- 1Department of Psychology and Neuroscience, Emmanuel College, Boston, MA, United States
- 2Department of Chinese and Bilingual Studies, Faculty of Humanities, The Hong Kong Polytechnic University, Hong Kong, China
In this paper, we present a computational approach to bilingual speakers’ non-native (L2) lexical-semantic representations. Specifically, based on detailed analyses of the error patterns shown in our previous simulation results (Zhao and Li Int. J. Bilingual. Educ. Bilingual., 2010, 13, 505–524; Zhao and Li, Bilingualism, 2013, 16, 288–303), we aim at revealing the underlying learning factors that may affect the extent of fuzzy category boundaries within bilinguals’ L2 representation. Here, we first review computational bilingual models in the literature that have focused on simulating L2 lexical representations, including the Developmental Lexicon II (DevLex-II) model (Zhao and Li, Int. J. Bilingual. Educ. Bilingual., 2010, 13, 505–524; Zhao and Li, Bilingualism, 2013, 16, 288–303), on which the current study is based. The DevLex-II modeling results indicate a strong age of acquisition (AoA) effect: When the learning of L2 is early relative to that of native language (L1), functionally distinct lexical representations may be established for both languages; when the learning of L2 is significantly delayed relative to that of L1, fuzzy L2 representations may occur due to the structural consolidation (or the entrenchment) of the L1 lexicon. Next, we explore the error patterns shown in both lexical comprehension and production in DevLex-II. A novel contribution of the current study is that we systematically compare the computational simulation results with empirical findings. Such model-based error analyses extend our previous findings by indicating, especially in the late L2 learning condition, that fuzzy L2 semantic representations emerge and lead to processing errors, including errors in unstable phonology-semantic and semantic-phonemic mappings. The DevLex-II model provides a computational account of the development of bilinguals’ L2 representation with reference to the dynamic interaction and competition between the two lexicons. We point to future directions in which fuzzy L2 representations may be overcome, through a framework that highlights the social learning of L2 (SL2) and the embodied semantic representation of the lexicon in the new language (Li and Jeong, Npj Sci. Learn., 2020, 5, 1–9; Zhang, Yang, Wang and Li, Lang. Cogn. Neurosci., 2020, 35, 1223–1238).
Introduction
It is widely recognized that at least half of the world’s population can use more than one language in their daily lives (Grosjean and Li, 2013). Many of them are bilinguals who are fluent in both of their languages, but many more are individuals who are second language (L2) learners with varying levels of mastery of their L2 depending on various learning and learner factors, such as timing and history of learning L2 (Li, 2013), social interaction needs (Li and Jeong, 2020), context of usage (the Complementarity Principle; Grosjean, 2013), and cognitive abilities [such as working memory; see reviews by Kormos (2015), Wen et al. (2017)]. Behavioral evidence has shown that L2 learners often have comprehension and production problems in L2, particularly with decoding and producing the ambiguous sounds that they are unsure of (such as phonemes not available in their native language, see a review by Gor, 2015). Bilinguals, as compared with monolinguals, may have more difficulties in word recognition tasks (Lemhöfer et al., 2008) or generating fast and accurate names in picture naming or word naming tasks (Gollan et al., 2005; Gollan et al., 2008; Li et al., 2019; Peñaloza et al., 2019). They may also find it difficult to make accurate phonology-semantic or semantic-phonemic mappings in their L2 (Cook et al., 2016) and experience higher rate of tip-of-the-tongue state in L2 (Kreiner and Degani, 2015).
Many theoretical frameworks have been proposed to account for such patterns and difficulties in the L2, which include hypotheses on a fuzzy, weak, or less entrenched lexical-semantic representation/network of L2 (Hernandez and Li, 2007; Diependaele et al., 2013; MacWhinney, 2013; Cook and Gor, 2015). In their recent Ontogenesis Model (OM), Bordag et al. (2021, p.2) argued that a crucial property of L2 lexical representation is fuzziness, which “refers to inexact or ambiguous encoding of different components or dimensions of the lexical representation that can be caused by several linguistic, cognitive, and learning-induced factors.” (see also Gor et al., 2021 for the Fuzzy Lexical Representations account in this special research topic). Such a view is highly consistent with the concept of “parasitism” proposed by Hernandez et al. (2005), according to which factors such age of acquisition (AoA), proficiency, and in particular competition/interaction between L1 and L2, are responsible for a L2 lexical-semantic representations that become parasitic (and usually fuzzy) to L1 representations (also see Hernandez and Li, 2007). It is thus important to study the dynamic interaction between L1 and L2 and how the L2 learning history can shape the bilinguals’ L2 representations. Indeed, an issue of enduring interest in bilingualism research has been how the lexical-semantic system of L2, as a dynamic system, is represented and developed, and subsequently interacts with the L1 system in the bilingual’s mind (Li, 2013).
Since the 1950s, there have been many models and hypotheses of bilinguals’ lexical representations and processing (see, e.g., Jiang, 2015 for a historical review). These models often offer good explanatory power of bilingual language patterns and have made significant contributions to our understanding of bilingualism. However, most of the models so far are verbally descriptive in nature and have been designed to capture bilingual lexical processing for the mature adult bilingual speakers, rather than accounting for the developmental changes associated with the learning of a L2 or the processes underlying learning. In this paper, we advocate a dynamic approach of bilingual lexical-semantic representation, viewing it as constantly changing and evolving as learning progresses (e.g., Li, 2015). Rather than just taking a snapshot of the static situation (e.g., as end result or outcome), we focus on the underlying mechanisms that may affect the learning process that leads to fuzzy category boundaries within bilinguals’ L2 representation. To reach this goal, we use computationally implemented models, which are particularly helpful in helping us to understand the L1-L2 interaction and the emergence of fuzzy representations in L2. Computational models allow the researchers to bring multiple variables and the complex interaction between the variables under systematic control, and test hypotheses about the roles of variables of interest in bilingual representation while holding other variables constant. Such a systematic control of variables is particularly important for studying bilingualism, due to the multitude of potentially confounding variables existed in the natural contexts of bilingual learning that would be otherwise difficult to manipulate in behavioral studies (see Li and Zhao, 2017; Li and Zhao, 2021 for discussions of the role of computational modeling in psycholinguistics).
In this paper, we focus on systematically analyzing the patterns of the inaccurate lexical comprehension and production, along with their underlying mechanisms in a computational bilingual model. Error analysis of second language learners has been an important topic in applied linguistics, especially in foreign language education (see Chapter 2. Ellis, 1994; Swan, 1997). However, it attracts less attention in computational studies, which often focus on what a model structure is capable/competent to achieve in term of empirical behaviors, with simulation errors commonly treated as byproducts of statistical fluctuations (but see the classic connectionist models of U-shaped behavior in monolingual past-tense learning, Rumelhart and McClelland, 1986). Here we advocate a systematic comparison of computational modeling results with a detailed analysis of error patterns that occur in behavioral data from real second language learners, which can help us to understand the role of the interactive mechanisms embodied in the model on the emergence of fuzzy L2 lexical-semantic representations.
In the following sections, we first review computational bilingual models that have previously focused on simulating L2 lexical processing and representations1, including the Developmental Lexicon II (DevLex-II), an unsupervised connectionist model that includes three basic levels for the representation and organization of linguistic information (see Li et al., 2007; Zhao and Li, 2010; Zhao and Li, 2013). Our current computational simulations are based on the DevLex-II model. In the main part of this paper, we focus on analyzing the late L2 learning condition, in which fuzzy L2 representations (at both semantic and phonological levels) lead to processing errors or confusions, including errors in unstable phonology-semantic and semantic-phonemic mappings. The error patterns are further compared with behavioral data from previous literature and a Second Language Acquisition (SLA) corpus (COPA corpus on TalkBank, Zhang, 2009a). We argue that the DevLex-II model provides a computational account of the development of bilinguals’ L2 representation with reference to the dynamic interaction and competition between the two lexicons. We further point to future directions, including studies of fuzzy L2 representations that may be overcome through an approach that highlights the social learning of L2 (SL2) and the embodied semantic representation of the lexicon in the new language (Li and Jeong, 2020; Zhang et al., 2020).
Computational Models of Bilingual Lexical Representations
Interactive Activation Models
IA-based models have been mainly used in simulating patterns in bilingual language processing. The Bilingual Interactive Activation (BIA) model (Dijkstra and van Heuven, 1998) might be the best-known computational model of bilingual lexical processing so far. Similar to the famous monolingual IA model (McClelland and Rumelhart, 1981), BIA has three levels of nodes representing orthographic features, letters, and words. Unlike IA, however, the BIA has linguistic inputs from two languages, and is equipped with a language node level that provides top-down information regarding the language identity of perceived words.
As a successor of the BIA model, BIA+ (Dijkstra and van Heuven, 2002) incorporates semantic and phonological representations into its main component (i.e. the word identification system), along with a nonlinguistic task/decision system. The nonlinguistic task/decision system receives input from the identification system and computes processing steps and determines decision criteria for the simulation task, such as bilingual reading. Dijkstra et al. (2012) applied the BIA + structure to model lexical processing of Dutch-English bilinguals. In their simulations, proficiency and AoA were modeled by adjusting the relative frequency of the L2 words and the size of the lexicon, and the model predicted a gradual increase in processing speed in L2 for the late L2 learners.
Diependaele et al. (2013) used a bimodal interactive activation model to simulate the difference between the frequency effects in L1 and L2 word recognition. Specifically, they reduced the resting levels of the word nodes to simulate the “weaker” lexical memory representations in L2. They also reduced the level of word-word lexical inhibition in the model to simulate the increased competition between similar words caused by the less “precise” lexical representations in L2. Their simulation results were in line with the patterns shown in large-scale English word identification times from three bilingual populations. They concluded that L2 is less entrenched in late L2 learners’ lexical system due to low L2 proficiency, and this lower entrenchment could explain the stronger frequency effect in L2 word recognition.
