- 1Department of English, Languages and Applied Linguistics, School of Social Sciences and Humanities, College of Business and Social Science, Aston University, Birmingham, United Kingdom
- 2Department of English, Linguistics and Philosophy, School of Arts and Humanities, Nottingham Trent University, Nottingham, United Kingdom
Covert audio recordings feature in the criminal justice system in a variety of guises, either on their own or accompanied by video. If legally obtained, such recordings can provide important forensic evidence. However, the quality of these potentially valuable evidential recordings is often very poor and their content indistinct, to the extent that a jury requires an accompanying transcript. At present, in many international jurisdictions, these transcriptions are produced by investigating police officers involved in the case, but transcription is a highly complex, meticulous and onerous task, and police officers are untrained and have a vested interest in the influence of the transcript on a case, which gives rise to potential inaccuracy. This paper reports the design and results of a controlled transcription experiment in which eight linguistically trained professional transcribers produced transcripts for an audio recording of a conversation between five adults in a busy restaurant. In the context of covert recordings, this recording shares many of the typical features of covert forensic recordings, including the presence of multiple speakers, background noise and use of non-specialist recording equipment. We present a detailed qualitative and quantitative comparison of the transcripts, identifying areas of agreement and disagreement in (a) speaker attribution and (b) the representation of the linguistic content. We find that disagreement between the transcriptions is frequent and various in nature; the most common causes are identified as (i) omission of speech that is included in other transcripts, (ii) variation in the representation of turns, (iii) orthographic variation seemingly motivated by phonetic similarity, and (iv) orthographic variation seemingly not motivated by phonetic similarity. We argue that the variable nature of the transcription of “challenging” audio recordings must be considered in forensic contexts and make recommendations for improving practice in the production of forensic transcriptions.
1 Introduction
Covert audio recordings feature in the criminal justice system in a variety of guises, either on their own or accompanied by video. This can include clandestine ‘undercover’ recordings made by police, serendipitous recordings captured incidentally and recordings made by victims or witnesses on their mobile devices. If legally obtained, such recordings can provide important forensic evidence. They can capture a criminal offence being committed or can contain incriminating (or exculpating) material, including admissions of guilt, involvement, or knowledge of criminal activity. In other words, they can help in determining if a crime has been committed, what that crime is and who might be responsible. However, the quality of these potentially valuable evidential recordings is often very poor and their content indistinct, to the extent that a jury needs an accompanying transcript to assist in two tasks (i) working out what is being said (e.g. in cases of disputed utterances), and (ii) in multi-speaker recordings, working out who is saying what (cf. Fraser 2021a: 416).
At present, in many international jurisdictions, these transcriptions are produced by investigating police officers involved in the case “who are given the status of “ad hoc experts” to facilitate admission of their transcripts as opinion evidence” (French and Fraser 2018: 298). As is now well-documented, most comprehensively in the work of Fraser (e.g., Fraser, 2018a; Fraser, 2018b), current practice is problematic and risks producing unreliable evidence that can mislead the jury and result in miscarriages of justice. Transcription is a highly complex, meticulous and onerous task (Jenks 2013: 259). In a forensic context, although trained linguists and phoneticians can be involved in the production of transcripts, it is often the case that the police are responsible for producing transcripts for potentially incriminating audio, and this gives rise to some important problems (see Fraser 2021b for a nuanced discussion of the relative roles of experts and police in transcription). Police officers are untrained and have a vested interest in the influence of the transcript on a case. At best, this renders their transcripts as liable to being inaccurate. At worst, the effects of cognitive bias are such that they may “perceive something they expect, assume or want to be present” (Fraser 2014: 11).
Fraser (2021a: 428) provides an overview of the challenges facing forensic transcription and offers a solution to these problems:
[T]hat all audio admitted as evidence in criminal trials is accompanied by a demonstrably reliable transcript that sets out the content, provides translations where necessary and attributes utterances reliably to participants in the conversation.
The first step towards achieving this, according to Fraser (2021a: 429), is to ensure that appropriately trained experts in linguistic science create and evaluate forensic transcripts rather than the police. In turn, this requires a branch of linguistic science dedicated specifically to the study of transcription (Fraser 2021a: 429). The current study shares this belief and aims to make a contribution in this direction. The position taken in this paper is that any science of transcription must be committed to observing transcription in practice; describing and explaining the processes and products of transcription; and predicting factors that influence and affect transcription and transcribers. To that end, the analysis conducted in this paper reports on a controlled transcription experiment comparing the transcripts of the same speech recording produced by eight different professional transcribers. It proposes different approaches to comparing transcripts in terms of their similarity and difference and applies these approaches to provide empirical evidence of the extent of variation across transcripts and a categorisation of different sources of this variation. The results of the experiment and the findings of the analysis can be used by forensic transcribers in reflections on their professional practice, to identify any key areas of focus in transcription and provide a basis for future transcription research. The direction of this study is guided by two research questions:
1. To what extent are the eight transcripts different from one another and what are the main sources of variation?
2. What implications do the results have for the practice of forensic transcription?
Prior to the analysis there is a review of relevant literature from linguistics and forensic linguistics, before a necessarily detailed description and justification of the methodological decisions taken. The paper ends with a discussion of findings and implications and a look forward towards future research in the scientific study of transcription.
2 Literature Review
2.1 The Process of Transcription
Linguistic transcription can be characterised simply as the “transfer from speech to writing” (Kirk and Andersen 2016: 291). It is a common procedure in many approaches to linguistic research as well as a range of professional contexts outside of academia, including forensics. Its ubiquity as a method for preparing data in linguistic research has given rise to the identification of a range of challenges that researchers have been contemplating for several decades (see Davidson, 2009, for a review of early transcription literature). For instance, it has been posited that transcription is not an objective process but rather a subjective and selective one: “because it is impossible to record all features of talk and interaction from recordings, all transcripts are selective in one way or another” (Davidson 2009: 38). As such, while some consider transcription as the process of producing “data” (for analysis), others consider transcription to be the first step of analysis in and of itself (Tessier 2012: 447).
The inherent subjectivity and interpretivism of transcription allows for both macro and micro variations among transcribers in terms of the representation of spoken language in written form. Our use of “variation” (rather than “inconsistency”) in this instance follows Bucholtz (2007), who argues that transcription is simply one of many forms of the entextualisation of speech into writing and that, therefore, “there is no reason to expect or demand that it must remain unchanged throughout this process of recontextualization” (p. 802). While we do adopt Bucholtz’ view that variation in transcription should not be viewed as the exception but rather the norm, we do, unlike Bucholtz (2007), seek to “problematize variability” (p. 785) insofar as minimizing the chance that such variability may interfere with evidential processes, for instance by misrepresenting the contents of evidential recordings.
