- 1Institute for Oceanography, Center for Earth System Research and Sustainability, Universität Hamburg, Hamburg, Germany
- 2Helmholtz-Zentrum Hereon, Institute of Coastal Systems-Analysis and Modeling, Geesthacht, Germany
- 3International Max Planck Research School on Earth System Modelling, Max Planck Institute for Meteorology, Hamburg, Germany
- 4École Normale Supérieure, LMD, Institut Pierre Simon Laplace (IPSL), Paris, France
- 5Sorbonne Universités (SU/CNRS/IRD/MNHN), LOCEAN Laboratory, Institut Pierre Simon Laplace (IPSL), Paris, France
We combine a machine learning method and ensemble climate predictions to investigate windows of opportunity for seasonal predictability of European summer climate associated with the North Atlantic jet stream. We particularly focus on the impact of North Atlantic spring sea surface temperatures (SST) on the four dominant atmospheric teleconnections associated with the jet stream: the summer North Atlantic Oscillation (NAO) in positive and negative phases, the Atlantic Ridge (At. Ridge), and Atlantic Low (At. Low). We go beyond standard forecast practices by not only identifying these atmospheric teleconnections and their SST precursors but by making use of these identified precursors in the analysis of a dynamical forecast ensemble. Specifically, we train the neural network-based classifier Self-Organizing Maps (SOM) with ERA-20C reanalysis and combine it with model simulations from the Max Planck Institute Earth System Model in mixed resolution (MPI-ESM-MR). We use two different sets of 30-member hindcast ensembles initialized every May, one for training and evaluation between 1902 and 2008, and one for verification between 1980–2016, respectively. Among the four summer atmospheric teleconnections analyzed here, we find that At. Ridge simulated by MPI-ESM-MR shows the best agreement with ERA-20C, thereby representing with its occurrence windows of opportunity for skillful summer predictions. Conversely, At. Low shows the lowest agreement, which might limit the model skill for early warning of warmer than average summers. In summary, we find that spring SST patterns identified with a SOM analysis can be used to guess the dominant summer atmospheric teleconnections at initialization and guide a sub-selection of potential skillful ensemble members. This holds especially true for At. Ridge and At. Low and is unclear for summer NAO. We show that predictive skill in the selected ensemble exceeds that of the full ensemble over regions in the Euro-Atlantic domain where spring SST significantly correlates with summer sea level pressure (SLP). In particular, we find a significant improvement in predictive skill for SLP, geopotential height at 500 hPa, and 2 m temperature at 3–4 months lead time over Scandinavia, which is robust among the two sets of hindcast ensembles.
1. Introduction
Seasonal predictability of European summer climate is closely linked to the leading modes of atmospheric teleconnections associated with the North Atlantic jet stream. In the Euro-Atlantic region, the jet stream controls the location of the storm track and modulates the occurrence of weather systems, thus acting as a dynamical control for large-scale temperature and precipitation regimes (e.g., Bladé et al., 2012; Dong et al., 2013). Yet, current state-of-the-art seasonal prediction systems often show biased representations of the jet stream strength and position (Beverley et al., 2019), posing a constraint to the skillful prediction of large-scale features of the summer climate in the North Atlantic-European sector a season ahead (e.g., Dunstone et al., 2016). A further limitation is that dynamical seasonal prediction systems tend to produce overdispersive ensembles, for which the forecast uncertainty is higher than the forecast error (Ho et al., 2013). In contrast, relatively small forecast uncertainties presumably indicate more predictable climate states, which in turn reveal windows of opportunity for more skillful forecasts (Mariotti et al., 2020). Identifying conditions that favor more predictable states—the aim of the present study—is hence a crucial step to achieving improved seasonal forecasts.
Here, we go beyond standard forecast practices by combining an ensemble seasonal prediction system with the neural network-based classifier Self-Organizing Maps (SOM) (Kohonen, 1984). This approach identifies a sub-ensemble in which simulated North Atlantic sea surface temperatures (SST) at the initialisation of the prediction system (i.e., April) are linked to the seasonal predictability of the two dominant modes of variability associated with the North Atlantic jet stream: the summer North Atlantic Oscillation (NAO) and East Atlantic Pattern (EA) (e.g., Folland et al., 2009; Bastos et al., 2016).
Several studies suggested an influence of spring North Atlantic SST on the predictability of NAO and EA. Neddermann et al. (2018) showed that tropical North Atlantic SST in spring can be a predictor for a zonal pressure difference mode that resembles the EA, while (Ossó et al., 2018) found that the source of predictability for the EA lies in the temperature gradient between subpolar and subtropical gyres. Gastineau and Frankignoul (2015) and Hall et al. (2017) suggested that a similar temperature gradient may influence the predictability of NAO as well. Going beyond these studies, we use North Atlantic SST patterns in spring as predictors for both the NAO and the EA.
Traditionally, NAO and EA are defined as the first two empirical orthogonal functions (EOF) of summer sea level pressure (SLP) in the Euro-Atlantic region (Barnston and Livezey, 1987; Folland et al., 2009). Cassou et al. (2005) proposed an alternative approach using k-means clustering (k = 4) and defined four modes of summer variability: the NAO in positive and negative phases, and the Atlantic Ridge (At. Ridge), and Atlantic Low (At. Low). While the At. Low resembles the positive phase of the EA (Barnston and Livezey, 1987), the At. Ridge resembles the negative phase. NAO in a positive phase and the At. Low are associated with warmer and drier conditions in northern and central Euro-Atlantic regions and colder and wetter conditions over the south. Generally, a negative NAO phase and At. Ridge shows a reverse pattern (e.g., Cassou et al., 2005).
In this study, we use SOM as an alternative tool to EOF to identify the main atmospheric teleconnections. We compare how well simulations with the Max Planck Institute Earth System Model in mixed resolution (MPI-ESM-MR) represent the spatial and temporal variability of SLP and its co-variability with spring SST in the Euro-Atlantic sector. Specifically, we use two different sets of 30-member hindcast ensembles initialized every May, one for training and evaluation between 1902 and 2008, and one for verification between 1980 and 2016, respectively. We give particular focus to the influence of specific SST patterns in spring on the seasonal predictability of the main atmospheric teleconnections.
We adopt a SOM perspective over traditional EOF analysis for two main reasons. First, a clear limitation for EOF is that all decomposed basis vectors must be orthogonal, which may lead to nonphysical or blended patterns (e.g., Reusch et al., 2005). Second, an EOF analysis requires stationarity, which cannot be assumed for a century-long analysis of the North Atlantic jet stream, and likely neither to the SST-SLP relationship investigated here (e.g., Woollings et al., 2015; Weisheimer et al., 2019; Rieke et al., 2021).
Besides neglecting orthogonality and stationarity assumptions, SOM provides advantages to visualize and interpret spatial and temporal variability associated with the data. It assumes that the data exist on a continuum instead of in distinct categories, which are organized such that similar SOM modes are displayed close together in the SOM map. This fine classification allows for efficient application of SOM to explore large-scale, slow varying processes, with several successful applications for climate characterisation (e.g., Polo et al., 2011; Johnson, 2013). Furthermore, SOM has been recently applied as an assessment tool to pre-select both skillful models in a multi-model ensemble (Mignot et al., 2020), as well as skillful ensemble members in a single-model ensemble prediction system (Oliveira et al., 2020).
In this study, we combine the concept of ensemble subsampling (e.g., Dobrynin et al., 2018) with SOM, and evaluate the potential of spring North Atlantic SST at initialisation in predicting skillful ensemble members in MPI-ESM-MR. We further assess to what extent this selection affects the predictive skill of European summer climate, in comparison to a traditional predictive skill analysis. The manuscript is structured as follows: Section 2 describes our methodology, the SOM algorithm and datasets used for training and evaluation. We characterize the main observed and simulated summer atmospheric teleconnections in the Euro-Atlantic domain in Section 3.1. In Section 3.2 we perform SOM training to identify regions in the domain with potential for skill improvement, and in Section 3.3 we evaluate the hindcast skill. We present a discussion of the results in Section 4, followed by conclusions in Section 5.
2. Methodology
We organize our methodology in four main parts: data (Section 2.1), training (Section 2.2), evaluation (Section 2.3), and verification (Section 3.4). In Section 2.1, we describe the reanalysis and test ensemble used for training and evaluation, an independent ensemble used for verification, and the preprocessing methods adopted in our analysis. A brief introduction to the SOM algorithm, the steps taken for training SOM, and our predictor analysis are described in Section 2.2. We characterize ensemble subsampling and hindcast skill analysis in Section 2.3 and describe an independent verification in Section 3.4. In Figure 1 we show a sketch representing the workflow of our method.
