- Phonetics Laboratory, Linguistics Department, University of California, Davis, CA, United States
Speech alignment is where talkers subconsciously adopt the speech and language patterns of their interlocutor. Nowadays, people of all ages are speaking with voice-activated, artificially-intelligent (voice-AI) digital assistants through phones or smart speakers. This study examines participants’ age (older adults, 53–81 years old vs. younger adults, 18–39 years old) and gender (female and male) on degree of speech alignment during shadowing of (female and male) human and voice-AI (Apple’s Siri) productions. Degree of alignment was assessed holistically via a perceptual ratings AXB task by a separate group of listeners. Results reveal that older and younger adults display distinct patterns of alignment based on humanness and gender of the human model talkers: older adults displayed greater alignment toward the female human and device voices, while younger adults aligned to a greater extent toward the male human voice. Additionally, there were other gender-mediated differences observed, all of which interacted with model talker category (voice-AI vs. human) or shadower age category (OA vs. YA). Taken together, these results suggest a complex interplay of social dynamics in alignment, which can inform models of speech production both in human-human and human-device interaction.
Introduction
Speech is now a common mode for interfacing with technology; people of all ages regularly talk to voice-activated artificially intelligent (voice-AI) devices, such as Apple’s Siri and Amazon’s Alexa (Bentley et al., 2018). Across the world, millions of voice-AI devices are being used in people’s homes (Ammari et al., 2019) and almost half of Americans report using a digital assistant (Olmstead, 2017). In some ways, these systems exhibit more human-like qualities: conveyed by their names (e.g., “Alexa”), speech patterns, and personas. Also, many voice-AI systems display an apparent gender. Gender is an indexical variable in human speech that has been found to shape the communicative behavior of interacting speakers (Eckert and McConnell-Ginet, 1992). Do men and women communicate differently with voice-AI systems? Gender also has been shown to influence listeners’ assessment of the persuasiveness, attractiveness, and group membership of the speaker (Oksenberg et al., 1986; DePaulo, 1992; Babel, 2012). How do people respond to apparent gender in a voice-AI system? Theoretical accounts of computer personification, such as the Computers Are Social Actors framework (CASA) (Nass et al., 1994; Nass et al., 1997), propose that when a person detects a sense of humanness in a digital system, they automatically apply socially-mediated behavior from human-human interaction to their interactions with technology. In the case of modern voice-AI assistants, their apparent gender can be seen as one of their many cues of humanity, along with real-time speech recognition, human-like speech patterns, and other features. Some work has examined gender stereotyping of predominantly apparent-female voice-AI assistants (Piper, 2016; Hwang et al., 2019). Only a handful of studies have tested whether speakers display different gender-mediated behaviors toward voice-AI, such as for (apparent) male and female text-to-speech (TTS) voices. While Habler et al. (2019) found no differences in participants’ ratings of male and female TTS voices, other studies examining participants’ speech behavior suggest there are some differences. For example, participants show different speech patterns toward male and female Apple Siri TTS voices, in similar directions as for real human male and female voices (Cohn et al., 2019; Snyder et al., 2019). This suggests that more subconscious behavior may reveal gender-mediated patterns (if present) in human-device interactions. Yet, in all three of these studies (Cohn et al., 2019; Habler et al., 2019; Snyder et al., 2019), only a young adult population (e.g., college-age students) was examined. How might gender-mediated patterns emerge across different age groups? Critically, no prior work, to our knowledge, has investigated whether individuals varying in age and gender differentially apply behaviors toward these systems (with female and male voices), making a direct comparison to human interlocutors.
The current study was designed to investigate two primary questions: 1) Are socially mediated speech patterns (here, gender) from human-human interaction mirrored in human-voice AI interaction? 2) Do the same patterns hold across talkers’ age categories? To answer these questions, we examine a subconscious behavior in vocal interaction: phonetic alignment (also known as entrainment). When people talk to one another—or even hear voices over headphones—they show a tendency to subconsciously align their speech patterns (Natale, 1975; Gregory and Hoyt, 1982; Goldinger, 1998; Shockley et al., 2004; Babel and Bulatov, 2012; Zając, 2013; Zellou et al., 2016; Sonderegger et al., 2017; Zellou et al., 2017). Evidence for speech imitation toward voice technology has also been observed: people align toward the speaking rate (Bell, 2003) and amplitude (Suzuki and Katagiri, 2007) of synthetic computer voices. While some have proposed that imitation is an automatic process that reflects a tight, unmediated coupling of the linguistic representations used for production and perception (Goldinger, 1998; Shockley et al., 2004), there is growing evidence that it is also socially mediated. For example, speakers show different patterns of imitation based on social characteristics of their interlocutors: such as gender (e.g., Namy et al., 2002), attractiveness (e.g., Babel, 2012), likeability (Chartrand and Bargh, 1996), and race/ethnicity (e.g., Babel, 2012). Socially-mediated imitation patterns are often interpreted through the lens of Communication Accommodation Theory (CAT) (Giles et al., 1991; Shepard, 2001), which proposes that speakers use linguistic alignment to emphasize or minimize social differences between themselves and their interlocutors. The CAT framework can also be applied to understand human-device interaction: recent studies that make a direct comparison between human and voice-AI interlocutors found greater vocal imitation for the human, relative to the voice-AI speaker (e.g., Apple’s Siri in Cohn et al., 2019; Snyder et al., 2019; Amazon’s Alexa in Raveh et al., 2019; Zellou and Cohn, 2020). Less speech alignment toward digital device assistants suggests that people may be less inclined to demonstrate social closeness toward voice-AI, as they do for humans.
In the following sections, we consider the role of voice gender (Role of Voice Gender), speaker gender (Role of Participant Gender), and speaker age (Role of Participant Age) as potential variables shaping differences in alignment toward human and voice-AI talkers.
