- 1Department of Advertising and Public Relations, Michigan State University, East Lansing, MI, United States
- 2Department of Journalism and Creative Media, University of Alabama, Tuscaloosa, AL, United States
Type 1 diabetes (T1D) is diagnosed mostly during childhood or adolescent years. During such transitional phases of life, having support from others in similar situations can reduce feelings of isolation and loneliness. Instagram, a platform with high use among teens or young adults, acts as an alternative to traditional online health communities. To better understand individuals self-disclosure on Instagram related to T1D, we conducted an exploratory quantitative content analysis of a sample of 423 posts using the hashtag #t1dlookslikeme. These posts were collected using Netlytic between July—October 2018. Our research questions asked about the types of hashtags used, the content of the images, the sentiments of the posts, the relationship between post engagement and post sentiment, if and how the posts represented self-disclosure, and the presence of social support. A codebook containing 43 items on the image and 10 codes for captions was created for this study, and all data were analyzed using SPSS. Our dataset included 89% images compared to 6.4% video clips. Additionally, 83.5% of the posts were personal images whereas 11.6% were categorized as memes. We noted the most popular hashtags, and other characteristics of the images used by individuals to self-disclose their T1D. Overall, our random sample contained more positive sentiment posts rather than negative—and these positive sentiment posts were correlated with a higher number of hashtags in each post. Indicating a possible connection between self-disclosure and positive sentiment. This finding also reflected elements of empowerment (such as taking the “power” away from T1D and returning it to themselves), which is also discussed.
Introduction
Since the advent of social media platforms individuals have openly self-disclosed their health experiences, received interpersonal social support, and built communities around understanding of their illness on these platforms (Greene et al., 2011; Shaw and Johnson, 2011). These platforms were not created specifically to share health information however, they provide a place for people to receive social support from others experiencing the same issues. There have been several studies examining the impact of Instagram on a wide variety of health topics including e-cigarettes/vaping/ hookah use (Laestadius et al., 2016; Allem et al., 2017b; Chu et al., 2017; Ben Taleb et al., 2019), nutrition (Sharma and De Choudhury, 2015), and weight (Santarossa et al., 2016; Slater et al., 2017; Tiggemann and Zaccardo, 2018). Yet, relatively little research to date has explored Instagram's contribution to community building through individuals' self-disclosure and social support they receive in regard to Type 1 diabetes (T1D), which is also associated with stigma.
This exploratory study employed a quantitative content analysis approach to better understand self-disclosure through the hashtag “#t1dlookslikeme” on Instagram in order to examine how individuals with T1D self-present, share their experiences, and perceive social support. Further, to our knowledge, this is the first study exploring self-disclosure through the visual lens of Instagram in order to examine its role in community building. Therefore, the objective of this study is to explore this topic, by asking about the kinds of posts users share within this space, and then examine, and analyze them in further detail.
Literature Review
Type 1 Diabetes
T1D is a lifelong condition, in which the pancreas no longer produces insulin. Insulin is a hormone that breaks down sugar so that our bodies can use it for energy. Managing diabetes requires a carefully choreographed routine, including regularly testing blood glucose (sugar), monitoring carbohydrates, activity levels, and measuring and administering insulin. Approximately 1.25 million Americans have been diagnosed with T1D and an estimated 40,000 more people will be diagnosed each year (Centers for Disease Control, 1991). Often people with T1D use the internet, particularly online forums, and social media to connect to others living with the disease, to find information, receive social support, and feel a sense of belonging to a community (Greene et al., 2011; Shaw and Johnson, 2011).
Health and Online Sharing
It has been well documented through research that the internet provides a space for people to share their own health stories while also listening to experiences of others (Fox, 2011; Jin et al., 2015; Myrick et al., 2015; Huh et al., 2016; Kanthawala et al., 2016). These exchanges are more than a mere exchange of experiences–they provide people with information by answering questions, offer guidance, provide social support, and help develop a sense of community within the group (Kanthawala et al., 2016). This type of sharing of health information has been observed in many online health communities, for example WebMD, where sub-communities are formed around specific health conditions (Huh et al., 2013; Kanthawala et al., 2016; Willis and Royne, 2017). It is important to note, that the context of these studies has been from an information seeking perspective, rather than a sharing perspective. This means, that there is a body of work exploring what health content people search for when they go online (Eysenbach and Köhler, 2002; Efron and Winget, 2010; Nambisan, 2011; Perez et al., 2015; Zhao et al., 2015; Jin et al., 2016; Roberts and Demner-Fushman, 2016), but the work regarding what they share (often voluntarily and without being asked) has not been deeply explored.
Furthermore, not all online communities where health topics are discussed were necessarily designed for that purpose. For example, people post about their experiences on social media platforms, such as Facebook, Twitter, or Instagram. This might especially occur when people are just posting about their day-to-day experiences and not something targeted toward solely a health community. They are able to connect with others through the use of hashtags. Hashtags are keywords or tags which follow the “#” sign that are used to indicate the content of a post. The traditional role of hashtags was to “organize knowledge and facilitate access and enable retrieval of information” (Giannoulakis and Tsapatsoulis, 2016, p. 115). Instagram introduced hashtags in 2015, allowing images to be searchable by terms, instead of just looking for a specific person (Instagram, n.d.), thereby increasing the visibility of a picture (Giannoulakis and Tsapatsoulis, 2016).
The discussion of social media sharing around health becomes especially relevant when we discuss T1D which affects a younger population (most people are diagnosed before age 20, the average age of diagnosis is 14) (American Diabetes Association, 2015; Beyond Type 1, 2019), an age group that is heavily active on social media, but might not be as active in traditional health websites. Instagram is a particularly popular platform for this group to be active on and share their experiences. In fact, the Pew Research Center reports that 72% of individuals aged 13–17 years and 75% of individuals aged 18–24 are on Instagram (Anderson and Jiang, 2018; Perrin and Anderson, 2019), making this an appropriate platform for the discourse surrounding T1D. This makes Instagram the ideal platform to study because of the intersecting nature of its popularity with younger people and the young age of T1D diagnosis, in addition to disclosures occurring on this platform by primarily this age group. Since no study to our knowledge has examined T1D representations on Instagram, we sought to understand the general landscape of this context. Therefore, we pose the following research question:
RQ1: Overall, what do T1D posts look like? (a) How many hashtags are used? (b) Are diabetes management items present?
Self-Disclosure
Self-disclosure has been explained as an act of revealing personal information about oneself deliberately that others might be unable to discover through different ways (Derlega et al., 1993; Catona and Greene, 2016; Masaviru, 2016). Furthermore, self-disclosure does not always need to be deep or meaningful, superficial self-disclosure in the form of small-talk tends to initiate relationships that can lead to more personal self-disclosures (Masaviru, 2016). Being exposed to self-disclosure, leads people to a reciprocal disclosure, which further leads to trust and development of a relationship (Masaviru, 2016). Additionally, according to Derlega et al. (1993) self-disclosure causes cognitive, emotional, and behavioral reactions by both the person disclosing as well as the person receiving said disclosure.