At the macro level, we can consider transcription as a political exercise that interfaces with the transcriber’s world-view, cultural experiences and sociolinguistic biases (Jaffe 2000). There exists also the continuum between what has been termed “naturalism” and “denaturalism” (Oliver et al., 2005); these concepts relate to the extent to which transcription should aim to capture as much of the detail from the speech signal as possible (naturalism) as opposed to the transcription only capturing what is deemed necessary for a particular purpose (denaturalism). Naturalism, which may be considered “excessive” for some purposes (Clayman and Teas Gill 2012: 123), is commonly found in heavily qualitative approaches such as conversation analysis (CA), while transcription lower on the scale of naturalism (e.g., simple orthographic transcription) tends to be preferred in relatively quantitative approaches such as corpus linguistics (Love 2020) (however, even in this context, transcripts are not highly denaturalised, as there is an explicit focus on recording in orthography the exact linguistic content that was uttered, avoiding paraphrasing). This distinction lends itself to variation in transcription notation and formats according to the style of the transcription, as discussed by Bucholtz (2007). As such, there appears to be a consensus that transcription style should vary according to the purpose of the work: “transcriptions should provide the level of detail required for the job they have to do” (Copland and Creese 2015: 196).
At the micro level, there are issues such as the transcriber’s ability to decipher the spoken signal (e.g. due to poor audio quality; see Loubere, 2017), the question of how to select the appropriate orthographic representation of speech signals for which there may be multiple variants, and other sources of potential transcription error (Tessier 2012: 450). These challenges are well-documented, and researchers have discussed the difficulties of transcribing phenomena such as “non-standard” speech (Jaffe 2000), semi-lexical items (Andersen 2016) and the structure of dialogue (Nagy and Sharma 2013), among many others (see Bucholtz, 2007, for a discussion of “orthographic variation”). A crude example of such “orthographic choices” (Nagy and Sharma 2013: 238) is the question of how to transcribe contractions, such as gonna (a contraction of going to). Whether to represent the contraction orthographically (gonna) or standardise it (going to) depends upon the purpose of the transcription. Either way, the transcriber(s) should apply the convention consistently. Typically, it is recommended that transcription conventions be developed prior to transcription, to anticipate such issues and prescribe standards so that transcribers may apply such conventions consistently, thus maximising rigour (Lapadat and Lindsay 1999). For example, in the context of the transcription of filled pauses in orthographic spoken corpora, Andersen (2016: 343) advocates for “a ‘reductionist approach’ in which unmotivated variability is eliminated for the sake of consistency”. Conventions may be reviewed and revised during transcription in an iterative manner, as additional unmotivated variability is discovered; as Copland and Creese (2015) discuss (in the context of ethnographic research), “transcription requires the researcher to be reflective and reflexive so that decisions about transcription are consciously made and can be discussed and defended” (p. 191).
However, while transcription conventions may help to reduce unwanted variability, what they cannot control for is the transcriber’s perception of the original speech signal; “speech perception involves not recognising sounds but constructing them, via a suite of complex (though almost entirely unconscious) mental processes” (Fraser and Loakes 2020: 409). In other words, a convention about whether to transcribe gonna or going to assumes that the transcriber actually perceives the production of the word gonna in the first place, but this might not always be the case. The transcriber may simply mistake one word for another (Easton et al., 2000), and errors like this may be made more likely if there are complicating factors such as multiple speakers, background noise and/or poor audio quality (Love 2020: 138).
2.2 The Problem of Forensic Transcription
It is known that transcription is a highly challenging and subjective process that is influenced by many factors that are unique to (a) individual transcribers and (b) individual speakers. This has potential implications in contexts where the “accuracy” of a transcript is of critical importance, such as in legal cases. In a forensic context, covert recordings can provide powerful evidence, but are often too low quality to be understood by the jury without the assistance of a transcript. Usually, when transcripts are required they are produced by police officers investigating the case who are granted “ad-hoc expert” status (French and Fraser 2018: 298). The production of such transcripts and their presentation to juries can pose a risk to the delivery of justice in two main ways. The first relates to issues of accuracy and reliability of the transcript produced by the police; the second relates to the impact any (inaccurate) transcript can have on jurors’ perception of the content of the recording.
Regarding accuracy and reliability, as has been discussed, producing transcripts of recordings is not a straightforward task, particularly when the recording is of low quality. Therefore, since there is a wide range of factors affecting the accuracy and reliability of forensic transcripts (see Fraser, 2003, for a full discussion of these factors), it is very possible that a police-produced transcript may contain inaccuracy. Notwithstanding the difficulty of perceiving low-quality recording, the skill level and the relationship that police officers have with the material can lead to an inaccurate transcription (Fraser 2014: 10–11). On the one hand, although police officers may be highly trained and skilled in a range of different areas, they likely have no training in linguistics or phonetics and have a lack of reflective practice on speech perception. At the same time, although detailed knowledge of the case, exposure to the material and potential familiarity with the speakers on the recording can be valuable when used in the appropriate way, it can mislead police transcribers rather than help them when producing a transcript (Fraser 2018a: 55; French and Fraser 2018: 300). In the same way as anyone else tasked with listening to and transcribing a spoken recording, police officers rely on “cues” to help them construct words and phrases (Fraser 2021a: 418); that is, they draw on precisely their contextual knowledge of the case, the evidence and the speakers involved when determining what is being said. This can lead to a cognitive bias, over which they have little to no control, which leads transcribers to perceive what they think the recording contains, rather than what it necessarily does contain. Therefore, the police are not independent or impartial transcribers (Fraser 2014: 110) and this can lead to the resultant transcript including content that biases in favour of the prosecution case. This is the argument made by Bucholtz (2009), who demonstrates the ways in which recordings of wire-tapped phone calls between drug dealers are recontextualised in the FBI’s “logs” of these conversations. She states that this process is one which “systematically and dangerously disadvantages the speakers whose words are subject to professional representation” (Bucholtz 2009: 519).
The main challenge facing forensic transcription is that “‘ground truth’ (i.e., indisputable knowledge) regarding the content of the recording cannot be known with certainty” (Fraser 2021a: 428). That is to say that there is no way of knowing precisely what is said in the speech recording, and therefore how this is to be represented or reflected in any transcription. Indeed, it is uncertainty over the content of a recording that is very often the rationale for producing a transcript in the first place. So-called “disputed utterance” cases centre around a section (or sections) of a recording that (1) is potentially evidential or incriminating and (2) causes some disagreement over its content. Fraser (2018b) details a case of this kind in Australia in which a police transcript of an indistinct covert recording included the phrase at the start we made a pact and the defendant in question was convicted of being party to a joint criminal enterprise and sentenced to 30 years in prison. However, after being asked to re-examine the audio recording, Fraser (2018b: 595) concluded that “the police transcript was inaccurate and misleading throughout” and “the ‘pact’ phrase was not just inaccurate but phonetically implausible”. Therefore, this transcript, produced with the intention of assisting the jury, is likely to have misled them. This builds on earlier work by Fraser et al. (2011), who clearly demonstrate the extent of influence that transcripts can have on people’s perceptions and interpretations of ambiguous or disputed recordings. Their experiments, using a recording from a New Zealand murder case, found that participants’ opinions of what was said in the recording changed when they were exposed to different “evidence”, including expert opinions on suggested interpretations as to what the recording said. In other words, once the jury were “primed” to hear certain things in the recording, this had a significant impact on their perception and interpretation of the recorded evidence. It is not only disputed utterances that can be the source of dangerous inaccuracies in forensic transcripts; speaker attribution also causes difficulties. As well as transcribing the content of the talk, police transcripts also attribute specific, potentially incriminating, utterances to specific speakers (Fraser 2018a: 55). This challenge is investigated by Bartle and Dellwo (2015: 230), who report a case from the UK Court of Appeal in which police officers’ identification of speakers in a recording differed from that of two phoneticians. The police officers’ attributions, which were important evidence in the original trial, were ruled as inadmissible and the conviction was overturned.
