 10.3389/fmicb.2018.01278
Pham V. T., Mohajeri M. H. (2018). The application of in vitro human intestinal models on the screening and development of pre- and probiotics. Benef. Microbes 9 (5), 725–742. doi: 10.3920/bm2017.0164
Promadej N., Fiedler F., Cossart P., Dramsi S., Kathariou S. (1999). Cell wall teichoic acid glycosylation in Listeria monocytogenes serotype 4b requires gtcA, a novel, serogroup-specific gene. J. Bacteriol. 181 (2), 418. doi: 10.1128/JB.181.2.418-425.1999
Reiß S., Pané-Farré J., Fuchs S., François P., Liebeke M., Schrenzel J., et al. (2012). Global Analysis of the Staphylococcus aureus Response to Mupirocin. Antimicrob. Agents Chemother. 56 (2), 787. doi: 10.1128/AAC.05363-11
Reuter S., Connor T. R., Barquist L., Walker D., Feltwell T., Harris S. R., et al. (2014). Parallel independent evolution of pathogenicity within the genus Yersinia. Proc. Natl. Acad. Sci. U. S. A. 111 (18), 6768. doi: 10.1073/pnas.1317161111
Saarela M. H. (2019). Safety aspects of next generation probiotics. Curr. Opin. Food Sci. 30, 8–13. doi: 10.1016/j.cofs.2018.09.001
Saitou N., Nei M. (1987). The neighbor-joining method: a new method for reconstructing phylogenetic trees. Mol. Biol. Evol. 4 (4), 406–425. doi: 10.1093/oxfordjournals.molbev.a040454
San Millan A., Escudero J. A., Gifford D. R., Mazel D., MacLean R. C. (2016). Multicopy plasmids potentiate the evolution of antibiotic resistance in bacteria. Nat. Ecol. Evol. 1 (1), 0010. doi: 10.1038/s41559-016-0010
Seddik H. A., Bendali F., Gancel F., Fliss I., Spano G., Drider D. (2017). Lactobacillus plantarum and its probiotic and food potentialities. Probiotics Antimicrob. Proteins 9 (2), 111–122. doi: 10.1007/s12602-017-9264-z
Song Y., He Q., Zhang J., Qiao J., Xu H., Zhong Z., et al. (2018). Genomic Variations in Probiotic Lactobacillus plantarum P-8 in the Human and Rat Gut. Front. Microbiol. 9 (893), 893. doi: 10.3389/fmicb.2018.00893
Sotoudegan F., Daniali M., Hassani S., Nikfar S., Abdollahi M. (2019). Reappraisal of probiotics’ safety in human. Food Chem. Toxicol. 129, 22–29. doi: 10.1016/j.fct.2019.04.032
Srivastava S. K., Rajasree K., Fasim A., Arakere G., Gopal B. (2014). Influence of the AgrC-AgrA complex on the response time of Staphylococcus aureus quorum sensing. J. Bacteriol 196 (15), 2876–2888. doi: 10.1128/JB.01530-14
Stefanovic E., McAuliffe O. (2018). Comparative genomic and metabolic analysis of three Lactobacillus paracasei cheese isolates reveals considerable genomic differences in strains from the same niche. BMC Genom. 19, 205. doi: 10.1186/s12864-018-4586-0
Sukjung C., Gwi-Deuk J., Jongbin P., Inhwan Y., Eun Bae K. (2018). Pan-Genomics of Lactobacillus plantarum Revealed Group-Specific Genomic Profiles without Habitat Association. J. Microbiol. Biotechnol. 28 (8), 1352–1359. doi: 10.4014/jmb.1803.03029
Tatusov R. L., Galperin M. Y., Natale D. A., Koonin E. V. (2000). The COG database: a tool for genome-scale analysis of protein functions and evolution. Nucleic Acids Res. 28 (1), 33–36. doi: 10.1093/nar/28.1.33
Trost E., Al-Dilaimi A., Papavasiliou P., Schneider J., Viehoever P., Burkovski A., et al. (2011). Comparative analysis of two complete Corynebacterium ulcerans genomes and detection of candidate virulence factors. BMC Genom 12 (1), 383. doi: 10.1186/1471-2164-12-383