Self-disclosure has been studied many times in the context of health. Oftentimes, this has to do with the important role of disclosure in coping with health conditions and positive outcomes such as improved health, quality of health, and perceptions of social support (Greene et al., 2006; Catona and Greene, 2016). Self-disclosure in health has been studied in personal relationships to help cope with personal tragedy, such as a terminal health diagnosis (Catona and Greene, 2016), physical functioning in breast cancer patients (Figueiredo et al., 2004), and illness rates among individuals whose spouses have died (Pennebaker and O'Heeron, 1984). However, there may be many reasons that an individual may choose to not disclose their health conditions (Santuzzi et al., 2014; Chang and Bazarova, 2016). For example, employees may choose to not disclose to evade possible stigma from employers and co-workers or hurdles to promotions. This tends to occur for people concealing stigmatized disabilities or those that might be seen by others as preventing workers from doing their job (Santuzzi et al., 2014).
Stigma
Stigma often refers to a moral discrediting attribute (Chang and Bazarova, 2016). Individuals who are stigmatized are often “reduced in our minds from a whole and usual person to a tainted, discounted one” (Goffman, 1963, p.3) because they deviate from what society deems as normal. Stigma can be contextual in nature because how stigmatization occurs is often dependent upon time, place, and group (Meisenbach, 2010). Stigma also has lasting impacts on an individual's stress level (Link and Phelan, 2001, 2006; Major and O'Brien, 2005). Thus, in order to eliminate stigma, many individuals will try to manage feelings of stigmatization by turning to communities for social support which can help members regain pride in their illness instead of seeking to get well, it also buffers them from the impact of stress (Goffman, 1963; Cohen and Wills, 1985; Major and O'Brien, 2005; Rains and Keating, 2011). Traditionally, anonymous online forums were attractive primarily due to the anonymity they provide. However, with social media platforms such as Instagram, Twitter, or Facebook, discussions are more similar to face-to-face interactions due to the relative lack of anonymity with user profiles and curated friends and followers (Boudewyns et al., 2015).
Online Self-Disclosure
In traditional online health community sharing (i.e., PatientsLikeMe, where the sole reason that people are on there is specifically for health reasons), people discuss their health conditions and situations, often as a response to a question posted or in an effort to explain their situations to others in order to help guide them. However, there is another component about sharing health conditions—this is not necessarily about providing people with information to help with their situations (though that might occur as a positive side effect). Instead, people self-disclose their experiences as a way to express their daily lives and how their challenges are more than a sum of their health experiences (Andalibi et al., 2017). In the early internet era, such expressions might have occurred through blogging or individual websites dedicated to this. However, today, a lot of similar traffic has moved from independent blogs to social media sites like Facebook, Twitter, Instagram, etc. (De Choudhury and De, 2014).
In online spaces, self-disclosure has been explored in the contexts of mental health and disclosure, social support and anonymity on social media, disclosing stigmatic health conditions through weblogs (De Choudhury and De, 2014; Rains, 2014; Balani and De Choudhury, 2015), and disclosure of substance use on social media (Morgan et al., 2010). Barak et al. (2008) note how self-disclosure can foster empowerment within online support groups. Further, Catona and Greene (2016) state how the “changes in technology have fundamentally restructured some forms of interpersonal relationship contact (e.g., mobile technology or Facebook), and there is a need to explore how disclosure theories apply to or should be modified with these changes” (p. 4). The changing role of technologies are especially visible through social media, which itself is evolving at a rapid pace. What started as primarily text-based content being shared on these platforms is transforming to visual content being shared more and more and visual platforms growing in popularity (We Are Social, Hootsuite, and DataReportal, 2019). We also note that individuals who use platforms like Instagram, which are primarily visual in nature, are skewed more toward younger users (We Are Social, Hootsuite, and DataReportal, 2019). This in conjunction with visual sociology literature which situates imagery as a powerful means of expression and the large volumes of images shared by people about their daily lives, events, and opinions, often in the form of personal photographs, selfies, or memes (cultural picture or video, generally with a humorous caption, that is spread widely on social media), demonstrate the importance of the content on these platforms (Manikonda and De Choudhury, 2017). In fact, there is existing research that has focused on images in order to identify emotion (Joshi et al., 2011; Abdullah et al., 2015), abusive behaviors (Pang et al., 2015), public health challenges (Kiran et al., 2016), fitness (Weber and Mejova, 2016), and mental health (Manikonda and De Choudhury, 2017) among others.
In this paper, we explore the role of self-disclosure through Instagram posts about T1D. The intersecting nature of the platform, its familiarity with people within the same age range as those diagnosed with T1D, and the role of self-disclosure makes this a valuable area of research to explore. Therefore, we ask:
RQ2: Do, and if so, how, the posts represent self-disclosure?
Sentiment
Social media content generated by people, especially selfie posts (images where people take pictures of themselves by reversing their camera or through a mirror), contain nonverbal and social cues. These cues may include visual information about the person in the picture, such as demographic information or even personal characteristics like their mood or attitude, according to the Social Information Processing (SIP) theory (Walther, 2015). In addition to the self-disclosures discussed in detail above, these types of disclosures have been proven by research to lead to positive evaluations by other users on the platform (Hong et al., 2020). The focus of our study being a health condition, we considered the underlying, non-verbal cues of how people were presenting themselves (or their T1D artifacts) in their pictures—as positive (focus on overcoming, empowering or generally optimistic viewpoints, i.e., happiness, excitement, gratefulness, feeling blessed, strength/confidence, humor/fun), negative (depicting challenges or hardships, i.e., frustration, annoyance, anger, fear), or neutral. Since the purpose of this study is to gain a better understanding of the Instagram landscape of people who post, i.e., share themselves through a T1D lens, and all posts in our dataset represented self-disclosure (as described above). We chose to code for sentiment because it is an important form of self-disclosure that communicates valuable non-verbal information, such as through Instagram posts. To this end, we pose the following research question:
RQ3: (a) What is the overall sentiment of the posts? (b) Does sentiment of the posts predict the number of hashtags, comments, or likes?
Social Support
Social support, especially when managing a T1D, has been found to improve overall psychological well-being and physical function (Murphy et al., 2006). Additionally, research has also found that higher perceptions of social support are correlated with increased feelings of control over one's health condition (Evans and Connis, 1995; Wright, 2002). It is particularly important during the adolescent and teen years during which individuals develop their sense of self (Olsson et al., 2005; Webster et al., 2015). Social support is an overarching term that encompasses five types of support, including: informational, emotional, esteem, networking, and tangible (Cutrona and Suhr, 1992). Informational support is the provisioning of knowledge and resources regarding the illness. This type of support has been led to people feeling more empowered and in control of their condition (Wright, 2002). Emotional support is when another provides a sense of understanding and empathy to an individual. This has been found to be helpful for people who are in a situation that must be adapted to Albrecht and Adelman (1987) and Wright (2002). Esteem support is when the impacted individual is provided messages that can increase their perceptions of self-efficacy around the condition. Networking support allows for the connection between other similar individuals. Last, tangible support is something that is physically provided to the individual (i.e., financial, health care, driving, etc.) (Cutrona and Suhr, 1992).