As a new computational model of bilingual representation along this direction, Multilink (Dijkstra et al., 2019) represents the latest efforts by the researchers to scale up the BIA modeling enterprise to a larger and more realistic lexicon (over 1,500 words from both lexicons). Multilink is an interactive based model integrating certain features of BIA+ (Dijkstra and van Heuven, 2002) and the Revised Hierarchical Model (RHM; Kroll and Stewart, 1994). By considering the role of multiple factors such as the frequency, length, orthographic similarity, and phonological neighborhood of words, it has been used to test and verify against empirical data from bilingual word recognition and translation.
It is worth noting that many IA-based models shown above lacked learning/development mechanisms. Their representations were often fixed and their parameters (e.g., resting level) manually adjusted to capture adult bilingual speakers’ word processing (see Li and Grant, 2019 for a commentary on the Multilink model). In fact, a wide variety of computational developmental models with a learning mechanism have been implemented for bilingual lexical representations, and they often embrace an emergentist view that static linguistic representations (e.g., words, concepts, and grammatical structures) are emergent properties, dynamically acquired from the learning environment. Common learning algorithms can be classified roughly into supervised and unsupervised learning (see Zhao, 2017 for a brief introduction). These algorithms are often developed under the framework of connectionist or neural network models (aka Parallel Distributed Processing or PDP models; McClelland et al., 1986; for a bibliography and recent models based on connectionism, see Li and Zhao, 2020).
Developmental Models with Supervised Learning
French (1998) tested a Bilingual Simple Recurrent Network model (BSRN), which was based on the monolingual SRN model (Elman, 1990). The BSRN was trained on intermixed sentences from two artificially generated languages, with its input having a certain probability of switching between the two languages. The model’s immediate task was to predict the next word in a sentence given the current word input. After training, a hierarchical cluster analysis was conducted on the hidden-node activations of the BSRN model, and results showed that words from the two languages became separated in the network’s internal representations. The simulation results supported the hypothesis that the bilingual input environment itself is sufficient for the development of a distinct mental representation of each language, without invoking separate processing or storage mechanisms for the different languages.
Monner et al. (2013) developed a connectionist model in an effort to address a long-standing issue in bilingual language acquisition: To what extent the entrenchment of one’s first language influences the learning of a second language? They tested the “less is more” hypothesis using a recurrent network model (Long Short-Term Memory or LSTM, Hochreiter and Schmidhuber, 1997) that learns the gender assignment and agreement in Spanish and French. In their network, increases of working memory were simulated using new cell assemblies in the model, whereas L1 entrenchment was simulated by training of the network with different length of L1 exposure before the onset of L2 (see more discussion below on this in the DevLex-II model). This approach allowed the researchers to dissociate and specify the effects due to age of L2 onset and those due to memory capacity. The authors concluded that their model supported the “less is more” hypothesis while at the same time showing L1 entrenchment effect as a function of L2 onset time.
During the last decade, there has been a fast-growing use of building semantic representations through the so-called “embedding” methods, which allows researchers to derive words’ lexical-semantic representations for distributional properties in natural language usage. Many of the methods were based on supervised learning of large-scale monolingual database (such as Word2Vec, Mikolov et al., 2013). Following this direction, researchers have developed interests in cross-language word embeddings (see a brief review and the M2VEC model in Wang et al., 2019). A common strategy has been to build a mapping/transformation matrix between two, usually well pretrained, monolingual word embedding spaces (one as the source/input language and the other the target/output language). Such a strategy often needs a pre-built high-quality dictionary or parallel corpora to align the words/concepts in the two languages. Although this approach makes it a great addition to applied fields such as machine translation, it is less ideal for simulating L2 learners’ bilingual lexical representations, which are dynamic and interactive in nature and more than just a mapping between two fully developed monolingual lexical spaces (keeping in mind Grosjean’s earlier warning that bilinguals are not the sum of two monolinguals; Grosjean, 1989).
Developmental Models with Unsupervised Learning
In contrast to supervised learning, unsupervised learning algorithms do not use explicit error signal at the output level to adjust the network’s weights. Among them, a popular type for bilingual models is the self-organizing map (SOM; Kohonen, 2001). On a SOM, a group of nodes with input connections are arranged on a two-dimensional lattice for the organization of external stimulus patterns. Each node has a vector associated with it to represent the weights of its input connections. At each training step of SOM, all nodes on the map are presented with an external input pattern (e.g., the phonological or semantic representation of a word). Some nodes will be activated, according to how similar their input weight vectors are to the input pattern; the node that has the highest activation is declared as the Best Matching Unit (BMU). A BMU’s weight vectors are adjusted, along with the weights of its neighboring nodes to become more similar to the input pattern. As the result, they will respond to the same or similar inputs more strongly next time. Initially activation occurs in large areas of the map, but gradually learning becomes more focused and the neighborhood size reduces. This self-organizing process continues until all inputs have found some maximally responding nodes as their BMUs. Eventually, the map falls into a topography-preserving state, which means that inputs with similar features will activate nodes in nearby regions, yielding meaningful activity bubbles that can be visualized on the map. This property of the SOM enables researchers to explicitly examine and visualize the emergence of lexical-semantic structures in their models. It is a more desired feature that is absent in some supervised learning models as discussed above, where the internal representation is often “hidden” from the outside and needs to be analyzed through mathematical tools such as Principal Component Analysis (PCA) and Hierarchical Cluster Analysis (HCA).
Li and Farkas (2002) proposed a self-organizing model of bilingual processing (SOMBIP). The model was based on the SOM described above, with training data derived from linguistic corpora. The SOMBIP model included two SOM maps connected via Hebbian learning. One SOM was trained on the phonological representations of words and the other on the semantic representations of words. The SOMBIP learned bilingual input with mixed English and Chinese words simultaneously; and the frequency of the bilingual words exposed to the network was modulated according to the corpus data, rather than to an ad hoc probability of language switching as in BSRN. In the SOMBIP, the simultaneous learning of Chinese and English led to distinct lexical representations for the two languages, as well as structured semantic and phonological representations within each language. The SOMBIP also simulated a novice learner by having limited linguistic experience so that the network was exposed to fewer sentences in L2. It was shown that the novice network’s representation of the L2 was more compressed and less clearly delineated, compared to that of the “proficient” network. The SOMBIP possessed more realistic linguistic and developmental properties than previous bilingual models and later evolved into the DevLex (Developmental Lexicon) models (Li et al., 2004; Li et al., 2007; Zhao and Li, 2010; Zhao and Li, 2013, see below for further discussions).
Recently, Peñaloza, et al. (2019) presented a SOM-based model to simulate the effect of AoA and L2 exposure on bilingual lexical access. Their model, BiLex, includes three connected SOMs, with one for common semantic/conceptual representation, and two for separate phonological representations of different languages (i.e. English and Spanish). Specifically, the model was applied to simulate the picture naming data on a case-by-case basis for 28 bilingual participants with different L2 proficiency levels and 5 monolinguals (as the base line). Their model incorporated important variables underlying the patterns of bilingual behavior, including language history regarding age of L2 acquisition, proficiency, exposure and language use. The best-fit set of values for parameters representing these variables were found based on an evolutionary algorithm (Back, 1996) to model the data of each participant. The model’s close match with real behavioral data from individual participants is a testimony that computational models, when properly constructed, can closely reflect realistic linguistic processes. Importantly, their simulations showed that early AoA and increased exposure can lead to well-organized representations on L2 phonological map and higher picture naming performance, while late AOA and limited exposure can lead to poor representations on L2 phonological map and lower picture naming performance. This pattern is in line with our simulation results from the DevLex-II, which we discuss next. The basic structure of Bilex resembles the well-developed theoretical framework of the RHM (Kroll and Stewart, 1994). However, the use of two predefined phonological maps for L1 and L2 assumes separated lexical representations of the two languages from the beginning of L2 learning, which could be problematic and makes it hard to simulate cross-language interferences. As discussed below, distinct or intermixed bilingual representations may emerge in the same underlying system such as the DevLex-II model through learning in the SOM or other computational algorithms.
The DevLex-II Model: A Sketch
The DevLex-II model is the main computation architecture of the current study. As an unsupervised multi-layer neural network model, the DevLex-II model has been successfully implemented in both monolingual and bilingual language learning (Li et al., 2007; Zhao and Li, 2010; Zhao and Li, 2013). As depicted in Figure 1, it consists of three basic levels for the representation and organization of linguistic contents, corresponding to phonological information, semantic information, and the output sequence of the lexicon, respectively. The core of the DevLex-II model is a SOM that handles lexical-semantic representation. This SOM is connected with two other SOMs, one for input (auditory) phonology, and the other for articulatory sequences of output phonology. During the training of DevLex-II, the semantic representation, input phonology, and output phonemic sequence of a word are simultaneously presented to and processed by their corresponding maps of the network, and the associative connections between maps are trained by the Hebbian learning rule. After the cross-map connections are stabilized, the activation of a word form can evoke the activation of a word meaning via form-to-meaning links, and we define this process as word comprehension in our model. Similarly, the activation of a word meaning can trigger the activation of an output sequence via meaning-to-sequence links, and we define this process as word production.
We have applied the DevLex-II model in various studies to simulate bilingual language learning (e.g., Zhao and Li, 2010) and cross-language priming (Zhao and Li, 2013). Specifically, to increase the connection to empirical data, we presented the model with an English-Chinese bilingual lexicon made up of 1,000 real words extracted from MacArthur-Bates Communicative Development Inventories (the CDI; English: Dale and Fenson, 1996; Chinese: Tardif et al., 1999). The input to the model was coded as vector representation of the phonemic, phonological, or semantic information of these words (see Zhao and Li, 2010 for technical details).
Simulations in Zhao and Li (2010), Zhao and Li (2013) included three learning conditions: simultaneous, early, and late, and the target lexicons were sent to the model in stages. The simultaneous learning condition was designed to simulate a simultaneous bilingual learner who is exposed to both languages from early on. In the two sequential learning conditions, learning of L2 was delayed relatively to that of L1, either only slightly (early learning: after 100 L1 words were presented) or significantly (late learning: after 400 L1 words were presented). The exposure to L2 words in all three conditions was 10 stages, with 50 more new L2 words added at each stage.