Role of Voice Gender
As previously mentioned, the (apparent) gender of a given voice has been shown to mediate imitation patterns—both in human-human and human-device interaction. In a study of phonetic imitation between college roommates, Pardo et al. (2012) found stronger alignment toward males (relative to females). The same asymmetry has been observed in studies directly comparing human and TTS voices: greater alignment toward male voices (both human and TTS) than female voices (Cohn et al., 2019; Snyder et al., 2019). Recently, a study comparing alignment toward identical TTS voices across three device platforms (varying in human-like embodiment) also showed this pattern (greater alignment toward the male voices, relative to female voices) (Cohn et al., 2020). Yet, others have found conflicting findings for gender-mediated patterns during speech alignment (e.g., Pardo et al., 2017), suggesting that other factors (e.g., participant gender, attitude toward the voice, etc.) might be at play. While we do not ascertain the sources of these gender-based differences in the present study, there are theoretical proposals that gender-differentiated patterns of communication are learned (Eckert and McConnell-Ginet, 1992).
Role of Participant Gender
Imitator gender has also been shown to be an important mediating factor for speech alignment in human-human interaction (Namy et al., 2002; Pardo, 2006; Pardo et al., 2010). For example, Babel (2012) found that, for a white male model talker, the more attractive he was rated, the more his vowels were imitated by the female participants; however, male participants showed a reverse pattern and imitated the white male less when they rated him as more attractive. Namy et al. (2002) found that female shadowers aligned more toward male model talkers than toward female model talkers, while male shadowers aligned similarly toward both genders. Meanwhile, other studies find the opposite pattern (e.g., Pardo, 2006). One possibility for why findings for imitator gender on alignment vary is that since socially-mediated alignment reflects that men and women are “differentially socialized” in the ways that they communicate, and perform in psychological studies, this will vary across contexts, time, and situations in which experiments are being conducted (Namy et al., 2002). Thus, further identifying the factors that interact with gender to predict phonetic imitation patterns is one motivation for the current study and can inform social-theoretical accounts of alignment.
Gender and Technology
Understanding differences in how females and males communicate with voice-AI can reflect how speech patterns are transferred from human-human communication to linguistic interactions with technological agents. Indeed, there is much interest in understanding the role of gender in behavioral patterns during human-computer interaction (e.g., Abel et al., 2020). Recent work has begun to explore gender-differences in attitudes and perceptions toward technological agents and, in particular, during human-robot interaction (see Nomura (2017) for survey). For one, there is work suggesting that gender-differentiated linguistic patterns present in human-human interaction are mirrored in computer-mediated interaction; for example, the differences in politeness patterns, assertiveness, and use of profanity that are found between men and women in face-to-face conversations are also present when using language through a computer (e.g., Herring, 1996; Herring, 2003). More recent work has begun to explore gender-differences in attitudes and perceptions toward technological agents and, in particular, during human-robot interaction (Nomura, 2017). For example, Nomura et al. (2006) had participants complete the Negative Attitudes toward Robots Survey (NARS) and found that females reported more negative attitudes toward interactive situations with robots than male participants. If this holds true for voice-AI interaction as well, we expect greater alignment toward device voices by male participants than female participants in the present study. At the same time, recent work examining human-robot interaction has also revealed gender differences in vocal imitation: female speakers vocally aligned more strongly to female-voiced AI devices that were more human-like (from a cylindrical speaker to a humanoid robot) (Cohn et al., 2020). Following CAT, that speakers use alignment to convey social closeness, and CASA, that people apply social behaviors from human-human interaction to those with robots, we can pose an alternative hypothesis. For example, another possible outcome is greater alignment toward device voices matching in the gender of the shadower (i.e., male shadowers align more toward male device voices and female shadowers align more toward female device voices).
Role of Participant Age
An underexplored area is the effect of a shadower’s age category on speech alignment toward human and voice-AI interlocutors. The vast majority of alignment studies have been conducted on college-age students (e.g., Babel, 2012; Pardo et al., 2012; Walker and Campbell-Kibler, 2015, etc.). To our knowledge, only three studies have examined alignment beyond a cohort of college-aged participants, and only two of which have examined speech imitation: Nielsen (2014) found that children showed stronger vocal alignment relative to young adults. Szabó (2019) examined speech alignment of older adults (OAs) and younger adults (YAs) in the Switchboard corpus of phone conversations. Both age groups showed a willingness to accommodate; OAs and YAs align in phonetically selective ways, yet, they did not compare differences in patterns of alignment toward a single set of model talkers. Giles et al. (1992) examined discourse alignment, not phonetic alignment, but they found that YAs (ages 30–40) accommodated toward OAs (aged 70–87). Older adults, meanwhile, displayed strong under-accommodation, moving away from younger adult interlocutors. The lack of accommodation in discourse topics by older individuals was interpreted as a way to “garner social control of the conversation” (Giles et al., 1992: 285). Taken together, these three studies suggest that age is a relevant social variable in alignment in human-human interaction. The current study additionally tests whether age is a factor mediating imitation of voice-AI systems, comparing YAs and OAs.
Based on Age Differences With Technology
Differences in experience with, and beliefs about, technology across OAs and YAs can lead to different predictions about age-related variation in alignment toward modern voice-AI (e.g., Apple’s Siri) and human model talkers. OAs have different experiences with technology (Harrison and Rainer, 1992; Ezer et al., 2009) and previous exposure to less human-like TTS synthesized voices (e.g., Klatt synthesizer in Klatt (1980)). It is possible that OAs, in comparing their experience to more “robotic-sounding” TTS voices, conceptualize modern TTS voices like Siri as more “human-like”. In the present study, this leads us to predict 1) greater alignment toward voice-AI overall, and 2) more similar gender-mediated patterns for the voice-AI and human voices by OAs (relative to YAs). YAs, meanwhile, have grown up with a more constrained, and quickly increasing in naturalism, range of “robotic” and “human-like”. TTS voices. It is possible that YAs perceive the same Siri voices as being the more outdated ‘robotic’ variety of TTS voices. Therefore, we predict YAs will display 1) less alignment for voice-AI, and 2) less socially-mediated behavior toward voice-AI (here, gender-mediated alignment).
Current Study
The current study examines whether human-to-AI speech alignment occurs along similar social dimensions as it does in human-to-human alignment, comparing two age groups (mean ages of 67 vs. 20 years old). Our social category of interest is gender: with male and female shadowers responding to (apparent) male and female human and voice-AI talkers. To our knowledge, this is also the first experimental work to use the same set of model talkers to explore differences in alignment across older and younger adults. Experiment one is a word shadowing paradigm, where participants, in repeated (shadowed) words produced by four distinct model talker voices (2 naturally produced human voices and two TTS voices); pre-exposure productions of words by participants were collected before exposure to the model talkers. Experiment two is an AXB similarity rating task (Pardo et al., 2013) where a separate group of listeners rate whether participants’ pre-exposure and shadowed productions from Experiment one are more acoustically similar to the model talker’s production of the shadowed item, providing a holistic assessment of speech alignment.
