- 1Department of Engineering Science, Faculty of Innovation Engineering, Macau University of Science and Technology, Taipa, Macao SAR, China
- 2Respiratory Disease AI Laboratory on Epidemic and Medical Big Data Instrument Applications, Faculty of Innovation Engineering, Macau University of Science and Technology, Macao, Macao SAR, China
- 3Guangzhou Key Laboratory for Clinical Rapid Diagnosis and Early Warning of Infectious Diseases, KingMed School of Laboratory Medicine, Guangzhou Medical University, Guangzhou, China
- 4State Key Laboratory of Respiratory Disease, National Clinical Research Center for Respiratory Disease, Guangzhou Institute of Respiratory Health, The First Affiliated Hospital of Guangzhou Medical University, Guangzhou, Guangdong, China
- 5Guangzhou Laboratory, Guangzhou, Guangdong, China
Background: Influenza A virus have a distinctive ability to exacerbate SARS-CoV-2 infection proven by in vitro studies. Furthermore, clinical evidence suggests that co-infection with COVID-19 and influenza not only increases mortality but also prolongs the hospitalization of patients. COVID-19 is in a small-scale recurrent epidemic, increasing the likelihood of co-epidemic with seasonal influenza. The impact of co-infection with influenza virus and SARS-CoV-2 on the population remains unstudied.
Method: Here, we developed an age-specific compartmental model to simulate the co-circulation of COVID-19 and influenza and estimate the number of co-infected patients under different scenarios of prevalent virus type and vaccine coverage. To decrease the risk of the population developing severity, we investigated the minimum coverage required for the COVID-19 vaccine in conjunction with the influenza vaccine, particularly during co-epidemic seasons.
Result: Compared to the single epidemic, the transmission of the SARS-CoV-2 exhibits a lower trend and a delayed peak when co-epidemic with influenza. Number of co-infection cases is higher when SARS-CoV-2 co-epidemic with Influenza A virus than that with Influenza B virus. The number of co-infected cases increases as SARS-CoV-2 becomes more transmissible. As the proportion of individuals vaccinated with the COVID-19 vaccine and influenza vaccines increases, the peak number of co-infected severe illnesses and the number of severe illness cases decreases and the peak time is delayed, especially for those >60 years old.
Conclusion: To minimize the number of severe illnesses arising from co-infection of influenza and COVID-19, in conjunction vaccinations in the population are important, especially priority for the elderly.
1 Introduction
With the relaxation of COVID-19 policies worldwide, the management of COVID-19 has now entered a phase of normalization. The previously implemented Public Health and Social Measures (PHSM) during the COVID-19 pandemic also had a preventive effect on the influenza epidemic, which may result in an immune gap among the population (Ali et al., 2022; Yang et al., 2022). The immune evasion ability of SARS-CoV-2 is well recognized by encoding seven accessors (Zandi et al., 2022), and ORF9c and ORF10 play key roles (Zandi, 2022). Besides, highly variable characteristics of the influenza virus led to continuous antigen drift and change of susceptible populations, causing repeated global influenza epidemics Therefore, the co-epidemics of COVID-19 and influenza in the coming years are inevitable.
Since the early stages of the pandemic, several studies have emerged mixed infections involving SARS-CoV-2 and influenza (Yang et al., 2022; Rezaee et al., 2023). A meta-analysis indicated that the prevalence of influenza co-infection among COVID-19 patients is 0.8%, with a frequency of 4.5% in Asia and 0.4% in the Americas (Kim et al., 2020). In the initial outbreak of COVID-19 in Wuhan, co-infections of SARS-CoV-2 and influenza viruses were highly common, with a shift from IBV to IAV in terms of the co-infection viral types (Yue et al., 2020).
Currently, there is a large amount of clinical evidence indicating that co-infection with COVID-19 and influenza increases the risk of patient mortality and prolongs hospitalization (Dadashi et al., 2021; Garg et al., 2022; Cong et al., 2022; Fahim et al., 2022; Musuuza et al., 2021). In patients with co-infections, the risk of death is around twice as high as in those with COVID-19 infection alone (Stowe et al., 2021). These findings are also supported by basic experiments, as laboratory studies have confirmed the synergistic effect of influenza on the infection of SARS-CoV-2. Research by Bai et al. suggests that pre-infection with influenza A virus (IAV) significantly enhances the infection of SARS-CoV-2 in various cell types (Swets et al., 2022). Furthermore, co-infection with SARS-CoV-2 and IAV noticeably reduces the levels of total IgG and neutralizing antibodies against both IAV and SARS-CoV-2 (Kim et al., 2022).
Recent studies have shown that there is a certain degree of cross-protection between COVID-19 and influenza, and vaccines are effective in preventing co-infections (Alosaimi et al., 2021; Varshney et al., 2023). Influenza vaccination has been proven to reduce the risk of SARS-CoV-2 infection (Marín-Hernández et al., 2021; Wang et al., 2021). For example, Wilcox et al. found a significant correlation between receiving influenza vaccination and a decrease in hospitalization or all-cause mortality, with a 24% reduction in all-cause mortality (adjusted odds ratio: 0.76, 95% confidence interval: 0.64-0.90) (Wilcox et al., 2021). However, studies have revealed that older adults and high-risk individuals with chronic diseases have a lower willingness to receive influenza vaccination (Katsiroumpa et al., 2023). Early research has shown a link between influenza vaccination among older adults and a decrease in COVID-19 hospitalization rates and mortality (Candelli et al., 2021; Yang et al., 2021). Therefore, promoting vaccine uptake among specific populations is warranted.
Regarding the issue mentioned above, the World Health Organization (https://www.who.int/tools/flunet) has started publishing data on the positivity rates of influenza and COVID-19. Multiple countries, including the United States, Israel, and India, have advised paying special attention to the issue of co-infection. So far, the world has experienced three waves of COVID-19, and the Omicron variant is predominant in the fourth wave (Gao et al., 2022). SARS-CoV-2 is constantly evolving (Bedford et al., 2015), and the Influenza virus is experiencing interchangeable strain circulation. The impact of such diverse co-pandemics on population health is still unknown.、
Current models of co-infection between COVID-19 and influenza (Du et al., 2022; Pinky and Dobrovolny, 2022; Ojo et al., 2022) have not considered the combination of types or the influence of vaccination and cross-immunity. Therefore, optimizing models to address these research gaps would be crucial for accurately predicting the severity of co-infection and for the development of effective prevention and control strategies.
2 Materials and methods
To quantify the mutual influence of the two viruses, we developed a model to illustrate how the interactions of the viruses at the individual level affect the epidemiological situation at the population level. This modeling study relies on publicly available aggregated data only. As such, institutional review and informed consent are waived by the Institutional Review Board of the first affiliated hospital of Guangzhou Medical University (Guangzhou, China)
2.1 Model construction
Based on epidemiological data, mathematical models can be used to estimate the impact of interactions on transmission and help develop prevention and control strategies (Chang et al., 2021). Considering that statistical method could not formalize the transmission process or biological mechanism, and the interaction mechanism cannot be determined nor the strength of interaction quantified. However, compartment model could dissect the cause and effect of the different components (Opatowski et al., 2018). Therefore, we developed an age-specific compartment model to illustrate the transition patterns of different population groups during a simultaneous outbreak of influenza and COVID-19. The model diagram is given in Figure 1.
