- 1Department of Psychology, The University at Albany, SUNY, Albany, NY, United States
- 2Haskins Laboratories, New Haven, CT, United States
- 3Department of Communication, University of California, Los Angeles, Los Angeles, CA, United States
- 4Department of Artificial Intelligence, University of Groningen, Groningen, Netherlands
We explore how timing in identical keystroke sequences that form a stem morpheme are influenced by linguistic knowledge by manipulating lexical status and morphological complexity of words in a type-to-copy task. Starting from the second keystroke, we find that average keystroke latency within a stem morpheme varies according to whole-word frequency (Experiment 1) and lexicality defined by compatibility of the upcoming suffix (e.g., IZE vs IST) with the stem (e.g., NORMAL) that forms the target string (e.g., RENORMALIZE vs. RENORMAL; RENORMALIZE vs. RENORMALIST in Experiments 2 and 3, respectively). Further, although lexical and frequency effects persist over the string as a whole, non-linear mixed-effects regressions reveal position varying lexical effects on keystroke latencies within the stem morpheme. In addition, whole word frequency effects on the first keystroke were present. These results challenge hierarchical accounts of production with modular motor programs where the same letter sequence (for a morpheme) is realized independently of and only after lexical access to the full word in which the letters occur (cf. Crump and Logan, 2010a; Logan and Crump, 2011).
Introduction
In most accounts of language processing it is assumed that access to one's knowledge about a word is stored in a mental lexicon and that lexical access to activate the requisite linguistic knowledge is necessary to execute behavior relevant to particular experimental tasks. In this framework, the mental lexicon is treated as a repository of lexical knowledge and access based on orthographic or phonological form generally is conceptualized as all or none. Nonetheless, time to access knowledge about a particular word will vary across words due to its frequency and this effect of whole word frequency gets interpreted as a reflection of the layout or organization of word representations in the lexicon. In essence, recognition of a word is conceptualized as a search through the repository whose duration depends on the manner in which it is organized and lexical retrieval is treated as all or none.
In more dynamic lexical frameworks word meaning is not stored and accessed from a form (e.g., Elman, 2004, 2009; Jones and Mewhort, 2007; Milin et al., 2017, 2018). This general approach does not conceptualize a word as an independent representation within a mental store. Instead, the knowledge that underlies productive and receptive language use reflects the typicality and distinctiveness of the meaning and form-based properties of a word with respect to context (e.g., other words). This includes both those words that are physically present and those that are not. The emphasis is more on how we learn and use language rather than on the content of localized representations for individual words (cf. Christiansen and Chater, 2016). The implication is that in a dynamic framework, the processing of a word (or morpheme or other linguistic unit) is not governed by time to execute one isolated event such as retrieving its entry in the mental lexicon because word units are not typically processed independently from one another and from other “levels” of structure (Spivey et al., 2005; Spivey and Dale, 2006; Spivey, 2008). In essence, interdependent orthographic, phonological, lexical and semantic properties that emerge over time are essential, and form the basis for a more dynamic lexical framework that involves extensive interactivity (cf. Seidenberg and McClelland, 1989).
Words are processed more quickly and more accurately than non/pseudowords, and higher frequency words are processed more quickly and more accurately than lower frequency words in a variety of experimental tasks. Interpretations of these properties nicely capture the two differing perspectives on how we use what we know about words. In the lexical repository framework, word status depends on attesting its presence in a mental lexicon and any effect of frequency reflects ease of lexical access, often conceptualized as the work of a counting mechanism that keeps track of number of prior exposures and organizes the lexical entries or the activation thresholds for particular entries according to frequency (Baus et al., 2013). Interpretations of the effect of neighborhood density—meaning number of words that differ by a single letter or phoneme from the target word—diverge from the structural interpretation for lexical status as a word and of frequency. Instead, the effect of neighborhood density reflects convergent patterns of activation and sometimes competition based on similarity with many other entries within the lexicon.
Baayen et al. (2016) have delineated how word frequency effects are, in fact, much more nuanced and not straightforwardly characterized in terms of all or none lexical access. For starters whole-word frequency values vary depending on the type of corpus on which frequencies were counted. These days some are web-based such as the Corpus of Contemporary American English (COCA, Davies, 2008), Facebook (Herdagdelen and Marelli, 2017) or Google (Brants and Franz, 2006). Others are based on subtitle frequency (Brysbaert and New, 2009). Frequency measures from different corpora tend to be correlated with each other although there are systematic differences that reflect modality (written, spoken) as well as register (formal, spontaneous). More importantly, whole word frequency measures tend to be correlated with other measures that describe letter strings. But these correlations are not restricted to real words. Letter length, orthographic neighborhood density, and more semantic measures such as emotional valence and arousal and semantic diversity and dispersion can often correlate with processing of properties of non-word sequences as well (Baayen et al., 2006). More interesting is that the correlation between various corpus-based measures of frequency and other purportedly less structural word measures tends to vary across corpora (Baayen et al., 2016). For example, an effect on processing of valence based on a subtitle corpus is stronger than from a corpus based on conversation while an effect of multiple senses or meanings is better predicted from a subtitle than in the spoken BNC corpus1.
Most challenging for the repository account of frequency is that discrepancies with respect to frequency across corpora are not uniform across all words. Frequency estimates for high frequency words are less subject to distortions based on pockets of high usage or burstiness than are lower frequency words. In fact, some have argued that once burstiness and the concomitant contextual diversity are taken into account, the contribution of word frequency as a predictor in simple processing tasks is severely attenuated (Adelman et al., 2006, 2008).
Repository and dynamic lexical accounts of whole-word frequency invite different predictions about the role of frequency in a type-to-copy production task where target words are visually presented. Consistent with the repository account, it has been asserted that control processes in a type-to-copy production task are organized hierarchically with multiple encapsulated levels such that production constraints at one level may be impervious to constraints at another. Logan and Crump (2011) model control process for (production by) typing in terms of an outer and inner loop that are hierarchically nested. Accordingly, retrieval or selection of a particular word occurs in the outer loop while the inner loop initiates the letter and keystroke sequence for each word designated by the outer loop. Attention to an available visual template to copy is reported to be more important than visual or kinesthetic feedback in this typing task regardless of whether one types with all ten fingers or with a more limited set because the organization of keystrokes is an inner loop task and the value of a template is to the outer loop (Rieger and Bart, 2016).
Based on the usefulness of kinesthetic feedback to the inner loop and visual feedback to the outer loop in this model, Logan and Crump (2011) claim that the outer loop passes along lexical knowledge about the motor program to the inner loop but does not know about keystroke sequencing in the inner loop. In their hierarchical and sequential framework, any lexical effects on the inner loop should be constant across keystroke positions because component keystrokes are activated in parallel once a word is retrieved from the lexicon (Crump and Logan, 2010a,b; Logan and Crump, 2010). Note that after typing a word, latencies to retype a probed position have been interpreted to suggest that the activation that underlies the benefit of repetition is graded across positions and stronger earlier in a word (Logan et al., 2016). However, reaction times to type the probe letter also were faster for first position and decreased at later positions so this finding in isolation is more difficult to interpret as consistent with parallel activation without sequential execution of constituent letters (see their Table 4 and Figure 8). Accordingly, it may be more cautious to retain the option of a systematic reduction in keystroke latencies as one progresses through the word (Rumelhart and Norman, 1982).
Manipulations of lexicality based on the legal or illegal combination of real morphemes permit one to explore where lexical and frequency effects arise in the course of producing a word in a type to copy task. Similarly, manipulations of the ratio of words to pseudowords in an experiment may affect the degree to which keystroke latencies decrease in word final positions. Novel in our typing study is that differences in latency between finger movements to particular keys are controlled for by comparing words and pseudowords that share a morpheme and thus a letter sequence. As a result, the influence on keystroke latency of the distance a finger must move to the key (Fitts, 1954) as well as the decision of which fingers to use (Hick, 1952; Hyman, 1953) are weakened if not fully eliminated. An account of production that entails retrieving from the lexical repository a typing motor program and control for its execution in a loop that is immune to lexical influences would need to assert that the relationship between keystrokes (e.g., [N]ORMAL) should be stable across words that contain that letter sequence or morpheme. In many analyses the first letter [N] is not included because the initial keystroke of a word tends to be disproportionally longer than the others. We follow that practice here and indicate it by the bracket notation [N].
Essential in this framework is that strings may differ with respect to time for lexical retrieval but, because keystroke execution in the inner loop occurs automatically, the relative timing for the same sequence of letters such as [N]ORMAL within strings such as RENORMALIZE vs. *RENORMALIST should not vary with lexicality (Shaffer, 1975; Gentner et al., 1988). One outcome that is more compatible with a dynamic than with a repository framework is that predictability in various types of linguistic contexts interacts with keystroke dynamics (plausibly in complex ways) so as to influence the manner in which a word is produced or recognized. In essence the dynamic account, but not the retrieval account, would not only be compatible with but would anticipate non-linear changes in position by keystroke latencies with manipulations of lexicality or whole-word frequency.
Online typing tasks with dependent measures based on the execution of keystrokes within a morpheme have been useful to track the interdependence of morpheme and word structure in production (Gagné and Spalding, 2014; Feldman et al., 2017). In the present study, we attenuate the role of retrieving or selecting the target word by presenting the target word visually and then ask whether whole word frequency and other lexical effects are restricted to the outer loop or whether inner loop measures associated with execution of constituent letters are sensitive to linguistic factors as well.