Social support through the sharing of personal experiences is particularly important for young people with life-long medical conditions. Studies have demonstrated numerous benefits to individuals who connect and engage in discussions regarding their own life experiences (Gonzalez-Morkos et al., 2014; Lindberg et al., 2014). Opportunities to interact with people who experience a similar situation enable the participants to have indirect experiences and obtain useful information, including health information for self-management and better stress-coping strategies (Lindberg et al., 2017).
Recent studies have suggested that technology-mediated peer support interventions can empower the patients' self-management and enhance their adherence to their recommended management regimen by providing emotional support, motivation, and health information for various health conditions (Norman et al., 2007; Preuveneers and Berbers, 2008). Technology-mediated peer support offers a dispersed and diverse population, unhindered by constraints of geography and time (Braithwaite et al., 1999; Walther and Boyd, 2002; Drentea and Moren-Cross, 2005). Research has shown that participation in online support groups can result in improved perceptions of social support, quality of life, and self-efficacy. Other research has demonstrated that online support groups most frequently provide emotional and informational support (Winzelberg, 1997; Braithwaite et al., 1999). Emotional support, when provided by a person with the same condition, has been found to be an important emotional resource especially for coping (Bolger et al., 2000; Wright, 2000; Barrera, 1988).
There have been a variety of studies that demonstrate positive outcomes for adolescents when using a technology-based peer support interventions. This body of research demonstrated that these interventions provide a space for information exchange, reduction of social isolation, improved perceptions of normalcy, and to make friends (Greco et al., 2001; Franklin et al., 2003; Liu et al., 2015; Kramer et al., 2018; Maor, 2020). Specifically in the context of self-disclosure, Lee et al. (2013) found that social support played a full, mediating role between self-disclosure and well-being on social networking sites. Meaning that when people used social media they seem to form strong ties online, leading to high quality friendships and social companionship, which in turn led to improved well-being (Lee et al., 2013).
From specifically a social media perspective, research has shown that Instagram photos have been mobilized to seek as well as provide psychosocial support. Manikonda and De Choudhury (2017) explicate, in the context of mental health issues on Instagram, how individuals with socially stigmatized experiences would look for “sympathetic others” by adopting the visual mode. They also note how people utilize the “photo-sharing affordance of Instagram as a way for emotional release around a distressful experience” (p. 178). This customizable level of anonymity provides for increased self-disclosure that can further elevate the perceptions of emotional support (White and Dorman, 2001).
Social media platforms like Facebook have been identified as an important space for people to construct both personal and group identities. An individual's social media account can be aligned with their own social conceptions of identity, where users send signals in order to establish their identity through interaction with others. Liking content can be viewed as performing identities [as described by Goffman (1959)] in a way that mirrors offline social interaction (Fergie et al., 2016). Additionally, Wohn et al. (2016), defined one-click cues, such as likes or favorite as paralinguistic digital affordances (PDAs). These one-click cues provided meaningful communication from the person liking a post to the poster, without the use of words. More specifically, recipients of likes (or their equivalent depending on the platform) equated them to receiving social support, i.e., getting likes on their posts meant they felt more supported. Since the goal of this formative study was to better understand the environment of T1D on Instagram, specifically exploring self-disclosure and social support, the final research question guiding our study is:
RQ4: Does self-disclosure play a role in social support received?
Materials and Methods
Hashtag Identification
This exploratory study examines how people are using Instagram through self-disclosure and community building within the T1D context. As we were interested in self-disclosure and social support, we first did a general search on Instagram using #diabetes, #type1, and #t1d. After looking through the first 100 post of each, we probed the hashtags most commonly used. We discovered many people using the hashtag “#t1dlookslikeme” (as of January 2020, it has more than 360,000 posts). By skimming through several of these posts and keeping our objectives in mind, we decided to use this hashtag as it includes self-disclosure of their condition and seems to be a positive place for people to come together about living with T1D.
Data Collection
We used Netlytic, a commonly used, free, third-party data collection tool, to gather publicly available posts that used “#t1dlookslikeme.” A total of 3,000 posts were collected from July 19, 2018 through October 1, 2018. Using a random number generator we randomly selected 500 of those posts; this is similar to a number of other studies coding Instagram posts (Santarossa et al., 2016; Allem et al., 2017a; Slater et al., 2017; Ben Taleb et al., 2019). The university's institutional review board (IRB) determined that the study is not human subject research.
Codebook Development
For this quantitative content analysis, the initial codebook development came from reviewing posts on Instagram, additional refinement and revisions was done using a subset of posts from the initial sample (Weber, 1990; Downe-Wamboldt, 1992). The codebook included a variety of other factors, including the appearance of diabetes medical equipment (continuous glucose monitors, insulin pumps, etc.), food, blood sugar ranges, the individual(s) in the picture, if it was a meme, and sentiment of the post. We decided that overall sentiment of the post would be mutually exclusive (i.e., a post could not be both positive and negative so as to capture the overall sentiment a post was trying to communicate). The codebook also included the number of likes, comments, and hashtags. The codebook consisted of up to 43 items on the image (skip logic was used if an item was not present) and 10 codes for the captions. If the posts, contained multiple photos, the coders were instructed to code at the first photo only. If the posts contained a video, the coders were to note if it was more than one situation (e.g., multiple places, multiple times, etc.) and to bring it to the team. Neither coder experienced this, and this was further verified by the lead researcher.
Finally, “self-disclosure” was not coded for directly, but was operationalized by coding for things that posters were, in fact, disclosing (such as T1D artifacts like medication, devices, tattoos, etc.). This was because all the posts in our dataset were all things that people were already self-disclosing. Therefore, coding for presence and absence of self-disclosure was not a valid option. Coding, instead, for the kinds of self-disclosure led to an understanding of the extent of self-disclosure by users. Overall, the measures of our variables like, self-disclosure and social support were achieved through social media data, as opposed to primary, human subjects' data. Because of this we chose alternative forms of operationalization rather than more traditional forms of social media behaviors (like efforts to reach the correct audience, for instance).
Coding Process
We trained two coders (independent, undergraduate students with coding experience) on the codebook. They then independently coded 50 random posts (not the posts used in the final evaluation) to ensure inter-coder reliability. Once the posts were coded, we reviewed the results together and discussed all of the discrepancies. Cohen's Kappa statistic was used to assess the degree of agreement in the coding. We did this for three rounds, until an inter-coder reliability of κ ≥0.9 was achieved. Generally, Kappa values >0.80 are considered “substantial,” however, we wanted to achieve a higher rate of reliability because of the preliminary nature of the research (Landis and Koch, 1977; Salkind, 2012). Each of the coders was then provided 250 posts to code independently. The authors also conducted repeated spot-checks of the coded posts. Disagreements were discussed and resolved by the authors in a team setting.