In this model, the population is categorized into susceptible (S), exposed (E), infectious (I), recovered (R), and severe cases (H). Within the susceptible group (S), we consider the vaccine effectiveness (VE) against infection, subsequently dividing them into those protected by the influenza vaccine (Svf), those protected by the COVID-19 vaccine (Svc), and those unprotected by any vaccine (Suv). The probability of infection varies among these susceptible groups, influenced by two vaccine protection parameters (ef, ec), representing the protection rate of the influenza vaccine against influenza infection (ef) and the protection rate of the COVID-19 vaccine against COVID-19 infection (ec). These susceptible individuals may contract either influenza, COVID-19, or both, transitioning to the exposed group (E) at infection rates (λf, λc). Considering that individuals can be solely infected or co-infected, the exposed population (E) is subdivided into those exposed to influenza (Ef), those exposed to COVID-19 (Ec), and those co-exposed to both (Ecf). For those exposed solely to either influenza or COVID-19 (Ef, Ec), they transition to the infectious group (If, Ic) after an incubation period (δf, δc). As for the co-exposed group (Ecf), they evolve into two infectious states: those infectious for influenza but exposed to COVID-19 (IfEc) and those infectious for COVID-19 but exposed to influenza (IcEf), subsequently becoming co-infected with both (Ifc). Considering the pre-infection may enhance the susceptibility of the other virus, we have introduced two key parameters, represent the change in susceptibility to SARS-CoV-2 in patients with influenza virus infection, and represent the change in susceptibility to influenza virus in patients with SARS-CoV-2 infection. Infectious individuals (If, Ic, Ifc) will transit to the recovered group (Rf, Rc, Rfc) over a recovery period (ωf, ωc, ωfc). However, given the possibility of disease exacerbation, some will progress to severe cases (Hf, Hc, Hfc) with transition probabilities μf, μc, μfc, representing severity rates for influenza, COVID-19, and co-infections respectively. We also account for vaccine-mediated protection against severity, incorporating three parameters: the protection rate of the vaccine against severe influenza (βf), against severe COVID-19 (βc), and severe co-infection (βfc). As our model does not consider mortality, these severe cases will transition to the recovered group (Rf, Rc, Rfc) over the recovery period (γf, γc, γfc). Lastly, given the realistic scenario where immunity wanes leading to potential re-infections, those recovered will gradually lose their immunity and revert to the susceptible state (S) with waning immunity probabilities of σf, σc, σfc.
The model equations are as follows:
2.2 Model parameters
The model parameters mainly include the virus characters, as well as parameters related to the severity and recovery rates. Viral characters include the basic reproduction number and incubation period. Parameters related to severity consider the vaccination rates and are effective at preventing severe disease in both young and elderly populations. The epidemiological parameters are presented in Table 1.
2.3 Vaccination simulation
To capture the difference in VE against infection across age group groups, we divided the population (S) based on their vaccination status into protected by the COVID-19 vaccine (Svc), protected by the influenza vaccine (Svf), and unprotected by any vaccine (Suc). To estimate the number of populations protected by a vaccine, it is necessary first to identify the number of people vaccinated and then also to consider the effectiveness of the vaccine. Therefore, we introduce the following parameters: vaccination rate v and vaccine effectiveness against infection e.
For example, to estimate the number of populations protected by the influenza vaccine (Svf), we consider the proportion of the population vaccinated (vf) and the vaccine efficacy (ef), resulting in the following formula for Svf:
Similarly, the number of susceptible populations protected by the COVID-19 vaccine:
The model also incorporates the VE against severe cases. We consider the post-vaccination severe rate μ to be the remaining risk of developing severe illness excluding vaccine protection based on severe rate φ. The remaining risk of developing severe illness could be presented as “1 - β”.
Therefor μ is calculated as:
2.4 Sensitivity analysis
Our research employed PRCC to examine the relative impact of specific parameters on the simulation results in three distinct scenarios: influenza, COVID-19, and co-infection of influenza and COVID-19. The Partial Rank Correlation Coefficient (PRCC) serves as an essential sensitivity analysis tool, quantifying the influence of marginal changes in input parameters on the outputs of the Susceptible-Exposed-Infectious-Recovered (SEIR) model. A perfect linear relationship between the ranks yields a rank correlation coefficient of +1 (or -1 for a negative relationship) and no linear relationship between the ranks yields a rank correlation coefficient of 0. Partial rank correlation is the correlation between two variables after removing the effect of one or more additional variables.
3 Result
3.1 Co-infection of influenza and SARS-CoV-2
Due to the variety of influenza types and SARS-CoV-2 variants prevalent in different countries or regions globally, we have chosen the major influenza types (IAV, IBV) and SARS-CoV-2 variants (DELTA, BA.5, and XBB) for analysis. To elucidate the interactions between different types of viruses, we modeled six co-epidemic scenarios (Figure 2). Additionally, this study examines the impact of co-epidemic on SARS-CoV-2 spread, with the single epidemic in comparison, independently with identical initial infection and immune conditions.
