Revered and reviled: a sentiment analysis of female and male referents in three languages
- 1Neurobiology of Language Department, Max Planck Institute for Psycholinguistics, Nijmegen, Netherlands
- 2Centre for Language Studies, Radboud University, Nijmegen, Netherlands
- 3Department of Linguistics, Stockholm University, Stockholm, Sweden
Our study contributes to the less explored domain of lexical typology, focusing on semantic prosody and connotation. Semantic derogation, or pejoration of nouns referring to women, whereby such words acquire connotations and further denotations of social pejoration, immorality and/or loose sexuality, has been a very prominent question in studies on gender and language (change). It has been argued that pejoration emerges due to the general derogatory attitudes toward female referents. However, the evidence for systematic differences in connotations of female- vs. male-related words is fragmentary and often fairly impressionistic; moreover, many researchers argue that expressed sentiments toward women (as well as men) often are ambivalent. One should also expect gender differences in connotations to have decreased in the recent years, thanks to the advances of feminism and social progress. We test these ideas in a study of positive and negative connotations of feminine and masculine term pairs such as woman - man, girl - boy, wife - husband, etc. Sentences containing these words were sampled from diachronic corpora of English, Chinese and Russian, and sentiment scores for every word were obtained using two systems for Aspect-Based Sentiment Analysis: PyABSA, and OpenAI’s large language model GPT-3.5. The Generalized Linear Mixed Models of our data provide no indications of significantly more negative sentiment toward female referents in comparison with their male counterparts. However, some of the models suggest that female referents are more infrequently associated with neutral sentiment than male ones. Neither do our data support the hypothesis of the diachronic convergence between the genders. In sum, results suggest that pejoration is unlikely to be explained simply by negative attitudes to female referents in general.
1 Introduction
“Semantic derogation”, or pejoration of nouns applying to women, whereby such words acquire connotations and further denotations of social pejoration, immorality and/or loose sexuality, has been “[p]erhaps the most prominent diachronic question” (Salmons, 1990) in studies on gender and language (change). This process has been argued to be seldom observable in the corresponding male-related words, with examples such as lord vs. lady, bachelor vs. spinster, mister vs. mistress, etc. (Schulz, 1975; Bebout, 1984; Kleparski, 1997; Kim, 2008). In her seminal article Schulz (1975) considers numerous English terms referring to women that have undergone various kinds of semantic derogation, or pejoration, and points out that pejoration is especially prominent in certain semantic groups (e.g., female kinship terms, terms used as endearments, words for girls and young women). This is all the more striking given that their male equivalents have on the whole escaped pejoration. Notably, as pointed out in Borkowska and Kleparski (2007), even though examples of male words hit by pejoration are attested, as, e.g., Old English cnafa ‘boy’ developing into the by now archaic knave ‘a dishonest or unscrupulous man’, words for males demonstrate many more examples of the opposite, ameliorative developments, such as page, originally meaning ‘boy, lad’, at some point acquiring the meaning of ‘a youth employed as the personal attendant of a person of rank’. For female words, ameliorative developments are reported much less often. This recurrent semantic derogation has been seen as a powerful factor behind repeated lexical replacement of words referring to (young) women (Grzega, 2004, pp. 32–33), e.g., also Fr. garçon ‘boy’ vs. garce ‘bitch’ (earlier ‘girl’), fille ‘girl’.
But why should it be so? Scholars working on semantic change basically agree that the roots of pejoration are to be sought in attitudes toward the referent (Stern, 1931; Ullmann, 1957; Borkowska and Kleparski, 2007). To quote Schulz (1975), p. 64, “a language reflects the thought, attitudes, and culture of the people who make it and use it. A rich vocabulary on a given subject reveals an area of concern of the society whose language is being studied. The choice between positive and negative terms for any given concept (as, for example, in the choice between freedom fighter and terrorist) reveals the presence or absence of prejudicial feelings toward the subject”. Schulz (1975), p. 71 analyses her findings in light of the three different origins for pejoration as suggested by Ullmann (1957), pp. 231–32 – association with a contaminating concept, euphemism, and prejudice – and finds evidence for all the three. According to her, men tend to think of women in sexual terms, and, by association, this results in the male speakers attributing sexual suggestiveness to any female term. Euphemism underlies many terms for prostitutes. However, the major factor behind semantic derogation of words for women is, in Schulz’s view, prejudice, which has two main ingredients – denigration and gross generalization. Schulz (1975), p. 73 concludes, that the semantic change “by which terms designating women routinely undergo pejoration, both reflects and perpetuates derogatory attitudes toward women. They should be abjured”.
Schulz’s conclusion about the prevalence of derogatory attitudes toward females in the society, to a large extent following the male norms, does not sound unfounded, given the accumulated and constantly growing knowledge of women’s discrimination all over the world. However, as pointed out by Glick and Fiske (1996), p. 491, Allport’s (1954), p. 9 classical definition of prejudice as “an antipathy based upon a faulty and inflexible generalization” and primarily used for ethnic prejudice, is difficult to apply to the relations between women and men. First of all, no other two groups have been so interrelated as males and females. Moreover, while prejudice as antipathy is commonly indexed by such measures as negative stereotypes, “cultural images of women from ancient to modern times are not uniformly negative; women have been revered as well as reviled” (Glick and Fiske, 1996, p. 491; see Potts and Weare, 2018 for a telling modern example of the ambivalence in the representation of women who kill as degraded victims or dehumanized monsters in English Crown Court sentencing remarks). The authors argue that sexism has always been marked by a deep ambivalence, in which the subjectively positive feelings toward women are closely associated with antipathy. They suggest further to distinguish between hostile sexism and benevolent sexism, the latter encompassing a set of attitudes based on viewing women stereotypically and in restricted roles, in which they trigger subjectively positive feelings and elicit pro-social behavior.
There has been massive research on prejudice (e.g., Dovidio et al., 2005; Jackson, 2011), including gender prejudice, and gender stereotypes in social psychology. In a nutshell, prejudices constitute the affective component of intergroup bias, whereas stereotypes account for its cognitive component and denote general beliefs about the characteristics of particular groups, e.g., different genders or sexes. Stereotypical beliefs may thus concern the general appropriateness of various roles and activities for men and women (gender/sex roles), or psychological or behavioral characteristics that are believed to characterize one of the genders/sexes with much greater frequency than the other(s) (gender/sex traits) (Williams and Best, 1990, pp. 16–17). Given that it is beyond the scope of this paper to give justice to the accumulated knowledge in the field, we have chosen here to mention the research that we find particularly relevant to our study.
Williams and Best (1990) utilizes the 300-item Adjective Check List, which is normally employed in self-descriptive personality assessment procedures (Gough and Heilbrun (1965) for measuring sex-trait stereotypes by applying a relative judgment method. Males and females from 30 countries were asked to consider each of the 300 items on the list (translated into the relevant language) and assess whether it is equally applicable to both women and men or more frequently associated with either women or men. The resulting scores were used for defining the so-called “focused stereotypes” using a standard degree of association criterion: “items were included in the stereotype set for a particular sex if they were associated with that sex at least twice as often as with the other sex” (Williams and Best, 1990, p. 59). The leading male-associated items across the countries included “adventurous”, “dominant”, “forceful”, “independent”, “masculine” and “strong” (and, slightly less frequent, “aggressive”, “autocratic”, “daring”, “enterprising”, “robust” and “stern”), while the leading recurrent female-associated items included “sentimental”, “submissive” and “superstitious”(and, slightly less frequent, “affectionate”, “dreamy”, “feminine” and “sensitive”) (Williams and Best, 1990, pp. 75–76).
The resulting stereotypes have been analyzed from different perspectives, of which the most relevant here concerns the affective or connotative meanings associated with them, much in the tradition of Osgood et al.’s (1975) Affective Meaning Theory. Each of the adjectives on the list was scaled along the dimensions of favorability (good vs. bad), strength (strong vs. weak) and activity (active vs. passive), resulting in the mean affective meaning score for each of the focused female and male stereotypes. Interestingly, while the male stereotypes in all countries were stronger and more active, there was no consistency across countries in the evaluation of favorability: in some countries (primarily in Peru, Italy and France), the female stereotype was evaluated more favorably than the male one, whereas others (primarily Nigeria, Japan and South Africa) showed the opposite trend (Williams and Best, 1990, pp. 97–99).
Williams and Best’s (1990) finding that the stereotyping evaluation of females vs. males is not easily captured by the good – bad dimension is much in line with both the Ambivalent Sexism idea in Glick and Fiske (1996) and with the more general and highly influential Stereotype Content Model, related to it (Fiske et al., 2002; Fiske, 2018). The latter claims that stereotypes are captured by two dimensions, according to which people tend to categorize others (and themselves) on the basis of interpersonal and intergroup interactions – warmth (trustworthiness, sociability) and competence (capability, agentivity). Moreover, stereotypes can be subjectively positive on one dimension, but negative (unflattering) on the other.