We track three basic measures of typing performance. All are sensitive to which finger moves and to what key. The simplest and best investigated is latency to key contact for the initial keystroke in a word (K1). This measure is assumed by Logan and Crump (2011) to reflect response preparation and initiation and it is known to be sensitive to whole-word frequency (West and Sabban, 1982; Inhoff, 1991; Pinet et al., 2016) and also to word length (Gagné and Spalding, 2014). Second is average latency between keystrokes for letters within a letter string or inter keystroke interval (IKSI). This measure is interpreted to reflect execution of the motor plan and is sensitive to bigram frequency (Pinet et al., 2016) and again to word length (Gagné and Spalding, 2014, 2016). Finally, it is possible to examine average keystroke latency by position within a stem morpheme or word. As a rule when typing text, the timing between keystrokes (IKSI) is faster at the end of a word and faster for a higher frequency letter or letter sequence (e.g., bigram, trigram) than for a less frequent letter sequence (Gagné and Spalding, 2016; Pinet et al., 2016).
Overall, IKSIs covary with multiple measures of predictability defined within as well as across words (van Rij et al., 2019a). For example, even the word “the,” perhaps the most typed word in all of English, can be sped up or slowed down slightly depending on how predictable it is in context (van Rij et al., 2019a). The implication is that one should account for differences in keystroke latencies by letter and bigram frequency before attempting to examine their interaction with various word properties including frequency or lexicality. At issue is whether position by keystroke latencies for identical constituent letters vary systematically according to lexical properties (letter length, orthographic neighborhood density, whole word frequency) of the words or stems in which they appear. The study we report utilizes effects on processing of a stem morpheme that varies systematically according to the other morphemes with which it appears. What we find does not support a characterization of morphological processing that assumes decomposition, or a characterization that emphasizes processing of a stem in isolation from the other constituents with which it typically appears. Effects of lexicality that arise from an incompatible affix positioned after the stem such as RENORMALIST are potentially informative in this regard.
We report the results of three experiments that use non-linear mixed-effects regressions to compare measures based on variation of keystroke timing. Comparisons focus on the same stem morpheme (e.g., NORMAL) in a variety of morphological contexts in an online typing-to-copy task. The key comparison in Experiment 1 is stem keystroke latencies between words that differ in whole word frequency such as NORMALLY and NORMALCY. In Experiment 2 the critical comparison is between strings that differ in affixation and resultant lexical status such as *RENORMAL which is not a word and RENORMALIZE which is and in Experiment 3 it is prefixed and suffixed strings that differ in lexical status such as *RENORMALIST and RENORMALIZE. Regardless of any preoccupation with morphological decomposition, morphological knowledge in production is particularly interesting to examine in its own right as it can provide a framework that highlights interactions of lexical effects on typing speed across the keystroke positions within a letter string.
In fact, the term lexical status is deceptive because letter strings can vary in their degree of wordiness. As consideration of *RENORMAL and *RENORMALIST demonstrate, a letter string need not be in full compliance or in full violation with lexical knowledge about word formation. Patterning can be graded. Readers know what these particular combinations of morphemes would mean, even though neither is an attested word in the language. Because we compare conditions where stems repeat, comparisons of the same pattern of keystrokes in more or less predictable morphological contexts allow us to answer various questions about the time course over which linguistic knowledge emerges in a production task. For example, differences between keystroke measures in RENORMALIZE vs. RENORMALIST speak to when an upcoming lexical deviation becomes evident on IKSI. Differences between keystroke measures in NORMALIZE vs. RENORMALIZE, words that differ in whole word frequency because of a prefix show how long the effect of a prefix and the concomitant reduction in whole word frequency persist on IKSI.
In summary, our focus on morphemes in production provides a method to control for differences in keystroke latencies by letter and bigram and permits an examination of the interaction of lexicality and various word properties like whole-word frequency and word length on morphological processing. Our focus on keystroke latency measures shows that these measures vary across types of morphological combinations in a type-to-copy production task. The results do not have strong compatibility with an account based on search and all-or-none access to a lexical repository. For example, work based on time to initiate first keystroke or average keystroke latency (less the initial keystroke) are fully compatible with models that assume lexical access and retrieval of a motor program before initiation of a motor response. In contrast, keystroke-to-keystroke latencies that vary across positions of a letter sequence within a word raise the possibility of a more dynamic option as when the timing variation in the execution of keystroke movements for a stem vary systematically with the lexical or morphological properties of the string as a whole.
These keystroke sequence latencies across positions within a word pose a challenge to the notion that response preparation based on lexical access and retrieval of a motor program is completed in its entirety before the initiation of keystroke movements. Similar claims for ongoing (re) assessment of lexicality have been made in the domain of comprehension when the lexical determination for a morphologically simple letter string is indicated by the velocity profile of mouse movement (Barca and Pezzulo, 2012, 2015).
Of particular relevance in the type-to-copy task is whether variation in IKSIs as one progresses through a morpheme or a word reflect a systematic and continuous updating based on lexical status, predictability, whole word frequency and perhaps other linguistic factors or whether effects remain constant over letter positions because decisions about which keystrokes to activate occur before movement to keystrokes begins. In support of prolonged linguistic influences on typing measures, Gagné and Spalding (2014, 2016) have reported a slowing in IKSI at the boundary between morphemes in a word and this effect is sensitive to the semantic consistency of the critical morpheme to the meaning of the full word in which it appears (Libben et al., 2012, 2014; Gagné and Spalding, 2016). Semantic influences on keystroke differences within a word can vary by position. They generally appear at but may appear earlier than the stem boundary. For the time being, we ask whether differences between conditions that vary according to the combination of morphemes are salient when aligned to the beginning of a morpheme stem and leave analyses aligned to the end for future work. Stem-initial alignment invites a focus on anticipatory influences whereas reductions with stem final alignment could reflect a later wrapping up that maximizes the semantics of the stem morpheme with respect to the suffixes with which it can combine. Both could be semantic in nature but to differing degrees and ultimately are worthy of consideration. Most relevant for the time being is evidence that lexical influences can be revised and updated during the course of producing a word.
Methods
To investigate lexical and frequency effects on keystroke measures, we conducted three on-line typing experiments. All used the same procedure with slightly different materials all of which consisted of triplets formed around a morpheme stem.
Participants
Recruitment and Payment
For each experiment, we aimed to recruit 100 (target N = 100) participants on Amazon's Mechanical Turk (AMT; requester.mturk.com). We report below procedures for discarding any participants and data, so that our N in each experiment was lower than this target. Participants were given the opportunity to participate once in the task for a payment of $1.25.
Participant Demographics
We restricted recruitment to the US, to people with at least 100 prior approved tasks, with an approval rate of 95%. One hundred participants were recruited for each of the three experiments. A small number of payments on AMT were rejected, no more than 2 per dataset. For example, participants who submitted a response on AMT had their work rejected due to lack of an appropriate payment confirmation number. The modal age range was 26–34 (~50% of participants). For highest education level, the two most common responses included 48 with “high-school” and 43 with “bachelors.” All participants reported gender: 62% of participants reported gender as male, 38% female. One hundred percent reported QWERTY keyboards. Eighty one percent were right-handed, and 1 participant reported ambidextrous.
Participant Time and Exclusion
The mode of the time required to complete the task was approximately 5 min. The average was 15 min. Data from participants that appeared not to be complete were excluded. We also excluded participants who did not appear to have typed responses to all trials in the experiment. This left data for 258 participants (85 in Experiment 1, 87 in Experiment 2, and 86 in Experiment 3), each of whom typed 25–58 words correctly.
Procedure
We adapted an Internet typing task that facilitates rapid data collection through AMT (Vinson et al., under revision). This online task uses a JavaScript framework to track IKSIs while participants type a word that is displayed on the screen. The framework records milliseconds associated with each keystroke, and tracks which key was pressed. This offers an easy-to-use type-to-copy task that rapidly crowdsources large amounts of typing data. An additional resource of the interface and raw data, in addition to our supplementary data, can be found online2.
After ~2 s (ISI), participants were presented a word and a textbox. Their cursor was automatically focused on the textbox so they did not have to use the mouse. They then typed words back and hit ENTER to move on. At any point an error was made, the interface reported it to the participants and began the next trial. About 7 practice items preceded the experimental items. The interface is depicted in Figure 1A. Informed consent was incorporated into the instructions for the task. See Figure 1B.
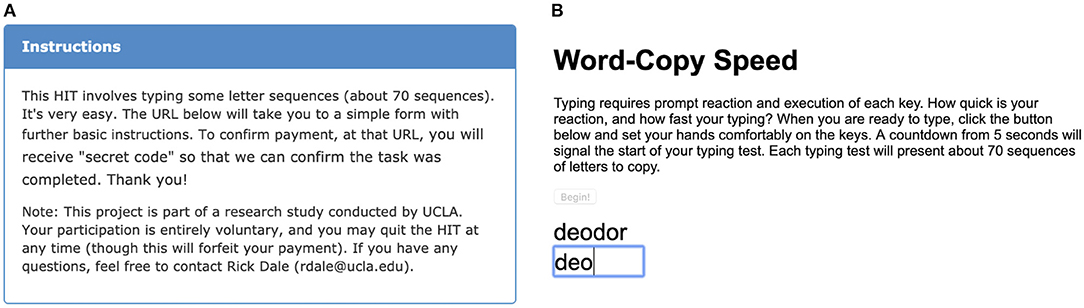
Figure 1. (A) Screen interface on Amazon Mechanical Turk. (B) Informed consent in interface instructions.