In summary, it is known that transcription is a highly subjective task that is vulnerable to the influence of transcribers’ level of skill, cultural awareness and internal biases. In the context of forensic transcription, this has the potential to lead to errors in the judicial process. In this paper, we seek to explore how variation in transcription manifests linguistically in the written record of what was said and by whom, with the aim of making recommendations to improve the practice of forensic transcription.
3 Methodology
3.1 Data
This paper reports on the design and results of a controlled transcription experiment in which eight linguistically trained, professional transcribers each transcribed the same audio recording using the same transcription conventions. The transcriptions were generated in the pilot phase of data collection for a large corpus of orthographically transcribed audio recordings known as the Spoken British National Corpus 2014 (Spoken BNC 2014; Love et al., 2017), which was gathered by Lancaster University and Cambridge University Press. The audio recording selected for our experiment is 4 minutes and 4 seconds in length and comprises five adult speakers (3 F, 2 M) having a conversation while dining in a busy restaurant in the north east of England. The recording itself, while not completely indecipherable, contains lots of background noise from other guests in the restaurant, and our assessment of its overall intelligibility is that the recording presents a challenging transcription task. The conversation was recorded using the in-built audio recording function on a smartphone. In the context of covert recordings, this recording shares many of the typical features of covert forensic recordings, including the presence of multiple speakers, background noise and the use of non-specialist recording equipment. Furthermore, the recording was transcribed orthographically, which is a technique commonly used in criminal investigations. It is important to acknowledge that there are some elements of forensic covert recordings that are not simulated here–for example, the device was visible to all speakers (rather than being concealed); all speakers were aware they were being recorded; and, despite the presence of some background noise, the speech signals were not affected by poor quality arising from the recording device being distant from the speakers. Furthermore, the context of transcription is not identical either; our recording was transcribed in a lower-stakes environment than would be the case for forensic transcription, and the transcribers were told beforehand that the recording features five speakers. Therefore, although the recording was not obtained–nor transcribed–in a forensic context, and some elements of our choice of recording may seem advantageous when compared to forensic recordings–we believe there to be enough similarity between our experimental conditions and real-world conditions to warrant use in this study.
As part of the pilot phase of the Spoken BNC2014 compilation, the recording was transcribed independently by eight highly experienced professional transcribers employed by Cambridge University Press. All transcribers are L1 speakers of British English and specialise in producing transcripts for linguistic contexts, for example the English language teaching (ELT) industry. They are based in the south of England and do not share the same accent or dialect as the speakers in our recording; however, they were selected for the Spoken BNC2014 project on the basis that they have proficiency in transcribing a diverse range of varieties of English from across the United Kingdom. All transcribers were trained to transcribe the recordings orthographically and received specialist linguistic training in common features of casual British English speech that can be difficult to transcribe (e.g., contractions). Although the transcribers do not possess forensic or phonetic expertise, they are to be considered the industry standard with regard to detailed orthographic transcription.
Consent for the transcriptions to be used in future research was gained from the transcribers at the time of this work in accordance with the ethical procedures of Cambridge University Press, and permission was granted from Cambridge University Press to re-use the transcripts for the present study.
As shown by Table 1, the length of the transcripts alone ranges from 656–883 words (mean 775) and 82–134 turns (mean 100), demonstrating that there appears to be substantial variation among the transcripts in terms of the amount of linguistic content transcribed.
3.2 Analytical Procedure
In order to gain a nuanced understanding of the nature and possible causes of the apparent variation–not only in quantity but also in quality–we compared the transcripts against each other, identifying areas of agreement and disagreement in (a) the attribution of the speakers and (b) the representation of the linguistic content. What we do not seek to measure in our analysis is accuracy, since no “ground truth” transcript of the recording exists, i.e. there is no set of “correct answers” with which to compare the transcripts. Our analysis is divided into three parts.
3.2.1 Speaker Attribution
In the first part of our analysis, we investigate the consistency with which transcribers performed speaker attribution, which refers to “the annotation of a collection of spoken audio based on speaker identities” (Ghaemmaghami et al., 2012: 4185). Based on previous research on the manual transcription of casual spoken interactions by Love (2020), we expect speaker attribution to be an area of potential difficulty when transcribing a recording comprising more than two speakers, such as the recording used in this study, which has five speakers. Specifically, Love (2020) found that transcribers tend to attribute speaker ID codes with a high degree of confidence, even when inter-rater agreement and accuracy are only at moderate levels; in other words, it is possible (and perhaps likely, with several speakers) that transcribers will unknowingly attribute the incorrect speaker ID codes to a turn on a routine basis–they will “regularly and obliviously get it wrong” (Love 2020: 156). The main reasons for this are likely to be similarities in the accent and/or voice quality of two or more speakers, and insufficiently clear audio quality. In our recording, four of the five speakers (three of which are females of a similar age) have similar northeast English accents, so we expect accent similarity to be a potential cause of difficulty with regard to speaker attribution.
The first step of this part of our analysis involved aligning the turns in each transcript, so that the speaker attribution of each turn could be compared. We did this firstly by separating the turns in the original transcripts from their corresponding speaker ID codes (labelled 1–5), so that they could be viewed alongside each other as columns in a spreadsheet. Secondly, due to differences in the presentation of turns in the transcripts (which we explore in detail in Section 4.3), it was not the case that each turn constituted the same row in the spreadsheet. Some transcribers, for example, split a turn across two lines, with an intervening turn from another speaker–for instance a backchannel–in between; representing a multi-unit turn (Schegloff 2007), while others represented the entire turn on one line. Therefore, the transcripts required editing manually in order to align the turns row by row and facilitate a comparison of the speaker attributions.
The transcripts were produced according to the Spoken BNC2014 transcription conventions (Love et al., 2018), which afforded transcribers three types of speaker attribution to represent the level of confidence with which transcribers could attribute each turn to a speaker:
(1) CERTAIN
mark the turn using a speaker ID code (e.g. “<0211>”)
(2) BEST GUESS
mark the turn using a ‘best guess’ speaker ID code (e.g. “<0211?>”)
(3) INDETERMINABLE
mark the turn according to the gender of the speaker (i.e. “<M>” or “<F>”) or show that many speakers produced the turn (i.e. “<MANY>”)
(Love 2020: 137).
