- 1Department of General Psychology and Methodology, University of Bamberg, Bamberg, Germany
- 2Research Group EPÆG (Ergonomics, Psychological Æsthetics, Gestalt), Bamberg, Germany
- 3Bamberg Graduate School of Affective and Cognitive Sciences (BaGrACS), Bamberg, Germany
Selfies are taken to communicate about mental conditions, aims, loves, and commitments. Until now, we lack a unified nomenclature or established classification system for selfies. We can retrieve information about the different types of selfies from various indicators. Most commonly, this is done by analyzing metatags, hashtags, or the linked message of the respective post. Alternatively, we can categorize the depicted selfie's subject or analyze how viewers describe the impression a selfie has on them. We refer to this latter approach as Semantics of Selfies (SoS). In the present study, participants (N = 132) were asked to generate spontaneous associations of selfies from a pool of 1,001 selfies in total. Cluster analyses revealed five main categories (Aesthetics, Imagination, Trait, State, and Theory of Mind) constituting a characteristic semantic profile for selfies. Consequently, the present article provides an understanding of how certain selfies affect viewers to perceive specific qualities in the self-portrayed person in a very compact visual form.
1. Introduction
Self-portraits have been a powerful means to communicate about our inner selves for over five centuries (Carbon, 2017). Since the arrival of easy-to-handle devices that allow quick and effortless self-photographs, we have seen a dramatic increase in people who take “selfies.” Taking selfies and disseminating them via social media has become a common habit in our daily lives. According to estimates by leading Internet platforms (e.g., Instagram and Google), the number of uploaded self-portraits per year increased dramatically during the last decade. For instance, Google estimated a yearly upload of ~24 billion images in 2019 (Google, 2019). Indeed, most people in today's world have experience with taking and sharing selfies. We use them as a very compact format of self-reference (Carbon, 2017) to express ourselves, tell stories about our current life, and show our moods in a compressed form—disseminating selfies is nowadays one of the core means to communicate about body images, our physical appearance, and most importantly, about the mental image of feelings, perceptions, thoughts, and beliefs about the own body (Hanna et al., 2017; Saunders and Eaton, 2018). Selfies are a perfect medium to create a so-called digital identity (Belk, 2013) which can be used for various digital platforms such as messenger services or dating portals. Similarly, research from the field of marketing and communication investigated how individuals can create their so-called “human brand” through classic channels of communication such as curricula vitae or, e.g., via blogging or social marketing (Arsel and Bean, 2013; McQuarrie et al., 2013; Parmentier et al., 2013). The human brand is described as a celebrity, a person who is well-known for being well-known (Boorstin, 1964) with the power that public recognition affords them (Rojek, 2001; Marshall, 2014). However, nowadays social media platforms also allow non-celebrities to build an image and present themselves to a broad audience without any great effort and the necessity of becoming famous.
In the field of visual perception, it has been shown that we can (positively) influence the perception of personality-, health-, and mating-related variables by simply applying standard selfie techniques such as manipulating the camera perspective using our arm or a selfie stick (Schneider and Carbon, 2017). Rotating the camera (around the head) for just some degrees is known to affect the perception of certain personality variables: Presenting the left hemiface by pronouncedly showing the left side of the face leads to an increase in attractiveness, whereas showing the right hemiface positively affects the perception of helpfulness, sympathy, and intelligence. Lowering the camera by 30° (taking a selfie below the head or raising the head) leads to a large effect of higher perceived body weight (up to 10 kilograms), whereas raising the camera by 30° (taking a selfie above the head or lowering the head) results in substantially lower perceived body weight (up to 10 kg), see, e.g., Schneider et al. (2012).
To better understand the goals of the present article and the nature of selfies in general, we would first like to present a theoretical framework based on (still relatively sparse) empirical findings from the research field of selfies. Selfies have attracted a series of perceptual researchers to conduct empirical studies on or analyzing several qualities of selfies (Bruno and Bertamini, 2013; Bruno et al., 2014, 2018; Sorokowska et al., 2016; Jarreau et al., 2019). Past research mainly focuses on what (e.g., personality) we can derive from selfies (Qiu et al., 2015; Sorokowska et al., 2016; Musil et al., 2017), how we take and perceive selfies (e.g., Schneider and Carbon, 2017, for a systematic examination), and why we are taking them (e.g., Diefenbach and Christoforakos, 2017). Interestingly, there are apparent great differences in how selfies are taken. Most of the depictions might still be “classical self-ies,” but we also see “we-fies” that are easier to produce since the advent of aids such as selfie sticks. Selfies also differ in the way of using such aids; for instance, some shoot by using the prostrated hand, while others use extensions or photograph themselves through a mirror; for an overview, see Table 1. However, up to now, there is no consistent or established terminology or systematic scientific investigation on such typical characteristics, similarities, and varieties in selfies. We can qualify selfies as a specific mode of communication (Parmentier et al., 2013), but which kinds of communication are aimed to be conveyed (see “main aims” column in Table 1)? Correspondingly, Carbon (2017) hypothesized that there are three main aims of a selfie-taker: (A) self-expression, (B) documentation, and (C) performance. The author argues that (A) self-expression is the idiosyncratic value of any kind of self-portrait. It refers to the principal motive of a selfie-taker to share emotional and cognitive states. It can also make an “off” or even express one's uniqueness and extravagance—a very good example to illustrate this self-expression principle is Dürer's famous 1500 AD self-portrait (Carbon, 2017). The author provides a comprehensive overview and a preliminary classification system of the types of contemporary photographic selfies and compares them with painted self-portraits of historic dimensions. Carbon (2017) suggests that selfies, as well as self-portraits (e.g., paintings), can be used as a tool for presenting the self and refer to the “conditio humana.” (B) Documentation is suggested to refer to the aim of sharing a certain status quo, e.g., showing significant achievements or milestones in one's life. Within this category of aims, a selfie-taker often holds their new driver's license to express the new sensation of freedom or takes a selfie from the hospital bed to state that the surgery was luckily overcome (see, e.g., Figure 7, first selfie upper row). (C) Performance refers to artistic and esthetic abilities of the self-portrayer. This specific aspect of performance might be mostly important for classical self-portraits (e.g., paintings), but we would like to expand Carbon (2017) definition of performance by adding further performance fields. Within the performance aim category, we would also include aspects of physical or mental abilities in a situational and a personality-related sense. Paradigmatic examples might be the portraying of coping a challenging situation (driving through deep water with a 4WD car) or the depicting of a general performance ability, e.g., taking a selfie while routinely doing the Ironman Triathlon.
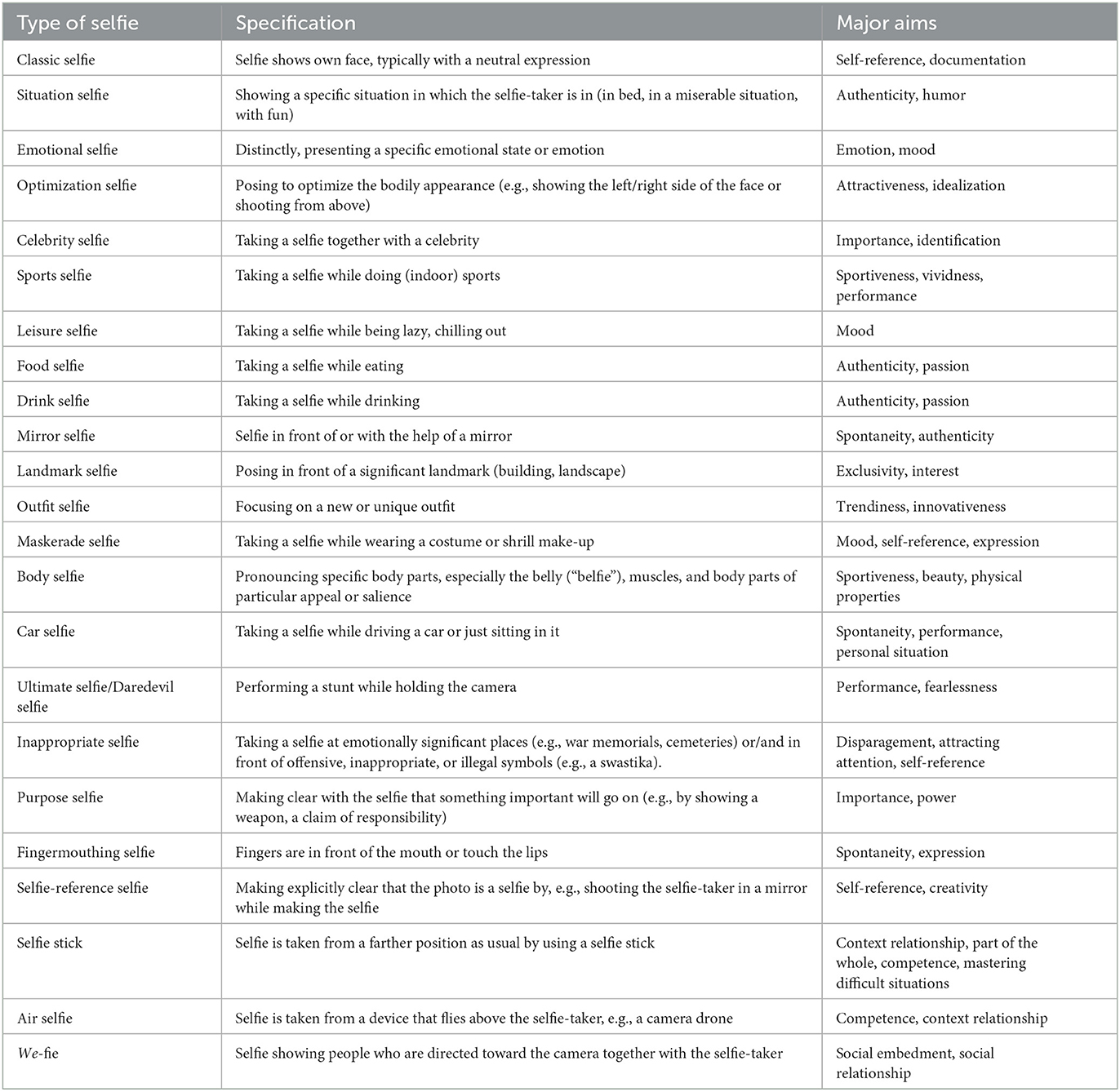
Table 1. Typical types of selfies, along with short specifications plus the major aims of the respective selfie-taker, based on the initial classification system of Carbon (2017).
