- 1Phonetics Work Group, Bielefeld University, Bielefeld, Germany
- 2CITEC, Bielefeld University, Bielefeld, Germany
- 3Centre for Industrial Electronics, University of Southern Denmark, Sonderborg, Denmark
In the present work, we describe and discuss two studies in the field of motivating-speech research. The studies investigate voice-quality features (study 1) and pragmatic aspects (study 2) in German motivating speech, thereby adding to the current state-of-the-art in understanding motivating speech and language1. We find indications that a low amount of breathiness, a more periodic signal and a balanced distribution of specific pragmatic elements contribute to a motivating impact in German.
Introduction
Be it in teaching, in the workplace, in sports or health care, there are numerous scenarios in which it is essential that an individual motivates their interlocutor toward a specific action. In this work, we focus on motivation in the context of sports and healthy nutrition and define the term motivation as a process which results in a readiness to act within an individual (Mook, 1987, cited in Rudolph, 2013). This process is complex and can be influenced by several factors, among which are leadership communication (Mayfield and Mayfield, 2018) and phonetics (Voße and Wagner, 2018). It has been discussed and partially shown that specific patterns on the level of language and speech influence the motivating impact of a person positively, while other patterns are assumed to be detrimental in the context of motivation.
Mayfield and Mayfield (2018) investigate the role of language within motivation from a pragmatic perspective. Central in their work is the Motivating Language Theory by Sullivan (1988) which argues that motivation within the workplace is enhanced by communication from the leadership that uses and balances explaining elements [meaning making language (MM)], empathetic elements [empathetic language (E)], and instructive elements [direction-giving language (DG)]. MM, E, and DG are claimed to be essential and, therefore, should all occur in motivating language.
Voße and Wagner (2018) focus on the acoustic-phonetic expression of motivation. Their hypotheses are inspired by recent studies on emotional speech and charismatic speech. Voße and Wagner (2018) see charisma and motivation as related concepts, as both can evoke an inner change within an individual (Niebuhr and Gonzalez, 2019). Although both concepts overlap in some aspects, charisma is usually described in the context of leadership (Klein and House, 1995; Antonakis et al., 2016). Motivation is more multifaceted and not necessarily tied to a leader-follower-relation (see Rheinberg and Engeser, 2018 for an overview). Due to the pragmatic proximity of motivation and charisma, Voße and Wagner (2018) expect that acoustic-phonetic characteristics observed for charismatic speech may also apply toward motivating speech.
Regarding motivation and emotion, Voße and Wagner (2018) argue for a systematic connection between these concepts. Striving for a positive emotional state is a basic motive within human behavior and it plays a central role in motivational processes (Schultheiss and Wirth, 2018). The emotional state of an individual, in turn, can directly be influenced by an interlocutor via the display of specific emotions (“emotional contagion”, Giner-Sorolla, 2012). Following from this, Voße and Wagner (2018) assume that the expression of positive emotions supports the motivating impact of a speaker.
Consistent with their assumption, Voße and Wagner (2018) found a high and variable pitch, a fast articulation, and a consistently loud voice within the speech units they analyzed as well as a longer duration of the analyzed speech units. This feature set shows several parallels to the acoustic-phonetic expression of charisma and positive emotions (e.g., Burkhardt and Sendlmeier, 2000; Niebuhr et al., 2016).
Understanding the characteristics of motivating speech, however, is still far from being achieved. In the present work, we want to contribute to the current state-of-the-art by presenting our analyses of voice-quality features (study 1) and pragmatic aspects (study 2) in German motivating speech. We designed the two studies with the aim to provide a first insight into these topics, so our findings might constitute a basis for future studies in this area.
Voice quality features have not been investigated in motivating speech so far. We assume these features add to the expression of motivation, as voice quality has been shown to be involved in the acoustic-phonetic characterization of emotions, affect, moods, and attitudes. However, the results regarding positive emotional speech from these studies are not congruent. For example, while Murray and Arnott (1993) report a rather breathy voice for the expression of happiness, Gobl and Ní Chasaide (2003) report a tenser voice setting.