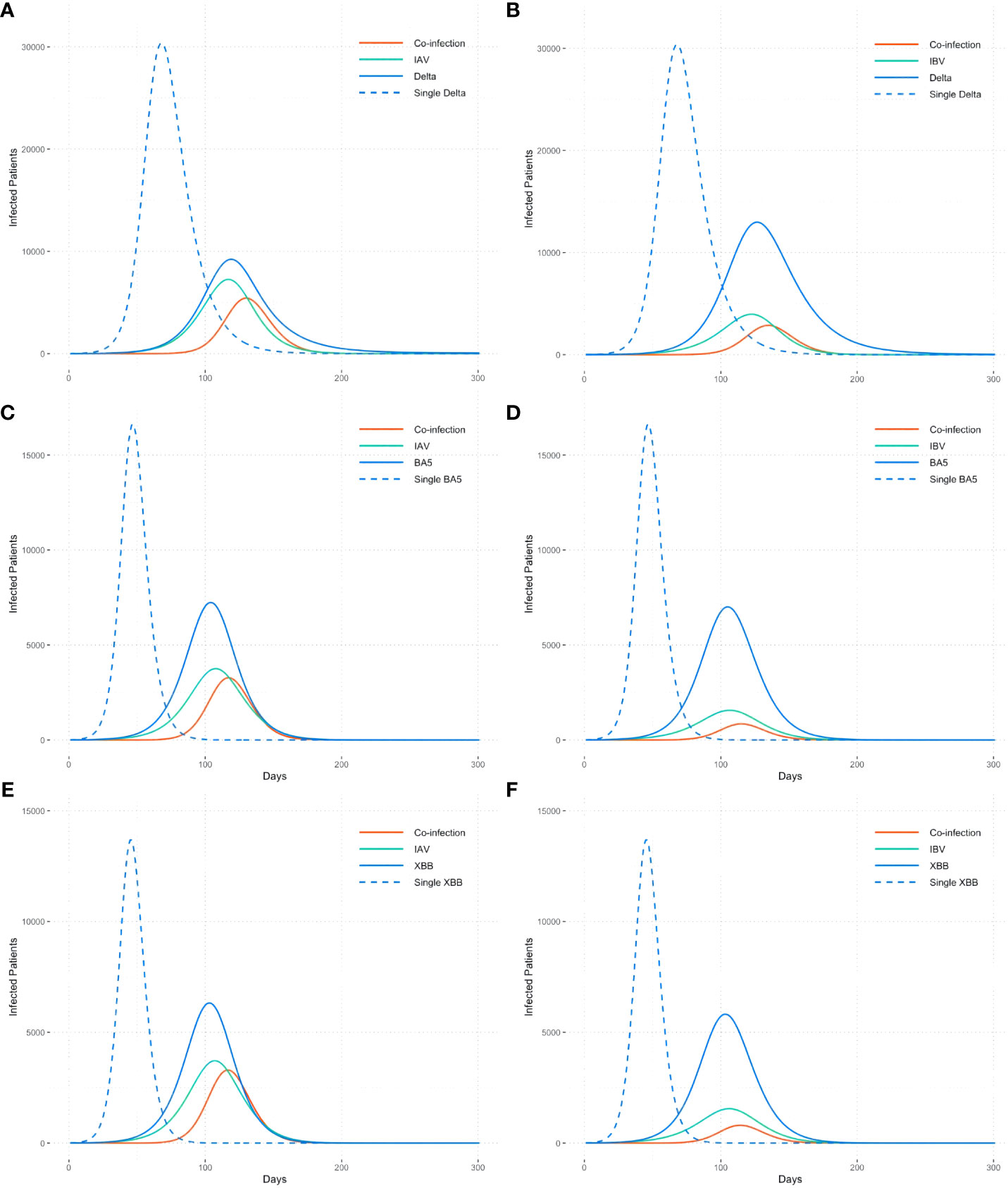
Figure 2 Co-epidemic of influenza types with different SARS-CoV-2 variants. (A)IAV+DELTA, (B)IBV+DELTA, (C)IAV+BA5, (D)IBV+BA5, (E)IAV+XBB, (F)IBV+XBB. The dotted line is the single epidemic curve of COVID-19, and the solid line refers to the number of co-infection and the trend of flu and COVID-19 when co-epidemic.
Number of cases co-infected with IAV and SARS-CoV-2 is higher than that with IBV, approximately 2 times, demonstrating differences in interactions towards SARS-CoV-2 between different influenza virus genotype. For the same type of influenza virus, the number of co-infected cases increases as SARS-CoV-2 becomes more transmissible, like from the Delta strain to the Omicron strain (Figures 2A, C, E).
Compared to the single epidemic, the transmission curve of the SARS-CoV-2 exhibits a lower trend when co-epidemic with influenza. Meanwhile, the occurrence of co-infection leads to a delayed peak time of SARS-CoV-2, with an average delay of 57 days compared to the single epidemic.
3.2 Protective effect of vaccination against severe illness due to co-infection
To investigate the impact of vaccination on preventing patients with co-infection from severe, we vary the proportion of individuals vaccinated with the third dose of COVID-19 vaccine (vertical axis) with the proportion vaccinated against influenza (horizontal axis) and examine the impact on severe co-infection (Hfc).
We plot some color-coded simulations to visualize the variation of the peak size, cumulative number, and peak time in Figure 3. Overall, as the proportion of individuals vaccinated with the COVID-19 vaccine and influenza vaccines increases, the peak number of co-infected severe illnesses and the number of severe illness cases decreases and the peak time is delayed.
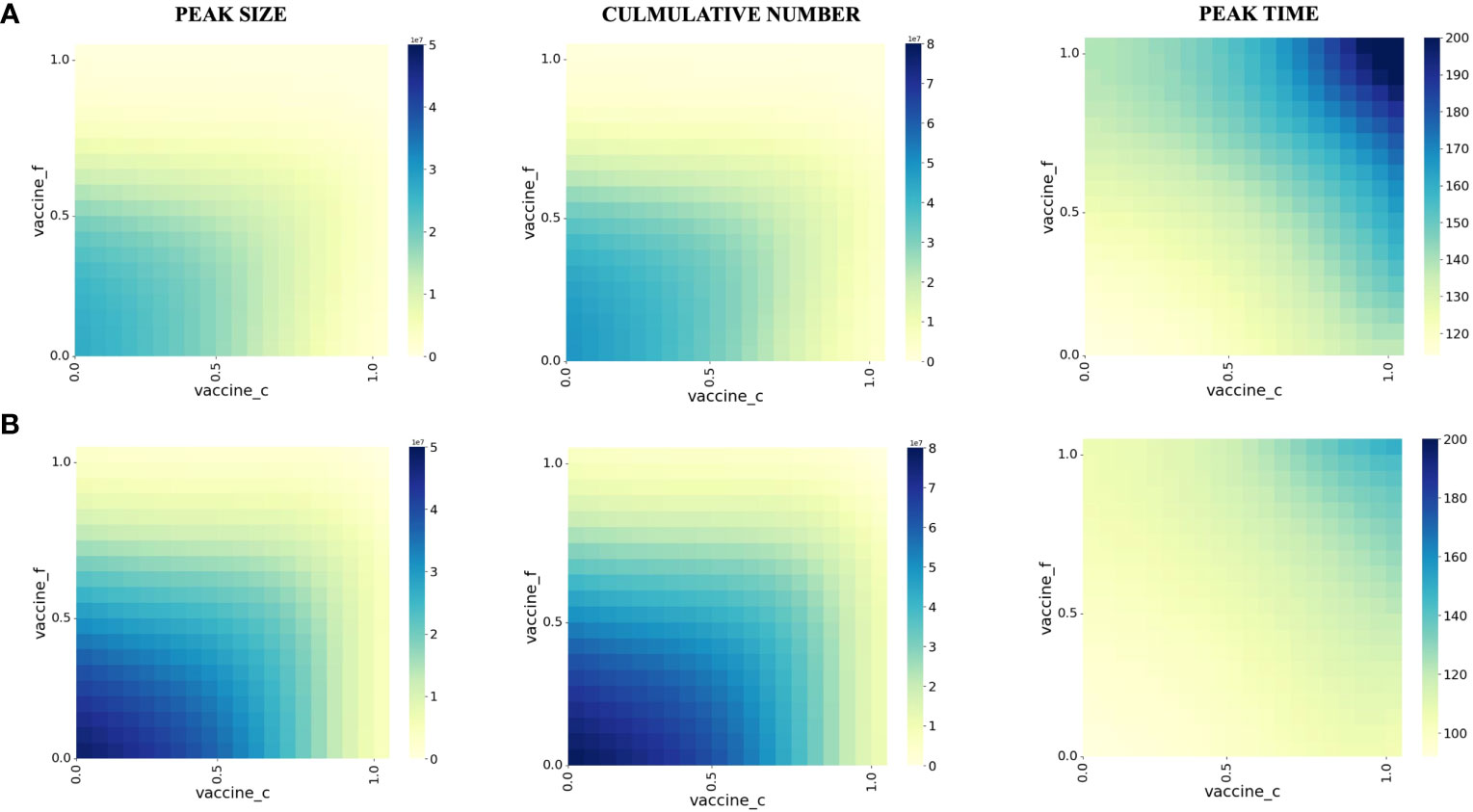
Figure 3 Effect of vaccination on peak size, peak time, and cumulative number of co-infection in different populations. (A) People younger than 60 years old years old (B) People older than 60 years old years old. The proportion of the susceptible population vaccinated against influenza during the initial pandemic period is shown on the vertical axis. The proportion vaccinated against COVID-19 is shown on the horizontal axis, and each square in the figure represents the modeled outcome of the model at the two current vaccination rates.