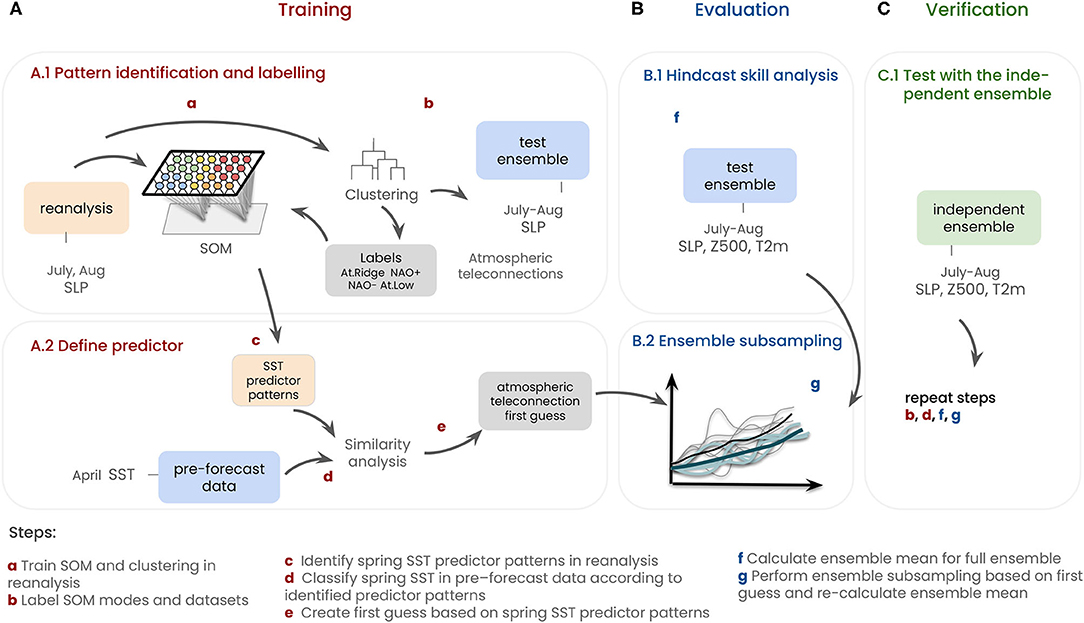
Figure 1. Sketch of workflow. A.1: We use Self-Organizing Map (SOM) to identify atmospheric teleconnections dominating the large-scale variability over the Euro-Atlantic region in 1902–2008. The final SOM output is a map consisting of twelve SOM modes. We train SOM with monthly July and August (JA) sea level pressure (SLP) in the reanalysis data. This same data is used to train a k-means clustering (k = 4) to be used as a reference, whose centroids are labeled NAO+, NAO−, At. Ridge or At. Low as proposed in Cassou et al. (2005). A.2: We calculate pattern correlation between SOM modes and the k-means centroids to associate each summer SOM mode to a summer atmospheric teleconnection. We repeat this process with the k-means centroids to label each JA SLP field of the reanalysis and the hindcast. Finally, we identify sea surface temperature (SST) predictor patterns by calculating composite April SST patterns in the reanalysis with respect to SOM modes. Those SST predictor patterns are used to classify each North Atlantic April SST field in the pre-forecast data using pattern correlation. Given that each SST predictor pattern precedes a summer SOM mode associated with NAO+, NAO−, Atlantic Ridge (At. Ridge) or Atlantic Low (At. Low), we are able to define a summer atmospheric teleconnection “first guess” before each initialization. B.1: Using the test ensemble, we evaluate the hindcast skill for 3–4 month lead time considering the full ensemble to calculate the ensemble mean. B.2: We perform ensemble subsampling to re-calculate the ensemble mean by selecting only ensemble members whose labels agree with the “first guess,” and comparing it with the full ensemble. C.1: We label, classify, and subsample the independent ensemble as verification of our method.
2.1. Data
2.1.1. Reanalysis
We use monthly means of sea level pressure (SLP), geopotential height at 500 hPa (Z500), air temperature at 2m (T2m), and SST from ERA-20C (Poli et al., 2016) and ERA-Interim (Dee et al., 2011) reanalysis products, spanning 1902–2008 and 1980–2016, respectively. For the three atmospheric variables, we evaluate the North Atlantic-European sector covering 70°W-40°E, 25°-80°N, and for SST covering 90°W-40°E, 5°-80°N.
2.1.2. Ensemble simulations
We use two sets of 30-member hindcast simulations with the MPI-ESM-MR setup (Dobrynin et al., 2018). The atmospheric component ECHAM6 (Stevens et al., 2013) has a resolution of T63L95, with an approximate horizontal resolution of 200 km (1.875°) and 95 vertical layers up to 0.01 hPa. The oceanic component MPI-OM (Jungclaus et al., 2013) has a resolution of TP04L40, with an approximate horizontal resolution of 40 km (0.4°) and 40 vertical layers. External forcing is taken from CMIP5 (Giorgetta et al., 2013). The first set of hindcasts is used for training and evaluation and covers the period 1902–2008 (hereafter: test ensemble), and the second is used for independent verification (Section 2.4, refer to Figure 1) and covers 1980–2016 (hereafter: independent ensemble).
The test ensemble is initialized on the 1st of May every year from 1902 to 2008, with initial conditions taken from an assimilation experiment. The assimilation experiment is performed using the MPI-ESM-MR with full-field nudging by Newtonian relaxation toward all atmospheric and ocean levels except in the boundary layer. The atmospheric conditions of vorticity, divergence, three-dimensional temperature, and two-dimensional pressure are taken from ERA-20C. In the ocean, three-dimensional daily mean salinity and temperature anomalies are nudged at a relaxation time of approximately 10 days. The ocean state is derived in an ocean-only simulation performed with MPI-OM forced with the atmospheric variables from ERA-20C. The three-dimensional atmospheric and ocean fields of the assimilation experiment form the initial conditions, and ensemble members are generated by small perturbations of the atmospheric state by disturbing the diffusion coefficient in the uppermost layer.
Similarly, the independent ensemble is initialized in 1st of May every year from 1980 to 2016 by an assimilation experiment in which ERA-Interim data (Dee et al., 2011) is assimilated into the atmospheric model component, and ORA-S4 data (Balmaseda et al., 2013) and National Snow and Ice Data Center observations (Comiso, 1995) are assimilated into the ocean/sea ice component.
We use July and August monthly means (3–4 months lead time) of SLP, Z500, and T2m from test and independent ensembles. At every gridpoint, we compute anomalies by removing the mean seasonal cycle and linear trend, in order to eliminate the centennial-scale climate change signal. We use July-August seasonal means (JA) to focus on the low-frequency dominant summer atmospheric teleconnections. In addition, we calculate spatial averages weighted by the cosine of latitude to take into account the dependence of the gridpoint density on latitude.
2.2. Training
2.2.1. Pattern Identification and Labeling
We use monthly JA SLP fields from ERA-20C reanalysis to train Minisom, a Python implementation of Self-Organizing Map (SOM) (Vettigli, 2019). SOM is a non-linear method based on unsupervised learning with two-layer neural networks (Kohonen, 1984). SOM's architecture allows for a reduced and ordered representation of high-dimensional datasets by a smaller set of variables. In a typical two-layer SOM, the input layer corresponds to feature vectors from the training dataset, while the output layer is the SOM map. The SOM map is a topological ordering of neurons usually in the 2D grid (denoted SOMij, where i and j are the grid indices of the SOM map). This layer is fully connected to the input layer via weight vectors with the same dimension as the feature vectors. The lattice structure of the layers permits to calculate a measure of distance (here Euclidean distance) and identify the shortest path between neurons of both layers, thereby assigning as Best Matching Unit the respective closest neuron in the SOM map, iteratively. A fundamental property of SOM is the topological ordering: neighboring neurons SOMij represent similar neurons in the input data space and therefore share similar properties. Hereafter, we adopt mode as terminology to refer to neurons in the SOM map. For details on the SOM algorithm, refer to Kohonen (2013).
We train a 3 x 4 rectangular lattice of neurons (i.e., SOM34) and choose training parameters as a compromise to keeping low quantisation and topological errors while achieving a detailed view on the representative SOM modes associated with the summer atmospheric teleconnections defined in Cassou et al. (2005). We find that optimum parameters are (i) initial spread of the neighborhood function σ(0) = 0.01 (Gaussian) and (ii) learning rate 0.8, for a maximum of 100,000 iterations in batch training mode. Our tests with larger SOM sizes (e.g., 4x4, 5x5) showed qualitatively similar modes to the chosen 3x4 lattice, although showing duplicate patterns. Hence, a 3x4 SOM lattice balanced the need to represent the main summer atmospheric teleconnections with the least number of modes possible. The final output of the SOM training is a 12-mode SOM map (hereafter: SOM master). In addition to the SOM master, we perform a similar SOM training for each individual ensemble member of the test ensemble to allow comparison between model and reanalysis.
Next, we label each SOM mode as either At. Ridge, At. Low or NAO in positive or negative phase to allow comparison with other studies using EOF or k-means. Labelling is achieved by calculating pattern correlation between each SOM mode and the four centroids of a k-means clustering of ERA-20C JA SLP means, previously labeled according to Cassou et al. (2005); Cattiaux et al. (2013). We obtain similar labels if classifying the SOM modes with hierarchical agglomerative clustering using ward dissimilarity (Jain and Dubes, 1988). Note that during learning, each observation in the training dataset is associated with only one mode SOMij in the SOM map. In other words, this analysis assigns one dominant atmospheric teleconnection per summer each year.