Materials
The final set of experimental materials for each experiment included about 60 words. The materials for Experiment 1 consisted of only 57 triplets formed around a shared stem morpheme because three stem triples contained spelling errors and were eliminated from all analyses. The triplet based on the stem “NORMAL” provides an example throughout this report. In Experiment 1, for each triplet, one item was the stem such as NORMAL. The second and third items were a legal stem-suffix combination of that same stem such as NORMAL-LY and NORMAL-CY, with the former occurring with a higher frequency than the latter.
In Experiment 2, for each triplet, one item was a legal stem-suffix combination such as NORMAL-IZE. The second was a legal prefixed version of that same stem plus suffix sequence such as RE-NORMAL-IZE. The third was a nonword formed by combining the stem morpheme of the former with an incompatible prefix such as RE-NORMAL. The *RENORMAL constraint required that we present different materials than in Experiment 1.
In Experiment 3, for each triplet, one item was a legal stem-suffix combination such as NORMAL-IZE. The second was a legal prefixed version of that same stem plus suffix sequence such as RE-NORMAL-IZE. The third was a nonword formed by combining the prefix and the stem morpheme of the former with an incompatible suffix such as RE-NORMAL-IST. Suffixes in the prefixed word and prefixed nonword condition were matched triple by triple for length but not number of syllables with a median of 4 and a range of 2 and 4. Prefixes in those two conditions had a median of 2 and ranged only between 2 and 3. Median suffix length was 4 letters but varied between 2 and 4.
In no case did the stem morpheme undergo a spelling change when affixed as in the derivation of SEVERITY from SEVERE where the final E in SEVERE gets dropped before affixation. Each participant viewed and typed one formation from the stem morpheme. Members of each stem triplet were distributed across three different lists and presented to different participants. Each participant viewed and typed a total 57 unique words. For example across lists, the same stem morpheme NORMAL appeared in different morphological contexts e.g., NORMALIZE, RENORMALIZE, and RENORMALIST.
Table 1 presents the conditions (types of word structures) that were tested in each experiment. Materials are listed in Appendix A.
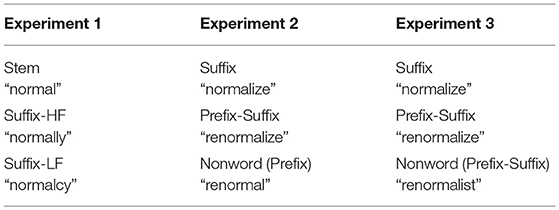
Table 1. Overview of the experimental conditions in the three experiments, with example stimuli derived from the stem “normal.”
Results
To consolidate presentation of the data and facilitate comparisons of a measure across experiments and types of word structures, we present the results of the three experiments in parallel. Materials for Experiments 2 and 3 only differed in whether or not nonwords were suffixed. Thus, the experimental stimuli in Experiments 2 and 3 were almost identical in that they were derived from the same stems. Experiment 2 included three additional stems, however. Experiment 1 only shared four stems with Experiment 2 and 3. We focus on keystroke latencies across positions within a stem that is nested within a letter string because systematic variation poses a challenge to the notion that response preparation based on lexical access and retrieval of a motor program is completed in its entirety before the initiation of keystroke movements.
Preprocessing of Data
The trials were terminated at the end of the presented string or when a typing error was made. Incorrect trials were not included in the analysis (2,298 trials out of 14,712; 15.6%). The overall accuracy varied slightly between the three experiments (86.9, 84.8, and 81.6% respectively, for Experiments 1, 2, and 3) but this variation is likely attributable to variations in word length (average number of characters: 8.3, 8.9, and 9.9 respectively, for Experiments 1, 2, and 3): As a rule, longer words were typed less accurately than shorter words. After excluding all incorrect trials, 70 trials (out of 12,414; 0.56%) were excluded because the first keystroke latency was longer than 6,000 ms, and another 7 trials were excluded because one or more later keystroke latencies were longer than 3,000 ms (0.056%).
Analysis
A range of measures can be used to investigate when lexical and orthographic information would become available during the time course of typing a word. These include the first keystroke latencies, the sum of keystroke latencies for letters in the stem (normalized for length), all keystroke latencies for letters in the stem, and the trajectory meaning the keystroke latencies by letter position within the string. In this paper we will present only the three most important measures, namely the first keystroke latency (K1), which is purported to reflect lexical access (Crump and Logan, 2010b), the keystroke latencies for the stem as a whole less K1, which reflects execution of the motor program to type the stem and is most compatible with the morphological word recognition literature where stem processing is the primary focus, and the trajectory of keystroke latencies by letter position, which has the potential to provide more insight into keystroke by keystroke execution of the motor program to type the stem. To reiterate the logic, systematic differences in the same (series of) keystrokes depending on co-occurring morphemes within a word call into question the claim that all keystrokes are activated in parallel without regard to lexical context.
The typing measures were analyzed with Generalized Additive Mixed Modeling (GAMM; Wood, 2017), a mixed-effects regression approach that allows a non-linear relation between the measure and the covariates (see for introductions Wieling, 2018; van Rij et al., 2019a,b). The data were analyzed in R version 3.4.4 (2018-03-15; (R Core Team, 2018)) using the package mgcv version 1.8-24 (Wood, 2017) for modeling GAMMs and the package itsadug version 2.3 (van Rij et al., 2017) for evaluation and visualization of the results. The data of the three experiments were separately analyzed with similar statistical models, unless stated otherwise. We used an iterative backward-fitting model comparison procedure for determining the best-fitting model, but we also inspected the summary statistics and visualizations of the effects to verify the conclusions (cf. Wieling, 2018; van Rij et al., 2019b). The models were fitted using the maximum likelihood optimization score.
To investigate the effect of lexical frequency, we used the frequencies in the Google Books Corpus (Total word counts for English, version 20120701; 543,081 words and 6,640,052,764 tokens). We excluded the occurrences in books published before 1950 (leaving 541,040 words and 3,345,974,073 tokens). The frequency was converted to frequency per million words, and log-transformed to approach a normal distribution. We additionally calculated a measure of orthographic similarity, OLD50, which is the average Levenshtein distance between a word from the experiment and its 50 nearest neighbors in the Google Books Corpus of books after 1950 (cf. Yarkoni et al., 2008), using the R package vwr version 0.3.0 (Keuleers, 2013). The OLD50 scores were log-transformed.
First Keystroke Latencies
Figure 2 shows average log frequency per condition in each experiment. Figure 3 presents the grand averages of the first keystroke latencies (K1) for the three experiments and the conditions within each experiment. On average, the first keystroke latency is 850 ms. These are the most noticeable differences: in Experiment 1, the low frequency suffix words (“Suffix-LF”) seem to start with a longer first keystroke latency than the other two conditions (855 ms vs. 812 ms Stem/816 ms Suffix-HF); in Experiments 2 and 3 the Prefix-Suffix words (882 and 858 ms, respectively) seem to start with a longer first keystroke latencies than the Suffix words (856 and 830 ms, respectively). Faster K1 latencies for higher as compared to lower frequency words replicate reports in the typing literature (Crump and Logan, 2010a,b; Logan and Crump, 2011).
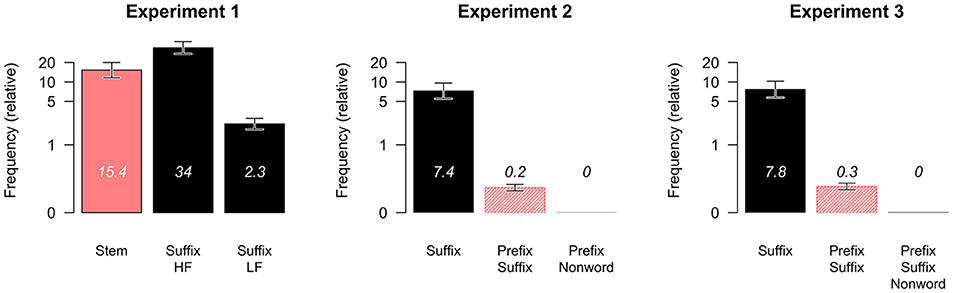
Figure 2. Mean (±1 SE) of the relative frequencies (frequency per million; on log scale) of the stimuli in Experiments 1, 2, and 3.
Differences Between Conditions
For the analyses, the keystroke latencies were transformed with an inverse transformation to approach normality (−1,000/keystroke latency). GAMM analyses were performed on the data for each experiment separately, with random intercepts included for the stem-triplets (i.e., words in three different conditions that were derived from the same stem), and for the first keystroke letter, and with by-participant non-linear random smooth's for Trial (i.e., the position of the word in the presentation sequence) to capture fluctuations in typing latencies over the course of the experiment that could cause autocorrelation in the residuals (Baayen and Milin, 2010). Model comparisons were utilized to determine whether the predictor Condition, which marks the three different experimental conditions, improved the model.
The GAMM analyses indicated that the first keystroke latencies in Experiment 1 were significantly influenced by condition [ = 12.32; p < 0.001; ΔAIC = 24.4]. Latencies on Stem words were shorter than in high-frequency suffix (Suffix-HF) words (βStem = −0.0191, SE = 0.0083; t-value = −2.30; p = 0.021). More importantly, the first keystroke latencies in low-frequency suffix words (Suffix-LF) were significantly longer than in high-frequency suffix words (βS−LF = 0.0224, SE = 0.0084; t-value = 2.65; p = 0.008). The first keystroke latencies in Experiment 2 were also significantly influenced by condition [ = 3.05; p = 0.048; ΔAIC = 5.18]: latencies for Prefix-Suffix words were longer than for Suffix words (βP−S = 0.040, SE = 0.020; t-value = 2.03; p = 0.042). While, the first keystroke latencies in Experiment 3 did not differ between Prefix-Suffix words and Suffix words (βP−S = 0.013, SE = 0.023; t-value = 0.57; p > 0.1), the effect of Condition [ = 9.58; p < 0.001; ΔAIC = 18.11] was reliable for the difference between length matched words like RENORMALIZE and nonwords like RENORMALIST.
