- 1Center for Language and Cognition Groningen, University of Groningen, Groningen, Netherlands
- 2Department of Experimental Psychology, University of Groningen, Groningen, Netherlands
- 3Department of Experimental Psychology, University of Oxford, Oxford, United Kingdom
- 4College of Information Sciences and Technology, The Pennsylvania State University, State College, PA, United States
- 5Department of Statistical Methods and Psychometrics, University of Groningen, Groningen, Netherlands
Language processing is not an isolated capacity, but is embedded in other aspects of our cognition. However, it is still largely unexplored to what extent and how language processing interacts with general cognitive resources. This question can be investigated with cognitively constrained computational models, which simulate the cognitive processes involved in language processing. The theoretical claims implemented in cognitive models interact with general architectural constraints such as memory limitations. This way, it generates new predictions that can be tested in experiments, thus generating new data that can give rise to new theoretical insights. This theory-model-experiment cycle is a promising method for investigating aspects of language processing that are difficult to investigate with more traditional experimental techniques. This review specifically examines the language processing models of Lewis and Vasishth (2005), Reitter et al. (2011), and Van Rij et al. (2010), all implemented in the cognitive architecture Adaptive Control of Thought—Rational (Anderson et al., 2004). These models are all limited by the assumptions about cognitive capacities provided by the cognitive architecture, but use different linguistic approaches. Because of this, their comparison provides insight into the extent to which assumptions about general cognitive resources influence concretely implemented models of linguistic competence. For example, the sheer speed and accuracy of human language processing is a current challenge in the field of cognitive modeling, as it does not seem to adhere to the same memory and processing capacities that have been found in other cognitive processes. Architecture-based cognitive models of language processing may be able to make explicit which language-specific resources are needed to acquire and process natural language. The review sheds light on cognitively constrained models of language processing from two angles: we discuss (1) whether currently adopted cognitive assumptions meet the requirements for language processing, and (2) how validated cognitive architectures can constrain linguistically motivated models, which, all other things being equal, will increase the cognitive plausibility of these models. Overall, the evaluation of cognitively constrained models of language processing will allow for a better understanding of the relation between data, linguistic theory, cognitive assumptions, and explanation.
Introduction
Language is one of the most remarkable capacities of the human mind. Arguably, language is not an isolated capacity of the mind but is embedded in other aspects of cognition. This can be seen in, for example, linguistic recursion. Although linguistic recursion (e.g., “the sister of the father of the cousin of…”) could in principle be applied infinitely many times, if the construction becomes too complex we will lose track of its meaning due to memory constraints (Gibson, 2000; Fedorenko et al., 2013). Even though there are ample examples of cognitive resources like memory playing a role in language processing (e.g., King and Just, 1991; Christiansen and Chater, 2016; Huettig and Janse, 2016), it is still largely unexplored to what extent language processing and general cognitive resources interact. That is, which general cognitive resources and which language processing-specific resources are used for language processing? For example, is language processing supported by the same memory system that is used in other cognitive processes? In this review, we will investigate to what extent general cognitive resources limit and influence models of linguistic competence. To this end, we will review cognitively constrained computational models of language processing implemented in the cognitive architecture Adaptive Control of Thought—Rational (ACT-R) and evaluate how general cognitive limitations influence linguistic processing in these models. These computational cognitive models explicitly implement theoretical claims, for example about language, based on empirical observations or experimental data. The evaluation of these models will generate new insights about the interplay between language and other aspects of cognition.
Memory is one of the most important general cognitive principles for language processing. In sentence processing, words have to be processed rapidly, because otherwise the memory of the preceding context, necessary for understanding the complete sentence, will be lost (Christiansen and Chater, 2016). Evidence that language processing shares a memory system with other cognitive processes can be found in the relation between general working memory tests and linguistic tests. For example, individual differences in working memory capacity have been found to play a role in syntactic processing (King and Just, 1991), predictive language processing (Huettig and Janse, 2016), and discourse production (Kuijper et al., 2015). Besides memory, other factors like attentional focus (Lewis et al., 2006) and processing speed (Hendriks et al., 2007) have been argued to influence linguistic performance. Thus, it seems apparent that language processing is not an isolated capacity but is embedded in other aspects of cognition. This claim conflicts with the traditional view that language is a specialized faculty (cf. Chomsky, 1980; Fodor, 1983). It is therefore important to note that computational cognitive models can be used to investigate both viewpoints, i.e., to investigate to what extent general cognitive resources can be used in language processing but also to investigate to what extent language is a specialized process. It has also been argued that language processing is a specialized process that is nevertheless influenced by a range of general cognitive resources (cf. Newell, 1990; Lewis, 1996). Therefore, we argue that the potential influence and limitations of general cognitive resources should be taken into account when studying theories of language processing.
To be able to account for the processing limitations imposed by a scarcity of cognitive resources, theories of language need to be specified as explicitly as possible with regards to, for example, processing steps, the incrementality of processing, memory retrievals, and representations. This allows for a specification of what belongs to linguistic competence and what belongs to linguistic performance (Chomsky, 1965): competence is the knowledge a language user has, whereas performance is the output that a language user produces, which results from his competence in combination with other (cognitive) factors (see Figure 1 for examples). Many linguistic theories have been argued to be theories of linguistic competence that abstract away from details of linguistic performance (Fromkin, 2000). These theories rarely make explicit how the step from competence to performance is made. In order to create a distinction between competence and performance, an increasing emphasis is placed on grounding linguistic theories empirically by creating the step from an abstract theory to concrete, testable predictions (cf. e.g., Kempen and Hoenkamp, 1987; Roelofs, 1992; Baayen et al., 1997; Reitter et al., 2011). Formalizing language processing theories explicitly thus means that the distinction between linguistic competence and linguistic performance can be explained and makes it possible to examine which cognitive resources, according to a language processing theory, are needed to process language (see also Hale, 2011).
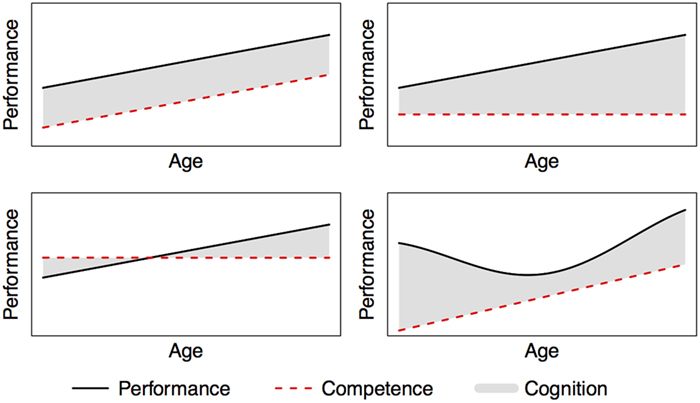
Figure 1. The above graphs show four possible relationships between competence, cognition and performance. Performance is influenced by competence and cognition. If someone’s performance (black solid line) increases over age, this could be due to the competence (red dashed line) increasing (as displayed in the upper left graph), or due to cognition (shaded area) increasing, while competence stays constant (as displayed in the upper right graph). Cognitive limitations can prevent performance from reaching full competence (lower left graph). Competence and cognition can also both change over age and influence performance. The lower right graph shows the classical performance curve of U-shaped learning, in which performance initially decreases even though competence is increasing. The graphs are a simplification, as factors other than competence and cognition could also influence performance, for example motor skills.
The importance of explicitly specified linguistic theories that distinguish between competence and performance can be seen in the acquisition of verbs. Children show a U-shaped learning curve (see Pauls et al., 2013 for an overview, U-shaped learning curve is depicted in Figure 1) when learning past tenses of verbs, using the correct irregular form first (e.g., the past tense ate for eat), then using the incorrect regular form of irregular verbs (e.g., eated), before using the correct irregular form again. It is conceivable that whereas children’s performance initially decreases, children are in the process of learning how to correctly form irregular past tenses and therefore have increasing competence (cf. Taatgen and Anderson, 2002). In this example, explicitly specifying the processing that is needed to form verb tenses and how this processing uses general cognitive resources could explain why children’s performance does not match their competence. Another example of performance deviating from competence can be seen in the comprehension and production of pronouns: whereas 6-year-old children generally produce pronouns correctly (they have the competence, see Spenader et al., 2009), they often make mistakes in pronoun interpretation (they show reduced performance, Chien and Wexler, 1990).
Especially when different linguistic theories have been put forward to explain similar phenomena, it is important to be able to compare and test the theories on the basis of concrete predictions. Linguistic theories are often postulated without considering cognitive resources. Therefore, it is important to investigate how well these theories perform under realistic cognitive constraints; this will provide information about their cognitive plausibility. Cognitively constrained computational models (from now on: cognitive models) are a useful tool to compare linguistic theories while taking into account the limitations imposed by a scarcity of cognitive resources and can be used to investigate the relation between underlying linguistic competence and explicit predictions about performance. Thus, by implementing a linguistic theory into a cognitive model, language processing is embedded in other aspects of cognition, and the extent can be investigated to which assumptions about general cognitive resources influence models of linguistic competence.
As cognitive models, we will consider computational models simulating human processing that are constrained by realistic and validated assumptions about human processing. Such cognitive models can generate new predictions that can be tested in further experiments, generating new data that can give rise to new implementations. This theory-model-experiment cycle is a promising method for investigating aspects of language processing that are difficult to investigate with standard experimental techniques, which usually provide insight into performance (e.g., behavior, responses, response times), but not competence. Cognitive models require linguistic theories, that usually describe competence, to be explicitly specified. This way, the performance of competing linguistic theories, which often have different approaches to the structure and interpretation of language, can be investigated using cognitive models. Contrary to other computational modeling methods, cognitive models simulate the processing of a single individual. Because of this, it can be investigated how individual variations in cognitive resources (which can be manipulated in a model) influence a linguistic theory’s performance.
The comparison of cognitive models that use different linguistic approaches is most straightforward when they make use of the same assumptions about cognitive resources, and thus are implemented in the same cognitive architecture. This review will therefore focus on cognitive models developed in the same domain-general cognitive architecture, ACT-R (Anderson et al., 2004). There are several other cognitive architectures available (e.g., EPIC: Kieras and Meyer, 1997; NENGO: Stewart et al., 2009), but in order to keep the assumptions about general cognitive resources roughly constant, this review will only consider models implemented in ACT-R. Over the past years, several linguistic phenomena have been implemented in ACT-R, such as metaphors (Budiu and Anderson, 2002), agrammatism (Stocco and Crescentini, 2005), pronominal binding (Hendriks et al., 2007), and presupposition resolution (Brasoveanu and Dotlačil, 2015). In order to obtain a broad view of cognitively constrained models of linguistic theories, we will examine three models of different linguistic modalities (comprehension, production, perspective taking), that all take a different linguistic approach, in depth: the syntactic processing model of Lewis and Vasishth (2005), the syntactic priming model of Reitter et al. (2011), and the pronoun processing model of Van Rij et al. (2010). By examining models of different linguistic modalities that take different linguistic approaches, we aim to provide a more unified understanding of how language processing is embedded within general cognition, and investigate how proficient language use is achieved. The selected models are all bounded by the same assumptions about cognitive capacities and seriality of processing as provided by the cognitive architecture ACT-R, which makes them optimally comparable. Their comparison will provide insight into the extent to which assumptions about general cognitive resources influence models of linguistic competence.
