- 1Laboratorio Nacional de Ciencias de la Sostenibilidad (LANCIS), Instituto de Ecología, Universidad Nacional Autónoma de México (UNAM), Ciudad Universitaria, Mexico City, Mexico
- 2Centro de Ciencias de la Atmósfera, Universidad Nacional Autónoma de México (UNAM), Mexico City, Mexico
- 3Agriculture, Forestry, and Ecosystem Services Research, International Institute for Applied Systems Analysis, Laxenburg, Austria
- 4Institute for Environmental Studies (IVM), Vrije Universiteit Amsterdam, Amsterdam, Netherlands
- 5Programa de Investigación en Cambio Climático (PINCC), Universidad Nacional Autónoma de México (UNAM), Mexico City, Mexico
Evidence suggests that climate change could drastically reduce Mexico's agricultural productivity with severe socio-ecological consequences. Population growth and the increasing demand of resources will exacerbate these impacts. Focusing on rainfed maize production, we evaluate the socio-ecological risk that municipalities currently face and how climate change could modify it. Municipalities were classified based on their biophysical and socioeconomic traits like temperature, precipitation, population, gross domestic product, marginalization, and agricultural subsidies. The study identifies municipalities that would face higher risk under climate change conditions, and it evaluates whether increases in agricultural subsidies could be effective for reducing the farmers' future risk. Our results show that during the 2010's, 36.8% of the municipalities and 15% of the population were at very high and high risk, respectively. By 2070, under a high-warming scenario these figures increase to 56.5 and 18.5%. We find that a generalized augment in agricultural subsidies is not enough to compensate for the effects of climate change on the socio-ecological risk of rainfed maize producers. We suggest that transformative adaptation is required for managing the agricultural risk that socio-ecological systems experience under climate change conditions. Such adaptation strategies should include poverty alleviation, promotion of resistant and native varieties of crops, capacity building to improve management and water use, sustainable technification, and soil restoration.
Introduction
Agriculture is the main livelihood option and one of the primary sources of people's household self-consumption and income in rural and peri-urban areas of low-and middle-income countries (Stevens and Madani, 2016). Nevertheless, this activity is highly sensitive to changes in temperature and precipitation regimes (IPCC, 2014). Due to observed changes in climate, almost every aspect of food security (i.e., production, access, and price stability) has been affected, especially in poor and vulnerable societies. Such situation puts agricultural livelihoods at risk and in a state of insecurity, threatens food production at the local level, and it can lead to cascade impacts on regional and global population (Cohn et al., 2016; Donatti et al., 2019; Ray et al., 2019).
Climate change risk arises from the interaction between hazard, vulnerability, and exposure (IPCC, 2014). Various social and economic processes influence these latter attributes of risk. That means that risk is not an isolated entity constructed solely by the possible impacts of biophysical components. It is linked to socioeconomic, cultural, and ecological traits that modify the systems' resistance and resilience (Kotzee and Reyers, 2016). Changes in exposure to adverse climatic elements like high temperatures, change in precipitation patterns, or heat weaves could negatively impact the disadvantaged individuals and communities whose livelihoods depend on climatic conditions (Leichenko and Silva, 2014).
Agriculture is a dynamic socio-ecological system (SES) that results from human-environment interactions in a process shaped by uncertainty, errors, learning, and adaptation (Rivera-Ferre et al., 2013; Maass, 2017). Agriculture is a SES that bridges the biophysical and social elements. Although it is a system that humans widely manage, it could not exist without the biophysical component on which it depends. The agricultural development depends entirely on the benefits of provision (e.g., food) and regulation (e.g., climate, pollination) of nature to people (Díaz et al., 2018). Nevertheless, it also depends on the management that individuals give to their farming systems. Moreover, agriculture responds to other large-scale processes, such as climate and macroeconomy, social and cultural contexts. Besides, agriculture integrates the coevolution of the relationship between biophysical elements like temperature and precipitation, socioeconomic and cultural practices of farmers like different management types, and willingness to change crop varieties (Fuller et al., 2015). The SES approach identifies complex patterns and non-linear dynamics between social and natural systems with different organization and hierarchy levels (Liu et al., 2007). The SES framework allows us to analyze the biophysical and the social subsystems, their complexity, and their synergies at different spatial-temporal scales (Turner et al., 2003; Bennett et al., 2016).
We applied a SES approach to maize agriculture because it is the most important crop in terms of land area and production, besides being the staple component of Mexican diets (Bellon et al., 2018). Consequently, maize production is essential not only in cultural terms but also in food security and people's livelihoods (Eakin, 2000). Smallholder farmers ( ≤ 5 ha with no technification) produce around 60% of the country's national maize production. Maize farmers are highly dependent on rainfed agriculture, and novel climates will impose new challenges to the continuity of this livelihood (e.g., yield reduction, fulfill self-consumption and local demand, income decrease) (Monterroso et al., 2011).
Many studies assess the agricultural risk to climate change focusing on the economic and production impacts. Such studies use biophysical crop models that provide gross estimates of changing yields (Howden et al., 2007; De Salvo et al., 2013; Rosenzweig et al., 2014). Climate change scientists have analyzed the impacts of climatic variables over rainfed maize in Mexico (Murray-Tortarolo et al., 2018; Ureta et al., 2020), while others have explored the linkage between institutions, climate risk, and vulnerability of maize production (Eakin et al., 2018). Although these studies are useful to identify coarse trends and impacts of climate change on maize production, they do not consider the diversity of the socio-ecological contexts which can affect their risk.
Because of the socio-ecological challenges that people can face to deal with the effects of new climate conditions linked to maize production, we combine climate and socioeconomic data in an integrative modeling framework to develop a multivariate risk index. It identifies which municipalities would be at higher risk under climate change, paying particular attention to rainfed maize production. In Mexico, most of the governmental strategies to support the agricultural sector are subsidies that are mainly target cereals producers. These subsidies aim to raise yields by increasing fertilizers' use (Galeana-Pizaña et al., 2021), which does not necessarily improve capacities to decrease the risk of environmental threats. In the risk index, we include increases in agricultural subsidies to assess how effectively this strategy shapes future climate change risk for maize producers. Finally, based on the municipalities' regional risk context, we proposed potential adaptation strategies to alleviate future climate threats on maize producers.
Data and Methods
Dataset
We use a set of socioeconomic and biophysical data to represent the socio-ecological systems' traits. The information of the socioeconomic variables is taken from different information sources. Population and GDP data are from the national census from the National Institute for Statistics and Geography (INEGI, 2010, 2015). We downscaled the economic data assuming a constant municipality share, based on the historical contribution reported by the National Information Systems for Municipalities (SNIM, 2013).1 A national marginalization index developed by the National Population Council (CONAPO, 2010)2 was included to quantify different elements related to poverty, education, housing, population distribution, and incomes (Table 1). The monetary support (subsidies) provided by the Programa Producción para el Bienestar (2019) for farmers was from the Ministry of Agriculture and Rural Development (SADER).3 Data on the percentage of the population working in the primary sector (2010) was taken Geoinformation Portal of the National Commission for Biodiversity (CONABIO, 2012).4 Rainfed maize yield data from 2003 to 2018 were from the National System of Agricultural Information (SIAP, 2018).5 We averaged the yield of the two cultivation periods (spring-summer and autumn-winter) to obtain the annual yield values.
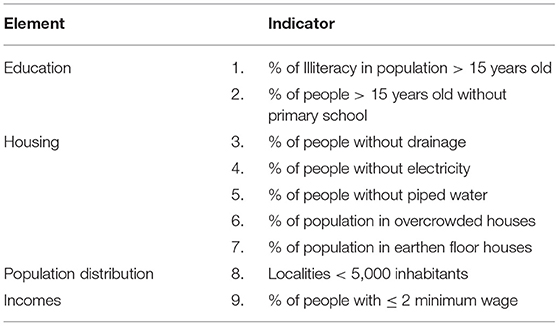
Table 1. Elements that integrate the national index of marginalization CONAPO, 2010.
Bioclimatic variables were obtained from the WorldClim 1.4 database (Hijmans et al., 2005).6 These layers are globally interpolated surfaces obtained from climatic stations' records and represent annual, seasonal, and extreme temperature and precipitation trends for a period between 1960 and 1990. These scenarios are bias-corrected and readily available in raster format. For our analysis, we used a spatial resolution of 2.5 min (about 4.5 km). We averaged each socio-economic and climatic variable for each municipality across Mexico.
Rotated Principal Components Analysis (PCA)
Principal Component Analysis (PCA) allows to reduce the dimensionality of a set of interrelated variables, while retaining most of the variance of the original dataset (Jolliffe, 2002). This technique provides insights about the interrelations of variables and suggest new and more simple interpretations of the original data (Jolliffe, 2002; Wilks, 2011). PCA uses the variance-covariance structure of the data to produce linear combinations that are orthogonal to the original dataset X. This is done by finding the eigenvalues λ and eigenvectors u of the equation (Σ−λI)u = 0 where Σ is the covariance or correlation matrix of X. Due to the differences in measurement scales of the variables, the correlation matrix is used in our analysis (Jolliffe, 2002). The first principal component (PC1) is the linear combination that maximizes subject to the constraint . The remaining principal components (PC) are the linear combinations of that maximize subject to the constraints and for all j≠k. Principal component rotation is used to simplify the interpretation of the PC and to further separate the main direction of variability. In this paper, varimax rotation normalized is applied (Jolliffe, 2002). In the case of rotated PCA, the scores are calculated as F = BZ where F is the matrix of scores, Z is the matrix of standardized values of X and B = L(L′L)−1 is the matrix of loadings (Harman, 1976; Jollife, 2002). If the number of eigenvectors to be rotated that is selected is too small, this could lead to the problem of underrotation which can distort or lead to mixed modes of variability. On the contrary, selecting too many eigenvectors for rotation produces overrotation which can contaminate the analysis as it would lead to excessive separation of modes (O'Lenic and Livezey, 1988). To avoid such problems, we follow O'Lenic and Livezey (1988) and analyze the existence of “shelves” in the scree plot of eigenvalues which would suggest the mixing of signals and, depending on the amount of explained variance, they could indicate that the remaining PC represent noise. We combine this truncation criteria with the Kaiser rule which suggests that the PC with associated eigenvalues smaller than 1 should be discarded (Johnson and Wichern, 2007). It is important to note that while the total amount of explained variance of the set of selected PC is the same before and after rotation, the explained variance is redistributed among the PC (also called factors after rotation), and their relative contribution can be different.