The availability of big digital corpora and development of corpus linguistic methods for extracting information from them has enabled large-scale research on collective representations of men and women, where the term “collective representation”, introduced by Durkheim (1989/1953) and further developed, among others, by Moscovici (1988), “refers[s] to societal-level systems of meaning that pervade everyday social life” (Charlesworth et al., 2021, p. 218). As repeatedly argued (or at least assumed), “[t]he spoken and written language of a society affords a unique way to measure the magnitude and prevalence of these widely shared collective representations” (Charlesworth et al., 2021, p. 218), also because it may provide access to implicit, hidden attitudes, much less visible in studies based on participants’ reports, where the participants tend to reply in a socially desirable manner and engage in self-deception (Nosek et al., 2007; DeFranza et al., 2020, p. 9; Charlesworth et al., 2021). By studying language in use, researchers ask the questions of how often and in which ways females and males are spoken / written about in different contexts, primarily in texts of different genres and produced during different time periods. A particularly useful method for approaching these issues builds on a comparison of collocations, i.e., words and expressions that frequently occur in close proximity, for pairs of gendered nouns. There is a bulk of studies along these lines, predominantly on English (but see Zasina, 2019 on Czech), comparing the number of occurrences and collocations for such pairs as “woman” vs. man” (Pearce, 2008; Caldas-Coulthard and Moon, 2010), “boy” and “girl” (Macalister, 2011, Taylor, 2013, Norberg, 2016), “bachelor” vs. spinster” (Romaine, 2000, pp. 108–109), the two pairs “woman” vs. man” and “girl” vs. boy” together (Romaine, 2000, p. 110; Caldas-Coulthard and Moon, 2010), or, more generally, for expressions referring to females vs. males (Herdağdelen and Baroni, 2011; cf. Baker, 2014: Chapter 6 for a useful overview).
All these studies unveil significant gender biases in the representation of females and males, with interesting differences among the genres and time periods. To give an example, Pearce (2008) analyses collocations of “man” and “woman” with modifying adjectives and verbs that have them as their subject or object in British National Corpus (BNC) across five different domains, commonly reflecting persistent gender differences in the representation of males and females – power and deviance, social categories, personality and mental capacity (the “Big Fives” of human personality), appearance, and sexuality. For instance, women are more often characterized by adjectives signaling marital/reproductive status (childless, married, separated) and sexual orientation (heterosexual) and are saliently or exclusively modified by adjectives of nationality (American, Bangladeshi), ethnicity (African-American, Asian, gipsy), and class (high-caste, lower-class) (Pearce, 2008, p. 12). Men are strongly associated with attributive adjectives referring to physical strength, prowess, physical size, weight and bulk, while the corresponding adjectives for women show a more limited range of bodily types and shapes, with some referring to weight and size (pear-shaped, slender) and others to breasts (big-bosomed, large-breasted). Men’s facial appearance and expression is likewise more variously described than women’s (Pearce, 2008, p. 17). Some of the differences within the domain of personality and mental capacities include the stronger association of such words as brilliant, clever, gifted and wise with men, while adjectives with negative associations in the domain of sexuality are more strongly associated with women (fallen, promiscuous, frigid, butch). Pearce concludes that the collocates of “man” and “woman” in the BNC seem often to represent gender in stereotypical ways, but also points out a number of important caveats stemming from the composition of the corpus and the limitations of the analytic tools.
The recent years have seen studies using more advanced Natural Language Processing techniques, such as word embedding (Garg et al., 2018; DeFranza et al., 2020; Charlesworth et al., 2021) – a machine-learning technique that captures the meaning of words by the context in which they occur. These studies use impressively big corpora representing different kinds of media, covering relatively long time periods and therefore allowing researchers to study trends in gender bias in society.
Noteworthy, while the unequal representation of the gender per se is either explicitly acknowledged or at least assumed to be reprehensible in all research on gender in corpora, very few studies approach the issue of the overall sentiment of the language used to describe the different genders. Romaine (2000), pp. 109-110 claims that “words with negative overtones are still more frequently used together with girl/woman than with man/boy”, supporting the claim with the frequencies of occurrences in the 3 mln (sub) corpus of BNC for such adjectives as hysterical, silly, loose and ugly vs. honest and intelligent. But this is a bit of cherry-picking: in Pearce’s (2008) study attractive, beautiful, glad are used predominantly about women, while ignorant, cruel and mad are more frequently applied to men. In other words, it is a priori unclear whether gender biases in the representation of females vs. males will go hand in hand with the overall prevalence of negative collocations or other overt linguistic markers of negativity in their descriptions.
We have found two recent studies aiming at quantifying the degree to which the language used to describe females and males differs in being more positive or negative. DeFranza et al. (2020) use word embedding to test whether the male vs. female members of 218 gendered noun and pronoun pairs differed in their overall semantic similarities to 25 positively vs. 25 negatively valenced words in Wikipedia and in the Common Crawl corpus (containing snapshots of all the texts available to the general public on the Internet since 2013) in 45 languages. It turns out that a substantial portion of the corpora (Wikipedia in 21 languages and the Common Crawl corpus in 19 languages) manifest a higher degree of association of male words with positively valenced words.
Hoyle et al. (2019) have used a list of 22 gendered noun pairs and the pronouns he and she for pulling out collocations in a huge corpus (11 bln words) by means of a generative latent-variable model that jointly represents adjective or voice choice with its sentiment. While there are great differences in the exact semantics and semantic class of the positive and negative adjectives and verbs applying to females versus males, there is only one significant difference in the overall sentiment of these combinations: adjectives applying to men are more often neutral than those applying to women.
To summarize, previous research shows several main opinions. While some researchers highlight predominantly derogatory attitudes toward females in language and society, others find ambivalence; according to the latter, the stereotypical representations of both men and women include positive and negative features. Finally, some studies show that words representing males more often occur in neutral contexts in comparison with words representing females. In our study, we want to investigate which of the opinions is best supported by usage data. Another important question is whether the situation has changed over recent decades or not. Thanks to the effort of feminists and movements like #Metoo, many countries have witnessed progress in the political, economical and cultural role of women in the society. If there was indeed a bias for negative or less neutral sentiment toward women, it may have become weaker recently.
While most of the previous quantitative work has been on English and has used synchronic data, our study takes a cross-linguistic and diachronic approach. We use state-of-the-art NLP methods – in particular, Aspect-Based Sentiment Analysis – to look for evidence of derogation or non-neutral status of nouns referring to women in language use. More specifically, we want to find if there are differences between pairs of nouns denoting male and female humans, in terms of their sentiment – positive, negative or neutral, and, in case there are gender differences, whether they have decreased with time. For this purpose, we use large diachronic corpora of Chinese, English and Russian. The choice of languages is motivated by their diversity: these languages represent two language families (Chinese: Sino-Tibetan, English and Russian: Indo-European) and are typologically very different – from the isolating Chinese to the analytic English and finally to the synthetic Russian. They are also typologically different in their relation to grammatical gender: Russian has a three-gender distinction into masculine, feminine and neuter in its nouns and pronouns and an obligatory gender agreement in adjectives and several other groups of words (including verbs in their past forms); English has an obligatory three-gender distinction in personal pronouns, with he and she restricted to animate referents, while Chinese lacks any obligatory gender distinctions, but can optionally distinguish between “he”, “she” and “it” in writing (see also 2.1). This is relevant for the ongoing debate on whether “gendered” languages, i.e., languages with a differentiation between masculine and feminine genders, display more gender prejudice than genderless languages (cf. DeFranza et al., 2020).
The second consideration for the choice of Chinese, English and Russian was availability of large diachronic corpora. Originally we wanted to study fiction in different languages. Fiction has important properties that make it attractive for a diachronic analysis of sentiment. First, it often contains fragments resembling everyday language use. Second, it is possible to obtain data from different historical periods and perform a diachronic analysis. But most importantly, fiction authors tend to express emotions and feelings of their characters toward other people. However, finding diachronic corpus data turned out to be more problematic than we had expected. For English and Russian, which we originally started with, we have found data representing fiction covering the time from 1950 to 2019. While the English and Russian data are comparable, we were not able to find a completely matching corpus of Chinese. The time span of the Chinese data is smaller – only 20 years, from 1991 to 2010. Unfortunately, it was difficult to access diachronic fiction data in Chinese, so we had to use data from newspapers. While this difference between the Russian and English data, on the one hand, and the Chinese data, on the other, is a limitation of our study, the similarity of the results based on the different sources is striking (cf. also fn. 3).
Our approach differs from the large-scale diachronic studies of biases and stereotypes, which employ word embeddings to compute average distributional vectors that represent social constructs of interest, such as gender or race (e.g., Garg et al., 2018; Morehouse et al., 2023). The results of such studies are often difficult to interpret because the dimensions of the embeddings are a “black box” without inherent meaning. In our study, we investigate the lexical categories representing female and male categories directly, obtaining their sentiment values in every context of use.
The remaining part of our paper is organized as follows. Section 2 discusses the corpora, the process of data extraction, the computational and statistical methods, and a few methodological caveats that may influence the interpretation of our data. In Section 3, we report the results of our analyses. Finally, Section 4 concludes the paper, discussing the main findings and providing a perspective.
2 Materials and methods
2.1 Materials
Table 1 displays the words we analyzed in this study. We focused on nouns because pronouns would not be directly comparable across the languages. In English, the pronouns he and she are used only for animate referents (with a few exceptions). Spoken Chinese has no gender distinctions in the 3rd person singular, although one can differentiate between the equivalents of “he”, “she” and “it” in writing. In Russian, both animate and inanimate referents can be anaphorically referred to with gendered pronouns on ‘he’, ona ‘she’ and ono ‘it’ depending on the lexical gender.
