- Department of Speech Research and Technology, Institute of the Estonian Language, Tallinn, Estonia
The increasing prevalence of audio advertising has provided a challenge to find out more about voices and performance styles used in advertisements. In this study, we were interested in the listeners' preferences when a synthesizer performs the advertisements. As training an advertisement style synthesizer requires big corpora, the creation of which is time-consuming and expensive, we have chosen to use less resource-intensive style transfer on already extant synthesized voices trained on neutral speech. We used a corpus of advertisements created out of 120 male and 120 female voices reading one text in both an energetic and calm advertisement style, the styles most commonly provided by advertising agencies, to train four style transfer models: energetic and calm for both male and female voices. These were used to convert two synthesized female and two male voices that had been created using a Merlin-based speech synthesizer for Estonian. Each converted voice performed three short advertisements. Adult listeners rated the likability of the performances on a 7-point Likert scale. The results showed that the calm performance style was overwhelmingly preferred. We also ascertained the acoustic features of the calm and energetic performances using the open-source toolkit openSMILE to calculate the 88 parameters of the extended Geneva Minimalistic Acoustic Parameter Set. The calm style differed from the energetic in acoustic features that are related to a lower, quieter, and more sonorous voice and a more neutral speaking style. Considering the difference in style ratings, it is worth taking the target audiences' style preferences into account.
1. Introduction
Every day we are exposed to advertisements on the radio, Internet, and in urban open spaces. To present their products, businesses select suitable voices from voicebanks of media companies, which offer recordings of diverse speakers presenting various speech styles. Although advertisements intended for audio media are widely produced, there are few studies on voices used in advertising and this leads to voices being chosen by intuition (Westermann, 2008; Rodero and Larrea, 2021). More consideration given to the choice of voice might increase the effectiveness of an advertisement. Without such consideration, there is the risk of making a choice, like having a celebrity as the spokesperson, who may draw all the attention onto themselves and the audience remembers them instead of the product (Kuvita and Karlíček, 2014; Erfgen et al., 2015; Grigaliunaite and Pileliene, 2015). The effectiveness of an advertisement may also be affected by intracultural experience. Desmarais and Vignolles (2019) have shown that vocal preferences are not universal, but are influenced by the dominant sales strategy of the culture. If the culture is dominated by a hard sell strategy, which aims to get the client to make a purchase quickly, the commercial messages are direct, explicit, rational, and based on information. The style of performance in this case is rapid, emphatic, and loud. Male voices used in such advertisements are masculine, dramatic, aggressive, or very enthusiastic; female voices do not emphasize femininity and use a happy or casual tone of voice. In the case of a soft sell strategy, where the client is influenced emotionally into making the purchase, the advertising voices are seductive and softer, the speech is slower, and the intonation is kept flat. Consumers prefer voices and advertising styles which are familiar in their cultural environment (Desmarais, 2000; Desmarais and Vignolles, 2019). Rodero et al. (2013) and Martín-Santana et al. (2015, 2017) have noted the overuse of male voices in advertisements in the belief that deep male voices sound more convincing, believable, and persuasive. Their studies have shown that the gender of the spokesperson had no impact on the efficacy of advertisements of non-gender imaged products. However, in the selection of the spokesperson the likability of the spokesperson's voice should be taken into account, which in turn is impacted by both culture and speech style (Altrov et al., 2018; Baus et al., 2019; Pajupuu et al., 2019; Weiss et al., 2021).
The speech style of advertisements is clearly recognizable due to sounding unnatural in both speech tempo and its exaggerated way of speaking with strong emphases (Chattopadhyay et al., 2003; Rodero, 2020; Rodero and Potter, 2021). Listeners have learned to automatically associate this speech style with advertisements and in order to resist the influence of the advert, have learned to tune it out (Michelon et al., 2020). To make advertisements more acceptable to the listener, it is important to choose a suitable voice for the specific product or goal, but to also consider intracultural preferences (Desmarais and Vignolles, 2019).
Predictions forecast an increase in ad spending on both traditional radio advertisements as well as digital audio advertisements (Statista, 2022). Therefore, the need for effective and engaging audio advertising remains. Studies have shown that emotions fostered in listeners by speech features influence their willingness to buy, which is why the importance of choice of voice type cannot be underestimated (Nagano et al., 2021; Rodero and Larrea, 2021). To save time and money in ad production, it is sensible to search for alternatives. One option is to use text-to-speech synthesis instead of a human voice. Rodero (2017) has shown the effectiveness, attention, and recall of synthesized and human voices in a narrative advertising story. The study came to the conclusion that although current synthesizers are now able to produce a relatively natural and intelligible sound, the importance of expressiveness in advertisements and its absence in synthesized speech gave rise to negative ratings for advertisements performed by non-human voices. Synthesized voices are expected to exhibit the same characteristics as human voices which means the acoustic variety of synthesized voices needs to be further improved. Rodero's (2017) findings also showed gender differences. Most participants in the listening test preferred advertisements performed by male voices. There was also a crossover effect – women gave higher ratings to male voices and men lower. The preference for male voices may be explained by the wider use of them in Spanish radio advertising, leading to listeners being accustomed to such a voice environment (see Rodero et al., 2013).