This paper is organized as follows. First, we will discuss the components of ACT-R that are most relevant in our discussion of language processing models, in order to explain how cognitive resources play a role in this architecture. Then, we will outline the different linguistic approaches that are used in the models. Finally, we will discuss the selected ACT-R models of language processing in more detail. Importantly, it will be examined how general cognitive resources are used in the models and how these cognitive resources and linguistic principles interact.
Basic ACT-R Components
Adaptive Control of Thought—Rational (Anderson, 1993, 2007; Anderson et al., 2004) is a cognitive architecture in which models can be implemented to simulate a certain process or collection of processes. Of specific interest for this review is the simulation of language-related processes, such as interpreting or producing a sentence. Cognitive models in ACT-R are restricted by general cognitive resources and constraints embedded in the ACT-R architecture. Examples of such cognitive resources, that are of importance when modeling language, are memory, processing speed, and attention. By implementing a model of a linguistic theory in ACT-R, one can thus examine how this linguistic theory behaves in interaction with other aspects of cognition.
Adaptive Control of Thought—Rational aims to explain human cognition as the interaction between a set of functional modules. Each module has a specific function, such as perception, action, memory, and executive function [see Anderson et al. (2004) for an overview]. Modules can be accessed by the model through buffers. The information in these buffers represents information that is in the focus of attention. Only the information that is in a buffer can be readily used by the model. An overview of the standard ACT-R modules and buffers is shown in Figure 2. The modules most relevant for language processing, the declarative memory module and the procedural memory module, will be discussed in more detail below.
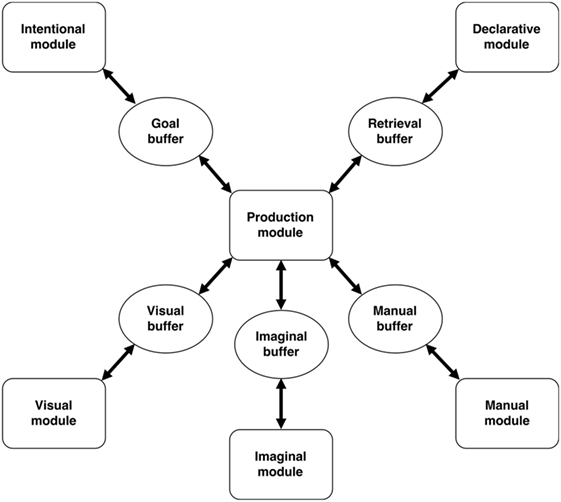
Figure 2. An overview of the standard modules and buffers in Adaptive Control of Thought—Rational [based on Anderson et al. (2004)].
The declarative memory stores factual information as chunks. Chunks are pieces of knowledge that can store multiple properties, such as that there is a cat with the name “Coco,” whose color is “gray.” The information in a chunk can only be used after the chunk has been retrieved from the declarative memory and has been placed in the corresponding retrieval buffer. In order to retrieve information from memory, a retrieval request must be made. Only chunks with an activation that exceeds a predetermined activation threshold can be retrieved. The higher the activation of a chunk, the more likely it is to be retrieved. The base-level activation of a chunk increases when a chunk is retrieved from memory, but decays over time. This way, the recency and frequency of a chunk influence a chunk’s activation, and thereby its chance of recall and its retrieval time (in line with experimental findings, e.g., Deese and Kaufman, 1957; Allen and Hulme, 2006). Additionally, information that is currently in the focus of attention (i.e., in a buffer) can increase the probability that associated chunks are recalled by adding spreading activation to a chunk’s base-level activation. The activation of chunks can additionally be influenced by noise, occasionally causing a chunk with less activation to be retrieved over a chunk with more activation.
Whereas the declarative memory represents factual knowledge, the procedural memory represents knowledge about how to perform actions. The procedural memory consists of production rules, which have an if-then structure. An example of the basic structure of a production rule is as follows:
IF
a new word is attended
THEN
retrieve lexical information about this word from memory
The THEN-part of a production rule is executed when the IF-part matches the current buffer contents. Production rules are executed one by one. If the conditions of several production rules are met, the one with the highest utility is selected. This utility reflects the usefulness the rule has had in the past and can be used to learn from feedback, both positively and negatively (for more detail on utilities, see Anderson et al., 2004). New production rules can be learned on the basis of existing rules and declarative knowledge (production compilation, Taatgen and Anderson, 2002).
Several general cognitive resources and further resources that are important for language processing are incorporated in the ACT-R architecture, such as memory, speed of processing, and attention. Long-term memory corresponds to the declarative module in ACT-R. Short-term or working memory is not incorporated as a separate component in ACT-R (Borst et al., 2010) but emanates from the interaction between the buffers and the declarative memory. Daily et al. (2001) proposed that the function of working memory can be simulated in ACT-R by associating relevant information with information that is currently in focus (through spreading activation). Thus, working memory capacity can change as a result of a change in the amount of spreading activation in a model.
Crucially, all above mentioned operations take time. Processing in ACT-R is serial, meaning that only one retrieval from declarative memory and only one production rule execution can be done at any point in time (this is known as the serial processing bottleneck, see Anderson, 2007). The retrieval of information from declarative memory is faster and more likely to succeed if a chunk has a high activation (for details see Anderson et al., 2004). Because a chunk’s activation increases when it is retrieved, chunks that have been retrieved often will have a high activation and will therefore be retrieved more quickly. Production rules in ACT-R take a standard amount of time to fire (50 ms). Rules that are often used in succession can merge into a new production rule. These new rules are a combination of the old rules that were previously fired in sequence, making the model more efficient. Thus, increasing activation and production compilation allow a model’s processing speed to increase through practice and experience.
As described, memory and processing speed are examples of general cognitive principles in ACT-R, that will be important when implementing models that perform language processing. In the next section, three linguistic approaches will be discussed. These approaches are relevant for the three cognitive models reviewed in the remainder of the paper.
Linguistic Approaches
Cognitive models can be used to implement any linguistic approach, and as such are not bound to one method or theory. In principle any of the theories that have been proposed in linguistics to account for a speaker’s linguistic competence, such as Combinatorial Categorial Grammar (Steedman, 1988), construction grammar (Fillmore et al., 1988), generative syntax (Chomsky, 1970), Head-driven Phrase Structure Grammar (Pollard and Sag, 1994), Lexical Functional Grammar (Bresnan, 2001), Optimality Theory (OT) (Prince and Smolensky, 1993), Tree-Adjoining Grammar (Joshi et al., 1975), and usage-based grammar (Bybee and Beckner, 2009) could be implemented in a cognitive model. Note that this does not imply that any linguistic theory or approach can be implemented in any cognitive model, as cognitive models place restrictions on what can and cannot be modeled. Different linguistic approaches tend to entertain different assumptions, for example about what linguistic knowledge looks like (universal principles, violable constraints, structured lexical categories, grammatical constructions), the relation between linguistic forms and their meanings, and the levels of representation needed. This then determines whether and how a particular linguistic approach can be implemented in a particular cognitive model.
In this review, we will discuss three specific linguistic approaches that have been implemented in cognitive models, which allows us to compare how general cognitive resources influence the implementation and output (e.g., responses, response times) of these modeled linguistic approaches. The three linguistic approaches that will be discussed have several features in common but also differ in a number of features: X-bar theory (Chomsky, 1970), Combinatorial Categorial Grammar (Steedman, 1988), and OT (Prince and Smolensky, 1993). These linguistic approaches are implemented in the cognitive models discussed in the next section.
Generative syntax uses X-bar theory to build syntactic structures (Chomsky, 1970). X-bar theory reflects the assumption that the syntactic representation of a clause is hierarchical and can be presented as a binary branching tree. Phrases are built up around a head, which is the principal category. For example, the head of a verb phrase is the verb, and the head of a prepositional phrase is a preposition. To the left or right of this head, other phrases can be attached in the hierarchical structure.
Combinatory Categorial Grammar (CCG) (Steedman, 1988) builds the syntactic structure of a sentence in tandem with the representation of the meaning of the sentence. It is a strongly lexicalized grammar formalism, that proceeds from the assumption that the properties of the grammar follow from the properties of the words in the sentence. That is, each word has a particular lexical category that specifies how that word can combine with other words, and what the resulting meaning will be. In addition, CCG is surface-driven and reflects the assumption that language is processed and interpreted directly, without appealing to an underlying—invisible—level of representation. For one sentence, CCG can produce multiple representations (Steedman, 1988; Reitter et al., 2011). This allows CCG to build syntactic representations incrementally, from left to right.
The linguistic framework of OT (Prince and Smolensky, 1993) reflects the assumption that language is processed based on constraints on possible outputs (words, sentences, meanings). Based on an input, a set of output candidates is generated. Subsequently, these potential outputs are evaluated based on hierarchically ranked constraints; stronger constraints have priority over weaker constraints. The optimal output is the candidate that satisfies the set of constraints best. The optimal output may be a form (in language production) or a meaning (in language comprehension).
Commonalities and Differences
X-bar theory, CCG, and OT have different assumptions about how language is structured. X-bar theory builds a syntactic structure, whereas CCG builds both a syntactic and a semantic representation, and OT builds either a syntactic representation (in language production) or a semantic representation (in language comprehension). Nevertheless, these theories can all be used for the implementation of cognitive models of language processing. In the next section, three cognitive models of language processing will be discussed in detail, with a focus on how the linguistic approaches are implemented and how they interact with other aspects of cognition.
Cognitive Models of Language Processing
In the following sections, three cognitive language models will be described: the sentence processing model of Lewis and Vasishth (2005), the syntactic priming model of Reitter et al. (2011), and the pronoun processing model of Van Rij et al. (2010). The model of Lewis and Vasishth (2005) uses a parsing strategy that is based on X-bar theory, the model of Reitter et al. (2011) uses CCG, and the model of Van Rij et al. (2010) uses OT. The models will be evaluated based on their predictions of novel empirical outcomes and how they achieve these predictions (for example how many parameters are fitted, cf. Roberts and Pashler, 2000). After describing the models separately, the commonalities and differences between these models will be discussed. Based on this, we will review how the interaction between general cognitive resources in ACT-R and linguistic principles from specific linguistic theories can be fruitful in studying cognitive assumptions of linguistic theories.
Modeling Sentence Processing as Skilled Memory Retrieval
The first model that we discuss is the sentence processing model of Lewis and Vasishth (2005). This model is a seminal model forming the basis for many later language processing models (a.o., Salvucci and Taatgen, 2008; Engelmann et al., 2013; Jäger et al., 2015). Lewis and Vasishth’s (Lewis and Vasishth, 2005) sentenced processing model (henceforth the L&V model) performs syntactic parsing based on memory principles: when processing a complete sentence, maintaining the part of the sentence that is already processed in order to integrate it with new incoming information requires (working) memory. The aim of the L&V model is to investigate how working memory processes play a role in sentence processing.
Theoretical Approach
The L&V model uses left-corner parsing (Aho and Ullman, 1972), based on X-bar theory (Chomsky, 1970), to build a syntactic representation of the sentence. The left corner (LC) parser builds a syntactic structure of the input sentence incrementally, and predicts the upcoming syntactic structure as new words are encountered. Thus, LC parsing uses information from the words in the sentence to predict what the syntactic structure of that sentence will be. In doing this, LC parsing combines top-down processing, based on syntactic rules, and bottom-up processing, based on the words in a sentence. An example sentence is (1).
(1) The dog ran.