The choice of the nouns was motivated by the following reasons. First, these lexical categories exist in all three languages and can be easily found in corpora (although the semantic extensions may differ). Second, these nouns indicate the gender of the referent in all three languages (with a small number of exceptions discussed in Section 2.4). Finally, they occur frequently enough to allow for a comparison of their sentiment values and tracing their changes over time.
We extracted examples of these words in context from large corpora. The data and extraction procedure are described below.
To collect English data, we used the Corpus of Historical American English (COHA) (Davies, 2010). We extracted contexts containing the word forms of interest from the fiction component of the corpus covering years from 1950 to 2019. For data extraction, we used the online version of the corpus at https://www.english-corpora.org/coha/. We downloaded a random sample of 500 sentences per decade with the nouns in the singular or plural form, with the part-of-speech tag NOUN. If the form was infrequent, we included all available examples. The total number of examples was 75,736 sentences. We also saved information about the book in which every example appeared.
To find Chinese sentences, we used a local copy of the Chinese Gigaword Corpus Fifth Edition, Xinhua (XIN) and CNA sections. The data represented news from 1991 to 2010. We used a script to extract the examples and metadata. We took all sentences we could find in the corpus that contained the words representing “mother-in-law” and “father-in-law”, which were relatively infrequent. We sampled 7,000 observations representing each of the other lexical categories, which were more frequent. The total sample size was 91,095 sentences from the news agencies CNA and Xinhua.
As for Russian, we extracted sentences from the Russian National Corpus (RNC, ruscorpora.ru), using the online interface. We pre-selected the subcorpus of fiction (“xudožestvennaja literatura”) from 1950 to 2019. We searched separately for the lemmas in the RNC in texts written by female and male authors, and with grammatical features “Singular” and “Plural”. We had to perform a manual check of ambiguous word forms because not all forms were disambiguated in the corpus. For example, the form teste is the singular locative case of the words ‘father-in-law’ and ‘dough’. The irrelevant forms were excluded, as well as ungrammatical and archaic forms, e.g., otče ‘father’ in the vocative case. We downloaded all available examples. Because the total size of the data was very large, we sampled 7,000 sentences for each of the concepts, with the exception of the words representing “mother-in-law” and “father-in-law”, which were infrequent in the corpus compared to the other nouns and of which we took all examples. The total number of sentences in the final dataset was 86,020.
2.2 Methods
2.2.1 Aspect-based sentiment analysis
In order to obtain sentiment polarity values for the instances of the female and male terms in the corpora, we employed state-of-the-art Aspect-Based Sentiment Analysis (ABSA). It is a subtype of sentiment analysis and opinion mining, which allows companies to analyze customer opinions and sentiments expressed in reviews of products and services and helps improve marketing campaigns. Traditional sentiment analysis provides sentiment polarity values (usually positive, negative or neutral) to entire sentences or texts. In contrast, Aspect-Based Sentiment Analysis can deal with situations when a sentence expresses different sentiments to different entities. For example, in the sentence I love the pizza at this restaurant, but the service is terrible, there are different sentiments toward pizza and service: positive and negative, respectively. The words pizza and service are called aspect terms (for more detail, see Zhang et al., 2022).
We obtained ABSA polarity values using two approaches. One of them was using a multilingual model from the PyABSA toolkit (Yang and Li, 2023).1 For illustration, consider several examples from the COHA. The aspect term is underlined.
1. a. The woman extended her hand. [NEUTRAL].
2. b. I cannot think of anything more exciting than drinking champagne in a pretty woman’s bedroom. [POSITIVE].
3. c. She looked socially prominent, but the type of society woman that could be easily induced to lend her name and face to a cold-cream advertisement. [NEGATIVE].
4. a. The man opened his eyes. [NEUTRAL].
5. b. He was a man in a million. [POSITIVE].
6. c. Wherever that man goes, there is trouble, he said. [NEGATIVE].
The model was trained on different datasets, which contain mostly customer reviews of different products and restaurants. This represents a limitation. There is a danger that human beings will be evaluated based on the same criteria as laptops or shampoos, or at best as service personnel. This is why we also used a second method, employing GPT-3.5-turbo (Brown et al., 2020; Ouyang et al., 2022) from OpenAI. GPT models have been used previously for ABSA and related tasks (Hosseini-Asl et al., 2022), but to the best of our knowledge not involving human referents. Because of the costs involved in processing of large numbers of tokens, we only annotated a part of the datasets, drawing random samples of 400 examples of every lexeme. For English and Russian, the examples of the singular and plural forms were sampled separately, for a total of 800 samples per lexeme. Some of forms were less frequent than 400 instances (e.g., the forms representing PARENTS-IN-LAW), in those cases all instances were used. This resulted in 10,214 annotated examples for the English data, 10,070 for the Russian data and 5,600 for the Chinese data.
In order to obtain ABSA judgments from the GPT models, we used a few-shot approach. In few-shot learning, a small number of example questions with human-assigned answers are given as context, followed by a question. The language model is then queried for the most likely continuation to this context, with the expectation that the final question is answered. Such a context is referred to as a prompt. In our case, we used a prompt according to the following pattern:
“You will guess the sentiment of the author toward a particular person. Answer only with a single word, one of the following: Positive, Negative, Neutral. When in doubt, answer Neutral.
Question 1: what is the attitude toward “sister” in the following text? < << His sister won an Olympic gold medal. >>>
Question 2: what is the attitude toward “mothers” in the following text? < << Their mothers were all above the age of 80. >>>
Question 3: what is the attitude toward “mother-in-law” in the following text? < << Her mother-in-law likes to watch her suffer. >>>
Answer 1: Positive.
Answer 2: Neutral.
Answer 3: Negative.
Question 1: what is the attitude toward “fathers” in the following text? < << Their fathers were all very interested in chess. >>>
Question 2: what is the attitude toward “brother” in the following text? < << Her brother won an Oscar. >>>”
After the above prompt, we would expect a good language model to generate the following text, from which we then extract the sentiment labels of “father” and “brother” in the last two sentences above:
“Answer 1: Neutral.
Answer 2: Positive.”
Our reasoning in this case is that a person winning an Olympic medal or an Oscar are clear contexts where most readers would be expected to gain positive impressions of the people behind these achievements, as opposed to for instance a person simply liking chess.
We used a fixed set of examples for each language (English: 10; Chinese: 13; Russian: 15), divided into two question/answer blocks with the examples approximately evenly split between them. These were sent through the OpenAI API, using the model gpt-3.5-turbo. To ensure maximal consistency, we used a very small temperature parameter of 10−6. For efficiency, we sent queries in batches of 10. In other words, our prompts ended with 10 questions, and we expected a text containing the corresponding 10 answers in return.
To see how reliable the models are, we compared a small number of randomly selected and manually annotated sentences with the labels provided by PyABSA and GPT-3.5. The results are shown in Table 2. The Accuracy score represents the proportions of correct labels in the total number of annotated examples. With three categories, the baseline accuracy is obtained by randomly guessing is 33.3%. However, since most sentences in the data carry neutral sentiment, it is possible to obtain a much higher accuracy by assigning the label “neutral” to all sentences (cf. the prompt fragment “When in doubt, answer Neutral” for GPT-3.5). The macro-averaged F1-score is the mean of F1 scores across the three sentiment labels, and each such F1 score is the harmonic mean between precision and recall for that label. The F1 score considers how good the model is at identifying each of the categories, and always predicting “neutral” would yield zero F1-scores for the positive and negative categories. The performance metrics show that the GPT-3.5 model strongly outperforms the PyABSA model. This is explained largely by different proportions of neutral sentiment assigned by each system, which is the sentiment observed in most of the test sentences. We have also evaluated the more recent GPT-4 model, and found it to be roughly equal in performance to GPT-3.5. The higher cost prevented us from applying GPT-4 to larger amounts of data.
2.2.2 Generalized linear mixed-effect models
To test the effect of gender on sentiment, we used Generalized Linear Mixed-effect Models with logit as the link function. For every dataset, we fitted two types of models to test the main expectations based on previous findings. The first one predicted if the sentiment was neutral or not, as a follow-up of the results reported by Hoyle et al. (2019). The second one, which was inspired by the claims about pejoration summarized in Section 1, predicted if the sentiment was positive or negative, excluding the examples with neutral sentiment. Because of the multiple comparisons performed on the same data, we used a Bonferroni correction for model selection.
The fixed effects in all models contained the gender of the referent (female or male) and a scaled and centered version of the year. These variables are directly relevant for our expectations about the gender differences in synchrony and diachrony. In addition, we tested several covariates, which could potentially influence the results. The English and Russian models contained the number of the referents (singular or plural) because one could not exclude that writers have different attitudes to a woman or man as an individual and as a group. The number of referents in the Chinese sentences was, unfortunately, too difficult to control for, because Chinese nouns are usually not marked for number. The Russian data and one Chinese dataset contained the author’s gender (female or male). This was an important factor to consider because it is possible that female and male writers have different attitude toward persons of their own and of the other gender(s). All pairwise interactions were tested, and the ones with the corrected p-value of the likelihood ratio test less than 0.05 were added to the model. In the Chinese dataset without the authors’ data, we tested the source (one of the two news agencies, XIN and CNA) as a fixed effect.2
There were also variables that were treated as random effects. The concept pairs were treated as random intercepts in all models. The individual books were random intercepts in English and Russian, and individual authors were random intercepts in the Chinese model that included author’s data. This was necessary because the assumption of independence of observations was violated, with more than one sentence coming from one and the same book or individual author. Different authors could have their individual biases toward male and female referents they wrote about. All potential random slopes were tested using the likelihood ratio test.