Besides its role in positive emotional speech, Niebuhr et al. (2018) found specific voice quality characteristics for charismatic speech. Following Voße and Wagner's (2018) understanding of charisma and motivation as intertwined concepts, we expect that voice quality characteristics observed for charismatic speech may also apply toward motivating speech.
Study 1 of this work focuses on voice quality features in motivating speech in German. As the picture regarding voice quality characteristics of positive emotional speech is less clear in the literature than the picture regarding voice quality features of charismatic speech, we consider only findings of the latter for the hypothesis to be tested in study 1:
• H1 (study 1): Motivating speech involves a specific voice quality, that is, a bundle of acoustic-phonetic voice quality features all of which conspire to contribute to the impression of a “fuller voice” and a lower amount of breathiness (see Niebuhr et al., 2018, and 2.2 for an explanation of “full”).
To test this hypothesis 1, we compare speech samples from two different levels of motivation, namely more motivating speech (MMS) and less motivating speech (LMS) (see Data collection and annotation for a definition of MMS and LMS).
In addition to investigating the role of voice quality in the expression of motivation, it would be insightful to see whether claims of the Motivating Language Theory generalize to domains outside of leadership communication in the workplace. We dedicate study 2 in this article to this purpose. Here, we transfer a central claim of Motivating Language Theory–namely that an occurrence of the element types MM, E, and DG is essential for a motivating impact–to motivating speech in the area of sports and healthy nutrition. To this purpose, we compare the distributions of the element types in more motivating speech (MMS) and in less motivating speech (LMS) and hypothesize the following:
• H2 (study 2): We assume that all three elements MM, E, and DG occur in the MMS condition, but not or less balanced in the LMS condition.
We are further interested in whether the individual element types show specific acoustic-phonetic profiles so that their variable combinations cause dynamic changes within motivating speech. Findings from Repp (2020) show that different types of speech acts are marked differently in the prosodic dimension, which lets us assume that also other pragmatic units such as the element types MM, E, and DG might differ in their acoustic-phonetic realization. Additionally, we expect to observe differences in the realization of each element type between the conditions MMS and LMS, as acoustic-phonetic differences between these two conditions have been reported by Voße and Wagner (2018) for other types of units. In sum, we expect to observe the following regarding the acoustic-phonetic form of the element types MM, E, and DG:
• H3 (study 2): We will observe element-specific, differentiated acoustic-phonetic characteristics between the conditions MMS and LMS for the elements MM, E, and DG.
Methods
Data collection and annotation
We use audio tracks from non-professional motivating videos from the Internet that were freely accessible. We opt for non-professionals to avoid any confounds caused by an expert status of a speaker or due to monetary reasons. Additionally, we ensure that the data is suitable for acoustic analyses and that factors which potentially influence motivational processes are constant. We decide to focus on female motivating speakers of a defined age span (~18–30 years), who address a specific audience (young adults, approximately 18–30 years, probably mostly females). The assumptions about the target audience are made on the basis of the topics/arguments provided in the videos. Furthermore, we consider only data which show a similar argumentation structure and do not make exhaustive use of visualization tools.
We use the audience rating “view” to distinguish between more motivating videos [representing more motivating speech (MMS)] and videos with a less motivating impact [representing less motivating speech (LMS)], in this way identifying acoustic-phonetic patterns that potentially either promote or inhibit a motivating impact. In our scheme, more motivating videos gain at least 33,000 views and less motivating videos at most 1,000 views over approximately 1.5 years. These thresholds do not refer to any standard. They are chosen after inspecting the views of all videos suitable for the analyses with the intention to maximize the distance between the MMS and LMS conditions.
We are aware that the popularity of a speaker is a potential confound in our categorization strategy that limits our conclusions. To counteract that, we chose videos of non-professionals who do not receive a high level of recognition outside the fitness community. Doing so, we assume that the majority of the users who watched the analyzed videos did not do so because of the speakers but because of a genuine interest in sports and healthy nutrition. Of course, we cannot fully control the factor popularity with this approach, as it might be that more popular fitness bloggers receive more views than less popular ones. However, we believe that the popularity of a fitness blogger is at least partially founded in its motivating impact. Accordingly, it appears rather unlikely that a video receives “views” exclusively for the popularity of the speakers without considering their motivating impact.