Left corner parsing is based on structural rules, such as those given below as (a)–(d). These structural rules for example state that a sentence can be made up of a noun phrase (NP) and a verb phrase [rule (a)], and that a NP can be made up of a determiner and a noun [rule (b)]. An input (word) is nested under the lefthand-side (generally an overarching category) of a structural rule if that rule contains the input on its LC. For example, in sentence (1), the is a determiner (Det) according to structural rule (c), which itself is on the LC of rule (b) and thus it is nested under an NP. This NP is on the LC of rule (a). The result of applying these rules is the phrase-structure tree shown in Figure 3.
(a) S → NP VP
(b) NP → Det N
(c) Det → the
(d) N → dog
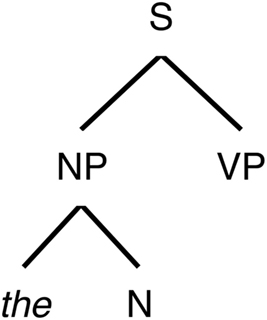
Figure 3. A tree structure generated by left corner parsing of the word the from Example (1) by applying rules (c), (b), and (a) consecutively [based on Lewis and Vasishth (2005)].
Importantly, the generated tree also contains syntactic categories that have not been encountered yet (like N and VP in Figure 3), so it contains a prediction of the upcoming sentence structure. When the next word, dog, is now encountered, it can be integrated with the existing tree immediately after applying rule (d).
Implementation
The L&V model parses a sentence on the basis of guided memory retrievals. Declarative memory is used as the short- and long-term memory needed for sentence processing. The declarative memory holds lexical information as well as any syntactic structures that are built during sentence processing. The activation of these chunks is influenced by the standard ACT-R declarative memory functions, and so their activation (and with this their retrieval probability and latency) is influenced by the recency and frequency with which they were used. Similarity-based interference occurs because the effectiveness of a retrieval request is reduced as the number of items associated with the specific request increases.
Grammatical knowledge however is not stored in the declarative memory but is implemented as procedural knowledge in production rules. That is, the knowledge about how sentences are parsed is stored in a large number of production rules, which interact with the declarative memory when retrieving lexical information or constituents (syntactic structures).
The L&V model processes a sentence word for word using the LC parsing algorithm described in Section “Theoretical Approach.” An overview of the model’s processing steps is shown in Figure 4. After a word is attended [for example, the from Example (1), Box 1], lexical information about this word is retrieved from memory and stored in the lexical buffer (Box 2). Based on the syntactic category of the word and the current state of the model, the model looks for a prior constituent that the new syntactic category could be attached to (Box 3). In our example, the is a determiner and it is the first word, so a syntactic structure with a determiner will be retrieved. The model then creates a new syntactic structure by attaching the new word to the retrieved constituent (Box 4). A new word is then attended [dog in Example (1), Box 1]. This cycle continues until no new words are left to attend.
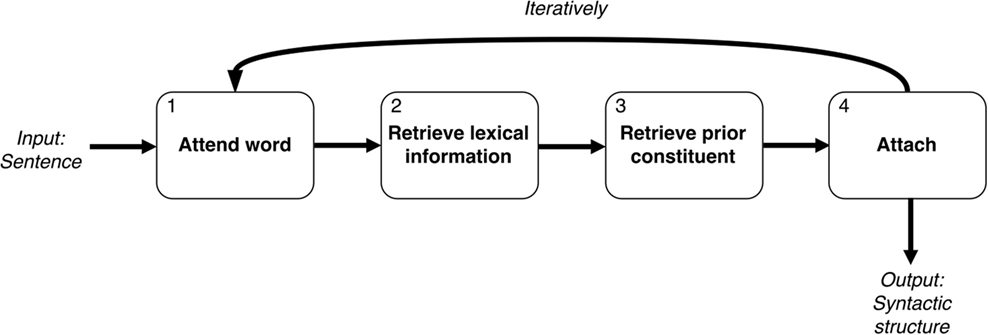
Figure 4. Overview of the processing steps of L&V’s sentence processing model [based on Lewis and Vasishth (2005)]. The model processes one word at a time when processing a sentence such as Example (1), first retrieving its lexical information and then retrieving a prior constituent for the new word to be attached to.
Evaluation
Lewis and Vasishth (2005) presented several simulation studies, showing that their model can account for reading times from experiments. The model also accounts for the effects of the length of a sentence (short sentences are read faster than long sentences) and structural interference (high interference creates a bigger delay in reading times than low interference) on unambiguous and garden-path sentences. With a number of additions (that are outside the scope of this review), the model can be made to cope with gapped structures and embedded structures, as well as local ambiguity (see Lewis and Vasishth, 2005, for more detail).
Predictions
Lewis and Vasishth (2005) compared their output to existing experiments, rather than making explicit predictions about new experiments. The model does however provide ideas about why any discrepancies between the model and the fitted data occur, which could be seen as predictions, although these predictions have not been tested in new experiments. For example, in a simulation comparing the L&V models’ simulated reading times of subject relative clauses vs. object relative clauses to data from Grodner and Gibson (2005), the model overestimates the cost of object-gap filling for object relative clauses. The prediction following from the model is that adjusting the latency, a standard ACT-R parameter that influences the time it takes to perform a chunk retrieval, would reduce the difference between model and data. Thus, the prediction is that the retrieval latency of chunks may be lower in this type of language processing than in other cognitive processes.
Linguistic Principles
X-bar theory is a widely known approach to syntactic structure. Although already previously implemented as an LC parser (Aho and Ullman, 1972), it is interesting to examine this linguistic approach in interaction with memory functions. Importantly, the use of LC parsing allowed the L&V model to use a top-down (prediction-based, cf. Chesi, 2015) as well as bottom-up (input-based, cf. Chomsky, 1993) processing, which increases its efficiency.
Cognitive Principles
Many of the cognitive principles used in the L&V model are taken directly from ACT-R: memory retrievals are done from declarative memory, the grammatical knowledge needed for parsing is incorporated in production rules, and sentences are processed serially (word by word). Memory plays a very important role in the model, as processing sentences requires memory of the recent past. For all memory functions, the same principles of declarative memory are used as would be used for non-linguistic processes. For the L&V model, the standard ACT-R architecture was expanded with a lexical buffer, which holds a lexical chunk after it is retrieved from the declarative memory. Thus, the model assumes the use of general memory functions for language processing, but added a specific attention component to store linguistic (lexical) information that is in the focus of attention.
The speed of processing required for language processing is achieved in the L&V model by keeping the model’s processing down to the most efficient way to do things: the processing of a word takes a maximum of three production rules and two memory retrievals, serially. This however includes only the syntactic processing, and not, for example, any semantic processing. It remains to be investigated therefore how the model would function if more language processing elements, that take additional time to be executed due to the serial processing bottleneck, are added.
Limitations and Future Directions
Although the simulations show a decent fit when compared to data from several empirical experiments, there are a number of phenomena for which a discrepancy is found between the simulation data and some of the experimental data. Specifically, the L&V model overestimates effects of the length of a sentence and underestimates interference effects. Lewis and Vasishth (2005) indicated that part of this discrepancy may be resolved by giving more weight to decay and less weight to interference in the model, but leave the mechanisms responsible for length effects and interference effects open for future research.
Lewis and Vasishth (2005) acknowledged that the model is a first step to modeling complete sentence comprehension and indicated that future extensions might lie in the fields of semantic and discourse processing, the interaction between lexical and syntactic processing, and investigating individual performance based on working memory capacity differences. Indeed, this sentence processing model is an influential model that has served as a building block for further research. For example, Engelmann et al. (2013) used the sentence processing model to study the relation between syntactic processing and eye movements, Salvucci and Taatgen (2008) used the model in their research of multitasking, and Van Rij et al. (2010) and Vogelzang (2017) build their OT model of pronoun resolution on top of L&V’s syntactic processing model.
Modeling Syntactic Priming in Language Production
A second model discussed in this paper is the ACT-R model of Reitter et al. (2011). Their model (henceforth the RK&M model) investigates syntactic priming in language production. Speakers have a choice between different words and grammatical structures to express their ideas. They tend to repeat previously encountered grammatical structures, a pattern of linguistic behavior that is referred to as syntactic or structural priming (for a review, see Pickering and Ferreira, 2008). For example, Bock (1986) found that when speakers were presented with a passive construction such as The boy was kissed by the girl as a description of a picture, they were more likely to describe a new picture using a similar syntactic structure. Effects of priming have been detected with a range of syntactic constructions, including NP variants (Cleland and Pickering, 2003), the order of main and auxiliary verbs (Hartsuiker and Westenberg, 2000), and other structures, in a variety of languages (Pickering and Ferreira, 2008), and in children (Huttenlocher et al., 2004; Van Beijsterveldt and Van Hell, 2009), but also syntactic phrase-structure rules in general (Reitter et al., 2006; Reitter and Moore, 2014).
In the literature, a number of factors that interact with priming have been identified:
• Cumulativity: priming strengthens with each copy of the primed construction (Jaeger and Snider, 2008).
• Decay: the probability of occurrence of a syntactic construction decays over time (Branigan et al., 1999).
• Lexical boost: lexically similar materials increase the chance that priming will occur (Pickering and Branigan, 1998).
• Inverse frequency interaction: priming by less frequent constructions is stronger (Scheepers, 2003).
Besides these factors, differences have been found between fast, short-term priming and slow, long-term adaptation, which is a learning effect that can persist over several days (Bock et al., 2007; Kaschak et al., 2011b). These two different priming effects have been suggested to use separate underlying mechanisms (Hartsuiker et al., 2008), and as such may rely on different cognitive resources.
Syntactic priming is seen as an important effect by which to validate models of syntactic representations and associated learning. Several other models of syntactic priming were proposed (Chang et al., 2006; Snider, 2008; Malhotra, 2009), but none of these are able to account for all mentioned factors as well as short and long term priming. The goal of the RK&M model is thus to account for all types of syntactic priming within a cognitive architecture.
Theoretical Approach
The RK&M model is based on a theoretical approach that explains priming as facilitation of lexical-syntactic access. The model bases its syntactic composition process on a broad-coverage grammar framework, CCG (see Linguistic Approaches, Steedman, 1988, 2000). Categorial Grammars use a small set of combinatory rules and a set of parameters to define the basic operations that yield sentences in a specific language. Most specific information is stored in the lexicon. With the use of CCG, the RK&M model implements the idea of combinatorial categories as in Pickering and Branigan’s (Pickering and Branigan, 1998) model.
In CCG, the syntactic process is the result of combinations of adjacent words and phrases (in constituents). Unlike classical phrase-structure trees, however, the categories that classify each constituent reflect its syntactic and semantic status by stating what other components are needed before a sentence results. For example, the phrase loves toys needs to be combined with a NP to its left, as in Example 2. This phrase is assigned the category S\NP. Similarly, the phrase Dogs love requires a NP to its right to be complete, thus, its category is S//NP. Many analyses (derivations) of a given sentence are possible in CCG.
(2) Dogs love toys.
Combinatory Categorial Grammar allows the RK&M model to generate a syntactic construction incrementally, so that a speaker can start speaking before the entire sentence is planned. However, it also allows the planning of a full sentence before a speaker starts speaking. CCG is generally underspecified and generates more sentences than would be judged acceptable. The RK&M model at least partially addresses this over-generation by employing memory-based ACT-R mechanisms, which also help in providing a cognitively plausible version of a language model.
Implementation
In the RK&M model, lexical forms and syntactic categories are stored in chunks in declarative memory. The activation of any chunk in ACT-R is determined by previous occurrences, which causes previously used, highly active chunks to have a higher retrieval probability, creating a priming effect.