Results
The 500 posts were manually coded to determine their fit for our study. The coders eliminated posts that were no longer available on Instagram (27 posts); not in English (either the photo and/or the caption) (22 posts), nothing in the picture or caption related to type 1 diabetes (20 posts), or posts that specifically asked for a like (8 posts). Based on these criteria, 77 posts were eliminated, for a total of 423 posts analyzed.
Post Characteristics
Research question 1 asks about the descriptive information regarding the types of posts within the T1D community, namely the types of posts, the types of hashtags, and the presence of devices or management tools in the post.
After elimination, our dataset consisted of 88.9% images vs. 6.4% video clips. Furthermore, 83.5% of the posts were personal images, out of which 25.3% had a device in the image (with 10.9% of devices being on the individual's body), 8.2% showing their face, 21.2% contained something related to diabetes in the image (not necessarily medical, but a T1D artifact such as clothing, a tattoo, candy, etc.), 16.3% had a food or beverage, 11.11% had more than one person in the image, 3.1% had an animal, 6.8% were travel shots, and 7.1% were during a social activity. Additionally, overall, 25.3% of images included management items for T1D. The remaining 11.6% (non-personal posts) were categorized as memes by the coders. Of the videos that were coded, 55% were of physical activity (i.e., lifting weights, exercising, dancing, etc.) and three used the “boomerang” effect (short videos, no audio that loops). No images are included here for privacy of users.
Types of Hashtags
As described in the Methods section, a general search was conducted on Instagram using #diabetes, #type1, and #t1d. While we discovered many people using the hashtag “#t1dlookslikeme,” we still noted the use of other popular hashtags. Overall, our dataset included the several hashtags (excluding #t1dlookslikeme which was in all posts) as can be seen in Table 1.
Presence of Device Management Tools
A quarter (25.29%, N = 107) of the sample posts included management items for T1D. These were items people used to manage their T1D conditions, examples include diabetes blood glucose meters, insulin pumps, syringes, etc. (Table 2).
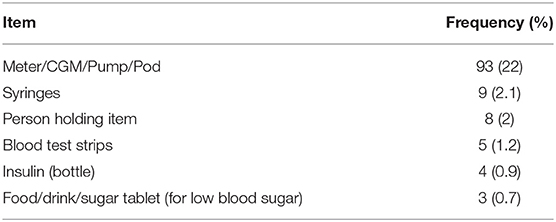
Table 2. Diabetes self-management items in sample posts 107 posts (25.3%) contained devices; multiple devices could be in the same post (N = 423).
Post Sentiment
Each post was classified as either positive or negative in sentiment. Our goal for classification of post by sentiment was to understand the nature of the self-disclosure involved in these posts and if it came from a positive or negative place. The categories for positive sentiment included posts that were happy, excited, grateful, blessed, strong/confident, or funny/humorous. Table 3 provides a breakdown of all the positive and negative sentiment posts. Similarly, the categories for negative sentiment included posts that were frustrated, annoyed, angry, fear/afraid. These results indicate that the sharing carried out by people on Instagram about their T1D was more positive in nature, rather than negative.
Engagement With Posts and Sentiment
Research question 2 asked if the number of hashtags, likes, or comments could predict the sentiment of the post. Of our sample posts, 83.8% contained hashtags (ranging from 0 to 35 per post), 83.1% contained likes (ranging from 8 to 1,612 per post), and 83.8% contained comments (ranging from 0 to 376 per post). To determine the correlation of post engagement (hashtags, likes, and comments) with post sentiment, we ran chi square tests. We found that while the presence of likes and comments were not significant predictors on sentiment, the presence of hashtags was. These results can be found in Table 4.
Self-Disclosure in Posts
Research question 3 asked about how self-disclosure is presented in T1D. To answer this question, we specifically focused on three characteristics of each post, namely (1) whether or not there was a T1D device in the image, (2) the presence of a T1D related artifact (i.e., an item that specifically mentions or is in reference to diabetes, a t-shirt, tattoo, etc.), and (3) whether or not the post contained T1D management items (e.g., blood test strips, syringes, insulin, etc.). These three characteristics were specifically selected because they are representative of people voluntarily disclosing things related to their T1D condition, but not for reasons of information sharing or answering others' questions, like what has been found in traditional online health communities (Kanthawala et al., 2016). However, here we focused specifically on those posts that included content people did not need to share, but instead volunteered it, seemingly in an act of self-disclosure. From our sample, 73.8% contained a T1D device in the image, 53.3% contained a diabetes related artifact, and 22.3% contained T1D management items.
Furthermore, we also conducted a series of chi-square tests to determine if there was a significant effect of various characteristics stated above on the sentiment of each post. As can be seen in Table 5, the presence of a T1D device or a diabetes-related artifact did have significant effects on the sentiment of the posts.
Social Support in Posts
Research question 4 asked about the social support in the posts. Since we did not have any human subjects contact in this initial study, but instead observed real conversations on Instagram, we operationalized social support as exchanged through the likes the posts received [as noted by (Carr et al., 2016; Wohn et al., 2016)]. To determine if self-disclosure actually affected social support, we operationalized self-disclosure through the number of hashtags in each post and social support as the number of likes on the post. We ran a correlation of hashtags with likes and found a significant correlation (r = 0.167, p = 0.001) indicating that a higher self-disclosure was related to a higher amount of social support the post received.
Discussion
The primary goal of this exploratory study was to comprehend the Instagram landscape regarding T1D, specifically the hashtag #t1dlookslikeme. Through this study we were particularly interested in issues of self-disclosure and social support. The findings suggest that people are self-disclosing their condition and receiving support back from the community. The following paragraphs focus on self-disclosure, including self-presentation, and social support. Last, we discuss what we believe are additional links to empowerment that should be considered in future studies.
Our review of Instagram posts around T1D led us to the community hashtag (#t1dlookslikeme) used by people who have T1D, which we subsequently utilized to collect data. Our findings aligned with past work that has identified social media as a space to create identity, by extending it through similar observations on a visual platform like Instagram. Here, individuals who go through life with T1D use images to depict artifacts related to their condition in relation to their everyday lives. We observed artifacts ranging from medical devices to tattoos, depicting how people present their own versions of life with T1D which are forms for self-disclosure.
Oftentimes, health conditions are accompanied with stigma that might prevent people from disclosing them. While the posts that we looked at are simply a sample of individuals who self-select disclosure, it is interesting to note the type of disclosure involved here. Whether this should be attributed to T1D and its community or the fact that this population skews younger, we cannot be certain and hope to explore in future research. However, it is clear that with higher positive sentiments among posts and self-expression and disclosure of medication, medical devices, challenges, and optimism, these individuals are choosing not to stay hidden.
Our research suggests that the more positive sentiment a post has, the more hashtags it has. Hashtags are a meaningful form of engagement that come from the user when posting a picture to Instagram. They are the only form of engagement that come from the poster, as opposed to the person engaging with a post. Further, they could be thought of as a form of network support, in showing others other similar groups or networks. Therefore, hashtags in this dataset depicts an element of self-disclosure.