2.2.2. Define Predictor
We derive a set of 12 SST predictor patterns by calculating monthly April North Atlantic SST composites in the reanalysis with respect to the SOM master. That is, each SST composite pattern is a mean over the years associated with the input vector connected to a specific SOM mode in summer. We assume that each pattern is a potential predictor for one of the four atmospheric teleconnections (At. Low, At. Ridge, NAO+ or NAO−). To test this in the model, we classify each April North Atlantic SST field in the pre-forecast data according to the SST predictors via maximum pattern correlation. Thus, the most similar SST predictor defines a summer atmospheric teleconnection “first guess” before each initialization, which we use as subsampling criteria in Section 2.3.
2.3. Evaluation
2.3.1. Hindcast Skill Analysis and Ensemble Subsampling
The hindcast skill of MPI-ESM-MR against reanalysis data is assessed using point-wise detrended anomaly correlation coefficient (ACC) (Collins, 2002), which describes the model's ability to reproduce the reference anomalies.
For single-model initialized ensemble prediction systems, ensemble subsampling consists of a post-processing technique that pre-selects potentially skillful ensemble members prior to a predictive skill assessment, giving a statistical link to sources of high predictability (e.g., Dobrynin et al., 2018). We perform an ensemble subsampling with the aim of leveraging the MPI-ESM-MR ensemble prediction system before calculating the ensemble mean and assessing the hindcast skill. This procedure retains only ensemble members anticipated by the SST predictor to re-calculate the ensemble mean, i.e., selects a subsample of potential skillful ensemble members per year. That is, it allows us to investigate the conditional predictability of the SST predictor on the summer atmospheric circulation. This selection of ensemble members is used to re-calculate the ensemble mean of SLP, Z500, and T2m.
2.4. Verification
We use the independent ensemble (Section 2.1.2) to evaluate the robustness of our spring SST predictors in selecting potential skillful ensemble members at the initialization of summer predictions for the period 1980–2016. Even though “test” and “independent” ensembles derive from completely independent seasonal prediction systems, we acknowledge that an overlapping period (1980–2008) is present in the verification, implying that the conclusions drawn in this section must be taken with care. We stress, however, that excluding this overlapping period would lead to a very limited statistical analysis of the model predictive skill covering only the 8-year period from 2009 to 2016 and, thus, preventing us from reproducing the analysis performed in Section 2.3. Once more observed and predicted years become available, it would be relevant to perform this analysis without overlap with the training period.
To perform the verification, we firstly label the independent ensemble as described in Section 2.2.1. Next, using the assimilation experiment as our pre-forecast data, we classify SST as described in Section 2.2.2. Finally, we perform a hindcast skill analysis and ensemble subsampling (Section 2.3), comparing to ERA-Interim (Dee et al., 2011) reanalysis.
3. Results
3.1. Dominant Summer Atmospheric Teleconnections
We use a 3x4 SOM to represent the summer (JA) SLP variability spanning 1902–2008 in the Euro-Atlantic region using the reanalysis (Figure 2 shows the labeled SOM master, where the position of some modes differ from the original SOM map, refer to Supplementary Figure S1). We group together SOM modes associated with the same main atmospheric teleconnections (i.e., NAO+, NAO−, At. Ridge, or At. Low) and identify two main groups based on the meridional position of cyclonic and anticyclonic pressure centers. The first group comprises SOM modes 1–6, with NAO+ and At. Ridge modes, the second group comprises SOM modes 7–12, with NAO- and At. Low modes. In terms of the preferred jet stream position, the first group of atmospheric teleconnections is usually associated with northerly or central positions, while the second with southerly or central (e.g., Woollings et al., 2010; Trouet et al., 2018). For simplicity, we refer to the former as the northern jet group and the latter as the southern jet group.
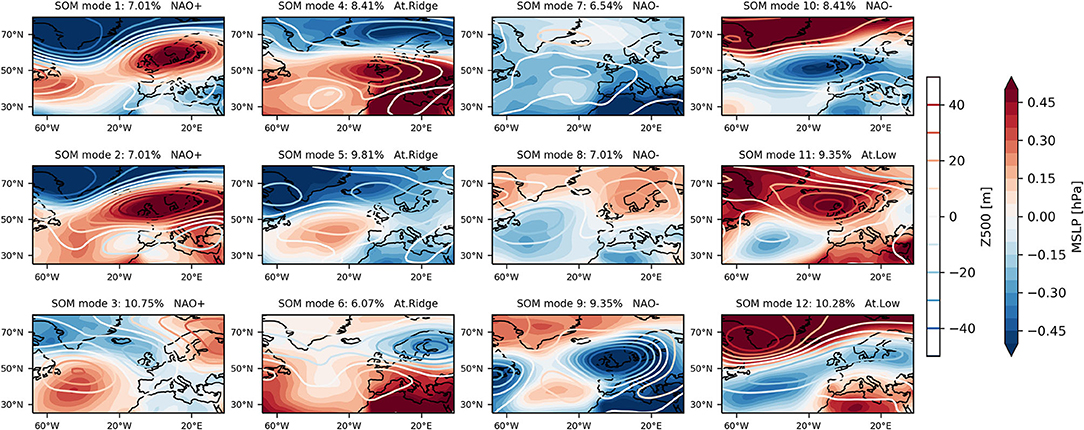
Figure 2. Self-Organizing Maps master representing the dominant summer atmospheric teleconnections in the North Atlantic European sector during 1902–2008, trained with ERA-20C JA SLP. Note that some modes were re-positioned with respect to the original SOM map to facilitate the interpretation of results.
North Atlantic Oscillation modes in positive (SOM modes 1–3) and negative (SOM modes 7–10) phases are located in opposite corners of the original SOM master, showing the highest topological distance and, therefore, the least level of similarity (c.f. Supplementary Figure S1). Clear spatial asymmetries revealed by the non-linear method can be observed between the two phases. SOM modes 4–6 and 11–12 cover a range of wavy patterns related to At. Ridge (SOM modes 4–6), and At. Low (SOM modes 11–12). While NAO modes show significant moderate correlation to the correspondent first EOF of SLP (e.g., SOM mode 1: 0.36, p < 0.05 and SOM mode 10: –0.54, p < 0.05), no significant pattern correlation can be found with the second EOF of SLP. Yet, some modes bear high similarity with At. Ridge (SOM modes 4–6) and At. Low (SOM modes 11–12), reported in Cassou et al. (2005).
Next, we assess the agreement between atmospheric teleconnections in the reanalysis and those simulated by MPI-ESM-MR in the test ensemble (Figures 3A,B). As opposed to a traditional evaluation using the ensemble mean, we instead analyse each ensemble member separately to assess the intra-ensemble variability. Spatially, we find overall moderate pattern correlation values, with At. Low modes (SOM modes 11–12) and one NAO+ mode (SOM mode 3) differing the most between model and reanalysis. In contrast, At. Ridge modes SOM 4–5 show the best agreement. While pattern frequency results (Figure 3B) show high intra-ensemble variability, the model fails at times to encompass the observed frequency (e.g., SOM modes 1, 6, 8). The model tends to underestimate the frequency of At. Low (SOM modes 11–12), and overestimate NAO+ modes (SOM modes 1–2). We hypothesize that these limitations in the model representation of the observed summer variability pose a constraint on the credibility of summer predictions in the Euro-Atlantic region one season ahead based on this prediction system.
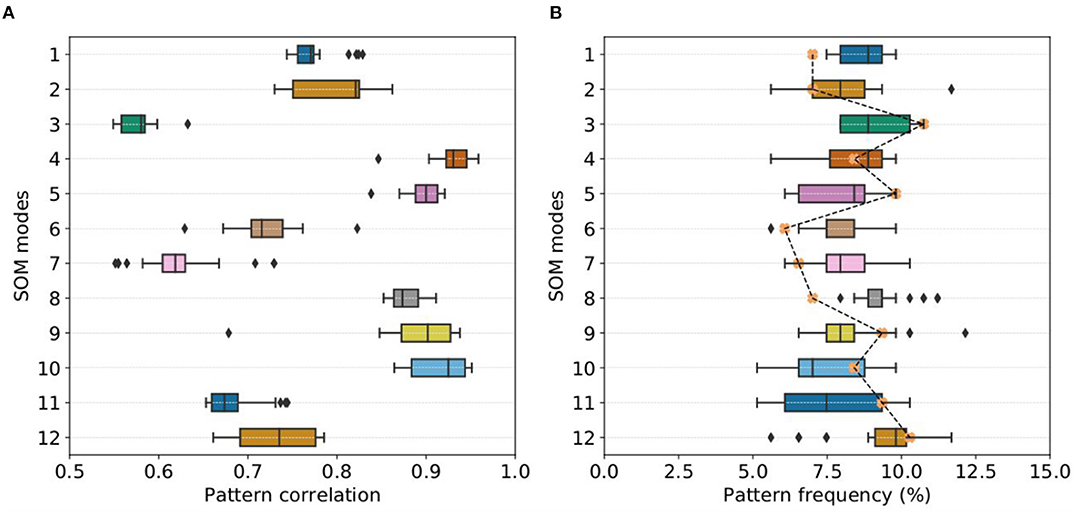
Figure 3. Model agreement with reanalysis: (A) Pattern correlation between observed and simulated SOM modes (per ensemble member). (B) Associated frequency of observed and simulated SOM modes (per ensemble member). X markers and dashed lines represent observed frequencies.