The RK&M model additionally uses spreading activation to activate all syntax chunks that are associated with a lexical form, creating the possibility to express a meaning in multiple ways. Some ways of expressing a meaning are more frequent in language than others, and therefore the amount of spreading activation from a lexical form to a syntax chunk is mediated by the frequency of the syntactic construction. This causes more frequent forms to have a higher activation and therefore to be more likely to be selected. However, a speaker’s choice of syntactic construction can vary on the basis of priming and noise.
To make its theoretical commitments to cue-based, frequency- and recency-governed declarative retrieval, as well as its non-commitments to specific production rules and their timing more clear, the RK&M model was implemented first in ACT-R 6, and then in the ACT-UP implementation of the ACT-R theory (Reitter and Lebiere, 2010).
Syntactic Realization
The RK&M model takes a semantic description of a sentence as input and creates a syntactic structure for this input. The serially executed processing steps of the model are shown in Figure 5 and will be explained on the basis of Example (3).
(3) Sharks bite.
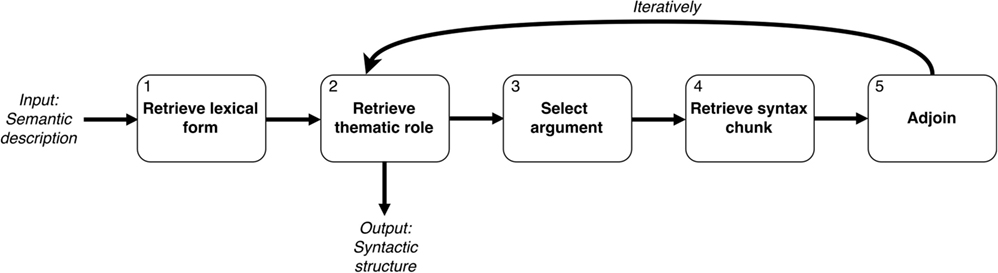
Figure 5. Overview of the processing steps of RK&M’s syntactic priming model, which produces the syntactic structure of a sentence such as Example (3) [based on Reitter et al. (2011)]. First, retrievals of the lexical form of the head and a thematic role are done. Then, the model selects an argument for the thematic role and retrieves a syntax chunk before combining the information according to combinatorial rules of Combinatory Categorial Grammar in the adjoin phase.
First, the model retrieves a lexical form for the head of the sentence (Box 1). In Example (3), this head will be the verb bite. Then the most active thematic role is retrieved from memory (Box 2), which would be the “agent-role” in our example. If no next thematic role can be retrieved, the entire sentence has been generated and an output can be given. The model then identifies the argument associated with the retrieved thematic role and retrieves a lexical form for this argument (Box 3). In the case of the agent-role in Example (3), this will be sharks. Following, the model retrieves a syntax chunk that is associated with the retrieved lexical form (Box 4). The lexical form was sharks, and the corresponding syntax chunk will thus indicate that this is an NP, and that it needs a verb to its right (S/VP). Finally, the model adjoins the new piece of syntactic information with the syntactic structure of the phrase thus far (Box 5), according to the combinatorial rules of CCG. The model then goes back to retrieving the next thematic role (Box 2) and repeats this process until the entire sentence has been generated.
Priming
Within the language production process, syntactic choice points (Figure 5, Box 4) will occur, during which a speaker decides between several possible syntactic variants. The model needs to explicate the probability distribution over possible decisions at that point. This can be influenced by priming.
The time course of priming is of concern in the RK&M model. Immediately after a prime, repetition probability is strongly elevated. The model uses two default ACT-R mechanisms, base-level learning and spreading activation, to account for long-term adaptation and short-term priming. Short-term priming emerges from a combination of two general memory effects: (1) rapid temporal decay of syntactic information and (2) cue-based memory retrieval subject to interfering and facilitating semantic information (Reitter et al., 2011). Long-term priming effects in the model emerge from the increase in base-level activation that occurs when a chunk is retrieved.
Evaluation
In the RK&M model, base-level learning and spreading activation account for long-term adaptation and short-term priming, respectively. By simulating a restricted form of incremental language production, it accounts for (a) the inverse frequency interaction (Scheepers, 2003; Reitter, 2008; Jaeger and Snider, 2013); (b) the absence of a decay in long-term priming (Hartsuiker and Kolk, 1998; Bock and Griffin, 2000; Branigan et al., 2000; Bock et al., 2007); and (c) the cumulativity of long-term adaptation (Jaeger and Snider, 2008). The RK&M model also explains the lexical boost effect and the fact that it only applies to short-term priming, because semantic information is held in short-term memory and serves as a source of activation for associated syntactic material.
The model uses lexical-syntactic associations as in the residual-activation account (Pickering and Branigan, 1998). However, learning remains an implicit process, and routinization (acquisition of highly trained sequences of actions) may still occur, as it would in implicit learning accounts.
The RK&M model accounts for a range of priming effects, but despite providing an account of grammatical encoding, it has not been implemented to explain how speakers construct complex sentences using the broad range of syntactic constructions found in a corpus.
Predictions
Because semantic information is held in short-term memory and serves as a source of activation for associated syntactic material, the RK&M model predicts that lexical boost occurs with the repetition of any lexical material with semantic content, rather than just with repeated head words. This prediction was confirmed with corpus data (Reitter et al., 2011) and also experimentally (Scheepers et al., 2017). The RK&M model also predicts that only content words cause a lexical boost effect. This prediction was not tested on the corpus, although it is compatible with prior experimental results using content words (Corley and Scheepers, 2002; Schoonbaert et al., 2007; Kootstra et al., 2012) and semantically related words (Cleland and Pickering, 2003), and the insensitivity of priming to closed-class words (Bock and Kroch, 1989; Pickering and Branigan, 1998; Ferreira, 2003).
The model predicted cumulativity of prepositional-object construction priming, and it suggested that double-object constructions are ineffective as primes to the point where cumulativity cannot be detected. In an experimental study published later by another lab (Kaschak et al., 2011a), this turned out to be the case.
Linguistic Principles
An important aspect of the RK&M model is that it uses CCG. This allows the model to realize syntactic constructions both incrementally and non-incrementally, without storing large amounts of information. CCG can produce multiple representations of the input at the same time, which reflect the choices that a speaker can make. CCG has enjoyed substantial use on large-scale problems in computational linguistics in recent years. Still, how much does this theoretical commitment (of CCG) limit the model’s applicability? The RK&M model relies, for its account of grammatical encoding, on the principles of incremental planning made possible by categorial grammars. However, for its account of syntactic priming, the deciding principle is that the grammar is lexicalized, and that syntactic decisions involve lower-frequency constructions that are retrieved from declarative (lexical) memory. Of course, ACT-R as a cognitive framework imposes demands on what the grammatical encoder can and cannot do, chiefly in terms of working memory: large, complex symbolic representations such as those necessary to process subtrees in Tree-Adjoining Grammar (Joshi et al., 1975), or large feature structures of unification-based formalisms such as Head-driven Phrase Structure Grammar (Pollard and Sag, 1994) would be implausible under the assumptions of ACT-R.
Cognitive Principles
The RK&M model’s linguistic principles are intertwined with cognitive principles in order to explain priming effects. Declarative memory retrievals and the accompanying activation boost cause frequently used constructions to be preferred. Additionally, the model uses the default ACT-R component of spreading activation to give additional activation to certain syntax chunks, increasing the likelihood that a specific syntactic structure will be used. Working memory capacity is not specified in the RK&M model.
The RK&M model is silent with respect to the implementation of its grammatical encoding algorithms. Standard ACT-R provides for production rules that represent routinized skills. These rules are executed at a rate of one every 50 ms. Whether that is fast enough for grammatical encoding when assuming a serial processing bottleneck, and how production compilation can account for fast processing, is unclear at this time. Production compilation, in ACT-R, can combine a sequence of rule invocations and declarative retrievals into a single, large and efficient production rule. An alternative explanation may be that the production rule system associated with the syntactic process is not implemented by the basal ganglia, the brain structure normally associated with ACT-R’s production rules, but by a language-specific region such as Broca’s area. This language-specific region may allow for faster processing.
Limitations and Future Directions
Some effects related to syntactic priming remain unexplained by the RK&M model. For example, the repetition of thematic and semantic assignments between sentences (Chang et al., 2003) is not a consequence of retrieval of lexical-syntactic material. A future ACT-R model can make use of working memory accounts (cf. Van Rij et al., 2013) to explain repetition preferences leading to such effects.
Modeling the Acquisition of Object Pronouns
The third and final model that is discussed, is Van Rij et al.’s (2010) model for the acquisition of the interpretation of object pronouns (henceforth the RR&H model). In languages such as English and Dutch, an object pronoun (him in Example 4) cannot refer to the local subject (the penguin in Example 4, cf. e.g., Chomsky, 1981). Instead, it must refer to another referent in the context, in our example the sheep. In contrast, reflexives such as “zichzelf” (himself, herself) can only refer to the local subject.
(4) Look, a penguin and a sheep. The penguin is hitting him/himself.
Children up to age seven allow the unacceptable interpretation of the object pronoun “him” (the penguin), although children perform adult-like on the interpretation of reflexives from the age of four (e.g., Chien and Wexler, 1990; Philip and Coopmans, 1996). Interestingly, children as early as 4 years old show adult-like production of object pronouns and reflexives (e.g., De Villiers et al., 2006; Spenader et al., 2009). The ACT-R model is used to investigate why children show difficulties interpreting object pronouns, but not interpreting reflexives or producing object pronouns or reflexives.
Theoretical Account
To explain the described findings on the interpretation of object pronouns and reflexives, Hendriks and Spenader (2006) proposed that children do not lack the linguistic knowledge needed for object pronoun interpretation but fail to take into account the speaker’s perspective. According to this account, formulated within OT (Prince and Smolensky, 1993, see Linguistic Approaches), object pronouns compete with reflexives in their use and interpretation.
In the account of Hendriks and Spenader (2006), two grammatical constraints guide the production and interpretation of pronouns and reflexives. “Principle A” is the strongest constraint, which states that reflexives have the same reference as the subject of the clause. In production, Hendriks and Spenader assume a general preference for producing reflexives over pronouns, which is formulated in the constraint “Avoid Pronouns.”
Hendriks and Spenader (2006) argue that the interpretation of object pronouns is not ambiguous for adults, because they take into account the speakers’ perspective: if the speaker wanted to refer to the subject (e.g., the penguin in Example 4), then the speaker would have used a reflexive in accordance with the constraint Principle A. When the speaker did not use a reflexive, therefore, an adult listener should be able to conclude that the speaker must have wanted to refer to another referent. Although this account can explain the asymmetry in children’s production and interpretation of object pronouns, it does not provide a theory on how children acquire the interpretation of object pronouns. To investigate this question, the theoretical account of Hendriks and Spenader was implemented in ACT-R (Van Rij et al., 2010; see also Hendriks et al., 2007).
Implementation
An overview of the RR&H model is presented in Figure 6. The process of finding the optimal meaning for a form (in comprehension) or finding the optimal form for a meaning (in production) was implemented in ACT-R as a serial process. To illustrate the process, consider the interpretation of the pronoun him.