Consequently, we postulate distinct categories of selfies directly linked to the selfie-taker's specific intentions and motives. In other words, for different narratives to be told via selfies, we need specific types of selfies. This makes it necessary that selfies contain semantic information that assists the viewer of the selfie in easily making conclusions about the meaning and aim of the scene and, ultimately, about the selfie-taker. This information is what we call Semantics of Selfies (SoS) in the following. The SoS will always be susceptible to interpretations as we hardly ever know the motive behind the shooting (and post-processing) of selfies—and even if we know the motive (given the impression of a Daredevil selfie, see Table 1), it is improbable that the incorporated message can correctly and universally be read and understood by the perceivers. Eagar and Dann (2016) provided necessary pre-work by analyzing respective hashtags added to selfies by selfie-takers across a large number of selfies. They revealed nine types (so-called “genres”) of different selfies, namely autobiographic selfies (selfies to document a person's life, e.g., important events like birthdays, but also different states of daily life, e.g., shopping or simply being bored), which is similar to Carbon (2017) documentation aim, self-parody selfies, social media parody selfies (both as a stylistic device of telling stories), propaganda selfies (aim to gain medial attraction and responses), romance of togetherness selfies, romance of aloneness (both describe the efforts of the selfie-taker to present her/his social connections in real life), self-help selfies (as a function of self-achievement in fields such as beauty and physical fitness), travel diary selfies (referring to the location and surrounding of the selfie-taker), and coffee table book selfies (selfies as a solely esthetic stylistic device for non-verbal communication). Focusing on the intentions and posting habits of persons, Williamson et al. (2017) investigated who posts self-presentations (such as selfies) across popular social media platforms (Snapchat, Instagram, LinkedIn, Facebook, and Twitter). The authors could show that, e.g., Twitter users post selfies to clarify their personality or other personal attributes to a public who does not know them on an interpersonal level. In contrast, other platforms such as Facebook, Instagram, and Snapchat tend to be used to target messages to “friends.” In another large-scale investigation of 2.5M selfies from Instagram, Deeb-Swihart et al. (2017) revealed a typology of emergent selfie categories that represent emphasized identity statements. However, they also emphasize in their conclusion that the question remains how the viewer perceives and interprets these selfies in the sense of the SoS. Accordingly, we raise the question of whether we can find standard semantic information in the selfies to provide a consistent terminology. This semantic information can neither be derived solely from objective visual information [e.g., metric image-related data, image statistics or face-specific information such as the face-ism index (Archer et al., 1983), or facial expression classification] nor from text-based information (e.g., hashtags) because a fundamental level of information is missing: the assigned message to a selfie, for instance, the interpreted motive of the selfie-taker (e.g., attempting to be perceived as authentic, trendy, attractive, or even fearless). Although individual interpretations of what message a selfie transports will differ, we will be able to identify common impressions a selfie makes. Such an approach based on individual interpretations might help us identify different selfie categories based on such semantics.
2. Methods
2.1. Participants
A total of 132 persons participated in an online-based assessment voluntarily. No demographic data were collected since we had no specific hypotheses regarding such variables as age or male/female/other categories of gender. Most participants were recruited by online announcements on social networks (e.g., Facebook groups). Students of the University of Bamberg were mainly recruited by announcement boards, and specifically all undergraduate students (n = 52) gained additional course credit to fulfill course requirements. Participants had to give a web-based signed consent to participate in the study. All procedures and treatments of participants were under the Declaration of Helsinki and followed the ethical guidelines of the University of Bamberg, which had been approved by an umbrella declaration on psychophysical studies by the University Ethics Committee on 18 August 2017.
2.2. Materials
For the present study, we used the “Selfiecity” database (Tifentale and Manovich, 2015) with k = 3,200 pictures. We applied our strict definition of a selfie as being a self-portrait, taken by a mobile camera (smartphone or camera) using the own hands or a selfie stick. We excluded all selfies not meeting these inclusion criteria. There was no limitation regarding the depicted person exposing the whole body or only her or his face. The number was further reduced by our selection criterion of selfies showing no text passages or ads on the pictures. Our selected selfies should be maximally interpreted by the specific way of portraying the person but not by explicit information conveyed by written information. The final selection of pictures comprised k = 1,001 (692 female) selfies. As the assessment of all these selfies in a row would be too laborious for single persons causing fatigue and probably invalid data, we selected the target pictures by a random “pull without replacement” algorithm, retrieving 15 pictures from the entire picture base for each participant. We established a picture-assignment procedure that ensured that (a) every selfie was evaluated by approximately the same number of observers and that (b) a sufficient variance of selected selfies was achieved. Each picture was presented in color on a middle gray background and was standardized to a size of 640 × 640 pixels (please note that all pictures of the Selfiecity database were cropped and are only available in a resolution of 640 × 640 pixels).
2.3. Procedure
To maximize the comparability across viewers and to offer a viewing mode where the whole selfie can be perceived at once, we asked our participants to avoid the use of mobile devices (such as mobile phones and tablets). However, we propagated the use of desktop or high-resolution notebook PCs. Furthermore, we asked the participants to use the full-screen mode of their browser to reduce distracting visual cues. Participants were given five free text fields beneath the presented stimuli, where they had to input their first five free associations concerning the presented selfie. To enforce spontaneous impressions to be reported, we provided the following instruction for all trials: “Please take a look at the presented picture and write down your first five spontaneous associations which intuitively come up to your mind. Such an association can be single words, a sentence, or whatever you think about the depiction. There are no false or correct answers—we are interested in your spontaneous impression. Do not think too long, but still take your time. Do not leave out a text field and click ‘resume’ before you finish the trial.” The next trial automatically started when all five text fields had been filled out—at least by one word each (no solitary special characters or numbers were accepted). Since 1,001 (pictures) × 5 (associations) = 5,005 responses in total were too much for a single observer, each participant was allocated to 15 randomly selected selfies by the algorithm mentioned above. As a result, each selfie was rated by more than one participant on average (M = 1.63). Accordingly, we decided to aggregate the response of >2 raters according to the procedure described in the section data pre-processing below. This step finally resulted in a single superordinate category per selfie. Second- or third-rater answers were considered in case of missed answers or denied answers (e.g., “no answer” or letter salad to skip the trial). The whole online study was automatically terminated after all pictures were processed to gain at least five associations per selfie. We set it to five instances to widen the variety of possible associations.
2.4. Data pre-processing
For data pre-processing, we oriented to an approach in the field of qualitative data analysis introduced by Mayring (2000) and practically applied to the field of empirical esthetics by Augustin et al. (2012), where the core concepts and associations are extracted from participant's listings by processing the data via the following steps within phase 1:
1. Correction of spelling and typos. The response was considered a missing value if we could not identify the correct word.
2. Extraction of task-related parts from sentences. Example: the sentence bad colored hair was reduced to bad color and hair; the sentence with a jacket in the bathroom was split into jacket and bathroom. Importantly, such a separation was only done if several parts of a phrase were clear and had informative value. In contrast, phrases like blurry picture remained as they were.
3. Removal of articles for nouns (e.g., “the” or “a”).
4. Removal of qualifiers, such as very or too. Example: Very likable was shortened to likable. However, in the case of the phrase, e.g., not vain, it remained as it was due to the fact that the qualifier was used to denote an opposite. Consequently, such phrases were later processed in the first- and second-reduction steps (see details in the following).
5. Pooling different spellings of the same concept (e.g., extrovert vs. extravert).
6. Pooling singulars and plurals of the same noun.
7. Pooling words that have the same stem and are synonyms, such as Abendbrot and Abendessen (both for dinner) or Straßenlaterne and Straßenlampe (both stand for street light).