For the sake of analysing inter-rater agreement in this study, the “best guess” codes (those marked with a question mark to indicate lower confidence in their own attribution) were merged with the “certain” codes, i.e., we did not make a distinction between a turn attributed to speaker “4” as opposed to speaker “4?”; we considered both of these as positive attributions of the turn to speaker 4, which contribute to agreement.
Once aligned, we compared the speaker ID codes on a turn-by-turn basis in order to calculate inter-rater agreement for speaker attribution. Using the online tool ReCal OIR (Freelon 2013), we calculated Krippendorff’s alpha (Krippendorff 1970), which, in many fields, is a widely applied measure of inter-rater reliability (Zapf et al., 2016), i.e., it can tell us the extent to which the transcribers are in agreement about speaker attributions. Unlike other commonly used measures of inter-rater reliability between three or more coders (e.g., Fleiss’ kappa, Fleiss 1971), Krippendorff’s alpha (α) accounts for cases where the coders (transcribers, in our case) did not provide a speaker ID code at all. This occurred due to variation among transcribers in terms of the inclusion or omission of entire turns (as discussed in Section 4.3), meaning that there are many cases where some (but not all) transcribers included a particular turn, and therefore indicated a speaker ID code. In other words, some turn “slots” in the aligned transcripts are empty and thus were not assigned a speaker ID code.
Krippendorff’s α ranges from 0.0 to 1.0, indicating the percentage of the speaker ID codes that are attributed with agreement better than chance. Krippendorff (2004: 241) makes two clear recommendations for the interpretation of the alpha:
Rely only on variables with reliabilities above α = 0.800.
Consider variables with reliabilities between α = 0.667 and α = 0.800 only for drawing tentative conclusions.
Based on this, an α of less than 0.667 is to be considered poor inter-rater agreement.
3.2.2 Frequency-Based Lexical Similarity
In the second part, we investigated the extent to which the content of the transcripts, measured in both types and tokens, are shared across the transcripts. Starting with types, we used the detailed consistency relations function in WordSmith Tools (Scott 2020) to calculate the number of types that are present in each pair of transcripts and, among those, the number of types that are shared between each pair. We then calculated the Dice coefficient (Dice 1945) for each pair, which indicates the extent of the overlap between each pair. The Dice coefficient is calculated by dividing the number of types or tokens that is shared among two transcripts by the total number of types or tokens present in both transcripts taken together, as per the following formula:
where J = shared types or tokens; F1 = transcript 1 total types or tokens; F2 = transcript 2 total types or tokens (adapted from Scott 2007).
The resulting Dice coefficient ranges from 0.0 to 1.0 and can be taken as a proportion of overlap between the two transcripts, i.e. the closer the coefficient to 1.0, the more overlap in the types or tokens present in the two transcripts (where 0.0 is no overlap whatsoever and 1.0 is complete overlap).
An admittedly crude measure of similarity between transcripts, what our approach does reveal is the extent to which transcripts differ in the quantity of content they contain. In an ideal world, each transcript would be identical, and therefore they would each fully overlap with each other in terms of the types and the frequency of tokens present (as indicated by a Dice coefficient of 1.0). Thus, differences in the number of types and tokens in the transcripts would be indicative of differences in the transcriptions.
3.2.3 Turn-Based Transcription Consistency
In the final stage of our analysis, we investigated the representation of linguistic content among the transcripts on a turn-by-turn basis. In an ideal world, all eight transcribers would produce identical transcripts of the recording, and this would be maximally desirable in forensic transcription. For that reason, in this analysis, we refer to transcribers being “consistent” with each other when they produce exactly the same linguistic content for a given turn.
Using the aligned transcripts, we compared the linguistic representation of each turn across all transcribers quantitatively and then qualitatively. We started by quantifying the extent to which each version of a given turn was transcribed identically. We did this by comparing the transcription of each turn and counting how many versions of each turn across transcripts were completely identical (out of a possible total of eight, which would indicate perfect agreement across all transcribers). We then counted how many of the turns were matching for each number of transcribers–a match for only one transcriber meant that each version of the transcribed turn was different to the other, i.e., no two (or more) versions matched. In doing so, we considered the presence of empty turn “slots”, as caused by the omission of turns by some of the transcribers. If two or more transcribers omitted the same turn, we did not consider this a form of matching, as we cannot prove that the omission of a turn is a deliberate transcription choice, as opposed to being a result of a transcriber simply not having perceived the turn in the audio recording. Therefore, we deemed this an unreliable measure of consistency, and only considered matching among turns that had actually been transcribed.
This approach provides a broad overview of the consistency of transcription, but it is a blunt instrument, making no distinction between minor and major discrepancies between transcribers; nor does it take into account the nature or apparent causes of the discrepancies. Therefore, our next step was to manually examine each set of turns, qualitatively categorising the main cause of variation for each. This was conducted together by both authors in order to maximise agreement in our coding.
To conduct this analysis, we made some further methodological decisions with regard to features of the Spoken BNC2014 transcription scheme (Love et al., 2018). In the transcription scheme, transcribers are instructed to mark the presence of a turn even if they could not decipher the linguistic content of the turn. For the purposes of our analysis, we disregarded such cases and treated them as omissions, as they did not provide any linguistic content to be compared against other versions of the same turn. Of course, in forensic contexts, for an expert transcriber to acknowledge that a section of speech is not transcribable may be meaningful in some cases; however, our focus is on investigating the linguistic content that has been transcribed, and so we chose to omit turns marked as “unclear” from our analysis. Additionally, we decided to disregard the presence or absence of question marks (the only punctuation character allowed as part of the transcription scheme, besides tags; Love et al., 2018: 37) as a marker of transcription variation, as we focussed solely on the consistency of the linguistic content.
Once each turn was coded according to the main source of inconsistency (where present), these were categorised to form the basis of our discussion in Section 4.3.
4 Results
4.1 Speaker Attribution
Using Krippendorff’s alpha (Krippendorff 1970), we calculated the extent of inter-rater agreement for speaker attribution among the eight transcripts. This revealed that across all transcripts and turns, α = 0.408, meaning that only a little over 40% of the turns were attributed to speakers with better-than-chance agreement. While not a direct measure of speaker attribution accuracy (as no 100% correctly attributed “ground truth” transcript exists), the extent of disagreement between transcribers with regards to speaker attribution is a clear indication of inaccuracy; if two (or more) transcribers disagree about a turn, then at least one of the transcribers must have attributed the turn incorrectly.
The possible implications of such a low level of agreement between transcribers in terms of the representation of linguistic content are explored in Section 5.
4.2 Frequency-Based Lexical Similarity: Types and Tokens
Next, we present the comparison of similarity between transcripts with regard to the types present in each transcript and the number of tokens that are shared. Figure 1 is a heatmap displaying the Dice coefficient values for each pairwise comparison of type overlap between transcripts. The Dice coefficient results range from 0.77 (pairs AH and CH) to 0.90 (pairs DE and EF), with a mean of 0.82, indicating that a majority of types occur at least once in each transcript pair. However, this also shows that (a) across each pair, there are some types (between 10–23%) that occur in one but not the other transcript, and (b) there is a fair amount of variation between pairs of transcripts, i.e., some transcribers are more consistent with some of their fellow transcribers than others.