Our findings suggest that disclosure is related to more positive sentiment within the post. Past literature has demonstrated that in many social contexts, especially those online, people selectively self-present their lives (Ellison et al., 2006), this also includes health and health related behaviors (Catona and Greene, 2016). Perhaps when a person is feeling more negative, they might be seeking more support from a closer, more intimate group of people. But in situations with positive sentiment, there seems to be more sharing and disclosing of their well-being and a depiction of their daily lives. Future research should seek to better understand this relationship.
We also considered the presence of social support through the likes received by posts. Since hashtags are indicative of self-disclosure and likes of social support (Wohn et al., 2016), we conclude that there is a positive relationship between how much an individual self-discloses and what support they receive in return. This finding is important to note, especially because of the importance of social support in self-management of chronic health conditions (like T1D). For this study, we are unable to determine their motivations for disclosure and what social outcomes they received through posting, such as relationship maintenance, social capital, or social support (Ellison et al., 2006, 2007; Bazarova and Choi, 2014; Sosik and Bazarova, 2014). A future study that seeks to understand the motivations underlying people's self-disclosure regarding their diabetes on Instagram could further enhance this research area.
Self-presentation online does not always reflect exactly how the individual is feeling, it is an outlet through which they can feel in control, get social validation, and relieve stress (Bazarova and Choi, 2014). This act of positively disclosing about one's own health condition can be empowering. We, however, cannot be certain about whether the users from our dataset felt empowered. Empowerment has been defined as a multi-dimensional and social process that is used to give power back to the individual, group, or society (Page and Czuba, 1999). In fact, we were surprised at how many of the #t1dlookslikeme posts could be classified as depicting a way the posters were feeling empowered. In this context, individuals are posting and seeking to take the “power” away from their T1D and giving it back to themselves. For example, they may live with T1D, but they do not live for T1D. Posting these images on Instagram is disclosing information which could be considered a stigma in a young person's peer group and gives them the power to share with others like themselves. Through this sharing a community of social support is formed and research has shown that this type of sharing enhances group cohesion (White and Danis, 2013). The authors could not find previous work that examined the use of Instagram for empowerment for people with T1D. Thus, the next step will be designed to study this phenomenon.
This study was an early investigation into the Instagram environment of how people with T1D self-present. To the authors' knowledge this is one of the first papers to explore chronic illnesses on Instagram. We hope that this can help provide other researchers with further research questions to explore this area. Armed with this knowledge, we are better able to use theory to guide the research and make meaningful predictions that could be useful to health care providers, educators, and caregiving family members.
Limitations
The sampled posts were selected using a random number generator. It is possible that this might not have captured enough posts to represent the entire population of #t1dlookslikeme Instagram posts. Additionally, this study was limited to those posts that were publicly available, which may have limited the number of posts that had a negative salience or that were of a more personal nature. Also, while we had several training sessions for the coders and reached a high intercoder reliability, there could have been information that was coded incorrectly. Last, as the authors both developed the codebook and trained the coders and there were no external reviewers, which could have introduced some bias in the coding.
Posting personal pictures on Instagram is a popular way for teens and young adults to express themselves online. Sometimes this expression can have negative consequences especially when considering body image, smoking/using tobacco or marijuana products, and self-harm. However, Instagram can also be a place to connect with similar individuals and to boost positive feelings. Therefore, this exploratory study sought to better understand the self-disclosure of T1D status of individuals on Instagram using #t1dlookslikeme. This study can guide a more thorough examination of content on Instagram, to help us better understand the potentially positive role of self-disclosure in disease management in future studies.
Data Availability Statement
The datasets generated for this study are available on request to the corresponding author.
Author Contributions
BH and SK contributed to the design and implementation of the research, to the analysis of the results, and to the writing of the manuscript. All authors contributed to the article and approved the submitted version.
Funding
BH currently has funding from the American Diabetes Association, Translational Research, Grant No. 1-16-ICTS-045.
Conflict of Interest
The authors declare that the research was conducted in the absence of any commercial or financial relationships that could be construed as a potential conflict of interest.
References
Abdullah, S., Murnane, E. L., Costa, J. M. R., and Choudhury, T. (2015). “Collective smile: Measuring societal happiness from geolocated images,” in CSCW 2015 - Proceedings of the 2015 ACM International Conference on Computer-Supported Cooperative Work and Social Computing (Vancouver, BC), 361–374.
Albrecht, T. L., and Adelman, M. B. (1987). Communicating Social Support. Thousand Oaks, CA: Sage Publications, Inc.
Allem, J. P., Chu, K. H., Cruz, T. B., and Unger, J. B. (2017a). Waterpipe promotion and use on instagram: #Hookah. Nicotine Tobacco Res. 19, 1248–1252. doi: 10.1093/ntr/ntw329
Allem, J. P., Escobedo, P., Chu, K. H., Cruz, T. B., and Unger, J. B. (2017b). Images of little cigars and cigarillos on instagram identified by the Hashtag #Swisher: thematic analysis. J. Med. Internet Res. 19:e255. doi: 10.2196/jmir.7634
American Diabetes Association (2015). Statistics About Diabetes. Retrieved from National Diabetes Statistics Report. Avaliable online at: http://www.diabetes.org/diabetes-basics/statistics/ (accessed August 27, 2019).
Andalibi, N., Ozturk, P., and Forte, A. (2017). “Sensitive self-disclosures, responses, and social support on instagram,” in Proceedings of the 2017 ACM Conference on Computer Supported Cooperative Work and Social Computing, (CSCW) (Portland, OR), 1485–1500.
Anderson, M., and Jiang, J. (2018). Teens, Social Media & Technology 2018 | Pew Research Center. Avaliable online at: www.pewresearch.org/internet/2018/05/31/teens-social-media-technology-2018/ (accessed January 15, 2020).
Balani, S., and De Choudhury, M. (2015). “Detecting and characterizing mental health related self-disclosure in social media,” in Conference on Human Factors in Computing Systems–Proceedings (Seoul), 1373–1378.
Barak, A., Boniel-Nissim, M., and Suler, J. (2008). Fostering empowerment in online support groups. Comput. Hum. Behav. 24, 1867–1883. doi: 10.1016/j.chb.2008.02.004
Bazarova, N. N., and Choi, Y. H. (2014). Self-disclosure in social media: extending the functional approach to disclosure motivations and characteristics on social network sites. J. Commun. 64, 635–657. doi: 10.1111/jcom.12106
Ben Taleb, Z., Laestadius, L. I., Asfar, T., Primack, B. A., and Maziak, W. (2019). #Hookahlife: the rise of waterpipe promotion on instagram. Health Educ. Behav. 46, 106–113. doi: 10.1177/1090198118779131
Beyond Type 1 (2019). Type 1 Diabetes Statistics. Available online at: https://beyondtype1.org/type-1-diabetes-statistics/ (accessed July 30, 2020).