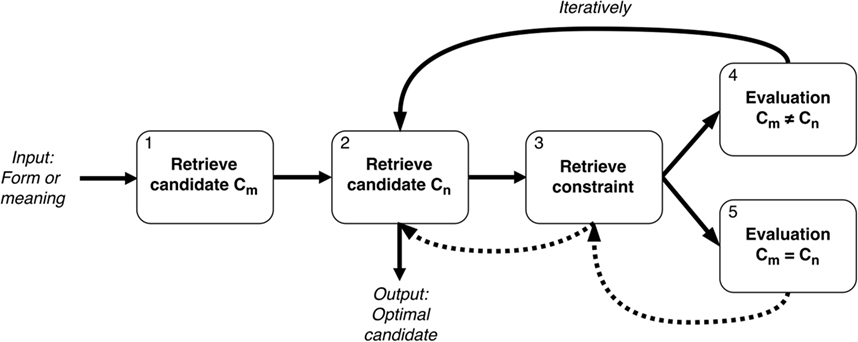
Figure 6. The optimization process as implemented in the RR&H model to determine the optimal meaning for a form such as him in Example (4) (in comprehension), and the optimal form for a meaning such as co-reference [(in production), based on Van Rij et al. (2010)].
Using Grammatical Constraints
When interpreting a pronoun, two consecutive production rules request the retrieval of two candidate interpretations from the model’s declarative memory (Box 1 and Box 2 in Figure 6). The two candidate interpretations are the co-referential interpretation (i.e., reference to the referent expressed by the local subject, e.g., the penguin in Example 4) and the disjoint interpretation (i.e., reference to another referent in the discourse, such as the sheep in Example 4). Consequently, a production rule requests the retrieval of a grammatical constraint from declarative memory. The chunk that represents the constraint Principle A has the highest activation because it is the strongest constraint and is retrieved from memory first (see Box 3).
On the basis of the retrieved constraint, the two candidate interpretations are evaluated (Box 4 and 5). If one of the candidates violates the constraint, the RR&H model tries to replace that candidate by a new candidate (Box 4 and Box 2). If it cannot find a new candidate in memory, the remaining candidate is selected as the optimal interpretation.
If the input was a pronoun, however, none of the candidate interpretations violates Principle A. Therefore, both candidate interpretations are still possible (Box 5). In this situation, the RR&H model retrieves a new constraint (Box 3), Avoid Pronouns. This constraint cannot distinguish between the two candidate meanings either, because it only applies to forms. As both the co-referential and the disjoint interpretation are still possible, the model randomly selects one of the two candidates as the optimal interpretation. The random choice between two optimal candidates reflects children’s behavior in the interpretation of object pronouns.
Perspective Taking
After selecting the optimal interpretation, the RR&H model takes the speaker’s perspective to verify whether the speaker indeed intended to express the selected interpretation (see Figure 7). Taking the speaker’s perspective, the model uses the same optimization mechanism, but now the input is the meaning (optimal interpretation) selected in the previous step when taking the listener’s perspective (m1), and the output is the optimal form to express that meaning (f2).

Figure 7. An optimization process from the perspective of the listener as well as an optimization process from the perspective of the speaker is performed [based on Van Rij et al. (2010)].
Continuing with the example of processing an object pronoun, the model could have selected the co-referential interpretation as the interpretation of the object pronoun when taking the listener’s perspective. In that situation, the input (m1) for the second optimization step, using the speaker’s perspective, would be the co-referential interpretation. The output of the second optimization step (f2) is the reflexive form, because the constraint Avoid Pronouns favors the use of a reflexive over a pronoun.
After the two optimization steps, a new production rule fires that compares the initial input (the object pronoun) with the output (a reflexive, Figure 7 Box 3). As these forms are not identical in our example, the model concludes that a co-referential interpretation is not intended by the speaker: the speaker would have used a reflexive rather than a pronoun to express a co-referential interpretation. As a consequence, the model will take an alternative candidate interpretation, the disjoint interpretation, and will check if the speaker could have intended a disjoint interpretation.
Alternatively, if the model had selected a disjoint interpretation for a pronoun during the first optimization step, the input for the speaker’s perspective (m1) would be a disjoint interpretation. The constraint Principle A would cause the model to select a pronoun rather than a reflexive for expressing the disjoint interpretation (f2). As the original input (f1, a pronoun) and the output (f2, also a pronoun) are identical, the model concludes that the speaker indeed intended a disjoint interpretation.
Although children are expected to use the same perspective taking mechanism as adults, it is assumed that children’s processing is initially too slow to complete this process. The time for pronoun resolution is limited: When the next word comes, the model stops processing the pronoun and redirects its attention to the new word. Gradually however, children’s processing becomes more efficient due to ACT-R’s default mechanism of production compilation (Taatgen and Anderson, 2002). This way, the process becomes more efficient, and over time it is possible to take the perspective of the speaker into account in interpretation.
Evaluation
The RR&H model explains the delay in object pronoun acquisition as arising from the interaction between general cognitive principles and specific linguistic constraints. The model simulations show that children’s non-adult-like performance does not necessarily arise from differences in linguistic knowledge or differences in processing mechanism but may arise because children lack processing efficiency.
Predictions
From the RR&H model simulations, a new prediction was formulated: when children receive sufficient time for pronoun interpretation, they will show more adult-like performance on object pronoun interpretation. Van Rij et al. (2010) tested this prediction by slowing down the speech rate. They found that children indeed performed significantly more adult-like on object pronoun interpretation when they were presented with slowed-down speech compared to normal speech. A second prediction of the RR&H model is that the use of perspective taking in pronoun interpretation is dependent on the input frequency of pronouns. With higher input frequency, the process becomes more efficient in a shorter time (Van Rij et al., 2010; Hendriks, 2014).
Linguistic Principles
The linguistic principles incorporated in the RR&H model is rooted in OT. The underlying idea in OT is that an in principle infinite set of potential candidates is evaluated on the basis of all constraints of the grammar. The serial optimization mechanism implemented in the model is a more constrained version of optimization: the two most likely candidates are compared using the constraints that are most relevant in the context. In this respect, the optimization mechanism could be applied to other linguistic (and non-linguistic) phenomena and is thus potentially generalizable.
Cognitive Principles
Several general cognitive principles are used in the RR&H model. Production compilation learning allowed the model to gradually derive an efficient variant of the general cognitive skill of perspective taking that is specialized for object pronoun interpretation. This specialization mechanism has been applied to model other linguistic and non-linguistic phenomena (e.g., Taatgen and Anderson, 2002). Through the increased efficiency of production rules, as well as through increasing activation of candidates and constraints that were used for pronoun interpretation, the model’s processing speed increases over time.
The RR&H model uses ACT-R’s declarative memory for the storage and retrieval of candidates and constraints. However, no discourse processing was included in the model, and no working memory component was used. Therefore, a remaining question is whether, contrary to what is assumed in other research (Christiansen and Chater, 2016), processing speed limitations on pronoun processing are not imposed by working memory limitations, but by processing efficiently limitations (cf. Kuijper, 2016).
RR&H’s account of the difference between children’s and adults’ processing of pronouns crucially follows from the serial processing bottleneck assumption, as it assumes that children have the knowledge necessary to use bidirectional optimization, including all relevant linguistic knowledge, but cannot make use of it due to time limitations. Proceduralization is used as the explanation for how children arrive at adult performance given the serial processing bottleneck.
Limitations and Future Directions
A potential limitation of RR&H’s object pronoun processing model is that it is not yet clear how to determine the two most likely candidates or how the model can decide what the most relevant constraint is. Another simplification is that both candidate referents were introduced in the previous sentence. An interesting extension of the model would be one in which the discourse status of the referents would also be taken into account (cf. Van Rij et al., 2013). The extended model would need to integrate factors such as first-mention, frequency, recency, grammatical role and role parallelism (Lappin and Leass, 1994), and semantic role (Kong et al., 2009) to account for topicality and the discourse prominence of referents (Grosz et al., 1995), which plays an important role in pronoun resolution (Spenader et al., 2009).
Another future direction for this research would be to investigate why children as early as 4 years old in languages such as Italian and Spanish do not allow unacceptable reference to the local subject for object pronouns (Italian: McKee, 1992; for an overview on Italian see Belletti and Guasti, 2015; Spanish: Baauw, 2002), in contrast to children in languages such as English and Dutch. Thus, this cognitive model could be applied to investigate cross-linguistic variation.
Commonalities and Differences
In the previous sections, we discussed three language processing models in ACT-R that were based on different linguistic approaches. The models were all implemented in the same cognitive architecture, so they are all constrained by the same limitations on cognitive resources. This allows for their comparison, which can provide information about how different aspects of language processing interact with non-linguistic aspects of cognition, and how models addressing different linguistic phenomena can be integrated. In this section, we will discuss the commonalities and differences between these models in more detail, so it can be examined to which extent assumptions about general cognitive resources influence implementations of these specific linguistic approaches. Additionally, their comparison will provide an overview of some choices that can be made when implementing a language processing model, such as how to represent (grammatical) knowledge, and how these choices can directly impact how cognitive resources influence the model. The models’ main differences lie in (1) the language modality, (2) the linguistic approach they take, and (3) how grammatical knowledge is represented.
As for the different language modalities investigated in the three models, the model of Lewis and Vasishth (2005) focuses on sentence interpretation and builds the syntactic representations needed for interpretation. In contrast, the model of Reitter et al. (2011) focuses on sentence production. The model of Van Rij et al. (2010) again focuses on sentence interpretation but includes a sentence production component in its implementation of perspective taking. So, the selected models show that cognitive models can perform both sentence processing as needed for interpretation and sentence processing as needed for production. As the selected models are merely example implementations of linguistic approaches, this shows how versatile cognitive modeling can be.
A second difference between the three models is that the models all take a different linguistic approach, as Lewis and Vasishth (2005) used LC parsing based on X-bar theory, Reitter et al. (2011) used CCG, and Van Rij et al. (2010) used OT. Although a working cognitive model does not prove the necessity of a particular linguistic approach, it shows its sufficiency: the model of Lewis and Vasishth (2005), for example, shows that LC parsing is sufficient to account for experimental data on sentence processing. It should be noted that the three linguistic approaches need not be mutually exclusive. For example, it is conceivable that a model processes sentences based on LC parsing and uses OT to interpret ambiguous pronouns (cf. Van Rij, 2012; Vogelzang, 2017). Additionally, it should be noted that all three theories have been treated as approaches that have remained unquestioned, whereas variations of these approaches may be worth while to consider (cf., e.g., Osborne et al., 2011).
A final important difference between the models is how grammatical knowledge is represented. In Lewis and Vasishth’s (Lewis and Vasishth, 2005) model, lexical information and syntactic structures are stored in declarative memory, but grammatical rules are incorporated as procedural knowledge in production rules. Therefore, their grammatical rules are not subject to the activation functions associated with the declarative memory but are subject to the time constraints of production rule execution. This is different from the model of Reitter et al. (2011), which stores lexical forms as well as syntactic categories as chunks in the declarative memory, and therefore also incorporates the grammatical rules in the declarative memory. The model of Van Rij et al. (2010) incorporates grammatical rules as chunks in the declarative memory. So, the models incorporate grammatical knowledge in different ways, which has consequences for the influence of general cognitive resources on grammatical knowledge. Specifically, knowledge stored in declarative memory is subject to ACT-R’s principles concerning memory activation and retrieval time, whereas knowledge stored in procedural memory is subject to ACT-R’s principles concerning production rule execution time.
Although the three models differ in several respects, they also have a number of important features in common. The most important ones that we will discuss are (1) the restrictions placed on the model performance by general cognitive resources, (2) the assumption of a serial processing bottleneck, and (3) the generation of quantitative predictions.
As all models were implemented in ACT-R, the performance of all models is constrained by the same restrictions on cognitive resources. So, although the models focus on different linguistic phenomena and use different representations, they all use, for example, the same functions of declarative memory for the activation of chunks. Furthermore, they all use the same distinction between procedural and declarative memory and incorporate the constraint that information can only be actively used by the model once it is retrieved from declarative memory. Using the same cognitive architecture therefore makes these different models comparable with regard to how the representations are influenced by cognitive resources.