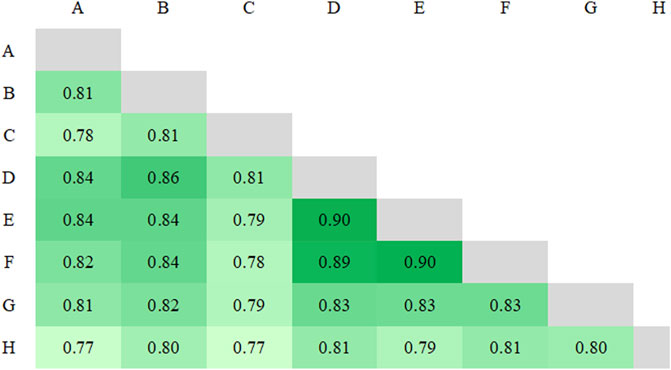
FIGURE 1. Dice coefficient heatmap for types, graded from lower (light green) to higher (dark green) values.
Our analysis of similarity in terms of types is limited in that it does not take into account the frequency of each type; it calculates overlap in a binary fashion, based simply on the presence or absence of types (regardless of how many times the type occurs, if present). Therefore, we repeated our analysis using the raw frequencies of each individual token in the transcripts. The heatmap displaying the Dice coefficients results for each pairwise comparison of token frequency are shown in Figure 2. The values range from 0.76 (pair CH) to 0.88 (pair EF), with a mean of 0.80, indicating a slightly lower range of overlap when compared to that of the comparison of types. Again, while the values indicate a majority overlap between each pair, between 12–24% of tokens that are present in a given transcript are absent in another.
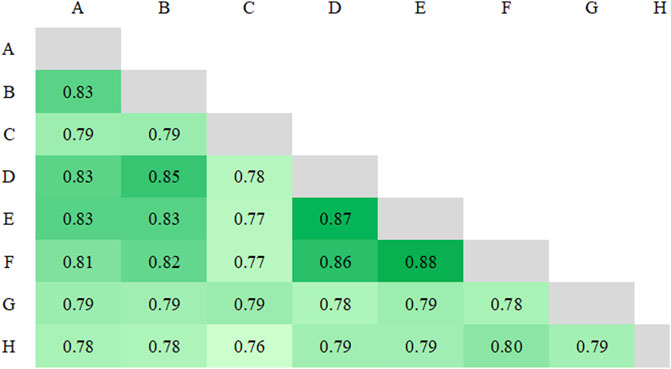
FIGURE 2. Dice coefficient for tokens, graded from lower (light green) to higher (dark green) values.
These comparisons provide a crude indication that there are substantial differences in the content of the transcripts, the specific nature of which requires qualitative examination, which we discuss in the next section.
4.3 Turn-Based Transcription Consistency
Finally, we present the findings of our analysis of the linguistic content on a turn-by-turn basis. Starting with a broad measure of the extent to which turns matched exactly, we found generally low levels of consistency across the eight transcribers in terms of how they transcribed each of the 170 turns. Only five of the 170 turns (2.94%) are transcribed identically by all eight transcribers. All five of these represent minimal speech, with the longest consistently transcribed turn being yeah it is. There are two instances where yeah was transcribed by all eight transcribers and the remaining two turns are the non-lexical agreement token mm. Therefore, this leaves 165 of the 170 turns in which there was inconsistency across the eight transcribers. This ranges from cases in which there was consistency across seven of the eight transcribers, with only one transcriber differing from the others, to cases where all eight transcribers transcribed a given turn differently. Figure 3 shows that the lack of consistency between transcribers is striking. By far the most common occurrence, accounting for 78 of the 170 turns (45.88%), sees only one transcriber “in agreement”, meaning in reality that each of the eight transcribers transcribed the turn differently to the other. In fact, in only 24 of 170 turns (14.12%) do any two of the eight transcribers agree on the content of the recording, and this number reduces as the number of transcribers increases. To generalise, only 39 out of the 170 turns (22.94%) were transcribed consistently by the majority of transcribers (i.e., more than four of the eight).
This binary measuring of (in)consistency on the basis of transcribers producing an identical transcription for each turn masks the fact that, while some versions of the transcribed turns produced by different transcribers are very similar, others vary substantially. In turn, this variation and difference is manifest in a number of different ways–what we refer to here as “sources of variation”. In each of the 165 turns where there was some variation among the transcribers, we qualitatively identified and categorised the source of variation in terms of precisely how the transcripts differed or on what basis they disagreed with one another. We identified the following sources of variation:
• Omitted or additional speech
• Splitting of turns
• Phonetic similarity
• Lexical variation
There is also one instance of inconsistency based on the transcription convention itself; this relates to a part of the recording in which a place name was mentioned, and some transcribers anonymised the place name while some did not. Because this inconsistency relates to the parameters of the transcription set out in the experiment, rather than the content of the recording itself, we will not consider this instance any further. The remainder of this analysis will describe and demonstrate each of the other types of inconsistency, drawing on examples in the data to show how transcribers varied in their transcriptions of the same recording.
4.3.1 Omitted or Additional Speech
Some transcriptions of the turn contained more or less speech content than others. The most straightforward example of this is in turns where some of the transcribers identify and transcribe a speaker turn while others do not. In some cases, there is a high level of consistency across transcribers, and the amount or nature of omitted or additional speech is minimal. In one turn, shown in Table 2, all eight transcribers agreed on the transcription because I can’t eat it any other way. The only variation here is that Transcriber B included an ooh as a preface to the utterance and this is something that was not found in any of the other transcripts.
In other cases, however, there is less consistency across transcribers. In one turn, for instance, four of the eight transcribers agreed that the turn in the recording was don’t get too excited, while the other half of the transcribers not only left that turn blank but did not include don’t get too excited anywhere in their transcript. Table 3 shows an example of this by comparing two of the transcribers. In cases such as this, it is evident that some transcribers are hearing some talk that others are not, or are at least including talk in their transcripts that is absent from others’. This is perhaps the starkest type of difference or inconsistency between transcribers. When tasked with representing the same recording in a transcript, some identify elements of talk that others do not, including full utterances. The implications of this in a forensic context are clear and problematic; it might be that an evidentially significant utterance that is identified in one transcript is missing altogether from another.