The speaking style of synthesized speech results from the type of speech data used for training the text-to-speech systems. While there are large-scale neutral speech training corpora available for synthesizing neutral speech, collecting or recording similar quantities of expressive speech in all its variety of emotions, attitudes, and styles is time-consuming and expensive, which is why other methods are being explored (see Zhu and Xue, 2020; Schnell and Garner, 2022). Lately a solution has been sought in speech style transfer, which means transferring the style from one signal to another while preserving the latter's content and speaker's identity. A small expressive speech corpus, possibly one with multiple speakers, can be used to train a model of the desired speech style which can then be applied to synthesized neutral speech (see Gao et al., 2019; Kulkarni et al., 2021; Pan and He, 2021; Li et al., 2022; Ribeiro et al., 2022).
In our own study, we set the goal of determining which performance style of advertisement is preferred in Estonia when the advertisement is performed by a synthesized voice. For human voices, we know that Estonians prefer speech styles that do not require a loud voice (Altrov et al., 2018). It is not known what kinds of voices Estonians prefer in advertisements, nor whether the dominant sales strategy in Estonia is hard or soft sell, but the voicebanks of media companies offer voices in two styles – energetic and calm, and advertisements of both types can be heard on the radio. In our study, we used human-like female and male synthesized voices on which we applied two style models trained on a multi-speaker corpus – an energetic and calm one.
We formulated the following research questions:
Q1: Do Estonian listeners prefer synthetic voices speaking in an energetic or calm synthesized advertising style?
Q2: What speech features differentiate the calm and energetic synthesized advertising style?
2. Method
2.1. Text-to-speech synthesis
The speech models were trained using Merlin (Wu et al., 2016). A neural network based speech synthesis system developed at the Center for Speech Technology Research (CSTR), University of Edinburgh. The system relies on the Theano numerical computation library. To convert text to full-context labels, a front-end text processor developed locally in the Institute of the Estonian Language was used (Kiissel, 2022a). We converted generated parameters to signal using the WORLD vocoder (see samples Kiissel, 2022b).
Four voice models were trained on existing available Estonian corpora of emotionally neutral sentences: Female 1 (365 min, 4,701 sentences), Female 2 (449 min, 5,172 sentences), Male 1 (376 min, 4,165 sentences), and Male 2 (330 min, 2,838 sentences). For the purpose of this study, one sample sentence and three short advertising texts were synthesized with every voice.
2.2. Speech style transfer
For voice conversion we used a Tensorflow based style transfer tool (Gao, 2019; Gao et al., 2019). This is a nonparallel emotional speech conversion tool, one that does not require any paired data, transcripts, or time alignment. It enables the transfer of style-related speech characteristics, while preserving the speaker's identity and linguistic content. It is capable of producing conversions of acceptable quality from relatively small corpora. We have tested it, applying models trained on various corpora to Estonian speech (Pajupuu, 2022).
In this study, to train the style transfer models we used voice samples of 120 female actors and 120 male actors reading the same Estonian pretend-advertisement in two styles – calm and energetic – which were sourced from the database of the audio-visual post-production studio Orbital Vox Studios (wav 44.1 kHz, 16 bit, stereo, average length of advertisement 20 sec). We trained the style transfer models (CycleGAN) between energetic and calm female voices and between energetic and calm male voices. Then we applied these models to neutral style synthesized advertisements.
2.3. Listening test
A web based listening test was created. The test consisted of two parts. In the first part, the likability of two male synthesized voices (M1 and M2) and two female synthesized voices (F1 and F2) had to be rated on a 7-point Likert scale, where 1 = not likable at all … 7 = very likable, before style transfer had been applied. For all voices, this sentence was provided for listening to: Olen kõnerobot (nimi) ja õpin reklaame lugema. [I am speech robot (name) and I am learning to read advertisements]. In the second part, an energetic and calm advertising style had been applied to the synthesized voices and every voice read aloud the following three advertisement fragments in both styles. The fragments were selected to avoid their Estonian semantic content from clashing with either performance style:
- Hullud päevad kolmapäevast pühapäevani Vesiku kaubakeskuses. [Crazy days Wednesday to Sunday at Vesiku shopping center.]