In total, the data set for studies 1 and 2 consists of audio tracks from six videos, each presented by a different female speaker in German. The data is divided into three videos (35 min in total) that represent a higher level of motivation and three videos (18 min in total) that represent a lower level. In total, about 53 min of speech material were analyzed.
The data for the study on voice quality is annotated semi-automatically with WebMAUS (Kisler et al., 2017) on phone level, followed by manual corrections of phone labels and segment boundaries.
For the study on pragmatics, we manually annotated the data for the element types MM, E and DG and supplemented this annotation with an additional, parallel annotation of speech acts from Speech Act Theory (Austin, 1975). The latter facilitated the annotation procedure, as the temporal extension of MM, E and DG elements is often hard to define, whereas speech acts are more clear-cut categories along the time axis and easy to associate with MM, E and DG. From the categories provided by the Speech Act Theory, we considered representative speech acts, direct speech acts and indirect speech acts, as they closely match with the element types MM, E, and DG (see Sullivan, 1988 and Austin, 1975 for more detailed information on the element types and speech act types).
As we applied the Motivating Language Theory outside the field it was originally developed for, it was necessary to adapt the element types MM, E, and DG accordingly. In the present study, MM, E, and DG are defined as follows:
• Meaning making language (MM): representative speech act expressing factual knowledge or personal opinions; Example: “It is fun to do sports.”
• Empathetic language (E): representative speech act expressing personal experience; Example: “I really enjoy doing sports.”
• Direction giving language (DG): (in)direct speech act expressing commands or suggestions for an action; Example: “Give it a try to do sports.”
These definitions form the basic segmentation criteria during the annotation process of study 2.
Data analyses
Study 1: Voice quality
As specified in the introduction, we expect motivating speech to resemble charismatic speech in terms of voice quality. That is, motivating speech should show a low amount of breathiness and a fuller voice (Niebuhr et al., 2018). As acoustic correlates of amount of breathiness and full voice, we consider the features cepstral peak prominence smooth (CPPS), spectral slope, and H1-H2.
CPPS describes the distance between the amplitude of the first rhamonic and its corresponding quefrency point on the regression line in the cepstrum (Hillenbrand and Houde, 1996). This distance is an indicator of the harmonicity of the spectrum, as a prominent first rhamonic speaks for a more clearly pronounced harmonic structure in the spectrum (Mayer, 2017). A more harmonic structure in the spectrum implies a higher periodicity of the signal, for which a lower amount of breathiness is a prerequisite (Hillenbrand and Houde, 1996). We assume that signals with a high periodicity and a low amount of breathiness are more strongly associated with the auditory impression of a “fuller voice” than signals with a low periodicity and a higher amount of breathiness.
Breathy voices are furthermore characterized by long open phases and short closed phases of the glottis, while the opposite is true for creaky voices (Mayer, 2017). Long open phases of the glottis are usually associated with a larger energy drop in higher frequency bands in comparison to shorter opening phases. The amount of this energy loss toward higher spectral frequencies can be estimated via measures of the spectral slope (Mayer, 2017). We use the Praat (Boersma and Weenink, 2018) command “Get slope…” (settings: low band: 50–1,000 Hz, high band: 1,000–5,000 Hz; averaging method: energy) based on a long-term average spectrum.
Besides its relation to breathiness, studies found that the spectral slope also varies due to stress and accentuation, with lower stress/accent levels showing steeper spectral slopes than higher stress/accents levels of syllables and words (see Heldner, 2001 for an overview). Yarra et al. (2017) report a relation between stressed syllables and sonority such that sonority-based features improved the accuracy of their stress-detection algorithm. We infer from these findings that a less steep spectral slope correlates with a more sonorous voice and hence contributes to the impression of a “fuller voice.”
With H1-H2, we include a central, robust measure of breathiness in our analysis. H1-H2 describes the dB difference in amplitude between the first and the second harmonic in the signal, which directly relates to the proportion of open and closed phases of the glottis (Mayer, 2017) on the acoustic level.