The rotated PCA analysis was performed to identify factors that could be used as a proxy for climate change risk at the municipal level for maize yields and farmers. We interpret the resulting PC or factors as risk indices that combine biophysical and socioeconomic elements and that aim to represent climate and socioeconomic challenges affecting rainfed maize production. Mexican municipalities (n = 2,457) were classified according to these risk indices. Table 2 shows the variables included in our PCA analysis.
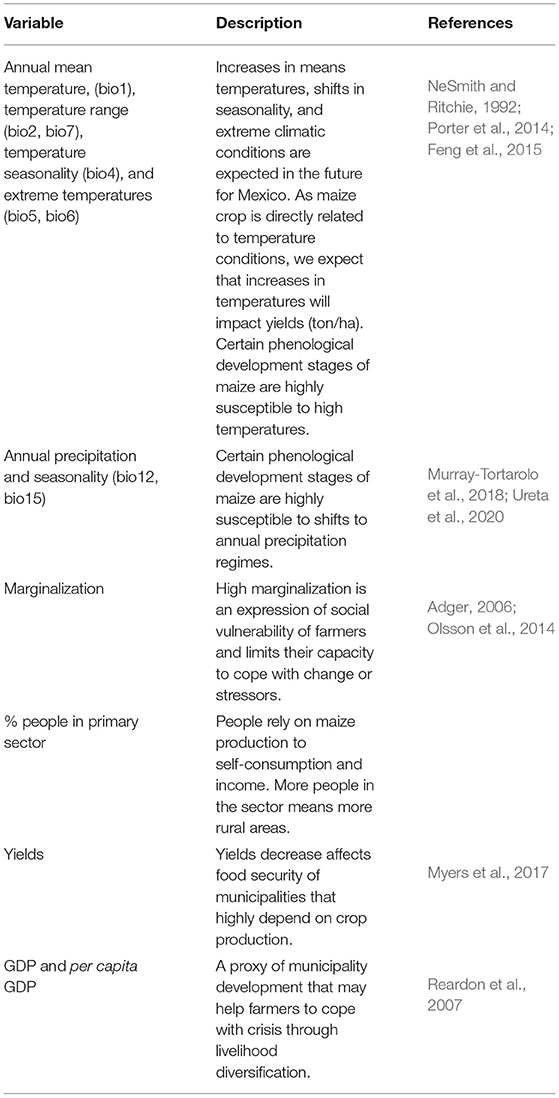
Table 2. Description of socioeconomic and climatic variables and their assumed effects in maize agriculture.
Multivariate Risk Index
Our analysis focuses on characterizing the historical socio-ecological conditions (i.e., bioclimatic and socioeconomic variables) at the municipality level and on how risk levels would change under future climate conditions and the corresponding rainfed maize yields.
A composite risk index is proposed to summarize the information contained in the rotated PCs to help rank municipalities according to their implied risk in different dimensions. The purpose of the aggregation of variables of different nature is to identify municipalities at risk from a socio-ecological perspective. The index also identifies municipalities that would potentially face higher impacts from future climate change in maize production and farmers' livelihood given the current socio-ecological conditions. In this form, we integrate in a coupled and additive way not only climate conditions that affect the maize phenology (e.g., higher temperatures), but also contextual socioeconomic issues that could reinforce potential negative impacts (Table 2). The proposed index is based on the use quadrants for analyzing and classifying PCA scores (Chakraborty et al., 2019; Estrada et al., 2020). This is illustrated in a two-dimensional space and then generalized to an n-dimensional space. Consider a scatterplot of two factors on the Cartesian plane which is divided in four quadrants that intersect in the origin (0,0). The quadrants represent four possible combinations in which the factor scores can fall: quadrant I contains the combination of score values that are positive in both factors, (+,+); quadrant II is defined by negative values in the x-axis and positive values in the y-axis (−,+); quadrant III contains the score values that are negative in both axes (−,−); and quadrant IV defined by positive values in the x-axis and negative values in the y-axis (+,−). In PCA analysis, the interpretation of each quadrant is derived from the meaning that is assigned to each factor. For example, assume that factor 1 and factor 2 are interpreted as aridity and income indices, respectively, and that high positive values indicate more arid conditions and higher income. Then, the municipalities with higher levels of aridity and income would be found in quadrant I, while quadrant II would contain those that are less arid and that are characterized by high income levels, and so on. Positive/negative values in the PCA indices can be associated to challenges or advantages certain municipalities have in terms of socioecological risk. This use of factors for classification can be generalized to n-dimensions once the interpretation of each factor is assigned and can be used to define multivariate risk indices as follows. First, positive and negative scores in each factor are associated with higher or lower risk levels. Second, for each factor, if the sign of the i-th factor score (i.e., municipality) contributes to a higher risk, then a value of 1 is assigned and added to the multivariate risk index. Third, the total score for each municipality is normalized by the number of factors used, and thus the risk index is bounded between zero and one. Values close to 1 in this composite index represent municipalities with higher risk from climatic elements (i.e., high temperatures, low precipitation) and socioeconomic elements (i.e., low GDP, high marginalization, and high dependency of primary sector income) that can affect agricultural producers focused on rainfed maize. Supplementary Table 1 provides a description of factor conditions for the construction of the proposed multivariate risk index.
Risk Index Under Future Scenarios
We use a supervised ensemble machine learning Random Forest (RF) algorithm to predict current maize yields across municipalities under a set of socioeconomic and climatic predictors. RF is a technique based on bootstrap aggregation, which reduces the variance in predictions, it is highly flexible allowing it to incorporate different types of variables, and exhibits a high predictive power (Hastie et al., 2004). This algorithm is extensively used to generate predictions of crop yields at global and regional scales (Everingham et al., 2016; Jeong et al., 2016) and particularly maize crops (Márquez-Ramos et al., 2020). Five bioclimatic (bio1, bio4, bio5, bio6, bio12) and four socioeconomic variables (population, GDP, marginalization, and subsidies for maize production) (Supplementary Figure 1) at the municipality level were selected to train RF models. Rows with missing data were removed from the original dataset, and therefore the final dataset for this part was composed of 2,300 municipalities. First, we explored a wide range of parameters (mtry, maxnodes, ntree, and nodesize) with the aim to select the best combination that minimizes the prediction error using the RMSE (root mean square error). The best parameterization for the final model was: ntree = 1,000, importance = T, mtry = 7, nodesize = 10, maxnodes = NULL. We implemented a cross-validation strategy to avoid model overfitting and potential spatial autocorrelation effects (Supplementary Figure 2). This strategy consists of generating a random partition of the data into two sets: calibration (70% of the data) and another for validation (30%). The final model's fit and predictive ability was evaluated using R-squared and RMSE (Supplementary Figure 2)7.
Based on our calibrated RF model, we generate a projection under a future high-warming climate change scenario (RCP8.5) produced by the Beijing Climate Center Climate System Model (BCC-CSM1.1) for 2070. We generated an additional scenario that includes an increase of 25% in agricultural subsidies (scenario RCP8.5+25%). This scenario is motivated by the fact that ImpProd19 was the most important variable in all RF models trained (Supplementary Figure 3). Accordingly, it could be theorized that an increase in agricultural subsidies could alleviate the reduction in maize yields produced under a high-emission climate change scenario. This scenario was used to evaluate if economic subsidies can be considered as an effective adaptation policy to reduce the risk farmers would face in the future.
Finally, the projected bioclimatic variables (RCP8.5 from BCC-CSM1.1), future maize yields (estimated from projections of a RF model), and a plus of 25% more subsidies were used to evaluate the future risk for all municipalities using the multivariate risk index proposed in section Multivariate Risk Index.
Results
PCA
We selected the first five components from the PCA, which account for 84% of the total variance of the dataset. Varimax normalized rotation was applied to the retained PCs, and Table 3 shows the resulting factor loadings. The resulting rotated PCs (referred to as factors) can be separated into two groups. The first group refers to biophysical elements like minimum, mean and maximum temperatures (factor 1), and precipitation, and temperature variability (factor 3). The second group combines socioeconomic characteristics like the municipality size in terms of population and GDP (factor 2), marginalization, proportion of people working in the primary sector, and per capita GDP (factor 4) (Figure 1).
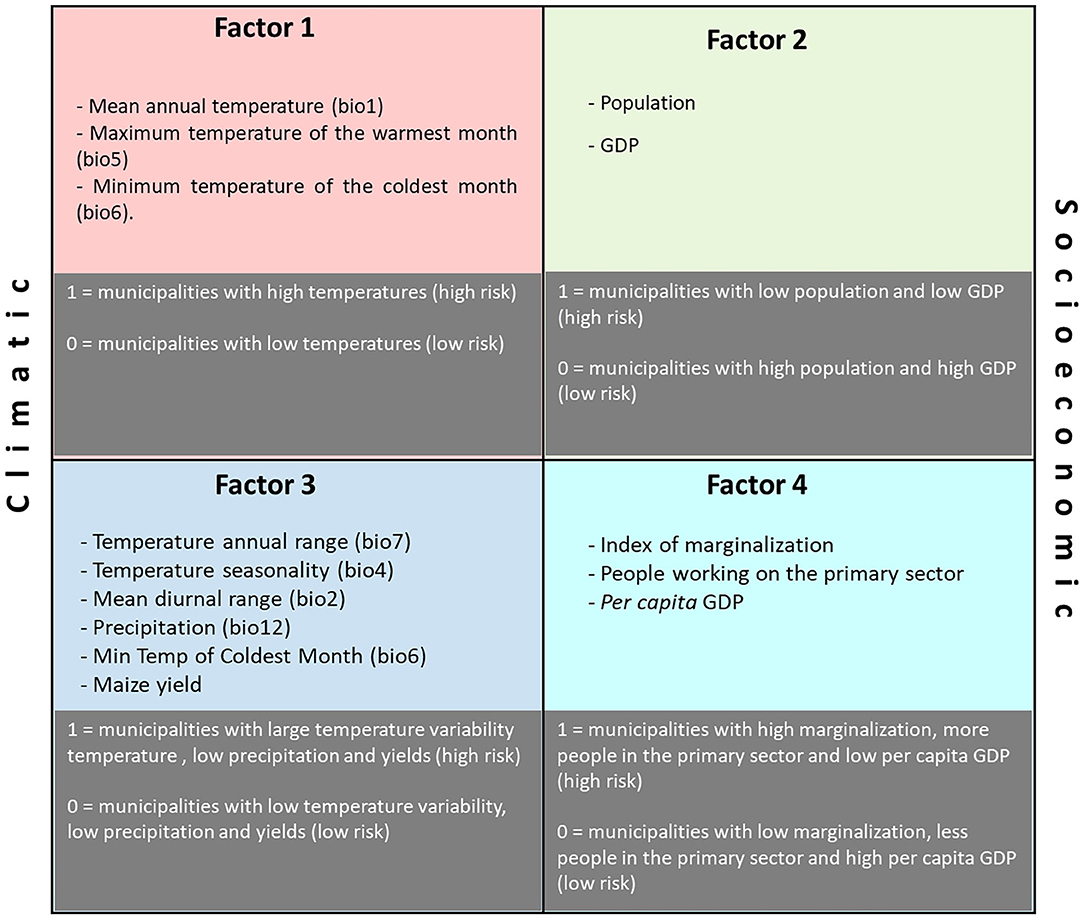
Figure 1. Explanatory variables used in the PCA and the resulting factors. Gray rectangles explain the value given to the risk index.