Experiment one: Word Shadowing Task
Methods
Stimuli
Target words consisted of 12 monosyllabic English low-frequency words: bomb, sewn, vine, pun, shun, chime, yawn, shone, wane, tame, wren, hem (mean log frequency: 1.6; range: 1.1–2.1 (Brysbaert and New, 2009)) used in related imitation experiments with human and device interlocutors (Cohn et al., 2019; Snyder et al., 2019; Cohn et al., 2020). Stimuli consisted of recordings of the words produced by four distinct voices: two human and two AI device voices. First, items were recorded by female and male humans, native English speakers in their 20s, using a Shure WH20 XLR head-mounted microphone in a sound-attenuated booth. The AI voices were created using the command line on an Apple computer (OSX 10.13.6), and changing the Siri voice setting (US-female: “Samantha”, US-male: “Alex”).
Participants
A total of 46 participants completed the lexical shadowing task. Older adult participants (n = 22; 10 F, 12 M) were recruited from the community via a flyer or word of mouth. OAs’ mean age was 66.5 years (range = 53–81; sd = 8.7). Younger adult participants (n = 24; 12 F, 12 M) were recruited from the undergraduate subject pool and received course credit for their participation. The YAs’ mean age was 20.4 years (range = 18–39, sd = 4.3). All participants were native English speakers. Participants were asked to indicate whether they had any known hearing problems. Three OA and 1 YA participant indicated that they did have known hearing problems and their productions were excluded from the perceptual similarity ratings task.
Procedure
First, subjects were told they would be repeating words produced by four talkers: “Siri” and “Alex” (female and male devices); “Melissa” and “Carl” (female and male humans). The introduction included pictures of the talkers, to ensure that participants had the same top-down knowledge of the voice categories (as device or human). As seen in Figure 1, the images for Siri and Alex were separate iPhones displaying “active” Siri modes; the human images were stock photos of adults (selected from the first Google image results of “male” and “female stock image” at the time).
Next, participants' pre-exposure productions of the words were recorded in order to get their baseline production of each item prior to exposure to the model talkers. In this pre-exposure block, each of the 12 target words were displayed on a computer screen one at a time and participants produced each word in isolation twice, randomly selected. Then, participants completed the lexical shadowing portion of the experiment. In the shadowing block, participants heard one of four interlocutors saying the word and were asked to repeat (e.g., “Carl says ‘shone’”). Each trial consisted of a randomly selected word-model pairing. A block containing one repetition of each item per talker was repeated twice in the experiment. Each participant repeated the 12 words twice for each model (4 talkers x 12 words x two repetitions = 96 shadowed tokens). Productions were recorded, digitized at a 44 kHz sampling rate, using a Shure WH20 XLR head-mounted microphone in a sound-attenuated booth. Finally, subjects completed a background questionnaire about their voice-AI experience. For those who had experience with Apple’s Siri, subjects responded to a question aimed to probe their beliefs about it: “Does Siri sound like a real person?”. They responded “Yes” or “No” and then were asked to explain their choice (responses provided in Supplementary Material).
Experiment two: AXB Similarity Ratings Task
The purpose of Experiment two is to have independent raters assess the perceptual similarity between the participants’ shadowed productions of the target items and the model’s produced target item, relative to participants’ pre-exposure productions of the words (recorded at the beginning of the experiment, prior to exposure to the model talkers). To this end, we designed an AXB similarity ratings task, following the methods outlined in Pardo et al. (2017), using the stimuli and participant recordings from Experiment one.
Methods
Stimuli
The stimulus items for Experiment two were taken from model and shadower productions from Experiment one. The model utterances consisted of the stimuli presented to shadowers. The shadower utterances consisted of the second pre-exposure and shadowed productions of the target items collected. For each shadower, the second pre-exposure repetition was selected. All shadower items were trimmed to exclude latencies (provided in Appendix I). In order for a shadower’s items to be included in Experiment two, they had to have no mispronunciations across the 12 items in the pre-exposure or shadowed production. For example, one young adult male shadower produced many mispronunciations in the pre-exposure block, therefore his stimuli were not included. In total, 22 young adult shadowers (12 F, 10 M) and 19 older adult shadowers (9 F, 10 M) had full target word sets of pre-exposure and shadowed productions for use in Experiment two (41 total shadowers).
In order to present the stimuli in an online experiment, each AXB set of items was concatenated into a single sound file and played on a given trial. Prior to concatenation, all items were amplitude-normalized to 60 dB.
Participants
A total of 166 participants (mean age = 20 years old; 119 female, 46 male, one genderqueer), all native English speakers, provided holistic AXB similarity ratings of the shadowers’ and model talkers’ word productions. Raters were recruited through the UC Davis Psychology subjects’ pool and received course credit for their participation.
Procedure
The experiment was conducted online, using the Qualtrics survey platform. On a given trial, raters heard three items separated by a short silence (ISI = 400 ms): a shadower’s pre-exposure production and shadowed production of a word occurred as the first and third items (e.g., “A” and “B”; order counterbalanced evenly across trials), and the model talker’s production of that same word was the second item (“X”). The rater’s task is to identify the shadower’s token that sounded most similar to “X” (i.e., the model talker’s production). The option “first” and “third” was provided on the computer screen as a two-option selection. Raters would select one of these options before the experiment would advance to the next trial. Order of pre-exposure and shadowed token (i.e., “A” and “B”) was balanced within each shadower and counterbalanced across model talkers.
We limited the number of shadowers each participant rated in order to keep the experiment a reasonable length. Pardo et al. (2017) assessed the reliability in performance as the number of raters decreased, from 10 to one; they found that having at least 4 raters per shadower leads to high reliability. Thus, 23 lists were constructed, containing the full set of stimuli from two shadowers each. In total, each list contained 96 AXB similarity ratings (2 shadowers x 12 words x four model talkers). Each shadower was assessed fully by six to eight raters (raters were randomly assigned to one of the lists).