The difference between high- and low-frequency suffixed words formed from the same stem in Experiment 1 is consistent with reports that word frequency influences the first keystroke latency such that first keystroke takes less time in the higher frequency suffix words than in the lower frequency suffix words. Words in those conditions differed in their frequency, but not in word length. The difference between the high-frequency suffix words and the Stem words, on the other hand, suggests that word length may play a role. The first keystrokes of the Stem words take less time to produce than in high-frequency suffixed words, but their frequency is lower on average than the high-frequency suffixed words (see Figure 2). Thus, both frequency and word length can affect first keystroke latencies. Based on these conclusions, we would expect to find a difference in the first keystroke latencies in Experiments 2 and 3 between the Suffix words and the Prefix-Suffix words, because they differ in word length and in frequency (Prefix-Suffix words are longer and have a lower average frequency than Suffix words, see Figure 2). However, this difference reached significance in Experiment 2, but not in Experiment 3.
Another factor that may influence the first keystroke latencies is the morphological complexity of the words: Words composed only of a stem should be easier to process than those affixed words with a suffix or prefix, and those affixed words with both (Prefix-Suffix) should be most difficult. To isolate an effect of frequency from morphological complexity, we investigated the effects of frequency on suffixed words by combining materials across experiments.
Effect of Frequency
To investigate the effects of whole word frequency on the first keystroke latencies more directly, we combined all Suffix-words from the three Experiments into one analysis (i.e., the conditions “Suffix-LF” and “Suffix-HF” from Experiment 1, and the conditions called “Suffix” from Experiments 2 and 3 –the black bars in Figure 1). Random intercepts for participants, stem-triplets, and the typed letters were included in the GAMM model, along with a by-participant random smooth for log Frequency and a by-participant random slope for OLD50. We included the predictor Experiment to test for differences between the experiments, and non-linear smooth's for whole word Frequency, and OLD50, our measure of orthographic distance from the 50 most similar words. Word length was not included as a predictor, because the experimental stimuli did not exhibit sufficient variation in stem length (range 5–6).
The statistical model indicated that the first keystroke latencies for suffixed words in Experiment 1 were significantly faster than for those in Experiments 2 and 3 (β1−2 = −0.080, SE = 0.043, t-value = 1.88, p = 0.061; β1−3 = 0.095, SE = 0.035, t = 2.71, p = 0.007). This could reflect at least in part the inclusion of pseudowords in the latter two experiments. In addition, the effect of Frequency was significantly different from zero [F(1.00,5121.559) = 11.74; p < 0.001], and linear (edf = 1.00); an edf (effective degrees of freedom of the smooth term) of 1 indicates a straight line)3. Visualization of the effect of frequency across experiments indicated that Suffix-words with lower frequency result in a longer first keystroke latency than Suffix-words with higher frequency. This is illustrated in Figure 4 (Left panel). OLD50 did not contribute to the model (see Figure 4, Right panel). Finally, the interaction between Experiment and Frequency did not improve the model [a model without the interaction resulted is a lower ML score (ΔML = 10.04), fewer degrees of freedom (Δdf = 4), and a lower AIC (ΔAIC = 18.11)].
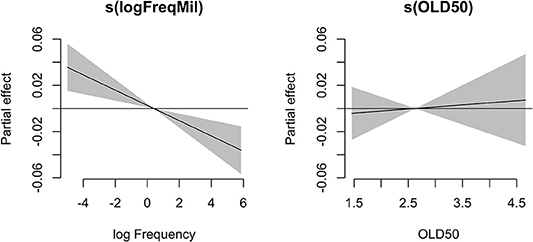
Figure 4. Partial effect estimates from the GAMM model. Left: Effect of frequency on the first keystroke latencies of Suffix-words. Right: (Non-significant) effect of OLD50 (orthographic similarity measure) on the first keystroke latencies of Suffix-words.
In summary, we have replicated the effect of whole word frequency on initial keystroke latency (in this experiment only marginally significant) and extended it to words composed of a stem and a suffix. Thus, we add a finding from a production task to the literature showing a robust effect of whole word frequency thereby complementing those identified in recognition tasks. In the next section we explore keystroke latencies to the stem independent from any effect of initial keystroke. We ask whether lexical effects are evident in processes associated with a purportedly encapsulated inner loop that controls keystroke execution.
Keystroke Latencies on the Stem (Normalized for Length)
The keystrokes following the initial keystroke, were typed considerably faster than the first. The average latency of the later keystrokes was 194 ms, which is considerably shorter than the first keystroke latency of 850 ms. With deference to the visual word recognition literature for morphology, we analyzed the keystroke latencies of the stem. To avoid redundancy with the analysis of the first keystroke of the word, in the stem latencies we always excluded the first keystroke of the stem. Further, the stem latency was normalized for stem length by dividing the sum of the latencies by the number of keystrokes (i.e., , with n the stem length and ki the keystroke latencies). Figure 4 presents the stem latencies for the conditions of the three experiments. Similarly to the first keystroke latencies (Figure 1), Experiment 1 shows the longest latencies for the low-frequency suffix words, and the shortest latencies for the stem words, but note that the difference between the stem and the high-frequency suffix words seems to be larger here than in the first keystroke latencies. In Experiments 2 and 3 the difference between the Prefix-Suffix and Suffix words also is more systematic than in the first keystroke latencies.
We adhered to the same procedure in analyzing the stem latencies as with the first keystroke latencies: first we investigated the differences between the conditions in each experiment, and then we combined the Suffix words from all experiments to investigate the effect of frequency and orthographic neighborhood density.
Differences Between Average Stem Keystroke Latencies Across Conditions
For the analyses, the keystroke latencies were again transformed with an inverse transformation to approach normality (−1,000/keystroke latency). GAMM analyses were performed on the data for each experiment separately, with random intercepts included for the word-triplets (i.e., words in three different conditions that were derived from the same stem), and with by-participant non-linear random smooth's for Trial (i.e., the position of the word in the course of the experiment) to capture fluctuations in typing latencies that can result in autocorrelation in the residuals. Model comparisons were conducted to evaluate whether inclusion of Condition improved the model.
The GAMM analyses indicated that the average stem keystroke latencies in Experiment 1 were significantly influenced by condition [ = 101.96; p < 0.001; ΔAIC = 206.96]: latencies in Stem words were shorter than in high-frequency suffix (Suffix-HF) words (βStem = −0.0787, SE = 0.0099; t-value = −7.98; p < 0.001), but the average stem keystroke latencies in low-frequency suffix words (Suffix-LF) was significantly longer than in high-frequency suffix words (βS−LF = 0.0641, SE = 0.0101; t-value = 6.37; p < 0.001). The average stem keystroke latencies in Experiment 2 were also significantly influenced by condition [ = 98.08; p < 0.001; ΔAIC = 198.45]: latencies in Prefix-Suffix words were longer than Suffix words (βP−S = 0.077, SE = 0.011; t-value = 6.90; p < 0.001), but latencies in Prefix-Nonwords were significantly shorter than Suffix words (βPN−S = −0.080, SE = 0.011; t- value = −7.27; p < 0.001). Similarly, for Experiment 3 the average stem keystroke latencies were significantly influenced by condition [ = 179.90; p < 0.001; ΔAIC = 355.62): latencies in Prefix-Suffix words were longer than Suffix words (βS−PS = −0.107, SE = 0.011; t-value = −9.39; p < 0.001), but shorter than the Prefix-Suffix nonwords (βPSN−PS = 0.111, SE = 0.012; t- value = 9.53; p < 0.001).
Different from the first keystroke latencies, the average stem latencies show reliable and systematic differences among all conditions. These differences are much stronger than the differences found with the first keystroke measure and indicate lexical effects on keystroke dynamics independent of the first keystroke. To investigate the effect of frequency and orthographic neighborhood density, again we analyzed the Suffix words.
Effect of Frequency
To ascertain the effects of frequency on the stem latencies, we combined all Suffix-words from the three Experiments in one analysis (i.e., the conditions “Suffix-LF” and “Suffix-HF” from Experiment 1, and the conditions called “Suffix” from Experiments 2 and 3—the black bars in Figure 5). As above, random intercepts for participants, and stem-triplets were included in the GAMM model, and a by-participant random smooth for log Frequency along with a by-participant random slope for OLD50. We included the predictor Experiment to test for differences between the experiments, and non-linear smooth's for Frequency, and OLD50, a measure of orthographic distance from the 50 most similar words that is similar in function to measures of bigram and trigram frequency. Word length was not included as a predictor, because the experimental stimuli did not show sufficient variation in word length.
The statistical model indicated that the stem latencies did not differ between the experiments even though the ratio of words to pseudowords varied. However, the effects of Frequency [edf = 1.77; F(1.770,5016.967) = 12.34; p < 0.001] and OLD50 [edf = 1.00; F(1.000,5016.967) = 7.18; p < 0.01] were significantly different from zero and followed a linear trend. As there were some words included which were not found in the corpus, we ran the model again without those extremely low frequencies to verify whether the effects of Frequency and OLD50 could be attributed to those outliers. In this new model the effects of Frequency [F(1.000,4496.278) = 13.71; p < 0.001] and OLD50 [F(1.000,4496.278) = 6.28; p = 0.012] remained significantly different from zero. Visualization of the effects depicted that for the same keystrokes, Suffix-words with lower frequency resulted in a longer stem latency than Suffix-words with higher frequency (Figure 6, Left panel). In addition, Suffix words with a shorter average distance (i.e., higher orthographic similarity) with the 50 most similar words resulted in shorter stem latencies than words with less orthographically similar neighbors (Figure 6, Right panel).