The factors' values are related to the risk that municipalities can face considering the socio-ecological elements. High values of factor 1 refer to municipalities with high temperatures (Figure 1), and high values of factor 3 relate to temperature variability, low precipitation, and low maize yields. The socioeconomic factors show that high positive values in factor 2 correspond to highly populated municipalities with large GDP values with better access to services. On the contrary, high values of factor 4 represent marginalized municipalities (see Supplementary Figure 4) with more people depending on the agricultural activities and low per capita GDP (Figure 2). Using these relationships, a composite risk index was constructed. Each of the calculated factors was converted to a Boolean variable according to the sign of the factor's value for each municipality. A value of 1 was assigned to represent a high risk, while zero signifies low risk (Figure 1, see section Multivariate Risk Index; Supplementary Table 1).
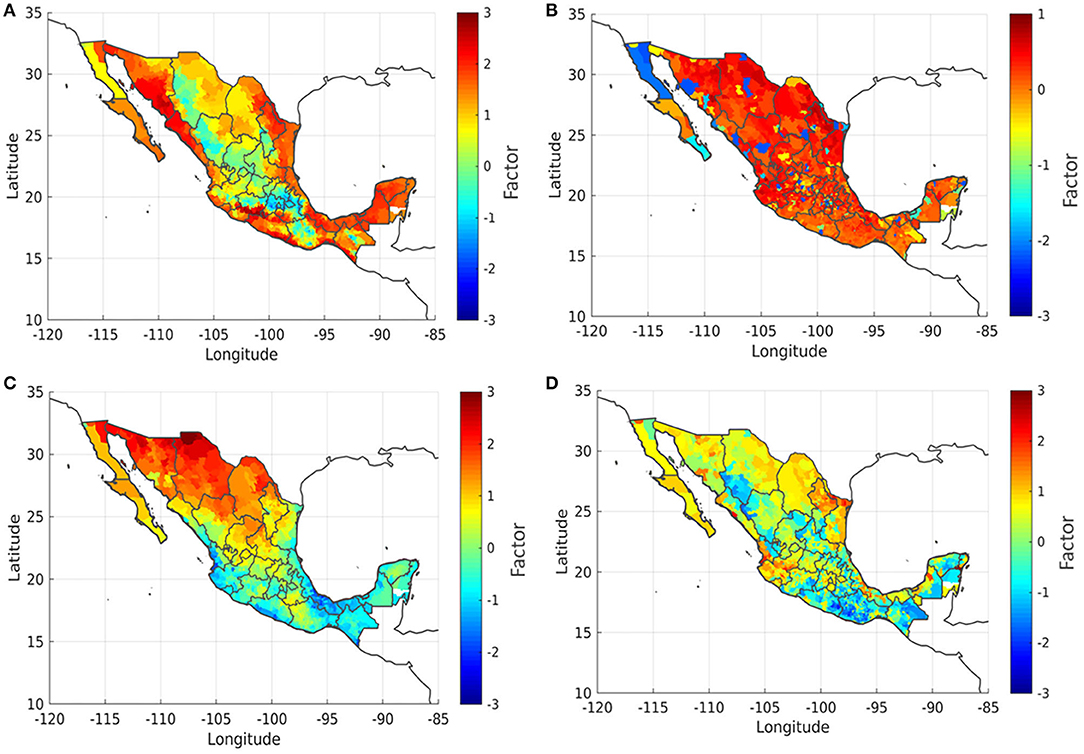
Figure 2. Climatic and Socio-economic factors regionalization. (A) Combination of variables related to temperature, (B) Combination of Population and GDP size, (C) Combination of temperature variables, precipitation, and rainfed maize yield, (D) Combination of Index of marginalization, people working on the primary sector and per capita GDP.
Historical Risk Index
In the 2010's, 37% of the Mexican municipalities (Table 4) showed a very high and high-risk category; these municipalities represent 46.9% of the country and 14.9% of its population. The municipalities at risk are mainly in the north of Mexico (Figure 3). Northern municipalities highlight because they face very high temperatures and high-temperature variability. However, those areas are not recognized as large producers of rainfed maize. All the Mexican states and almost all municipalities grow rainfed maize (95.7%), and the production is concentrated in three states which account for nearly half of the national rainfed maize (48.6%) (Figure 4). The municipalities with the largest rainfed production are in the Pacific Coast (Jalisco), which account for 27.7% of national output, followed by the municipalities of the State of Mexico (11.6%), and those from Chiapas (9.3%). During 2003–2019 the mean yield of rainfed maize was 2.09 ton/ha, and 55.6% of the municipalities (n = 1,308) had lower values than the national mean, and 29.5% of the municipalities (n = 694) showed yields lower than 1.0 ton/ha. Many municipalities (90.5%) in the very high-risk category showed yields lower than 3.0 ton/ha. Municipalities of two states (Chihuahua and Durango) represent 30.6% of the national area in the very high-risk category. Low yields of these municipalities impact their risk jointly with high temperatures, temperature variability, and low precipitation (Figure 2). The trend is similar for the high-risk category, where 80.8% of the municipalities have yields lower than 3.0 ton/ha. Besides, 7.3% of the municipalities in this risk class—mainly located in the north of the country (Chihuahua and Sonora)—do not produce rainfed maize. These municipalities are at risk linked to the bioclimate elements driven by factor 1 and factor 3 (Figure 2). Several municipalities in the north show low yields (less than 3 ton/ha), this in combination with climatic variables (high temperatures and low precipitation), contribute to their high-risk category. The risk decreases in municipalities across the Pacific Coast, and it becomes more heterogeneous toward southern states and the Gulf Coast, including the Yucatán Peninsula. Here, the diversity of risk links to the heterogeneity in terms of the municipalities' social, economic, and urban-rural context. Municipalities in the center of the country stand out for a predominance of low to very low risk, due to the socioeconomic context of large cities and predominantly industrial areas (i.e., State of Mexico, Mexico City, Guanajuato, Tlaxcala) (Supplementary Figure 5).
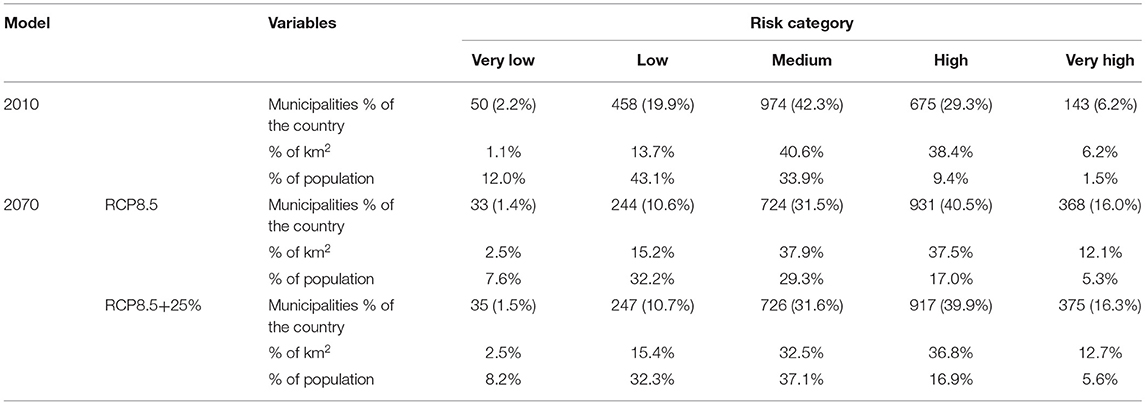
Table 4. Risk categories of municipalities, based on historical data (2010), and the RCP8.5 and RCP8.5+25% scenarios.
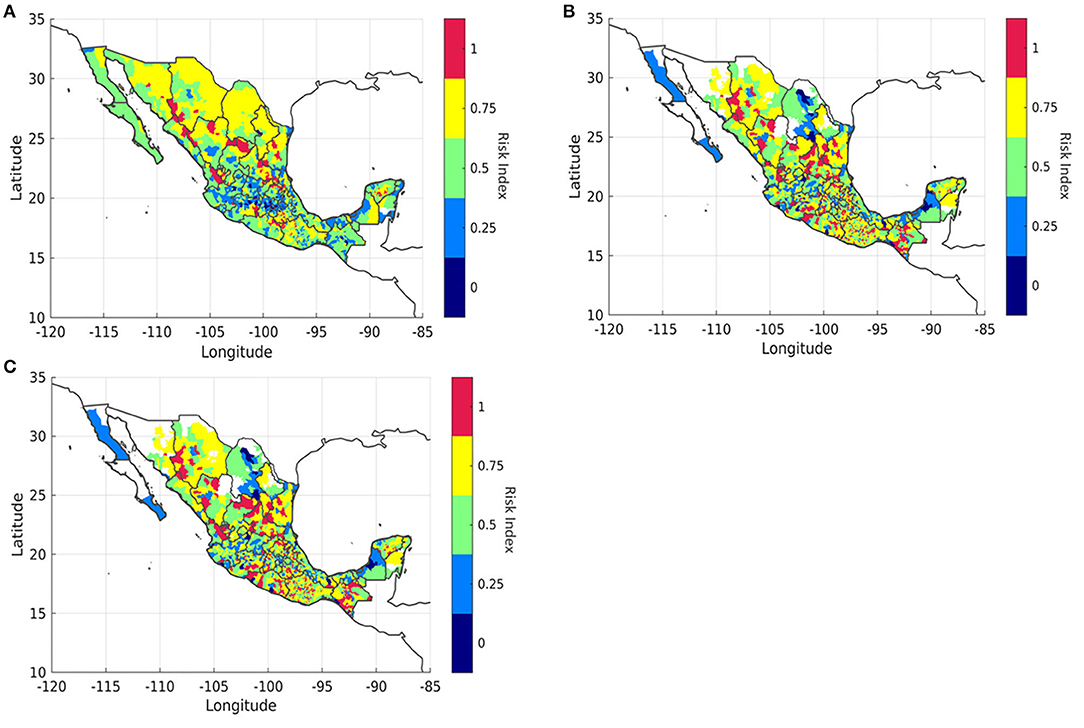
Figure 3. (A) Historical Risk Index, (B) Future Risk Index 2070 and the RCP8.5 scenario, (C) Future Risk Index 2070 and the RCP8.5+25% scenario.