The setup in Zhao and Li (2010), Zhao and Li (2013) allows a meaningful comparison of the three learning conditions on the effects that the consolidation of lexical organization in one language (usually L1) has on the lexical representation in the other language (usually L2). The modeling results indicate a strong age of acquisition (AoA) effect: When the learning of L2 is early relative to that of L1, functionally distinct lexical representations may be established for both languages; when the learning of L2 is significantly delayed relative to that of L1, fuzzy L2 representations occur due to the structural consolidation (or the “entrenchment”) of the L1 lexicon and its impact on the L2 lexicon.
The present study extends the work of (Zhao and Li, 2010; Zhao and Li, 2013) to examine error patterns, using the same model structure and training parameters (see the Methods section in Zhao and Li, 2010 for technical details). Our analyses below focus on the sequential bilingual learning stages, specifically, on a variety of errors produced by the DevLex-II model under the early and late L2 learning conditions. These error patterns provide a window into the developmental changes underlying lexical-semantic representation, for not only the bilingual’s L2 but also its interaction with the L1. They are also evaluated against empirical data that reflect patterns of L2 learners’ pronunciation errors (e.g., Cutler et al., 2004; Zhang, 2009b; Wang and Chen, 2020).
Analyses of Error Patterns in Bilingual DevLex-II
L2 learners often have comprehension and production problems in their L2, producing errors that deviate from L1 speakers or listeners. In the current study, we have found interesting error patterns in DevLex-II’s comprehension and production performance from our computational modeling. It is important to note a few general points here: 1) These errors show similar general patterns when either language is the L2 (Chinese or English), but the specific errors are language-dependent; 2) Our modeling parameters were held constant; being able to simulate both languages as the target L2 while holding modeling parameters constant shows an important and flexible feature of computational modeling; 3) We determined our modeling errors through examination of BMUs in the SOM maps: 1) If the activated unit on the semantic map is the BMU of the correct word meaning, it is taken that our network correctly comprehends this word; otherwise, the network makes a comprehension error; 2) If the activated units on the phonemic map match the BMUs of the phonemes making up the word in the correct order, it is taken that our network correctly produces this word; otherwise, the network makes an error in production.
Comprehension Errors
In Tables 1 and 2, we listed the average numbers of different types of comprehension errors that DevLex-II made at different stages of the late L2 learning condition (Table 1) and early L2 learning condition (Table 2). The results were averaged over 5 simulations trained with different random seeds, and the standard deviations were listed within the parentheses. Overall, four types of patterns were observed.

TABLE 1. Number of each type of comprehension errors in L2 (English) across different late L2 learning stages. Results averaged over 5 simulations for each learning stage. Standard deviations are listed within the parentheses.

TABLE 2. Number of each type of comprehension errors in L2 (English) across different early L2 learning stages. Results averaged over 5 simulations for each learning stage. Standard deviations are listed within the parentheses.
First, our model for late L2 learners showed a large proportion of errors related to phonological confusions/interferences, errors that were mainly with the comprehension of L2 (45.2 out of 104.8 total errors at the final stage in Table 1). For example, an activation of the English word think on the input phonology map led to the activation of sink on the semantic map. This type of error might be caused by the similarity in sound between two words in the L2 (and their representation similarities on the input phonology map): the/θ/versus/s/difference in L2 English does not exist in L1 Chinese, and therefore/s/took the place of/θ/. Other examples included stove-stone, bump-jump, glass-grass, pull-pool, she-see, bug-big, light-like, blue-blow, chair-hair, wash-watch (English as L2); qing3 (“invite”)-qin1 (“kiss”)2, zang1 (“dirty”)—zhang1 (“piece”), bai2 (“white”)—bei4 (“carry”) (Chinese as L2), and many more.
Second, semantic similarities also led to a large proportion of comprehension errors in our model (32.2 out of 104.8 total errors at the final stage). For example, an activation of the Chinese word ge1ge (“older brother”) on the input phonology map led to the activation of di1di (“younger brother”) on the semantic map. This is an example of incorrect comprehension (from phonology to semantics) due to within-language semantic interference. Most of these errors were within the L2 itself, such as dog-cat, car-boat, pen-pencil, kick-drop, cut-tear, a-an, bench-couch; and hei1 (“black”)-lv4 (“green”), mi4feng1 (“bee”)-ma3yi3 (“ant”), ya1zi (“duck”)-gong1ji1 (“rooster”). Such types of error might reflect the overlap/similarities between the representations of the words on the semantic map of our model.
Third, for the late L2 condition, certain comprehension errors could not be clearly categorized (25.4 out of 104.8 total errors at the final stage). For example, some activations on the input phonology map were not able to generate the activations of a BMU associated directly with a meaning (i.e., the model failed to comprehend the sound of the word). Also, some word forms evoked the activation of word meanings that were not related in any meaningful way. Examples included will-jelly, nurse-little, sun-cheese. Such errors were rare in our early L2 learning condition (see Table 2) and might reflect the unstable/inaccurate form-to-meaning links inconsistently built under our late L2 learning condition.
Finally, a very small proportion of comprehension errors were due to cross-language similarities (6.4 out of 104.8 total errors at the final stage as shown in Table 1). Most of them were due to phonetic similarities (i.e., cross-language homophones) and originated from L2: a-e2 (“goose”), tongue-tang2 (“sugar”), hair-hei1 (“black”), ear-ye2ye (“grandpa”), when-wan3 (“bowl”) (see Li and Farkas 2002, for similar errors). Cross-language comprehension errors due to semantic similarities were found too, but their occurrence was extremely rare and only in the direction from L2 sound representation to L1 semantic representation. We only observed a few examples at the beginning of late L2 learning condition, such as Mao1 (“cat”)-bear; shou3 (“hand”)-toe (Chinese as L2), and kiss-qin1 (“kiss”), owl-ya1zi (“duck”), touch-reng1 (“throw”) (English as L2).
Overall, in Table 1, we could observe the increment of total number of comprehension errors as more L2 words entered the training. In addition, roughly similar proportions of the three main types of comprehension errors occurred across the training stages of late learning condition (with a relative larger portion of phonological confusions towards the end of learning).
For the early L2 learning shown in Table 2, it is interesting to note that the total number of comprehension errors stayed at a low level across most stages, and most errors were regular confusions in either meaning or sound (13.4 and 12 out of 28.2 total errors respectively at the end), and the proportion of uncategorized errors was small (4.4 out of 28.2 at the end)3. The cross-language comprehension errors occurred rarely under the early L2 condition (0 at the end). Such a pattern was different from the late L2 learning where uncategorized errors were common, and it implied that the unstable form-to-meaning links (which often caused uncategorized errors) might not be a main driving force for early L2 learners’ comprehension errors (which was low in number in the first place). In addition, from a developmental perspective, our model made fewer comprehension errors generally as L2 learning progressed.
Production Errors
In monolingual simulations (see Table 1 in Zhao and Li, 2013), DevLex-II showed lexical confusions, omissions, replacements, and incorrect sequencing of phonemes in production. The current bilingual simulations also showed many of the same patterns. However, there were certain production error patterns unique to our bilingual simulations, in particular for the late L2 learning condition. In Table 3, we present a summary of four types of production errors made by DevLex-II under both the late and early L2 (English) learning conditions: phoneme confusions, phoneme replacement, incorrect sequencing, and semantic confusions. The results were the averaged values across 5 simulations trained with different random seeds, and the standard deviations were in parentheses.
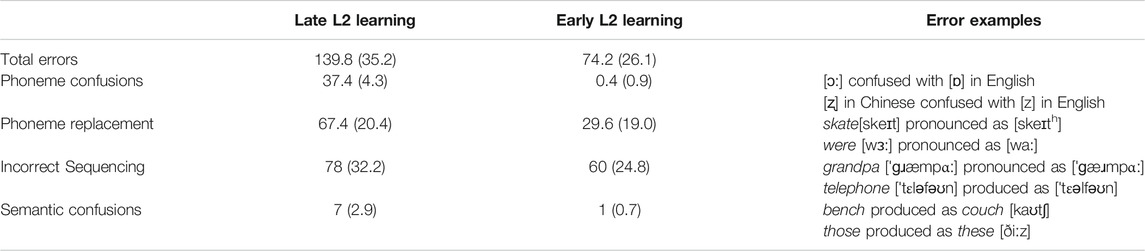
TABLE 3. Number of each type of production errors in L2 (English). Results averaged over 5 simulations for each learning condition. Standard deviations are listed within the parentheses.
Under the late L2 learning condition, a large amount of production errors was due to confusions on the phonemes unique to L2 (37.4 out of 139.8 total errors). As shown on the row labeled as “phoneme confusions”, sometimes our late L2 learning simulations could not distinguish between L2-unique phonemes and similar but not identical phonemes in L1 on the output phonemic map. Examples of such confusions included an indistinguishable English phoneme [z] (as in zebra) with a Chinese phoneme [ʐ] (as r in ri4, “sun”), and English phonemes [ɔ:] (as in born) or [ɒ] (as in pot) with [o] (as o in wo3, “I”) in Chinese. Other times our late L2 learning model could not distinguish between different L2-unique phonemes that are themselves similar on the output phonemic map. For example, English phonemes [ɔ:] as in born and [ɒ] as in pot are two phonemes not found in Chinese and therefore, they were often confused with each other on the output phonemic map when English was learned as L2. Other examples included [z] as in zebra with [ð] as in then (see Cutler et al., 2004 for phoneme confusion patterns of non-native listeners of English). Similarly, error patterns of this type of phonemic confusion were observed when Chinese was learned as L2 in our simulations. For instance, c ([ts’]) and ch [tʂ’] are two phonemes not found in English and therefore they were often confused with each other on the phonemic map. Other examples included confusion of phonemes such as j, q, x ([tɕ], [tɕ’], [ɕ]), z and zh ([ts], [tʂ]), s and sh ([s], [ʂ]). Such phonemic confusions were very rare under the early L2 learning condition though (0.4 out of 74.2 total errors), given that the output phonemic map of early L2 learning was often much clearer and more organized (see the discussion section). These simulated patterns match up well with empirical findings (Yang and Yu, 2019; Wang and Chen, 2020; see further error analyses of an SLA corpus in next subsection). They are also consistent with speech learning theories indicating that early learners can create new phonetic categories more easily than late learners, and that such differences are due to the stabilization of the phonetic representation of L1 versus L2 over the lifespan of learning (see Flege, 1995; Flege, 2007). Flege’s Speech Learning Theory also suggests that phonemes in the L2 that are similar to the L1 can actually cause more difficulty in learning, which is supported by our phonemic confusion data.