Analysis
Responses were coded for whether the shadowed item was selected as more similar to the model talker (=1) or not (=0). These data were analyzed in a mixed effects logistic regression using the glmer() function in the lme4 package in R (Bates et al., 2014). Estimates for p-values were computed using Satterthwaite approximation in the lmerTest package (Kuznetsova et al., 2015). Fixed effects included the Shadower Age group (2 levels: younger, older), the Model Talker Humanness (2 levels: human, digital device), Model Talker Gender (2 levels: female, male), and Shadower Gender (2 levels: female, male). Each fixed effect was contrast-coded. Chi-square tests, using the anova() function, revealed that the inclusion of each additional factor and interaction significantly improved model fit. The final model included all two-, three-way interactions between the fixed effects, since the addition of each improved model fit. Inclusion of the four-way interaction did not improve model fit, therefore it was not included in the final model. Random effects structure was maximal, given the design of the experiment, which included by-Shadower random intercepts and by-Shadower random slopes for Model Talker Humanness and Model Talker Gender and the interaction between them. By-Word random intercepts, by-Rater random intercepts and by-Rater random slopes for ordering of pre-exposure and post-exposure production were also included. To explore significant interactions, Tukey’s HSD pairwise comparisons were performed within the model, using the emmeans() function in the lsmeans R package (Lenth and Lenth, 2018).
Results
The output of the logistic regression run on raters’ responses is provided in Table 1. Numerically, with an overall AXB rating of 53%, listeners were greater than chance (50%) at selecting shadowing productions as more similar to the model talkers’ productions. Hence, shadowers aligned to the model talkers, in rates comparable to those reported in prior studies (e.g., Pardo et al., 2017).
The model also computed several significant interactions. First, there was an interaction between Shadower Gender and Model Talker Humanness, which is plotted in Figure 2: male shadowers align more to human voices overall. Post-hoc pairwise comparison using lsmeans revealed that Female shadowers show no difference in alignment toward device voices and human voices (z = 0.6, p = 0.5).
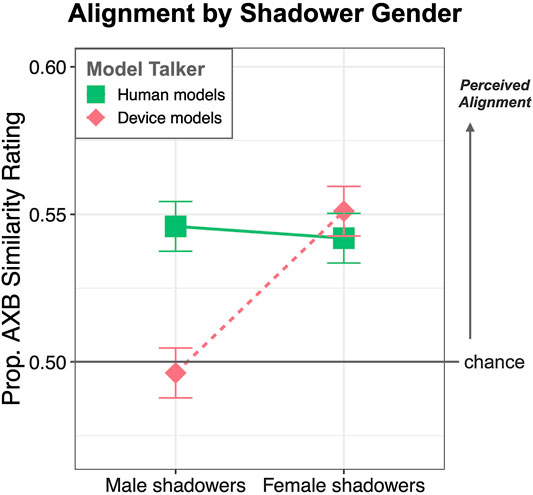
FIGURE 2. Mean proportion and standard errors of AXB perceptual similarity ratings for the interaction between Model Talker Humanness (Device vs. Human) and Shadower Gender (F, M).
There was also an interaction between Shadower Age and Model Talker Gender: as seen in Figure 3, YAs align more toward male model talkers (relative to female model talkers). Post-hoc pairwise comparison using lsmeans revealed the opposite for OA, who aligned more to female model talkers (relative to male model talkers) (z = 2.8, p < 0.01). Critically, though, this effect is part of a 3-way interaction between Shadower Age Group, Model Talker Humanness, and Model Talker Gender, also seen in Figure 3: YA showed the largest degree of alignment toward the human male model talker. Post-hoc pairwise comparison using lsmeans showed that YA alignment toward male and female device model talkers was the same (p = 0.7).
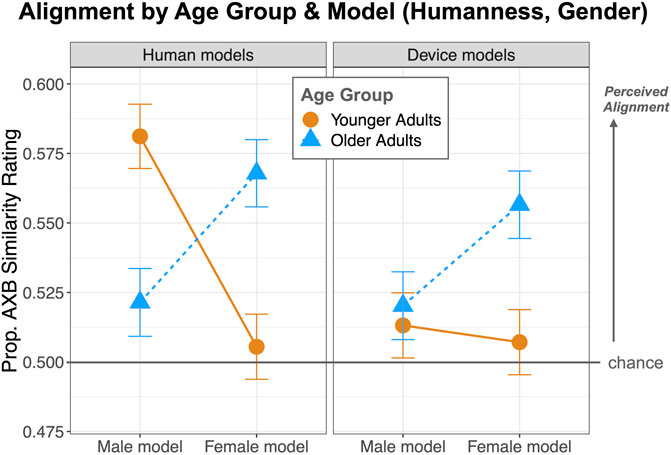
FIGURE 3. Mean proportion and standard errors of AXB perceptual similarity ratings for each Model Talker, by Humanness (Device vs. Human) and Gender (F, M).
Post-hoc Apparent Age Rating Survey
In the present study, one possibility is that the different age groups align toward the voices closest in apparent age category to them. To explore whether the apparent age category of the model talkers’ voices mediated shadowing, we conducted a post-hoc age rating study. An apparent age ratings survey was conducted on the model talkers’ productions. We hypothesize that the human voices, produced by adults in their 20s, might convey different apparent ages from the device voices and that this could explain alignment patterns across age groups.
Participants and Procedure
Eighteen native English-speaking participants (mean age = 19.67 years, sd = 1.41; 11 F), who had not participated in either Experiment one or Experiment two, were recruited from the UC Davis Psychology subjects’ pool and received course credit for their participation. Stimuli consisted of the 12 target words produced by each interlocutor from Experiment one, concatenated with a 1 s pause between each word in the same order. Participants completed the study in a sound-attenuated booth, facing a computer monitor and mouse, and wearing headphones (Sennheiser Pro). First, subjects were given instructions: “You will hear four talkers (Melissa, Carl, Siri, Alex). On each trial, play the recording and rate each talker's voice using the sliding scale (e.g., how old does Melissa sound?)”. On each trial, subjects heard all of one model talkers’ stimuli (with the same images used in Experiment one; see Figure 1); subjects could re-play the audio as many times as they liked. Then, subjects provided an estimate of the talker’s age (in years) using a sliding scale that ranged from 0–100. Each participant completed age ratings for the four model talkers (randomized for each subject).