3.2. Target Regions for Skill Improvement and Link to SST
In this section, we evaluate the relationship between spring SST and summer SLP to target regions for potential skill improvement in the model. The set of spring SST predictors (Figure 4) show reasonable agreement with previous studies (e.g., Gastineau and Frankignoul, 2015). Preceding summers dominated by At. Ridge (hereafter: pre-At. Ridge), we find SST tripole patterns with warm SST anomalies in the tropical North Atlantic and western subpolar gyre off Newfoundland, and mostly cold SST in the subtropical western North Atlantic. At. Low summers follow major North Atlantic basinwide cooling, with a warming pattern near Greenland and off Newfoundland (hereafter: pre-At. Low). In contrast, negative NAO summers mostly follow horseshoe-like SST patterns with warm anomalies except over the subtropical gyre (hereafter: pre-NAO-). One case stands out, however, showing instead a SST predictor similar to the At. Low ones (c.f. Figure 4, pre-SOM mode 8). Finally, we find no consistent mean SST pattern preceding positive NAO summers, but a set of three different SST tripole patterns with a common cooling over the North Sea and off the coast of northwest Africa (hereafter: pre-NAO+).
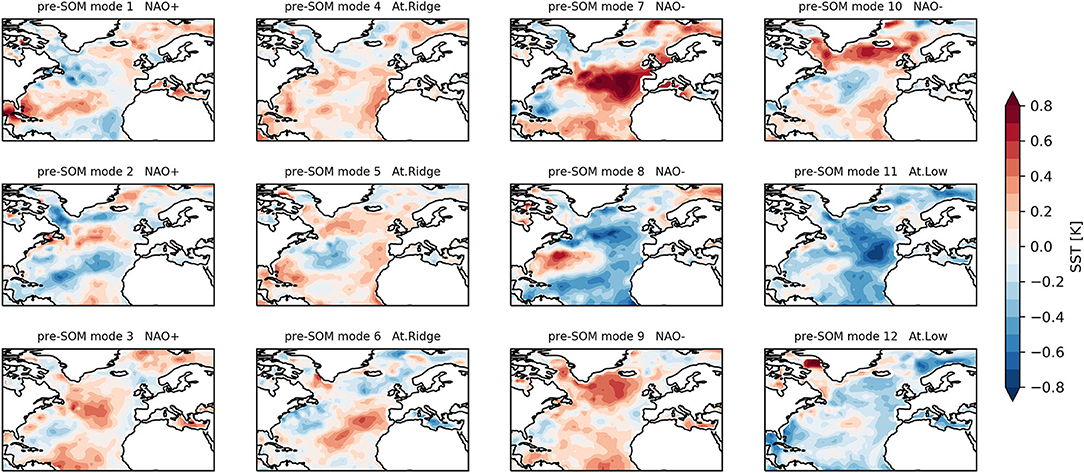
Figure 4. Spring sea surface temperature (April SST) predictor patterns in the reanalysis with respect to the SOM master (Figure 2), for the period 1902–2008. Each pattern of SST precedes a summer SOM mode, as labeled.
We evaluate the linear relationship between April North Atlantic SST predictors and JA SLP using the reanalysis (Figure 5). Areas of significant correlation indicate where the specific SST predictor might influence the summer circulation and, thus, a serve as reference to interpret any skill improvement in the model. We find that SST predictors have a significant influence on JA SLP in At. Ridge (pre-SOM modes 4–6) and At. Low cases (pre-SOM modes 11–12), and in less extent to NAO+ (pre-SOM modes 1–2) and NAO- (pre-SOM modes 8–10). We find reasonable agreement with correlation results for the SST predictors and JA T2m or JA Z500 (not shown). From Figure 5 we might expect to find skill improvement only for the summer circulation over Greenland, Scandinavia, central Europe, and a region over the ocean west of the Iberia Peninsula using our SST predictors.
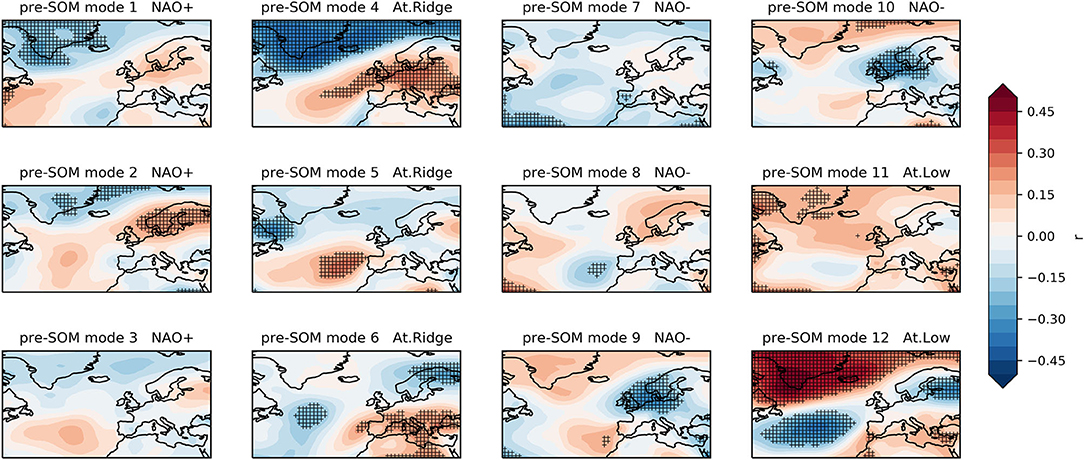
Figure 5. Summer sea level pressure (JA SLP) point-wise correlated to timeseries associated with April SST predictor patterns for the reanalysis over the period 1902–2008. Stippling represents correlation significant at the 95% level.
3.3. Windows of Opportunity for MPI-ESM-MR Based on SST
First, we use the test ensemble to evaluate whether the SST-SLP relationship found in the reanalysis holds in the model. Next, we use the independent ensemble to test the robustness of this relationship and to assess the potential of skill improvement for other variables.
We evaluate the predictive skill at 3–4 months lead time for JA SLP in the test ensemble, for SST predictors of each of the four main summer atmospheric teleconnections separately to distinguish the contribution of SST (Figure 6). As a first step, we analyse ACCs before subsampling in the ensemble space, considering the full ensemble (Figures 6A,D,G,J). We find distinct predictive skills for each group of SST predictors, with SST predictors for At. Ridge (i.e., pre-At. Ridge) showing highest skill off the Iberian coast but no skill over land. The remaining groups show very limited skill overall.
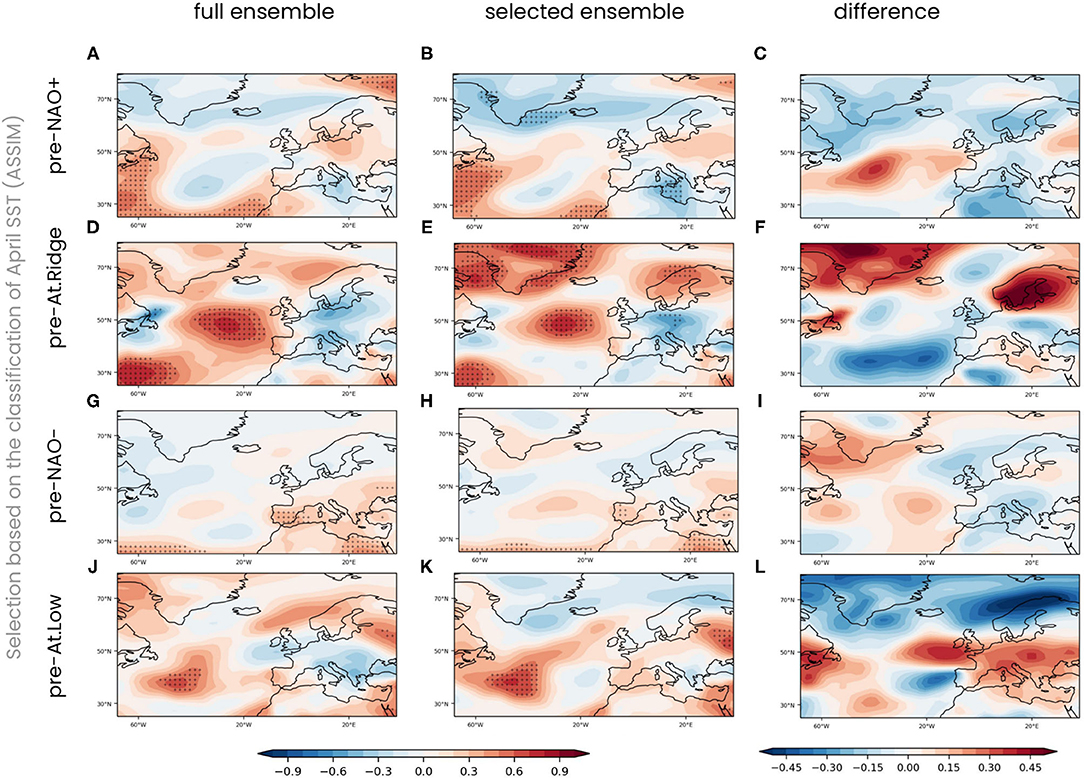
Figure 6. Evaluation of a SST as predictor in the test ensemble. Anomaly correlation coefficients (ACC) for SLP, comparing the test ensemble to the reanalysis in 1902–2008. On the first row (uppermost) ACCs are calculated for years where April SST months in the pre-forecast data are classified as preceding NAO+, i.e., pre-NAO+. Similarly, the remaining three rows show ACCs for the case of pre-Atl. Ridge, pre-NAO− and pre-At. Low, respectively. Column-wise, ACCs are presented as follows: (A,D,G,J) the ensemble mean is taken over the full ensemble; (B,E,H,K) the ensemble mean is taken over the selected ensemble; (C,F,I,J) differences in ACC between the full and selected ensemble mean. Stippling represents correlation significant at the 95% level.