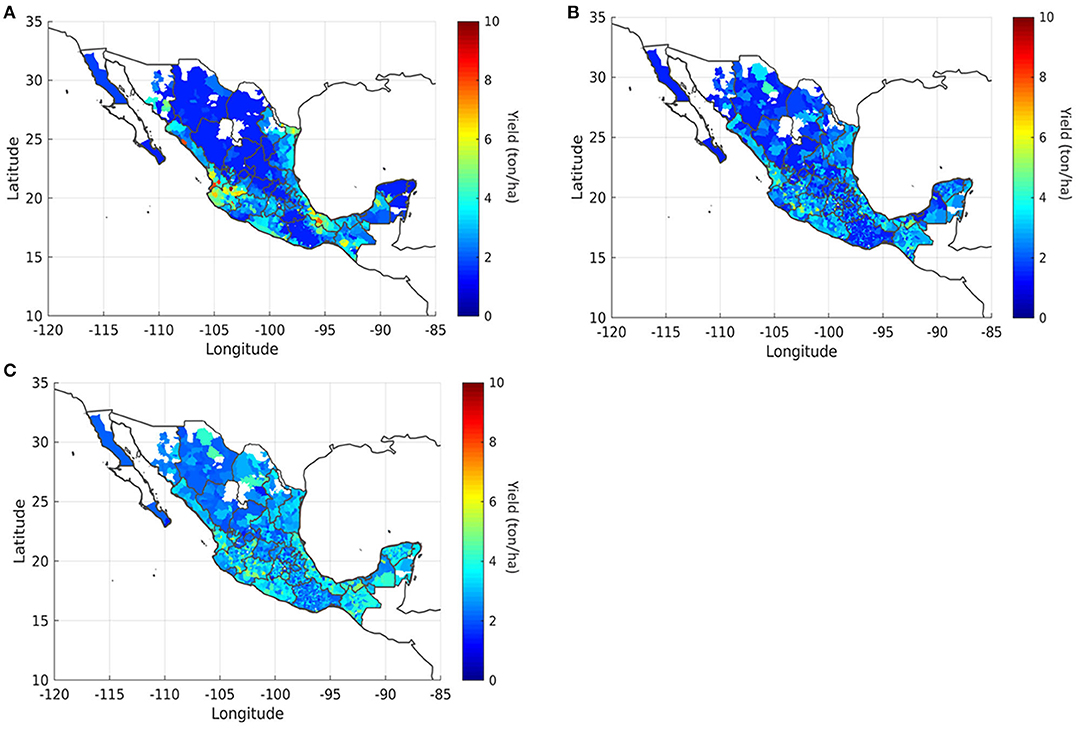
Figure 4. (A) Historical mean rainfed maize yield (2003–2019), (B) Maize future yield 2070 and the RCP 8.5 scenario, (C) Maize future yield 2070 and the RCP8.5+25% scenario.
Future Risk Index
Future Risk With Current Subsidies Level
Future risk for the scenario RCP 8.5 shows that by 2070, 16.0 and 40.5% of Mexico could be at very high-, and high-risk categories, respectively, which includes 4.5 and 14% of the Mexican population (Table 4). Three states account for more than 30% of the very high-risk category, Chihuahua (13.2%), Durango (10.3%), and Chiapas (11.5%). The northern states are at risk mainly due to their increased exposure to high temperatures and low precipitation. Moreover, at a very high-risk class, 87% of the municipalities (n = 320) show maize yields <3.0 ton/ha and 24.7% <1.0 ton/ha. Risk increases in some municipalities in the southern part of the country, in a more dispersed way in Oaxaca state, and central, north, and southern municipalities of Chiapas state (Table 3). Municipalities of Chiapas in the very high-risk category have a historical mean yield of 2.4 ton/ha, and by 2070, it decreases to 1.1 ton/ha. It means that maize producers will face higher risks and challenges imposed not only for climate change but also for its historically high degrees of marginalization in several municipalities of southern states. At a national level, 89.3% of the municipalities reduce their yields, and 370 of them (16.1%) decrease it by more than 70% compared to their historical mean. This reduction seems to be the trend for most of the Mexican rainfed maize producers' municipalities. For instance, the RCP 8.5 shows a national mean yield reduction of 38%, which impacts the risk of the municipalities. In terms of risk related to climate, by 2070, 78.7% of the studied municipalities (n = 1,811) will have increases higher than 3.5°C. Of these, 28 municipalities showed decreases in annual precipitation ≥ 50 mm. The municipalities that are projected to exhibit more constraints for higher temperature tendency and lower reductions in precipitation are in seven states, on the Pacific Coast (Jalisco, Michoacán, and Nayarit), north of Mexico (Chihuahua and Durango), and center of the country (Hidalgo and Morelos) (Supplementary Figures 6, 7 in https://github.com/abiharo/risk_index).
Future Risk With 25% Increase in Subsidies
Although increasing subsidies (i.e., monetary support mainly use for fertilization) are projected to reduce the drop in the mean national yields (14.0 vs. 30.0%), the scenario RCP8.5+25% does not produce differences in future risk in comparison to the RCP8.5 (Figure 4). For instance, there is a slight increase in the percentage of municipalities and extent in the very high-risk category between both scenarios (1.0 and 0.6%, respectively) (Table 4). We suggest that this is due to a non-linear relationship between subsidies and risk categories. The complex and non-linear relationship between maize yields and subsides was also identified in the RF models (Supplementary Figure 5). However, this scenario shows a much lower reduction of mean rainfed maize yield of 14.0%, suggesting that overall socioenvironmental risk would not decrease by augmenting agricultural subsidies to mitigate the yield reduction. That means that the negative impacts of climate change overpass the benefits of the economic support for increasing yields, making evident the complexity to tackle the agricultural risk to climate change. However, for some municipalities, the increment in subsidies modifies their risk category. For example, some municipalities of some states (i.e., Jalisco and Zacatecas) reduce their risk category (Figure 5; Supplementary Figure 5), while others on the Pacific Coast (Guerrero, Oaxaca, and Chiapas) increase their risk (Figure 5; Supplementary Figure 5). These two cases could be an expression of regional socioeconomic conditions that predominantly drive risk in certain municipalities.
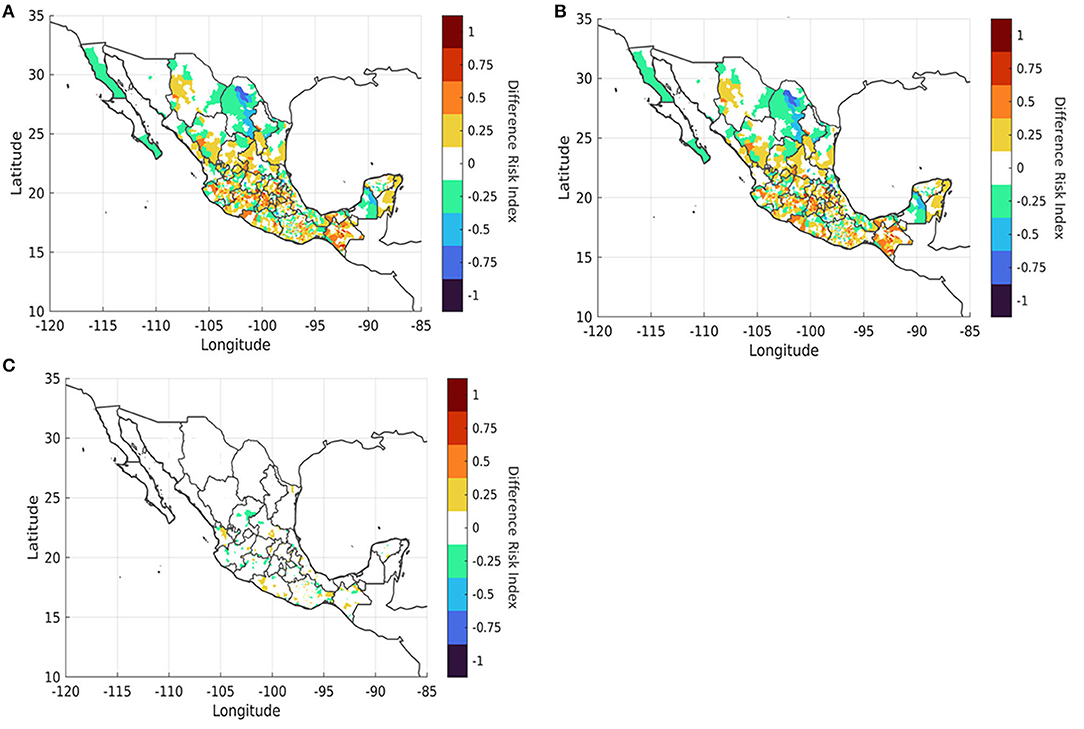
Figure 5. (A) Difference between future Risk RCP8.5 scenario and the Historical Risk, (B) Difference between future Risk RCP8.5+25% scenario and the Historical Risk, (C) Difference between future Risk RCP8.5 and RCP8.5+25% scenarios.