- Sinu tegemiste õnnestumised saavad alguse heast ideest. Laenumarket – kõik tarbimislaenud ühest kohast. [The success of your endeavors starts from a good idea. Loan market – all consumer loans from one source.]
- Tule Diili ja vaheta vana uue vastu! [Come to Deal and swap the old one for a new one!]
Both performers and sentences were listened to in a random order. The rating had to be assigned to the likability of the performance on a 7-point Likert scale, where 1 = not likable at all … 7 = very likable. The instruction text was as follows:
We are teaching speech robots to read advertisements. We need your help to find out which advertisement style you prefer as performed by a robot. Please listen to the advertisement fragments and give a rating to the performance style of the advertisement. First get to know the robots that are learning to read advertisements and let us know how you like their voices. You do not have to listen to all of the performances at once, you can save and return later. You can also change previous ratings.
The test was designed to have a duration of ~10 min. The audio files for the listening test and the data of the listening test are included in the dataset (https://figshare.com/projects/Robot_reads_ads/151404).
2.4. Data analysis
The participating raters of the listening test were adults with tertiary education, 8 women (aged 39–56, M = 45.4 years, SD = 6.4) and 10 men (aged 36–53, M = 45.9 years, SD = 7.0). Raters participated voluntarily. The listening test caused no harm to any participant, the identity of the participants has been kept confidential, and no conflict of interest can be identified.
All scores for each rater were normalized using the formula
where, x is the score, X is the mean of the rater's scores, and s is the standard deviation of the rater's scores. We classified performances with scores above zero as likable, and those below zero as unlikable.
To find out the degree of agreement among the raters (inter-rater reliability), the intra-class correlation coefficient (ICC2k) was calculated using the “psych” package in R (Revelle, 2021). A Welch Two Sample t-test was used to determine whether the advertisement style affected likability ratings (R Core Team, 2022).
2.5. Acoustic analysis
For the acoustic analysis of the calm and energetic synthesized advertising styles we used the open-source toolkit openSMILE (Eyben et al., 2010, 2013). The parameters of the extended Geneva Minimalistic Acoustic Parameter Set (eGeMAPS) were calculated for each performance. The use of eGeMAPS is promising due to the set of acoustic parameters extracted from speech having been specially developed for paralinguistic speech analysis (Eyben et al., 2016). These 88 parameters include statistical properties (arithmetic mean, coefficient of variation, percentiles, etc.) calculated for a set of time-varying low-level acoustic features, including frequency-related, energy-/amplitude-related, spectral, and temporal features (Eyben et al., 2016). All parameters were normalized with the R function scale. To identify the acoustic features that distinguish the calm and energetic advertising styles, the Kruskal–Wallis Test was used, and the statistically significant parameters were ordered by the test statistic (R Core Team, 2022).
3. Results
A moderate to good reliability was found within rater measurements. The average measure ICC2k was 0.81 with a 95% confidence interval from 0.70 to 0.90 F(27, 513) = 5.3, p < 0.0001.
The results of the listening test revealed that the original neutral synthesized voices were considered more likable than voices with advertising styles transferred on them [Moriginal = 0.39 vs. Mcalm =0.09, t(130) = 2.48, p = 0.014; Moriginal = 0.39 vs. Menergetic = −0.21, t(141) = 4.81, p < 0.001]. Of the advertising styles transferred on the original voices, the listeners overwhelmingly preferred the calm advertisement style [Mcalm = 0.09 vs. Menergetic = −0.21, t(474) = 3.4, p < 0.001], see Figure 1.
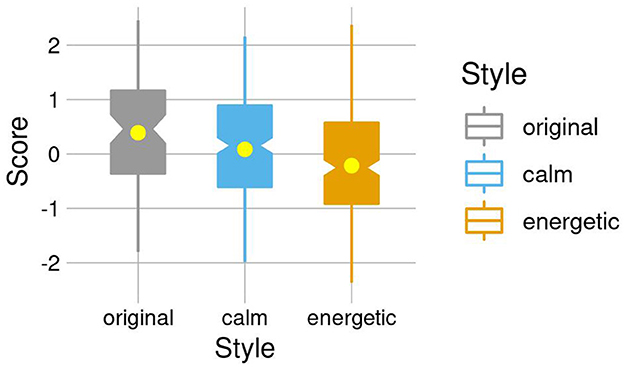
Figure 1. The normalized likability scores for the original synthesized speech and the style-transferred calm and energetic advertising styles.