We also took age-specific vaccine effectiveness into account, mainly for<60 (Panel A) and >60 years old (Panel B). For those<60 years old, the effectiveness of vaccine protection against severe illness is more pronounced in those >60 years of age. Based on our modeling results, for the< 60-year-old population, when the vaccination proportion of the population reaches at least 70% for COVID-19 and 55% for influenza, the cumulative number of co-infections and the number of severe illnesses is substantially reduced.
For those >60 years of age, the cumulative number of co-infections and the number of severe illnesses were substantially reduced when the vaccination proportion of the population reached at least 85% for COVID-19 and 70% for influenza.
3.3 Sensitivity analysis
We conduct the sensitivity analysis using the partial rank correlation coefficients (PRCC) method on different outcomes important to public health intervention, like peak number (Figure 4A), the peak time of co-infections (Figure 4B), and the total number of co-infections (Figure 4C), to identify the key parameters of the epidemic with the hope of determining public health measures that can be implemented to epidemic control and decreasing disease burden. We focus on parameters with the absolute value of PRCC greater than 0.06.
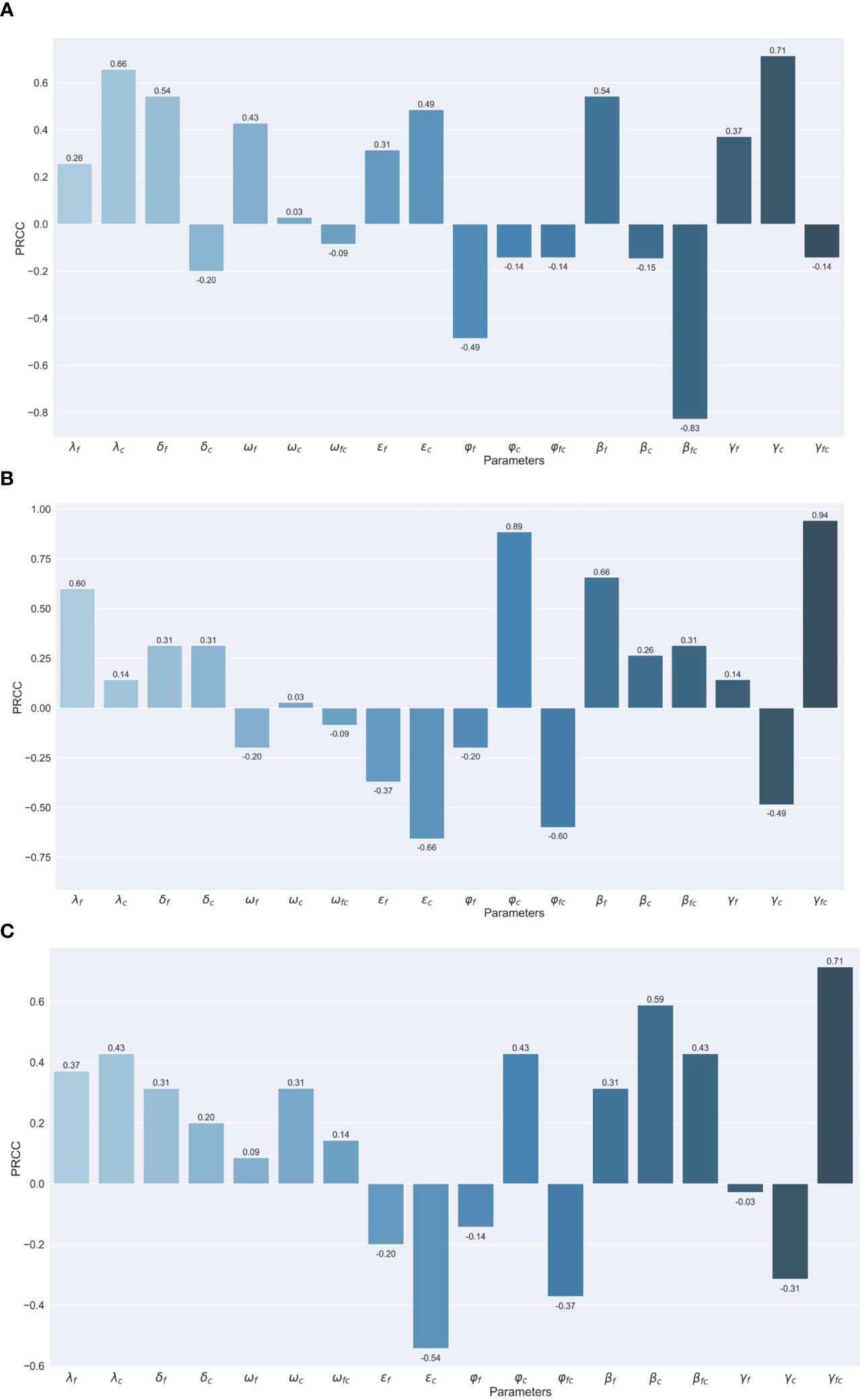
Figure 4 Assessing the Effects of Different Parameters on Co-infection Cases through PRCC Analysis (A) Maximum number of co-infection cases. (B) Peak Timing of co-infection cases. (C) Total number of co-infection cases.
For the peak number of coinfections, the transmission rate of COVID-19 (indicated as '') and recovery period for severe cases of COVID-19 ('') have a positive impact, while the vaccine protection rate against severe co-infection ('') has a negative impact. The positive correlations suggest that with the escalation of the transmission rate of COVID-19 and the recovery period for severe cases of COVID-19, the peak size also experiences a notable increase. Conversely, the vaccine protection rate against severe co-infection is unique due to its considerable negative correlation. This implies that an improvement in the VE against co-infection from severe can potentially lead to a decline in such cases.
For the peak time for co-infection population, infection rates of influenza ('') severity rates for COVID-19 ('') the protection rate of the vaccine against severe influenza ('') and the recovery period of severe co-infection cases ('') ‘demonstrate a positive correlation with PRCC values of 0.60, 0.89, 0.66 and 0.94, respectively (Figure 2B). While susceptibility of influenza patients to COVID-19 (' ') and severity rates of co-infection ('') posed a negative correlation.
As to the total number of coinfections, severe co-infection cases ('') are strongly positively correlated and susceptibility of influenza patients to COVID-19 ('') is in reverse.