As shown on the row labeled as “phoneme replacement”, some of the production errors were due to the phonemes in the pronouncing sequence replaced by other phonemes. For example, were [wɜ:] was wrongly pronounced as [wa:] in one simulation with English as L2. Similar examples included [ʃea] for share [ʃeə], [skeɪth] for skate[skeɪt], [pʊi:] for poor [pʊə], and so on. Incorrect sequencing of phonemes in production was another salient error pattern that could be found in our simulations. For example, grandpa [ˈɡɹæmpαː] was wrongly pronounced as [ˈɡæɹmpαː] in one simulation with English as L2. Similarly, telephone [ˈtɛləfəʊn] was wrongly produced as [ˈtɛəlfəʊn] in another simulation. These types of patterns could also be found in the empirical error analyses of a Second Language Acquisition (SLA) corpus as shown in next section.
It is worth noting that incorrect sequencing could be accompanied with phoneme replacement and phoneme confusions, and it happened more often with words of greater length (such as multisyllabic words). For example, in one of the simulations, closet ['klαːzət] was pronounced as ['klαːəzd]. The effect of word length on lexical production was reported in our previous monolingual simulations and could be associated with the impact of individual working memory capacities on word articulation (see Li et al., 2007). This type of error reflects the challenges that language learners face when they need fine control of their articulatory organs to coordinate and execute sequential sound patterns, especially when the sequence belongs to an unfamiliar language (see the sensorimotor integration hypothesis from Hernandez and Li, 2007). Both late and early L2 learning showed a large amount of incorrect sequencing (78 and 60 respectively) along with phoneme replacement errors, but for the early L2 learning, such errors occurred less frequently and were more evenly distributed between L1 and L2.
Finally, our simulations also showed production errors caused by the confusions at the semantic level. Such errors were most salient in the late L2 learning condition and could be associated with the fuzzy L2 representations on the semantic map. For example, in one simulation, the word bench was wrongly named as couch [kaʊtʃ] and the word those was produced as these [ðiːz]. A close examination of the trained semantic map showed that the conceptual representation of bench and couch activated the same BMU on the map; therefore, they were confused in the first place during lexical production. Given that the late L2 learning condition produced much denser and fuzzier L2 semantic representation than the early L2 condition, it carried more semantic confusions as such (see discussion below on displaying and measuring density in representation). Such a type of semantic confusion could also be found in empirical studies. For example, contributors of Swan and Smith (1987), as quoted in Swan (1997), collected many vocabulary confusions at the semantic level (e.g., think/hope, beat/hit/strike/knock) in second language learners’ L2 (English) production. An example observed from Chinese L2 learners of English, as quoted in Swan (1997), was that they often had confusions on the usage of “small verbs” such as come/go, do/make, bring/take, which are semantically similar but with subtle differences. Similar examples such as 爱love/喜欢like and 预约appointment/约会date could also be found in American L2 learners of Chinese (Yuan, 2017).
Production Errors in SLA Corpus
The production errors shown in our model could also be compared with those from an SLA corpus (COPA Corpus on TalkBank, Zhang, 2009a), which included the elicited responses from L2 learners of Chinese to a fixed series of questions designed to measure the growth of their proficiency in Chinese (Zhang, 2009b). Specifically, we analyzed data from 46 learners of Chinese with English as their L1 in the corpus, aged between 19 and 56 years (M = 27.79, SD = 9.96). Although AoA information was not indicated in the corpus and despite many participants could speak Chinese relatively fluently, these participants were clearly not balanced bilingual or early L2 learners of Chinese. In the COPA Corpus, each participant’s production errors at the word level were clearly annotated with error codes of the CHILDES (Child Language Data Exchange System; MacWhinney, 2000), and we conducted a frequency analysis of these errors using the CLAN program of the CHILDES.
As shown in Table 4, three main types of production errors were annotated in COPA Corpus by Zhang (2009). They were phonological errors (coded with [*p]), semantic errors (coded with [*s]), and neologisms (coded with [*n]). Generally consistent with our simulation results from late L2 learning condition (see the sections above), there were overall more phonological errors than the semantic errors [t (45) = 2.21, p = 0.032 in a paired-samples t test]. Within the phonology category, many errors were caused by the confusions of tones, a salient feature of Chinese as a tonal language but a difficult linguistic feature for learners of L2 Chinese (Hao, 2012; see also; Pelzl et al., 2021). We could also observe many phonemic confusions/replacements as in previous simulations. Examples included, but not limited to, s[s] for sh[ʂ], c[ts'] for ch [tʂʻ], j[tɕ] for q[tɕʻ], an[an] for ang [αŋ]. Incorrect sequencing could also be observed in the corpus. For example, a participant mistakenly pronounced the word xin1xian1 (新鲜 fresh) as xian1xin1.4
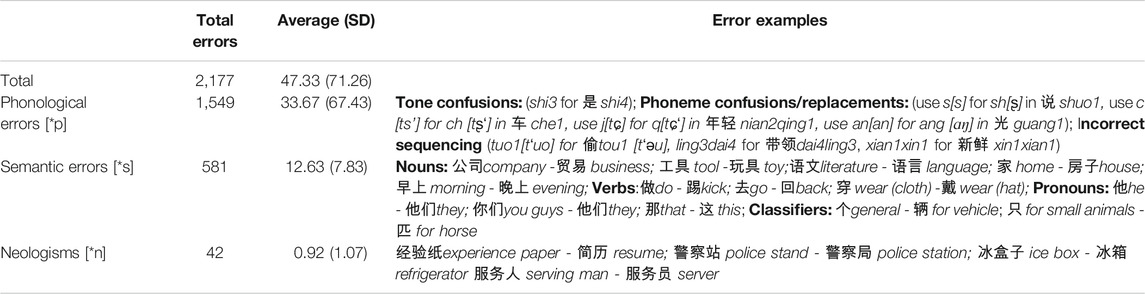
TABLE 4. Total and average number of production errors shown in second language learners’ L2 (Chinese). Data is averaged over 46 participants (with English as L1) from the COPA Corpus on TalkBank (Zhang, 2009a).
Similar to our simulations in the late L2 learning, semantic errors in the COPA Corpus included confusion of a word with another word more or less semantically related to it, such as 那that-这 this, 穿 wear (cloth)-戴 wear (hat), 工具 tool-玩具 toy, and 只 classifier for small animals-匹 classifier for horse. Understandably, the confused words were often in the same grammatical category. As discussed below, we believe that this reflects the fuzzy and dense representations within the semantic categories of late L2 learners. There were also a small number of neologisms in the COPA Corpus, which were participant-generated pseudo words based on meaning of the target words. However, phonological information from the target word might also be mixed in the neologism, given that participants might keep the most part of a compound word in its original form but only changed one morpheme/character into a similar but inappropriate morpheme, like the case in using 警察站 (police stand) for 警察局 (police station). Neologisms were not able to be simulated in our current study given that DevLex-II does not have a separate morpheme layer, although this could be one of the future research directions.
Displaying and Measuring Density in Representation
As discussed previously, the topography-preserving property of SOM allows the researchers to visually examine the emergence of lexical-semantic structures in their models. Here we show how lexical items from the two languages are represented under the late L2 learning (Figure 2) and early L2 learning (Figure 3) conditions. Figure 2A shows a semantic map5 at the final stage of a late L2 learning condition. The circled gray regions indicate the nodes that represent the L2 (English) words and the other regions represent the L1 (Chinese) words learned by the model. Inspecting the bilingual representations, we can find that the words are not evenly represented for L1 and L2 by the model. Some areas are very dense while others very sparse. Lexical items in L2 are represented more densely on the maps in a more disorganized fashion, and they have higher chances to be confused with each other (being projected to the same BMU). Compared with L1 words, L2 words occupy only small and fragmented regions (neighborhoods on the map), dispersing throughout the map. In addition, the boundary between L1 items and L2 items on the maps is fuzzy.
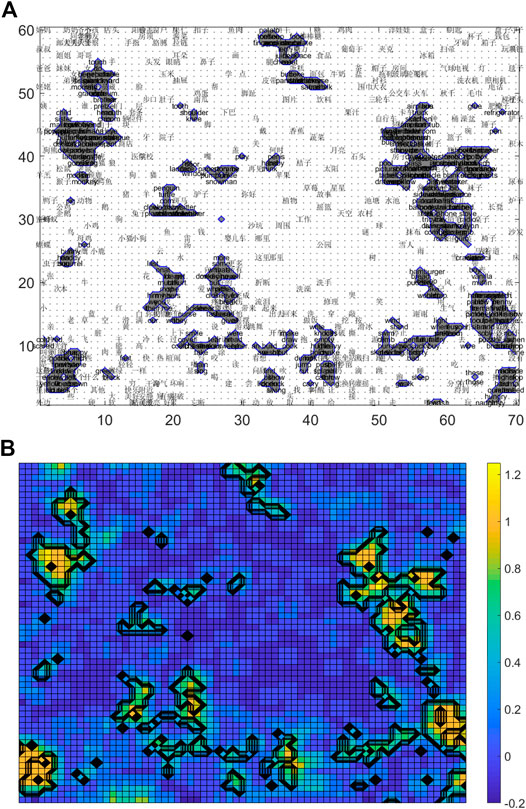
FIGURE 2. Bilingual lexical-semantic representations of late L2 learning in the DevLex-II model. Circled areas correspond to L2 (English) words. Top: (A) Semantic map; Bottom (B) Heat map with density measure of each node, brighter color indicates higher density level.