Based on Bellon's et al. (2021) data, we calculated the number of smallholder farmers in the very high- and high-risk municipalities. We found that 1,259 and 4,254 farmers with a mean yield of <1 ton/ha are in these two risk categories, respectively (Table 5). By 2070, the number increased in the RCP 8.5 to 2,085 farmers at very high-risk and 4,323 in high-risk. The scenario RCP8.5+25% shows that an increment of subsidies does not reduce the number of farmers in the very high-risk category. Nevertheless, in the high-risk category, subsidies could slightly alleviate farmers, avoiding that 179 of them fall in this category (Table 5).
Adaptation Strategies for Reducing Socio-Ecological Risk to Climate Change
To decrease the risk through the Mexican municipalities, we propose some adaptation strategies based on the heterogeneity of the municipalities (Table 6). We can notice that municipalities from the northwest region (Supplementary Figure 9), followed by those from the southwest, show the highest risk (Table 6). As we mentioned, the risk in the northern region is strongly driven by climatic elements like high temperatures, low precipitation, and high-temperature variability. We suggest that to reduce the risk, the municipalities should diversify their crops by promoting native races of maize and growing other products that can be more tolerant to heat and water stress. Some transformative adaptation strategies include improving the water management to avoid overexploitation of water tables by exploring some sustainable alternatives. On the contrary, adaptation in municipalities in the Southern region should focus on capacity building to sustainable increase production to alleviate poverty while decreasing the impacts on soil degradation and land-use change. Municipalities of the West and Southwest need to promote their native races of maize, from which some are tolerant to heat conditions. Municipalities from the North Center and East region face threats to both climatic and socioeconomic conditions. For these municipalities, transformative adaptation can include the diversification of economic activities like sustainable forestry or ecotourism. Municipalities of the regions respond differently to the risk, and consequently, adaptation and transformative strategies need to integrate their threats and their context to better implement adaptations to climate change. In most regions, we identified the need for transformative adaptations, particularly in regions with high marginalization levels and the most vulnerable groups, such as indigenous communities and smallholder farmers. To these transformative strategies, we added incremental strategies that are still necessary to face the impacts of climate change.
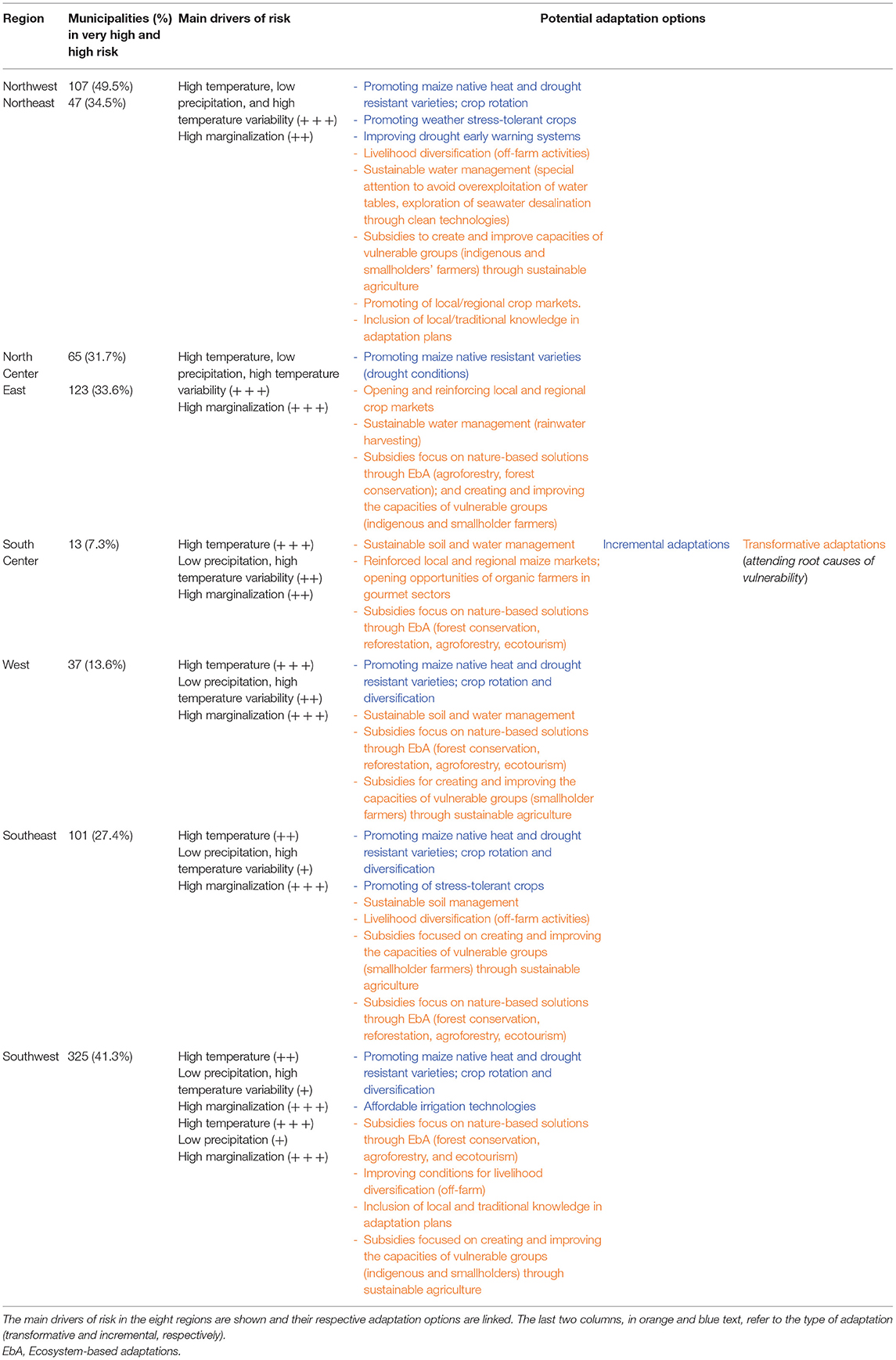
Table 6. Municipalities in the category of very high-risk and high-risk, according to their regionalization.
Discussion
Our analysis shows that more than half of the Mexican municipalities could be at high and very high risk of climate change due to their socio-ecological conditions. The SES approach allows us to identify areas that could be more affected by shifting climate (e.g., increases in extremes temperatures and reduced levels of precipitation) matching with challenging socioeconomic conditions (i.e., poverty, marginalization, and low GDP). The identification of these elements is crucial to develop an effective adaptation policy for the agricultural sector (Fedele et al., 2019). Our findings highlight how spatial risk variation under shifting climates and socioeconomic factors can guide adaptation to prioritize municipalities. There is a very high level of variation among and within the Mexican maize for climate adaptation (Hellin et al., 2014). The general overall climatic ranges for maize included 0 to 2,900 m altitude, 11.3° to 26.6°C annual mean temperature, 12.0° to 29.1°C growing season mean temperature, and 426–4245 mm annual rainfall (Ruiz-Corral et al., 2008). However, it seems that rainfed maize are strongly correlated to annual precipitation and precipitation seasonality (Challenger, 1998; Murray-Tortarolo et al., 2018). The RF models identified that annual precipitation was the second most important predictor in rainfed maize yields (Supplementary Figure 3). Therefore, larger variations in climatic conditions in the future will have a higher impact on rainfed agriculture and farmers' economy. Mexico's geographical characteristics (physical and human) are playing a direct role in the suitability of rainfed maize as we found in our modeling approach implemented here. In arid and semi-arid regions (in northern Mexico), the risk was driven by extreme temperatures, high seasonality, and low precipitation regimes. These regions are more prone to extreme climatic events
(Pontifes et al., 2018). For instance, the north of Mexico is not suitable for rainfed agriculture because of the threat to droughts (mean annual precipitation <350 mm) (Moreno and Huber-Sannwald, 2011). This threat will impact the already low yields (<3.0 ton/ha) which will reduce by climate change (Murray-Tortarolo et al., 2018). To overcome the climatic challenges for the agricultural sector in the north of Mexico, farmers have implemented irrigation management to grow animal feed crops such as sorghum and wheat, leaving staple foods production to the southern and central Mexico (Eakin et al., 2014b). The south seems prone to be affected not only by climate change (Monterroso et al., 2011; López-Blanco et al., 2018; Murray-Tortarolo et al., 2018) but also by socioeconomic constraints. Southern states of Mexico, like Chiapas, have lagged in investment (Fox and Haight, 2010), and the adoption of mechanization is impossible due to topography restrictions (Eakin et al., 2014c). However, Chiapas has many maize low-yield native varieties which subsistence can be related more to the cultural value than the commercial one. These native varieties can explain, in part, the climatically robust varieties of maize in that region (Eakin, 2005; Mercer et al., 2012). That means that Chiapas preserves its native maize varieties, avoiding the commercial varieties (Perales et al., 2003; Brush and Perales, 2007). A priority national strategy should be to study the potential of native varieties to increasing temperatures as an adaptation to climate change. In this context, subsidies should change their aim to support native races in contrast to commercial ones.
The focus on commercial varieties and markets is related to agricultural subsidies based on the global north's economic and commercial model (Pingali, 2007). They do not consider the country's wide socio-environmental heterogeneity, particularly among agricultural groups (Evenson and Gollin, 2003). In Mexico, the national programs to support farmers like The Programa de Apoyos Directos al Campo (PROCAMPO which means, Direct Field Support Programme), does not reach the majority of the most marginalized peasant communities (Fox and Haight, 2010). Also, the Productive Program (PROAGRO) has not positively impacted the smallholder farmers' maize production (Valentín-Garrido et al., 2016). Most of the time, these programs are focused only on a single part of the system, like crop production and profitability. Therefore, they only target large-size farmers with large cultivation areas, irrigation infrastructure, and economic solvency. While PROCAMPO was promoted to facilitate the transition of smallholder farmers of basic grains into higher-value crops, in practice, it has become a steady source of cash income for farmers of all scales (Eakin et al., 2014b).