The second phase consisted of a two-step data reduction. In the first step, we reduced the data to 48 categories (out of 5,005 single associations in total) which were then processed by the second step of the data reduction (see Table 2). In this final step, categories were merged to describe umbrella constructs, yielding 26 final categories (see Table 2 and Figure 1). All the described analyses in the Methods section are based on these superordinate categories (n = 26).
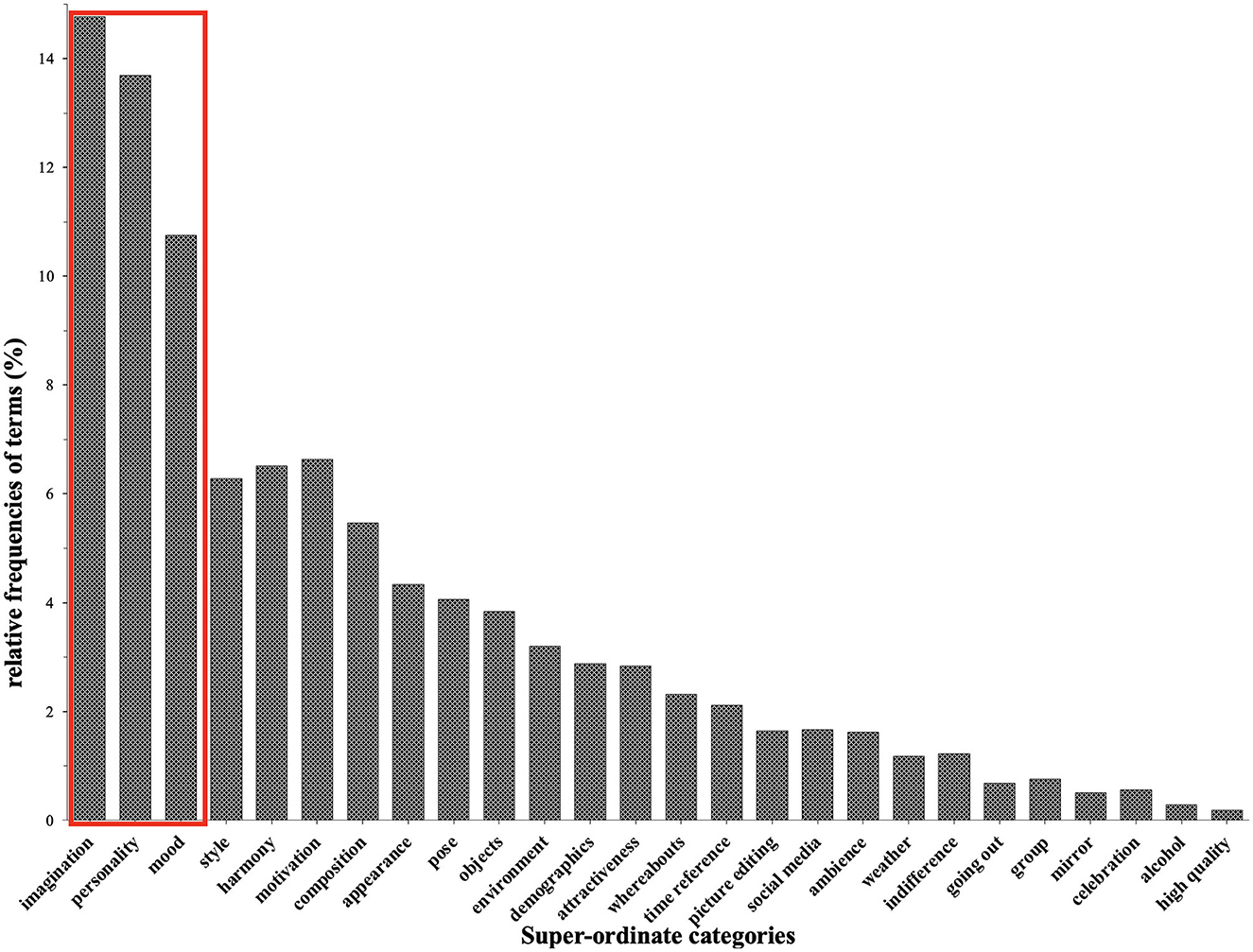
Figure 1. Relative frequencies of terms for each of the 26 final superordinate categories (in percent). The red box indicates the three most important categories (imagination, personality, and mood). We revealed that Imagination was the most used category (14.8%) to describe the presented selfies, followed by Personality (13.7%) and Mood (10.8%).
Exemplarily categories for the first step of data reduction:
I. Clothes: Pooling cloth-related terms, e.g., scarf, shirt, or hat.
II. Composition: Pooling terms that were related to the picture composition, e.g., mirrored, blurry, or bad picture detail.
III. Dividing personality-related terms into two subcategories that were used to describe either positive or negative personality attributions. Examples of positive personality attributions (“personality positive”): likable, confident, or honest. Examples of negative personality attributions (“personality negative”): strict, compulsive, or naive.
IV. Imagination: Pooling terms that had an interpretative character and were related to assumptions of the viewers (participants) about the depicted person or the presented scene in general. We call this category imagination as it refers to associations of the viewer that are not necessarily directly visible in the picture and therefore were indirectly linked to the picture's content. Examples: artistically talented, waiting, on the way to work, or she wears the working pants.
V. Mood: Similar to imagination but with the main difference that the mood of the depicted person or the scene can be derived from the picture, e.g., sad, happy, or bad mood.
Please note that we assumed some of the aforementioned categories were already at the superordinate category level since they showed the highest abstraction level in our data (e.g., imagination, personality, and mood). Consequently, these categories were not further subsumed in step 2.
In the following, we want to exemplarily list categories for the second step of data reduction, which were later the basis of all further statistical analyses (see also Table 2 for details):
1. Pooling imagination-related subcategories: Identity (underlying terms were, e.g., student or blogger), which was defined as an assumption of the viewer regarding the depicted person's identity (please note that we certainly do not know whether the depicted person is actually, for instance, a student; however, we can imagine that she or he is a student). Identity association (underlying terms were, e.g., Heidi Klum, mermaid, or looks like Barbie), which was defined as an imaginative association of an actual or fictional identity or individual. of course, the respective individual was actually not visible on the presented selfie, but the participant felt that there was a link in some way to the respective individual. All other terms were already subsumed to imagination in the first-step data reduction (see examples above).
2. Re-combining “personality positive” and “personality negative” to the superordinate category Personality. This re-combination was necessary since we aimed to have semantic-neutral superordinate categories.
3. There was only one mood-related subcategory: Smiling [underlying terms were, e.g., (no) smiling or evil grin]. All other terms were already subsumed to Mood in the first-step data reduction.
4. Pooling motivation-related subcategories: Motivation (underlying terms were, e.g., wants to look cool, craving for recognition, or wants to look older), which was defined as a potential motivation of the selfie-taker. Fun picture (underlying terms were, e.g., fun picture, fooling around, or funny), which is similar to the aforementioned self-parody selfie described by Eagar and Dann (2016). Here, the selfie-taker wants to show a snapshot of a funny moment in her or his life in a humorous way. Self-expression (underlying terms were, e.g., self-expression, staged, or intentional picture) is similar to the concept mentioned above of self-reference by Carbon (2017).
5. Harmony. Underlying terms were, e.g., natural, beautiful, or authentic, and described positive esthetic aspects of a selfie. in contrast, odd or even disarranging aspects of a given selfie (absence of harmony) were described by terms such as, e.g., artificial, strange, or unflattering.
6. Pooling style-related subcategories: the aforementioned (see first-step reduction) subcategory clothes and the subcategory body modification (underlying terms were, e.g., tattoo, make-up, or mascara) were subsumed. Other style-related terms, such as, e.g., fancy, casual, or modern, were already pooled and subsumed into Style in the first-step reduction.
7. The superordinate category Appearance contained mainly subcategories regarding body parts such as facial features, hair, or body shape in general.
8. The category Pose consisted of two subcategories: (1) Pose in general (underlying terms were, e.g., posing/pose, seductive, or convulsed) and (2) Mimic was described by terms such as duckface, quizzical, or serious expression.
9. Pooling objects-related subcategories: the subcategory accessories was described as objects that can be used as body jewelry (e.g., earrings, necklace, or barrette) or as tools such as smartphones, headphones, or glasses. The second subcategory of Objects was surroundings which was described by terms such as, e.g., emptiness, wall, or ceiling.
3. Results
Based on the aforementioned pre-processing procedure, the resulting level of measurement was categorical. We first focused on the frequency distribution of the resulting categories (see Figure 1). In the second step, we analyzed the distribution of common categories across all selfies. In other words, we investigated how many selfies shared the same categories (see details below). Finally, we aimed to obtain clusters of selfies that share the same categories. To do so, we investigated the relative frequency distributions of used categories per cluster to analyze which categories are most representative for each cluster—to get first insight on which types of and combinations of categories are predominant for the respective clusters to define and label them semantically.