The variables are summarized in Table 3.
2.3 A caveat: replaceability of female and male terms
An important caveat is that the gender-specific words may have their own contribution to the sentiment scores. In order to check whether the sentiment classification depends on the target noun itself, we selected a random sample of 1,000 sentences from each corpus and obtained the sentiment values as described above. After this, we replaced the target words with their correspondences of the opposite gender, e.g., woman was replaced with man, boys was replaced with girls, and so on. The sentiment analysis was then run on the modified sentences with the help of PyABSA. Finally, we computed the proportions of the same classification of the sentences before and after the modification. The results showed that for the overwhelming majority of the sentences the gender did not matter. But some of the concepts were slightly more sensitive to this transformation than others, although we found no systematic patterns across the languages.
In the English sample, the same label was assigned in the same context in 87.1% of all cases. The greatest effect of changing the gender was in sentences with “brother” (overlap 82.1%) and “sister” (overlap 83.1%). The weakest effect was in sentences with “daughter” (overlap 92%) and “son” (overlap 91%). “Mother-in-law” and “father-in-law” had only a few occurrences in the random sample, so they were not considered.
As for Chinese, the same label was assigned in the same context in 89% of all cases. The concepts “wife” and “woman” had the greatest effect of replacement (77.8 and 82.1% overlap, respectively). The replacement had the weakest effect for the concepts “boy” (96% overlap) and “daughter” (93.4%).
In the Russian sample, the same label was assigned in the same context in 89.3% of all cases. The greatest effect of replacement was in sentences with “daughter”, “mother” and “father” (overlap less than 85%). The smallest effect was in sentences with “man” and “woman” (overlap more than 94%), as well as the low-frequency “mother-in-law” and “father-in-law” (overlap 100%).
It is very difficult to say whether these differences have to do with the inherent sentiment associated with the individual words, or with their interaction with the context. For example, a pretty woman can be perceived positively in a heteronormative culture, but a pretty man may not. Moreover, as Romaine (2000), p. 109 observes, even seemingly gender-neutral terms have different connotations when applied to men and women. For example, to call a man a professional is a compliment, but in some languages, such as English, Japanese or French, if a woman is called a professional, this may be a euphemism for a prostitute. All this means that the sentiment value depends on the complex interaction of the target word with its context, which requires further investigation.
2.4 Another caveat: polysemy and male bias
In our large corpus study, we did not have tools to control for polysemy of the nouns. One widely spread type of polysemy is the use of male terms to represent male and female referents, which serves as evidence of the unmarked status of male forms in structuralist theories of semantic markedness (Jakobson 1971[1932]). This type of polysemy is common across languages and represents an example of the so-called male bias (Aikhenvald, 2016). In English, it is observed in the semantics of the English word man. An example from COHA is below.
(3) The hydrogen bomb represented the ultimate refinement in man’s search for the means of self-destruction. (Morgan’s Passing by Tyler, 1980)
This type of polysemy may distort the results. In order to estimate the size of the problem, we performed a manual check of 500 occurrences of the form man in our dataset and found only 17 instances where the form could be interpreted as referring to a human being regardless of their gender. This accounts for only 3.4% of the data. We can conclude that this type of polysemy does not play an important role in English. In Russian, a similar polysemy is observed in the word brat ‘brother’, as in All people are brothers. A manual check of 500 randomly selected sentences revealed, again, only 17 cases (3.4%) where this word could be potentially interpreted in this sense. This means that this type of polysemy was unlikely to cause major distortion in our analysis.
Other types of polysemy include the use of the Russian nouns sestra ‘sister’ and brat ‘brother’ in the meaning ‘nurse’. In some cases, the words mat’ ‘mother’, otec ‘father’ and brat ‘brother’ are used as terms of address that do not imply any kinship, similar to bro in English. This extended use of kinship terms is also common in Chinese, where for instance 兄弟 ‘brother(s)’ is frequently found in contexts such as ‘brother peoples’. In Chinese, we also find polysemy within the kinship domain, with 剬剬 ‘father-in-law’ also sometimes being used for ‘grandfather’. We included all these uses, as well. Our statistical models allowed us to control for the potential biases associated with individual concepts with the help of random effects.
3 Results
3.1 English
3.1.1 Descriptive statistics
3.1.1.1 PyABSA
Figure 1 displays the proportions of positive, neutral and negative scores in the English data across the genders and conceptual pairs, obtained with PyABSA. We can see that the proportions vary in a subtle way across the genders and more substantially across the pairs. For example, the pair ADULT (“woman” and “man”) has less often neutral sentiment than the pair CHILD (“daughter” and “son”), but the proportions within each pair are almost equal. In some of the pairs, however, female referents are less often evaluated neutrally than male ones, e.g., NOT ADULT (“girl” has fewer neutral scores than “boy”), PARENT (“mother” vs. “father”) and PARENT-IN-LAW (“mother-in-law” vs. “father-in-law”). These female nouns are also more often used both in positive and in negative contexts than their male counterparts.
3.1.1.2 GPT-3.5
Figure 2 shows the proportions of the positive, negative and neutral scores obtained with the help of GPT-3.5. The neutral scores are predominant for all lexemes. We observe no large differences between the female and male lexemes, with the exception of the pair PARENT-IN-LAW, where “mother-in-law” has visibly more negative scores than “father-in-law”. Unlike what we saw above, we find no indications of the male lexemes being more often used in neutral contexts than the female lexemes.
3.1.2 Generalized linear mixed-effect models
3.1.2.1 PyABSA
The first model was fitted to predict whether a sentiment score was neutral or not. We included random intercepts for each Concept Pair and Source (the book). We also tested all possible random slopes and ended up having random slopes for Gender, Number and the interaction between Gender and Number. The coefficients of the fixed effects, as well as their 95% confidence intervals and Bonferroni-corrected p-values, are shown in Table 4. Positive log-odds ratios of the coefficients (or simply log-odds, for the intercept term) show that the variable increases the chances of neutral sentiment. Negative log-odds ratios decrease the likelihood of neutral sentiment. A log-odds ratio very close to zero means that there is no effect. Log-odds ratios can be transformed into odds ratios, which represent the ratio of odds of neutral sentiment in the presence of the specified value (or increase in one unit, for numeric variables) and the odds of in the absence of this value (or 0 for numeric variables). If a variable has no effect, the odds ratio will be 1.
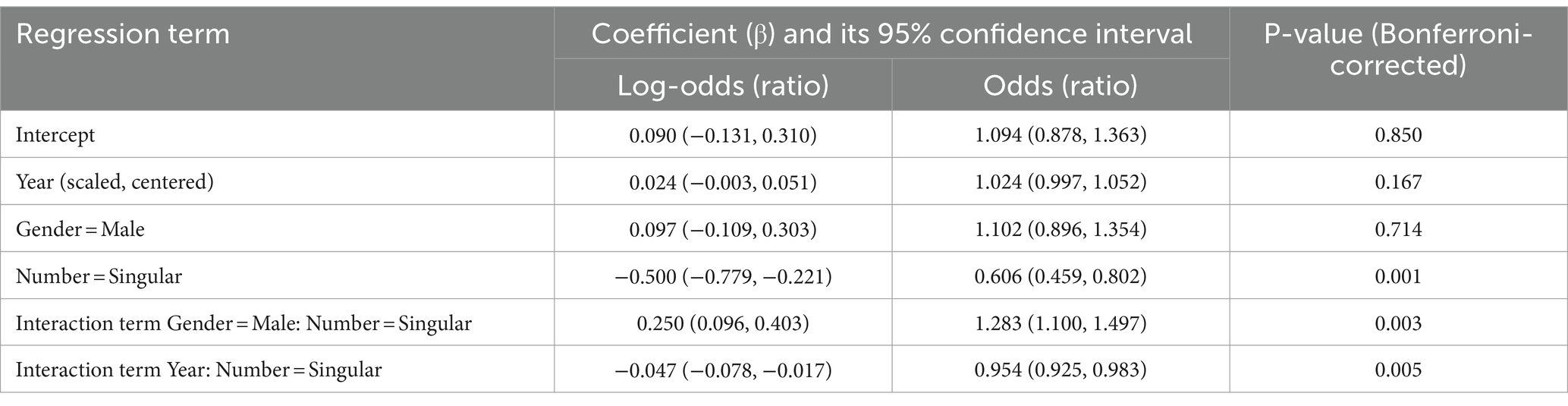
Table 4. Coefficients and their Wald 95% confidence intervals (in parentheses) of the fixed effects in the generalized linear mixed-effect model with the response variable “neutral or non-neutral” based on the English data and PyABSA.