Five northern states in Mexico have received the largest rural per capita shares of public investment in agriculture, linked to two factors: the large farm and thus larger benefits via PROCAMPO, and support programs that target capital intensive and mechanized agriculture (Scott, 2010; Eakin et al., 2014a). One state (Sinaloa) received over 12.0% of public agriculture subsidies, accounting only for 4.0% of the population in the primary sector. Contrastingly, the State of Mexico receives 3.0% of public expenditures with 5% of people in the same sector. Moreover, the same state (Sinaloa) received 68 and 100% of the budget allocated to two programs to support national agriculture (Ingreso Objetivo program and Cobertura de Precios) between 2006 and 2009 (Appendini, 2014). This highlights the national interest to entail substantial subsidies for commercial agribusiness and the limitation that smallholder farmers face to deal with climate adversities. We integrated subsidies in the future risk assessment to assess the positive impacts in decreasing the risk, but it is to notice that subsidies have promoted intensive use of chemical inputs with high environmental impacts (OECD, 2005). In this study, the increase of the agricultural subsidies seems to positively impact the mean national yield of rainfed maize. However, these augments did not significantly reduce the risk to climate change because of the complexity of elements involved, like the marginalization and poverty in the south and the lack of infrastructure and capacities to sustainably deal with the lack of water in the north of Mexico. We support exploring other alternatives like promoting heat and drought resistant and native varieties, crop rotation and diversification, alternatives to tackle the lack of water like environmentally friendly desalinization of seawater for agriculture, and sustainable technological support to increase crop production.
Our risk index included population and GDP size, which relates to cities that show lower risk. However, there are urban municipalities at high risk not only because of high temperatures but also because some peri-urban areas grow maize (Losada et al., 2011). Besides the urban agricultural risk to climate change, there are other risks related to cities like diseases, pollution (Elmqvist et al., 2013), flooding (Jha et al., 2012), and heatwaves (Li and Bou-Zeid, 2013). However, these elements were not considered in our risk index.
The spatial identification of risk helps identify patterns of drivers that increase the risk to tackle them through specific strategies (Howden et al., 2007), like addressing adaptive management for different regions based on specific contexts. Risk characterization is linked to developing strategies to decrease the negative impacts by increasing the resistance, resilience, mitigation, and adaptation of the SES. Notably, the relationship between risk and adaptation to climate change has been extensively explored under the concept of double exposure (O'Brien and Leichenko, 2000). This concept indicates how the dynamic interrelationship of the social, economic, and political spheres interact with climate.
Our findings highlight the variation of risk across regions due to the combination of climate and socioeconomic factors. We support that transformative adaptation actions should be specific to each municipality to tackle the causes of the vulnerability to climate change (Fedele et al., 2019). Risk and adaptation related to agriculture are not only a matter of increasing agricultural subsidies. Farm subsidies are only one of many incremental actions, which works in the short term because they are mainly used for fertilizer acquisition and not to improve social and ecological conditions in rural areas (Zarazúa-Escobar et al., 2011). Besides, subsidies have adverse effects in the medium and long term, affecting the ecosystem's conditions (e.g., soil and water pollution) and dynamics that generate negative feedbacks (e.g., erosion, pest resistance), sometimes leading the SES toward an unsustainable trajectory (Eriksen et al., 2011; Rivera-Ferre et al., 2013; Fedele et al., 2020). Nevertheless, adaptation should be a combination of transformative strategies like poverty alleviation, capacity building to improve management and water use, sustainable technification, and soil restoration. Moreover, considering that Mexico has more than 59 races of maize (Perales and Golicher, 2014), the country could implement strategies to promote the use of native resistant varieties in some regions, which will be favored by climate change (Ureta et al., 2012). Also, adaptation based on combinations of local and technical knowledge that use traits that cope better with the heat and drought stress of native races should allow to cope better with climate change (Bellon and Hellin, 2011; Hellin et al., 2014). For example, transformative adaptations like sustainable water management in the north of Mexico could reduce the overexploitation of water tables or programs to develop capacities for the most vulnerable groups (indigenous and smallholder farmers) in the south of Mexico. These social programs can integrate agricultural diversification of crops through sustainable production to support an increasing regional or international market (e.g., organic fertilizers, soil improvement). These strategies can complement nature-based solutions (Cohen-Shacham et al., 2016) through ecosystem-based adaptations (i.e., forest conservation, reforestation, agroforestry, and sustainable watershed management). It should be noted that the actions at the municipal level cannot exclude the linking strategies at regional or international policies and plans. If these agendas remain isolated, the implementation of transformative adaptations will be more difficult.
In Mexico, the national climate change policy currently focuses on transformative adaptation programs through nature-based solutions (e.g., Ecosystem-based adaptation, Community-based adaptation) (INECC, 2019). These programs consider the conditions and ecosystem characteristics of which individuals could take advantage of to link social well-being (Jones et al., 2012). Furthermore, at the same time is developing a greater capacity and resilience of the SES to climate change impacts. Moreover, it can improve their livelihoods or even expand them in a sustainable way (Wamsler et al., 2016; Scarano, 2017). We suggest that a way to promote adaptation strategies is to link the distribution of risk to information regarding the distribution and differentiation in environmental conditions, land-use change rates, poverty, economic activities, and areas with a high expansion urban-rural transition. Programs should incorporate local and municipality characteristics to complement adaptation and mitigation strategies. However, there is a lack of linkage between the available information at different levels that impose an extra challenge. There is not yet a database that concentrates data on farms, farmers, and environmental traits. These elements make it challenging to evaluate a complete analysis of risk fully. We support that gathering information at different levels will improve this kind of evaluation.
Conclusions
We developed a municipality risk index using a socio-ecological framework that integrates climatic and socioeconomic data linked to maize production in Mexico. Our results show that currently more than one third of the Mexican municipalities are at very high and high-risk, and by 2070 this will could increase to more than half of the country. The risk in the north of Mexico is principally driven by climate factors like high temperatures, high-temperature variability, and low precipitation. However, in future scenarios, we found that suitable areas for rainfed maize migth experience the synergistic effects of climate change and the challenges of poverty and marginalization. It means that incrementing the agricultural subsidies is not enough to reduce the risk of climate change. Impacts of climate change on agriculture require adaptation plans to address adaptive transformative strategies that consider the SES's complexity, such as poverty alleviation, native resistant varieties, capacity building to improve management and water use, sustainable technification, and soil restoration.
Data Availability Statement
The original contributions presented in the study are included in the article/Supplementary Material, further inquiries can be directed to the corresponding author/s.
Author Contributions
AH, AM-P, and FE developed the idea. FE, ÓC-B, and JV processed and analyzed the data. AH, AM-P, FE, and JV wrote the paper. All authors contributed to the article and approved the submitted version.
Funding
This work was supported by UNAM-DGAPA Grant No. IN111221 and IT200618. JV acknowledges support from PINCC-UNAM project grants 2020. AM-P acknowledges support from UNAM-DGAPA.
Conflict of Interest
The authors declare that the research was conducted in the absence of any commercial or financial relationships that could be construed as a potential conflict of interest.
Acknowledgments
AH thanks the Ph.D. Program in Sustainability Sciences of the National Autonomous University of Mexico (UNAM). And to the Consejo Nacional de Ciencia y Tecnología (CONACYT) for the offering the scholarship (No. 728645).
Supplementary Material
The Supplementary Material for this article can be found online at: https://www.frontiersin.org/articles/10.3389/fclim.2021.674693/full#supplementary-material
Footnotes
3. ^https://www.suri.agricultura.gob.mx:8017/buscadorBeneficiario
4. ^http://www.conabio.gob.mx/informacion/gis/
6. ^https://www.worldclim.org/data/worldclim21.html#
7. ^Further details on predictive accuracy of Random Forest models and dependence plots are in the (Supplementary Figure 8).
References
Adger, W. N. (2006). Vulnerability. Glob. Environ. Change 16, 268–281. doi: 10.1016/j.gloenvcha.2006.02.006
Appendini, K. (2014). Reconstructing the maize market in Rural Mexico. J. Agrarian Change 14, 1–25. doi: 10.1111/joac.12013
Bellon, M. R., and Hellin, J. (2011). Planting hybrids, keeping landraces: agricultural modernization and tradition among small-scale maize farmers in Chiapas, Mexico. World Dev. 39, 1434–1443. doi: 10.1016/j.worlddev.2010.12.010
Bellon, M. R., Mastretta-Yanes, A., Ponce-Mendoza, A., Ortiz-Santa María, D., Oliveros-Galindo, O., Perales, H., et al. (2021). Beyond subsistence: the aggregate contribution of campesinos to the supply and conservation of native maize across Mexico. Food Security 13, 39–53. doi: 10.1007/s12571-020-01134-8
Bellon, M. R., Mastretta-Yanes, A., Ponce-Mendoza, A., Ortiz-Santamaría, D., Oliveros-Galindo, O., Perales, H., et al. (2018). Evolutionary and food supply implications of ongoing maize domestication by Mexican campesinos. Proc. Royal Soc. B Biol. Sci. 285:20181049. doi: 10.1098/rspb.2018.1049
Bennett, N. J., Blythe, J., Tyler, S., and Ban, N. C. (2016). Communities and change in the anthropocene: understanding social-ecological vulnerability and planning adaptations to multiple interacting exposures. Region. Environ. Change 16, 907–926. doi: 10.1007/s10113-015-0839-5
Brush, S. B., and Perales, H. R. (2007). A maize landscape: ethnicity and agro-biodiversity in Chiapas Mexico. Agri. Ecosyst. Environ. 121, 211–221. doi: 10.1016/j.agee.2006.12.018
Chakraborty, T., Hsu, A., Manya, D., and Sheriff, G. (2019). Disproportionately higher exposure to urban heat in lower-income neighborhoods: a multi-city perspective. Environ. Res. Lett. 14:105003. doi: 10.1088/1748-9326/ab3b99
Challenger, A. (1998). Utilización y conservación de los ecosistemas terrestres de México: Pasado, presente y futuro. Mexico City: Conabio-Instituto de Biología, UNAM, Agrupación Sierra Madre.
Cohen-Shacham, E., Walters, G., Janzen, C., and Maginnis, S. (2016). Nature-based Solutions to address global societal challenges. Gland: IUCN. doi: 10.2305/IUCN.CH.2016.13.en
Cohn, A., Vanwey, L., Spera, S., and Mustard, J. (2016). Cropping frequency and area response to climate variability can exceed yield response. Nat. Climate Change 6:2934. doi: 10.1038/nclimate2934
CONABIO (2012). 'Actividades económicas en México por municipio, 2010', escala: 1:250000. Comisión Nacional para el Conocimiento y Uso de la Biodiversidad. México: Datos estadísticos del 2010, del Instituto Nacional de Estadísitca y Geografía (INEGI).