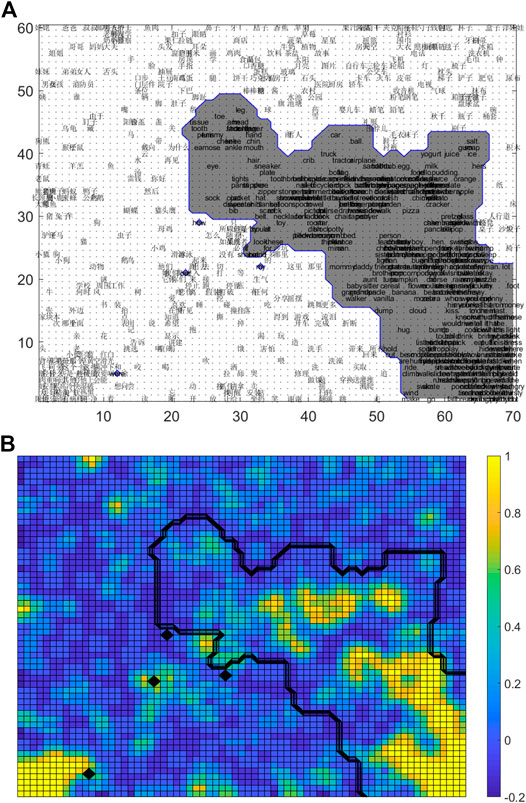
FIGURE 3. Bilingual lexical-semantic representations of early L2 learning in the DevLex-II model. Circled areas correspond to L2 (English) words. Top: (A) Semantic map; Bottom (B) Heat map with density measure of each node, brighter color indicates higher density level.
To explore the differences in the density of the regions that L1 and L2 occupied on the map, we developed a method to calculate the density of units in their semantic and phonological neighborhoods. Specifically, we defined a unit’s density as the number of words represented as BMUs in its direct neighborhood divided by the total number of units of its neighborhood, which is usually nine, but can be six or four, depending on whether the tested unit is on the border or in the corner of the map. The value of this density measure ranges from 0 when a unit has no words represented as BMUs in its neighborhood, to 1.0 when its entire neighborhood including itself are occupied by words. The results of this calculation showed that under the late L2 learning conditions the density of the L2 regions reached a very high level (0.64 and 0.75 on average for the semantic maps and phonological maps, respectively). Figure 2B shows a heat map of Figure 2A with each unit’s density level represented by color. The high density of the small and isolated regions occupied by L2 can be clearly observed with the bright colors, reflecting the compact and fuzzy representation of L2 items.
Figure 3 presents a semantic map and its corresponding heatmap under the early L2 learning condition. Comparing it with Figure 2, we can find that the relative onset time of L2 vs. L1 plays an important role in modulating the overall representational structure of L2. For the early L2 learning condition, our network shows clear distinct lexical representations of the two languages at both the semantic (Figure 3A) and phonological level (not shown here). The results imply that the early learning of two languages allows the system to easily separate the lexicons during learning. In addition, as shown by the heatmap in Figure 3B, L2 representations are less crowded with lower density measures (bluer in color) on the L2-occupied regions on both phonological and semantic maps (0.35 and 0.29 respectively).
To further explore the density distributions of L2 and L1 on the maps under different learning conditions, a simulation-based 2 by 2 mixed-design ANOVA was conducted with learning condition (early vs. late) as the between-subject factor and language (L2 vs. L1) as the within-subject factor. The data were based on 10 simulations (5 for early and 5 for late learning), and only results for semantic maps are reported here. Significant main effects were found for both factors. The main effect of learning condition [F (1,8) = 369.51, p < 0.001, partial η2 = 0.979] suggested that overall late L2 learning generated more crowded representation than early L2 learning. The main effect of language was significant too [F (1,8) = 900.20, p < 0.001, partial η2 = 0.991]; and it showed that L2 had more crowded representation than L1.
A significant interaction between learning condition and languages can also be observed in our simulations [F (1,8) = 179.70, p < 0.001, partial η2 = 0.957]. The interaction can be clearly found on the line graph of the average density levels of the four groups (Figure 4). Specifically, a post-hoc test on the simple effects of learning condition showed a significantly (p < 0.001) more crowded L2 representation under the late L2 learning condition (M = 0.64, SD = 0.025) than under the early L2 learning condition (M = 0.29, SD = 0.043); however, there is no significant difference (p = 0.999) between L1 density levels under the two learning conditions (L1 representations are always clear).
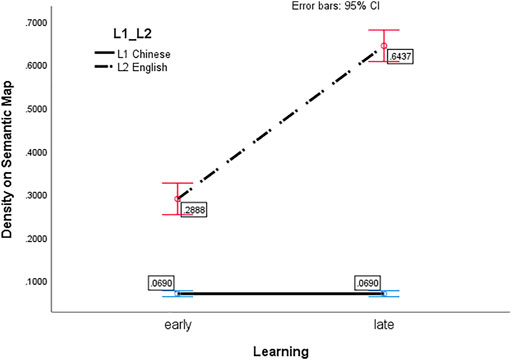
FIGURE 4. A line graph of the average density levels of the L1 and L2 regions on the semantic map under the two learning conditions.
General Discussions
The comprehension and production errors as shown in our bilingual learning models reflect the dynamic interactions among bilinguals’ two lexicons in the DevLex-II model. We suggest that these errors mainly come from two sources, namely the fuzzy linguistic representations of L2 on each map and the inaccurate/unstable connections between the maps. Such considerations based on computational models are informative to our understanding of the nature of fuzzy representations in the bilingual lexicon.
Fuzzy L2 Representations
One possible source of these error-prone difficulties in production and comprehension could be the fuzzy L2 representations of the L2 phonology and semantics, and the output sequence maps. As can be found in Figure 2 and the associated analyses of word density, there is a very compact and fuzzy representation of L2 items under the late L2 learning condition. There are two ways in which we characterize the fuzziness in the dense L2 areas: on the one hand, many L2 words are densely (and sometimes imprecisely) represented on the adjacent units and even by the same BMUs; on the other hand, the organization of L2 lexicon is fuzzy in the sense that the boundaries among word categories are blurred and overlapped, especially for those words that are similar phonologically or semantically. As a consequence, the retrieval of the sound or the semantic content of a word could be difficult because the competition between words is strong and could thus result in a higher confusion rate, contributing to the higher number of comprehension errors of L2. Word density is relatively low for the L1 words in general, and the organization of L1 representations is clearer and more precise. They are more robust than words in high density areas and thus more resistant to competition. Consequently, a clearer, more precise, and less crowded lexical-semantic representation of L2 makes early bilinguals less prone to lexical errors in their L2, compared with late L2 learners.
In late L2 learning, L2 representations are fuzzy because they are often parasitic on or auxiliary to those of L1 words, in the sense that the locations of many isolated L2 words depend on how similar they are to the L1 words in meaning (for the semantic map) and in sound (for the phonological map). For example, on the semantic map shown in Figure 2A, the English word go and walk is located next to the Chinese verbs like pao3 (“run”) and pa2 (“crawl”) since they are similar in meaning. Similar examples could also be found on one phonological map (not shown here): English word cat is close to the Chinese word kai1 (“open”) since they sound similar; other examples include ear close to ye2ye (“grandpa”), hair close to hei1 (“black”). Interestingly, these are the places where few cross-language comprehension errors occurred at the beginning stages of late L2 learning. As shown in Table 1, the ratio of such cross-language comprehension errors over the total number of errors gradually decreases as learning progresses, indicating a gradually reduced parasitism of L2 on L1, even for the late L2 learning condition. Another interesting finding is that the cross-language interference is unidirectional, that is, the comprehensions of L2 words are affected by L1 knowledge only. There is little evidence of direct interference from L2 to L1 in our simulations. This is perhaps because in late L2 learning, the system has not reached a level of proficiency to produce backward L2-to-L1 influence. Such findings are consistent with the theoretical perspectives of emergentism, according to which parasitism arises not simply as a function of AoA, but as a function of the interaction between L1 and L2 (Li and Zhao, 2013; Hernandez et al., 2005). Specifically, the bilingual’s L1 may be “entrenched”, such that the lexical structure established early on becomes resistant to radical changes when L2 learning occurs late in life, causing only L2 to be parasitic on L1 rather than the other way around.
The compact and fuzzy L2 representations on the semantic map could also contribute to more production errors of L2. As shown in Table 3, semantic confusions (e.g. bench-couch) may generate production errors between synonyms. These two words are represented by the same BMU on the semantic map in one simulation with English as the L2, indicating that our model confuses these two L2 words in the first place. As a result, the model produces the sound of a wrong but semantically related word. A real-world example of this type of error would be that a participant mistakenly called a bench as couch in a picture naming task in her L2 (see similar examples in McMillen et al., 2020). Additionally, for those L2 words that are not overlapped with each other but are close enough at the semantic level, the high competition among them could result in shorter reaction times and less accuracy when being produced in picture naming tasks (Gollan et al., 2008), or result in higher rate of tip-of-the-tongue state in L2 (Kreiner and Degani, 2015). As discussed earlier, such production errors can be found in real SLA corpus as shown in Table 4.
On the output phoneme map, confusions between similar phonemes were also found, and these confusions contribute to many production errors as described above. Specifically, in late L2 learning, the subtle differences between some L2-unique phonemes are not highly distinguishable in a system that has already committed itself to the L1 phonemic inventory, thus these similar phonemes are projected to the same BMU on the map. Also, some of the new phonemes in L2 are conveniently “attracted” to similar but not identical L1 phonemes, acting like the “magnets” in the phonemic space for late L2 learners. Phonological errors shown in Table 4 also provide many such examples with Chinese as L2. This pattern is highly consistent with the well-documented findings that adult L2 learners often have greater difficulties in accurately perceiving or producing the phonemic contrasts that do not exist in their L1 (e.g.,/r/and/l/ for Japanese learners of English, Flege, 1995; Flege, 2007; see also Zhang and Yin, 2009; Han, 2013 for detailed discussions on the commonly observed pronunciation problems of Chinese learners of English as L2).