4 Discussion
In this article, we developed the SEIHR model to simulate the co-prevalence of influenza viruses and SARS-CoV-2 to determine the impact on the health of the population and to facilitate the adoption of targeted strategies. At the same time, we elucidated the benefit of vaccination for co-infection populations based on age stratification.
WHO has declared an end to COVID-19 as a public health emergency, and some articles note that COVID-19 is now entering mini-waves rather than seasonal surges (Callaway, 2023). Reduced exposure due to PHSM during the COVID-19 pandemic resulted in insufficient preexisting immunity to influenza in the population, which could shift the immune landscape, build up a susceptible pool, and further alter the timing, trajectories, and severity of influenza in future seasonal epidemics (Ashraf et al., 2022). The above conditions make it much more likely that influenza and COVID-19 will be co-prevalent at the same time in the short term. It is important to raise awareness of co-infection with COVID-19 and influenza viruses and to assess the risk of co-infection manner promptly.
In vivo, experiments have demonstrated that infection with influenza causes a slight upregulation of ACE2 expression levels (2-3 folds), and that co-infection with influenza and COVID-19 strongly upregulates ACE2 expression levels (20 folds) (Swets et al., 2022), but the studies did not compare the differences between the different influenza virus types. Typically, influenza A and B viruses take turns to be the dominant types. The impact of different types of influenza and COVID-19 co-prevalent is still unknown. Our study suggests that influenza A, when co-prevalent with COVID-19, will cause a higher peak of co-infection than influenza B, which may be because influenza A viruses spread at a greater rate than influenza B (Lei et al., 2022; Yuan et al., 2013) and have a shorter incubation period (Zhong et al., 2011).
Existing studies have reported variable prevalence of co-infection. However, it is well recognized that co-infections can exacerbate damage in immunocompromised populations and significantly increase rates of severe illness and mortality. And it’s noted that simultaneous vaccination against H1N1 and SARS-CoV-2 is an effective prevention strategy for the coming winter (Bao et al., 2021). Our study found that simultaneous vaccination of populations against influenza and COVID-19 before the onset of the co-prevalent season did reduce the number and peak of cumulative severe illnesses, which effectively reduced the burden of disease and the impact on the healthcare system in co-infected populations. Moreover, the results of the age stratification of the model suggest that the increasing vaccination rate of people >60 years old is more effective in reducing and delaying the peak of severe illness, which suggests that areas with scarce vaccine resources may choose to prioritize vaccination of people >60 years old, At the same time, reducing the number of severe cases of co-infections, influenza vaccination seems more effective than COVID-19 vaccination, but the two vaccines are administered at similar rates, which encourages the development of bivalent vaccines.
Sensitivity analyses of the model showed that the number of peak co-infections was mainly positively correlated with the rate of transmission of the COVID-19 virus and the length of recovery from COVID-19 severe illness, and therefore the transmission and virulence of the COVID-19 epidemic strains should be continuously monitored. The protective effect of vaccines against severe disease from co-infections is a significant negative correlate, so timely assessment of the ability of virulent strains to escape from infection or vaccination to produce neutralization antibodies is also important.
In the construction of the model, we considered the infection order of the two viruses, the cross-protective effect of the vaccine, and the attenuation of the neutralization antibody, to restore as much as the influencing factors of co-infection in the population, providing a scientific basis for vaccine resource allocation as well. At the same time, we selected strains with potential pandemics. We presented scenarios of co-prevalence of different types of influenza viruses and SARS-CoV-2, which provides an assessment of the health risk of the population raised by coinfection. There are still some limitations in our study. Firstly, we only considered co-infection in the population under co-prevalence of COVID-19 and influenza. Therefore, we believe that there is no widespread co-infection in the population when the two viruses are not co-pandemic. Secondly, few data have been reported on the rate of protection against severe disease in co-infected patients by vaccination. Therefore, for parameters such as the vaccine protection rate against co-infection, we refer to the COVID-19 protection rate, as there are more real-world studies on this data. It is important to note that the model parameters utilize conventional seasonal parameters. However, due to previous large-scale outbreaks of COVID-19, the parameters for XBB may encompass the influence of population immunity levels.
5 Conclusion
To minimize the number of severe illnesses arising from co-infection of influenza and COVID-19, in conjunction vaccinations in the population are important, especially priority for the elderly.
Data availability statement
The data that support these findings are available on reasonable request from the corresponding author. Data are not publicly available due to concerns regarding research participant privacy.
Author contributions
JL: Conceptualization, Data curation, Investigation, Writing – original draft, Methodology. YW: Conceptualization, Methodology, Writing – original draft. ZL: Data curation, Writing – review & editing, Software. WH: Writing – review & editing, Investigation, Visualization. JS: Data curation, Writing – review & editing. QL: Data curation, Writing – review & editing. MZ: Data curation, Writing – review & editing. ZC: Data curation, Writing – review & editing. YG: Data curation, Writing – review & editing. WZ: Data curation, Writing – review & editing. TL: Data curation, Writing – review & editing. ZZ: Writing – review & editing, Supervision. CH: Funding acquisition, Supervision, Writing – review & editing. ZY: Writing – original draft, Funding acquisition, Supervision.
Funding
The author(s) declare financial support was received for the research, authorship, and/or publication of this article. This work was supported by the National Basic Research Priorities Program of China (Grant No. 2023YFC3041600), the National Key Research and Development Program of China (No. 2022YFC2600705); Self-supporting Program of Guangzhou Laboratory (Grant No. SRPG22-007); Science and Technology Development Fund of Macau SAR (005/2022/ALC); Science and Technology Program of Guangzhou (No. 2022B01W0003); Science and Technology Program of Guangzhou (Grant No. 202102100003); Science and Technology Development Fund of Macau SAR(0045/2021/A); Macau University of Science and Technology (FRG-20-021-MISE); and National Natural Science Foundation of China (Grant No. 82361168672).
Conflict of interest
The authors declare that the research was conducted in the absence of any commercial or financial relationships that could be construed as a potential conflict of interest.
Publisher’s note
All claims expressed in this article are solely those of the authors and do not necessarily represent those of their affiliated organizations, or those of the publisher, the editors and the reviewers. Any product that may be evaluated in this article, or claim that may be made by its manufacturer, is not guaranteed or endorsed by the publisher.
Abbreviation
IAV, Influenza A virus; IBV, Influenza B virus; VE, vaccine effectiveness; PHSM, Public Health and Social Measures; PRCC, Partial Rank Correlation Coefficient; SEIR, Susceptible-Exposed-Infectious-Recovered.