3.1. Qualitative analysis of data
Frequency-based similarity analyses revealed that there are aforementioned terminological commonalities across many selfies that were used to describe them. Accordingly, we conducted a cluster analysis on the (un)similarity matrix based on the common categories across all selfies. Initially, we aimed to obtain clusters of selfies that share the same categories (we call this category semantic profiles). In the second step, we investigated the relative frequency distributions of used categories per cluster to analyze which categories most represent each cluster. Based on this information, clusters were then labeled with superordinate terms reflecting the qualities of the categories they mainly contain. For the presented analyses, we oriented toward a well-established approach (see details, Schneider and Carbon, 2021): Partitioning clustering (k-means) was applied by using R 4.2.1 (R Core Team, 2013) for macOS, utilizing the R package factorextra (ver. 1.0.6) by Lê et al. (2008) and Kassambara and Mundt (2019), and R package NbClust (ver. 3.0) by Charrad et al. (2014) applying the gap statistic criterion (Tibshirani et al., 2001) for determining the appropriate number of clusters. For all analyses, we used the Euclidean distance metric.
As shown in Figure 2, partitioning clustering revealed five distinct semantic profiles (clusters) of selfies that share common categories supporting the assumption of terminological commonalities across selfies. Figures 3–7 show the relative frequency distributions of used categories per cluster. Please note that higher relative frequencies of a given category indicate the relatively higher importance of the respective category within a cluster. Based on this information, we labeled the clusters with superordinate terms.
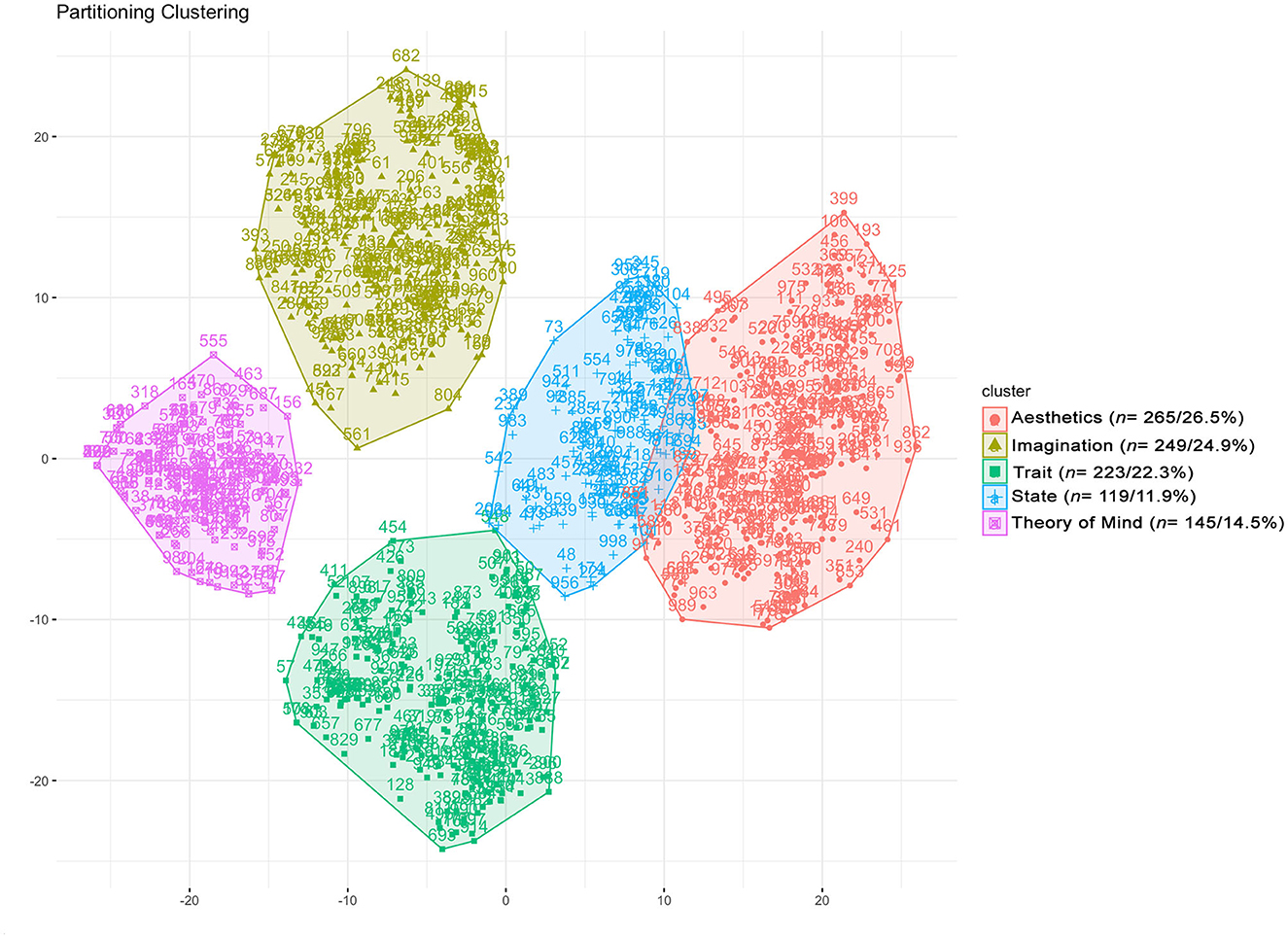
Figure 2. Visualized results of partitioning clustering show a clear 5-cluster structure (n = total number of selfies per cluster + the relative number of selfies per cluster in percent). Please note that each point indicates a single selfie (out of n = 1,001 selfies in total). Further details (cluster memberships for all single selfies and the respective associations) can be found at https://osf.io/39zhe/.
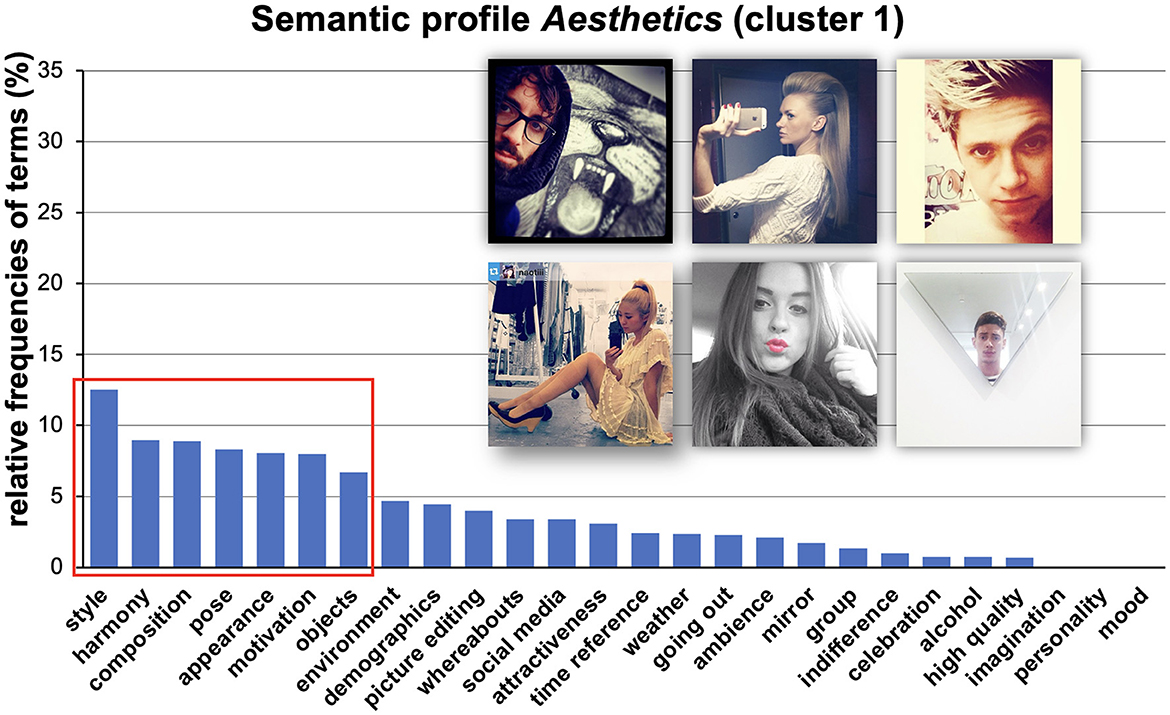
Figure 3. Visualized results of relative frequencies of used categories. We called this semantic profile esthetics because it refers to typical esthetic-related terms (see red box). Please note that we used a minimum-distance classification concerning the cluster centroid to choose a cluster's most typical selfies (see example pictures). The order of presented selfies is not related to their typicality. This procedure was applied to all the following visualizations. Images are re-used images from Selfiecity licensed under CC BY-NC-SA.