We expect CPPS, spectral slope, and H1-H2 to differ significantly between the conditions MMS and LMS in the following ways:
• CPPS: higher for MMS than for LMS, that is, a higher signal periodicity, a lower amount of breathiness and, therefore, a fuller voice
• Spectral slope: lower for MMS than for LMS, that is, a lower amount of breathiness and a fuller voice
• H1-H2: closer to 0 for MMS than for LMS, that is, a lower amount of breathiness.
Our analysis is performed exclusively on instances of /a / (n = 246) with a duration of at least 0.05 s (50 ms), as Mayer (2017) finds this vowel to be most suitable for H1-H2 analyses. To have a consistent data set for this analysis, we took the other measurements on these vowel-sized segments as well.
The analysis is conducted automatically with Praat (Boersma and Weenink, 2018) scripts2. The statistical analysis is performed in R. For each of the three voice quality parameters specified in our hypothesis, we determine the following three fundamental statistical features and compare them between LMS and MMS:
• Location of distribution, that is, where the center of the distribution is located
• Dispersion of distribution, that is, the range of the values in a distribution
• Form of distribution, that is, how the distribution is shaped.
During the analysis, we collect visual impressions from the inspection of density plots and supplement them with findings from Bonferroni-corrected non-parametric tests [Wilcoxon-Rank-Sum in the package “stats” (R Core Team., 2019) for location of distribution, Brown-Forsythe in the package “lawstat” (Gastwirth et al., 2015) for dispersion of distribution, Kolmogorov-Smirnov in the package “stats” (R Core Team., 2019) for form of distribution] due to a non-normal distribution of half of the variables.
Study 2: Pragmatics
The data is annotated manually for the element types MM, E, and DG as well as for speech acts (see section Introduction and Data collection and annotation for further information) on a separate tier by one trained phonetician. To check for a consistent annotation procedure, we calculate an intra-annotator agreement and an inter-annotator agreement with Cohen's kappa on a subset of the data (about 10% of the data per speaker). For the intra-annotator agreement, the annotator redoes the labeling task on an already completed subset of the data about 1 year and 3 months later. For the inter-annotator agreement, we delete the labels from a subset of the data annotated by the first annotator, so the annotation contains only boundaries. A second annotator then inserts the respective MM, E and DG element-type and speech-act labels again.
The analysis is conducted in two steps. First, we look at the distribution of elements within the conditions MMS and LMS by applying a Chi-squared test in R (see R Core Team, 2021). Second, we analyze a set of acoustic-phonetic features for each element type automatically via Praat (Boersma and Weenink, 2018) scripts3. The set of features comprises:
• Duration of the element types MM, E, and DG (sec.)
• RMS intensity (Pa)
• Intensity variation coefficient ()
• F0 median (st rel. to 200 Hz)
• F0 variation coefficient ().
The statistical assessment is conducted in R on z-score normalized data per speaker. Here, we employ generalized mixed models with the package “lme4” (Bates et al., 2015) to predict the acoustic-phonetic features as a function of Condition*Label. By investigating this interaction effect, we check for element-specific, differentiated acoustic-phonetic characteristics between the conditions for the elements MM, E, and DG as specified in the hypotheses.
Results
Study 1: Voice quality
Regarding location of distribution, a clear difference in location of distribution between the conditions MMS and LMS can only be observed for CPPS, where the mean for MMS is noticeably higher than the mean for LMS, see Figure 1. This observation is confirmed by the Wilcoxon rank-sum test, which yields a significant result only for CPPS (W = 1970, p < 0.001***). On an auditory level, the observed difference in CPPS level corresponds to the impression of a fuller and less breathy voice in MMS than in LMS.