Results
The results of the apparent age rating survey indicate distinct apparent ages of the human and device model talkers. Participants rated the human female and male as being younger (x̅ = 27.2, sd = 5.2 and x̅ = 23.5 years, sd = 2.4, respectively) than the female device (x̅ = 51.8, sd = 16.9) and male device (x̅ = 45, sd = 18.2). A mixed effects linear regression model was fit to age ratings, with fixed effects of voice Gender and Humanness, and their interaction, as well as random intercepts for rater. The model revealed that Humanness was a significant predictor of age rating, with the digital device voices rated as older sounding than the human voices (Coef = 11.5, SE = 1.3, p < 0.001). No other main effects or interactions were significant.
Individual Variation
Belief About Devices
One prediction as to why OAs and YAs might differentially respond to voice-AI interlocutors was based on differences in their beliefs about the system: OAs, we hypothesized, might perceive voice-AI as more human-like than YAs, when comparing the system to prior TTS productions they had heard (e.g., formant-based synthesizers Klatt (1980)). To probe this, we asked shadowers from Experiment one, who reported that they had experience with voice-AI, if “[the system] sounded like a real person” and to explain why or why not. Summaries of the responses and participants’ rationales are provided in Supplemental Materials. We found that both YAs and OAs predominately responded “No” (27/33) and gave similar rationales (e.g., sounding “robotic”). Only 1 YA and four OAs responded “Yes” (with one OA responding “Yes” for one system, but “No” for another). That the majority of participants did not think voice-AI sounds like a “real person” suggests that differences in alignment for OAs and YAs are not due to differences in perceptions across age groups of the systems as sounding more “human-like”.
Device Usage
As previously mentioned, we hypothesized the OA and YA would have different experiences with technology over their lifespans that could influence the way they engage with modern voice-AI systems. In order to explore whether their reported experience with voice-AI might have affected alignment patterns, we examined their reported voice-AI usage for the age groups. The majority of participants in both age groups reported experience with voice-AI: 12/19 OAs and 21/23 YAs reported that they used a voice-AI assistant on at least a weekly basis. The remaining participants (8/42) reported that they did not engage with voice-AI at all. Those who did use voice-AI reported using Apple’s Siri (9 OAs, 20 YAs), Amazon’s Alexa (9 OAs, eight YAs), and/or Google Assistant (2 OAs, six YAs).
Since there was little variation in device usage within the younger adult group, individual differences for YAs could not be explored. However, since there was variation within the older adult group (12 reported previous regular digital device experience, seven reported none), we ran a logistic mixed effects model on AXB responses to only OA shadower tokens with fixed effects of Model Humanness (2 levels: human, digital device) and Device Usage (2 level: regular, none), and their interaction. The model included by-Shadower random intercepts and by-Shadower random slopes for Model Talker Humanness, as well as by-Word random intercepts, by-Rater random intercepts, and by-Rater random slopes for AXB ordering. The model did not reveal any significant effects. Model Humanness was not significant (p = 0.7). Furthermore, there was no main effect of device usage (p = 0.19), nor a significant interaction between Usage and Humanness (p = 0.9).
General Discussion
The present study examined older and younger adults’ speech alignment toward human and device voices. First, we find that in a short laboratory experiment, individuals of both age groups display alignment toward human and voice-AI model talkers, consistent with prior work comparing alignment toward human and device voices for YAs only (e.g., Cohn et al., 2019; Snyder et al., 2019). This finding is informative to the impact of voice-AI assistants on human speech and language patterns, especially as increasing numbers of households adopt them (Ammari et al., 2019). This is one step toward understanding the influence of voice-AI on human behavior. As people increasingly use speech to interface with technology, understanding how voice-AI influences human language patterns will be more important.
Moreover, patterns of alignment toward voice-AI and human model talkers vary based on the characteristics of the shadower (their gender and age category) as well as the model talker (gender). Table 2 summarizes the patterns of results observed in the present study.
On the one hand, there is some evidence in the present findings for transfer of social behaviors from human-human to human-AI interaction, supporting predictions made by the CASA framework (Nass et al., 1994; Nass et al., 1997). For example, female shadowers align toward human and voice-AI model talkers to the same degree, in line with findings by Abel et al. (2020) who found that female participants showed no difference in perception of human-likeness for movements produced by a virtual robot and a digital human. However, in the same study, males did show a distinction (rating the digital human movements as more human-like than the robot). Similarly, this asymmetry is observed in the present study, where male shadowers aligned more toward human voices than device voices (with no perceived alignment toward the device). While these patterns are in contrast with studies reporting that male users display more positive attitudes and social behaviors toward robots (e.g., Schermerhorn et al., 2008), they are consistent with other work examining speech alignment toward interactive systems, where females showed stronger alignment in general for three different types of interactive AI systems (Cohn et al., 2020) and studies showing that female users respond with greater engagement and more positively to expressive embodied agents than male users (Foster, 2007). Our findings suggest that learned gender behaviors might be at play during human-AI interaction in some cases (e.g., for female participants) but that the gender of the participant is a critical mediating factor.
Additionally, there is more evidence for possible transfer of human-human alignment behaviors to voice-AI for older adults: OAs demonstrated similar gender-based asymmetries for voice-AI and human model talkers (i.e., greater alignment toward female, than male, model talkers). At the same time, younger adults showed no difference in alignment for device voices by gender, but a large difference for human model talkers based on gender, with more alignment to male than female human talkers. Greater alignment toward male voices for YAs is consistent with prior work (e.g., Namy et al., 2002; Cohn et al., 2019; Snyder et al., 2019). Yet, one possibility for the difference across age groups is that the perceived age of the model talkers might explain these patterns (e.g., if there were large differences in perceived age by female/male model talkers across categories). For example, there is work suggesting that differences in alignment across ages might be influenced by the differences in age of the interlocutors [e.g., discourse topics in Giles et al. (1992)]. Our post-hoc apparent age ratings survey did reveal differences in apparent age of the model talkers: the device voices were rated as older-sounding than the human voices. But, critically, we do not see greater alignment by OA toward devices than humans; this seems to suggest that the differences in alignment for OAs is predominantly driven by model talker gender. One possibility is that the distinct patterns of speech alignment toward male and female model talkers by OAs and YAs in the present study reflect different gender-mediated alignment patterns across generations. Indeed, it is well established that gender-differentiated patterns of language use are learned (Eckert and McConnell-Ginet, 1992), which might vary for different generations. That both age and gender influence alignment patterns is in line with socially-mediated theories of alignment (e.g., CAT, Shepard, 2001).