Looking at the synthesized voices separately, the original neutral synthesized voices ranked best to worst as follows: F2 (M = 0.55), M2 (M = 0.41), F1 (M = 0.39), M1 (M = 0.20), but there was no significant difference in their scores. The calm advertising style was rated significantly higher than the energetic style in the cases of F1 and M2 [MF1_calm = 0.39 vs. MF1_energetic = −0.29, t(113) = 2.42, p = 0.017; MM2_calm = 0.02 vs. MM2_energetic = −0.69, t(117) = 4.21, p < 0.0001]. There was no significant difference between the two styles for F2 and M1 [MF2_calm = 0.03 vs. MF2_energetic = 0.14, t(117) = −0.58, p = 0.527; MM1_calm = 0.19 vs. MM1_energetic = −0.01, t(118) = 1.15, p = 0.252], see Figure 2.
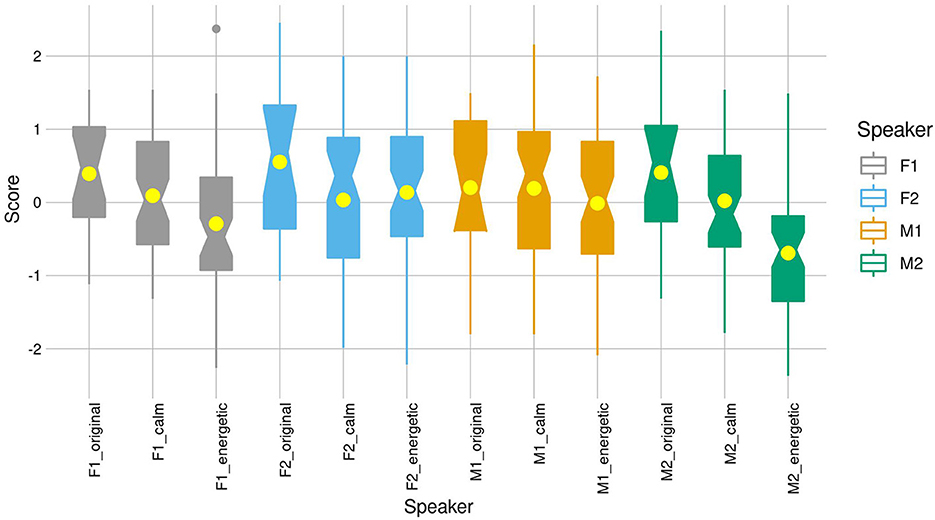
Figure 2. The normalized likability scores for the original synthesized speech and the style-transferred calm and energetic advertising styles for each voice separately.
The calm and energetic advertisement performance styles were acoustically quite different: out of the 88 eGeMAPS parameters, 37 significantly differentiated these styles, of which 7 were frequency related, 10 energy/amplitude related, and 20 were spectral parameters. Tempo parameters did not belong among the differentiating features. The amount of significant parameters differentiating the styles reveals that styles might not be characterizable by a single feature, but rather a combination of features, some of which might lack a specific perceptible counterpart. The acoustic eGeMAPS parameters that differentiate the styles are presented with descriptions in the Supplementary material (see List of eGeMAPS parameter abbreviations and Supplementary Table 1).
The parameters that offer a more evident interpretation indicate that a calm advertisement style was characterized by lower pitch (lower f0), quieter voice (lower loudness, smaller mean alpha ratio), with no abrupt changes in loudness (smaller rising and falling slopes of loudness), and a sonorous voice (less steep spectral slope). The calm advertising style was also characterized by a more neutral rather than an emotional performance (see Liu and Xu, 2014; Pralus et al., 2019; Voße et al., 2022; lower spectral flux, lower f0, bigger rising slope f0, and smaller falling slope of f0, higher Hammarberg index), and the calm style is also differentiated from the energetic by parameters related to timbre (higher MFCC2, MFCC3, MFCC4, see Nordström, 2019, p. 27). See Figure 3.