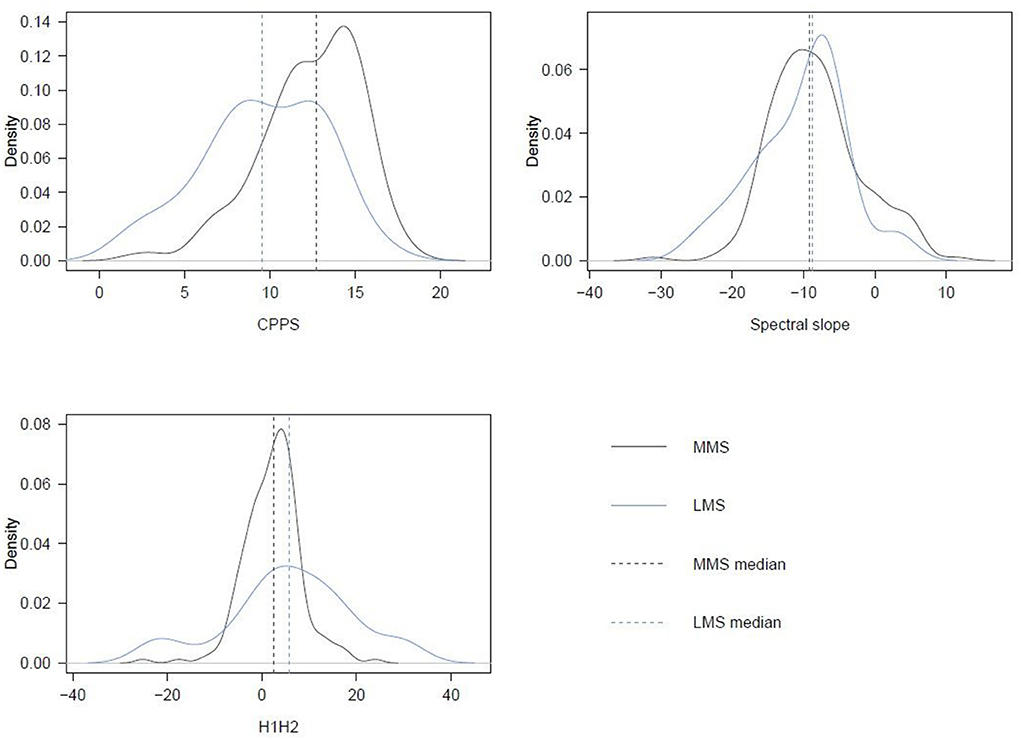
Figure 1. Density plots representing the distributions of MMS and LMS for the investigated parameters in study 1.
The dispersion of distribution differs most between LMS and MMS for H1-H2, where LMS shows a wider dispersion. Differences in this respect are not clearly visible for CPPS and spectral slope, see Figure 1. The Brown-Forsythe test supports this impression, as only the difference in H1-H2 is statistically significant (F = 41.312, p < 0.001***). This difference indicates that LMS speakers employ a wider spectrum of voice qualities than MMS speakers.
In terms of form of distribution, we observe clear differences in the distributions for CPPS and H1-H2, see Figure 1. The statistical significance between these differences is confirmed by the Kolmogorov-Smirnov test (D = 0.37597, p < 0.001***; D = 0.36099, p < 0.01**), which supports the impression that LMS speakers behave differently than MMS speakers with respect to these two features.
Study 2: Pragmatics
Regarding the annotator agreement, the calculation of Cohen's kappa yields κ = 0.98 for the intra-annotator comparison and κ = 0.77 for the inter-annotator comparison.
For investigating hypothesis 2, we compare the frequencies of the elements MM, E, and DG in the conditions LMS and MMS. Instances of all three elements can be found in both conditions. However, the elements show different distributions in MMS and LMS, χ2 (2, N = 442) = 64.386, p < 0.001***. In Figure 2A it can be seen that in MMS the elements are distributed in a more balanced manner compared to LMS where E dominates and DG is hardly present.

Figure 2. (A) Mosaic plot representing the distributions of E, DG, and MM in the conditions MMS and LMS; (B) Boxplots for RMS intensity in the elements DG, E and MM with respect to the conditions MMS and LMS.
Regarding hypothesis 2, we employ linear mixed effect models [package “lme4” (Bates et al., 2015)] to investigate the potential interaction effect Condition*Label. The models contain the variable Speaker as random intercept. An interaction effect is observed in the model for RMS intensity between LMS and E (estimate = −1.0151, SE = 0.4793, t = −2.118). This effect underlies a lower RMS intensity relative to DG labels in the LMS condition, see Figure 2B. A similar trend occurs in the RMS intensity model between the condition LMS and the label MM (meaning making language) (estimate = −0.8628, SE = 0.4933, t = −1.749), but without statistical significance.