References
Ali, S. T., Lau, Y. C., Shan, S., Ryu, S., Du, Z., Wang, L., et al. (2022). Prediction of upcoming global infection burden of influenza seasons after relaxation of public health and social measures during the COVID-19 pandemic: a modelling study. Lancet Global Health 10, e1612–e1622. doi: 10.1016/S2214-109X(22)00358-8
Alosaimi, B., Naeem, A., Hamed, M. E., Alkadi, H. S., Alanazi, T., Al Rehily, S. S., et al. (2021). Influenza co-infection associated with severity and mortality in COVID-19 patients. Virol. J. 18, 127. doi: 10.1186/s12985-021-01594-0
Altarawneh, H. N., Chemaitelly, H., Ayoub, H. H., Tang, P., Hasan, M. R., Yassine, H. M., et al. (2023). Effects of previous infection, vaccination, and hybrid immunity against symptomatic Alpha, Beta, and Delta SARS-CoV-2 infections: an observational study. EBioMedicine 95. doi: 10.1016/j.ebiom.2023.104734
Ampomah, P. B., Lim, L. H. (2020). Influenza A virus-induced apoptosis and virus propagation. Apoptosis 25, 1–11. doi: 10.1007/s10495-019-01575-3
Ashraf, M., Rajaram, S., English, P. M. (2022). How the COVID 19 pandemic will shape influenza public health initiatives: The UK experience. Hum. Vaccines Immunotherapeutics 18, 2056399. doi: 10.1080/21645515.2022.2056399
Bao, L., Deng, W., Qi, F., Lv, Q., Song, Z., Liu, J., et al. (2021). Sequential infection with H1N1 and SARS-CoV-2 aggravated COVID-19 pathogenesis in a mammalian model, and co-vaccination as an effective method of prevention of COVID-19 and influenza. Signal Transduction Targeted Ther. 6, 200. doi: 10.1038/s41392-021-00618-z
Bedford, T., Riley, S., Barr, I. G., Broor, S., Chadha, M., Cox, N. J., et al. (2015). Global circulation patterns of seasonal influenza viruses vary with antigenic drift. Nature 523, 217–220. doi: 10.1038/nature14460
Callaway, E. (2023). COVID's future: mini-waves rather than seasonal surges. Nature. doi: 10.1038/d41586-023-01437-8
Candelli, M., Pignataro, G., Torelli, E., Gullì, A., Nista, E. C., Petrucci, M., et al. (2021). Effect of influenza vaccine on COVID-19 mortality: a retrospective study. Internal Emergency Med., 1–7. doi: 10.1007/s11739-021-02702-2
Chang, S., Pierson, E., Koh, P. W., Gerardin, J., Redbird, B., Grusky, D., et al. (2021). Mobility network models of COVID-19 explain inequities and inform reopening. Nature 589, 82–87. doi: 10.1038/s41586-020-2923-3
Cheung, P.-H. H., Chan, C.-P., Jin, D.-Y. (2022). Lessons learned from the fifth wave of COVID-19 in Hong Kong in early 2022. Emerg. Microbes Infect. 11, 1072–1078. doi: 10.1080/22221751.2022.2060137
Chin, E. T., Leidner, D., Zhang, Y., Long, E., Prince, L., Li, Y., et al. (2021). Effectiveness of the mRNA-1273 Vaccine during a SARS-CoV-2 Delta Outbreak in a Prison. New Engl. J. Med. 385, 2300–2301. doi: 10.1056/NEJMc2114089
Cong, B., Deng, S., Wang, X., Li, Y. (2022). The role of respiratory co-infection with influenza or respiratory syncytial virus in the clinical severity of COVID-19 patients: A systematic review and meta-analysis. J. Glob Health 12, 05040. doi: 10.7189/jogh.12.05040
Cowling, B. J., Ip, D. K., Fang, V. J., Suntarattiwong, P., Olsen, S. J., Levy, J., et al. (2014). Modes of transmission of influenza B virus in households. PloS One 9, e108850. doi: 10.1371/journal.pone.0108850
Dadashi, M., Khaleghnejad, S., Elkhichi Abedi, P., Goudarzi, M., Goudarzi, H., Taghavi, A., et al. (2021). COVID-19 and influenza co-infection: A systematic review and meta-analysis. Front. Med. 8. doi: 10.3389/fmed.2021.681469
Du, Z., Fox, S. J., Ingle, T., Pignone, M. P., Meyers, L. A. (2022). Projecting the combined health care burden of seasonal influenza and COVID-19 in the 2020-2021 season. MDM Policy Pract. 7, 23814683221084631. doi: 10.1177/23814683221084631
Fahim, M., Roshdy, W. H., Deghedy, O., Kamel, R., Naguib, A., Showky, S., et al. (2022). Epidemiology, disease severity and outcome of severe acute respiratory syndrome coronavirus 2 and influenza viruses coinfection seen at Egypt integrated acute respiratory infections surveillance, 2020–2022. Can. J. Infect. Dis. Med. Microbiol. 2022, 7497500. doi: 10.1155/2022/7497500
Faycal, A., Ndoadoumgue, A., Sellem, B., Blanc, C., Dudoit, Y., Schneider, L., et al. (2022). Prevalence and factors associated with symptom persistence: a prospective study of 429 mild COVID-19 outpatients. Infect. Dis. Now 52, 75–81. doi: 10.1016/j.idnow.2021.11.003
Fernandes, Q., Inchakalody, V. P., Merhi, M., Mestiri, S., Taib, N., Moustafa Abo El-Ella, D., et al. (2022). Emerging COVID-19 variants and their impact on SARS-CoV-2 diagnosis, therapeutics and vaccines. Ann. Med. 54, 524–540. doi: 10.1080/07853890.2022.2031274
Fielding, J. E., Kelly, H. A., Glass, K. (2015). Transmission of the first influenza A(H1N1)pdm09 pandemic wave in Australia was driven by undetected infections: Pandemic response implications. PloS One 10, e0144331. doi: 10.1371/journal.pone.0144331
Gao, L., Zheng, C., Shi, Q., Xiao, K., Wang, L., Liu, Z., et al. (2022). Evolving trend change during the COVID-19 pandemic. Front. Public Health 10. doi: 10.3389/fpubh.2022.957265
Garg, I., Gangu, K., Shuja, H., Agahi, A., Sharma, H., Bobba, A., et al. (2022). COVID-19 and influenza coinfection outcomes among hospitalized patients in the United States: A propensity matched analysis of national inpatient sample. Vaccines (Basel) 10. doi: 10.3390/vaccines10122159
Gras-Valentí, P., Chico-Sánchez, P., Algado-Sellés, N., Gimeno-Gascón, M. A., Mora-Muriel, J. G., Sánchez-Payá, J. (2021). Efectividad de la vacuna de la gripe para prevenir casos graves. Temporada 2018/2019. Gaceta Sanitaria 35, 339–344. doi: 10.1016/j.gaceta.2020.02.008
Hirve, S., W.H. Organization (2015). Seasonal influenza vaccine use in low and middle income countries in the tropics and subtropics: a systematic review (Geneva: World Health Organization). Available at: https://iris.who.int/bitstream/handle/10665/188785/9789241565097_eng.pdf.