CONAPO (2010). “Indice de Marginación Municipal,” in Available online at: http://www.conapo.gob.mx/es/CONAPO/Indices_de_Marginacion_2010_por_entidad_federativa_y_municipio (accessed December 12, 2020), 54.
De Salvo, M., Begalli, D., and Signorello, G. (2013). Measuring the effect of climate change on agriculture: a literature review of analytical models. J. Dev. Agri. Econ. 5, 499–509. doi: 10.5897/JDAE2013.0519
Díaz, S., Pascual, U., Stenseke, M., Martín-López, B., Watson, R. T., Molnár, Z., et al. (2018). Assessing nature's contributions to people. Science 359:270. doi: 10.1126/science.aap8826
Donatti, C. I., Harvey, C. A., Martinez-Rodriguez, M. R., Vignola, R., and Rodriguez, C. M. (2019). Vulnerability of smallholder farmers to climate change in Central America and Mexico: current knowledge and research gaps. Climate Dev. 11, 264–286. doi: 10.1080/17565529.2018.1442796
Eakin, H. (2000). Smallholder maize production and climatic risk: a case study from Mexico. Climatic Change 45, 19–36. doi: 10.1023/A:1005628631627
Eakin, H. (2005). Institutional change, climate risk, and rural vulnerability: cases from Central Mexico. World Dev. 33, 1923–1938. doi: 10.1016/j.worlddev.2005.06.005
Eakin, H., Bausch, J. C., and Sweeney, S. (2014a). Agrarian winners of neoliberal reform: the “maize boom” of Sinaloa, Mexico. J. Agrarian Change 14, 26–51. doi: 10.1111/joac.12005
Eakin, H., Perales, H., Appendini, K., and Sweeney, S. (2014b). Selling maize in Mexico: the persistence of peasant farming in an era of global markets. Dev. Change 45, 133–155. doi: 10.1111/dech.12074
Eakin, H., Sweeney, S., Lerner, A. M., Appendini, K., Perales, H., Steigerwald, D. G., et al. (2018). Agricultural change and resilience: agricultural policy, climate trends and market integration in the Mexican maize system. Anthropocene 23, 43–52. doi: 10.1016/j.ancene.2018.08.002
Eakin, H. C., Lemos, M. C., and Nelson, D. R. (2014c). Differentiating capacities as a means to sustainable climate change adaptation. Glob. Environ. Change 27, 1–8. doi: 10.1016/j.gloenvcha.2014.04.013
Elmqvist, T., Redman, C. L., Barthel, S., and Costanza, R. (2013). “History of urbanization and the missing ecology,” in Urbanization, Biodiversity and Ecosystem Services: Challenges and Opportunities: A Global Assessment, eds T. Elmqvist, M. Fragkias, J. Goodness, B. Güneralp, P. J. Marcotullio, R. I. McDonald, S. Parnell, M. Schewenius, M. Sendstad, K. C. Seto and C. Wilkinson (Dordrecht: Springer Netherlands), 13–30. doi: 10.1007/978-94-007-7088-1_2
Eriksen, S., Aldunce, P., Martins, R., Sygna, L., Ulsrud, K., O'Brien, K., et al. (2011). When not every response to climate change is a good one: identifying principles for sustainable adaptation. Climate Dev. 3, 7–20. doi: 10.3763/cdev.2010.0060
Estrada, F., Velasco, J. A., Martinez-Arroyo, A., and Calderón-Bustamante, O. (2020). An analysis of current sustainability of Mexican cities and their exposure to climate change. Front. Environ. Sci. 8:25. doi: 10.3389/fenvs.2020.00025
Evenson, R. E., and Gollin, D. (2003). Assessing the impact of the green revolution, 1960 to 2000. Science 300:758. doi: 10.1126/science.1078710
Everingham, Y., Sexton, J., Skocaj, D., and Inman-Bamber, G. (2016). Accurate prediction of sugarcane yield using a random forest algorithm. Agron. Sustain. Dev. 36:27. doi: 10.1007/s13593-016-0364-z
Fedele, G., Donatti, C. I., Harvey, C. A., Hannah, L., and Hole, D. G. (2019). Transformative adaptation to climate change for sustainable social-ecological systems. Environ. Sci. Policy 101, 116–125. doi: 10.1016/j.envsci.2019.07.001
Fedele, G., Donatti, C. I., Harvey, C. A., Hannah, L., and Hole, D. G. (2020). Limited use of transformative adaptation in response to social-ecological shifts driven by climate change. Ecol. Soc. 25:125. doi: 10.5751/ES-11381-250125
Feng, S., Oppenheimer, M., and Schlenker, W. (2015). Weather Anomalies, Crop Yields, and Migration in the US Corn Belt. New York, NY: S. U. o. F. a. E. School of Economics, Department of Geosciences and Woodrow Wilson School Princeton University and School of International and Public Affairs (SIPA) and The Earth Institute, Columbia University.
Fox, J., and Haight, L. (2010). Subsidios para la desigualdad. Las políticas del maíz en México a partir del libre comercio. México: Woodrow Wilson International Center for Scholars.
Fuller, A., Min, Q., Jiao, W., and Bai, Y. (2015). Globally Important Agricultural Heritage Systems (GIAHS) of China: the challenge of complexity in research. Ecosyst. Health Sustain. 1:art6. doi: 10.1890/EHS14-0007.1
Galeana-Pizaña, J. M., Couturier, S., Figueroa, D., and Jiménez, A. D. (2021). Is rural food security primarily associated with smallholder agriculture or with commercial agriculture? an approach to the case of Mexico using structural equation modeling. Agri. Syst. 190:103091. doi: 10.1016/j.agsy.2021.103091
Hastie, T., Tibshirani, R., Friedman, J., and Franklin, J. (2004). The elements of statistical learning: data mining, inference, and prediction. Math. Intell. 27, 83–85. doi: 10.1007/BF02985802
Hellin, J., Bellon, M. R., and Hearne, S. J. (2014). Maize landraces and adaptation to climate change in Mexico. J. Crop Improv. 28, 484–501. doi: 10.1080/15427528.2014.921800
Hijmans, R., Cameron, S., Parra, J., Jones, P., and Jarvis, A. (2005). Very high resolution interpolated climate surfaces for global land areas. Int. J. Climatol. 25, 1965–1978. doi: 10.1002/joc.1276
Howden, S. M., Soussana, J.-F., Tubiello, F. N., Chhetri, N., Dunlop, M., and Meinke, H. (2007). Adapting agriculture to climate change. Proc. Natl. Acad. Sci. U. S. A. 104:19691. doi: 10.1073/pnas.0701890104
INECC (2019). Criterios para el monitoreo y evaluación de las medidas de adaptación al cambio climático. Nota técnica. Proyecto “Construcción de esquemas de monitoreo y evaluación de la adaptación en México para la formulación de políticas públicas basadas en evidencia” (INECC-CONACYT). México: Instituto Nacional de Ecología y Cambio Climático (INECC).
INEGI (2010). Censos de Población y Vivienda. Available online at: https://www.inegi.org.mx/programas/ccpv/2010/#Microdatos (accessed October 10, 2020).
IPCC (2014). Climate Change 2014: Impacts, Adaptation, and Vulnerability. Part A: Global and Sectoral Aspects. Contribution of Working Group II to the Fifth Assessment Report of the Intergovernmental Panel on Climate Change. Cambridge, New York, NY.
Jeong, J., Resop, J., Mueller, N., Fleisher, D., Yun, K., Butler, E., et al. (2016). Random forests for global and regional crop yield predictions. PLoS ONE 11:e0156571. doi: 10.1371/journal.pone.0156571
Jha, A., Bloch, R., and Lamond, J. (2012). “Cities and Flooding : A Guide to Integrated Urban Flood Risk Management for the 21st Century. World Bank. doi: 10.1596/978-0-8213-8866-2 Available online at: https://openknowledge.worldbank.org/handle/10986/2241 (accessed January 17, 2021).
Johnson, R., and Wichern, D. (2007). Applied Multivariate Statistical Analysis. Essex: Pearson Prentice Hall.
Jones, H. P., Hole, D. G., and Zavaleta, E. S. (2012). Harnessing nature to help people adapt to climate change. Nat. Climate Change 2, 504–509. doi: 10.1038/nclimate1463
Kotzee, I., and Reyers, B. (2016). Piloting a social-ecological index for measuring flood resilience: a composite index approach. Ecol. Indicat. 60, 45–53. doi: 10.1016/j.ecolind.2015.06.018
Leichenko, R., and Silva, J. (2014). Climate change and poverty: vulnerability, impacts, and alleviation strategies. Wiley Interdiscipl. Rev. Climate Change 5:287. doi: 10.1002/wcc.287
Li, D., and Bou-Zeid, E. (2013). Synergistic interactions between Urban Heat Islands and heat waves: the impact in cities is larger than the sum of its parts. J. Appl. Meteorol. Climatol. 52, 2051–2064. doi: 10.1175/JAMC-D-13-02.1
Liu, J., Dietz, T., Carpenter, S. R., Alberti, M., Folke, C., Moran, E., et al. (2007). Complexity of coupled human and natural systems. Science 317:1513. doi: 10.1126/science.1144004
López-Blanco, J., Pérez-Damián, J. L., Conde-Álvarez, A. C., Gómez-Díaz, J. D., and Monterroso-Rivas, A. I. (2018). Land suitability levels for rainfed maize under current conditions and climate change projections in Mexico. Outlook Agri. 47, 181–191. doi: 10.1177/0030727018794973
Losada, H., Rivera, J., Cortes, J., and Vieyra, J. (2011). Urban agriculture in the metropolitan area of Mexico city. Field Actions Sci. Rep. 5. Available online at: http://journals.openedition.org/factsreports/781
Maass, M. (2017). Integrating food-water-energy research through a socio-ecosystem approach. Front. Environ. Sci. 5:48. doi: 10.3389/fenvs.2017.00048
Márquez-Ramos, A., Prado, L., Elis, D., Nunes, W., Cordeiro, D., Pereira, L., et al. (2020). A random forest ranking approach to predict yield in maize with uav-based vegetation spectral indices. Comput. Electr. Agri. 178:105791. doi: 10.1016/j.compag.2020.105791
Mercer, K. L., Perales, H. R., and Wainwright, J. D. (2012). Climate change and the transgenic adaptation strategy: smallholder livelihoods, climate justice, and maize landraces in Mexico. Glob. Environ. Change 22, 495–504. doi: 10.1016/j.gloenvcha.2012.01.003
Monterroso, R., Conde, A., Rosales, D., Gomez, D., and Gay, G. (2011). Assessing current and potential rainfed maize suitability under climate change scenarios in México. Atmósfera 24, 53–67.