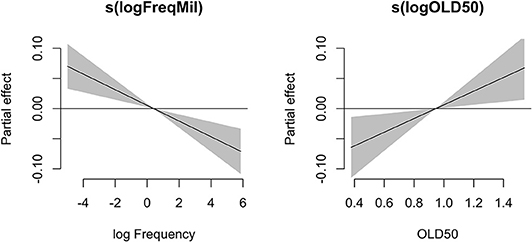
Figure 6. Partial effect estimates from the GAMM model. Left: Effect of frequency on the first keystroke latencies of Suffix-words. Right: Effect of OLD50 (orthographic similarity measure) on the first keystroke latencies of Suffix-words.
These combined analyses indicate that when typing the stem, both lexical knowledge based on whole word frequency as well as orthographic knowledge based on similarity with other words is available and influences the typing speed. Whereas, effects of orthographic knowledge on keystroke latencies have been documented frequently in the past, effects of whole word frequency on keystroke latencies after the initial keystroke have not.
Keystroke Latencies by Position in Stem
If variation in non-initial keystroke latencies across positions within a stem depends on the letter string within which it is nested then preparation and retrieval of a motor program cannot be completed in its entirety before the initiation of keystroke movements. Here we use GAMMs (Wood, 2017) to compare the trajectories for keystroke timing across stem position in lexical and morphological contexts formed around the same stem. We expect decreases in latencies across position and ask whether rate of keystroke execution decreases uniformly across stems that differ with respect to position of affix(es) and the lexical status of the particular combination when position within the word is held constant. Here, we examine the time course of morphological effects in production when keystrokes are aligned to stem onset but abutting morphemes differ.
Perhaps most obvious in Figure 7 is that keystroke latencies are not uniform across the stem and further, they vary according to the structure of the string in which the stem appears. Consistent with previous reports of slowing around the boundary between morphemes, increased latencies are visible at the onset of the stem after a prefix in Experiments 2 and 3. Stem-suffix boundary effects are difficult to detect, however, at least in part because of variation in suffix length. As a rule, stem by position latencies decrease both in the absence of a suffix and, to a lesser degree, in its presence. More interestingly, whole word frequency contributions introduced by manipulations of suffix are evident not only when typing the letters of the suffix but also in the course of typing the letters of the stem (Experiment 1). Evidently, production of keystroke latencies for the stem are not independent of the context in which it appears. For example in Experiment 1, the possibility of competing suffixes such as CY and LY as one transitions out of a stem such as NORMAL seems to offset the typical speeding up that occurs as one approaches the end of the word (e.g., positions 8–12). In both Experiments 2 and 3, stem latencies are faster in the production of a suffixed only word than in the production of that same string when accompanied by a prefix. See Figure B1 (Appendix B) for the same non-initial keystroke latencies aligned on the offset of the stem.
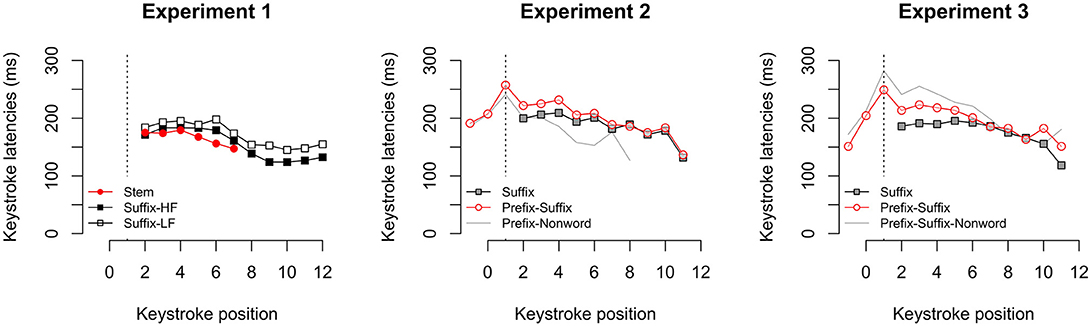
Figure 7. Mean keystroke trajectories (excluding the first keystroke) aligned to the onset of the stem for all conditions in Experiments 1, 2, and 3. The onset of the stem is indicated with a dashed vertical line.
Results such as these highlight some of the ways in which processing of the stem is interdependent with that of the affixes with which it co-occurs. We examine this interaction in more detail below because it may identify a potential weakness of an account of morphological processing restricted to the stem, and an account of typing where keystrokes are executed in series irrespective of emerging lexical or non-lexical context based on the particular combination of morphemes which accompany the stem.
Analyses of Keystroke Trajectories Aligned to Stem Onset
In order to further examine whether rate of keystroke execution was stable or decreased uniformly across positions in the word, we compared keystroke by position within stems that appeared in contexts composed of various combinations of affixes in keystroke trajectory analyses. The keystroke latencies again were transformed with a log-transformation to approach normality and we then excluded the first keystroke of the word from the analyses. We included the following predictors in our statistical models: Condition, which marks the three different morphological structures within an experiment, Keystroke Position, which captures the position of the keystroke within the stem relative to its onset, Key, indicating the particular letter that was typed, and Stimulus, describing the word-triplets, i.e., words in three different conditions that were derived from the same stem, and Participant. GAMM analyses were performed on the data for each experiment separately, with non-linear random smooth's included for Keystroke Position by Stimulus, and non-linear random smooth's for Keystroke Position by Participant by Condition, and a random intercept for Key, capturing the variation in typing caused by the different letters. The models were fitted using the smoothing parameter estimation method fREML (fast restricted maximum likelihood) for estimating the smoothing parameters, because the data were too large to use ML (maximum likelihood). As a consequence, the model comparisons may be less reliable. Therefore, we used both the model summary information and a model-comparison procedure to determine whether the predictors Condition and Keystroke Position and their interaction explained significantly more variance in the data than the baseline model with only random effects included.
For Experiment 1, we ran the GAMM analysis on the keystroke latencies in the stem (excluding the first keystroke), because the Stem words did not contain a suffix. Figure 8 (left panel) illustrates the estimated effects from the best-fitting statistical model. The model with the interaction between Condition and Keystroke Position included had a lower AIC value than the model without the interaction (ΔAIC = 8.69), but the fREML scores were not significantly different. Inspection of the estimated effects suggests that when typing the stem, there was no significant difference in keystroke latencies by position between the conditions Suffix-HF and Suffix-LF, although the latencies were faster when typing the Stem-words than in the other two suffixed conditions. Any distinctiveness of Suffix-HF keystrokes arose mainly at the end of the stem (see Figure 8, left). In addition, the summary statistics indicate a non-linear trend for typing keystrokes in the Stem words, that was significantly different from zero [FStem(3.732, 20421.281) = 4.28; p < 0.01], but nothing comparable for Suffix words. This outcome indicates that there is no difference between timing of keystroke positions for the stems in HF and LF productions as one produces the word.
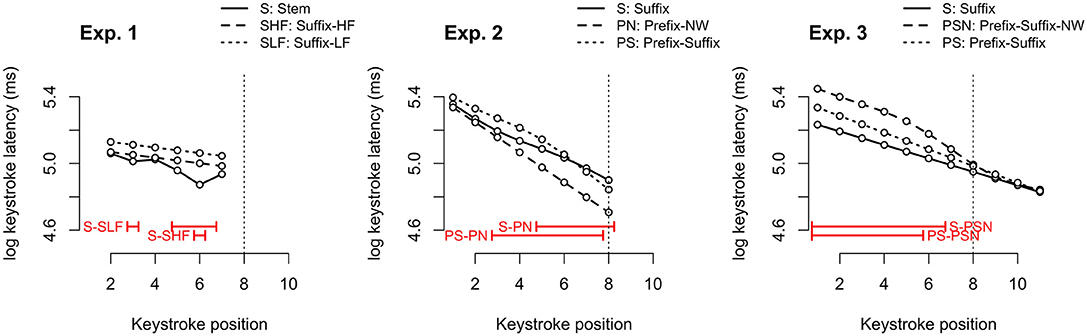
Figure 8. Estimates from the GAMM models fitting the keystroke trajectories (excluding the first keystroke) aligned to the onset of the stem for all conditions ([stem, stem + suffix low frequency; stem + suffix high frequency]; [stem + suffix; prefix + stem (NW); prefix + stem + suffix]; [stem + suffix; prefix + stem + suffix (NW); prefix+ stem + suffix] in Experiments 1, 2, and 3 respectively. The onset of the stem is labeled as 1. Positions with significant latency differences are indicated in red. The vertical dashed line indicates the median onset of the suffixes, which are only included in the analysis of Experiment 3.
These results fail to be consistent with the analysis of the average stem latencies where there was a significant difference between the two Suffix-conditions. It seems to be the case that the latencies on the low frequency Suffix-words are slightly longer than on the high frequency Suffix-words. The difference was not sufficient to establish significantly different keystroke trajectories but did indicate a difference when we summed the latencies across positions to calculate the average stem latencies. Note that if the program to produce all keystrokes were retrieved in parallel and executed according to the same sequencing constraints then the pattern of keystroke latencies should not differ depending on the upcoming morphemes. Either trajectories should not vary by position within the word or perhaps they should decrease uniformly in later positions within the word but an effect on the stem of an upcoming affix and the lexical acceptability of the stem-affix combination are not anticipated in a repository account.