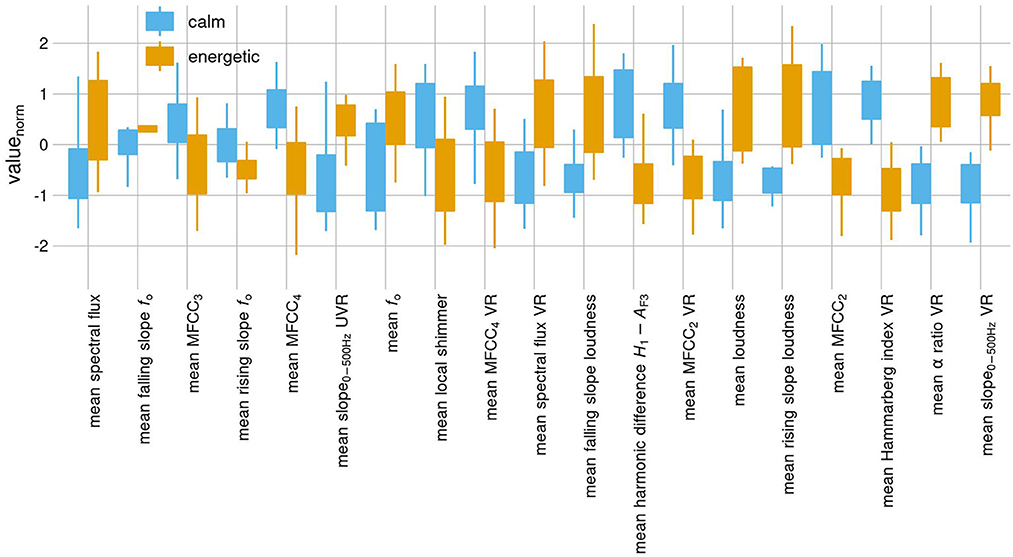
Figure 3. The acoustic parameters differentiating the advertising styles. Spectral parameters: slope 0–500 Hz = linear regression slope of the logarithmic power spectrum for 0–500 Hz region, alpha ratio = ratio of the summed energy from 50–1000 Hz, Hammarberg index = the ratio of the strongest energy peaks in the 0–2 kHz vs 2–5 kHz regions, MFCC2,3,4 = second, third, and fourth Mel-frequency cepstral coefficient; harmonic difference H1-AF3 = ratio of energy of the first f0 harmonic (H1) to the energy of the highest harmonic in the third formant range (A3), spectral flux = difference of the spectra of two consecutive frames; Energy/Amplitude related parameters: loudness = estimate of perceived signal intensity from an auditory spectrum, rising/falling slope loudness = slope of rising/falling signal parts of loudness, shimmer = difference of the peak amplitudes of consecutive f0 periods; Frequency related parameters: f0 = logarithmic fundamental frequency on a semitone frequency scale, starting at 27.5 Hz (semitone 0), rising/falling slope f0 = slope of rising/falling signal parts of f0; VR, voiced regions; UVR, unvoiced regions (see descriptions in Eyben et al., 2016).
4. Discussion
In this study we attempted to answer the question of which performance style Estonians prefer in audio advertisements, if the advertisement is performed by a synthesizer. We were also interested in which acoustic features characterize the performance styles. The audio advertisements we obtained from media companies were described by them as calm or energetic based on performance style. A similar division has arisen in intercultural studies, where speech styles in advertising have been associated with the dominant sales strategy in the culture: a calmer style corresponds to the soft sell strategy while the energetic style corresponds to the hard sell strategy (see Desmarais, 2000; Desmarais and Vignolles, 2019).
We trained transferrable style models on 120 advertisements in the calm style and 120 in the energetic style, performed by female and male actors. These were then transferred on already extant voices trained on a neutral speech text-to-speech synthesizer corpus.
In the listening test, the participants rated all the original neutral synthesized voices without style transferred speech styles as more likable than the average (see Figure 2). Of the voices that had advertisement performance styles transferred on them, the calm style was considered likable and the energetic style unlikable (Figures 1, 2). Acoustically, the calm performance style was differentiated from the energetic by 37 eGeMAPS spectral, frequency, and energy/amplitude related properties, which summarily showed that compared to the energetic, the calm performance style is more neutral, the voice lower and quieter without rapid changes in loudness, but also more sonorous rather than breathy (see Figure 3, Supplementary Table 1). Using eGeMAPS makes the results from the various studies that use it easy to compare, but it is not always obvious how these parameters link to acoustically perceived speech characteristics. The use of eGeMAPS would also provide a framework for comparing advertisement styles performed by synthetic voices and humans.
The calm style preferred by listeners aligns with the soft sell sales strategy (Desmarais, 2000; Desmarais and Vignolles, 2019), but we cannot conclude that the soft sell strategy dominates Estonia. Earlier studies on the human voice conducted in Estonia have shown that for other speech styles, Estonians also prefer a quieter and more neutral voice (Altrov et al., 2018). As far as we know, different speech styles in advertisement performances have not been compared in other cultures; rather, the advertising style described in studies seems to correspond to the energetic style that studies found is both unnatural in speech tempo and excessive emphaticism (Chattopadhyay et al., 2003; Rodero, 2020; Rodero and Potter, 2021). Therefore, it is not known whether advertisements performed in the calm style may be a better fit for listeners in other cultures as well, regardless of the sales strategy.