Unstable Connections
Another major source of L2 lexical errors could be the weak or inaccurate form-to-meaning or meaning-to-sequence links between the maps, simulating the unstable connections between these different linguistic aspects in real language learning situations. The fuzzy L2 presentations again may contribute to some failures of building reliable between-map links. In the DevLex-II model, associative connections between maps are trained via the Hebbian learning rule, a biologically inspired mechanism, whose success requires a consistent co-occurrence of different linguistic aspects belonging to the same word. Under the late L2 learning condition, due to the fuzzy boundaries between L2 and L1 and within the compacted L2 region, BMUs corresponding to the same word may be subject to quick change of their coordinates on the maps. As a consequence, associative connections might be weak or inaccurate and cannot overcome the randomness in the model (which is generated by the connections’ initial random weights). Indeed, Cook et al. (2016) showed that a fuzzy nonnative phono-lexical representation may lead to inaccurate form-to-meaning mappings in a Pseudo-Semantic Priming (PSP) task of L2 for American adult L2 learners of Russian. Many uncategorized comprehension errors occurred in late L2 learning (see Table 1) may be caused by these unreliable associative connections. It is worth noting that, under the early L2 learning condition, the boundaries between and within the two languages are much clearer on both phonology and semantic maps (Figure 3), thus effectively reducing the number of uncategorized (or arbitrary) comprehension errors.
Adult L2 learners often face a big challenge in adjusting themselves to better coordinate their articulatory apparatus to execute sequential sound patterns in an unfamiliar language. Different from comprehension, the articulation of sounds must be a sensorimotor process, and the accuracy of L2 pronunciation depends on the speaker’s motor control and effortful coordination of the articulatory apparatus (tongue, lips, jaw, larynx, etc.). The difficulties in building correct meaning-to-phonemes links for L2 words in our late L2 learning condition reflect this challenge, especially for words with considerable length. The longer the target word is, the more ordered one-to-more links the model needs to learn, and the higher the chance of incorrect sequencing of phonemes and the replacement of certain ambiguous L2 phonemes (see also similar patterns in child L1 based on the DevLex model; Li et al., 2007).
The Dynamic Interplay Between L1 and L2
The current study clearly demonstrates that to understand effects of AoA on L2 acquisition, the computational modeling approach is important, especially with regard to understanding the dynamic interplay between L1 and L2, the process of L1 entrenchment, L2 parasitism, and the semantic, orthographic, and phonological organizations of lexical structures. Our simulations with the DexLex-II model show that such interactions play an important role in the development of the lexical representation systems across learning stages. When L2 is introduced late relative to L1 learning, L2 learners develop L2 representations parasitic on their L1 representations due to previously consolidated L1. Late L2 representations are fuzzy and under-differentiated in both phonological and semantic systems. Such fuzzy representations contribute to errors in L2 word comprehension and production as shown in our simulations. When L2 is introduced early relative to L1 learning, clear and distinct lexical representations of the two languages emerge in learning, and fewer errors are observed.
Why does late L2 learning lead to lexical representations so different from those in the early L2 learning condition? We believe that this “age” effect in L2 learning may reflect the changing learning dynamics and neural plasticity of the learning system. In the late learning condition, L2 is introduced at a time when the learning system has already dedicated its resources and representational structure to L1, and L1 representations has been consolidated. So, L2 can only use existing structures and associative connections that are already established by the L1 lexicon. This is the sense in which we say that the L2 lexicon is parasitic to the L1 lexicon (Hernandez et al., 2005). In terms of the network’s plasticity, the decrement of the neighborhood sizes on each map at a later stage of learning also significantly constrains its plasticity for radical re-organization. Therefore, as reflected at multiple levels of our model, L2 representations are constrained by well-developed L1 to fragmented areas. In contrast, for early L2 learning, the network still has significant plasticity and can continually reorganize the lexical space for L2. Rather than becoming parasitic to the L1 lexicon, early learning allows the L2 lexicon to present significant competition against the L1 lexicon. Our computational modeling findings suggest that the nature of bilingual representation is the result of a highly dynamic and competitive process in which early learning significantly constrains later development, shaping the time course and structure of later language systems.
Our simulation results are consistent with many previous theoretical frameworks that emphasize the dynamic interactive nature of bilingualism (Hernandez et al., 2005; Hernandez and Li, 2007; Li, 2015). Moreover, newer theoretical formulations highlight this dynamic interaction in terms of emergentism and the ecosystem (see Claussenius-Kalman et al., 2021), influenced by strong competitions between bilinguals’ two languages across a developmental timeline. For late L2 learners, their L1 knowledge and skills are already well established, and highly resistant to change (i.e., “entrenched”) in the face of new input from a new language. Once the structural consolidation in L1 has reached a point of entrenchment, the organization of L2 will have to tap into existing representational resources and structure of L1. According to the “sensorimotor integration hypothesis” (Hernandez and Li, 2007), entrenchment is accompanied by changes in neural plasticity, particularly in sensorimotor integration, such that highly flexible neural systems for developing fine-grained articulatory motor actions and for sequence processing are in deficit or are no longer available. Indeed, recent neurocognitive findings provide evidence that points to differences in neuroplasticity: L2 speakers fail to establish a neural network that connects L2 lexico-semantic representation with sensorimotor integration, in contrast to the L1 network that establishes strong connections between language processing areas and sensorimotor brain systems; see Figure 5 in Zhang et al. (2020). Such findings have significant theoretical and practical implications for L2 learning and representation, as discussed in Li and Jeong (2020) from a neurocognitive perspective.
Future Directions
DevLex-II has been proven to be a powerful tool for studying both monolingual and bilingual lexical development. In the future, we plan to further extend its scope to help us better understand L2 learning and representation.
First, DevLex-II is essentially a developmental model, but it could be extended to integrate both lexical learning and processing, and simulate a wide variety of empirical findings quantitatively. By incorporating a spreading activation mechanism on the semantic map, Zhao and Li (2013) has successfully simulated the effects of age of acquisition on cross-language semantic priming. Similar spreading activation mechanism, if added onto the phonological map and cross-map connections, will have the potential to simulate the phonology-based priming effects and word recognitions as shown in empirical studies such as (Cook et al., 2016; Gor, 2018).
Second, DevLex-II can be used to systemically examine how individual L2 learners’ different cognitive abilities can affect their L2 learning outcomes, given other factors (such as AoA, L2 exposure) being equal. Cognitive scientists have been interested in whether executive control abilities such as working memory and processing speed might predict individual learners’ success in L2 (see Miyake and Friedman, 1998; Kormos, 2015; Wen et al., 2017). In the original monolingual version of DevLex-II, we have successfully simulated individual differences in word production by adjusting our model’s serial-recall ability in phonological short-term memory with a “memory gating parameter” (Li et al., 2007, p.593). Such a strategy could also be used for modeling bilingual processing and is consistent with the current trend of testing computational models on individual differences data (as shown in Peñaloza et al., 2019).
Finally, it will also be interesting to examine if fuzzy L2 representations may eventually be overcome in our model by integrating new modules. Zhang et al. (2020) showed that, compared with L2 speakers, L1 speakers engaged a more integrated brain network connecting key areas for language and sensorimotor integration during lexico-semantic processing. Naturally, a related question would be if late L2 learners can eventually acquire L1-like representations in L2 by utilizing extra cognitive resources or using new learning strategies. In a recent article, Li and Jeong, (2020) proposed the approach of Social L2 Learning (SL2) that focuses on grounding L2 learning in a social interaction framework, which focuses on “learning through real-life or simulated real-life environments where learners can interact with objects and people, perform actions, receive, use, and integrate perceptual, visuospatial, and other sensorimotor information, which enables learning and communication to become embodied.” Most recently, Li and Lan (2021) also pointed out that digital language learning (DLL) may enable “L1-like representations in the L2, through the use of interactive and socially relevant contexts and multimodal/multisensory information”, and such DLL approach may lead to brain changes in both function and structure. Social learning has been well accepted in L1 studies as an important contributor to successful language acquisition in children, and computational modeling research has also compared models with and without social cues. For example, Yu and Ballard (2007) incorporated social-interactive cues that are based on mother-child interactions, suggesting that such a model performed significantly better than models without such cues.
Along this new line of research that highlights social learning, the DevLex-II model could consider methods to build embodied semantic representations into the L2 lexicon by incorporating sensorimotor cues, social cues, and even affective-emotional cues from the learning environment. In addition, one could consider a growing SOM mechanism (e.g., Farkas and Li, 2002) to enable more resources for late L2 for the processing of embodied perceptual-spatial-sensorimotor features. Such studies could incorporate important information based on neurocognitive evidence that involves processing in both the neocortical and subcortical brain regions (see Green and Abutalebi, 2013; Stocco et al., 2014; see Grant et al., 2019, for a review). This new direction using the computational modeling approach, in conjunction with behavioral and neurocognitive studies, will lead to significant insights into the mechanisms and principles underlying individual difference in L2 learning and representation.
Data Availability Statement
The raw data supporting the conclusion of this article will be made available by the authors, without undue reservation.
Author Contributions
XZ and PL both contributed to the conception, design, and data analysis of the study. They contributed equally to the preparation of the manuscript.
Conflict of Interest
The authors declare that the research was conducted in the absence of any commercial or financial relationships that could be construed as a potential conflict of interest.
Publisher’s Note
All claims expressed in this article are solely those of the authors and do not necessarily represent those of their affiliated organizations, or those of the publisher, the editors and the reviewers. Any product that may be evaluated in this article, or claim that may be made by its manufacturer, is not guaranteed or endorsed by the publisher.
Acknowledgments
Preparation of this article was made possible in part by a grant from the Hong Kong Research Grants Council (GRF-15601520) and a Faculty Startup Fund from the Hong Kong Polytechnic University to PL, and a Faculty Development Grant from Emmanuel College to XZ. The authors wish to express gratitude to Jing Wang for help on an earlier draft of the article.
Footnotes
1It is worth noting that there have been many computational studies of other important issues in bilingualism (code switching as one example; see recent publications by Xu et al. (2021), Tsoukala et al. (2021). It is beyond the scope of this paper to review all computational models of bilingualism. Readers are referred to various resources such as the special issue in Bilingualism: Language and Cognition [edited by Li (2013), Shirai (2019) book, and the online bibliography of (Li and Zhao, 2020)].