We perform ensemble subsampling by selecting ensemble members according to the April SST classification in the pre-forecast data in predicting northern (At. Ridge or NAO+) or southern jet (At. Low or NAO-) groups. For example, Figure 6B shows the ACC calculated for the ensemble mean over members which predict either NAO+ or At. Ridge, for those years where April SST in the pre-forecast data classifies as pre-NAO+. A more strict selection allowing only one dominant atmospheric teleconnection per summer leads to a weak improvement. We include difference plots to illustrate the effect of performing ensemble subsampling on the predictive skill, in comparison to the traditional analysis using the full ensemble mean. Physically, a positive difference in correlation (selected minus full ensemble) thus represents the model predictive skill that could be achieved by this prediction system, if corrected using our method. In contrast, a negative difference in correlation suggests that the SST predictor is not sufficient to perform the first guess, or that the model is unable to accurately simulate the atmospheric teleconnection under the circumstances given.
Comparing selected and full ensemble in Figure 6, we find significant regional improvement in the predictive skill of JA SLP for SST predictors of At. Ridge and Low. Improvement is highest for At. Ridge over Greenland and Scandinavia, reaching ACCs above 0.6. These regions of improvement in skill agree with the expectation based on Figure 5, SOM modes 4–6. This finding further agrees with Figures 2B,C, which shows that At. Ridge is the atmospheric teleconnection best represented by MPI-ESM-MR, thus, having the potential to benefit the most from a physics-informed subsampling. For NAO+ and NAO−, improvement is limited to the region over the ocean off the Iberian Peninsula, insignificant at the 95% level. We speculate that this is partly due to the less accurate representation of some NAO SOM modes by MPI-ESM-MR (refer to Figures 2B,C), in addition to a weaker SST-SLP relationship than for At. Ridge and Low modes (refer to Figure 5).
To contribute to the interpretation of predictive skill improvement, we test the benefit of refining the model ensemble with a "perfect" selection using the test ensemble. The "perfect" ensemble selection assumes that the dominant summer atmospheric teleconnection in the Euro-Atlantic domain is known each year in advance, thereby estimating the potential skill if such atmospheric teleconnection would be perfectly predicted by the model. We stress that, as opposed to the "selected" ensemble, such an analysis is only possible in hindcast mode, thus, not being reproducible in real forecast mode. We analyse northern and southern jet groups separately to distinguish the effect of subsampling for SLP. In Figure 7, we select only ensemble members which agree with the atmospheric teleconnection label predicted by the reanalysis in a given year to calculate the ensemble mean. We find major skill improvement in the Euro-Atlantic sector for SLP predictions at 3–4 months lead time, with about half of the ensemble members selected to re-calculate the ensemble mean (Figure 7G). The main area of improvement fairly agrees with the position of the jet: over Scandinavia for the northern group and over south-western Europe for the southern group. This suggests that the area of skill improvement depends on the skill of the model in simulating the relationship between predictor and target.
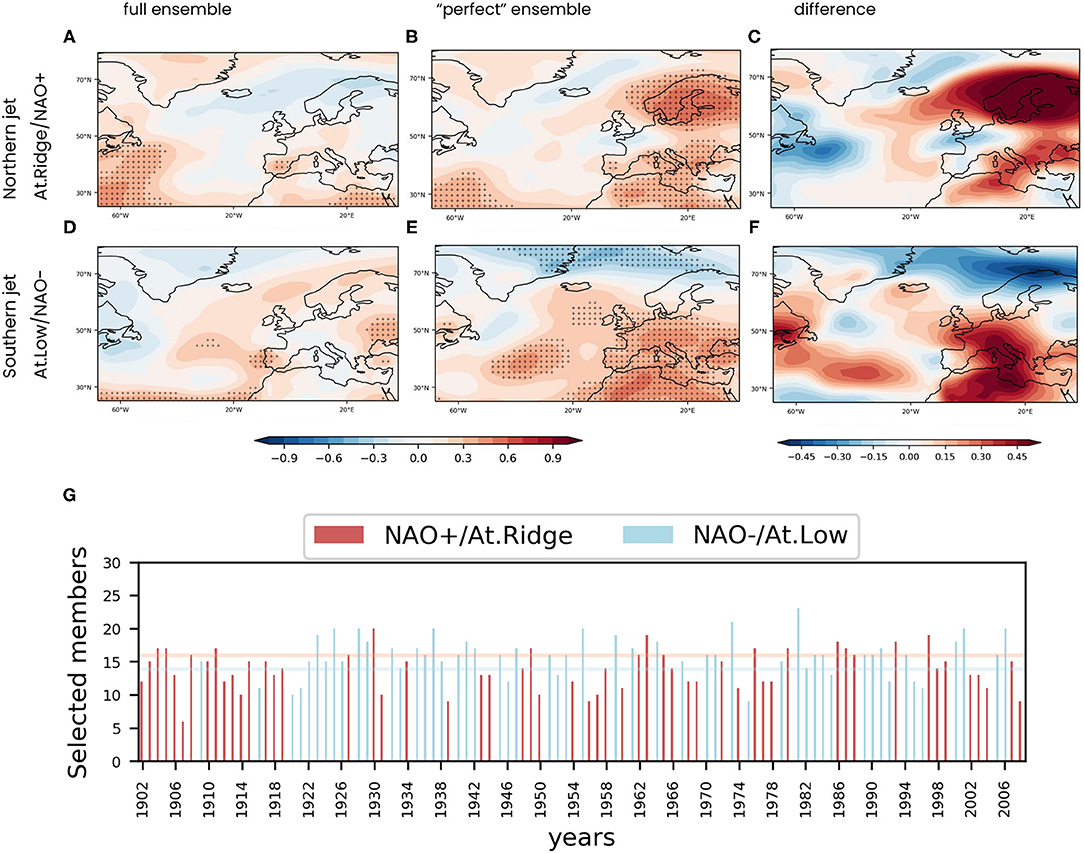
Figure 7. “Perfect” model approach. ACC for SLP, comparing the test ensemble to the reanalysis in 1902–2008. On the upper row, ACCs are calculated for years where the observed dominant summer atmospheric teleconnection is classified as either At.Ridge or NAO+. Conversely, in the lower row, ACCs are calculated for At. Low or NAO− cases. ACCs are presented as follows: (A,D) the ensemble mean is taken over the full ensemble; (B,E) only members whose classification matches the observed are selected to calculate the ensemble mean; (C,F) differences in ACC between full and selected ensemble mean. Stippling represents correlation significant at the 95% level. (G) Bar plots show the fraction of ensemble members selected per year: red bars for observed At.Ridge or NAO+, blue bars for At. Low or NAO- cases. Horizontal lines show the mean fraction for each case.
3.4. Test With the Independent Ensemble
Next, we use the independent ensemble to test whether these findings hold for an independent hindcast dataset covering the period of 1980–2016 (Figure 8). In addition to SLP, we test how the selection of ensemble members based on the SST-SLP relationship impacts the predictive skill of T2m and Z500. We analyse two groups and calculate ACCs for SST predictors for the northern jet (pre-At. Ridge or NAO+) and southern jet (pre-At. Low or NAO-), similarly to Figure 7. Analyzing the full ensemble (first column), we find for both SLP and Z500 that the northern jet group (Figures 8A,G) shows a higher skill for northern Europe in comparison to the southern jet group (Figures 8J,P). Still, improvement for SLP and Z500 skill (Figures 8C,I,L,R) takes place in similar areas as for the independent ensemble (Figure 6 and Supplementary Figure S3), albeit less pronounced. For T2m in the selected ensemble (Figures 8D–F), we find skill improvement for north-western Europe, with ACCs over Scandinavia reaching significant values above 0.5 (at 95% level) for spring SST indicating northern jet group (pre-At. Ridge/NAO+). We find no significant T2m skill improvement for the southern jet group (Figures 8M–O). Despite not reaching statistical significance, correspondence in the spatial pattern improvement for SLP, Z500, and T2m (Figures 8C,F,I) alludes that the ensemble subsampling based on the spring SST - summer SLP relationship influences the predictive skill of Z500 and T2m. This suggests the effect of the large-scale atmospheric circulation as a dynamical driver of temperature variability at the seasonal timescale and illustrates the importance of improving the simulation of summer atmospheric teleconnections within the ensemble as a step to achieve skillful predictions of European climate.