Concerning the gender of the performer of the advertisement, unlike Rodero's (2017) study on synthesized advertisements, our research did not show a gender preference in synthetic voices (see Figure 2). Whether female or male voices are preferred in advertisements is likely tied to cultural habits: if there are more male voices heard in advertisements in a culture, as Rodero et al. (2013) and Rodero (2017) have noted about Spain, then the preference is for male voices.
Our study reaffirmed the necessity of studying style preferences intraculturally. Considering the results, advertisers can make audio advertisements more palatable for the listeners of the corresponding cultural environment and thereby more effective. The impact of language alongside culture could also be further researched by studying the style preferences of listeners from different language groups within one culture.
From a technical standpoint, our study has shown that style transfer has potential and an effect can be achieved even when training style models on small corpora. Yet, the styles were rated significantly lower than the original neutral synthesized voices and the effect of style transfer was different on every voice (Figure 2). Going forward, the scale of the training corpus could be increased to see if that would improve the quality of the transfer. For a better comparison, the original voices should be trained on an equal amount of emotionally neutral sentences, so that style characteristics would not be amplified to different degrees. Furthermore, the advertisement fragments that are synthesized for rating should be longer than a sentence or two, so as to highlight the applied style model better.
5. Conclusion
Using style transfer on already existing text-to-speech synthetic voices, we discovered that Estonian listeners prefer a synthesized voice performing in a calm advertising style over an energetic one. We conclude that when using synthesizers for voicing advertisements, they could benefit from using a calm style when advertising in Estonia. Further research could show if there are differences between listener preferences of advertisement styles as performed by synthesized voices and humans.
Data availability statement
The datasets presented in this study can be found in online repositories. The names of the repository/repositories and accession number(s) can be found below: https://figshare.com/projects/Robot_reads_ads/151404.
Ethics statement
Ethical review and approval was not required for the study on human participants in accordance with the local legislation and institutional requirements. Written informed consent for participation was not required for this study in accordance with the national legislation and the institutional requirements.
Author contributions
HP, JP, and RA contributed to the conception of the study. HP, JP, and IK wrote sections of the manuscript. IK and JP were responsible for the speech stimuli generation. HP and RA compiled the listening test and conducted it. HP and JP did the data analysis and interpretation. All authors contributed to the manuscript revision, approved the submitted version, and had full access to all the data in the study.
Funding
This work was financed by the European Union through the European Regional Development Fund (Center of Excellence in Estonian Studies), by the National Programme for Estonian Language Technology 2018–2027, and by basic governmental financing of the Institute of the Estonian Language from the Estonian Ministry of Education and Research.
Acknowledgments
The authors thank Orbital Vox Studios, an audio-visual post-production studio, for the opportunity to use their voice database in this study. The authors wish to express their gratitude to the participants of the listening test.
Conflict of interest
The authors declare that the research was conducted in the absence of any commercial or financial relationships that could be construed as a potential conflict of interest.
Publisher's note
All claims expressed in this article are solely those of the authors and do not necessarily represent those of their affiliated organizations, or those of the publisher, the editors and the reviewers. Any product that may be evaluated in this article, or claim that may be made by its manufacturer, is not guaranteed or endorsed by the publisher.
Supplementary material
The Supplementary Material for this article can be found online at: https://www.frontiersin.org/articles/10.3389/fcomm.2023.1089577/full#supplementary-material
References
Altrov, R., Pajupuu, H., and Pajupuu, J. (2018). Phonogenre affecting voice likability. Proc. Int. Conf. Speech Prosody 2018, 177–181 doi: 10.21437/SpeechProsody.2018-36
Baus, C., McAleer, P., Marcoux, K., Belin, P., and Costa, A. (2019). Forming social impressions from voices in native and foreign languages. Sci. Rep. 9, 1–14. doi: 10.1038/s41598-018-36518-6
Chattopadhyay, A., Dahl, D. W., Ritchie, R. J. B., and Shahin, K. N. (2003). Hearing voices: the impact of announcer speech characteristics on consumer response to broadcast advertising. J. Consum. Psychol. 13, 198–204. doi: 10.1207/S15327663JCP1303_02
Desmarais, F. (2000). Authority versus seduction: the use of voice-overs in New Zealand and French television advertising. Media Int. Austr. Cult. Policy 96, 135–152. doi: 10.1177/1329878X0009600116
Desmarais, F., and Vignolles, A. (2019). Customer engagement through the vocal touchpoint: an exploratory cross-cultural study. Adv. Adv. Res. 2019, 67–78. doi: 10.1007/978-3-658-24878-9_6
Erfgen, C., Zenker, S., and Sattler, H. (2015). The vampire effect: when do celebrity endorsers harm brand recall? Int. J. Res. Market. 32, 155–163. doi: 10.1016/j.ijresmar.2014.12.002
Eyben, F., Scherer, K., Schuller, B., Sundberg, J., Andre, E., Busso, C., et al. (2016). The Geneva minimalistic acoustic parameter set (GeMAPS) for voice research and affective computing. IEEE Trans. Aff. Comput. 7, 190–202. doi: 10.1109/TAFFC.2015.2457417
Eyben, F., Weninger, F., Gro,ß, F., and Schuller, B. (2013). Recent developments in openSMILE, the Munich open-source multimedia feature extractor. Proc. ACM Int. Conf. Multimedia. 2013, 835–838. doi: 10.1145/2502081.2502224
Eyben, F., Wöllmer, M., and Schuller, B. (2010). openSMILE: The Munich versatile and fast open-source audio feature extractor. Proc. ACM Int. Conf. Multimedia. 2010, 1459–1462. doi: 10.1145/1873951.1874246
Gao, J. (2019). Emotional Speech Conversion Using Nonparallel Data. Available online at: https://github.com/bottlecapper/EmoCycleGAN (accessed November 3, 2018).