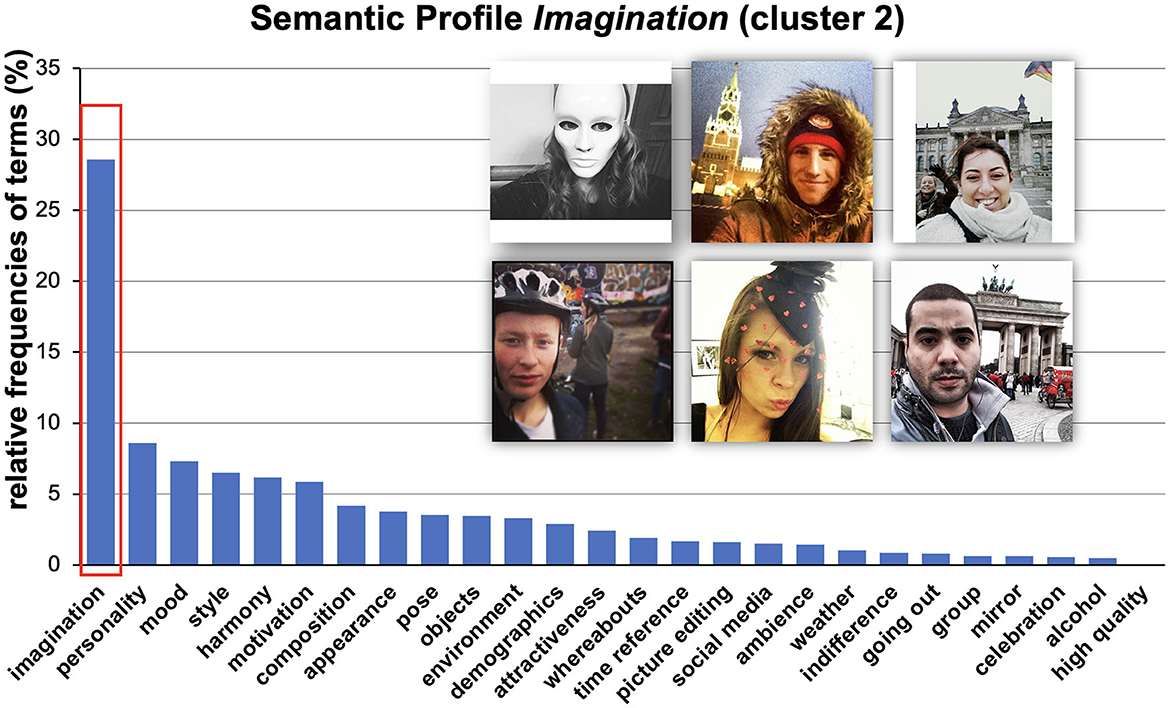
Figure 4. Visualized results of relative frequencies of used categories. We called this profile Imagination because it refers to typical imagination-related terms (see red box). Images are re-used images from Selfiecity licensed under CC BY-NC-SA.
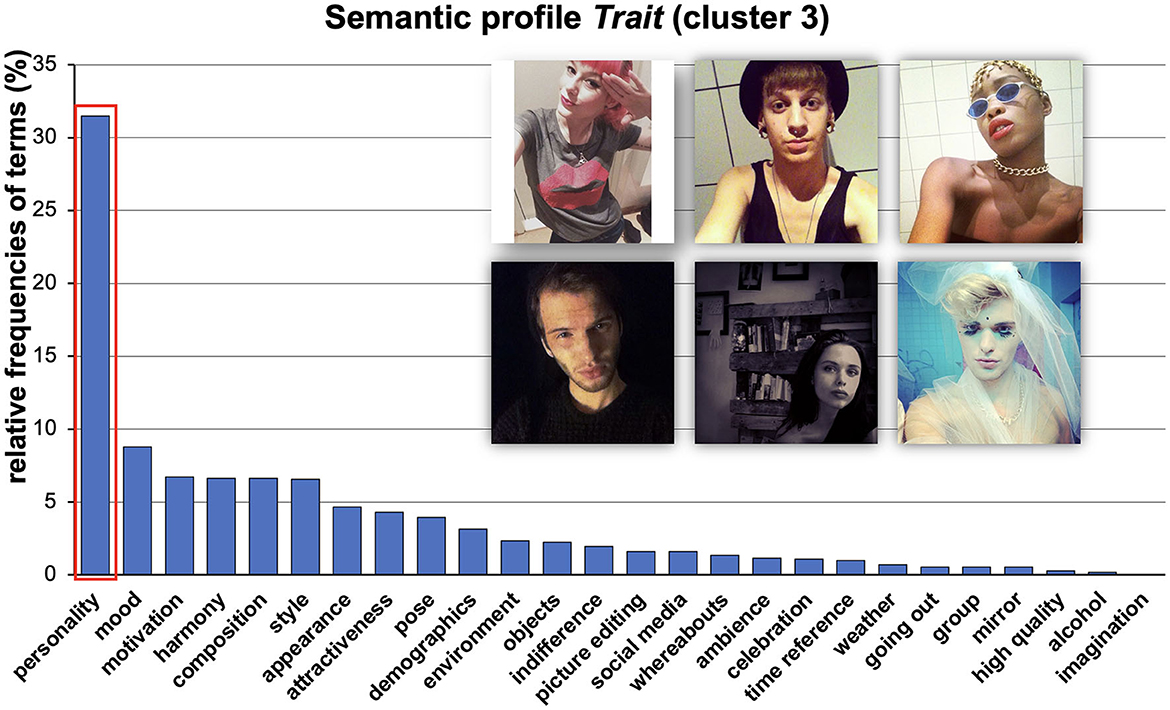
Figure 5. Visualized results of relative frequencies of used categories. We called this semantic profile Trait because it mainly refers to typical personality trait-related terms (see red box) in a long-lasting and consistent sense. Images are re-used images from Selfiecity licensed under CC BY-NC-SA.
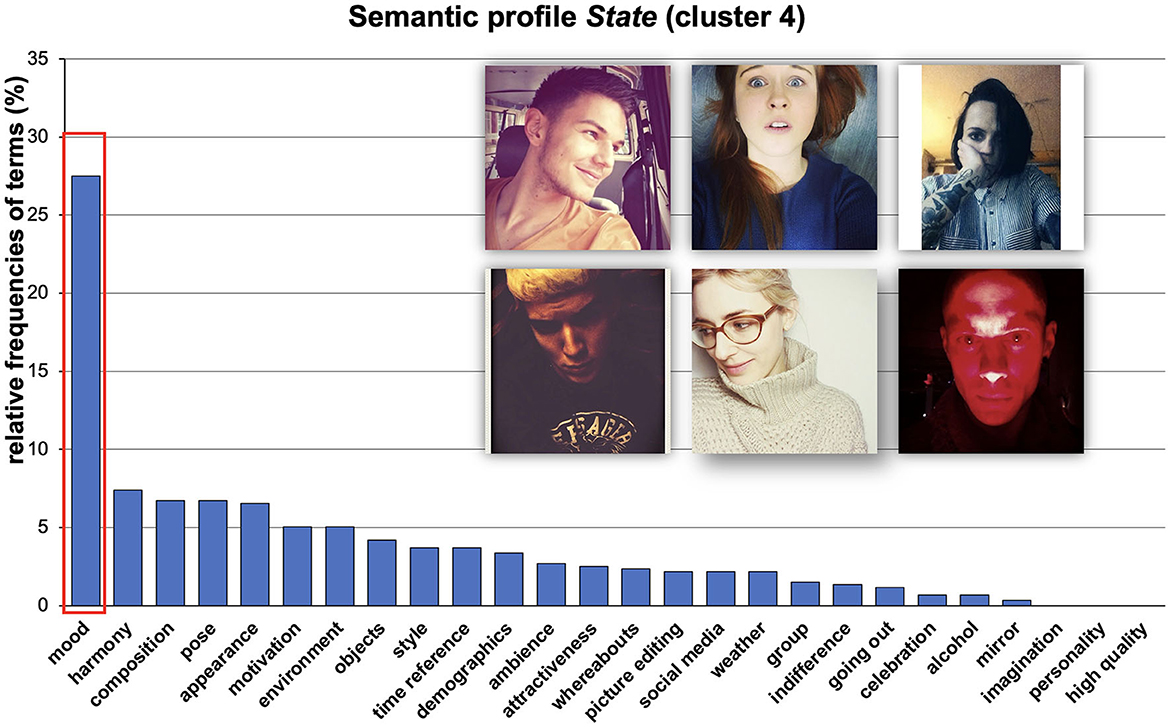
Figure 6. Visualized results of relative frequencies of used categories. We called this semantic profile State because it mainly refers to typical state-related terms (e.g., mood, see red box) in the case of a rather situation-dependent manner (non-long-lasting personality states). Images are re-used images from Selfiecity licensed under CC BY-NC-SA.