For Experiment 2, we ran the GAMM analysis on the keystroke latencies in the stem only (excluding the first keystroke of the word), because the Prefix-Nonwords did not contain a suffix, and the Suffix words did not contain a prefix. Here again, model comparisons suggested that the model with the interaction between Keystroke Position and Condition explained significantly more variance than a model without this interaction [ = 8.17, p = 0.003; ΔAIC = 7.05]. In contrast with the results of Experiment 1, the summary statistics of this model indicate that the trends over Keystroke Position for all three conditions are significantly different from zero [FSuffix(2.247, 22396.844) = 5.05, p < 0.01; FPrefix−Suffix(2.545, 22396.844) = 7.94, p < 0.001; FPrefix−Nonword(1.019, 22396.844) = 32.00, p < 0.001]. Further inspection of the estimated effects suggests that there was no significant difference in keystroke latencies between the word conditions Suffix and Prefix-Suffix when typing the stem, but that the latencies were faster when typing the Prefix nonwords than in the other two word conditions (see Figure 8, center). An effect of lexicality on prefixed strings emerged early in the stem, which was followed by a suffix in the word conditions, but not in the nonword condition. The difference between the words and nonwords seems to point to the absence of a word final speed up as arose in the presence of competing suffixes in Experiment 1. In Experiment 3 we included nonwords with a suffix, to further probe lexicality effects.
In contrast to the analyses for Experiments 1 and 2, we included the keystrokes on the stem and the suffix when analyzing the data of Experiment 3 (continuing to exclude the first keystroke of the word). Once again, model comparisons suggest that the model with the interaction between Keystroke Position and Condition explained significantly more variance than did a model without this interaction [ = 6.19, p = 0.015; ΔAIC = 13.02]. The summary statistics reveal that both word conditions, Prefix-Suffix and Suffix words, were better fitted with a linear regression line (edf = 1) rather than a non-linear trend. All three conditions show a significant decrease in latencies with increasing Keystroke Position [FSuffix(1.001, 31387.140) = 22.00, p < 0.001; FPrefix−Suffix(1.000, 31387.140) = 38.11, p < 0.001; FPrefix−Suffix−Nonword(3.394, 31387.140) = 21.09, p < 0.001]. Inspection of the estimated effects suggests that there is no significant difference in keystroke latencies between the conditions Suffix and Prefix-Suffix when typing the stem. Importantly however, the latencies were slower when typing the Prefix-Suffix nonwords than in the other two conditions (see Figure 8, right). This finding supports the idea that nonwords are more difficult to type than words with the same stem and same morphological (affixation) structure. Note that the differences in keystroke latencies between the words and nonwords disappears at the end of the stem (see also Figure 8, right panel). Lexicality of the prefix-stem-suffix sequence influences early keystroke latencies but by the time that participants are typing the suffix, any effect of lexicality has dissipated.
Taken together, the analyses of all three experiments show a speed-up in latencies at the end of the word and an early lexicality effect, with slower latencies for nonwords than words on the stem, but not on the suffix.
Keystroke Variation as a Function of Whole Word Frequency
Our final insights into the production of morphologically complex words derive from analyses of the conditions under which effects of orthographic similarity interact with frequency. We again combine the two Suffix-conditions from Experiments 2 and 3 into one analysis. In contrast with the earlier analysis of the first keystroke latency and the stem latencies, we did not include the Suffix conditions from Experiment 1 in this analysis, because these showed larger variation in suffix as well as stem length, which necessarily makes aligning the trajectories more complex: Suffix words in Experiment 1 varied in the length of suffix between 1 (e.g., “jealousy”) and 6 characters (e.g., “satisfactory”). Materials in Experiments 2 and 3 underwent less variation in suffix length (2–4 characters).
Random non-linear smooth's for Keystroke Position by Stimulus, Keystroke by Participant, and Frequency by Participants were included in the GAMM model, along with a random intercept for Key. We included the predictor Experiment to test for differences between the experiments, and non-linear smooth's for Keystroke Position (aligned on the onset of the stem), Frequency, and OLD50, a measure of orthographic distance with the 50 most similar words. Word length was not included as predictor, because the experimental stimuli were constructed so as to restrict variation in word length. Of most interest were potential non-linear interactions between Keystroke Position and Frequency and between Keystroke Position and OLD50. Here again, the models were fitted using fREML for estimating the smoothing parameters, but because the data were too large for using ML, the model comparisons may be less reliable. Therefore, we report the summary statistics when these provide information on the contribution of a predictor or interaction. We excluded 10 words that did not occur in the Google Books Corpus as outliers from the analyses presented below, but we verified that this did not change the results by rerunning the models on all data. As the differences are small, we present here the data without the outliers.
The interaction between Keystroke Position on the stem plus suffix and OLD50 was significantly different from zero [F(3.478,16833.605) = 4.08; p < 0.01], but the interaction between Keystroke Position and Frequency made only a weak contribution to the model [F(2.524,16833.605) = 2.78; p = 0.034] (it did not reach significance with all data included). Further, there was a linear main effect for Frequency [F(1.000,16833.605) = 15.27; p < 0.001] and for Keystroke Position [F(3.248,16833.605) = 5.44; p < 0.001] with no effect of experiment. Figure 9 presents the model estimates of the partial effects (i.e., individual model terms) for Keystroke Position, Frequency, and the interaction between Keystroke Position and Frequency on the top row. The effect of Keystroke Position showed a general decrease in keystroke latencies, along with an even steeper decrease after the stem (the stem length ranges between 4 and 8 characters, with a median stem length of 6 characters).
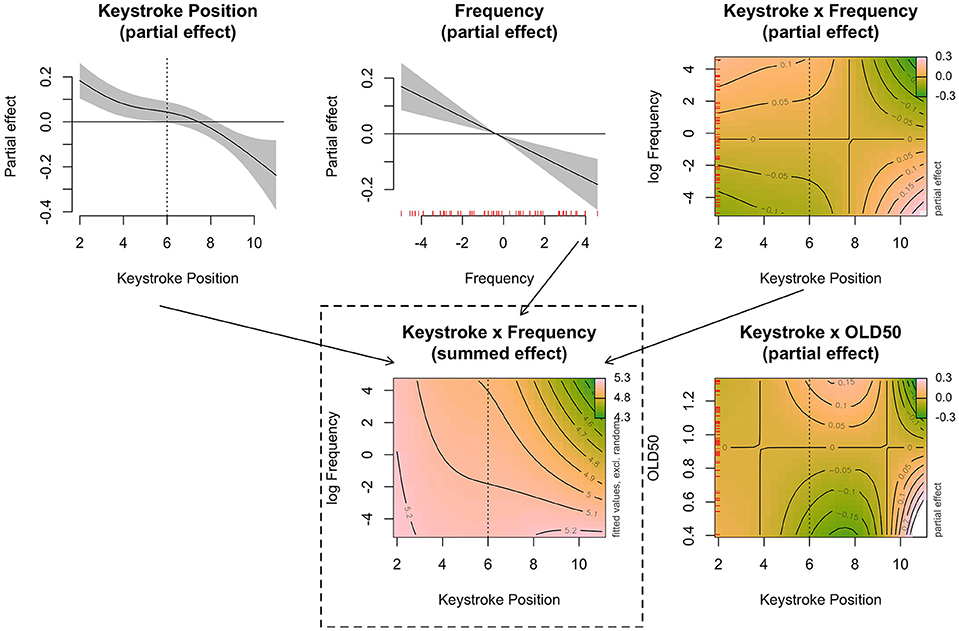
Figure 9. Estimates from the best-fitting statistical models of the keystroke trajectories (excluding the first keystrokes of the words) aligned to the onset of the stem for the Suffix words in Experiments 2, and 3. The top row shows the partial effects of Keystroke Position (left), Frequency (center), and Keystroke Position by Frequency (right). The bottom row shows the partial effect of Keystroke Position by OLD50 (right) and the summed effects of Keystroke Position by Frequency, with random effects excluded (center).
In the analysis of the stem trajectories of Experiment 3 suffixes are included because all conditions had suffixes. In this case, no boundary pattern was detectable in the analysis. There was an overall frequency effect, with lower word frequencies resulting in generally longer keystroke latencies. The partial interaction effect indicates that the word frequency effect is stronger when typing the affix than when typing the stem. The center panel (surrounded by a box) shows the summed effects for Keystroke Position and Frequency, including the partial effects in the top row and the intercept. Of particular relevance is that higher frequency words show a much steeper decrease in keystroke latencies at the end of the word than do lower frequency words. Finally, the bottom left panel shows the estimated interaction (partial effect) of Keystroke Position by OLD50. This interaction suggests that the effect of orthographic uniqueness (lower OLD50 values) increases the latencies at the end of the stem and on the suffix. For the longer words, this effect seems to be reversed around the last characters. Basically, any effect of orthographic similarity comes in later than the effects of whole word frequency.
At a minimum, it is evident that effects of frequency on keystrokes latencies persist throughout an entire word and that they are not uniform across position; as a rule, they decrease across positions in the word. Perhaps most important is that the rate of speeding up varies with whole word frequency. Stated succinctly, keystrokes latencies in higher frequency words show a more dramatic reduction at the end of the word than in lower frequency words.