Even obtaining two transcripts of a given recording may not suffice in insuring against omitted utterances. There are other instances in our data where an utterance is transcribed by only one of the eight transcribers. For example, Table 4 compares the work of two transcribers and shows that, not only is there a lack of agreement on who spoke the second turn (albeit the transcription of this turn is very similar in terms of content), but each transcript sees an utterance transcribed that does not appear in any of the other seven transcripts. For Transcriber E, this is an attribution of Speaker 2 saying it’s hard to find exactly what this stuff is, while Transcriber H represents Speaker 1 as saying if you just count it you just count the calories. The fact that these utterances are only found in the transcripts of one of the eight transcribers reflects the extent of the problem of omitted/additional speech and the discrepancies in the output of different transcribers. However, it also raises an important question as to which is the best interpretation of such instances. It is unclear whether cases such as these should be viewed as seven transcribers missing talk that one hears, or whether one transcriber is contaminating their transcript with talk that only they (think) they hear. In other words, in a forensic context, a question arises as to whose transcript(s) do we trust the most. There is a judgement to be made as to whether more weight is given to the one transcript that does include an utterance or the fact that seven other transcribers do not report hearing that utterance.
4.3.2 Splitting of Turns
The omission of speech that we have seen above can have further consequences for the transcription. Namely, the decision to include an utterance or not can affect the representation of the turn sequences in the transcript. Table 5 is a case in point. Here, transcribers C and D choose to represent overlapping speech by an unidentifiable but “female” speaker in yeah. The way in which this overlapping speech is included is such that it splits the turn of Speaker 1 before seven hundred and ninety six calories, and this is the same in both transcripts. Transcriber A and B, on the other hand, do not choose to represent the overlapping yeah. Therefore, for them, Speaker 1’s utterance is represented in full and uninterrupted, forcing a difference between their version and those of transcriber C and D.
Such differences in turn splitting do not only appear as a result of the inclusion or omission of overlapping speech. In Table 6, for example, all transcribers transcribed the mm feedback by Speaker 5 (for reasons of space, only four transcripts are shown here). However, despite all transcribers agreeing that some overlapping speech can be heard, they disagreed on how they represented the initial turn; while transcribers A and D chose to place chicken balti as a new turn, transcribers C and H did not. The inclusion and/or placement of overlapping speech in a transcript is an important element of the talk being represented in terms of the implications that it has for other turns and the chronology of the unfolding talk.
A final factor that can result in transcriptions varying in terms of turn completion and turn splitting is variation in speaker attribution. Table 7 shows three transcribers–B, C and F–who vary in terms of to which speaker they attribute a turn. With transcriber B and C, this is a straightforward disagreement; the speaker is identified as Speaker 4 and Speaker 3 respectively. Even though the transcribers disagree on which speaker uttered the turn, they do agree that the full turn was spoken by the same speaker. Transcriber F, in contrast, believes this not to be one turn, but in fact two turns spoken by two different speakers (Speaker 4 and then Speaker 3). Disagreement in terms of “who said what” can have clear implications in a forensic context, and an example such as this brings into sharp focus how differing speaker attributions can result in problematically different transcripts.
4.3.3 Phonetic Similarity
The phonetic similarity between words that gives rise to ambiguity and the resultant challenges to transcription are well-documented. Coulthard et al. (2017: 132) describe a drug case in which there was a dispute over whether a word in a recording was hallucinogenic or German in a police transcript. A second example from Coulthard et al. (2017) is a murder case which involved a transcript of talk from a murder suspect in which the utterance show[ed] a man ticket was erroneously transcribed as the phonetically similar shot a man to kill. The mistaking of one word (or phrase) for another that shares some sound similarities with another word can have serious implications in a forensic transcript, particularly when the words have different meanings and, in the context of the case, those differences are significant. It may be, for example, that an innocuous word is transcribed as an incriminating word.
In our data, we found many instances of transcripts containing different but similar-sounding words in the same turn. For our purposes, phonetic similarity was determined impressionistically on the basis of a judgement of two words sharing phonemes. Table 8 is an example of this, showing a turn in which the same word is transcribed three different ways: *ostensively, ostensibly and extensively. Across all eight transcribers, five transcribed this word as ostensibly, two as extensively and one as *ostensively. It is worth noting that, besides the variation in this word, the content of three transcripts is very similar. Notwithstanding that *ostensively is not a word, although ostensibly and extensively sound similar, they have very different meanings. In this experimental context, this difference is not of great significance, but in a forensic context this difference could have serious implications.
In the case of ostensibly/extensively the choice of either word has implications for the meaning of the full turn. However, the variation across the transcripts is essentially restricted to one word. There are other cases in our data in which longer phrases with phonetically similar properties are found to differ across transcripts. An example of this is in Table 9, where two transcribers vary in their transcription of what’s in and want some. This shows that the influence of phonetic similarity can stretch beyond individual words and affect the perception and transcription of multi-word utterances. In deciding between ostensibly and extensively, contextual cues can be used by transcribers to determine which of the two words makes the most “sense” within the given utterance, and this can influence the choice between two words which sound similar, but which match the semantics of the sentence to different degrees. In the case of Table 8, it might be that ostensibly makes more semantic sense than extensively in the broader context of the talk. In contrast, neither what’s in or want some is the obvious candidate in the context of the turn in Table 9. In such cases, the ambiguity may be insurmountable, and to choose one option over the other would do more damage than marking the word as indecipherable or inaudible.
Finally, where phonetic similarity accounts for variation in transcription between different transcribers, this variation not only has the potential to affect individual words or larger multi-word units (changing the semantics of the utterance in the process), but can also change the perceived pragmatic purpose or force of a given turn. This is exemplified in Table 10, in which the phonetic indistinguishability of can and can’t and light and late can see the same turn be transcribed as a statement by some transcribers (B and C) and a question by others (A). As we saw above, these three transcripts are generally very similar, but diverge on the basis of phonetic similarity. In almost all communicative contexts, the pragmatic difference between a question and a statement is significant in terms of speaker intent and knowledge, both of which can be central to (allegedly) criminal talk.
4.4.4 Lexical Variation
In the previous section, we showed how transcripts can include different versions of the same utterance and how those differences can be accounted for by some sound similarity between the different versions. However, in our data, we also found many instances where the lexical content of the transcribed turns differed in contexts where there was seemingly no phonetic explanation for that difference. We have called this lexical variation.
In Table 11, for example, we see three versions of the same turn across three transcribers. The location of the variation here is in the verb phrase, I could quite go for, I think I might go for and I quite like the look of. The versions by transcribers A and B at least share the same main verb go for, but there is variation in the premodification. What is key here is that there is a clear lexical intrusion between could quite and think I might in the latter that cannot be straightforwardly accounted for by phonetic similarity.
Another, possibly more noteworthy, example of this is shown in Table 12. Here, the three transcripts are consistent in their inclusion of reckons if you go large. However, the key lexical difference is that each of the transcripts has a different pronoun as the subject of reckons: he (D), it (E) and I (G). Although this is a very small lexical difference, it has significant consequences insofar as it attributes agency to different people or things. In a casual conversation such as that recorded here, this may not be important, but the implications of the difference between he, it and I in a forensic context are clear in terms of responsibility and agency.