Another constraint within all the models, also imposed by the cognitive architecture, is the serial processing bottleneck (Anderson, 2007). In ACT-R, only one production rule execution or memory retrieval can be performed at a time. Using serial processing increases the time it takes to perform multiple processing steps. Therefore, the serial processing bottleneck creates timing constraints for the models, influencing predictions about performance. We will discuss the implications of this serial processing bottleneck in more detail in the Section “Discussion.”
Finally, the last commonality is that all models can generate quantitative predictions. In general, linguistic theories only discuss competence and do not address performance and do not explain why the observed performance may not match the competence. Thus, linguistic theories do not explain, for example, why speakers may use a certain form in 80% of the cases, but a different form in the other cases. By implementing theoretical approaches in cognitive models, quantitative predictions about why performance does not match competence can be generated.
Discussion
In this review, we investigated to what extent general cognitive resources influence concretely implemented models of linguistic competence. To this end, we examined the language processing models of Lewis and Vasishth (2005), Reitter et al. (2011), and Van Rij et al. (2010). In this section, we will discuss the benefits and limitations of using a cognitive architecture to implement and investigate theories of linguistic competence, and to what extent general cognitive resources influence performance on the basis of these theories.
Cognitive architectures provide a framework for implementing theories of linguistic competence in a validated account of general cognitive resources related to learning and memory. The three specific models that we discussed showed that the cognitive architecture ACT-R on the one hand provides sufficient freedom to implement different linguistic theories in a plausible manner, and on the other hand sufficiently constrains these theories to account for several differences between linguistic competence and performance. Implementing a linguistic theory in a cognitive architecture forces one to specify, among other things, assumptions about how lexical, syntactic, and semantic knowledge is represented and processed in our mind. These specifications are necessarily constrained by general cognitive resources. Therefore, general cognitive resources such as memory and processing speed also constrain performance on the basis of linguistic theories and are crucial for investigating this performance in a cognitively plausible framework.
By implementing a theory of linguistic competence in a cognitive model, it can be evaluated whether a linguistic theory can account for experimental performance data. The distinction between competence and performance is an advantage of cognitive models over abstract linguistic theories (reflecting competence) and standard experimental measures (measuring performance). A cognitive model thus can not only be used to model performance but can also be used to investigate the reason why full competence may not be reached (e.g., because of memory retrieval limitations: Van Maanen and Van Rijn, 2010, processing speed limitations: Van Rij et al., 2010, or the use of an incorrect strategy: Arslan et al., 2017). As such, cognitive models can account for patterns of linguistic performance that were traditionally accounted for by positing a separate parsing module in the mind specifically for language processing (e.g., Kimball, 1973; Frazier and Fodor, 1978). This line of argumentation has also been explored by Hale (2011), who argues that linguistic theories need to be specified not just on Marr’s computational level, but that it is necessary to specify theories at a level of detail so that they can be implemented, step-by-step, in an algorithmic-level framework and yield precise predictions about behavior. The comparison of models described in this review makes explicit which assumptions have to be made in the cognitive model to incorporate particular linguistic theories. All three cognitive models discussed in this review have been applied to fit human data. In many of these cases, the model could account for the general trends in the data, if not the complete data set. As such, all three models provided an explicit relation between data, theory, and explanation. Although not all models made novel predictions that could be tested in new experiments, this is a strength of cognitive modeling and therefore something every paper on cognitive modeling should include. Adding novel predictions shows that (1) the model was not just fitted to existing data and (2) the model is falsifiable. The latter is important, because falsifiable models allow a theory to be disproven. Providing novel predictions allows other researchers to test these, and gather either support for or evidence against a specific theory.
An additional benefit of cognitive modeling is that individual differences can be investigated. By manipulating, for example, the amount of experience (Van Rij et al., 2010), the amount of working memory capacity (Van Rij et al., 2013), or the rate of forgetting in memory (Sense et al., 2016), different performance levels can be achieved. This way, different individuals can be modeled and it can be investigated why certain mistakes may be made (explanations could be, for example limited experience, limited memory capacity, limited attention span). By combining different simulated individuals, group effects may be explained (Van Rij et al., 2010).
There are, however, also some limitations to modeling language processing in a cognitive architecture. First, all three models that were discussed can account for specific linguistic phenomena, but these only form a small part of language. Scalability is an issue for many models, as expanding their coverage and making them more complex (for example, by combining a model that performs full semantic processing with a model that performs full syntactic processing) will make models slower in any architecture that assumes serial processing. Specifically, although the model of Van Rij et al. (2010) uses the serial processing bottleneck explicitly to account for children’s performance errors, both Lewis and Vasishth (2005) and Reitter et al. (2011) suggest that their models may struggle with this assumption when expanded. It is thus important to keep in mind that the discussed, relatively small, serially implemented models of language processing were sufficient to fit to experimental data, but the serial processing bottleneck may prove to be too strict for sentence processing when a complete language processing model is developed. Moreover, the discussed models are abstractions and simplifications of reality and take into account neither additional internal factors influencing language processing, such as attentional state or focus (Lappin and Leass, 1994; Lewis et al., 2006), emotion (Belavkin et al., 1999), and motivation (Belavkin, 2001), nor external factors such as visual context (Tanenhaus et al., 1995). Once a model has found support for underlying mechanisms of sentence processing, it can be used as a basis for investigating the effects of these additional factors. Therefore, the models discussed can be seen as a first step toward investigating such factors in the future.
A second limitation is related to a concern that Lewis and Vasishth (2005) raised: the degrees of freedom in cognitive models. For any set of cognitive models to be optimally comparable, they should be restricted by the same cognitive resources. However, cognitive architectures provide much freedom regarding different parameters (for example, the memory decay parameter in ACT-R can be changed manually). Therefore, models should generally strive to keep the quantitative parameters constant. If this is done, any variation between models will originate from the production rules and the content of the declarative memory, which is also where (linguistic) theory is implemented.
As a final limitation, any cognitive architecture that does not specify different types of memory (short-term memory, episodic long-term memory, semantic long-term memory) will make it difficult to model language processing in all its complexity. For example, long-term memory is difficult to implement in ACT-R, because all chunks are subject to the same decay in activation over time. Thus, it is a puzzle why people do not forget certain pieces of knowledge that are not retrieved frequently (like, for example, what a hedgehog is). Recent research has found that different types of facts may actually have different decay rates (Sense et al., 2016). This can be important for language processing, because even infrequent words are not forgotten and can still be recognized and used after a long time. A related issue is that cognitive architectures with only one type of memory make it challenging to implement and manipulate working memory capacity. So, although the possibility of manipulating cognitive resources in cognitive models can be seen as a benefit, not restricting how these cognitive resources should be modeled limits its application. As language processing is known to be constrained by working memory capacity, manipulations of working memory capacity would be useful in order to study its effects on linguistic performance. Moreover, when modeling language acquisition or language attrition, working memory may be of great influence, as it can differ between ages (Grivol and Hage, 2011) and in clinical populations (e.g., ADHD: Martinussen et al., 2005; autism spectrum disorder: Barendse et al., 2013; cochlear implant users: AuBuchon et al., 2015). Although the function of working memory can be simulated indirectly through other processes like spreading activation (Daily et al., 2001), restrictions on their implementation in the cognitive architecture would make models more comparable and potentially more cognitively plausible.
Thus, using a cognitive architecture to investigate theories of linguistic competence has clear benefits as well as a number of current limitations. The main question in this review was to what extent general cognitive resources influence concretely implemented models of linguistic competence. An examination of the different cognitive models of linguistic performance provides evidence that well-studied general cognitive resources such as working memory influence language processing. In addition, less well-studied cognitive factors may also play a role, such as number of processing steps (Lewis and Vasishth, 2005) and processing efficiency (Van Rij et al., 2010). The influence of these factors can differ due to differences in, for example, experience, processing strategy, or possibly developmental disorder. Thus, our investigation of different cognitive models emphasizes that not only memory-related resources but also other timing-related resources and factors influence language processing.
As stated, implementations of linguistic theories into a cognitive model can, on the one hand, provide information about whether the theory can sufficiently account for observed performance. On the other hand, they can also be used to investigate cognitive processes. For example, the speed of language processing is so high that it may not be met by the time-consuming processing steps provided by a cognitive model (cf. Vogelzang, 2017), or by the same memory processes that underlie other cognitive processes. So, from the viewpoint of linguistics, but also from the viewpoint of cognitive modeling, the puzzle of highly fast and efficient language processing compared to other cognitive processes is an interesting direction for future research.
Overall, cognitively constrained models can be used to investigate whether a linguistic theory can account for specific linguistic data. The interactions between a particular linguistic approach and general cognitive resources can be investigated through such models, which formalize of relation between competence and performance. Additionally, cognitive models can generate quantitative predictions of the basis of theories of linguistic competence. Because of this, cognitive models of linguistic theories are very suitable for investigating the relation between data, theory and experiments. Moreover, the possibility to model differences in cognitive resources allows for the investigation of individual differences in performance, as well as deviating performance due to aging or developmental disorders. In some cases, the high efficiency of language processing is currently not met by some of the constraining assumptions about cognitive resources. In this sense, cognitive models of language processing can also be used to investigate human cognition, for example in which ways currently adopted cognitive assumptions fail to meet the requirements for language processing. In conclusion, investigating specific linguistic phenomena through cognitive modeling can provide new insights that can complement findings from standard experimental techniques.
Author Contributions
All authors listed have made a substantial, direct, and intellectual contribution to the work and approved it for publication.
Conflict of Interest Statement
The authors declare that the research was conducted in the absence of any commercial or financial relationships that could be construed as a potential conflict of interest.
Acknowledgments
The authors would like to thank Michael Putnam for his comments on an earlier draft of the paper.
Funding
This research was supported by a grant from the Netherlands Organization for Scientific Research (NWO) awarded to Jacolien Van Rij (grant no. 275-70-044). David Reitter acknowledges support from the National Science Foundation grant BCS-1734304.
References
Aho, A. V., and Ullman, J. D. (1972). The Theory of Parsing, Translation, and Compiling. Upper Saddle River, NJ: Prentice-Hall, Inc.
Allen, R., and Hulme, C. (2006). Speech and language processing mechanisms in verbal serial recall. J. Mem. Lang. 55, 64–88. doi: 10.1016/j.jml.2006.02.002
Anderson, J. R. (2007). How Can the Human Mind Occur in the Physical Universe? New York, NY: Oxford University Press.
Anderson, J. R., Bothell, D., Byrne, M. D., Douglass, S., Lebiere, C., and Qin, Y. (2004). An integrated theory of mind. Psychol. Rev. 111, 1036–1060. doi:10.1037/0033-295X.111.4.1036
Arslan, B., Taatgen, N. A., and Verbrugge, R. (2017). Five-year-olds’ systematic errors in second-order false belief tasks are due to first-order theory of mind strategy selection: a computational modeling study. Front. Psychol. 8:275. doi:10.3389/fpsyg.2017.00275
AuBuchon, A. M., Pisoni, D. B., and Kronenberger, W. G. (2015). Short-term and working memory impairments in early-implanted, long-term cochlear implant users are independent of audibility and speech production. Ear Hear. 36, 733–737. doi:10.1097/AUD.0000000000000189
Baauw, S. (2002). Grammatical Features and the Acquisition of Reference. A Comparative Study of Dutch and Spanish. New York: Routledge.