Discussion
In the present study we asked whether average keystroke latencies and related measures for identical constituent letters in a stem vary systematically according to their lexical properties (letter length, orthographic neighborhood density) or to those of the words in which they appear (whole word frequency). As a rule, higher frequency words were typed more quickly and more accurately than lower frequency words, and words were typed more quickly and more accurately than nonwords when length was matched. In the framework where the mental lexicon is treated as a repository of lexical knowledge and access to it is conceptualized as all or none, time to access knowledge about a particular word will vary across words due to frequency and this effect of whole word frequency gets reflected in the layout or organization of word representations in the lexicon. In essence, recognition of a word or the motor program to type it is described as a search through a repository of words where search duration depends on the manner in which the repository is organized.
A prominent recent model of expert typing is compatible with this tradition and posits two independent loops (Crump and Logan, 2010a,b; Logan and Crump, 2010, 2011). In this framework, the outer loop passes along lexical knowledge about the requisite motor program to the inner loop but is blind to keystroke sequencing or timing which are the responsibility of the inner loop. It is successful in accounting for a number of interesting effects (see Logan, 2018 for review). As we have highlighted above, the model does not predict that lexical effects that persist into the inner loop should vary across keystroke positions because component keystrokes are activated in parallel and executed in series once a word is retrieved (Crump and Logan, 2010a,b; Logan and Crump, 2010, 2011). As noted above, however, effects of retyping a probed position seem more consistent with graded activation across positions, because effects are stronger earlier in a word (Logan et al., 2016). In this case, the higher-level word unit may be activating all of the keystrokes in parallel but there is some indication that execution varies with position within the word. Similarly, degree of disruption to typical keystroke position vary according to the position of the target letter within the word (Yamaguchi and Logan, 2014).
In the present study, we provide novel evidence that activation as measured by keystroke latency does vary with position within the word and that it is not uniform across contexts when length is controlled. Rather, measures based on keystroke latencies can be influenced by stem position within the string, by string lexicality and affixation, and by similarity of the target string to other words. It is important to note that in prior discussion, Logan and Crump (2011, p. 7) do acknowledge the potential cross-talk between these loops, but argue that these relationships are unlikely to contribute substantially to explaining variation in production. Such a comparison of effect size between purported outer and inner loops is outside the scope of the present study. However, the methods we described in our three experiments may permit new investigation of these distinctions through new tasks and, importantly, new statistical models. We elaborate below.
Most novel in our study was the analysis of keystroke trajectories which revealed not only that rate of keystroke execution decreased across positions in the word but also that those changes were not uniform over different morphological structures and word frequencies. In order to test these effects, we took a multilevel model-building approach, integrated various item- and subject-level factors contributing to the sequence of interkey intervals, and thereby controlled for a variety of factors. Across three experiments, these analyses helped quantify subtle aspects of word production in typing.
Of particular note were the anticipatory effects of an upcoming affix on keystroke trajectories according to the lexical acceptability of the combination. In the tradition of morphological decomposition in the recognition literature, one might have expected the contribution of the stem to predominate over that of any affixes that were produced at the same time. In fact, the effect of Keystroke Position showed a general decrease in keystroke latencies, along with an even steeper decrease after the stem. Effects of whole word frequency on keystroke timing could be documented with several keystrokes measured on the stem but, here again, the trajectory analysis in Figure 9 indicated that the word frequency effect was more pronounced when typing the affix than when typing the stem. Finally, higher frequency words showed a steeper decrease in keystroke latencies at the end of the word than did lower frequency words. Similarly, we observed an interaction (partial effect) of Keystroke Position with OLD50 such that orthographic uniqueness (lower OLD50 values) increased keystroke latencies at the end of the stem and into the suffix.
In these results, keystroke latencies are not retrieved and executed in a uniform manner. Finally, the dynamic but not the retrieval account anticipates interactions of typing measures with orthographic similarity of the target to other words or to predictability of the affix given the particular stem. Processes at different levels (visual form recognition, morphological segmentation, semantic processing, etc.) seem to be fluidly interacting throughout performance. This interaction among processes could be the mechanistic underpinning of language processing and production. Finding new echoes of this parallelism in behavioral metrics offers a promising new direction to test such predictions about styles of interaction among levels of control.
Summary and Conclusion
In the word recognition literature that focuses on morphological processing, the repository account typically asserts that access to the lexicon entails decomposing a morphologically complex word into its constituent morphemes by a process that is blind with respect to the semantics of the stem. Recent reports demonstrating the salience of whole word as contrasted with stem frequency in the course of morphological processing have substantially weakened this account (Baayen et al., 2007; Milin et al., 2017; Schmidke et al., 2017). Once one accesses the lexicon, one can retrieve the motor program to produce a word as by typing it, but we have demonstrated here that that process is not executed independently from its lexical and morphological properties.
We reported the results of three experiments where the critical comparison focuses on a repeated stem morpheme in a variety of morphological and lexical contexts. As in the decomposition account in word recognition, if morphological decomposition and stem access dominated processing in an online typing-to-copy task, then structures that accompanied the stem could have been ignored or played only a secondary role. In this framework one might have expected latencies for the keystrokes that comprise the stem should have been more stable over morphological and lexical contexts. On the contrary, as depicted in Figures 5–7, this was not the case. Rather, patterns of keystroke latencies for letters in the stem highlight the interactions of lexical and morphological effects on stem production. Further, an effect of lexicality based on an incompatible stem- suffix combination emerged while executing the keystrokes of the stem in anticipation of the upcoming deviation. The theoretical upshot is an unencapsulated parallelism—from motor control to morphological semantics, these patterns and systematicities are whispering to each other in a manner that is measurable in performance.
Ethics Statement
Data were collected online and we had no face to face contact with participants. The UCLA Institutional Review Board (UCLA IRB) has determined that the above referenced study meets the criteria for an exemption from IRB review. UCLA's Federal wide Assurance (FWA) with Department of Health and Human Services is FWA00004642.
Author Contributions
RD designed the web interface for data collection. LF designed the materials. JvR created the analyses. All authors were engaged in writing the manuscript.
Funding
The development of this publication was supported by the Words in the World Partnership Project funded by the Social Sciences and Humanities Research Council of Canada (895-2016-1008).
Conflict of Interest Statement
The authors declare that the research was conducted in the absence of any commercial or financial relationships that could be construed as a potential conflict of interest.
Footnotes
1. ^When it comes to predicting reaction times (RTs) in a lexical decision or a naming task, Baayen et al. (2016) interpret the ostensible superiority of the subtitle corpus as reflecting a confound of frequency with other variables that affect processing in those particular time-limited tasks. By comparison, eye-tracking measures while reading prose from English novels are better predicted from the written British National Corpus (BNC) than from subtitles (Hendrix, 2015).
2. ^https://github.com/racdale/keystroke-timing-feldman-dale-van-rij
3. ^An edf (effective degrees of freedom of the smooth term) of 1 indicates a straight line.
References
Adelman, J. S., Brown, G. D. A., and Quesada, J. F. (2006). Contextual diversity, not word frequency, determines word-naming and lexical decision times. Psychol. Sci. 17, 814–823. doi: 10.1111/j.1467-9280.2006.01787.x
Adelman, J. S., Brown, G. D. A., and Quesada, J. F. (2008). Modeling lexical decision: the form of frequency and diversity effects. Psychol Rev. 115, 214–229. doi: 10.1037/0033-295X.115.1.214
Baayen, R. H., Feldman, L. F., and Schreuder, R. (2006). Morphological influences on the recognition of monosyllabic monomorphemic words. J. Mem. Lang. 53, 496–512 doi: 10.1016/j.jml.2006.03.008
Baayen, R. H., Milin, P., and Ramscar, M. (2016). Frequency in lexical processing, Aphasiology 30, 1174–1220. doi: 10.1080/02687038.2016.1147767
Baayen, R. H., Wurm, H. L., and Aycock, J. (2007). Lexical dynamics for low-frequency complex words. A regression study across tasks and modalities. The Mental Lexicon 2.3, 419–463. doi: 10.1075/ml.2.3.06baa
Barca, L., and Pezzulo, G. (2012). Unfolding visual lexical decision in time. PLoS ONE 7:e35932. doi: 10.1371/journal.pone.0035932
Barca, L., and Pezzulo, G. (2015). Tracking second thoughts: continuous and discrete revision processes during visual lexical decision. PLoS ONE, 10:e0116193. doi: 10.1371/journal.pone.0116193
Baus, C., Strijkers, K., and Costa, A. (2013). When does word frequency influence written production? Front Psychol. 4:963. doi: 10.3389/fpsyg.2013.00963
Brants, T., and Franz, A. (2006). Web 1T 5-gram, Version 1. Philadelphia: Linguistic Data Consortium, University of Pennsylvania
Brysbaert, M., and New, B. (2009). Moving beyond Kucera and Francis: a critical evaluation of current word frequency norms and the introduction of a new and improved word frequency measure for American English. Behav. Res. Methods. 41, 977–990. doi: 10.3758/BRM.41.4.977
Christiansen, M. H., and Chater, N. (2016). The now-or-never bottleneck: a fundamental constraint on language. Behav. Brain Sci. 39, 1–72. doi: 10.1017/S0140525X1500031X
Crump, M. J. C., and Logan, G. D. (2010a). Hierarchical control and skilled typing: Evidence for word-level control over the execution of individual keystrokes. J. Exp. Psychol. Learn. Memory Cogn. 36, 1369–1380. doi: 10.1037/a0020696
Crump, M. J. C., and Logan, G. D. (2010b). Warning: This keyboard will deconstruct—The role of the keyboard in skilled typewriting. Psychon. Bull. Rev. 17, 394–399. doi: 10.3758/PBR.17.3.394
Davies, M. (2008). The Corpus of Contemporary American English (COCA): 410+ Million Words, 1990-Present. Available online at: www.americancorpus.org
Elman, J. L. (2004). An alternative view of the mental lexicon. Trends Cogn. Sci. 8, 301–306. doi: 10.1016/j.tics.2004.05.003
Elman, J. L. (2009). On the meaning of words and dinosaur bones: lexical knowledge without a lexicon. Cogn. Sci. 33, 547–582. doi: 10.1111/j.1551-6709.2009.01023.x
Feldman, L. B., Vinson, D., and Dale, R. (2017). “Production of morphologically complex words as revealed by a typing task: Interkeystroke measures challenge the viability of morphemes as production units,” in Paper Presented at The Mental Lexicon, October (Ottawa, ON).