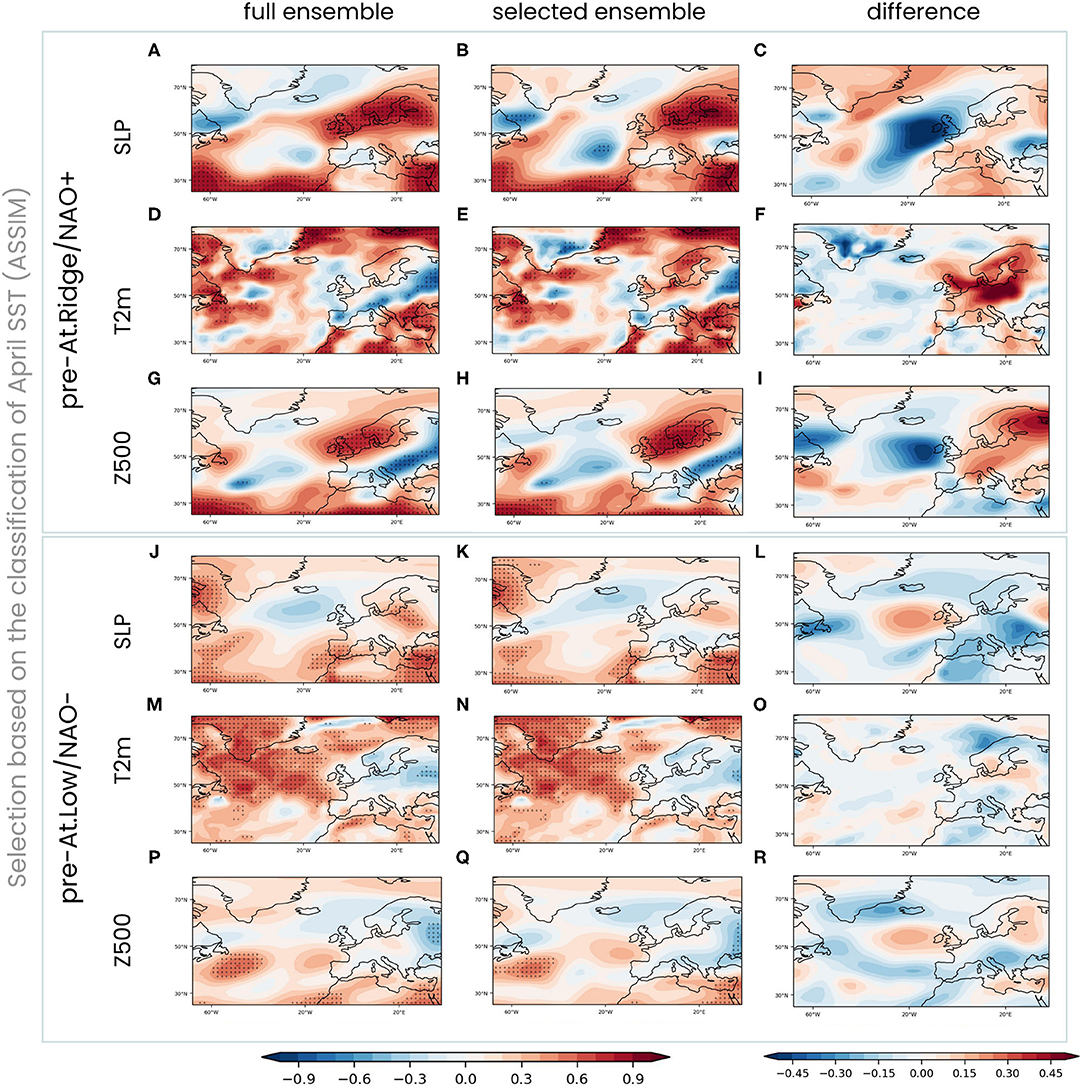
Figure 8. Evaluation of SST as a predictor in the independent ensemble. ACC for SLP, temperature at 2 m height (T2m), and geopotential height at 500 hPa (Z500), as labeled, comparing the independent ensemble to Era-Interim in 1980–2016. On the upper three rows, ACCs are calculated for years where April SST months in the pre-forecast data are classified as preceding either At.Ridge or NAO+, i.e., pre-At.Ridge/NAO+. Conversely, the lower three rows show ACCs for the case of pre-Atl.Low/NAO-. Column-wise, ACCs are presented as follows: (A,D,G,J,M,P) the ensemble mean is taken over the full ensemble; (B,E,H,K,N,Q) the ensemble mean is taken over the selected ensemble; (C,F,I,L,O,R) differences in ACC between the full and selected ensemble mean. Stippling represents correlation significant at the 95% level.
4. Discussion
Dynamical seasonal forecasts of European summer climate have until recently mostly shown negligible skill (e.g., Mishra et al., 2019), presumably a consequence of model errors in representing important drivers of summer climate variability (e.g., Beverley et al., 2019; Ossó et al., 2020). While recent work has shown evidence for skillful European summer rainfall predictions (Dunstone et al., 2018), model skill seemed to stem primarily from thermodynamical drivers. Identifying processes and predictors that provide forecast opportunities for skillful long-lead predictions is hence of paramount importance.
In this article, we use SOM to enable the identification of dynamical predictors for a pre-defined set of SOM modes. These SOM modes represent 12 stages of the four main atmospheric teleconnections driving the large-scale summer variability in the Euro-Atlantic region (Cassou et al., 2005). While defining these discrete samples is a clear limitation of our approach, using SOM allows us to distinguish spring SST patterns that can be used to indicate conditions of potentially high summer predictive skill at prediction start. Conversely, we were unable to find skillful SST predictors for composites based on a k-means clustering (k = 4), possibly due to the blending of patterns and loss of information (not shown).
We show in this study that April North Atlantic SST patterns can be used to select potentially skillful ensemble members for MPI-ESM-MR summer hindcasts over specific target regions. We find that these regions depend on the dominant atmospheric teleconnection in a given summer and the strength of its relationship with spring SST. Based on these two criteria, we find that spring SST precursors for At. Ridge summers correspond to conditions under which the MPI-ESM-MR seasonal predictions may be expected to have particularly high skill over target regions, thus, representing forecasts of opportunity (Mariotti et al., 2020).
We identify spring SST precursor patterns for At. Ridge summers which show reasonable agreement with results reported by Ossó et al. (2018) using Maximum Covariance Analysis. The authors described a pattern consisting of a spring SST dipole between subpolar and subtropical North Atlantic that persists into summer, forced by anomalous winter atmospheric circulation. Ossó et al. (2020) showed that this SST pattern drives a poleward displacement of the jet stream through changes in the background baroclinicity. Ossó et al. (2018) suggested that the summer atmospheric response to this oceanic forcing is imprinted at the surface as an anticyclonic anomaly that resembles the At. Ridge.
Identifying SST initial conditions that are linked to summers dominated by At. Ridge 3–4 months ahead is an important step toward skillful European seasonal summer predictions. At. Ridge has been associated with northerly wind anomalies over western Europe (Craig and Allan, 2021), leading to a widespread below-average surface temperature distribution (Cassou et al., 2005; Comas-Bru and Hernández, 2018). We find a similar imprint on the surface climate for SOM modes associated with At. Ridge (Supplementary Figure S2), illustrated by composites of summer air temperature anomalies. In combination with cyclonic conditions over the Mediterranean region, At. Ridge has been additionally associated with easterly wind anomalies, thereby influencing the occurrence of dry spells and drought conditions over western Europe (Haarsma et al., 2009; Rousi et al., 2021).
In addition to At. Ridge, our analysis of the relationship between spring SST and summer SLP (Figure 5) suggests that spring North Atlantic SST precursors for At. Low could predict potentially skillful ensemble members for MPI-ESM-MR summer hindcasts (e.g., Figure 5, pre-SOM mode 12). While we find improvement in skill over large areas of central Europe and off the coast of England, the improvement does not reach significance (95%) over most areas, except over southeastern Europe. We speculate that this is due to the more limiting representations of this atmospheric teleconnection in MPI-ESM-MR (Figures 3A,B), which might imply a model limitation for early warning of warmer than average summers. This finding agrees with Neddermann (2019), which shows that a teleconnection pattern similar to At. Low in MPI-ESM-MR shows a different structure of the centers of action in comparison to the reanalysis.
In contrast to At. Ridge and Low, only a few studies have suggested an active role of spring North Atlantic SST as a driver for summer NAO at seasonal to interannual timescales (e.g., Gastineau and Frankignoul, 2015; O'Reilly et al., 2017). Osborne et al. (2020) investigated the effect of North Atlantic SST on atmospheric circulation responses over the Euro-Atlantic region, speculating that summer NAO-related SST anomalies might feedback onto the At. Ridge and Low teleconnections rather than the summer NAO. Our analysis using North Atlantic SST as a precursor for NAO show only marginal predictive skill improvement, for both NAO in positive and negative phases. This suggests that April North Atlantic SST has a limited impact on the seasonal predictability of summer NAO, potentially explained by this SST-NAO inconsistency.