Baayen, R. H., Dijkstra, T., and Schreuder, R. (1997). Singulars and plurals in Dutch: evidence for a parallel dual-route model. J. Mem. Lang. 37, 94–117. doi:10.1006/jmla.1997.2509
Barendse, E. M., Hendriks, M. P., Jansen, J. F., Backes, W. H., Hofman, P. A., Thoonen, G., et al. (2013). Working memory deficits in high-functioning adolescents with autism spectrum disorders: neuropsychological and neuroimaging correlates. J. Neurodev. Disord. 5, 14. doi:10.1186/1866-1955-5-14
Belavkin, R. V. (2001). “Modelling the inverted-U effect in ACT-R,” in Proceedings of the 2001 Fourth International Conference on Cognitive Modelling, eds E. M. Altmann, A. Cleeremans, C. D. Schunn, and W. D. Gray (Mahwah, NJ, London: Lawrence Erlbaum), 275–276.
Belavkin, R. V., Ritter, F. E., and Elliman, D. G. (1999). “Towards including simple emotions in a cognitive architecture in order to fit children’s behaviour better,” in Proceedings of the 1999 Conference of the Cognitive Science Society (CogSci) (Mahwah, NJ: Erlbaum).
Belletti, A., and Guasti, M. T. (2015). The Acquisition of Italian: Morphosyntax and Its Interfaces in Different Modes of Acquisition. Amsterdam, PA: John Benjamins Publishing Company.
Bock, J. K. (1986). Syntactic persistence in language production. Cogn. Psychol. 18, 355–387. doi:10.1016/0010-0285(86)90004-6
Bock, J. K., Dell, G. S., Chang, F., and Onishi, K. H. (2007). Persistent structural priming from language comprehension to language production. Cognition 104, 437–458. doi:10.1016/j.cognition.2006.07.003
Bock, J. K., and Griffin, Z. (2000). The persistence of structural priming: transient activation or implicit learning? J. Exp. Psychol. Gen. 129, 177–192. doi:10.1037/0096-3445.129.2.177
Bock, J. K., and Kroch, A. S. (1989). “The isolability of syntactic processing,” in Linguistic Structure in Language Processing, eds G. N. Carlson, and M. K. Tanenhaus (Dordrecht, The Netherlands: Springer), 157–196.
Borst, J. P., Taatgen, N. A., and Van Rijn, H. (2010). The problem state: a cognitive bottleneck in multitasking. J. Exp. Psychol. Learn. Mem. Cogn. 36, 363–382. doi:10.1037/a0018106
Branigan, H. P., Pickering, M. J., and Cleland, A. A. (1999). Syntactic priming in language production: evidence for rapid decay. Psychon. Bull. Rev. 6, 635–640. doi:10.3758/BF03212972
Branigan, H. P., Pickering, M. J., Stewart, A. J., and McLean, J. F. (2000). Syntactic priming in spoken production: linguistic and temporal interference. Mem. Cognit. 28, 1297–1302. doi:10.3758/BF03211830
Brasoveanu, A., and Dotlačil, J. (2015). Incremental and predictive interpretation: experimental evidence and possible accounts. Proc. SALT 25, 57–81. doi:10.3765/salt.v25i0.3047
Bresnan, J. (2001). “The emergence of the unmarked pronoun,” in Optimality-Theoretic Syntax, eds G. Legendre, J. Grimshaw, and S. Vikner (Cambridge, MA: The MIT Press), 113–142.
Budiu, R., and Anderson, J. R. (2002). Comprehending anaphoric metaphors. Mem. Cognit. 30, 158–165. doi:10.3758/BF03195275
Bybee, J., and Beckner, C. (2009). “Usage-based theory,” in The Oxford Handbook of Linguistic Analysis, eds B. Heine, and H. Narrog (Oxford: Oxford University Press), 827–855.
Chang, F., Bock, J. K., and Goldberg, A. E. (2003). Can thematic roles leave traces of their places? Cognition 99, 29–49. doi:10.1016/S0010-0277(03)00123-9
Chang, F., Dell, G. S., and Bock, K. (2006). Becoming syntactic. Psychol. Rev. 113, 234–272. doi:10.1037/0033-295X.113.2.234
Chesi, C. (2015). On directionality of phrase structure building. J. Psycholinguist. Res. 44, 65–89. doi:10.1007/s10936-014-9330-6
Chien, Y.-C., and Wexler, K. (1990). Children’s knowledge of locality conditions in binding as evidence for the modularity of syntax and pragmatics. Lang. Acquis. 1, 225–295. doi:10.1207/s15327817la0103_2
Chomsky, N. (1970). “Remarks on nominalization,” in Reading in English Transformational Grammar, eds R. Jacobs, and P. Rosenbaum (Waltham, MA: Ginn), 184–221.
Chomsky, N. (1980). Rules and representations. Behav. Brain Sci. 3, 1–15. doi:10.1017/S0140525X00001515
Chomsky, N. (1981). Lectures on Government and Binding: The Pisa Lectures. Dordrecht, The Netherlands: Foris Publications.
Chomsky, N. (1993). “A minimalist program for linguistic theory,” in Tile View from Building 20: Essays in Linguistics in Honor of Sylvain Bromberger, eds K. Hale, and S. J. Keyser (Cambridge, MA: MIT Press), 1–52.
Christiansen, M., and Chater, N. (2016). The now-or-never bottleneck: a fundamental constraint on language. Brain Behav. Sci. 39, e62. doi:10.1017/S0140525X1500031X
Cleland, A. A., and Pickering, M. J. (2003). The use of lexical and syntactic information in language production: evidence from the priming of noun-phrase structure. J. Mem. Lang. 49, 214–230. doi:10.1016/S0749-596X(03)00060-3
Corley, M., and Scheepers, C. (2002). Syntactic priming in English sentence production: categorical and latency evidence from an internet-based study. Psychon. Bull. Rev. 9, 126–131. doi:10.3758/BF03196267
Daily, L. Z., Lovett, M. C., and Reder, L. M. (2001). Modeling individual differences in working memory performance: a source activation account. Cogn. Sci. 25, 315–353. doi:10.1016/S0364-0213(01)00039-8
De Villiers, J., Cahillane, J., and Altreuter, E. (2006). “What can production reveal about principle B?” in Proceedings of the Inaugural Conference on Generative Approaches to Language Acquisition – North America, Vol. 1, eds K. Deen, J. Nomura, B. Schulz, and B. Schwartz (Honolulu, HI: University of Connecticut), 89–100.
Deese, J., and Kaufman, R. A. (1957). Serial effects in recall of unorganized and sequentially organized verbal material. J. Exp. Psychol. 54, 180–187. doi:10.1037/h0040536
Engelmann, F., Vasishth, S., Engbert, R., and Kliegl, R. (2013). A framework for modeling the interaction of syntactic processing and eye movement control. Top. Cogn. Sci. 5, 452–474. doi:10.1111/tops.12026
Fedorenko, E., Woodbury, R., and Gibson, E. (2013). Direct evidence of memory retrieval as a source of difficulty in non-local dependencies in language. Cogn. Sci. 37, 378–394. doi:10.1111/cogs.12021
Ferreira, V. S. (2003). The persistence of optional complementizer production: why saying “that” is not saying “that” at all. J. Mem. Lang. 48, 379–398. doi:10.1016/s0749-596x(02)00523-5
Fillmore, C. J., Kay, P., and O’Connor, M. C. (1988). Regularity and idiomaticity in grammatical constructions: the case of let alone. Language 64, 501–538. doi:10.2307/414531
Frazier, L., and Fodor, J. D. (1978). The sausage machine: a new two-stage parsing model. Cognition 6, 291–325. doi:10.1016/0010-0277(78)90002-1
Fromkin, V. A. (2000). Linguistics: An Introduction to Linguistic Theory. Hoboken, NJ: Wiley-Blackwell Publishing.
Gibson, E. (2000). “The dependency locality theory: a distance-based theory of linguistic complexity,” in Image, Language, Brain, eds A. Marantz, Y. Miyashita, and W. O’Neil (Cambridge, MA: MIT Press), 95–126.
Grivol, M. A., and Hage, S. R. d. V. (2011). Phonological working memory: a comparative study between different age groups. J. Soc. Bras. Fonoaudiol. 23, 245–251. doi:10.1590/S2179-64912011000300010
Grodner, D., and Gibson, E. (2005). Consequences of the serial nature of linguistic input for sentenial complexity. Cogn. Sci. 29, 261–290. doi:10.1207/s15516709cog0000_7
Grosz, B. J., Weinstein, S., and Joshi, A. K. (1995). Centering: a framework for modeling the local coherence of discourse. Comput. Linguist. 21, 203–225.
Hale, J. T. (2011). What a rational parser would do. Cogn. Sci. 35, 399–443. doi:10.1111/j.1551-6709.2010.01145.x
Hartsuiker, R. J., Bernolet, S., Schoonbaert, S., Speybroeck, S., and Vanderelst, D. (2008). Syntactic priming persists while the lexical boost decays: evidence from written and spoken dialogue. J. Mem. Lang. 58, 214–238. doi:10.1016/j.jml.2007.07.003
Hartsuiker, R. J., and Kolk, H. H. J. (1998). Syntactic persistence in Dutch. Lang. Speech 41, 143–184. doi:10.1177/002383099804100202
Hartsuiker, R. J., and Westenberg, C. (2000). Word order priming in written and spoken sentence production. Cognition 75B, 27–39. doi:10.1016/S0010-0277(99)00080-3
Hendriks, P. (2014). Asymmetries between Language Production and Comprehension. Dordrecht, The Netherlands: Springer.
Hendriks, P., and Spenader, J. (2006). When production precedes comprehension: an optimization approach to the acquisition of pronouns. Lang. Acquis. 13, 319–348. doi:10.1207/s15327817la1304_3
Hendriks, P., Van Rijn, H., and Valkenier, B. (2007). Learning to reason about speakers’ alternatives in sentence comprehension: a computational account. Lingua 117, 1879–1896. doi:10.1016/j.lingua.2006.11.008
Huettig, F., and Janse, E. (2016). Individual differences in working memory and processing speed predict anticipatory spoken language processing in the visual world. Lang. Cogn. Neurosci. 31, 80–93. doi:10.1080/23273798.2015.1047459
Huttenlocher, J., Vasilyeva, M., and Shimpi, P. (2004). Syntactic priming in young children. J. Mem. Lang. 50, 182–195. doi:10.1016/j.jml.2003.09.003
Jaeger, T. F., and Snider, N. E. (2008). “Implicit learning and syntactic persistence: surprisal and cumulativity,” in Proceedings of the 30th Annual Conference of the Cognitive Science Society (CogSci) (Washington, DC: Cognitive Science Society), 1061–1066.