While we had predicted that OAs’ lifespan exposure with less advanced TTS systems would lead them to perceive voice-AI TTS productions to sound more human-like, this was not supported by the post-experiment survey. Instead, we found converging evidence that both age groups nearly unanimously did not think that the systems sounded like ‘real people’. Additionally, rationales for this belief were also similar across the groups, suggesting that OAs and YAs did not have systematically different beliefs or attitudes about voice-AI. At the same time, it is possible that a more gradient measure of technology anthropomorphism might reveal individual differences across people. Furthermore, we explored whether an individuals’ reported experience with voice-AI predicts their alignment patterns toward devices; while nearly all of the YAs reported experience, an individual differences analysis of OAs did not show any systematic difference between those who frequently use voice-AI and those who never used voice-AI.
At the same time, it is important to note that age category is not purely a social variable. For one, age-related cognitive and physiological decline on speech production patterns and hearing ability are well documented (e.g., Burke and Shafto, 2008; Gosselin and Gagné, 2011; Ferguson, 2012). While the present study did not include a pure tone audiometry test, we did exclude individuals who reported a hearing impairment (1 YA, three OAs). While it is possible that some of our OA participants may have had age-related hearing loss, this might lead to less alignment toward the model talkers if they are perceiving less acoustic information. As we do not see a systematic difference in degree of alignment among the YA and OA groups (i.e., no main effect of Age Group), this does not appear to be a factor in the present study. While speech-in-noise perception is a common challenge for OAs (Gosselin and Gagné, 2011) and those with hearing loss (Ferguson, 2012), the listening conditions in the current study were optimal: stimuli were presented in isolation (at 60dB) over headphones in a sound-attenuated booth. Additionally, potential cognitive differences in OAs did not appear to systematically affect the results: for instance, shadowing latencies were faster for OAs than YAs (provided in Appendix I), inconsistent with slower linguistic processing. Furthermore, the present study was a simple task that involved single word repetition, which did not appear to tax working memory evidenced by OAs’ faster latencies. Whether YAs and OAs vary in their speech behavior for voice-AI and humans in more interactive and/or cognitively demanding tasks (e.g., Pardo et al., 2010) remains an open area for future research. This work can contribute to understanding of age-related changes in speech behavior, an area warranting further extensive study across fields of speech perception as well as human-computer interaction.
Overall, it is clear that there are a complex array of factors that vary across age groups that are at play in alignment. This line of work opens up new avenues for exploring how people apply human norms from human-human speech communication to artificially intelligent systems. Such investigations can present novel tests and theoretical extensions to human-device interaction frameworks, as well as contribute to our understanding of human-human interaction (e.g., alignment). For example, studying cross-generational phonetic alignment can inform speech production models, which are often based on studies disproportionately run on college-age adults (Hazan, 2017). The current study makes an important contribution in investigating OAs, part of a larger gap in the scientific understanding of speech and language changes over the lifespan, as well as with different interlocutors (voice-AI vs. human). Future work exploring other age categories (e.g., children) and language backgrounds will be important in a larger scientific understanding of alignment and AI personification. Additionally, varying the types of stimuli used is another area for future work; while the current study used only low frequency words, looking at how alignment patterns apply in more contexts (e.g., low vs. high frequency words, words vs. nonwords) can reveal the mechanisms at play in alignment and further test the extent similar patterns hold in alignment toward other humans and voice-AI. Finally, alignment toward device speech has implications for more general human linguistic patterns. Specifically, speech imitation has been proposed as a mechanism for the spread of sound change (Babel, 2012; Garrett and Johnson, 2013); future work examining the extent to which alignment patterns persist over time—and how they might vary for different age groups—can begin to test whether interactions with voice-AI might play a role in sound change.
Data Availability Statement
The raw data supporting the conclusions of this article will be made available by the authors, without undue reservation.
Ethics Statement
The studies involving human participants were reviewed and approved by UC Davis Social & Behavioral Committee C. The patients/participants provided their written informed consent to participate in this study.
Author Contributions
GZ, MC, and BF designed the study. MC conducted the study. GZ statistically analyzed the data. GZ, MC, and BF wrote the paper. All authors contributed to the article and approved of the final version.
Funding
This material is based upon work supported by the National Science Foundation SBE Postdoctoral Research Fellowship under Grant No. 1911855 to MC and by an Amazon Faculty Research Award to GZ.
Conflict of Interest
The authors declare that the research was conducted in the absence of any commercial or financial relationships that could be construed as a potential conflict of interest.
Acknowledgments
Thank you to Melina Sarian and Riley Stray for their assistance in data collection.
Appendix i shadowing latencies
To determine whether there were systematic differences in shadower’s production latencies by speaker age group (e.g., older adults responding slower, or cutting off the model talker), we computed latencies from the offset of the model talker’s productions in shadowing trials by age group and model talker condition. All latencies were positive for both groups, indicating that the speakers produced their response after the model talker’s production had finished. Across all shadowing conditions, OAs responded more quickly than YAs (Device Female: OAs = 345 ms, YAs = 520 ms; Device Male: OAs = 460 ms, YAs = 565 ms; Human Female: OAs = 455 ms, YAs = 505 ms; Human Male: OAs = 415 ms, YAs = 481 ms).
References
Abel, M., Kuz, S., Patel, H. J., Petruck, H., Schlick, C. M., Pellicano, A., et al. (2020). Gender effects in observation of robotic and humanoid actions. Front. Psychol. 11, 797. doi:10.3389/fpsyg.2020.00797
Ammari, T., Kaye, J., Tsai, J. Y., and Bentley, F. (2019). Music, search, and IoT: how people (really) use voice assistants. ACM Trans. Comput. Hum. Interact. 26 (3), 1–28. doi:10.1145/3311956
Babel, M., and Bulatov, D. (2012). The role of fundamental frequency in phonetic accommodation. Lang. Speech. 55 (Pt 2), 231–248. doi:10.1177/0023830911417695
Babel, M. (2012). Evidence for phonetic and social selectivity in spontaneous phonetic imitation. J. Phonetics. 40 (1), 177–189. doi:10.1016/j.wocn.2011.09.001
Bates, D., Mächler, M., Bolker, B., and Walker, S. (2014). Fitting linear mixed-effects models using lme4. ArXiv Preprint. Available at: https://arxiv.org/abs/1406.5823 (Accessed June 23, 2020).