We observed two significant interactions. One of them was between Year and Number. We found that singular nouns tend to become slightly less neutral with time, while plural nouns become slightly more neutral. The more important interaction for us, however, is the interaction between Gender and Number. This interaction is displayed in Figure 3. Our model revealed that the male nouns are more likely to get neutral scores than the female nouns in the singular. However, there was only a small difference in the plural.
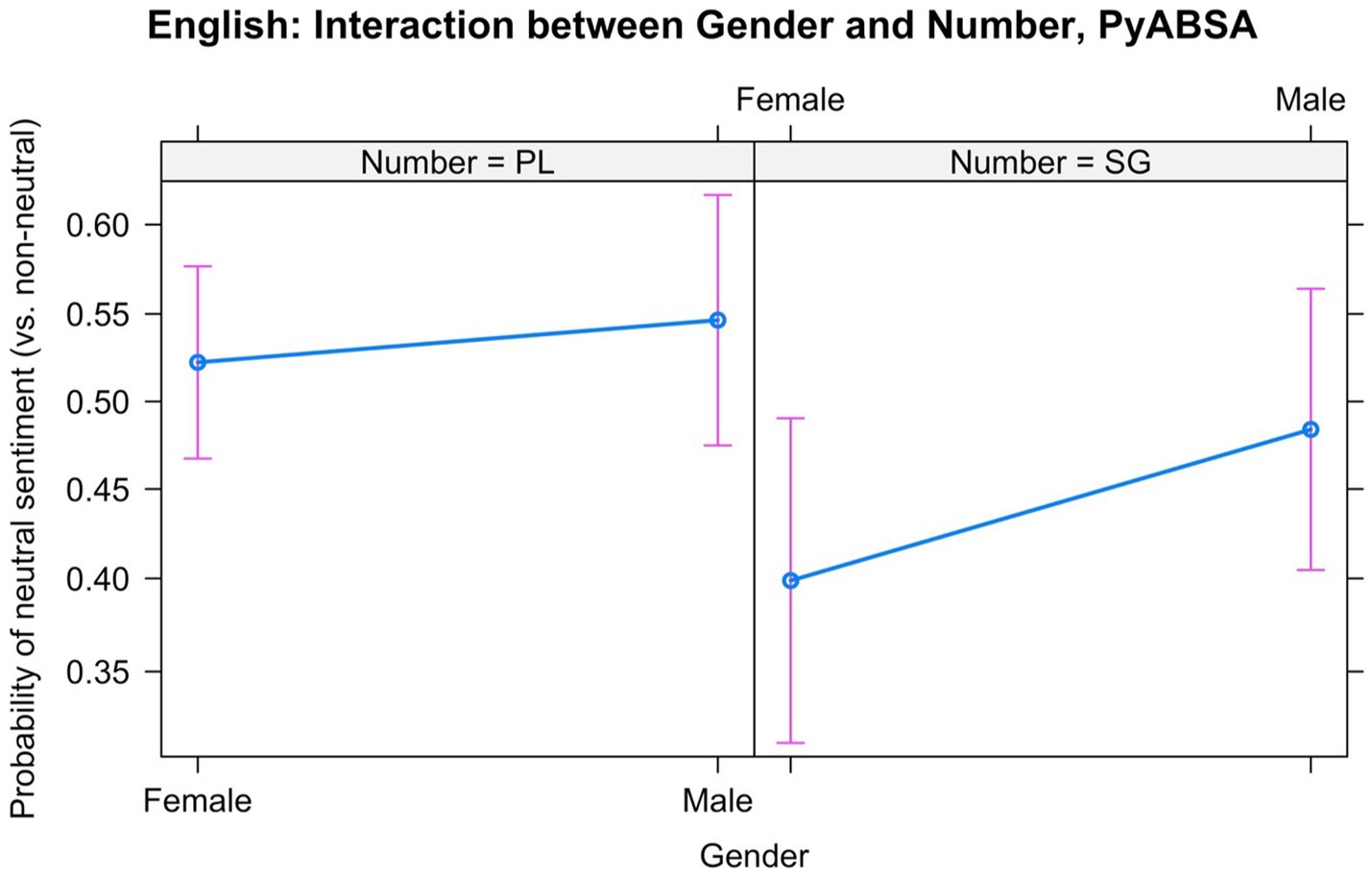
Figure 3. Interaction between Gender and Number in the English data: Neutral vs. non-neutral sentiment, based on PyABSA.
We fitted the second model to predict whether a gendered word has a positive or a negative sentiment score, excluding the neutral scores. The main statistics are provided in Table 5. In this case, positive log-odds ratios or odds ratios above 1 show that the variable increases the chances of positive sentiment, and negative log-odds ratios or odds ratios below 1 indicate that the chances of negative sentiment are higher.
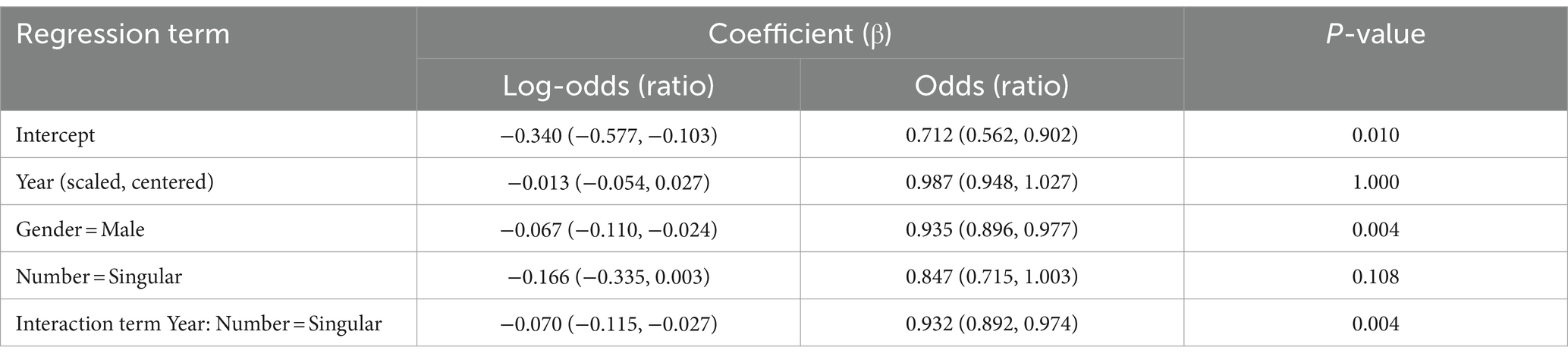
Table 5. Coefficients and their Wald 95% confidence intervals (in parentheses) of the fixed effects in the Generalized Linear Mixed-effect Model with the response variable “Positive or Negative” based on the English data and PyABSA.
In this model we observed a significant main effect of Gender. As shown in Figure 4, female nouns are more likely to have positive sentiment scores than male nouns, and male nouns are more likely to get negative scores. The effect is significant (p = 0.004), but very small: the odds of positive sentiment if the referent is male are 0.935 as large as the odds of positive sentiment if the referent is female. To reformulate this, the odds of positive sentiment if the referent is female are only 1.069, or about 7%, higher than the odds of positive sentiment if the noun is male.
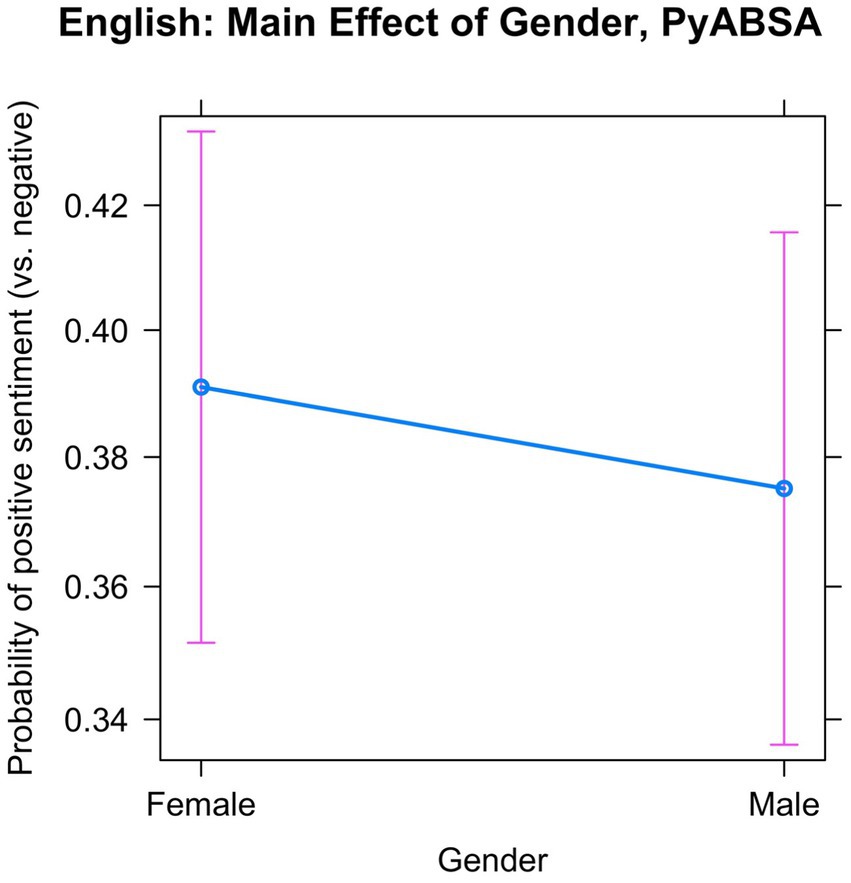
Figure 4. Main effect of Gender and Number in the English data, based on PyABSA: Positive vs. negative sentiment.