Gao, J., Chakraborty, D., Tembine, H., and Olaleye, O. (2019). Nonparallel emotional speech conversion. Proc. Interspeech 2019, 2858–62. doi: 10.21437/Interspeech.2019-2878
Grigaliunaite, V., and Pileliene, L. (2015). Determination of the impact of spokesperson on advertising effectiveness. Int. J. Manage. Account. Econ. 2, 810–822.
Kiissel, I. (2022a). Merlinil põhinev eesti keele kõnesüntesaator [Merlin based Estonian speech synthesizer]. Available online at: https://github.com/ikiissel/mrln_et (accessed April 3, 2023).
Kiissel, I. (2022b). Merlinil põhinevad sünteeshääled [Merlin-based synthetic voices for Estonian]. Available online at: https://www.eki.ee/~indrek/mrln_et/ (accessed April 3, 2023).
Kulkarni, A., Colotte, V., and Jouvet, D. (2021). “Improving transfer of expressivity for end-to-end multispeaker text-to-speech synthesis,” in Proeedings of 29th European Signal Processing Conference (Dublin), 31–35.
Kuvita, T., and Karlíček, M. (2014). The risk of vampire effect in advertisements using celebrity endorsement. Central Eur. Bus. Rev. 3, 16–22. doi: 10.18267/j.cebr.89
Li, X., Song, C., Wei, X., Wu, Z., Jia, J., Meng, H., et al. (2022). Towards cross-speaker reading style transfer on audiobook dataset. Proc. Interspeech 2022, 5528–5532. doi: 10.21437/Interspeech.2022-11223
Liu, X., and Xu, Y. (2014). “Body size projection by voice quality in emotional speech—Evidence from Mandarin Chinese,” in Social and Linguistic Speech Prosody: Proceedings of the 7th International Conference on Speech Prosody, Dublin, 974–977.
Martín-Santana, J. D., Muela-Molina, C., Reinares-Lara, E., and Rodríguez-Guerra, M. (2015). Effectiveness of radio spokesperson's gender, vocal pitch and accent and the use of music in radio advertising. BRQ rly 18, 143–160. doi: 10.1016/j.brq.2014.06.001
Martín-Santana, J. D., Reinares-Lara, E., and Reinares-Lara, P. (2017). Influence of radio spokesperson gender and vocal pitch on advertising effectiveness: the role of listener gender. Spanish J. Market. ESIC 21, 63–71. doi: 10.1016/j.sjme.2017.02.001
Michelon, A., Bellman, S., Faulkner, M., Cohen, J., and Bruwer, J. (2020). A new benchmark for mechanical avoidance of radio advertising. J. Adv. Res. 60, 407–416. doi: 10.2501/JAR-2020-007
Nagano, M., Ijima, Y., and Hiroya, S. (2021). Impact of emotional state on estimation of willingness to buy from advertising speech. Proc. Interspeech 2021, 2486–90. doi: 10.21437/Interspeech.2021-827
Nordström, H. (2019). Emotional Communication in the Human Voice. [dissertation thesis]. Stockholm: Stockholm University Sweden.
Pajupuu, H., Altrov, R., and Pajupuu, J. (2019). The effects of culture on voice likability. Trames J. Hum. Soc. Sci. 23, 239.−257. doi: 10.3176/tr.2019.2.08
Pajupuu, J. (2022). Samples of Speech Style Transfer for Estonian. Available online at: https://github.com/pajupuujh/CycleGAN (accessed April 3, 2023).