Bell, L. (2003). Linguistic adaptations in spoken human-computer dialogues—empirical studies of user behavior. Available at: http://urn.kb.se/resolve?urn=urn:nbn:se:kth:diva-3607 (Accessed January 2003).
Bentley, F., Luvogt, C., Silverman, M., Wirasinghe, R., White, B., and Lottridge, D. (2018). Understanding the long-term use of smart speaker assistants. Proc. ACM Interact. Mob. Wearable and Ubiquitous Technol. 2 (3), 1–24. 10.1145/3264901.
Brysbaert, M., and New, B. (2009). Moving beyond Kucera and Francis: a critical evaluation of current word frequency norms and the introduction of a new and improved word frequency measure for American English. Behav. Res. Methods. 41 (4), 977–990. doi:10.3758/BRM.41.4.977
Burke, D. M., and Shafto, M. A. (2008). Language and aging. In The handbook of aging and cognition. Editors F. I. M. Craik, and T. A. Salthouse (Hove, UK: Psychology Press), 373–443.
Chartrand, T. L., and Bargh, J. A. (1996). Automatic activation of impression formation and memorization goals: nonconscious goal priming reproduces effects of explicit task instructions. J. Pers. Soc. Psychol. 71 (3), 464–478. 10.1037/0022-3514.71.3.464.
Cohn, M., Ferenc Segedin, B., and Zellou, G. (2019). “Imitating Siri: socially-mediated alignment to device and human voices,” in Proceedings of International Congress of Phonetic Sciences, Melbourne, Australia, August 5–9, 2019, 1813–1817.
Cohn, M., Jonell, P., Kim, T., Beskow, J., and Zellou, G. (2020). “Embodiment and gender interact in alignment to TTS voices,” in Proceedings of the Cognitive Science Society, Montreal, Canada, July 29–August 1, 2020, 220–226.
DePaulo, B. M. (1992). Nonverbal behavior and self-presentation. Psychol. Bull. 111 (2), 203. doi:10.1037/0033-2909.111.2.203
Eckert, P., and McConnell-Ginet, S. (1992). Think practically and look locally: language and gender as community-based practice. Annu. Rev. Anthropol. 21 (1), 461–488. doi:10.1146/annurev.an.21.100192.002333
Ezer, N., Fisk, A. D., and Rogers, W. A. (2009). More than a servant: self-reported willingness of younger and older adults to having a robot perform interactive and critical tasks in the home. Proc. Hum. Factors Ergon. Soc. Annu. Meet. 53 (2), 136–140. doi:10.1177/154193120905300206
Ferguson, S. H. (2012). Talker differences in clear and conversational speech: vowel intelligibility for older adults with hearing loss. J. Speech Lang. Hear. Res. 55, 779. doi:10.1044/1092-4388(2011/10-0342)
Foster, M. E. (2007). “Enhancing human-computer interaction with embodied conversational agents,” in The International Conference on Universal Access in Human-Computer Interaction, Beijing, China, June 22–27, 2007, 828–837.
Garrett, A., and Johnson, K. (2013). “Phonetic bias in sound change,” in Origins of Sound Change: Approaches to Phonologization., 51–97.
Giles, H., Coupland, N., Coupland, J., Williams, A., and Nussbaum, J. (1992). Intergenerational talk and communication with older people. Int. J. Aging Hum. Dev. 34 (4), 271–297. doi:10.2190/TCMU-0U65-XTEH-B950
Giles, H., Coupland, N., and Coupland, I. (1991). Accommodation theory: communication, context, and consequence,” in Contexts of accommodation: developments in applied sociolinguistics. Cambridge, United Kingdom: Cambrigde University Press, 1, 1–68.
Goldinger, S. D. (1998). Echoes of echoes? An episodic theory of lexical access. Psychol. Rev. 105 (2), 251–279. doi:10.1037/0033-295x.105.2.251
Gosselin, P. A., and Gagné, J. P. (2011). Older adults expend more listening effort than young adults recognizing audiovisual speech in noise. Int. J. Audiol. 50, 786. doi:10.3109/14992027.2011.599870
Gregory, S. W., and Hoyt, B. R. (1982). Conversation partner mutual adaptation as demonstrated by Fourier series analysis. J. Psycholinguist. Res. 11 (1), 35–46. doi:10.1007/BF01067500
Habler, F., Schwind, V., and Henze, N. (2019). “Effects of smart virtual assistants’ gender and language,” in The Proceedings of Mensch und Computer 2019, Hamburg, Germany, September 8–11, 2019, 469–473.
Harrison, A. W., and Rainer, R. K. (1992). The influence of individual differences on skill in end-user computing. J. Manag. Inf. Syst. 9 (1), 93–111. doi:10.1080/07421222.1992.11517949
Hazan, V. (2017). Speech production across the lifespan. Acoust. Today. 13 (1), 36–43. doi:10.3758/s13428-011-0075-y
Herring, S. C. (2003). “Gender and power in on-line communication,” in The Handbook of Language and Gender., 202–228.
Herring, S. (1996). Posting in a different voice: gender and ethics in computer-mediated communication. Philosophical Perspectives on Computer-Mediated Communication. 115, 45.
Hwang, G., Lee, J., Oh, C. Y., and Lee, J. (2019). It sounds like a woman: exploring gender stereotypes in south Korean voice assistants,” in TheExtended abstracts of the 2019 CHI conference on human factors in computing systems, Glasgow, United Kingdom, May, 2019, 1–6.