In addition, an examination of the interaction, which is not displayed here due to space limitations, reveals that singular nouns become more often negatively tagged with time for both genders, but this trend is much weaker in the plural.
3.1.2.2 GPT-3.5
This subsection reports the regression modeling results based on GPT-3.5 with few-shot learning. The best model for neutral vs. non-neutral sentiment did not include Gender because it was not significant (corrected p = 1). No random slopes or interactions improved the model. The only significant fixed effects were Year and Number, shown in Table 6. We observe a decrease of neutral sentiment with time. We also find that singular nouns have lower chances of neutral sentiment.

Table 6. Coefficients and their Wald 95% confidence intervals (in parentheses) of the fixed effects in the Generalized Linear Mixed-effect Model with the response variable “Neutral or Non-neutral” based on the English data and GPT-3.5.
As for positive vs. negative sentiment, the best model included only one fixed effect: that of Number. As shown in Table 7, singular nouns have higher chances of being associated with positive sentiment than plural ones.

Table 7. Coefficients and their Wald 95% confidence intervals (in parentheses) of the fixed effects in the Generalized Linear Mixed-effect Model with the response variable “Positive or Negative” based on the English data and GPT-3.5.
3.1.2.3 Summary
The models of data annotated by PyABSA and GPT-3.5 agree in several important respects. First of all, we find no evidence of the female nouns to be more associated with negative sentiment than the male nouns. Secondly, there is no predicted interaction between Gender and Year, which would display a gradual convergence between the genders during the time period examined.
There are also intriguing differences between the approaches. While the PyABSA approach reveals a tendency for singular male nouns to be more often associated with neutral sentiment, we find no such tendency in the GPT-3.5 data. Also, the PyABSA data show that male referents tend to occur in more negative contexts, whereas the GPT-3.5 data yield no significant gender differences at all.
Can these differences be explained by the smaller size of the data annotated by GPT-3.5? Refitting the models on the smaller sample reveals that the preference for singular male nouns to be associated with more neutral speeches, which was found with the help of PyABSA, is robust. Notably, the preference for male referents to be accompanied by more positive sentiment is no longer found in the smaller dataset. In the full PyABSA analysis, the effect was significant but small [odds ratio confidence interval (0.896, 0.977)]. Also, no effect of Year and Number is detected.
3.2 Chinese
3.2.1 Descriptive statistics
Figure 5 shows the proportions of different sentiments in the Chinese data with PyABSA sentiment scores. We can see that the words are in general more often positively evaluated than in the English data. The proportion of neutral sentiment is smaller. Overall, the figure suggests that the female concepts are less frequently evaluated neutrally than the male ones in all concept pairs. They also have more often positive sentiment, with the exception of “mother-in-law”. The proportions of negative sentiment vary a lot across the pairs and genders.
Figure 6 displays the proportions of different sentiment values obtained for the smaller Chinese sample (see Section 2.2.1). In contrast with the PyABSA data, most of the sentiment values are neutral. There are also no systematic gender differences: for example, the concept “man” is used more often positively and less often negatively than “woman” in the conceptual pair ADULT, but “mother” is used more often positively than “father” in the pair PARENT. “Girl” appears less often in neutral contexts than “boy” (see NOT ADULT), but “wife” is used more frequently in neutral contexts than “husband” (see SPOUSE).
3.2.2 Generalized linear mixed-effect models
3.2.2.1 PyABSA
The best model predicting neutral vs. non-neutral sentiment fitted to the PyABSA scores had random slopes for the conceptual pairs, modifying the effects of Gender and Source. The coefficients for the fixed effects are provided in Table 8. The model displays a significant effect of the referent’s Gender. Male referents are presented neutrally more often than female referents, as shown in Figure 7. The log-odds ratio coefficient is 0.472 (p < 0.001), which means in simple odds that male referents have 1.6 times higher chances of neutral sentiment than female ones. There was also a change in the sentiment scores in the XIN news. With time, they became more neutral. In the CNA news, we detected no differences.
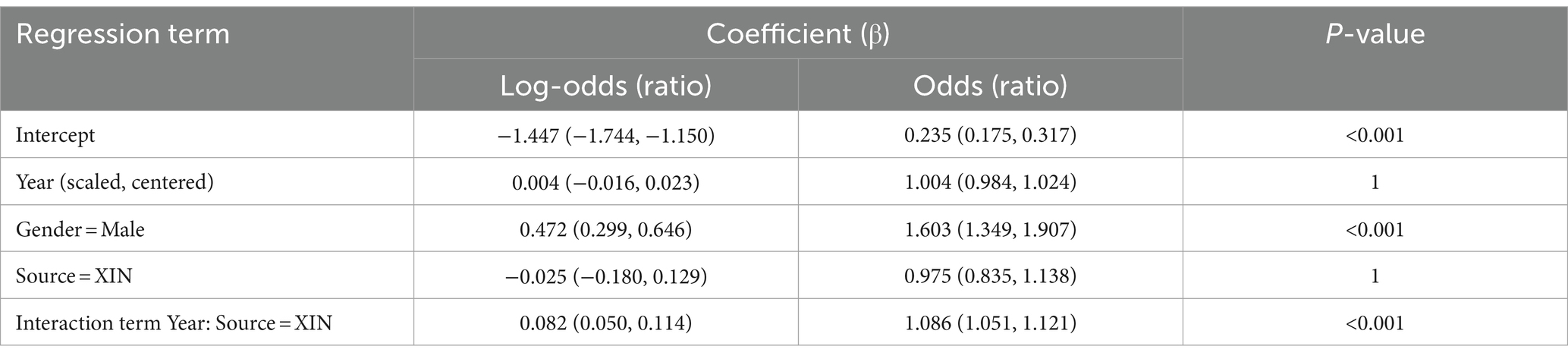
Table 8. Coefficients and their Wald 95% confidence intervals (in parentheses) of the fixed effects in the Generalized Linear Mixed-effect Model with the response variable “Neutral or Non-neutral” based on the Chinese data and PyABSA.
We also performed regression on the choice between positive and negative sentiment labels produced by PyABSA. The model had random slopes for Year, Gender and Source. The coefficients are presented in Table 9. The fixed effect of Gender is not statistically significant. As the interaction between Year and Source suggests (not shown due to space limitations), the sentiment values became more positive over time in XIN; in CNA, there is very little change.
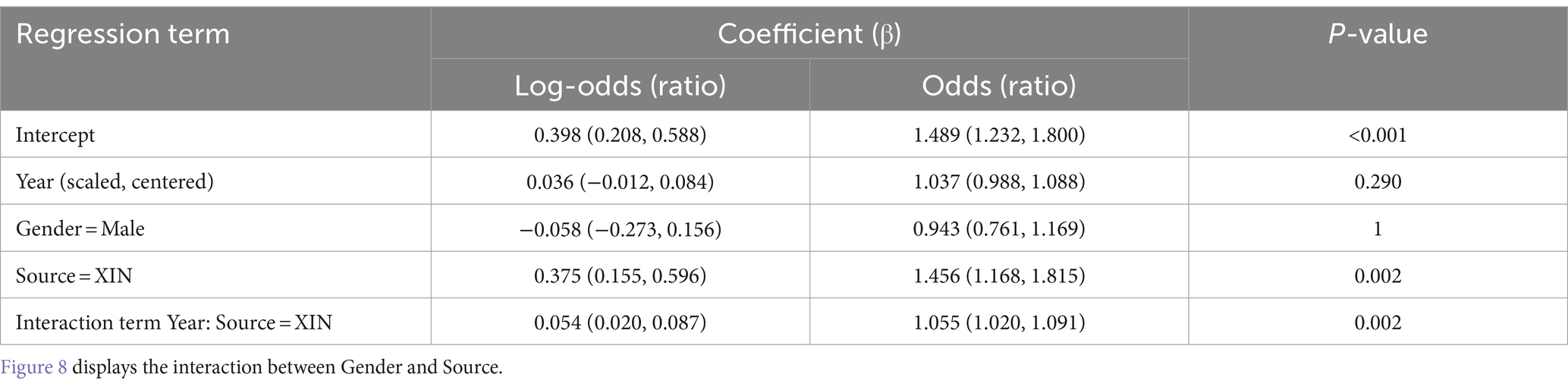
Table 9. Coefficients and their Wald 95% confidence intervals (in parentheses) of the fixed effects in the Generalized Linear Mixed-effect Model with the response variable “Positive or Negative” based on the Chinese data and PyABSA.