Karyakarte, R. P., Das, R., Rajmane, M. V., Dudhate, S., Agarasen, J., Pillai, P., et al. (2023). Chasing SARS-CoV-2 XBB.1.16 recombinant lineage in India and the clinical profile of XBB.1.16 cases in Maharashtra, India. Cureus 15, e39816. doi: 10.7759/cureus.39816
Katsiroumpa, A., Sourtzi, P., Kaitelidou, D., Siskou, O., Konstantakopoulou, O., Galanis, P. (2023). Predictors of Seasonal Influenza Vaccination Willingness among High-Risk Populations Three Years after the Onset of the COVID-19 Pandemic. Vaccines 11, 331. doi: 10.3390/vaccines11020331
Kim, D., Quinn, J., Pinsky, B., Shah, N. H., Brown, I. (2020). Rates of co-infection between SARS-CoV-2 and other respiratory pathogens. JAMA 323, 2085–2086. doi: 10.1001/jama.2020.6266
Kim, E.-H., Nguyen, T.-Q., Casel, M. A. B., Rollon, R., Kim, S.-M., Kim, Y.-I., et al. (2022). Coinfection with SARS-coV-2 and influenza A virus increases disease severity and impairs neutralizing antibody and CD4+ T cell responses. J. Virol. 96, e01873–e01821. doi: 10.1128/jvi.01873-21
Lei, H., Yang, L., Wang, G., Zhang, C., Xin, Y., Sun, Q., et al. (2022). Transmission patterns of seasonal influenza in China between 2010 and 2018. Viruses 14, 2063. doi: 10.3390/v14092063
Li, Z., Liu, S., Li, F., Li, Y., Li, Y., Peng, P., et al. (2022). Efficacy, immunogenicity and safety of COVID-19 vaccines in older adults: a systematic review and meta-analysis. Front. Immunol. 13, 965971. doi: 10.3389/fimmu.2022.965971
Liu, Q., Qin, C., Liu, M., Liu, J. (2021). Effectiveness and safety of SARS-CoV-2 vaccine in real-world studies: a systematic review and meta-analysis. Infect. Dis. Poverty 10, 132. doi: 10.1186/s40249-021-00915-3
Liu, S., Jiang, C., Wang, J., Liu, Y. (2023). The factors correlated with COVID-19 vaccination coverage in Chinese hypertensive patients managed by community general practitioner. Hum. Vaccin Immunother. 19, 2197839. doi: 10.1080/21645515.2023.2197839
Lyngse, F. P., Mortensen, L. H., Denwood, M. J., Christiansen, L. E., Møller, C. H., Skov, R. L., et al. (2021). SARS-CoV-2 Omicron VOC transmission in Danish households. MedRxiv 2021.12. 27.21268278. doi: 10.1101/2021.12.27.21268278
Marín-Hernández, D., Schwartz, R. E., Nixon, D. F. (2021). Epidemiological evidence for association between higher influenza vaccine uptake in the elderly and lower COVID-19 deaths in Italy. J. Med. Virol. 93, 64–65. doi: 10.1002/jmv.26120
Menni, C., Valdes, A. M., Polidori, L., Antonelli, M., Penamakuri, S., Nogal, A., et al. (2022). Symptom prevalence, duration, and risk of hospital admission in individuals infected with SARS-CoV-2 during periods of omicron and delta variant dominance: a prospective observational study from the ZOE COVID Study. Lancet 399, 1618–1624. doi: 10.1016/S0140-6736(22)00327-0
Moghadami, M. (2017). A narrative review of influenza: A seasonal and pandemic disease. Iran J. Med. Sci. 42, 2–13.
Musuuza, J. S., Watson, L., Parmasad, V., Putman-Buehler, N., Christensen, L., Safdar, N. (2021). Prevalence and outcomes of co-infection and superinfection with SARS-CoV-2 and other pathogens: A systematic review and meta-analysis. PloS One 16, e0251170. doi: 10.1371/journal.pone.0251170
Ogata, T., Tanaka, H. (2023). SARS-CoV-2 incubation period during the omicron BA.5–dominant period in Japan. Emerging Infect. Dis. J. 29, 595. doi: 10.3201/eid2903.221360
Ojo, M. M., Benson, T. O., and Peter, O. J., Goufo, E. F. D. (2022). Nonlinear optimal control strategies for a mathematical model of COVID-19 and influenza co-infection. Physica A: Stat. Mechanics its Appl. 607, 128173.
Opatowski, L., Baguelin, M., Eggo, R. M. (2018). Influenza interaction with cocirculating pathogens and its impact on surveillance, pathogenesis, and epidemic profile: A key role for mathematical modelling. PloS Pathog. 14, e1006770. doi: 10.1371/journal.ppat.1006770
Park, J.-E., Ryu, Y. (2018). Transmissibility and severity of influenza virus by subtype. Infection Genet. Evol. 65, 288–292. doi: 10.1016/j.meegid.2018.08.007
Pilz, S., Theiler-Schwetz, V., Trummer, C., Krause, R., Ioannidis, J. P.A. (2022). SARS-CoV-2 reinfections: Overview of efficacy and duration of natural and hybrid immunity. Environ. Res. 209, 112911. doi: 10.1016/j.envres.2022.112911
Pinky, L., Dobrovolny, H. M. (2022). Epidemiological consequences of viral interference: A mathematical modeling study of two interacting viruses. Front. Microbiol. 13. doi: 10.3389/fmicb.2022.830423
Pormohammad, A., Ghorbani, S., Khatami, A., Razizadeh, M. H., Alborzi, E., Zarei, M., et al. (2021). Comparison of influenza type A and B with COVID-19: A global systematic review and meta-analysis on clinical, laboratory and radiographic findings. Rev. Med. Virol. 31, e2179. doi: 10.1002/rmv.2179
Rees, E. M., Nightingale, E. S., Jafari, Y., Waterlow, N. R., Clifford, S., Pearson, C. A. B., et al. (2020). COVID-19 length of hospital stay: a systematic review and data synthesis. BMC Med. 18, 1–22. doi: 10.1186/s12916-020-01726-3
Rezaee, D., Bakhtiari, S., Jalilian, F.A., Doosti-Irani, A., Asadi, F. T., Ansari, N. (2023). Coinfection with severe acute respiratory syndrome coronavirus 2 (SARS-CoV-2) and influenza virus during the COVID-19 pandemic. Arch. Virol. 168, 53. doi: 10.1007/s00705-022-05628-y
Rondy, M., El Omeiri, N., Thompson, M. G., Levêque, A., Moren, A., Sullivan, S. G. (2017). Effectiveness of influenza vaccines in preventing severe influenza illness among adults: A systematic review and meta-analysis of test-negative design case-control studies. J. Infect. 75, 381–394. doi: 10.1016/j.jinf.2017.09.010
Stowe, J., Tessier, E., Zhao, H., Guy, R., Muller-Pebody, B., Zambon, M., et al. (2021). Interactions between SARS-CoV-2 and influenza, and the impact of coinfection on disease severity: a test-negative design. Int. J. Epidemiol. 50, 1124–1133. doi: 10.1093/ije/dyab081
Swets, M. C., Russell, C. D., Harrison, E. M., Docherty, A. B., Lone, N., Girvan, M., et al. (2022). SARS-CoV-2 co-infection with influenza viruses, respiratory syncytial virus, or adenoviruses. Lancet 399, 1463–1464. doi: 10.1016/S0140-6736(22)00383-X
Tanaka, H., Ogata, T., Shibata, T., Nagai, H., Takahashi, Y., Kinoshita, M., et al. (2022). Shorter incubation period among COVID-19 cases with the BA. 1 Omicron variant. Int. J. Environ. Res. Public Health 19, 6330.