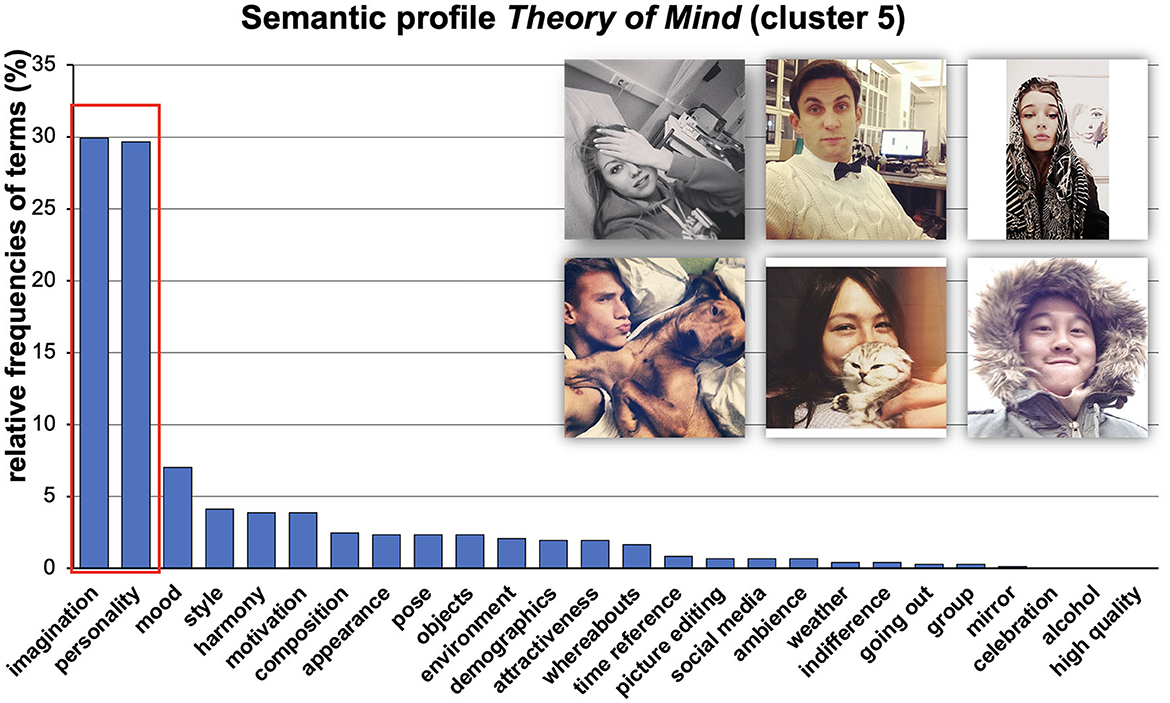
Figure 7. Visualized results of relative frequencies of used categories. We called this semantic profile Theory of Mind because it mainly refers to typical imaginative and personality-related terms. More in detail, participants' associations referred to theories about the personality of depicted persons, their motivations, etc. Images are re-used images from Selfiecity licensed under CC BY-NC-SA.
To illustrate and better understand the results, we first will provide sample associations for the most frequently used categories per cluster. Second, we selected the six most representative selfies (three female and three male) following a minimum-distance classification concerning the cluster centroid to give an impression of which images could usually be found in the different clusters. For this classification routine, we followed the so-called multidimensional face-space model (e.g., Valentine, 1991; Valentine and Endo, 1992; Busey, 1998; Schneider and Carbon, 2021). Here, facial exemplars are encoded as points in a multidimensional space (so-called face-space) and their distances correspond to the perceived (dis-)similarity. In contrast, the centroid of the face-space consists of the sum of all exemplars and is assumed to be the most typical presentation. Less typical exemplars are less densely clustered in the periphery. Accordingly, six selfies with the smallest distance to the cluster centroid were assumed as most typical for the respective cluster.
Semantic profile esthetics (Cluster 1). Analysis revealed that style, harmony, composition, pose, appearance, motivation, and objects, were the most frequently used categories to describe Cluster 1 (see Figure 3). Regarding the category style, typical phrases to describe selfies in this cluster were, e.g., “make-up,” “chic shirt,” or “typical stylish look of young modern women.” For harmony, typical phrases were “natural,” “very nice and natural laughing,” or “artificial” (in the sense of disharmony). Regarding composition, typical phrases were “blurry,” “tilted,” or “interesting interaction of shades and forms.” Pose was characterized by terms such as “duckface,” “listless smile,” or “posing.” Appearance was described by phrases such as “long face” or “colored hair.” The category motivation pointed at the intentions of the selfie-takers and was described by terms such as, “[selfie] taken for friends,” “showing the t-shirt,” or “wants to look beautiful.” The final category objects in cluster 1 referred to objects in the selfies that were not directly linked to the selfie-taker and were characterized by, e.g., “nice decorated tree,” “laminate floor,” or “bed.” Accordingly, we call this cluster esthetics as it refers to a broad range of main characteristics which were linked to esthetic experience in daily life (Faerber et al., 2010). The six most typical selfies (see Figure 3) of the esthetics-cluster (Cluster 1) illustrate major components of esthetic aspects in selfies (in the shown cases, e.g., posing, extraordinary hairstyle, making a duckface or wall paintings in the background, and artistic techniques such as using a mirror to crop picture details).
Semantic profile Imagination (Cluster 2). The second semantic profile was mainly described by the category imagination. In this case, exemplary associations to describe this cluster were, e.g., “traveling,” “tourist,” “fashionista,” or “at work.” These phrases were related to the assumptions of the viewers (participants) about the depicted person or the presented scene in general. Accordingly, we call this cluster imagination as it refers to associations of the viewer that are not necessarily directly visible in the picture and therefore were indirectly linked to the picture's content. For example, we certainly do not know whether the depicted person is traveling; however, we can imagine that the male person (third selfie, lower row, Figure 4) is on vacation in Berlin (Germany) and visited the Brandenburg Gate.
Semantic profile Trait (Cluster 3). For the third semantic profile, we found that participants mainly used personality-related terms to describe their respective selfies (see Figure 5). Exemplary terms were, e.g., “friendly,” “arrogant,” “self-confident”/“shy,” “crazy,” or “aggressive.” Accordingly, we call this cluster Trait in contrast to Cluster 4 (state cluster, see below) and used terms describing rather stable and long-lasting personality-related traits.
Semantic profile State (Cluster 4). As we stated before, this semantic profile was described by terms and phrases referring to the perceived mood of the depicted person or atmosphere of the respective scene. Typical phrases were, e.g., “relaxed,” “depressive,” “scary,” “happiness,” or “bored.” Consequently, we called this cluster state as most terms pointed at situational elements relating to the depicted person's mood or the selfie's general atmosphere.
Semantic profile Theory of Mind (Cluster 5). The fifth semantic profile mainly consisted of two categories: Imagination and Personality. We call this cluster Theory of Mind as it refers to the viewer's theories and assumptions about the personality, motives, or identity of the depicted persons. Notably, such variables are not necessarily directly visible but were derived from the respective selfie. Exemplary phrases were, e.g., “Frenchwoman,” “Muslim,” or “animal-loving.”
4. Discussion
The main goal of the present study was to apply a new approach to investigate how viewers describe the impression a selfie has on them (this routine we call Semantics of Selfies). Other approaches exist to gain information about the quality of selfies, among other things, analyzing metatags, hashtags, or the linked message of the respective post (e.g., Eagar and Dann, 2016). Our approach, in contrast, analyzes how viewers describe the impression a selfie has on them and thus is a genuine psycho-perceptual approach. By using this approach, we revealed five distinct categories which we call semantic profiles. These categories were described as Esthetics, Imagination, Trait, State, and Theory of Mind.
Esthetics can be denoted as a rather complex construct referring to a broad range of main characteristics linked to daily esthetic experience. The main components were style, harmony, composition, pose, appearance, motivation, and objects. Selfies of the Esthetics cluster mainly refer to aspects of how the picture is taken, e.g., stylistic, compositional, or even artistic techniques (e.g., photo filters or perspective), which were typically used by selfie-takers (e.g., Schneider et al., 2012; Schneider and Carbon, 2017). However, selfies from this cluster also refer to how a selfie-taker presents her- or himself (e.g., a facial expression like making a “duckface”) and what might be the motive to take the picture. These motives and aims are in accordance with results described by Carbon (2017), e.g., posing to optimize bodily appearance (e.g., showing the left/right side of the face or shooting from above). Accordingly, besides the fact that most of the selfies were assigned to this cluster (26.5% of all selfies), the aforementioned findings underline the importance of such esthetic aspects in selfies.
Regarding Imagination, selfies were related to the general assumptions of the viewer about the depicted person or the presented scene. This semantic profile refers to associations of the viewer that are not necessarily directly visible in the picture and therefore are indirectly linked to the content of the picture. Regarding State, the results indicate that this construct is related to aspects referring to the perceived mood of the depicted person or the situational aspects or atmosphere of the respective scene.
The semantic profile Theory of Mind mainly consists of Imagination and Personality-related associations and refers to the viewer's theories and assumptions about the personality, motives, or identity of the depicted persons (e.g., “Frenchwoman,” “Muslim,” “animal-loving,” or “she is concerned about her appearance”). Such variables are not necessarily directly visible but were derived from the respective selfies. Based on the results of the present study, we could show that such non-directly accessible visual cues make us speculate about what kind of a person the depicted person is (e.g., being an animal-loving person or being a very self-appearance-focusing person).