Klatt, D. H. (1980). Software for a cascade/parallel formant synthesizer. J. Acoust. Soc. Am. 67 (3), 971–995. doi:10.1121/1.383940
Kuznetsova, A., Brockhoff, P. B., and Christensen, R. H. B. (2015). Package ‘lmertest R Package Version. J. Statist. Software. 2. doi:10.18637/jss.v082.i13
Lenth, R., and Lenth, M. R. (2018). Package ‘lsmeans. Am. Statistician. 34 (4), 216–221. doi:10.1080/00031305.1980
Namy, L. L., Nygaard, L. C., and Sauerteig, D. (2002). Gender differences in vocal accommodation: the role of perception. J. Lang. Soc. Psychol. 21 (4), 422–432. doi:10.1177/026192702237958
Nass, C., Moon, Y., Morkes, J., Kim, E.-Y., and Fogg, B. J. (1997). Computers are social actors: a review of current research. Human Value Des. Comput. Technol. 72, 137–162. doi:10.1145/259963.260288
Nass, C., Steuer, J., and Tauber, E. R. (1994). “Computers are social actors,” in Proceedings of the SIGCHI Conference on Human Factors in Computing Systems, New York, NY, April 24–28, 1994, 72–78.
Natale, M. (1975). Convergence of mean vocal intensity in dyadic communication as a function of social desirability. J. Pers. Soc. Psychol. 32 (5), 790–804. doi:10.1037/0022-3514.32.5.790
Nielsen, K. (2014). Phonetic imitation by young children and its developmental changes. J. Speech Lang. Hear. Res. 57 (6), 2065–2075. doi:10.1044/2014_JSLHR-S-13-0093
Nomura, T., Kanda, T., and Suzuki, T. (2006). Experimental investigation into influence of negative attitudes toward robots on human–robot interaction. AI Soc. 20 (2), 138–150. doi:10.1007/s00146-005-0012-7.
Oksenberg, L., Coleman, L., and Cannell, C. F. (1986). Interviewers’ voices and refusal rates in telephone surveys. Publ. Opin. Q. 50 (1), 97–111.
Olmstead, K. (2017). Nearly half of Americans use digital voice assistants, mostly on their smartphones. Washington, DC: Pew Research Center.
Pardo, J. S., Jay, I. C., and Krauss, R. M. (2010). Conversational role influences speech imitation. Atten. Percept. Psychophys. 72 (8), 2254–2264. doi:10.3758/APP.72.8.2254
Pardo, J. S., Urmanche, A., Wilman, S., and Wiener, J. (2017). Phonetic convergence across multiple measures and model talkers. Atten. Percept. Psychophys. 79 (2), 637–659. doi:10.3758/s13414-016-1226-0
Pardo, J. S., Gibbons, R., Suppes, A., and Krauss, R. M. (2012). Phonetic convergence in college roommates. J. Phonetics. 40 (1), 190–197 doi:10.1016/j.wocn.2011.10.001
Pardo, J. S. (2006). On phonetic convergence during conversational interaction. J. Acoust. Soc. Am. 119 (4), 2382–2393. doi:10.1121/1.2178720
Piper, A. (2016). Stereotyping femininity in disembodied virtual assistants. PhD Thesis. Ames (Iowa): Iowa State University.
Raveh, E., Siegert, I., Steiner, I., Gessinger, I., and Möbius, B. (2019). Three’sa crowd? Effects of a second human on vocal accommodation with a voice assistant. Proc. Interspeech. 2019, 4005–4009.
Schermerhorn, P., Scheutz, M., and Crowell, C. R. (2008). “Robot social presence and gender: do females view robots differently than males?” in Proceedings of the 3rd ACM/IEEE International Conference on Human Robot Interaction, 263–270.
Shepard, C. A. (2001). “Communication accommodation theory,” in The New Hand-Book of Language and Social Psychology., 33–56.
Shockley, K., Sabadini, L., and Fowler, C. A. (2004). Imitation in shadowing words. Percept. Psychophys. 66 (3), 422–429. doi:10.3758/bf03194890
Snyder, C., Cohn, M., and Zellou, G. (2019). “Individual variation in cognitive processing style predicts differences in phonetic imitation of device and human voices,” in Proceedings of the Annual Conference of the International Speech Communication Association, Graz, Austria, September 15–19, 2020, 116–120.
Sonderegger, M., Bane, M., and Graff, P. (2017). The medium-term dynamics of accents on reality television. Language. 93 (3), 598–640 doi:10.1353/lan.2017.0038
Suzuki, N., and Katagiri, Y. (2007). Prosodic alignment in human–computer interaction. Connect. Sci. 19 (2), 131–141. doi:10.1080/09540090701369125
Szabo, I. (2019). “Phonetic Selectivity in accommodation: the effect of chronological age,” in Proceedings of the 19th International Congress of Phonetic Sciences, Canberra, Australia, August 5–9, 2019, 3195–3199.
Walker, A., and Campbell-Kibler, K. (2015). Repeat what after whom? Exploring variable selectivity in a cross-dialectal shadowing task. Front. Psychol. 6, 546. doi:10.3389/fpsyg.2015.00546
Zellou, G., Scarborough, R., and Nielsen, K. (2016). Phonetic imitation of coarticulatory vowel nasalization. J. Acoust. Soc. Am. 140 (5), 3560–3575. doi:10.1121/1.4966232
Zellou, G., and Cohn, M. (2020). Social and functional pressures in vocal alignment: differences for human and voice-AI interlocutors. Proc. Interspeech. 2020, 1634–1638. doi:10.21437/Interspeech.2020-1335
Keywords: voice-AI, human-device interaction, speaker age, speaker gender, speech alignment
Citation: Zellou G, Cohn M and Ferenc Segedin B (2021) Age- and Gender-Related Differences in Speech Alignment Toward Humans and Voice-AI. Front. Commun. 5:600361. doi: 10.3389/fcomm.2020.600361
Received: 29 August 2020; Accepted: 21 December 2020;
Published: 20 January 2021.
Edited by:
Junying Liang, Zhejiang University, ChinaReviewed by:
Zhen Qin, Hong Kong University of Science and Technology, Hong KongJing Shao, Hong Kong Baptist University, Hong Kong
Copyright © 2021 Zellou, Cohn and Ferenc Segedin. This is an open-access article distributed under the terms of the Creative Commons Attribution License (CC BY). The use, distribution or reproduction in other forums is permitted, provided the original author(s) and the copyright owner(s) are credited and that the original publication in this journal is cited, in accordance with accepted academic practice. No use, distribution or reproduction is permitted which does not comply with these terms.
*Correspondence: Georgia Zellou, Z3plbGxvdUB1Y2RhdmlzLmVkdQ==