Tolossa, T., Wakuma, B., Seyoum Gebre, D., Merdassa Atomssa, E., Getachew, M., Fetensa, G., et al. (2021). Time to recovery from COVID-19 and its predictors among patients admitted to treatment center of Wollega University Referral Hospital (WURH), Western Ethiopia: Survival analysis of retrospective cohort study. PloS One 16, e0252389. doi: 10.1371/journal.pone.0252389
Varshney, K., Pillay, P., Mustafa, A. D., Shen, D., Adalbert, J. R., Mahmood, M. Q., et al. (2023). A systematic review of the clinical characteristics of influenza-COVID-19 co-infection. Clin. Exp. Med. doi: 10.1007/s10238-023-01116-y
Wang, R., Liu, M., Liu, J. (2021). The association between influenza vaccination and COVID-19 and its outcomes: A systematic review and meta-analysis of observational studies. Vaccines 9, 529. doi: 10.3390/vaccines9050529
Wilcox, C. R., Islam, N., Dambha-Miller, H. (2021). Association between influenza vaccination and hospitalisation or all-cause mortality in people with COVID-19: a retrospective cohort study. BMJ Open Respir. Res. 8, e000857. doi: 10.1136/bmjresp-2020-000857
Wu, B. (2022) The vaccination rate is only 2.46%. Why is the flu vaccine not “popular”? Available online at: https://epaper.oeeee.com/epaper/A/html/2022-09/13/content_20997.htm#article.
Wu, Y., Kang, L., Guo, Z., Liu, J., Liu, M., Liang, W. (2022). Incubation period of COVID-19 caused by unique SARS-CoV-2 strains: a systematic review and meta-analysis. JAMA network Open 5, e2228008-e2228008. doi: 10.1001/jamanetworkopen.2022.28008
Yang, J., Gong, Y., Zhang, C., Sun, J., Wong, G., Shi, W., et al. (2022). Co-existence and co-infection of influenza A viruses and coronaviruses: Public health challenges. Innovation 3. doi: 10.1016/j.xinn.2022.100306
Yang, M.-J., Rooks, B. J., Le, T.-T. T., Santiago, I. O., Diamond, J., Dorsey, N. L., et al. (2021). Influenza vaccination and hospitalizations among COVID-19 infected adults. J. Am. Board Family Med. 34, S179–S182. doi: 10.3122/jabfm.2021.S1.200528
Yazici Özkaya, P., Turanli, E. E., Metin, H., Uysal Aydın, A., Çiçek, C., Karapinar, B. (2022). Severe influenza virus infection in children admitted to the PICU: Comparison of influenza A and influenza B virus infection. J. Med. Virol. 94, 575–581. doi: 10.1002/jmv.27400
Young, B., Zhao, X., Cook, A. R., Parry, C. M., Wilder-Smith, A., I-Cheng, M. C. (2017). Do antibody responses to the influenza vaccine persist year-round in the elderly? A systematic review and meta-analysis. Vaccine 35, 212–221. doi: 10.1016/j.vaccine.2016.11.013
Yuan, Q., Nsoesie, E. O., Lv, B., Peng, G., Chunara, R., Brownstein, J. S. (2013). Monitoring influenza epidemics in China with search query from baidu. PloS One 8, e64323. doi: 10.1371/journal.pone.0064323
Yue, H., Zhang, M., Xing, L., Wang, K., Rao, X., Liu, H., et al. (2020). The epidemiology and clinical characteristics of co-infection of SARS-CoV-2 and influenza viruses in patients during COVID-19 outbreak. J. Med. Virol. 92, 2870–2873. doi: 10.1002/jmv.26163
Zandi, M. (2022). ORF9c and ORF10 as accessory proteins of SARS-CoV-2 in immune evasion. Nat. Rev. Immunol. 22, 331. doi: 10.1038/s41577-022-00715-2
Zandi, M., Shafaati, M., Kalantar-Neyestanaki, D., Pourghadamyari, H., Fani, M., Soltani, S., et al. (2022). The role of SARS-CoV-2 accessory proteins in immune evasion. BioMed. Pharmacother. 156, 113889. doi: 10.1016/j.biopha.2022.113889
Zhang, M., Xiao, J., Deng, A., Zhang, Y., Zhuang, Y., Hu, T., et al. (2021). Transmission dynamics of an outbreak of the COVID-19 Delta variant B. 1.617. 2—Guangdong Province, China, May–June 2021. China CDC weekly 3, 584.
Zhao, H. T., Peng, Z. B., Ni, Z. L., Yang, X. K., Guo, Q. Y., Zheng, J. D., et al. (2022). [Investigation on influenza vaccination policy and vaccination situation during the influenza seasons of 2020-2021 and 2021-2022 in China]. Zhonghua Yu Fang Yi Xue Za Zhi 56, 1560–1564.
Zheng, J., Chen, F., Wu, K., Wang, J., Li, F., Huang, S., et al. (2021). Clinical and virological impact of single and dual infections with influenza A (H1N1) and SARS-CoV-2 in adult inpatients. PloS Negl. Trop. Dis. 15, e0009997. doi: 10.1371/journal.pntd.0009997
Zheng, C., Shao, W., Chen, X., Zhang, B., Wang, G., Zhang, W. (2022). Real-world effectiveness of COVID-19 vaccines: a literature review and meta-analysis. Int. J. Infect. Dis. 114, 252–260. doi: 10.1016/j.ijid.2021.11.009
Keywords: SARS-CoV-2, influenza, co-infection, vaccination, compartmental model
Citation: Liang J, Wang Y, Lin Z, He W, Sun J, Li Q, Zhang M, Chang Z, Guo Y, Zeng W, Liu T, Zeng Z, Yang Z and Hon C (2024) Influenza and COVID-19 co-infection and vaccine effectiveness against severe cases: a mathematical modeling study. Front. Cell. Infect. Microbiol. 14:1347710. doi: 10.3389/fcimb.2024.1347710
Received: 01 December 2023; Accepted: 01 February 2024;
Published: 04 March 2024.
Edited by:
Yong-Tang Zheng, Chinese Academy of Sciences (CAS), ChinaReviewed by:
Milad Zandi, Lorestan University of Medical Sciences, IranEduardo Becerril, National Institute of Respiratory Diseases-Mexico (INER), Mexico
Copyright © 2024 Liang, Wang, Lin, He, Sun, Li, Zhang, Chang, Guo, Zeng, Liu, Zeng, Yang and Hon. This is an open-access article distributed under the terms of the Creative Commons Attribution License (CC BY). The use, distribution or reproduction in other forums is permitted, provided the original author(s) and the copyright owner(s) are credited and that the original publication in this journal is cited, in accordance with accepted academic practice. No use, distribution or reproduction is permitted which does not comply with these terms.
*Correspondence: Zhiqi Zeng, emVuZ196aGlxQDEyNi5jb20=; Zifeng Yang, amVmZnlhaEAxNjMuY29t; Chitin Hon, Y3Rob25AbXVzdC5lZHUubW8=