Discussion
In study 1, we observe higher mean values in CPPS for MMS than for LMS. This supports our assumption that motivating speech is characterized by a more periodic signal, which we consider as an indicator of a fuller voice and a lower amount of breathiness (see chapter 2.3.1 for more details). The findings regarding H1-H2 indicate reduced and less variable breathiness in motivating speech. In total, these findings support our hypothesis for study 1, that is, a fuller and less breathy voice characterizes MMS as compared to LMS.
The results from study 1 suggest that voice quality-related features play a role in the acoustic-phonetic expression of motivating speech. However, it must be considered that the data selection for this study has been restricted to occurrences of the vowel /a / with a minimal duration of 0.05 s. Future studies on other vowel qualities might lead to different observations.
In study 2, we observe different frequency distributions of the elements MM, E, and DG in the conditions MMS and LMS. The nearly equal proportions of the elements in MMS suggest that a balanced occurrence of the three element types promotes a motivating impact, which is consistent with Mayfield and Mayfield (2018). Focusing on E elements and underrepresenting DG elements, however, could have a detrimental effect on motivation. It would be interesting to see in future studies if this presumably detrimental effect can be caused by any possible imbalance in the elements' distributions, or if it is specifically the underrepresentation of DG and/or the overrepresentation of E that reduces a speaker's motivational impact.
Regarding how well the acoustic-phonetic features determine the elements' tone of voice, it is surprising to find an interaction effect only in one of five investigated models, that is, in the RMS intensity model. Considering findings from the literature regarding prosodic realization in speech acts (e.g., Repp, 2020), we expected to find further element-specific acoustic-phonetic characteristics besides the interaction effect of LMS and E in the RMS intensity model. The lack of further element-specific observations might be due to our annotation scheme, especially the way we segmented our data. Severe conceptual problems with respect to labeling the data can be ruled out, as the inter-annotator agreement reached a fairly good result (κ = 0.77). Nevertheless, it would be interesting to run the study again with a revised annotation scheme to check whether it might lead to a clearer and more distinctive acoustic-phonetic characterization of the three element types. Furthermore, the chosen unit of analysis can have an impact, as Voße and Wagner's (2018) study based on inter-pausal units came up with prosodically more distinct results between the conditions LMS and MMS than our analysis based on the element-type units of study 2.
Another point that must be mentioned is the somewhat arbitrarily chosen number-of-views threshold for grouping the data into the conditions MMS and LMS. We are aware that our threshold lacks a validation and thus limits the generalization of our findings. However, we believe that the chosen threshold captures data with quite different levels of motivation, as we maximized the distance of views between MMS and LMS. We expect this strategy to be sufficient for the present goal: providing a first glimpse into the phonetics and pragmatics of motivating speech and, thereby, laying the foundation for more specific hypotheses that are put forward and tested in future studies. Nevertheless, we believe that follow-up studies in this area will definitely benefit from developing and applying a more robust threshold or categorization concept, for example, one that is based on additional perception tests.
It must be discussed that the data sets of study 1 and 2 are rather sparse and, therefore, the conditions are confounded with a low number of speakers. Also, our samples are restricted to female speakers of a specific age span and, therefore, might not generalize to other groups of speakers. Although we assume that the videos were addressed at young female adults, we have not directly controlled for the audience, so we cannot infer whether this factor might have influenced our results. Moreover, we have not controlled factors such as gestures and mimics, which will certainly have affected the rating of the videos and therefore our threshold concept. These problems result from the general difficulty of obtaining suitable speech material: Not only must potential data sources meet the requirements for acoustic analyses, they must also be constant with respect to factors that play a role in triggering motivation. As only a low number of available data sources fulfill these requirements, future studies might opt for recording larger data sets under controlled conditions.