Bolger, N., Zuckerman, A., and Kessler, R. C. (2000). Invisible support and adjustment to stress. J. Pers. Social Psychol. 79:953–961. doi: 10.1037//0022-3514.79.6.953
Boudewyns, V., Himelboim, I., Hansen, D. L., and Southwell, B. G. (2015). Stigma's effect on social interaction and social media activity. J. Health Commun. 20, 1337–1345. doi: 10.1080/10810730.2015.1018604
Braithwaite, D. O., Waldron, V. R., and Finn, J. (1999). Communication of social support in computer-mediated groups for people with disabilities. Health Commun. 11, 123–151. doi: 10.1207/s15327027hc1102_2
Carr, C. T., Wohn, D. Y., and Hayes, R. A. (2016). As social support: relational closeness, automaticity, and interpreting social support from paralinguistic digital affordances in social media. Comput. Hum. Behav. 62, 385–393. doi: 10.1016/j.chb.2016.03.087
Catona, D., and Greene, K. (2016). “The international encyclopedia of interpersonal communication,” in Edited Self-Disclosure, 1st Edn. eds C. R. Berger, and M. E. Roloff (Wiley), 1–5.
Centers for Disease Control (1991). The Prevention and Treatment of Complications of Diabetes. Avaliable online at: http://wonder.cdc.gov/wonder/prevguid/p0000063/p0000063.asp (accessed January 15, 2020).
Chang, P. F., and Bazarova, N. N. (2016). Managing stigma: disclosure-response communication patterns in pro-anorexic websites. Health Commun. 31, 217–229. doi: 10.1080/10410236.2014.946218
Chu, K. H., Allem, J. P., Cruz, T. B., and Unger, J. B. (2017). Vaping on instagram: cloud chasing, hand checks and product placement. Tobacco Control 26, 575–578. doi: 10.1136/tobaccocontrol-2016-053052
Cohen, S., and Wills, T. A. (1985). Stress, social support, and the buffering hypothesis. Psychol. Bull. 98, 310–357. doi: 10.1037/0033-2909.98.2.310
Cutrona, C. E., and Suhr, J. A. (1992). Controllability of stressful events and satisfaction with spouse support behaviors. Commun. Res. 19, 154–174. doi: 10.1177/009365092019002002
De Choudhury, M., and De, S. (2014). “Mental health discourse on reddit: self-disclosure, social support, and anonymity,” in Proceedings of the 8th International Conference on Weblogs and Social Media (ICWSM) (Ann Arbor, MI), 71–80.
Derlega, V. J., Metts, S., Petronio, S., and Margulis, S. T. (1993). “Developing close relationships,” in Self Disclosure (Newbury Park, CA: SAGE Publications Inc.), 8–42.
Downe-Wamboldt, B. (1992). Content analysis: method, applications, and issues. Health Care Women Int. 13, 313–321. doi: 10.1080/07399339209516006
Drentea, P., and Moren-Cross, J. L. (2005). Social capital and social support on the web: the case of an internet mother site. Sociol. Health Illness 27, 920–943. doi: 10.1111/j.1467-9566.2005.00464.x
Efron, M., and Winget, M. (2010). “Questions are content: a taxonomy of questions in a microblogging environment,” in Proceedings of the ASIST Annual Meeting (Pittsburgh, PA), 47.
Ellison, N., Heino, R., and Gibbs, J. (2006). Managing impressions online: self-presentation processes in the online dating environment. J. Comput-Med. Commun. 11, 415–441. doi: 10.1111/j.1083-6101.2006.00020.x
Ellison, N. B., Steinfield, C., and Lampe, C. (2007). The benefits of facebook “friends:” social capital and college students' use of online social network sites. J. Comput-Med. Commun. 12, 1143–1168. doi: 10.1111/j.1083-6101.2007.00367.x
Evans, R. L., and Connis, R. T. (1995). Comparison of brief group therapies for depressed cancer patients receiving radiation treatment. Public Health Rep. 110:306.
Eysenbach, G., and Köhler, C. (2002). How do consumers search for and appraise health information on the world wide web? qualitative study using focus groups, usability tests, and in-depth interviews. BMJ 324, 573–577. doi: 10.1136/bmj.324.7337.573
Fergie, G., Hunt, K., and Hilton, S. (2016). Social media as a space for support: young adults' perspectives on producing and consuming user-generated content about diabetes and mental health. Soc. Sci. Med. 170, 46–54. doi: 10.1016/j.socscimed.2016.10.006
Figueiredo, M. I., Fries, E., and Ingram, K. M. (2004). The role of disclosure patterns and unsupportive social interactions in the well-being of breast cancer patients. Psycho-Oncol. 13, 96–105. doi: 10.1002/pon.717
Fox, S. (2011). Peer-to-Peer Healthcare. Pew Research Center's Internet & American Life Project, 1–26.
Franklin, V., Waller, A., Pagliari, C., and Greene, S. (2003). “Sweet Talk”: text messaging support for intensive insulin therapy for young people with diabetes. Diabetes Technol. Therap. 5, 991–996. doi: 10.1089/152091503322641042
Giannoulakis, S., and Tsapatsoulis, N. (2016). Evaluating the descriptive power of Instagram hashtags. J. Innov. Digital Ecosyst. 3, 114–129. doi: 10.1016/j.jides.2016.10.001
Gonzalez-Morkos, B., Zavala, O., Malogolowkin, M., and Kuperberg, A. (2014). The teen impact experience: a webcast pilot project for teens with cancer and blood diseases. J. Pediatr. Oncol. Nurs. 31, 272–276. doi: 10.1177/1043454214529369
Greco, P., Pendley, J. S., McDonell, K., and Reeves, G. (2001). A peer group intervention for adolescents with type 1 diabetes and their best friends. J. Pediatric Psychol. 26, 485–490. doi: 10.1093/jpepsy/26.8.485
Greene, J. A., Choudhry, N. K., Kilabuk, E., and Shrank, W. H. (2011). Online social networking by patients with diabetes: a qualitative evaluation of communication with facebook. J. Gen. Intern. Med. 26, 287–292. doi: 10.1007/s11606-010-1526-3
Greene, K., Derlega, V. J., and Mathews, A. (2006). “Self-disclosure in personal relationships,” in The Cambridge Handbook of Personal Relationships (Cambridge, UK: Cambridge University Press), 409–427.
Hong, S., Jahng, M. R., Lee, N., and Wise, K. R. (2020). Do you filter who you are? Excessive self-presentation, social cues, and user evaluations of instagram selfies. Comput. Hum. Behav. 104:106159. doi: 10.1016/j.chb.2019.106159
Huh, J., Marmor, R., and Jiang, X. (2016). Lessons learned for online health community moderator roles: a mixed-methods study of moderators resigning from WebMD communities. J. Med. Internet Res. 18:e247. doi: 10.2196/jmir.6331
Huh, J., McDonald, D. W., Hartzler, A., and Pratt, W. (2013). “Patient moderator interaction in online health communities,” in AMIA Annual Symposium Proceedings/AMIA Symposium. (AMIA Symposium), 627–636.