Though we only investigate the role of spring North Atlantic SST, other predictors have been suggested to influence the predictability of the summer atmospheric teleconnections analyzed here. In particular, Hall et al. (2017) suggested that summer NAO is dependent on the positioning of the polar front jet and, thus, on Arctic sea ice. Another potential predictor for summer NAO is stratospheric temperature, which in winter establishes a downward connection from the stratosphere to the surface, leading to enhanced surface predictability (e.g., Ayarzagüena and Serrano, 2009). For winter NAO, a combination of these precursors in autumn with ensemble subsampling allowed unprecedented skillful prediction of the NAO index in MPI-ESM-MR (Dobrynin et al., 2018). Including such predictors to inform the ensemble subsampling would be an interesting focus for future study.
5. Conclusions
We combine SOM and seasonal climate predictions with MPI-ESM-MR to investigate the seasonal predictability of the European summer climate associated with the North Atlantic jet stream. We show that April North Atlantic SST patterns can be used to select potentially skillful ensemble members for MPI-ESM-MR summer hindcasts over specific target regions. Our main findings are:
• Our SOM analysis shows that among the four main summer atmospheric teleconnections MPI-ESM-MR best represents At. Ridge, showing the highest agreement with the reanalysis both spatially and in the frequency of occurrence. Conversely, spatial representation of At. Low in MPI-ESM-MR agrees the least with the reanalysis, and the model underestimates the frequency of occurrence.
• The use of SOM composites of North Atlantic SST patterns as spring SST predictors is relevant for At. Ridge and At. Low teleconnections and limited for NAO. Greenland and Scandinavia are the areas over land with the most potential spring SST influence on northern jet positions.
• Using the test ensemble (1902–2008), we find significant skill improvement for summer SLP at 3–4 month lead time over Greenland and Scandinavia for predictions initialized with SST predictors for At. Ridge, for a selected ensemble predicting northern jet atmospheric teleconnections (At. Ridge or NAO+).
• Using the independent ensemble (1980–2016), we find significant skill for summer SLP at 3–4 month lead time over northern Europe for predictions initialized with SST predictors for northern jet atmospheric teleconnections (At. Ridge or NAO+). For a selected ensemble predicting northern jet teleconnections, we find significant skill improvement over Scandinavia for both Z500 and T2m.
• A spatial correspondence in the improvement of Z500 and T2m for the northern jet group suggests the effect of large-scale atmospheric circulation as a dynamical driver of European temperature variability at the seasonal timescale. This finding highlights the importance of accurately representing summer atmospheric teleconnections in dynamical seasonal prediction systems as a necessary condition toward skillful predictions of the European climate.
Our findings offer an interesting avenue for the use of SOM in further research on windows of opportunity for seasonal climate predictions. Since our analysis only relies on SST information prior to the initialization of the prediction system, our methodology can be extended and further applied to operational ensemble prediction systems.
Data Availability Statement
The data analyzed in this study is subject to the following licenses/restrictions: All data are stored at the DKRZ in archive and can be made accessible upon request (https://www.dkrz.de/up). Requests to access these datasets should be directed to German Climate Computing Center (DKRZ) https://www.dkrz.de/up.
Author Contributions
JC-O wrote the article and performed the analysis. JC-O, LB, JB, and EZ conceived the study and contributed to the writing and interpretation of the results. All authors contributed to the article and approved the submitted version.
Funding
This research is a contribution to the Excellence Cluster CliCCS-Climate, Climatic Change, and Society at the University of Hamburg, funded by the DFG through Germany's Excellence Strategy EXC 2037 Project 390683824 (JB). This research has been additionally supported by the Helmholtz-Inkubator projector Reduced Complexity Models Redmod (JC-O and EZ); the European Commission, Horizon 2020 [grant no. EUCP (776613)]; and the ANR Tremplin-ERC project HARMONY, grant no. ANR-20-ERC9-0001 (LB).
Conflict of Interest
The authors declare that the research was conducted in the absence of any commercial or financial relationships that could be construed as a potential conflict of interest.
Publisher's Note
All claims expressed in this article are solely those of the authors and do not necessarily represent those of their affiliated organizations, or those of the publisher, the editors and the reviewers. Any product that may be evaluated in this article, or claim that may be made by its manufacturer, is not guaranteed or endorsed by the publisher.
Acknowledgments
The authors would like to thank the Climate Modelling group at Universität Hamburg for helpful discussions, and the reviewers for their thoughtful comments and suggestions.
Supplementary Material
The Supplementary Material for this article can be found online at: https://www.frontiersin.org/articles/10.3389/fclim.2022.844634/full#supplementary-material
References
Ayarzagüena, B., and Serrano, E. (2009). Monthly characterization of the tropospheric circulation over the euro-atlantic area in relation with the timing of stratospheric final warmings. J. Clim. 22, 6313–6324. doi: 10.1175/2009JCLI2913.1
Balmaseda, M. A., Mogensen, K., and Weaver, A. T. (2013). Evaluation of the ecmwf ocean reanalysis system oras4. Q. J. R. Meteorol. Soc. 139, 1132–1161. doi: 10.1002/qj.2063
Barnston, A. G., and Livezey, R. E. (1987). Classification, seasonality and persistence of low-frequency atmospheric circulation patterns. Month. Weather Rev. 115, 1083–1126. doi: 10.1175/1520-0493(1987)115<1083:CSAPOL>2.0.CO;2
Bastos, A., Janssens, I. A., Gouveia, C. M., Trigo, R. M., Ciais, P., Chevallier, F., et al. (2016). European land co 2 sink influenced by nao and east-atlantic pattern coupling. Nat. Commun. 7, 1–9. doi: 10.1038/ncomms10315
Beverley, J. D., Woolnough, S. J., Baker, L. H., Johnson, S. J., and Weisheimer, A. (2019). The northern hemisphere circumglobal teleconnection in a seasonal forecast model and its relationship to european summer forecast skill. Clim. Dyn. 52, 3759–3771. doi: 10.1007/s00382-018-4371-4
Bladé, I., Liebmann, B., Fortuny, D., and van Oldenborgh, G. J. (2012). Observed and simulated impacts of the summer nao in europe: implications for projected drying in the mediterranean region. Clim. Dyn. 39, 709–727. doi: 10.1007/s00382-011-1195-x
Cassou, C., Terray, L., and Phillips, A. S. (2005). Tropical atlantic influence on european heat waves. J. Clim. 18, 2805–2811. doi: 10.1175/JCLI3506.1
Cattiaux, J., Quesada, B., Arakélian, A., Codron, F., Vautard, R., and Yiou, P. (2013). North-atlantic dynamics and european temperature extremes in the ipsl model: sensitivity to atmospheric resolution. Clim. Dyn. 40, 2293–2310. doi: 10.1007/s00382-012-1529-3
Collins, M. (2002). Climate predictability on interannual to decadal time scales: The initial value problem. Clim. Dyn. 19, 671–692. doi: 10.1007/s00382-002-0254-8
Comas-Bru, L., and Hernández, A. (2018). Reconciling north atlantic climate modes: revised monthly indices for the east atlantic and the scandinavian patterns beyond the 20th century. Earth Syst. Sci. Data 10, 2329–2344. doi: 10.5194/essd-10-2329-2018
Comiso, J. C. (1995). SSM/I Sea Ice Concentrations Using the Bootstrap Algorithm, Vol. 1380. National Aeronautics and Space Administration, Goddard Space Flight Center, Linthicum Heights, MD, United States.