In view of these limitations, the results of the two studies must be treated as preliminary. Nevertheless, our studies provide a first impression of how motivating speech could possibly be characterized in acoustic-phonetic and pragmatic terms and form a valuable basis for the generation of hypotheses in future studies. In these, besides working with a larger and more controlled data set, we believe that a speaker-specific analysis of motivating speech could be a fruitful endeavor, as there might be differences between individual motivating strategies.
Data availability statement
The data analyzed in this study is subject to the following licenses/restrictions: To protect the privacy of the speakers, we will not publish the links to the material. Requests to access these datasets should be directed to ai52b3NzZUB1bmktYmllbGVmZWxkLmRl.
Author contributions
JV: planning, concepting, designing and conducting the studies, and writing and submitting the article. ON: contribution to concept and design of study 2 and feedback on the article draft. PW: supervision of the conduction of the studies, contribution to concept and design of the studies, and feedback on the article draft. JV, ON, and PW: revising the article. All authors contributed to the article and approved the submitted version.
Conflict of interest
The authors declare that the research was conducted in the absence of any commercial or financial relationships that could be construed as a potential conflict of interest.
Publisher's note
All claims expressed in this article are solely those of the authors and do not necessarily represent those of their affiliated organizations, or those of the publisher, the editors and the reviewers. Any product that may be evaluated in this article, or claim that may be made by its manufacturer, is not guaranteed or endorsed by the publisher.
Footnotes
1. ^We acknowledge support for the publication costs by the Open Access Publication Fund of Bielefeld University and the Deutsche Forschungsgemeinschaft (DFG).
2. ^We thank Marcin Wlodarczak for sharing his Praat (Boersma and Weenink, 2018) script for the voice quality analysis with us.
3. ^The scripts were written by the first author, who took inspiration from Mietta Lennes' Praat (Boersma and Weenink, 2018) scripts shared on https://lennes.github.io/spect/.
References
Antonakis, J., Bastardoz, N., Jacquart, P., and Shamir, B. (2016). Charisma: An ill-defined and ill-measured gift. Annu. Rev. Organ. Psychol. Organ. Behav. 3, 293–319. doi: 10.1146/annurev-orgpsych-041015-062305
Austin, J. L. (1975). “How to do things with words.” In: The William James lectures delivered at Harvard University in 1955. Ed. J. H. Urmson and M.Sbisà (New York: Oxford University Press). doi: 10.1093/acprof:oso/9780198245537.001.0001
Bates, D., Maechler, M., Bolker, B., and Walker, S. (2015). Fitting Linear Mixed-Effects Models Using lme4. J. Stat. Softw. 67, 1–48. doi: 10.18637/jss.v067.i01
Boersma, P., and Weenink, D. (2018). Praat: doing phonetics by computer [Computer program]. Version 6.1.09. Available online at: http://www.praat.org/
Burkhardt, F., and Sendlmeier, W. F. (2000). Verification of acoustical correlates of emotional speech using formant-synthesis. In: SpeechEmotion-2000, (Newcastle, Northern Ireland, UK), 151–156.
Gastwirth, J. L., Gel, Y. R., Hui, W. W., Miao, W., Noguchi, K., and Lyubchich, M. V. (2015). Package ‘lawstat'. Available online at: https://cran.microsoft.com/snapshot/2015-07-01/web/packages/lawstat/lawstat.pdf (accessed December 23, 2021).
Giner-Sorolla, R. (2012). Judging Passions: Moral Emotions in Persons and Groups. Psychology Press. doi: 10.4324/9780203123874
Gobl, C., and Ní Chasaide, A. (2003). The role of voice quality in communicating emotion, mood and attitude. Speech Commun. 40, 189–212. doi: 10.1016/S0167-6393(02)00082-1
Hillenbrand, J., and Houde, R. A. (1996). Acoustic correlates of breathy vocal quality: Dysphonic voices and continuous speech. J. Speech Lang. Hear. Res. 39, 311–321. doi: 10.1044/jshr.3902.311
Kisler, T., Reichel, U. D., and Schiel, F. (2017): Multilingual processing of speech via web services. Comput. Speech Lang. 45, 326–347. doi: 10.1016/j.csl.2017.01.005
Klein, K. J., and House, R. J. (1995). On fire: charismatic leadership and levels of analysis. Leadership Q. 6, 183–198. doi: 10.1016/1048-9843(95)90034-9
Mayer, J. (2017). Phonetische Analysen mit Praat. Ein Handbuch für Ein- und Umsteiger. Version 2017/09.