2The number in the Chinese phonetic transcription indicates the tone of the corresponding word
3Except at its earliest stage when the L1 was not fully developed, most such errors were comprehension failures without generating any meaningful BMU activations on the semantic map
4Please note that this example may also be categorized as semantic errors since the two characters (xin1新and xian1 鲜) of the word are also morphemes by themselves with the meaning of fresh
5Phonological maps are not displayed here, but their representations are similar
References
Bordag, D., Gor, K., and Opitz, A. (2021). Ontogenesis Model of the L2 Lexical Representation. Bilingualism 1, 1–17. doi:10.1017/S1366728921000250
Claussenius-Kalman, H., Hernandez, A., and Li, P. (2021). Expertise, Ecosystem, and Emergentism: Dynamic Developmental Bilingualism. Brain Lang. 222, 105013. doi:10.1016/j.bandl.2021.105013
Cook, S. V., and Gor, K. (2015). Lexical Access in L2: Representational Dificit or Processing Constraint Ml 10 (2), 247–270. doi:10.1075/ml.10.2.04coo
Cook, S. V., Pandža, N. B., Lancaster, A. K., and Gor, K. (2016). Fuzzy Nonnative Phonolexical Representations Lead to Fuzzy Form-To-Meaning Mappings. Front. Psychol. 7, 1345. doi:10.3389/fpsyg.2016.01345
Cutler, A., Weber, A., Smits, R., and Cooper, N. (2004). Patterns of English Phoneme Confusions by Native and Non-native Listeners. The J. Acoust. Soc. Am. 116, 3668–3678. doi:10.1121/1.1810292
Dale, P. S., and Fenson, L. (1996). Lexical Development Norms for Young Children. Behav. Res. Methods Instr. Comput. 28, 125–127. doi:10.3758/bf03203646
Diependaele, K., Lemhöfer, K., and Brysbaert, M. (2013). The Word Frequency Effect in First- and Second-Language Word Recognition: a Lexical Entrenchment Account. Q. J. Exp. Psychol. 66 (5), 843–863. doi:10.1080/17470218.2012.720994
Dijkstra, T., and van Heuven, W. J. B. (1998). “The BIA Model and Bilingual Word Recognition,” in Localist Connectionist Approaches to Human Cognition. Editors J. Grainger, and A. M. Jacobs (Mahwah, NJ: Erlbaum).
Dijkstra, T., and van Heuven, W. J. B. (2002). The Architecture of the Bilingual Word Recognition System: From Identification to Decision. Bilingualism 5 (3), 175–197. doi:10.1017/s1366728902003012
Dijkstra, T., Haga, F., Bijsterveld, A., and Sprinkhuizen-kuyper, I. (2012). “Lexical Competition in Localist and Distributed Connectionist Models of L2 Acquisition,” in Memory, Language and Bilingualism: Theoretical and Applied Approaches. Editors J. Altarriba, and L. Isurin (Cambridge: Cambridge University Press), 48–73.
Dijkstra, T., Wahl, A., Buytenhuijs, F., Van Halem, N., Al-Jibouri, Z., De Korte, M., et al. (2019). Multilink: a Computational Model for Bilingual Word Recognition and Word Translation. Bilingualism 22 (4), 657–679. doi:10.1017/S1366728918000287
Elman, J. L. (1990). Finding Structure in Time. Cogn. Sci. 14, 179–211. doi:10.1207/s15516709cog1402_1
Farkas, I., and Li, P. (2002). “Modeling the Development of Lexicon with a Growing Self-Organizing Map,” in Proceedings of the Sixth Joint Conference on Information Science, March 08–13, 2002. Editor H.J. Caulfield (North Carolina, USA: Research Triangle Park), 553–556.
Flege, J. E. (1995). “Second Language Speech Learning: Theory, Findings and Problems,” in Speech Perception and Linguistic Experience: Issues in Cross-Language Research. Editor W. Strange (Baltimore: York Press), 233–277.
Flege, J. E. (2007). Language Contact in Bilingualism: Phonetic System Interactions. Lab. Phonol. 9. Editor J. Cole, and J. I. Hualde (Berlin, Germany: Walter de Gruyter), 353–382.
French, R. M. (1998). “A Simple Recurrent Network Model of Bilingual Memory,” in Proceedings of the 20th Annual Conference of the Cognitive Science Society. Editors M. A. Gernsbacher, and S. J. Derry (Mahwah, NJ: Erlbaum), 368–373.
Gollan, T. H., Montoya, R. I., Fennema-Notestine, C., and Morris, S. K. (2005). Bilingualism Affects Picture Naming but Not Picture Classification. Mem. Cogn. 33 (7), 1220–1234. doi:10.3758/bf03193224
Gollan, T. H., Montoya, R. I., Cera, C., and Sandoval, T. C. (2008). More Use Almost Always Means a Smaller Frequency Effect: Aging, Bilingualism, and the Weaker Links Hypothesis☆. J. Mem. Lang. 58, 787–814. doi:10.1016/j.jml.2007.07.001
Gor, K., Cook, S., Bordag, D., Chrabaszcz, A., and Opitz, A. (2021). Fuzzy Lexical Representations in Adult Second Language Speakers. Front. Psychol. 12, 732030. doi:10.3389/fpsyg.2021.732030
Gor, K. (2015). “Phonology and Morphology in Lexical Processing,” in The Cambridge Handbook of Bilingual Processing. Editor J. Schwieter (Cambridge: Cambridge University Press), 173–199. Cambridge Handbooks in Language and Linguistics.
Gor, K. (2018). Phonological Priming and the Role of Phonology in Nonnative Word Recognition. Bilingualism 21 (3), 437–442. doi:10.1017/s1366728918000056
Grant, A., Legault, J., and Li, P. (2019). “What Do Bilingual Models Tell Us about the Neurocognition of Multiple Languages,” in The Handbook of the Neuroscience of Multilingualism. Editor J. Schwieter (Wiley-Blackwell), 48–74. doi:10.1002/9781119387725.ch3
Green, D. W., and Abutalebi, J. (2013). Language Control in Bilinguals: The Adaptive Control Hypothesis. J. Cogn. Psychol. 25 (5), 515–530. doi:10.1080/20445911.2013.796377
Grosjean, F., and Li, P. (2013). The Psycholinguistics of Bilingualism. New York, NY: John Wiley & Sons.
Grosjean, F. (1989). Neurolinguists, Beware! the Bilingual Is Not Two Monolinguals in One Person. Brain Lang. 36 (1), 3–15. doi:10.1016/0093-934x(89)90048-5
Grosjean, F. (2013). “Bilingualism: A Short Introduction,” in The Psycholinguistics of Bilingualism. Editors F. Grosjean, and P. Li (Hoboken, NJ: John Wiley & Sons), 5–25.
Hao, Y.-C. (2012). Second Language Acquisition of Mandarin Chinese Tones by Tonal and Non-tonal Language Speakers. J. Phonetics 40, 269–279. doi:10.1016/j.wocn.2011.11.001
Hernandez, A. E., and Li, P. (2007). Age of Acquisition: Its Neural and Computational Mechanisms. Psychol. Bull. 133, 638–650. doi:10.1037/0033-2909.133.4.638
Hernandez, A., Li, P., and MacWhinney, B. (2005). The Emergence of Competing Modules in Bilingualism. Trends Cogn. Sci. 9 (5), 220–225. doi:10.1016/j.tics.2005.03.003
Hochreiter, S., and Schmidhuber, J. (1997). Long Short-Term Memory. Neural Comput. 9 (8), 1735–1780. doi:10.1162/neco.1997.9.8.1735
Jiang, N. (2015). “Six Decades of Research on Lexical Representation and Processing in Bilinguals,” in The Cambridge Handbook Of Bilingual Processing. Editor J. Schwieter (Cambridge: Cambridge University Press), 29–84. Cambridge Handbooks in Language and Linguistics. doi:10.1017/CBO9781107447257.002
Kormos, J. (2015). “Individual Differences in Second Language Speech Production,” in The Cambridge Handbook Of Bilingual Processing. Editor J. Schwieter (Cambridge: Cambridge University Press), 369–388. Cambridge Handbooks in Language and Linguistics. doi:10.1017/CBO9781107447257.017
Kreiner, H., and Degani, T. (2015). Tip-of-the-tongue in a Second Language: The Effects of Brief First-Language Exposure and Long-Term Use. Cognition 137, 106–114. doi:10.1016/j.cognition.2014.12.011
Kroll, J. F., and Stewart, E. (1994). Category Interference in Translation and Picture Naming: Evidence for Asymmetric Connections between Bilingual Memory Representations. J. Mem. Lang. 33 (2), 149–174. doi:10.1006/jmla.1994.1008
Lemhöfer, K., Dijkstra, T., Schriefers, H., Baayen, R. H., Grainger, J., and Zwitserlood, P. (2008). Native Language Influences on Word Recognition in a Second Language: A Megastudy. J. Exp. Psychol. Learn. Mem. Cogn. 34, 12–31. doi:10.1037/0278-7393.34.1.12
Li, P., and Farkas, I. (2002). “A Self-Organizing Connectionist Model of Bilingual Processing,” in Bilingual Sentence Processing. Editors R. Heredia, and J. Altarriba (North-Holland: Elsevier Science Publisher), 59–85. doi:10.1016/s0166-4115(02)80006-1
Li, P., and Grant, A. (2019). Scaling up: How Computational Models Can Propel Bilingualism Research Forward. Bilingualism 22, 682–684. doi:10.1017/s1366728918000755
Li, P., and Jeong, H. (2020). The Social Brain of Language: Grounding Second Language Learning in Social Interaction. Npj Sci. Learn. 5, 1–9. doi:10.1038/s41539-020-0068-7
Li, P., and Lan, Y.-J. (2021). Digital Language Learning (DLL): Insights from Behavior, Cognition, and the Brain. Bilingualism, 1–18. doi:10.1017/S1366728921000353
Li, P., and Zhao, X. (2017). “Computational Modeling,” in Research Methods in Psycholinguistics and the Neurobiology of Language: A Practical Guide. Editors A. M. B. de Groot, and P. Hagoort (Hoboken, NJ: John Wiley & Sons), 208–229.