Craig, P. M., and Allan, R. P. (2021). The role of teleconnection patterns in the variability and trends of growing season indices across europe. Int. J. Climatol. 43, 1072–1091. doi: 10.1002/joc.7290
Dee, D. P., Uppala, S. M., Simmons, A., Berrisford, P., Poli, P., Kobayashi, S., et al. (2011). The era-interim reanalysis: configuration and performance of the data assimilation system. Q. J. R. Meteorol. Soc. 137, 553–597. doi: 10.1002/qj.828
Dobrynin, M., Domeisen, D. I., Müller, W. A., Bell, L., Brune, S., Bunzel, F., et al. (2018). Improved teleconnection-based dynamical seasonal predictions of boreal winter. Geophys. Res. Lett. 45, 3605–3614. doi: 10.1002/2018GL077209
Dong, B., Sutton, R. T., Woollings, T., and Hodges, K. (2013). Variability of the north atlantic summer storm track: mechanisms and impacts on european climate. Environ. Res. Lett. 8, 034037. doi: 10.1088/1748-9326/8/3/034037
Dunstone, N., Smith, D., Scaife, A., Hermanson, L., Eade, R., Robinson, N., et al. (2016). Skilful predictions of the winter north atlantic oscillation one year ahead. Nat. Geosci. 9, 809–814. doi: 10.1038/ngeo2824
Dunstone, N., Smith, D., Scaife, A., Hermanson, L., Fereday, D., O'Reilly, C., et al. (2018). Skilful seasonal predictions of summer european rainfall. Geophys. Res. Lett. 45, 3246–3254. doi: 10.1002/2017GL076337
Folland, C. K., Knight, J., Linderholm, H. W., Fereday, D., Ineson, S., and Hurrell, J. W. (2009). The summer north atlantic oscillation: past, present, and future. J. Clim. 22, 1082–1103. doi: 10.1175/2008JCLI2459.1
Gastineau, G., and Frankignoul, C. (2015). Influence of the north atlantic sst variability on the atmospheric circulation during the twentieth century. J. Clim. 28, 1396–1416. doi: 10.1175/JCLI-D-14-00424.1
Giorgetta, M. A., Jungclaus, J., Reick, C. H., Legutke, S., Bader, J., Böttinger, M., et al. (2013). Climate and carbon cycle changes from 1850 to 2100 in mpi-esm simulations for the coupled model intercomparison project phase 5. J. Adv. Model. Earth Syst. 5, 572–597. doi: 10.1002/jame.20038
Haarsma, R. J., Selten, F., Hurk, B. V., Hazeleger, W., and Wang, X. (2009). Drier mediterranean soils due to greenhouse warming bring easterly winds over summertime central europe. Geophys. Res. Lett. 36, L036617. doi: 10.1029/2008GL036617
Hall, R. J., Jones, J. M., Hanna, E., Scaife, A. A., and Erdélyi, R. (2017). Drivers and potential predictability of summer time north atlantic polar front jet variability. Clim. Dyn. 48, 3869–3887. doi: 10.1007/s00382-016-3307-0
Ho, C. K., Hawkins, E., Shaffrey, L., Bröcker, J., Hermanson, L., Murphy, J. M., et al. (2013). Examining reliability of seasonal to decadal sea surface temperature forecasts: the role of ensemble dispersion. Geophys. Res. Lett. 40, 5770–5775. doi: 10.1002/2013GL057630
Jain, A. K., and Dubes, R. C. (1988). Algorithms for Clustering Data. Upper Saddle River, NJ: Prentice-Hall, Inc.
Johnson, N. C. (2013). How many enso flavors can we distinguish? J. Clim. 26, 4816–4827. doi: 10.1175/JCLI-D-12-00649.1
Jungclaus, J., Fischer, N., Haak, H., Lohmann, K., Marotzke, J., Matei, D., et al. (2013). Characteristics of the ocean simulations in the max planck institute ocean model (mpiom) the ocean component of the mpi-earth system model. J. Adv. Model. Earth Syst. 5, 422–446. doi: 10.1002/jame.20023
Kohonen, T. (1984). Self-Organization and Associative Memory. Berlin; Heidelberg; New York, NY; Tokyo: Springer.
Kohonen, T. (2013). Essentials of the self-organizing map. Neural Netw. 37, 52–65. doi: 10.1016/j.neunet.2012.09.018
Mariotti, A., Baggett, C., Barnes, E. A., Becker, E., Butler, A., Collins, D. C., et al. (2020). Windows of opportunity for skillful forecasts subseasonal to seasonal and beyond. Bull. Am. Meteorol. Soc. 101, E608–E625. doi: 10.1175/BAMS-D-18-0326.A
Mignot, J., Mejia, C., Sorror, C., Sylla, A., Crépon, M., and Thiria, S. (2020). Towards an objective assessment of climate multi-model ensembles-a case study: the senegalo-mauritanian upwelling region. Geosci. Model Dev. 13, 2723–2742. doi: 10.5194/gmd-13-2723-2020
Mishra, N., Prodhomme, C., and Guemas, V. (2019). Multi-model skill assessment of seasonal temperature and precipitation forecasts over europe. Clim. Dyn. 52, 4207–4225. doi: 10.1007/s00382-018-4404-z
Neddermann, N. (2019). Seasonal prediction of European summer climate: a process-based approach (Ph.D. thesis). Universität Hamburg Hamburg.
Neddermann, N.-C., Müller, W. A., Dobrynin, M., Düsterhus, A., and Baehr, J. (2018). Seasonal predictability of european summer climate re-assessed. Clim. Dyn. 53, 3039–3056. doi: 10.1007/s00382-019-04678-4
Oliveira, J. C., Zorita, E., Koul, V., Ludwig, T., and Baehr, J. (2020). “Forecast opportunities for european summer climate ensemble predictions using self-organising maps,” in Proceedings of the 10th International Conference on Climate Informatics, (New York, NY: Association for Computing Machinery), 67–71.
O'Reilly, C. H., Woollings, T., and Zanna, L. (2017). The dynamical influence of the atlantic multidecadal oscillation on continental climate. J. Clim. 30, 7213–7230. doi: 10.1175/JCLI-D-16-0345.1
Osborne, J. M., Collins, M., Screen, J. A., Thomson, S. I., and Dunstone, N. (2020). The north atlantic as a driver of summer atmospheric circulation. J. Clim. 33, 7335–7351. doi: 10.1175/JCLI-D-19-0423.1
Ossó, A., Sutton, R., Shaffrey, L., and Dong, B. (2018). Observational evidence of european summer weather patterns predictable from spring. Proc. Natl. Acad. Sci. U.S.A. 115, 59–63. doi: 10.1073/pnas.1713146114
Ossó, A., Sutton, R., Shaffrey, L., and Dong, B. (2020). Development, amplification, and decay of atlantic/european summer weather patterns linked to spring north atlantic sea surface temperatures. J. Clim. 33, 5939–5951. doi: 10.1175/JCLI-D-19-0613.1
Poli, P., Hersbach, H., Dee, D. P., Berrisford, P., Simmons, A. J., Vitart, F., et al. (2016). Era-20c: An atmospheric reanalysis of the twentieth century. J. Clim. 29, 4083–4097. doi: 10.1175/JCLI-D-15-0556.1
Polo, I., Ullmann, A., Roucou, P., and Fontaine, B. (2011). Weather regimes in the euro-atlantic and mediterranean sector, and relationship with west african rainfall over the 1989-2008 period from a self-organizing maps approach. J. Clim. 24, 3423–3432. doi: 10.1175/2011JCLI3622.1
Reusch, D. B., Alley, R. B., and Hewitson, B. C. (2005). Relative performance of self-organizing maps and principal component analysis in pattern extraction from synthetic climatological data. Polar. Geography 29, 188–212. doi: 10.1080/789610199
Rieke, O., Greatbatch, R. J., and Gollan, G. (2021). Nonstationarity of the link between the tropics and the summer east atlantic pattern. Atmosphere. Sci. Lett. 22, e1026. doi: 10.1002/asl.1026
Rousi, E., Selten, F., Rahmstorf, S., and Coumou, D. (2021). Changes in north atlantic atmospheric circulation in a warmer climate favor winter flooding and summer drought over europe. J. Clim. 34, 2277–2295. doi: 10.1175/JCLI-D-20-0311.1
Stevens, B., Giorgetta, M., Esch, M., Mauritsen, T., Crueger, T., Rast, S., et al. (2013). Atmospheric component of the mpi-m earth system model: Echam6. J. Adv. Model. Earth Syst. 5, 146–172. doi: 10.1002/jame.20015
Trouet, V., Babst, F., and Meko, M. (2018). Recent enhanced high-summer north atlantic jet variability emerges from three-century context. Nat. Commun. 9, 1–9. doi: 10.1038/s41467-017-02699-3
Vettigli, G. (2019). Minisom: Minimalistic and Numpybased Implementation of the Self Organizing Map. Release 2.1.5. Available online at: http://github.com/JustGlowing/minisom
Weisheimer, A., Decremer, D., MacLeod, D., O'Reilly, C., Stockdale, T. N., Johnson, S., et al. (2019). How confident are predictability estimates of the winter north atlantic oscillation? Q. J. R. Meteorol. Soc. 145:140–159. doi: 10.1002/qj.3446
Woollings, T., Franzke, C., Hodson, D., Dong, B., Barnes, E. A., Raible, C., et al. (2015). Contrasting interannual and multidecadal nao variability. Clim. Dyn. 45, 539–556. doi: 10.1007/s00382-014-2237-y
Keywords: self-organizing maps, seasonal ensemble prediction, European summer, predictability, North Atlantic Oscillation, jet stream
Citation: Carvalho-Oliveira J, Borchert LF, Zorita E and Baehr J (2022) Self-Organizing Maps Identify Windows of Opportunity for Seasonal European Summer Predictions. Front. Clim. 4:844634. doi: 10.3389/fclim.2022.844634
Received: 28 December 2021; Accepted: 10 February 2022;
Published: 01 April 2022.
Edited by:
Nachiketa Acharya, The Pennsylvania State University, United StatesReviewed by:
Manmeet Singh, Indian Institute of Tropical Meteorology (IITM), IndiaRajib Chattopadhyay, Indian Institute of Tropical Meteorology (IITM), India
Copyright © 2022 Carvalho-Oliveira, Borchert, Zorita and Baehr. This is an open-access article distributed under the terms of the Creative Commons Attribution License (CC BY). The use, distribution or reproduction in other forums is permitted, provided the original author(s) and the copyright owner(s) are credited and that the original publication in this journal is cited, in accordance with accepted academic practice. No use, distribution or reproduction is permitted which does not comply with these terms.
*Correspondence: Julianna Carvalho-Oliveira, anVsaWFubmEuY2FydmFsaG8ub2xpdmVpcmFAdW5pLWhhbWJ1cmcuZGU=