What can we learn from the present study? There are mainly three important outcomes: First, we are able to analyze how viewers describe the impression a selfie has on them (this is what we call the Semantics of Selfies). Second, we could show that five main categories (Esthetics, Imagination, Trait, State, and Theory of Mind) constitute five distinct characteristic clusters (what we call a semantic profile). Third, previous research (e.g., Eagar and Dann, 2016; Carbon, 2017) postulated genres or types of selfies solely based on directly accessible information such as hashtags or picture elements. However, the assigned message to a selfie, for instance, the interpreted motive of the selfie-taker (e.g., attempting to be perceived as authentic, trendy, attractive, or even fearless) remained unclear. Particularly, the semantic profiles Imagination, Trait, State, and Theory of Mind highlight information that is not necessarily directly visible in the picture but is used by the viewer to make assumptions about the depicted person. Future research should consider the power of SoS in selfies as we postulate that people may use such semantic information to influence how they are perceived. This assumption is based on findings that people actually utilize, e.g., photographic techniques to optimize their appearance in many ways, for example, physical appearance (see, e.g., Schneider et al., 2012; Schneider and Carbon, 2017) or even emotional aspects (see, e.g., Lindell, 2013, 2017). Further research is needed to investigate whether and how SoS can be used to influence how a selfie-taker is perceived. Free associations might be the main technique to be applied, but cued-recognition and priming paradigms assessing the availability of concepts or microgenetic approaches unfolding the process of perceiving and categorizing selfies seem to be promising for such studies.
Finally, we would also like to mention some limitations of this study: The used dataset of selfies contains non-standardized depictions of random persons across different regions and cultures; we also had no access to the actual sex of a depicted person. Anyhow, we explicitly used such depictions as past research demonstrated the strength of using authentic and highly idiosyncratic material in face-related research (e.g., Schneider and Carbon, 2021). One may argue that such factors (own and foreign cultures) might strongly impact how we process a given selfie. There is scientific evidence that suggests that people of different cultures use their own familiar reference systems to make inferences about other people (see, e.g., Schneider et al., 2013). Applying this to the construct of SoS, we certainly expect an idiosyncratic pattern. However, there also might be some variables that could be stable across cultures (for example, State or Trait-related SoS). Accordingly, further research should focus on potential cross-cultural differences in SoS. We also like to mention that the “Selfiecity” database (Tifentale and Manovich, 2015) is composed solely of material from Instagram. As we learned from studies by, e.g., Deeb-Swihart et al. (2017) and Williamson et al. (2017), people use diverse social media platforms to communicate with their friends or even a public audience (e.g., Snapchat, Instagram, LinkedIn, Facebook, and Twitter). The authors of both studies revealed that people tend to use different platforms for communication in certain aspects of their lives. One may suggest that when investigating associations across diverse platforms, the pattern of semantic profiles may differ. However, there is currently no or even sparse knowledge of cross-platform usage regarding semantic profiles. Further research is needed to close this gap.
Concerning the recruited sample (participants), we did not collect any sociodemographic variables since we did not have a certain hypothesis on such aspects. For better representability, we did take care that at least half of the sample was recruited outside the University of Bamberg (n = 52 students out of 132 participants). However, in fact, regarding investigation in the field of art perception, there is scientific evidence that people differ in some ways they respond according to their esthetic appreciation (e.g., Leder et al., 2006). The authors could show that participants with more art knowledge (experts) have a better understanding of the paintings than participants with less art knowledge (novices). Similarly, regarding the perception of music, there is evidence that people differ in taste depending on, e.g., higher cognitive factors or personality-related variables (e.g., Chamorro-Premuzic et al., 2010; Nusbaum and Silvia, 2011; Andersen et al., 2012). People also differ in preference and response to music depending on their gender (e.g., Chamorro-Premuzic et al., 2009), age (e.g., Bonneville-Roussy et al., 2013), and mood (e.g., Vuoskoski and Eerola, 2011). Accordingly, from the present point, we cannot exclude the possibility that people may also have different associations when viewing a selfie depending on their personality, sex, age, or even how they currently feel. Further research should address this assumption. However, with that being said, the present study's focus was on how selfies are perceived and whether they can be used to express ourselves and send a message (SoS) to the viewers. Most importantly, even though we did not know who was responding, we revealed a clear pattern of different semantic profiles across many selfies.
Another limitation of the present study is that, in contrast to classical lab studies, we could not control experimental variables such as the actual size of the presented selfie on the monitor or the actual viewing distance. We also expected that contrast, brightness, and display color were not at the same level across all participants. However, classical lab conditions certainly do not reflect the typical daily life scenarios in which we see selfies on social media across many different devices (smartphones, tablets, computer screens, television, etc.) and in multiple situations (chatting in the subway, sitting comfortably on the sofa, and lying sleepily in the bed).
We hope that the Semantic of Selfies (SoS) will contribute to the understanding of how certain selfies affect viewers to perceive specific qualities in the self-portrayed person. We would be very happy to see our preliminary approach inspire more work in this important field of perceptual and media psychology—and society!
Data availability statement
The raw data supporting the conclusions of this article will be made available by the authors, without undue reservation. OSF Link is now public: https://osf.io/39zhe/.
Ethics statement
The studies involving humans were approved by the University Ethics Committee, University of Bamberg. The studies were conducted in accordance with the local legislation and institutional requirements. The participants provided their written informed consent to participate in this study. Written informed consent was obtained from the individual(s) for the publication of any potentially identifiable images or data included in this article.
Author contributions
TS was responsible for collecting, analyzing, and interpreting data as well as for writing this manuscript. C-CC was responsible for supervision (initial idea and experimental design improvements), interpretation of results, and critical manuscript reviewing. All authors agree to be accountable for the content of the article. All authors contributed to the article and approved the submitted version.
Funding
Open Access Availability was supported by University of Bamberg (funded by the German Research Foundation, DFG).
Acknowledgments
The authors would like to thank Caroline Tratz for collecting data and assisting in preliminary data pre-processing.
Conflict of interest
The authors declare that the research was conducted in the absence of any commercial or financial relationships that could be construed as a potential conflict of interest.
Publisher's note
All claims expressed in this article are solely those of the authors and do not necessarily represent those of their affiliated organizations, or those of the publisher, the editors and the reviewers. Any product that may be evaluated in this article, or claim that may be made by its manufacturer, is not guaranteed or endorsed by the publisher.
References
Andersen, N. E., Dahmani, L., Konishi, K., and Bohbot, V. D. (2012). Eye tracking, strategies, and sex differences in virtual navigation. Neurobiol. Learn. Mem. 97, 81–89. doi: 10.1016/j.nlm.2011.09.007
Archer, D., Iritani, B., Kimes, D. D., and Barrios, M. (1983). Face-Ism - 5 studies of sex-differences in facial prominence. J. Pers. Soc. Psychol. 45, 725–735. doi: 10.1037/0022-3514.45.4.725
Arsel, Z., and Bean, J. (2013). Taste regimes and market-mediated practice. J. Consum. Res. 39, 899–917. doi: 10.1086/666595
Augustin, M. D., Wagemans, J., and Carbon, C. C. (2012). All is beautiful? Generality vs. specificity of word usage in visual aesthetics. Acta Psychol. 139, 187–201. doi: 10.1016/j.actpsy.2011.10.004
Belk, R. W. (2013). Extended self in a digital world. J. Consum. Res. 40, 477–500. doi: 10.1086/671052
Bonneville-Roussy, A., Rentfrow, P. J., Xu, M. K., and Potter, J. (2013). Music through the ages: trends in musical engagement and preferences from adolescence through middle adulthood. J. Pers. Soc. Psychol. 105, 703–717. doi: 10.1037/a0033770
Bruno, N., and Bertamini, M. (2013). Self-portraits: smartphones reveal a side bias in non-artists. PLoS One 8, 141. doi: 10.1371/journal.pone.0055141
Bruno, N., Gabriele, V., Tasso, T., and Bertamini, M. (2014). ‘Selfies’ reveal systematic deviations from known principles of photographic composition. Art Percept. 2, 45–58. doi: 10.1163/22134913-00002027
Bruno, N., Pisanski, K., Sorokowska, A., and Sorokowski, P. (2018). Editorial: understanding selfies. Front. Psychol. 9, 44. doi: 10.3389/fpsyg.2018.00044
Busey, T. A. (1998). Physical and psychological representations of faces: evidence from morphing. Psychol. Sci. 9, 476–483. doi: 10.1111/1467-9280.00088
Carbon, C. C. (2017). Universal principles of depicting oneself across the centuries: From Renaissance self-portraits to selfie-photographs. Front. Psychol. 8, 245. doi: 10.3389/fpsyg.2017.00245
Chamorro-Premuzic, T., Fagan, P., and Furnham, A. (2010). Personality and uses of music as predictors of preferences for music consensually classified as happy, sad, complex, and social. Psychol. Aesthet. Creat. Arts 4, 205–213. doi: 10.1037/a0019210
Chamorro-Premuzic, T., Goma-i-Freixanet, M., Furnham, A., and Muro, A. (2009). Personality, self-estimated intelligence, and uses of music: a spanish replication and extension using structural equation modeling. Psychol. Aesth. Creat. Arts 3, 149–155. doi: 10.1037/a0015342
Charrad, M., Ghazzali, N., Boiteau, V., and Niknafs, A. (2014). Nbclust: an R package for determining the relevant number of clusters in a data set. J. Stat. Soft. 61, 1–36. doi: 10.18637/jss.v061.i06
Deeb-Swihart, J., Polack, C., Gilbert, E., and Essa, I. (2017). Selfie-presentation in everyday life: a large-scale characterization of selfie contexts on Instagram. Proc. Int. AAAI Conf. Web Soc. Media 11, 42–51. doi: 10.1609/icwsm.v11i1.14896
Diefenbach, S., and Christoforakos, L. (2017). The selfie Paradox: nobody seems to like them yet everyone has reasons to take them. An exploration of psychological functions of selfies in self-presentation. Front. Psychol. 8, 7. doi: 10.3389/fpsyg.2017.00007
Eagar, T., and Dann, S. (2016). Classifying the narrated #selfie: genre typing human-branding activity. Eur. J. Market. 50, 1835–1857. doi: 10.1108/EJM-07-2015-0509
Faerber, S. J., Leder, H., Gerger, G., and Carbon, C. C. (2010). Priming semantic concepts affects the dynamics of aesthetic appreciation. Acta Psychol. 135, 191–200. doi: 10.1016/j.actpsy.2010.06.006
Google (2019). Google Photos: One Year, 200 Million Users, and a Whole Lot of Selfies. Available online at: https://blog.google/products/photos/google-photos-one-year-200-million/ (accessed May 7, 2019).