Pan, S., and He, L. (2021). Cross-speaker style transfer with prosody bottleneck in neural speech synthesis. Proc. Interspeech.2021, 4678–4682, doi: 10.21437/Interspeech.2021-979
Pralus, A., Fornoni, L., Bouet, R., Gomot, M., Bhatara, A., Tillmann, B., et al. (2019). Emotional prosody in congenital amusia: impaired and spared processes. Neuropsychologia 134, 107234. doi: 10.1016/j.neuropsychologia.2019.107234
R Core Team (2022). R: A Language and Environment for Statistical Computing. Vienna: R Foundation for Statistical Computing.
Revelle, W. (2021). psych: Procedures for Psychological, Psychometric, and Personality Research. Evanston, IL: Northwestern University. R package version 2.1.6.
Ribeiro, M. S., Roth, J., Comini, G., Huybrechts, G., Gabry,ś, A., Lorenzo-Trueba, J., et al. (2022). “Cross-speaker style transfer for text-to-speech using data augmentation,” in ICASSP 2022 - 2022 IEEE International Conference on Acoustics, Speech and Signal Processing. England: ICASSP, 6797–6801.
Rodero, E. (2017). Effectiveness, attention, and recall of human and artificial voices in an advertising story. Prosody influence and functions of voices. Comput. Hum. Behav. 77, 336.−346. doi: 10.1016/j.chb.2017.08.044
Rodero, E. (2020). Do your ads talk too fast to your audio audience? J. Adv. Res. 60, 337–349. doi: 10.2501/JAR-2019-038
Rodero, E., and Larrea, O. (2021). “Audio design in branding and advertising,” in Innovation in Advertising and Branding Communication, ed. L. Mas-Manchón (New York, NY: Routledge Research in Communication Studies) 69–85.
Rodero, E., Larrea, O., and Vázquez, M. (2013). Male and female voices in commercials: Analysis of effectiveness, adequacy for the product, attention and recall. Sex Roles 68, 349–362. doi: 10.1007/s11199-012-0247-y
Rodero, E., and Potter, R. F. (2021). Do not sound like an announcer. The emphasis strategy in commercials. Psychol. Market. 38, 1417–1425. doi: 10.1002/mar.21525
Schnell, B., and Garner, P. N. (2022). Investigating a neural all pass warp in modern TTS applications. Speech Commun. 138, 26–37. doi: 10.1016/j.specom.2021.12.002
Statista (2022). Digital Audio Advertising – Worldwide. Available online at: https://www.statista.com/outlook/amo/advertising/audio-advertising/worldwide#ad-spending (accessed April 3, 2023).
Voße, J., Niebuhr, O., and Wagner, P. (2022). How to motivate with speech. Findings from acoustic phonetics and pragmatics. Front. Commun. 7, 910745. doi: 10.3389/fcomm.2022.910745
Weiss, B., Trouvain, J., and Burkhardt, F. (2021). “Acoustic correlates of likable speakers in the NSC database,” in Voice Attractiveness. Studies on Sexy, Likable, and Charismatic Speakers, eds. B. Weiss, J. Trouvain, M. Barkat-Defradas, and J. J. Ohala (Singapore: Springer Verlag) 245–262.
Westermann, C. F. (2008). “Sound branding and corporate voice–strategic brand management using sound,” in Usability of Speech Dialog Systems. Listening to the Target Audience, ed. T. Hempel (Berlin, Heidelberg: Springer), 147–155.
Wu, Z., Watts, O., and King, S. (2016). Merlin: an open source neural network speech synthesis system. Proc. ISCA Workshop SSW 9, 202–207. doi: 10.21437/SSW.2016-33
Keywords: speech styles, advertisements, synthesized speech, style transfer, acoustic features, GeMAPS
Citation: Pajupuu H, Pajupuu J, Altrov R and Kiissel I (2023) Robot reads ads: likability of calm and energetic audio advertising styles transferred to synthesized voices. Front. Commun. 8:1089577. doi: 10.3389/fcomm.2023.1089577
Received: 04 November 2022; Accepted: 28 March 2023;
Published: 17 April 2023.
Edited by:
Plinio Almeida Barbosa, State University of Campinas, BrazilReviewed by:
Luma Miranda, Eötvös Loránd University, HungaryJacek Kudera, University of Trier, Germany
Copyright © 2023 Pajupuu, Pajupuu, Altrov and Kiissel. This is an open-access article distributed under the terms of the Creative Commons Attribution License (CC BY). The use, distribution or reproduction in other forums is permitted, provided the original author(s) and the copyright owner(s) are credited and that the original publication in this journal is cited, in accordance with accepted academic practice. No use, distribution or reproduction is permitted which does not comply with these terms.
*Correspondence: Hille Pajupuu, aGlsbGUucGFqdXB1dUBla2kuZWU=