Instagram. (n.d.). How do I Use Hashtags on Instagram? Avaliable online at: https://help.instagram.com/351460621611097
Jin, J., Yan, X., Li, Y., and Li, Y. (2016). How users adopt healthcare information: an empirical study of an online Q&A community. Int. J. Med. Inform. 86, 91–103. doi: 10.1016/j.ijmedinf.2015.11.002
Jin, S. V., Phua, J., and Lee, K. M. (2015). Telling stories about breastfeeding through facebook: the impact of user-generated content (UGC) on pro-breastfeeding attitudes. Comput. Hum. Behav. 46, 6–17. doi: 10.1016/j.chb.2014.12.046
Joshi, D., Datta, R., Fedorovskaya, E., Luong, Q. T., Wang, J. Z., Li, J., et al. (2011). Aesthetics and emotions in images. IEEE Signal Process. Mag. 28, 94–115. doi: 10.1109/MSP.2011.941851
Kanthawala, S., Vermeesch, A., Given, B., and Huh, J. (2016). Answers to health questions: Internet search results versus online health community responses. J. Med. Internet Res. 18:e95. doi: 10.2196/jmir.5369
Kiran, V. R., Alfayad, A., and Weber, I. (2016). “Social media image analysis for public health,” in Conference on Human Factors in Computing Systems - Proceedings (San Jose, CA), 5543–5547.
Kramer, J. M., Ryan, C. T., Moore, R., and Schwartz, A. (2018). Feasibility of electronic peer mentoring for transition-age youth and young adults with intellectual and developmental disabilities: project teens making environment and activity modifications. J. Appl. Res. Intell. Disabil. 31, e118–29. doi: 10.1111/jar.12346
Laestadius, L. I., Wahl, M. M., and Cho, Y. I. (2016). #Vapelife: an exploratory study of electronic cigarette use and promotion on instagram. Subst. Use Misuse 51, 1669–1673. doi: 10.1080/10826084.2016.1188958
Landis, J. R., and Koch, G. G. (1977). An application of hierarchical kappa-type statistics in the assessment of majority agreement among multiple observers. Biometric 33, 363–374. doi: 10.2307/2529786
Lee, K. T., Noh, M. J., and Koo, D. M. (2013). Lonely people are no longer lonely on social networking sites: the mediating role of self-disclosure and social support. Cyberpsychol. Behav, Soc. Netw. 16, 413–418. doi: 10.1089/cyber.2012.0553
Lindberg, S., Svedberg, P., Bergquist, M., and Nygren, J. M. (2017). Evaluating digital peer support for children cured from cancer. Int. J. Hum-Comput. Interact. 33, 664–676. doi: 10.1080/10447318.2017.1278892
Lindberg, S., Wärnestål, P., Nygren, J., and Svedberg, P. (2014). “Designing digital peer support for children,” in Proceedings of the 2014 Conference on Interaction Design and Children - IDC '14 (Aarhus), 47–56.
Link, B. G., and Phelan, J. C. (2001). Conceptualizing stigma. Annu. Rev. Sociol. 27, 363–385. doi: 10.1146/annurev.soc.27.1.363
Link, B. G., and Phelan, J. C. (2006). Stigma and its public health implications. Lancet 367, 528–529. doi: 10.1016/S0140-6736(06)68184-1
Liu, L. S., Inkpen, K. M., and Pratt, W. (2015). ““I'm not like my friends”: understanding how children with a chronic illness use technology to maintain normalcy,” in Proceedings of the 18th ACM Conference on Computer Supported Cooperative Work & Social Computing - CSCW'15 (Vancouver, BC). doi: 10.1145/2675133.2675201
Major, B., and O'Brien, L. T. (2005). The social psychology of stigma. Annu. Rev. Psychol. 56, 393–421. doi: 10.1146/annurev.psych.56.091103.070137
Manikonda, L., and De Choudhury, M. (2017). “Modeling and understanding visual attributes of mental health disclosures in social media,” in Conference on Human Factors in Computing Systems - Proceedings (Denver, CO), 170–181.
Maor, D., and Mitchem, K. (2020). Hospitalized adolescents' use of mobile technologies for learning, communication, and Well-being. J. Adolesc. Res. 35, 225–247. doi: 10.1177/0743558417753953
Masaviru, M. (2016). Self-disclosure: theories and model review. J. Culture Soc. Dev. 18, 43–47. Available online at: https://iiste.org/Journals/index.php/JCSD/article/view/30022
Meisenbach, R. J. (2010). Stigma management communication: a theory and agenda for apdplied research on how individuals manage moments of stigmatized identity. J. Appl. Commun. Res. 38, 268–292. doi: 10.1080/00909882.2010.490841
Morgan, E. M., Snelson, C., and Elison-Bowers, P. (2010). Image and video disclosure of substance use on social media websites. Comput. Hum. Behav. 26, 1405–1411. doi: 10.1016/j.chb.2010.04.017
Murphy, H. R., Rayman, G., and Skinner, T. C. (2006). Psycho-educational interventions for children and young people with Type 1 diabetes. Diab. Med. 23, 935–943. doi: 10.1111/j.1464-5491.2006.01816.x
Myrick, J. G., Holton, A. E., Himelboim, I., and Love, B. (2015). #Stupidcancer: exploring a typology of social support and the role of emotional expression in a social media community. Health Commun. 31, 1–10. doi: 10.1080/10410236.2014.981664
Nambisan, P. (2011). Information seeking and social support in online health communities: Impact on patients' perceived empathy. J. Am. Med. Inform. Assoc. 18, 298–304. doi: 10.1136/amiajnl-2010-000058
Norman, G. J., Zabinski, M. F., Adams, M. A., Rosenberg, D. E., Yaroch, A. L., and Atienza, A. A. (2007). A review of eHealth interventions for physical activity and dietary behavior change. Am. J. Prev. Med. 33, 336–345. doi: 10.1016/j.amepre.2007.05.007
Olsson, C. A., Boyce, M. F., Toumbourou, J. W., and Sawyer, S. M. (2005). The role of peer support in facilitating psychosocial adjustment to chronic illness in adolescence. Clin. Child Psychol. Psychiatry 10, 78–87. doi: 10.1177/1359104505048793
Pang, R., Baretto, A., Kautz, H., and Luo, J. (2015). “Monitoring adolescent alcohol use via multimodal analysis in social multimedia,” in Proceedings - 2015 IEEE International Conference on Big Data (IEEE Big Data) (Santa Clara, CA), 1509–1518.
Pennebaker, J. W., and O'Heeron, R. C. (1984). Confiding in others and illness rate among spouses of suicide and accidental-death victims. J. Abnorm. Psychol. 93, 473–476. doi: 10.1037/0021-843X.93.4.473
Perez, S. L., Paterniti, D. A., Wilson, M., Bell, R. A., Chan, M. S., Villareal, C. C., et al. (2015). Characterizing the processes for navigating internet health information using real-time observations: a mixed-methods approach. J. Med. Internet Res. 17:e173. doi: 10.2196/jmir.3945
Perrin, A., and Anderson, M. (2019). Social Media Usage in the U.S. in 2019 | Pew Research Center. Avaliable online at: https://www.pewresearch.org/fact-tank/2019/04/10/share-of-u-s-adults-using-social-media-including-facebook-is-mostly-unchanged-since-2018/ (accessed January 15, 2020).