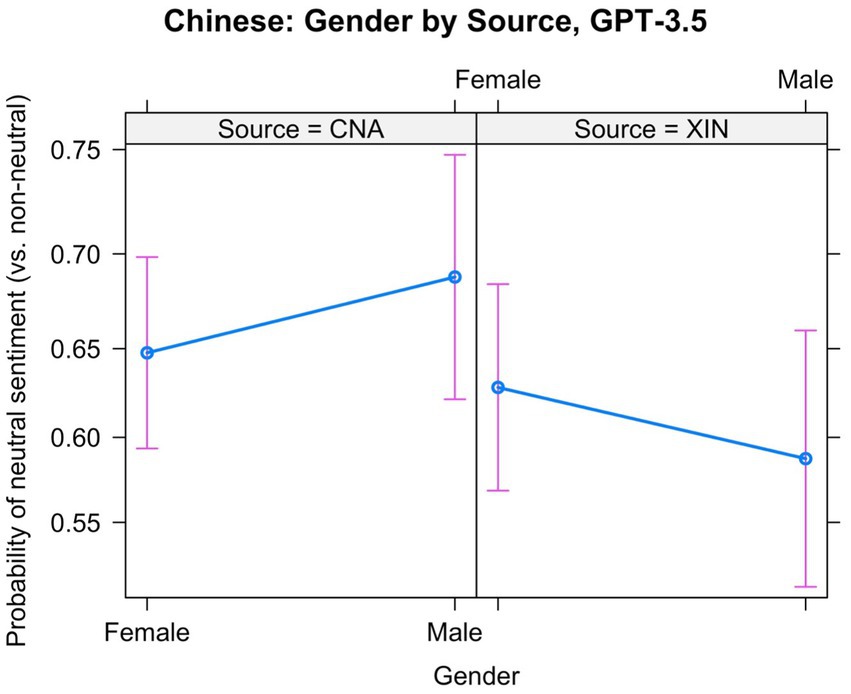
Figure 8. Interaction between Gender and Source in the Chinese data: Neutral vs. non-neutral sentiment, based on GPT-3.5.
3.2.2.2 GPT-3.5
We also fitted models based on the smaller sample annotated by GPT-3.5. The best model that predicts neutral vs. non-neutral labels had random slopes for Gender. The coefficients are shown in Table 10. The effect of Year was not significant, which is why this variable was excluded from the final model. There is a significant interaction between Gender and Source. As shown in Figure 8, we observe no consistent effect of Gender across the sources.

Table 10. Coefficients and their Wald 95% confidence intervals (in parentheses) of the fixed effects in the Generalized Linear Mixed-effect Model with the response variable “Neutral or Non-neutral” based on the Chinese data and GPT-3.5.
Finally, the best model that predicted positive vs. negative sentiment contained only Source (see Table 11). The odds of positive sentiment were higher in XIN than CNA. The effects of the other predictors were not significant.

Table 11. Coefficients and their Wald 95% confidence intervals (in parentheses) of the fixed effects in the Generalized Linear Mixed-effect Model with the response variable “Positive or Negative” based on the Chinese data and GPT-3.5.
3.2.2.3 Summary
The models based on PyABSA show that male referents are presented neutrally more often than female referents. In the GPT-3.5 sentiment labels, we find no stable effect of Gender, however. The direction of the effect depends on Source (the news agency). Neither approach has detected any gender-related differences with regard to positive vs. negative sentiment.
One should ask again if these differences between the approaches can be explained by the different sizes of the datasets used for the PyABSA and GPT-3.5. When we fitted the PyABSA neutral vs. non-neutral model on the smaller sample used for the GPT annotation, we found that the effect of Gender persists. At the same time, it is possible that the difference between the approaches is due to the greater bias toward neutral sentiment labels in the GPT-3.5 data.
3.3 Russian
3.3.1 Descriptive statistics
Figure 9 shows the proportions of each sentiment by Conceptual Pair and Gender in the Russian fiction data annotated by PyABSA. Notably, negative and positive sentiment prevail, whereas neutral sentiment is the least frequent. Still, one can discern that the male concepts tend to have neutral scores more often than the female ones. The female concepts are more often negative in most conceptual pairs, especially PARENT and PARENT-IN-LAW, but in some of them they are also more often positive (CHILD, NOT ADULT).
Figure 10 displays the proportions based on GPT-3.5. Unlike in the PyABSA results, the neutral sentiment prevails. The male referents are also no longer universally associated with more neutral sentiment: there is no difference in the pair SIBLING, and the male referent in the pair SPOUSE (that is, “husband”) is actually less often neutral than its female counterpart (“wife”). The differences between the proportions of positive and negative labels also vary across the conceptual pairs. While “mother-in-law” has the largest proportion of negative labels, “woman” and “mother” have relatively high proportions of positive labels.
3.3.2 Generalized linear mixed-effect models
3.3.2.1 PyABSA
The model that predicted neutral vs. non-neutral sentiment included the individual books and Conceptual Pairs as random intercepts, as well as random slopes of Conceptual Pairs for the variables Gender and Number. The coefficients of the fixed effects are shown in Table 12. There is an interaction of Gender with Year, which is displayed in Figure 11. The male nouns are always used more neutrally than the female nouns, but the sentiment labels of male referents become more neutral with time, whereas the labels of female referents become slightly less neutral with time. Contrary to our expectation, the gender gap in Russian literature increases.
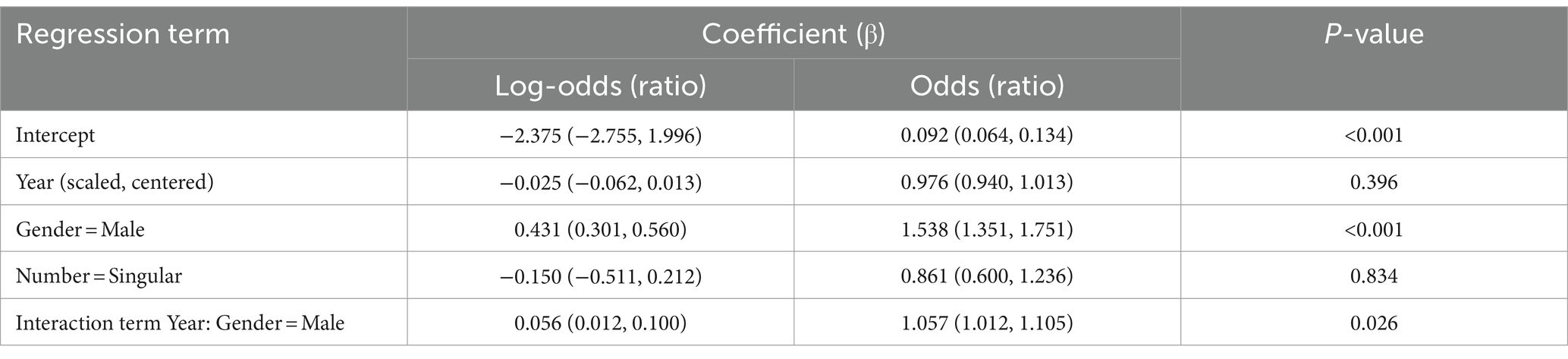
Table 12. Coefficients and their Wald 95% confidence intervals (in parentheses) of the fixed effects in the Generalized Linear Mixed-effect Model with the response variable “Neutral or Non-neutral” based on the Russian data and PyABSA.
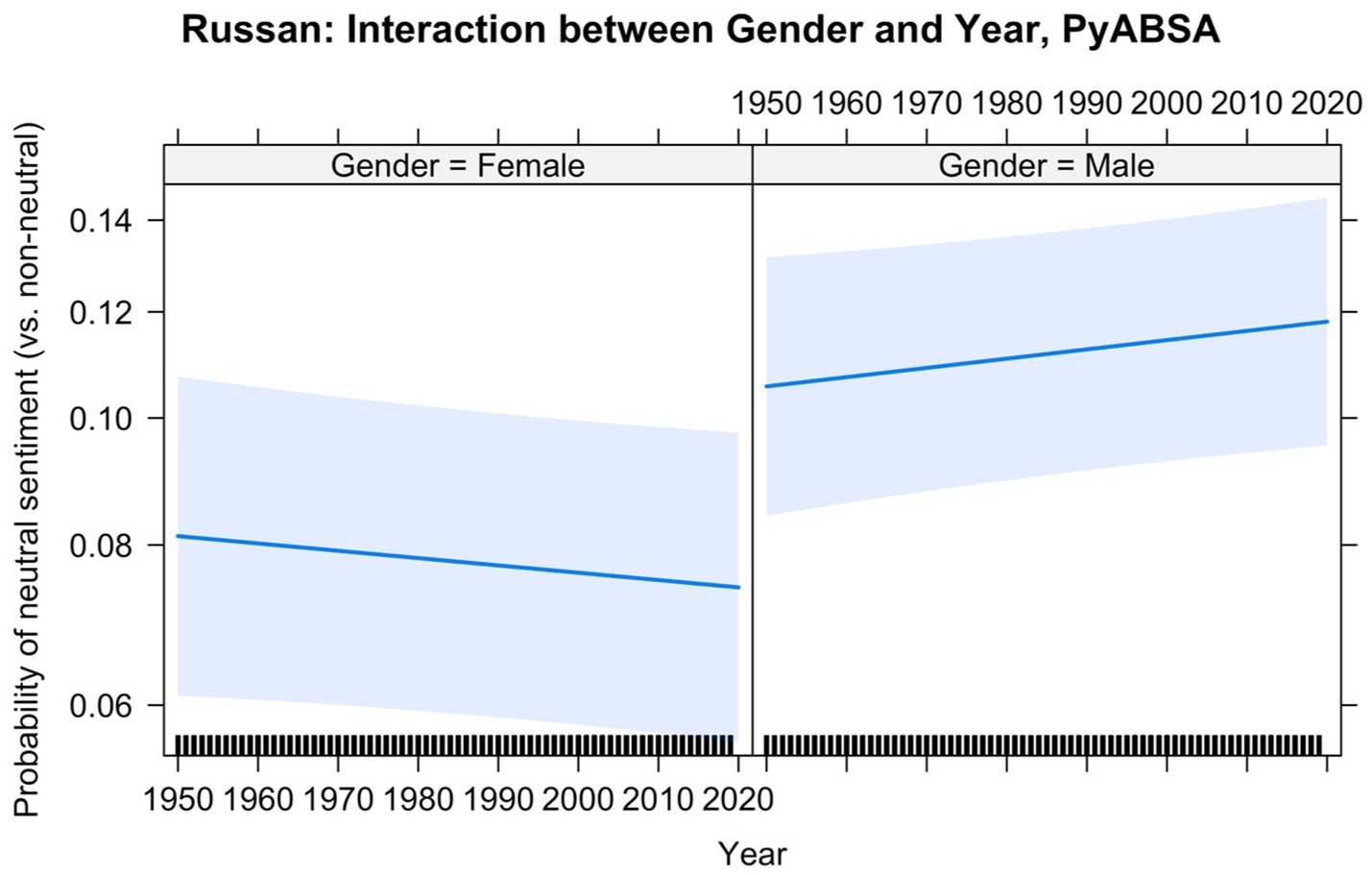
Figure 11. Interaction between Gender and Year in the Russian data, PyABSA: Neutral vs. non-neutral sentiment.