Mayfield, J., and Mayfield, M. (2018). Motivating Language Theory: Effective Leader Talk in the Workplace. New York, NY: Palgrave Macmillan. doi: 10.1007/978-3-319-66930-4
Murray, I. R., and Arnott, J. L. (1993). Toward the simulation of emotion in synthetic speech: a review of the literature on human vocal emotion. J. Acoust. Soc. Am. 93, 1097–1108. doi: 10.1121/1.405558
Niebuhr, O., and Gonzalez, S. (2019). Do sound segments contribute to sounding charismatic? Evidence from a case study of Steve Jobs' and Mark Zuckerberg's vowel spaces. Int. J. Acoust. Vib. 24, 343–355. doi: 10.20855/jav.2019.24.21531
Niebuhr, O., Skarnitzl, R., and Tylečková, L. (2018). The acoustic fingerprint of a charismatic voice-Initial evidence from correlations between long-term spectral features and listener ratings. In: Proc. 18th International Conference of Speech Prosody, Poland, 359–363. doi: 10.21437/SpeechProsody.2018-73
Niebuhr, O., Voße, J., and Brem, A. (2016). What makes a charismatic speaker? A computer-based acoustic-prosodic analysis of Steve Jobs tone of voice. Comput. Hum. Behav. 64, 366–382. doi: 10.1016/j.chb.2016.06.059
R Core Team. (2019). R: A language and environment for statistical computing. R Foundation for Statistical Computing, Vienna, Austria. Available online at: https://www.R-project.org/
Repp, S. (2020). The prosody of wh-exclamatives and wh-questions in German: Speech act differences, information structure, and sex of speaker. Lang. Speech. 63, 306–361. doi: 10.1177/0023830919846147
Rheinberg, F., and Engeser, S. (2018). Intrinsische Motivation und Flow-Erleben. In: Motivation und Handeln. Springer-Lehrbuch. Eds J. Heckhausen, H. Heckhausen (Berlin, Heidelberg: Springer). doi: 10.1007/978-3-662-53927-9_14
Schultheiss, O. C., and Wirth, M. M. (2018). Biopsychologische Aspekte der Motivation. In: Motivation und Handeln. Springer-Lehrbuch. Eds J. Heckhausen, H. Heckhausen (Berlin, Heidelberg: Springer). doi: 10.1007/978-3-662-53927-9_10
Sullivan, J. (1988). Three roles of language in motivation theory. Acad. Manage. Rev. 13, 104–115. doi: 10.5465/amr.1988.4306798
Voße, J., and Wagner, P. (2018). “Investigating the phonetic expression of successful motivation,” in Proceedings of the 9th Tutorial and Research Workshop on Experimental Linguistics, 117–120. doi: 10.36505/ExLing-2018/09/0028/000361
Keywords: motivation, speaking style, acoustic phonetics, pragmatics, voice quality
Citation: Voße J, Niebuhr O and Wagner P (2022) How to motivate with speech. Findings from acoustic phonetics and pragmatics. Front. Commun. 7:910745. doi: 10.3389/fcomm.2022.910745
Received: 01 April 2022; Accepted: 27 July 2022;
Published: 18 August 2022.
Edited by:
Donna Mae Erickson, Haskins Laboratories, United StatesReviewed by:
Puyang Geng, Academy of Forensic Science, ChinaMarisa Cruz, University of Lisbon, Portugal
Copyright © 2022 Voße, Niebuhr and Wagner. This is an open-access article distributed under the terms of the Creative Commons Attribution License (CC BY). The use, distribution or reproduction in other forums is permitted, provided the original author(s) and the copyright owner(s) are credited and that the original publication in this journal is cited, in accordance with accepted academic practice. No use, distribution or reproduction is permitted which does not comply with these terms.
*Correspondence: Jana Voße, ai52b3NzZUB1bmktYmllbGVmZWxkLmRl