Li, P., and Zhao, X. (2020). “Connectionism,”. originally published 2012, last updated 2020 in Oxford Bibliographies Online (Linguistics). Editor M. Aronoff (Oxford University Press). Available at: www.oxfordbibliographies.com.
Li, P., and Zhao, X. (2021). Computational Mechanisms of Development? Connectionism and Bilingual Lexical Representation. Bilingualism, 1–2. doi:10.1017/s1366728921000432
Li, P., Farkas, I., and MacWhinney, B. (2004). Early Lexical Development in a Self-Organizing Neural Network. Neural Networks 17, 1345–1362. doi:10.1016/j.neunet.2004.07.004
Li, P., Zhao, X., and Mac Whinney, B. (2007). Dynamic Self-Organization and Early Lexical Development in Children. Cogn. Sci. A Multidisciplinary J. 31, 581–612. doi:10.1080/15326900701399905
Li, P., and Zhao, X. (2013). “Connectionist Bilingual Representation,” in Foundations of Bilingual Memory. Editors R. R. Heredia, and J. Altaribba (New York, NY: Springer), 63–84.
Li, P. (2015). “Bilingualism as a Dynamic Process,” in Handbook of Language Emergence. Editors B. MacWhinney, and W. O’Grady (Malden, MA: John Wiley & Sons), 511–536. doi:10.1002/9781118346136.ch23
Li, R., Faroqi-Shah, Y., and Wang, M. (2019). A Comparison of Verb and Noun Retrieval in Mandarin-English Bilinguals with English-speaking Monolinguals. Bilingualism 22, 1005–1028. doi:10.1017/s1366728918000913
MacWhinney, B. (2000). The CHILDES Project: Tools for Analyzing Talk. Hillsdale, NJ: Lawrence Erlbaum.
MacWhinney, B. (2013). “The Logic of the Unified Model,” in The Routledge Handbook of Second Language Acquisition. Editors S. Gass, and A. Mackey (New York: Routledge), 211–227.
McClelland, J. L., and Rumelhart, D. E. (1981). An Interactive Activation Model of Context Effects in Letter Perception: I. An Account of Basic Findings. Psychol. Rev. 88, 375–407. doi:10.1037/0033-295x.88.5.375
McClelland, J., Rumelhart, D., and the PDP Research Group, (1986). Parallel Distributed Processing: Explorations in the Microstructure of Cognition, Vol. 2. Cambridge, MA: MIT Press.
McMillen, S., Griffin, Z. M., Peña, E. D., Bedore, L. M., and Oppenheim, G. M. (2020). "Did I Say Cherry ?" Error Patterns on a Blocked Cyclic Naming Task for Bilingual Children with and without Developmental Language Disorder. J. Speech Lang. Hear. Res. 63 (4), 1148–1164. doi:10.1044/2019_JSLHR-19-00041
Mikolov, T., Sutskever, I., Chen, K., Corrado, G. S., and Dean, J. (2013). “Distributed Representations of Words and Phrases and Their Compositionality,” in Advances in Neural Information Processing Systems, 3111–3119.
Miyake, A., and Friedman, N. P. (1998). “Individual Differences in Second Language Proficiency: Working Memory as Language Aptitude,” in Foreign Language Learning: Psycholinguistic Studies on Training and Retention, 339–364.
Monner, D., Vatz, K., Morini, G., Hwang, S.-O., and DeKeyser, R. (2013). A Neural Network Model of the Effects of Entrenchment and Memory Development on Grammatical Gender Learning. Bilingualism 16 (2), 246–265. doi:10.1017/S1366728912000454
Pelzl, E., Lau, E. F., Guo, T., and DeKeyser, R. M. (2021). Advanced Second Language Learners of Mandarin Show Persistent Deficits for Lexical Tone Encoding in Picture-to-word Form Matching. Front. Commun. 6, 689423. doi:10.3389/fcomm.2021.689423
Peñaloza, C., Grasemann, U., Dekhtyar, M., Miikkulainen, R., and Kiran, S. (2019). BiLex: A Computational Approach to the Effects of Age of Acquisition and Language Exposure on Bilingual Lexical Access. Brain Lang. 195, 104643. doi:10.1016/j.bandl.2019.104643
Rumelhart, D., and McClelland, J. L. (1986). “On Learning the Past Tenses of English Verbs,” in Parallel Distributed Processing: Explorations in the Microstructure of Cognition. Editors J. L. McClelland, and D. E. Rumelhart, and the PDP Research Group (MIT Press), Vol. 2, 216–271.
Stocco, A., Yamasaki, B., Natalenko, R., and Prat, C. S. (2014). Bilingual Brain Training: A Neurobiological Framework of How Bilingual Experience Improves Executive Function. Int. J. Bilingual. 18 (1), 67–92. doi:10.1177/1367006912456617
M. Swan, and B. Smith (Editors) (1987). Learner English: A Teacher’s Guide to Interference and Other Problems, Cambridge Handbooks for Language Teachers (Cambridge, UK: Cambridge University Press).
Swan, M. (1997). “The Influence of the Mother Tongue on Second Language Vocabulary Acquisition and Use,” in Vocabulary: Description, Acquisition and Pedagogy. Editors N. Schmitt, and M. McCarthy (Cambridge University Press), 156–180.
Tardif, T., Gelman, S. A., and Xu, F. (1999). Putting the “Noun Bias” in Context: a Comparison of English and Mandarin. Child. Dev. 70, 620–635. doi:10.1111/1467-8624.00045
Tsoukala, C., Frank, S. L., Van Den Bosch, A., Valdés Kroff, J., and Broersma, M. (2021). Modeling the Auxiliary Phrase Asymmetry in Code-Switched Spanish-English. Bilingualism 24, 271–280. doi:10.1017/S1366728920000449
Wang, X., and Chen, J. (2020). The Acquisition of Mandarin Consonants by English Learners: The Relationship between Perception and Production. Languages 5 (2), 20. doi:10.3390/languages5020020
Wang, H., Henderson, J., and Merlo, P. (2019). “Weakly-supervised Concept-Based Adversarial Learning for Cross-Lingual Word Embeddings,” in Proceedings of the 2019 Conference on Empirical Methods in Natural Language Processing and the 9th International Joint Conference on Natural Language Processing, Hong Kong, November 3–7, 2019 (EMNLP-IJCNLP), 4419–4430. doi:10.18653/v1/d19-1450
Wen, Z., Biedroń, A., and Skehan, P. (2017). Foreign Language Aptitude Theory: Yesterday, Today and Tomorrow. Lang. Teach. 50 (1), 1–31. doi:10.1017/S0261444816000276
Xu, Q., Markowska, M., Chodorow, M., and Li, P. (2021). Modeling Bilingual Lexical Processing through Code-Switching Speech: A Network Science Approach. Front. Psychol. 12, 662409. doi:10.3389/fpsyg.2021.662409
Yang, C., and Yu, A. (2019). The Acquisition of Mandarin Affricates by American L2 Learners. Taiwan J. Linguist. 17, 91–122. doi:10.6519/TJL.201907_17(2).0004
Yu, C., and Ballard, D. H. (2007). A Unified Model of Early Word Learning: Integrating Statistical and Social Cues. Neurocomputing 70 (13-15), 2149–2165. doi:10.1016/j.neucom.2006.01.034
Yuan, A. (2017). A Brief Analysis on the Common Mistakes Made by American Students in Learning Chinese as A Second Language. Chin. Lang. Teach. Methodol. Technol. 1 (2), 9–16. Article 3.
Zhang, F., and Yin, P. (2009). A Study of Pronunciation Problems of English Learners in China. Asian Soc. Sci. 5, 141–146. doi:10.5539/ass.v5n6p141
Zhang, X., Yang, J., Wang, R., and Li, P. (2020). A Neuroimaging Study of Semantic Representation in First and Second Languages. Lang. Cogn. Neurosci. 35, 1223–1238. doi:10.1080/23273798.2020.1738509
Zhang, Y. (2009a). SLABank COPA Corpus. [Data set]. TalkBank. Available at: https://sla.talkbank.org/TBB/slabank/Mandarin/COPA/engL1 (Retrieved November 9, 2021). doi:10.21415/T5ZK6W
Zhang, Y. (2009b). A Tutor For Learning Chinese Sounds through Pinyin. Pittsburgh, PA: Carnegie Mellon University. Unpublished doctoral dissertation.
Zhao, X., and Li, P. (2010). Bilingual Lexical Interactions in an Unsupervised Neural Network Model. Int. J. Bilingual. Educ. Bilingual. 13, 505–524. doi:10.1080/13670050.2010.488284
Zhao, X., and Li, P. (2013). Simulating Cross-Language Priming with a Dynamic Computational Model of the Lexicon. Bilingualism 16, 288–303. doi:10.1017/s1366728912000624
Zhao, X. (2017). “Connectionism in Linguistic Theory,” in The Oxford Research Encyclopedia of Linguistics. Editor M. Aronoff (New York, NY: Oxford University Press). Available at: http://linguistics.oxfordre.com/view/10.1093/acrefore/9780199384655.001.0001/acrefore-9780199384655-e-69. doi:10.1093/acrefore/9780199384655.013.69
Keywords: fuzzy lexical representation, bilingualism, computational modeling, emergentism, DevLex-II
Citation: Zhao X and Li P (2022) Fuzzy or Clear? A Computational Approach Towards Dynamic L2 Lexical-Semantic Representation. Front. Commun. 6:726443. doi: 10.3389/fcomm.2021.726443
Received: 16 June 2021; Accepted: 13 December 2021;
Published: 21 January 2022.
Edited by:
Svetlana V. Cook, University of MarylandUnited StatesCopyright © 2022 Zhao and Li. This is an open-access article distributed under the terms of the Creative Commons Attribution License (CC BY). The use, distribution or reproduction in other forums is permitted, provided the original author(s) and the copyright owner(s) are credited and that the original publication in this journal is cited, in accordance with accepted academic practice. No use, distribution or reproduction is permitted which does not comply with these terms.
*Correspondence: Xiaowei Zhao, eGlhb3dlaXpoYW9AZ21haWwuY29t; Ping Li, cGkybGlAcG9seXUuZWR1Lmhr