In terms of agency and action, we not only see inconsistencies in subject allocation but also main verbs themselves. Table 11 above saw variation in the premodification of main verbs, but Table 13 shows how, while six of the eight transcripts include one verb, another includes a different, unrelated verb. There is no phonetic similarity that would explain a disagreement between said and was thinking, and both make sense in context. Incidentally, the difference between saying something and thinking something could be the difference between committing and not committing a criminal offence. Although inconsequential in this recording, the (mis)identification of one verb as another could have substantial consequence in criminal and forensic contexts.
5 Discussion
Forensic transcription faces many difficult challenges regarding the accurate and reliable representation of spoken recordings and the effect that transcriptions have on juries’ perception of the evidence presented. Fraser (2021a) proposes that, in order to address these issues, and to ensure that transcripts used in forensic contexts are reliable, a branch of linguistic science dedicated specifically to the study of transcription is required. This study has aimed to move in this direction by providing empirical evidence from a transcription experiment that observes the extent and nature of variability across transcripts of the same recording. The primary motivation of this experiment and subsequent analysis has been to inform reflective practice and shed light on the process of transcription in new ways.
We have made the argument that the recording used for this experiment shares important similarities with the types of (covert) recordings that are likely to be central to forensic evidence. Relevant factors are that there are multiple speakers and the recording was taken on a smartphone in a busy environment with background noise. However, it should also be emphasised that the eight transcribers compared here did not anticipate their transcriptions to be analysed from a forensic perspective. For example, they were not directed to produce a transcript as if it were to be used as evidence in court. Had such an instruction been given, this may have motivated greater care and attention than was used (or indeed required) for the original task.
In terms of developing methodologies for a science of transcription, this paper proposes three ways in which different transcriptions of the same recording can be compared. We acknowledge that each of these methods have their own unique caveats and areas for refinement, but they are offered here as foundations for future work. They are: (i) measures of inter-rater reliability to evaluate speaker attribution, (ii) the use of the Dice coefficient to measure lexical similarity across transcripts in terms of types and tokens, and (iii) a qualitative approach to identifying patterns in variation at the level of the turn.
The findings of the analysis revealed that, generally, there is a substantial level of variation between different transcripts of the same recording. In terms of speaker attribution, agreement of who said what was just over 40%. In terms of lexical overlap, transcripts averaged 82% similarity in terms of word types, and 80% in terms of tokens. Finally, in terms of consistency across transcripts at the level of the turn, transcribers varied in terms of the speech included or omitted, the representation of overlapping speech and turn structure, and the representation of particular words or phrases, some of which seems to be motivated by phonetic similarity, while for others the source of difference is more difficult to ascertain.
It is clear that the interpretation of (indistinct) audio recordings, forensic or otherwise, is not simply a case of ‘common knowledge’, that can be left in the hands of the police or, indeed, the jury (Fraser 2018c: 101). Our results suggest that even trained transcribers do not produce transcripts “bottom-up”, and that disagreements between transcripts are common. Our interpretation of these findings is emphatically not that transcription is too difficult to be useful, or that forensic transcription should not be carried out at all. Rather, we believe our findings reveal that even professional transcribers vary in their perception and interpretation of recorded talk. The task of improving the practice of forensic transcription should not lie in attempting to completely eliminate variation, but rather to minimize the influence of variation on evidential and judicial processes. As such, at the most basic level, our findings emphasise and underline the argument that transcription should not be undertaken solely by police officers who are untrained in linguistics.
Our aim here is to take into consideration the findings of this work and use them to begin to develop frameworks and protocol for the management of forensic transcription. The extent to which this is achieved or achievable, in many ways, will be determined by future research and practice in this area.
Data Availability Statement
The data analyzed in this study is subject to the following licenses/restrictions: The data used in this study are part of the pilot phase data from the Spoken British National Corpus 2014 project. Requests to access these datasets should be directed to RL, ci5sb3ZlQGFzdG9uLmFjLnVr.
Ethics Statement
Written informed consent was obtained from the individual(s) for the publication of any potentially identifiable images or data included in this article.
Author Contributions
RL: Conceptualization, Methodology, Formal Analysis, Investigation, Data Curation, Writing–Original Draft, Writing–Review and Editing, Project Administration, Funding Acquisition. DW: Conceptualization, Methodology, Formal Analysis, Investigation, Writing–Original Draft, Writing–Review and Editing, Visualization, Funding Acquisition.
Conflict of Interest
The authors declare that the research was conducted in the absence of any commercial or financial relationships that could be construed as a potential conflict of interest.
Publisher’s Note
All claims expressed in this article are solely those of the authors and do not necessarily represent those of their affiliated organizations, or those of the publisher, the editors and the reviewers. Any product that may be evaluated in this article, or claim that may be made by its manufacturer, is not guaranteed or endorsed by the publisher.
Acknowledgments
We are grateful to Dr Claire Dembry for granting us permission on behalf of Cambridge University Press for the re-use of pilot data from the Spoken British National Corpus 2014 project.
References
Andersen, G. (2016). Semi-lexical Features in Corpus Transcription: Consistency, Comparability, Standardisation. Int. J. Corpus Linguistics 21 (3), 323–347. doi:10.1075/ijcl.21.3.02
Bartle, A., and Dellwo, V. (2015). Auditory Speaker Discrimination by Forensic Phoneticians and Naive Listeners in Voiced and Whispered Speech. Int. J. Speech, Lang. L. 22 (2), 229–248. doi:10.1558/ijsll.v22i2.23101
Bucholtz, M. (2007). Variation in Transcription. Discourse Stud. 9 (6), 784–808. doi:10.1177/1461445607082580
Bucholtz, M. (2009). Captured on Tape: Professional Hearing and Competing Entextualizations in the Criminal Justice System. Text & Talk. 29 (5), 503–523. doi:10.1515/text.2009.027
Clayman, S. E., and Teas Gill, V. (2012). “Conversation Analysis,” in The Routledge Handbook of Discourse Analysis. Editors J. P. Gee, and M. Hanford (London: Routledge), 120–134.
Copland, F., and Creese, A. (2015). Linguistic Ethnography: Collecting, Analysing and Presenting Data. London: SAGE.
Coulthard, M., Johnson, A., and Wright, D. (2017). An Introduction to Forensic Linguistics: Language in Evidence. London: Routledge.
Davidson, C. (2009). Transcription: Imperatives for Qualitative Research. Int. J. Qual. Methods 8, 35–52. doi:10.1177/160940690900800206
Dice, L. R. (1945). Measures of the Amount of Ecologic Association Between Species. Ecology 26 (3), 297–302. doi:10.2307/1932409
Easton, K. L., McComish, J. F., and Greenberg, R. (2000). Avoiding Common Pitfalls in Qualitative Data Collection and Transcription. Qual. Health Res. 10 (5), 703–707. doi:10.1177/104973200129118651
Fleiss, J. L. (1971). Measuring Nominal Scale Agreement Among many Raters. Psychol. Bull. 76 (5), 378–382. doi:10.1037/h0031619
Fraser, H. (2003). Issues in Transcription: Factors Affecting the Reliability of Transcripts as Evidence in Legal Cases. Int. J. Speech, Lang. L. 10 (2), 203–226. doi:10.1558/sll.2003.10.2.203
Fraser, H. (2014). Transcription of Indistinct Forensic Recordings: Problems and Solutions From the Perspective of Phonetic Science. Lang. L. / Linguagem e Direito. 1 (2), 5–21.