Hanna, E., Ward, L. M., Seabrook, R. C., Jerald, M., Reed, L., Giaccardi, S., et al. (2017). Contributions of social comparison and self-objectification in mediating associations between facebook use and emergent adults' psychological well-being. Cyberpsychol. Behav. Soc. Network. 20, 172–179. doi: 10.1089/cyber.2016.0247
Jarreau, P. B., Cancellare, I. A., Carmichael, B. J., Porter, L., Toker, D., Yammine, S. Z., et al. (2019). Using selfies to challenge public stereotypes of scientists. PloS one 14, 625. doi: 10.1371/journal.pone.0216625
Kassambara, A., and Mundt, F. (2020). Factoextra: Extract and Visualize the Results of Multivariate Data Analyses. R Package Version 1.0.7. Available online at: https://CRAN.R-project.org/package=factoextra
Lê, S., Josse, J., and Husson, F. (2008). FactoMineR: an R package for multivariate analysis. J. Stat. Soft. 25, 1–18. doi: 10.18637/jss.v025.i01
Leder, H., Carbon, C. C., and Ripsas, A. L. (2006). Entitling art: influence of title information on understanding and appreciation of paintings. Acta Psychol. 121, 176–198. doi: 10.1016/j.actpsy.2005.08.005
Lindell, A. K. (2013). The silent social/emotional signals in left and right cheek poses: a literature review. Laterality 18, 612–624. doi: 10.1080/1357650X.2012.737330
Lindell, A. K. (2017). Consistently showing your best side? Intra-individual consistency in #selfie pose orientation. Front. Psychol. 8, 246. doi: 10.3389/fpsyg.2017.00246
Marshall, P. D. (2014). Celebrity and Power: Fame in Contemporary Culture. Minnesota, MN: University of Minnesota Press.
Mayring, P. (2000). Qualitative content analysis. Forum Q. Soc. Res. 1, 20. doi: 10.17169/fqs-1.2.1089
McQuarrie, E. F., Miller, J., and Phillips, B. J. (2013). The megaphone effect: taste and audience in fashion blogging. J. Consum. Res. 40, 136–158. doi: 10.1086/669042
Musil, B., Preglej, A., Ropert, T., Klasinc, L., and Babic, N. C. (2017). What is seen is who you are: are cues in selfie pictures related to personality characteristics? Front. Psychol. 8. doi: 10.3389/fpsyg.2017.00082
Nusbaum, E. C., and Silvia, P. J. (2011). Shivers and Timbres: personality and the experience of chills from music. Soc. Psychol. Pers. Sci. 2, 199–204. doi: 10.1177/1948550610386810
Parmentier, M. A., Fischer, E., and Reuber, A. R. (2013). Positioning person brands in established organizational fields. J. Acad. Market. Sci. 41, 373–387. doi: 10.1007/s11747-012-0309-2
Qiu, L., Lu, J. H., Yang, S. S., Qu, W. N., and Zhu, T. S. (2015). What does your selfie say about you? Comput. Hum. Behav. 52, 443–449. doi: 10.1016/j.chb.2015.06.032
R Core Team (2013). R: A Language and Environment for Statistical Computing. Available online at: http://r.meteo.uni.wroc.pl/web/packages/dplR/vignettes/intro-dplR.pdf (accessed January 29, 2021).
Saunders, J. F., and Eaton, A. A. (2018). Snaps, selfies, and shares: how three popular social media platforms contribute to the sociocultural model of disordered eating among young women. Cyberpsychol. Behav. Soc. Netw. 21, 343–354. doi: 10.1089/cyber.2017.0713
Schneider, T. M., and Carbon, C. C. (2017). Taking the perfect selfie: investigating the impact of perspective on the perception of higher cognitive variables. Front. Psychol. 8, 1–16. doi: 10.3389/fpsyg.2017.00971
Schneider, T. M., and Carbon, C. C. (2021). The episodic prototypes model (EPM): On the nature and genesis of facial representations. I- Perception 12, 1–46. doi: 10.1177/20416695211054105
Schneider, T. M., Hecht, H., and Carbon, C. C. (2012). Judging body weight from faces: the height-weight illusion. Perception 41, 121–124. doi: 10.1068/p7140
Schneider, T. M., Hecht, H., Stevanov, J., and Carbon, C. C. (2013). Cross-ethnic assessment of body weight and height on the basis of faces. Pers. Ind. Diff. 55, 356–360. doi: 10.1016/j.paid.2013.03.022
Sorokowska, A., Oleszkiewicz, A., Frackowiak, T., Pisanski, K., Chmiel, A., Sorokowski, P., et al. (2016). Selfies and personality: who posts self-portrait photographs? Pers. Ind. Diff. 90, 119–123. doi: 10.1016/j.paid.2015.10.037
Tibshirani, R., Walther, G., and Hastie, T. (2001). Estimating the number of clusters in a data set via the gap statistic. J. Royal Stat. Soc. Series Methodol. 63, 411–423. doi: 10.1111/1467-9868.00293
Tifentale, A., and Manovich, L. (2015). “Selfiecity: Exploring photography and self-fashioning in social media,” in Postdigital Aesthetics: Art, Computation and Design, eds D. M. Berry and M. Dieter (London: Palgrave Macmillan), 109–122.
Valentine, T. (1991). A unified account of the effects of distinctiveness, inversion, and race in face recognition. The Q. J. Exp. Psychol. Hum. Exp. Psychol. 43, 161–204. doi: 10.1080/14640749108400966
Valentine, T., and Endo, M. (1992). Towards an exemplar model of face processing: the effects of race and distinctiveness. The Q. J. Exp. Psychol. Hum. Exp. Psychol. 44, 671–703. doi: 10.1080/14640749208401305
Vuoskoski, J. K., and Eerola, T. (2011). Measuring music-induced emotion: a comparison of emotion models, personality biases, and intensity of experiences. Music. Sci. 15, 159–173. doi: 10.1177/1029864911403367
Keywords: selfie, semantics, face-specific processing, selfie classification, verbal description
Citation: Schneider TM and Carbon C-C (2023) On the Semantics of Selfies (SoS). Front. Commun. 8:1233100. doi: 10.3389/fcomm.2023.1233100
Received: 01 June 2023; Accepted: 12 September 2023;
Published: 30 October 2023.
Edited by:
Wibke Weber, Zurich University of Applied Sciences, SwitzerlandReviewed by:
Fabienne Bünzli, The Pennsylvania State University (PSU), United StatesYvonne Eriksson, Mälardalen University, Sweden
Copyright © 2023 Schneider and Carbon. This is an open-access article distributed under the terms of the Creative Commons Attribution License (CC BY). The use, distribution or reproduction in other forums is permitted, provided the original author(s) and the copyright owner(s) are credited and that the original publication in this journal is cited, in accordance with accepted academic practice. No use, distribution or reproduction is permitted which does not comply with these terms.
*Correspondence: Tobias Matthias Schneider, VG9iaWFzQGh1bWFuLXBlcmNlcHRpb24uY29t
†ORCID: Tobias Matthias Schneider orcid.org/0000-0003-4014-1775
Claus-Christian Carbon orcid.org/0000-0002-3446-9347