Preuveneers, D., and Berbers, Y. (2008). “Mobile phones assisting with health self-care,” in Proceedings of the 10th International Conference on Human Computer Interaction with Mobile Devices and Services - MobileHCI '08 (Amsterdam), 177.
Rains, S. A. (2014). The implications of stigma and anonymity for self-disclosure in health blogs. Health Commun. 29, 23–31. doi: 10.1080/10410236.2012.714861
Rains, S. A., and Keating, D. M. (2011). The Social dimension of blogging about health: health blogging, social support, and well-being. Commun. Monograp. 78, 511–534. doi: 10.1080/03637751.2011.618142
Roberts, K., and Demner-Fushman, D. (2016). Interactive use of online health resources: a comparison of consumer and professional questions. J. Am. Med. Inform. Assoc. 23, 802–811. doi: 10.1093/jamia/ocw024
Santarossa, S., Coyne, P., Lisinski, C., and Woodruff, S. J. (2016). #fitspo on Instagram: a mixed-methods approach using netlytic and photo analysis, uncovering the online discussion and author/image characteristics. J. Health Psychol. 24, 376–385. doi: 10.1177/1359105316676334
Santuzzi, A. M., Waltz, P. R., Finkelstein, L. M., and Rupp, D. E. (2014). Invisible disabilities: Unique challenges for employees and organizations. Ind. Organization. Psychol. 7, 204–219. doi: 10.1111/iops.12134
Sharma, S. S., and De Choudhury, M. (2015). “Measuring and characterizing nutritional information of food and ingestion content in instagram,” in WWW 2015 Companion - Proceedings of the 24th International Conference on World Wide Web (Florence), 115–116. doi: 10.1145/2740908.2742754
Shaw, R. J., and Johnson, C. M. (2011). Health information seeking and social media use on the internet among people with diabetes. Online J. Public Health Inform. 3, 1–9. doi: 10.5210/ojphi.v3i1.3561
Slater, A., Varsani, N., and Diedrichs, P. C. (2017). #fitspo or #loveyourself? The impact of fitspiration and self-compassion Instagram images on women's body image, self-compassion, and mood. Body Image 22, 87–96. doi: 10.1016/j.bodyim.2017.06.004
Sosik, V. S., and Bazarova, N. N. (2014). Relational maintenance on social network sites: how facebook communication predicts relational escalation. Comput. Hum. Behav. 35, 124–131. doi: 10.1016/j.chb.2014.02.044
Tiggemann, M., and Zaccardo, M. (2018). ‘Strong is the new skinny’: a content analysis of #fitspiration images on instagram. J. Health Psychol. 23, 1003–1011. doi: 10.1177/1359105316639436
Walther, J. B. (2015). “Social information processing theory (CMC),” in The International Encyclopedia of Interpersonal Communication, eds C.R. Berger, M.E. Roloff, S.R. Wilson, J.P. Dillard, J. Caughlin, and D. Solomon. doi: 10.1002/9781118540190.wbeic192
Walther, J. B., and Boyd, S. (2002). “Attraction to computer-mediated social support,” in Communication Technology and Audience Adoption and Uses, eds C. A. Lin and D. Atkins (Cresskill, NJ: Hampton Press), 153–188.
We Are Social, Hootsuite, and DataReportal. (2019). Most Popular Social Networks Worldwide as of January 2019, Ranked by Number of Active Users (in Millions). Available online at: https://wearesocial.com/global-digital-report-2019 (accessed January 15, 2020).
Weber, I., and Mejova, Y. (2016). “Crowdsourcing health labels,” in DH '16: Proceedings of the 6th International Conference on Digital Health Conference (New York, NY: Association for Computing Machinery), 105–109. doi: 10.1145/2896338.2897727
Webster, M., Foster, E., Comber, R., Bowen, S., Cheetham, T., and Balaam, M. (2015). “Understanding the lived experience of adolescents with type 1 diabetes,” in Proceedings of the 14th International Conference on Interaction Design and Children - IDC '15 (Medford, MA). doi: 10.1145/2771839.2771854
White, A., and Danis, M. (2013). Enhancing patient-centered communication and collaboration by using the electronic health record in the examination room. JAMA 309, 2327–2328. doi: 10.1001/jama.2013.6030
White, M., and Dorman, S. M. (2001). Receiving social support online: implications for health education. Health Educ. Res. 16, 693–707. doi: 10.1093/her/16.6.693
Willis, E., and Royne, M. B. (2017). Online health communities and chronic disease self-management. Health Commun. 32, 269–278. doi: 10.1080/10410236.2016.1138278
Winzelberg, A. (1997). The analysis of an electronic support group for individuals with eating disorders. Comput. Hum. Behav. 13, 393–407. doi: 10.1016/S0747-5632(97)00016-2
Wohn, D. Y., Carr, C. T., and Hayes, R. A. (2016). How affective is a like? The effect of paralinguistic digital affordances on perceived social support. Cyberpsychol. Behav. Soc. Netw. 19, 562–566. doi: 10.1089/cyber.2016.0162
Wright, K. (2002). Social support within an on-line cancer community: an assessment of emotional support, perceptions of advantages and disadvantages, and motives for using the community from a communication perspective. J. Appl. Commun. Res. 30, 195–209. doi: 10.1080/00909880216586
Wright, K. B. (2000). Social support satisfaction, on-line communication apprehension, and perceived life stress within computer-mediated support groups. Commun. Res. Rep. 17, 139–147. doi: 10.1080/08824090009388760
Keywords: instagram, content analysis, type 1 diabetes (T1D), self-disclosure, social support
Citation: Holtz BE and Kanthawala S (2020) #T1DLooksLikeMe: Exploring Self-Disclosure, Social Support, and Type 1 Diabetes on Instagram. Front. Commun. 5:510278. doi: 10.3389/fcomm.2020.510278
Received: 05 November 2019; Accepted: 02 September 2020;
Published: 14 October 2020.
Edited by:
Danielle E. Jake-Schoffman, University of Florida, United StatesReviewed by:
Pamara Chang, University of Cincinnati, United StatesDanielle Arigo, Rowan University, United States
Copyright © 2020 Holtz and Kanthawala. This is an open-access article distributed under the terms of the Creative Commons Attribution License (CC BY). The use, distribution or reproduction in other forums is permitted, provided the original author(s) and the copyright owner(s) are credited and that the original publication in this journal is cited, in accordance with accepted academic practice. No use, distribution or reproduction is permitted which does not comply with these terms.
*Correspondence: Bree E. Holtz, YmhvbHR6QG1zdS5lZHU=