Fraser, H. (2018a). Covert Recordings Used as Evidence in Criminal Trials: Concerns of Australian Linguists. Judicial Officers’ Bull. 30 (6), 53–56. doi:10.3316/INFORMIT.728989125075618
Fraser, H. (2018b). 'Assisting' Listeners to Hear Words that Aren't There: Dangers in Using Police Transcripts of Indistinct Covert Recordings. Aust. J. Forensic Sci. 50 (2), 129–139. doi:10.1080/00450618.2017.1340522
Fraser, H. (2018c). Thirty Years Is Long Enough: It’s Time to Create a Process That Ensures covert Recordings Used as Evidence in Court Are Interpreted Reliably and Fairly. J. Judicial Adm. 27, 95–104.
Fraser, H. (2021a). “Forensic Transcription: The Case for Transcription as a Dedicated Area of Linguistic Science,” in The Routledge Handbook of Forensic Linguistics. Editors M. Coulthard, A. Johnson, and R. Sousa-Silva. 2nd edn. (London: Routledge), 416–431.
Fraser, H. (2021b). The Development of Legal Procedures for Using a Transcript to Assist the Jury in Understanding Indistinct covert Recordings Used as Evidence in Australian Criminal Trials A History in Three Key Cases. Lang. L. 8 (1), 59–75. doi:10.21747/21833745/lanlaw/8_1a4
Fraser, H., and Loakes, D. (2020). Acoustic Injustice: The Experience of Listening to Indistinct Covert Recordings Presented as Evidence in Court. L. Text Cult. 24, 405–429.
Fraser, H., Stevenson, B., and Marks, T. (2011). Interpretation of a Crisis Call: Persistence of a Primed Perception of a Disputed Utterance. Int. J. Speech, Lang. L. 18 (2), 261–292. doi:10.1558/ijsll.v18i2.261
Freelon, D. (2013). ReCal OIR: Ordinal, Interval, and Ratio Intercoder Reliability as a Web Service. Int. J. Internet Sci. 8 (1), 10–16. Available at: http://dfreelon.org/utils/recalfront/recal-oir/.
French, P., and Fraser, H. (2018). Why ‘Ad Hoc Experts’ Should Not Provide Transcripts of Indistinct Forensic Audio, and a Proposal for a Better Approach. Criminal L. J. 42, 298–302.
Ghaemmaghami, H., Dean, D., Vogt, R., and Sridharan, S. (2012). Speaker Attribution of Multiple Telephone Conversations Using a Complete-Linkage Clustering Approach. Speech Signal. Process. (Icassp)., 4185–4188. doi:10.1109/icassp.2012.6288841
Jaffe, A. (2000). Introduction: Non-Standard Orthography and Non-Standard Speech. J. Sociolinguistics. 4 (4), 497–513. doi:10.1111/1467-9481.00127
Jenks, C. J. (2013). Working With Transcripts: An Abridged Review of Issues in Transcription. Lang. Linguistics Compass. 7 (4), 251–261. doi:10.1111/lnc3.12023
Kirk, J., and Andersen, G. (2016). Compilation, Transcription, Markup and Annotation of Spoken Corpora. Int. J. Corpus Linguistics. 21 (3), 291–298. doi:10.1075/ijcl.21.3.01kir
Krippendorff, K. (1970). Estimating the Reliability, Systematic Error and Random Error of Interval Data. Educ. Psychol. Meas. 30 (1), 61–70. doi:10.1177/001316447003000105
Krippendorff, K. (2004). Content Analysis: An Introduction to its Methodology. Thousand Oaks, California: SAGE.
Lapadat, J. C., and Lindsay, A. C. (1999). Transcription in Research and Practice: From Standardization of Technique to Interpretive Positionings. Qual. Inq. 5 (1), 64–86. doi:10.1177/107780049900500104
Loubere, N. (2017). Questioning Transcription: The Case for the Systematic and Reflexive Interviewing and Reporting (SRIR) Method. Forum Qual. Soc. Res. 18 (2), 15. doi:10.17169/fqs-18.2.2739
Love, R., Dembry, C., Hardie, A., Brezina, V., and McEnery, T. (2017). The Spoken BNC2014. International Journal of Corpus Linguistics 22 (3), 319–344. doi:10.1075/ijcl.22.3.02lov
Love, R., Hawtin, A., and Hardie, A. (2018). The British National Corpus 2014: User Manual and Reference Guide. Lancaster: ESRC Centre for Corpus Approaches to Social Science. Available at: http://corpora.lancs.ac.uk/bnc2014/doc/BNC2014manual.pdf.
Love, R. (2020). Overcoming Challenges in Corpus Construction: The Spoken British National Corpus 2014. New York: Routledge.
Nagy, N., and Sharma, D. (2013). “Transcription,” in Research Methods in Linguistics. Editors R. Podesva, and D. Sharma (Cambridge, 235–256.
Oliver, D. G., Serovich, J. M., and Mason, T. L. (2005). Constraints and Opportunities With Interview Transcription: Towards Reflection in Qualitative Research. Social Forces. 84 (2), 1273–1289. doi:10.1353/sof.2006.0023
Schegloff, E. A. (2007). Sequence Organisation in Interaction: A Primer in Conversation-Analysis. Cambridge: Cambridge University Press.
Scott, M. (2007). Formulae. Retrieved From WordSmith Tools. Available at: https://lexically.net/downloads/version5/HTML/index.html?formulae.htm.
Tessier, S. (2012). From Field Notes, to Transcripts, to Tape Recordings: Evolution or Combination? Int. J. Qual. Methods. 11 (4), 446–460. doi:10.1177/160940691201100410
Keywords: forensic transcription, covert recordings, speaker attribution, transcription variation, inter-rater agreement analysis
Citation: Love R and Wright D (2021) Specifying Challenges in Transcribing Covert Recordings: Implications for Forensic Transcription. Front. Commun. 6:797448. doi: 10.3389/fcomm.2021.797448
Received: 18 October 2021; Accepted: 30 November 2021;
Published: 22 December 2021.
Edited by:
Helen Fraser, The University of Melbourne, AustraliaReviewed by:
Vincent Hughes, University of York, United KingdomGeorgina Brown, Lancaster University, United Kingdom
Copyright © 2021 Love and Wright. This is an open-access article distributed under the terms of the Creative Commons Attribution License (CC BY). The use, distribution or reproduction in other forums is permitted, provided the original author(s) and the copyright owner(s) are credited and that the original publication in this journal is cited, in accordance with accepted academic practice. No use, distribution or reproduction is permitted which does not comply with these terms.
*Correspondence: Robbie Love, ci5sb3ZlQGFzdG9uLmFjLnVr