Moreno, T. A., and Huber-Sannwald, E. (2011). “Impacts of drought on agriculture in Northern Mexico,” in Coping with Global Environmental Change, Disasters and Security: Threats, Challenges, Vulnerabilities and Risks, eds H. G. Brauch, Ú. Oswald Spring, C. Mesjasz, J. Grin, P. Kameri-Mbote, B. Chourou, P. Dunay, and J. Birkmann (Berlin, Heidelberg: Springer Berlin Heidelberg), 875–891. doi: 10.1007/978-3-642-17776-7_51
Murray-Tortarolo, G. J, Jaramillo, V., and Larsen, J. (2018). Food security and climate change: the case of rainfed maize production in Mexico. Agri. Forest Meteorol. 253–254, 124–131. doi: 10.1016/j.agrformet.2018.02.011
Myers, S. S., Smith, M. R., Guth, S., Golden, C. D., Vaitla, B., Mueller, N. D., et al. (2017). Climate change and global food systems: potential impacts on food security and undernutrition. Ann. Rev. Public Health 38, 259–277. doi: 10.1146/annurev-publhealth-031816-044356
NeSmith, D. S., and Ritchie, J. T. (1992). Maize (Zea mays L.) response to a severe soil water-deficit during grain-filling. Field Crops Res. 29, 23–35. doi: 10.1016/0378-4290(92)90073-I
O'Brien, K. L., and Leichenko, R. M. (2000). Double exposure: assessing the impacts of climate change within the context of economic globalization. Glob. Environ. Change 10, 221–232. doi: 10.1016/S0959-3780(00)00021-2
OECD (2005). Environmentally harmful subsidies. Challenges for reform. Organ. Econ. Co-operation Dev. 153. doi: 10.1787/9789264012059-en
O'Lenic, E. A., and Livezey, R. E. (1988). Practical considerations in the use of rotated principal component analysis (RPCA) in diagnostic studies of upper-air height fields. Monthly Weather Rev. 116, 1682–1689. doi: 10.1175/1520-0493(1988)116 <1682:PCITUO>2.0.CO;2
Olsson, L., Opondo, M., Tschakert, P., Agrawal, A., Eriksen, S., Ma, S., et al. (2014). “Livelihoods and poverty,” in Climate Change 2014: Impacts, Adaptation, and Vulnerability. Part A: Global and Sectoral Aspects. Contribution of Working Group II to the Fifth Assessment Report of the Intergovernmental Panel on Climate Change, eds C. B. Field, V. R. Barros, D. J. Dokken, K. J. Mach, M. D. Mastrandrea, T. E. Bilir, et al. (Cambridge; New York, NY: Cambridge University Press), 793–832.
Perales, H., Brush, S. B., and Qualset, C. O. (2003). Landraces of maize in Central Mexico: an altitudinal transect. Econ. Bot. 57, 7–20. doi: 10.1663/0013-0001(2003)0570007:LOMICM2.0.CO;2
Perales, H., and Golicher, D. (2014). Mapping the diversity of maize races in Mexico. PLoS ONE 9:e114657. doi: 10.1371/journal.pone.0114657
Pingali, P. (2007). Agricultural growth and economic development: a view through the globalization lens. Agri. Econ. 37, 1–12. doi: 10.1111/j.1574-0862.2007.00231.x
Pontifes, P. A., García-Meneses, P. M., Gómez-Aíza, L., Monterroso-Rivas, A. I., and Caso-Chávez, M. (2018). Land use/land cover change and extreme climatic events in the arid and semi-arid ecoregions of Mexico. Atmósfera 31, 355–372. doi: 10.20937/ATM.2018.31.04.04
Porter, J., Xie, L., Challinor, A., Cochrane, K., Howden, S., Iqbal, M., et al. (2014). “Food security and food production systems,” in Climate Change 2014: Impacts, Adaptation, and Vulnerability. Part A: Global and Sectoral Aspects. Contribution of Working Group II to the Fifth Assessment Report of the Intergovernmental Panel on Climate Change, eds C. B. Field, V. R. Barros, D. J. Dokken, K. J. Mach, M. D. Mastrandrea, T. E. Bilir, et al. (Cambridge: Cambridge University Press; New York, NY: New York Press), 485–533.
Ray, D., West, P., Clark, M., Gerber, J., Prishchepov, A., and Chatterjee, S. (2019). Climate change has likely already affected global food production. PLoS ONE 14:e0217148. doi: 10.1371/journal.pone.0217148
Reardon, T., Stamoulis, K., and Pingali, P. (2007). Rural nonfarm employment in developing countries in an era of globalization. Agri. Econ. 37, 173–183. doi: 10.1111/j.1574-0862.2007.00243.x
Rivera-Ferre, M., Ortega, M., and Baumgärtner, J. (2013). Rethinking study and management of agricultural systems for policy design. Sustainability 5, 3858–3875. doi: 10.3390/su5093858
Rosenzweig, C., Elliott, J., Deryng, D., Ruane, A. C., Müller, C., Arneth, A., et al. (2014). Assessing agricultural risks of climate change in the twenty-first century in a global gridded crop model intercomparison. Proc. Natl. Acad. Sci. U. S. A. 111:3268. doi: 10.1073/pnas.1222463110
Ruiz-Corral, J., Durán, N., Sánchez, J., Ron, J., González, D., Holland, J., et al. (2008). Climatic adaptation and ecological descriptors of 42 Mexican Maize Races. Crop Sci. 48, 1502–1512. doi: 10.2135/cropsci2007.09.0518
Scarano, F. R. (2017). Ecosystem-based adaptation to climate change: concept, scalability and a role for conservation science. Perspectiv. Ecol. Conserv. 15, 65–73. doi: 10.1016/j.pecon.2017.05.003
Scott, J. (2010). Subsidios agrícolas en México: ¿quién gana, y cuánto? en Subsidios para la desigualdad. Las políticas públicas del maíz en México a partir del libre comercio. Jonathan Fox and Libby Haight (coord). Santa Cruz, CA: Woodrow Wilson International Center for Scholars, Centro de Investigación y Docencia Económicas, University of California, 73–128.
SIAP (2018). Servicio de Información Agroalimentaria y Pesquera (SIAP). Sistema de Información Agroalimentaria y de Consulta (SIACON). Mexico City: Secretaría de Agricultura, Ganadería, Desarrollo Social, Pesca y Alimentación (SAGARPA).
SNIM (2013). Base de Datos del PIB Municipal 2005. Sistema Nacional de Información Municipal. Available online at: http://www.snim.rami.gob.mx/ (accessed July 20, 2020).
Stevens, T., and Madani, K. (2016). Future climate impacts on maize farming and food security in Malawi. Sci. Rep. 6:36241. doi: 10.1038/srep36241
Turner, B. L., Kasperson, R. E., Matson, P. A., McCarthy, J. J., Corell, R. W., Christensen, L., et al. (2003). Science and Technology for Sustainable Development Special Feature: a framework for vulnerability analysis in sustainability science. Proc. Natl. Acad. Sci. U. S. A. 100, 8074–8079. doi: 10.1073/pnas.1231335100
Ureta, C., González, E. J., Espinosa, A., Trueba, A., Piñeyro-Nelson, A., and Álvarez-Buylla, E. R. (2020). Maize yield in Mexico under climate change. Agri. Syst. 177:102697. doi: 10.1016/j.agsy.2019.102697
Ureta, C., Martínez-Meyer, E., Perales, H. R., and Álvarez-Buylla, E. R. (2012). Projecting the effects of climate change on the distribution of maize races and their wild relatives in Mexico. Glob. Change Biol. 18, 1073–1082. doi: 10.1111/j.1365-2486.2011.02607.x
Valentín-Garrido, J. M., León-Merino, A., Hernández-Juárez, M., Sangerman-Jarquín, D. M., and Valtierra-Pacheco, E. (2016). Evaluación del programa PROAGRO productivo en comunidades rurales de la sierra norte de Puebla. Revista Mexicana de Ciencias Agrícolas 7, 413–425. doi: 10.29312/remexca.v7i2.354
Wamsler, C., Niven, L., Beery, T. H., Bramryd, T., Ekelund, N., Jönsson, K. I., et al. (2016). Operationalizing ecosystem-based adaptation: harnessing ecosystem services to buffer communities against climate change. Ecol. Soc. 21:131. doi: 10.5751/ES-08266-210131
Wilks, D. S. (2011). Statistical Methods in the Atmospheric Sciences, 3rd ed., Methods. doi: 10.1016/S1572-0934(11)04009-1
Keywords: socio-ecological system, maize, risk assessment, Mexico, climate change
Citation: Haro A, Mendoza-Ponce A, Calderón-Bustamante Ó, Velasco JA and Estrada F (2021) Evaluating Risk and Possible Adaptations to Climate Change Under a Socio-Ecological System Approach. Front. Clim. 3:674693. doi: 10.3389/fclim.2021.674693
Received: 01 March 2021; Accepted: 11 May 2021;
Published: 10 June 2021.
Edited by:
Robyn Wilson, The Ohio State University, United StatesReviewed by:
Roopam Shukla, Potsdam Institute for Climate Impact Research (PIK), GermanyJeetendra Aryal, International Maize and Wheat Improvement Center, Mexico
Copyright © 2021 Haro, Mendoza-Ponce, Calderón-Bustamante, Velasco and Estrada. This is an open-access article distributed under the terms of the Creative Commons Attribution License (CC BY). The use, distribution or reproduction in other forums is permitted, provided the original author(s) and the copyright owner(s) are credited and that the original publication in this journal is cited, in accordance with accepted academic practice. No use, distribution or reproduction is permitted which does not comply with these terms.
*Correspondence: Francisco Estrada, ZmVwb3JydWFAYXRtb3NmZXJhLnVuYW0ubXg=
†These authors have contributed equally to this work and share first authorship