Turnbull J. D., Russell J. E., Fazal M.-A., Grayson N. E., Deheer-Graham A., Oliver K., et al. (2019). Whole-Genome Sequences of Five Strains of Kocuria rosea, NCTC2676, NCTC7514, NCTC7512, NCTC7528, and NCTC7511. Microbiol. Resour. Announc. 8 (44), e00256–e00219. doi: 10.1128/MRA.00256-19
Valeriano V. D. V., Oh J. K., Bagon B. B., Kim H., Kang D.-K. (2019). Comparative genomic analysis of Lactobacillus mucosae LM1 identifies potential niche-specific genes and pathways for gastrointestinal adaptation. Genomics 111 (1), 24–33. doi: 10.1016/j.ygeno.2017.12.009
Vatanen T., Plichta D. R., Somani J., Münch P. C., Arthur T. D., Hall A. B., et al. (2019). Genomic variation and strain-specific functional adaptation in the human gut microbiome during early life. Nat. Microbiol. 4 (3), 470–479. doi: 10.1038/s41564-018-0321-5
Wellenreuther M., Mérot C., Berdan E., Bernatchez L. (2019). Going beyond SNPs: The role of structural genomic variants in adaptive evolution and species diversification. Mol. Ecol. 28 (6), 1203–1209. doi: 10.1111/mec.15066
Wu M., Pan T., Wu Y., Chang S., Chang M., Hu C. (2010). Exopolysaccharide activities from probiotic Bifidobacterium: Immunomodulatory effects (on J774A.1 macrophages) and antimicrobial properties. Int. J. Food Microbiol. 144 (1), 104–110. doi: 10.1016/j.ijfoodmicro.2010.09.003
Zhai Q., Cen S., Jiang J., Zhao J., Zhang H., Chen W. (2019). Disturbance of trace element and gut microbiota profiles as indicators of autism spectrum disorder: A pilot study of Chinese children. Environ. Res. 171, 501–509. doi: 10.1016/j.envres.2019.01.060
Zhang J. (2008). Positive selection, not negative selection, in the pseudogenization of rcsA in Yersinia pestis. Proc. Natl. Acad. Sci. U. S. A. 105 (42), E69. doi: 10.1073/pnas.0806419105
Zhao S., Lieberman T. D., Poyet M., Kauffman K. M., Gibbons S. M., Groussin M., et al. (2019). Adaptive evolution within gut microbiomes of healthy people. Cell Host Microbe 25 (5), 656–667.e658. doi: 10.1016/j.chom.2019.03.007
Keywords: comparative genomics, Lactobacillus plantarum, niche-specific adaptive evolution, microbiome interaction, host physiology
Citation: Pan Q, Cen S, Yu L, Tian F, Zhao J, Zhang H, Chen W and Zhai Q (2021) Niche-Specific Adaptive Evolution of Lactobacillus plantarum Strains Isolated From Human Feces and Paocai. Front. Cell. Infect. Microbiol. 10:615876. doi: 10.3389/fcimb.2020.615876
Received: 10 October 2020; Accepted: 19 November 2020;
Published: 07 January 2021.
Edited by:
Andrew T. Gewirtz, Georgia State University, United StatesReviewed by:
Huaxi Yi, Ocean University of China, ChinaBailiang Li, Northeast Agricultural University, China
Copyright © 2021 Pan, Cen, Yu, Tian, Zhao, Zhang, Chen and Zhai. This is an open-access article distributed under the terms of the Creative Commons Attribution License (CC BY). The use, distribution or reproduction in other forums is permitted, provided the original author(s) and the copyright owner(s) are credited and that the original publication in this journal is cited, in accordance with accepted academic practice. No use, distribution or reproduction is permitted which does not comply with these terms.
*Correspondence: Qixiao Zhai, emhhaXFpeGlhb0BzaW5hLmNvbQ==; emhhaXFpeGlhb0BqaWFuZ25hbi5lZHUuY24=
†These authors have contributed equally to this work