Jaeger, T. F., and Snider, N. E. (2013). Alignment as a consequence of expectation adaptation: syntactic priming is affected by the prime’s prediction error given both prior and recent experience. Cognition 127, 57–83. doi:10.1016/j.cognition.2012.10.013
Jäger, L. A., Engelmann, F., and Vasishth, S. (2015). Retrieval interference in reflexive processing: experimental evidence from Mandarin, and computational modeling. Front. Psychol. 6:617. doi:10.3389/fpsyg.2015.00617
Joshi, A. K., Levy, L. S., and Takahashi, M. (1975). Tree adjunct grammars. J. Comput. Syst. Sci. 10, 136–163. doi:10.1016/S0022-0000(75)80019-5
Kaschak, M. P., Kutta, T. J., and Jones, J. L. (2011a). Structural priming as implicit learning: cumulative priming effects and individual differences. Psychon. Bull. Rev. 18, 1133–1139. doi:10.3758/s13423-011-0157-y
Kaschak, M. P., Kutta, T. J., and Schatschneider, C. (2011b). Long-term cumulative structural priming persists for (at least) one week. Mem. Cognit. 39, 381–388. doi:10.3758/s13421-010-0042-3
Kempen, G., and Hoenkamp, E. (1987). An incremental procedural grammar for sentence formulation. Cogn. Sci. 11, 201–258. doi:10.1207/s15516709cog1102_5
Kieras, D. E., and Meyer, D. E. (1997). An overview of the EPIC architecture for cognition and performance with application to human-computer interaction. Hum. Comput. Interact. 12, 391–438. doi:10.1207/s15327051hci1204_4
Kimball, J. (1973). Seven principles of surface structure parsing in natural language. Cognition 2, 15–47. doi:10.1016/0010-0277(72)90028-5
King, J., and Just, M. A. (1991). Individual differences in syntactic processing: the role of working memory. J. Mem. Lang. 30, 580–602. doi:10.1016/0749-596X(91)90027-H
Kong, F., Zhou, G., and Zhu, Q. (2009). “Employing the centering theory in pronoun resolution from the semantic perspective,” in Proceedings of the 2009 Conference on Empirical Methods in Natural Language Processing (Singapore: Association for Computational Linguistics), 987–996.
Kootstra, G. J., Van Hell, J. G., and Dijkstra, T. (2012). Priming of code-switches in sentences: the role of lexical repetition, cognates, and language proficiency. Bilingualism Lang. Cogn. 15, 797–819. doi:10.1017/S136672891100068X
Kuijper, S. J. M. (2016). Communication Abilities of Children with ASD and ADHD. Doctoral dissertation, University of Groningen, Groningen, The Netherlands.
Kuijper, S. J. M., Hartman, C. A., and Hendriks, P. (2015). Who is he? Children with ASD and ADHD take the listener into account in their production of ambiguous pronouns. PLoS ONE 10:e0132408. doi:10.1371/journal.pone.0132408
Lappin, S., and Leass, H. J. (1994). An algorithm for pronominal anaphora resolution. Comput. Linguist. 20, 535–561.
Lewis, R. L. (1996). Interference in short-term memory: the magical number two (or three) in sentence processing. J. Psycholinguist. Res. 25, 93–115. doi:10.1007/BF01708421
Lewis, R. L., and Vasishth, S. (2005). An activation-based model of sentence processing as skilled memory retrieval. Cogn. Sci. 29, 375–419. doi:10.1207/s15516709cog0000_25
Lewis, R. L., Vasishth, S., and Van Dyke, J. A. (2006). Computational principles of working memory in sentence comprehension. Trends Cogn. Sci. 10, 447–454. doi:10.1016/j.tics.2006.08.007
Malhotra, G. (2009). Dynamics of Structural Priming. Doctoral dissertation, University of Edinburgh, Edinburgh.
Martinussen, R., Hayden, J., Hogg-Johnson, S., and Tannock, R. (2005). A meta-analysis of working memory impairments in children with attention-deficit/hyperactivity disorder. J. Am. Acad. Child Adolesc. Psychiatry 44, 377–384. doi:10.1097/01.chi.0000153228.72591.73
McKee, C. (1992). A comparison of pronouns and anaphors in Italian and English acquisition. Lang. Acquis. 2, 21–54. doi:10.1207/s15327817la0201_2
Osborne, T., Putnam, M., and Gross, T. M. (2011). Bare phrase structure, label-less trees, and specifier-less syntax. Is minimalism becoming a dependency grammar? Linguist. Rev. 28, 315–364. doi:10.1515/tlir.2011.009
Pauls, F., Macha, T., and Petermann, F. (2013). U-shaped development: an old but unsolved problem. Front. Psychol. 4:301. doi:10.3389/fpsyg.2013.00301
Philip, W., and Coopmans, P. (1996). “The role of lexical feature acquisition in the development of pronominal anaphora,” in Amsterdam Series on Child Language Development, Vol. 5, eds W. Philip, and F. Wijnen (Amsterdam, The Netherlands: University of Amsterdam), 73–106.
Pickering, M. J., and Branigan, H. P. (1998). The representation of verbs: evidence from syntactic priming in language production. J. Mem. Lang. 39, 633–651. doi:10.1006/jmla.1998.2592
Pickering, M. J., and Ferreira, V. S. (2008). Structural priming: a critical review. Psychol. Bull. 134, 427–459. doi:10.1037/0033-2909.134.3.427
Pollard, C., and Sag, I. A. (1994). Head-Driven Phrase Structure Grammar. Chicago, IL: University of Chicago Press.
Prince, A., and Smolensky, P. (1993). Optimality Theory: Constraint Interaction in Generative Grammar. Technical Report CU-CS-696-93, Department of Computer Science, University of Colorado at Boulder, and Technical Report TR-2, Rutgers Center for Cognitive Science, Rutgers University, New Brunswick, NJ. Published by Blackwell in 2004.
Reitter, D. (2008). Context Effects in Language Production: Models of Syntactic Priming in Dialogue Corpora. Doctoral dissertation, University of Edinburgh, Edinburgh.
Reitter, D., Keller, F., and Moore, J. D. (2011). A computational cognitive model of syntactic priming. Cogn. Sci. 35, 587–637. doi:10.1111/j.1551-6709.2010.01165.x
Reitter, D., and Lebiere, C. (2010). “Accountable modeling in ACT-UP, a scalable, rapid-prototyping ACT-R implementation,” in Proceedings of the 10th International Conference on Cognitive Modeling (ICCM) (Philadelphia, PA), 199–204.
Reitter, D., and Moore, J. D. (2014). Alignment and task success in spoken dialogue. J. Mem. Lang. 76, 29–46. doi:10.1016/j.jml.2014.05.008
Reitter, D., Moore, J. D., and Keller, F. (2006). “Priming of syntactic rules in task-oriented dialogue and spontaneous conversation,” in Proceedings of the 28th Annual Conference of the Cognitive Science Society (CogSci) (Vancouver, Canada: Cognitive Science Society), 685–690.
Roberts, S., and Pashler, H. (2000). How persuasive is a good fit? A comment on theory testing. Psychol. Rev. 107, 358–367. doi:10.1037/0033-295X.107.2.358
Roelofs, A. (1992). A spreading-activation theory of lemma retrieval in speaking. Cognition 42, 107–142. doi:10.1016/0010-0277(92)90041-F
Salvucci, D. D., and Taatgen, N. A. (2008). Threaded cognition: an integrated theory of concurrent multitasking. Psychol. Rev. 115, 101–130. doi:10.1037/0033-295X.115.1.101
Scheepers, C. (2003). Syntactic priming of relative clause attachments: persistence of structural configuration in sentence production. Cognition 89, 179–205. doi:10.1016/S0010-0277(03)00119-7
Scheepers, C., Raffray, C. N., and Myachykov, A. (2017). The lexical boost effect is not diagnostic of lexically-specific syntactic representations. J. Mem. Lang. 95, 102–115. doi:10.1016/j.jml.2017.03.001
Schoonbaert, S., Hartsuiker, R. J., and Pickering, M. J. (2007). The representation of lexical and syntactic information in bilinguals: evidence from syntactic priming. J. Mem. Lang. 56, 153–171. doi:10.1016/j.jml.2006.10.002
Sense, F., Behrens, F., Meijer, R. R., and Van Rijn, H. (2016). An individual’s rate of forgetting is stable over time but differs across materials. Top. Cogn. Sci. 8, 305–321. doi:10.1111/tops.12183
Snider, N. E. (2008). An Exemplar Model of Syntactic Priming. Doctoral dissertation, Stanford University, Stanford.
Spenader, J., Smits, E.-J., and Hendriks, P. (2009). Coherent discourse solves the pronoun interpretation problem. J. Child Lang. 36, 23–52. doi:10.1017/S0305000908008854
Steedman, M. (1988). “Combinators and grammars,” in Categorial Grammars and Natural Language Structures, eds R. T. Oehrle, E. Bach, and D. Wheeler (Dordrecht, The Netherlands: Springer), 417–442.
Stewart, T., Tripp, B., and Eliasmith, C. (2009). Python scripting in the Nengo simulator. Front. Neuroinformatics 3:7. doi:10.3389/neuro.11.007.2009
Stocco, A., and Crescentini, C. (2005). Syntactic comprehension in agrammatism: a computational model. Brain Lang. 95, 127–128. doi:10.1016/j.bandl.2005.07.069
Taatgen, N., and Anderson, J. R. (2002). Why do children learn to say “broke”? A model of learning the past tense without feedback. Cognition 86, 123–155. doi:10.1016/S0010-0277(02)00176-2
Tanenhaus, M. K., Spivey-Knowlton, M. J., Eberhard, K. M., and Sedivy, J. C. (1995). Integration of visual and linguistic information in spoken language comprehension. Science 268, 1632–1634. doi:10.1126/science.7777863
Van Beijsterveldt, L. M., and Van Hell, J. G. (2009). Structural priming of adjective-noun structures in hearing and deaf children. J. Exp. Child Psychol. 104, 179–196. doi:10.1016/j.jecp.2009.05.002
Van Maanen, L., and Van Rijn, H. (2010). The locus of the Gratton effect in picture-word interference. Top. Cogn. Sci. 2, 168–180. doi:10.1111/j.1756-8765.2009.01069.x
Van Rij, J. (2012). Pronoun Processing: Computational, Behavioral, and Psychophysiological Studies in Children and Adults. Doctoral dissertation, University of Groningen, Groningen, The Netherlands.
Van Rij, J., Van Rijn, H., and Hendriks, P. (2010). Cognitive architectures and language acquisition: a case study in pronoun comprehension. J. Child Lang. 37, 731–766. doi:10.1017/S0305000909990560
Van Rij, J., Van Rijn, H., and Hendriks, P. (2013). How WM load influences linguistic processing in adults: a computational model of pronoun interpretation in discourse. Top. Cogn. Sci. 5, 564–580. doi:10.1111/tops.12029
Keywords: language processing, sentence processing, linguistic theory, cognitive modeling, Adaptive Control of Thought—Rational, cognitive resources, computational simulations
Citation: Vogelzang M, Mills AC, Reitter D, Van Rij J, Hendriks P and Van Rijn H (2017) Toward Cognitively Constrained Models of Language Processing: A Review. Front. Commun. 2:11. doi: 10.3389/fcomm.2017.00011
Received: 03 April 2017; Accepted: 23 August 2017;
Published: 08 September 2017
Edited by:
Ángel J. Gallego, Universitat Autònoma de Barcelona, SpainReviewed by:
Mireille Besson, Cognitive Neuroscience Laboratory, CNRS and Aix-Marseille University, FranceCristiano Chesi, Istituto Universitario di Studi Superiori di Pavia (IUSS), Italy
Copyright: © 2017 Vogelzang, Mills, Reitter, Van Rij, Hendriks and Van Rijn. This is an open-access article distributed under the terms of the Creative Commons Attribution License (CC BY). The use, distribution or reproduction in other forums is permitted, provided the original author(s) or licensor are credited and that the original publication in this journal is cited, in accordance with accepted academic practice. No use, distribution or reproduction is permitted which does not comply with these terms.
*Correspondence: Margreet Vogelzang, bWFyZ3JlZXR2b2dlbHphbmdAZ21haWwuY29t