Fitts, P. M. (1954). The information capacity of the human motor system in controlling the amplitude of movement. J. Exp. Psychol. 47, 381–391. doi: 10.1037/h0055392
Gagné, C. L., and Spalding, T. L. (2014). Typing time as an index of morphological and semantic effects during English compound processing. Lingue E Linguaggio 13, 241–262. doi: 10.1418/78409
Gagné, C. L., and Spalding, T. L. (2016). Effects of morphology and semantic transparency on typing latencies in english compound and pseudocompound words. J Exp Psychol Learn Mem Cogn. 42, 1489–95. doi: 10.1037/xlm0000258
Gentner, D. R., Larochelle, S., and Grudin, J. (1988). Lexical, sublexical, and peripheral effects in skilled typewriting. Cogn. Psychol. 20, 524–548. doi: 10.1016/0010-0285(88)90015-1
Hendrix, P. (2015). Experimental Explorations of a Discrimination Learning Approach to Language Processing. Doctoral Dissertation, University of Tuebingen.
Herdagdelen, A., and Marelli, M. (2017). Social Media and language processing: how facebookand twitter provide the best frequency estimates for studying word recognition. Cogn. Sci. 41, 976–995. doi: 10.1111/cogs.12392
Hick, W. E. (1952). On the rate of gain of information. Q J Exp Psychol. 4, 11–26. doi: 10.1080/17470215208416600
Hyman, R. (1953). Stimulus information as a determinant of reaction time. J Exp Psychol. 45, 188–96. doi: 10.1037/h0056940
Inhoff, A. W. (1991). Word frequency during copytyping. J Exp Psychol Hum Percep Perform. 17, 478–487. doi: 10.1037/0096-1523.17.2.478
Jones, M. N., and Mewhort, D. J. (2007). Representing word meaning and order information in a composite holographic lexicon. Psychol. Rev. 114, 1–37. doi: 10.1037/0033-295X.114.1.1
Keuleers, E. (2013). vwr: Useful Functions for Visual Word Recognition Research. R package version 0.3.0. Available online at: https://CRAN.R-project.org/package=vwr
Libben, G., Curtiss, K., and Weber, S. (2014). Psychocentricity and participant profiles: implications for lexical processing among multilinguals. Front. Psychol. 5:557. doi: 10.3389/fpsyg.2014.00557
Libben, G., Weber, S., and Miwa, K. (2012). A technique for the study of perception, production, and participant properties. Mental Lexicon 7, 237–248. doi: 10.1075/ml.7.2.05lib
Logan, G. D. (2018). Automatic control: How experts act without thinking. Psychol. Rev. 125, 453–485. doi: 10.1037/rev0000100
Logan, G. D., and Crump, M. J. C. (2010). Cognitive illusions of authorship reveal hierarchical error detection in skilled typists. Science 330, 683–686. doi: 10.1126/science.1190483
Logan, G. D., and Crump, M. J. C. (2011). “Hierarchical control of cognitive processes: the case for skilled typewriting,” in Psychology of Learning and Motivation, Vol. 54, ed B. H. Ross (Burlington: Academic Press), 1–27.
Logan, G. D., Ulrich, J. E., and Lindsey, D. R. B. (2016). Different (key)strokes for different folks: how standard and nonstandard typists balance Fitts' law and Hick's law. J Exp Psychol. 42, 2084–2102.
Milin, P., Feldman, L. B., Ramscar, M., Hendrix, P., and Baayen, R. H. (2017). Discrimination in lexical decision. PLoS ONE 12:e0171935. doi: 10.1371/journal.pone.0171935
Milin, P., Smolka, E., and Feldman, L. B. (2018). “Models of lexical access and morphological processing,” in The Handbook of Psycholinguistics, eds H. Cairns and E. Fernandez (London: Whiley).
Pinet, S., Ziegler, J. C., and Alario, F.-X. (2016). Typing is writing: Linguistic properties modulate typing execution. Psych Bull Rev. 23, 1898–906. doi: 10.3758/s13423-016-1044-3
R Core Team (2018). R: A Language and Environment for Statistical Computing. Vienna: R Foundation for Statistical Computing. Available online at: https://www.R-project.org/
Rieger, M., and Bart, V. K. E. (2016). Typing style and the use of different sources of information during typing: an investigation using self-reports. Front. Psychol. 7:1908. doi: 10.3389/fpsyg.2016.01908
Rumelhart, D. E., and Norman, D. A. (1982). Simulating a skilled typist: a study of skilled cognitive-motor performance. Cogn. Sci. 6, 1–36. doi: 10.1207/s15516709cog0601_1
Schmidke, D., Matsuki, K., and Kuperman, V. (2017). Analysis of the time-course of complex word recognition. J. Exp. Psychol. Learn. Memory Cogn. 43, 1793–1820 doi: 10.1037/xlm0000411
Seidenberg, M. S., and McClelland, J. L. (1989). A distributed, developmental model of word recognition and naming. Psychol. Rev. 96, 523. doi: 10.1037/0033-295X.96.4.523
Shaffer, L. H. (1975). Control processes in typing. Q. J. Exp. Psychol. 27, 419–432. doi: 10.1080/14640747508400502
Spivey, M. J., and Dale, R. (2006). Continuous dynamics in real-time cognition. Curr. Dir. Psychol. Sci. 15, 207–211. doi: 10.1111/j.1467-8721.2006.00437.x
Spivey, M. J., Grosjean, M., and Knoblich, G. (2005). Continuous attraction toward phonological competitors. Proc. Natl. Acad. Sci. U.S.A. 102, 10393–10398. doi: 10.1073/pnas.0503903102
van Rij, J., Hendriks, P., van Rijn, H., Baayen, R. H., and Wood, S. N. (2019a). Analyzing the time course of pupillometric data. Trends Hear. Sci. 23, 1–22. doi: 10.1177/2331216519832483
van Rij, J., Vaci, N., Wurm, L. H., and Feldman, L. B. (2019b). “Alternative quantitative methods in psycholinguistics: implications for theory and design,” in Word Knowledge and Word Usage: A Cross-Disciplinary Guide to the Mental Lexicon, eds V. Pirrelli, I. Plag, and W. U. Dressler. Berlin: Mouton de Gruyter.
van Rij, J., Wieling, M., Baayen, R. H., and van Rijn, H. (2017). itsadug: Interpreting Time Series and Autocorrelated Data Using GAMMs. R package version 2.3. Available online at: https://cran.r-project.org/web/packages/itsadug/index.html
West, L. J., and Sabban, Y. (1982). Hierarchy of stroking habits at the typewriter. J. Appl. Psychol. 67, 370–376. doi: 10.1037/0021-9010.67.3.370
Wieling, M. (2018). Analyzing dynamic phonetic data using generalized additive mixed modeling: a tutorial focusing on articulatory differences between L1 and L2 speakers of English. J. Phon. 70, 86–116. doi: 10.1016/j.wocn.2018.03.002
Wood, S. N. (2017). Generalized Additive Models: An Introduction With R, 2nd Edn. Boca Raton, FL: Chapman and Hall; CRC.
Yamaguchi, M., and Logan, G. D. (2014). Pushing typists back on the learning curve: revealing chunking in skilled typewriting. J. Exp. Psychol. Hum. Percept. Perform. 40, 592–612. doi: 10.1037/a0033809
Yarkoni, T., Balota, D., and Melvin Yap, M. (2008). Moving beyond coltheart's N: a new measure of orthographic similarity. Psychon. Bull. Rev. 15, 971–979. doi: 10.3758/PBR.15.5.971
Appendix A
Appendix B
Mean keystroke trajectories aligned on the offset of the stem.
Keywords: type-to-copy task, written production, morpheme, keystroke trajectory, lexicality, typing, letter position effects
Citation: Feldman LB, Dale R and van Rij J (2019) Lexical and Frequency Effects on Keystroke Timing: Challenges to a Lexical Search Account From a Type-To-Copy Task. Front. Commun. 4:17. doi: 10.3389/fcomm.2019.00017
Received: 07 November 2018; Accepted: 15 April 2019;
Published: 03 June 2019.
Edited by:
Gonia Jarema, Université de Montréal, CanadaReviewed by:
Laura Barca, Italian National Research Council (CNR), ItalyJianfeng Yang, Shaanxi Normal University, China
Copyright © 2019 Feldman, Dale and van Rij. This is an open-access article distributed under the terms of the Creative Commons Attribution License (CC BY). The use, distribution or reproduction in other forums is permitted, provided the original author(s) and the copyright owner(s) are credited and that the original publication in this journal is cited, in accordance with accepted academic practice. No use, distribution or reproduction is permitted which does not comply with these terms.
*Correspondence: Laurie Beth Feldman, bGZlbGRtYW5AYWxiYW55LmVkdQ==