The second model, in which we predicted positive vs. negative sentiment, included the same random effects as the first model. The coefficients of the fixed effects are displayed in Table 13. The year did not play any significant role, so it was excluded from the final model. All the other predictors interacted. We found that the male nouns had slightly more often positive labels than the female nouns in the singular, but not in the plural, as shown in Figure 12.
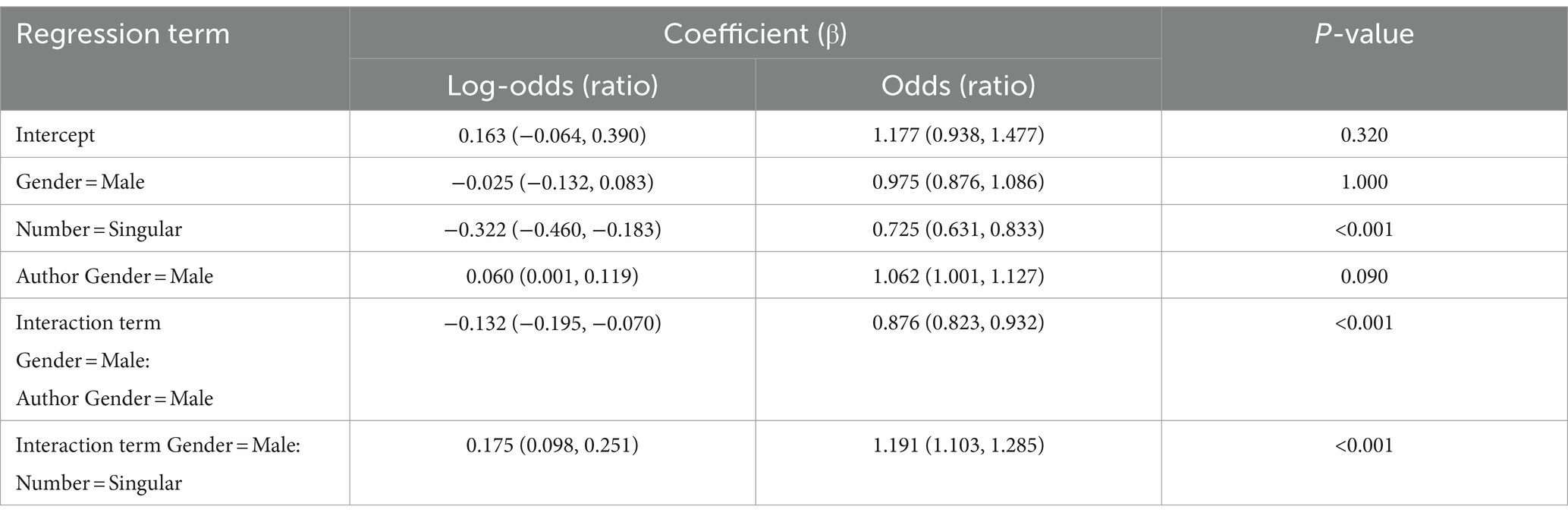
Table 13. Coefficients and their Wald 95% confidence intervals (in parentheses) of the fixed effects in the Generalized Linear Mixed-effect Model with the response variable “Positive or Negative” based on the Russian data and PyABSA.
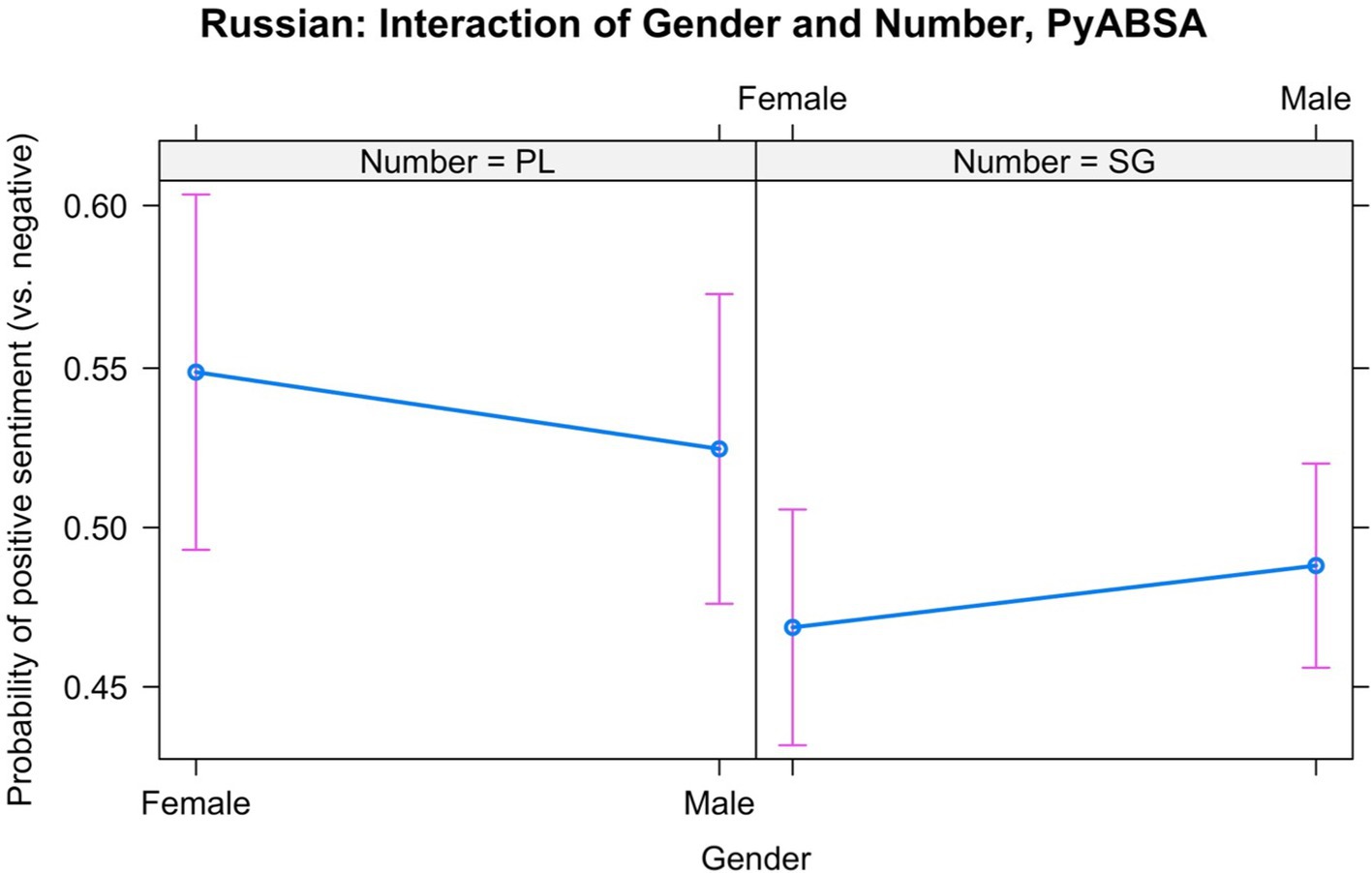
Figure 12. Interaction between Gender and Number in the Russian data, PyABSA: Positive vs. negative sentiment.
We also found an interaction between the referent’s gender and the author’s gender, which is displayed in Figure 13. Surprisingly, the male nouns in sentences written by female authors are slightly more likely to have positive scores than the female nouns. In the texts of male authors there is a very weak bias for the female nouns to get more positive scores than the male nouns.
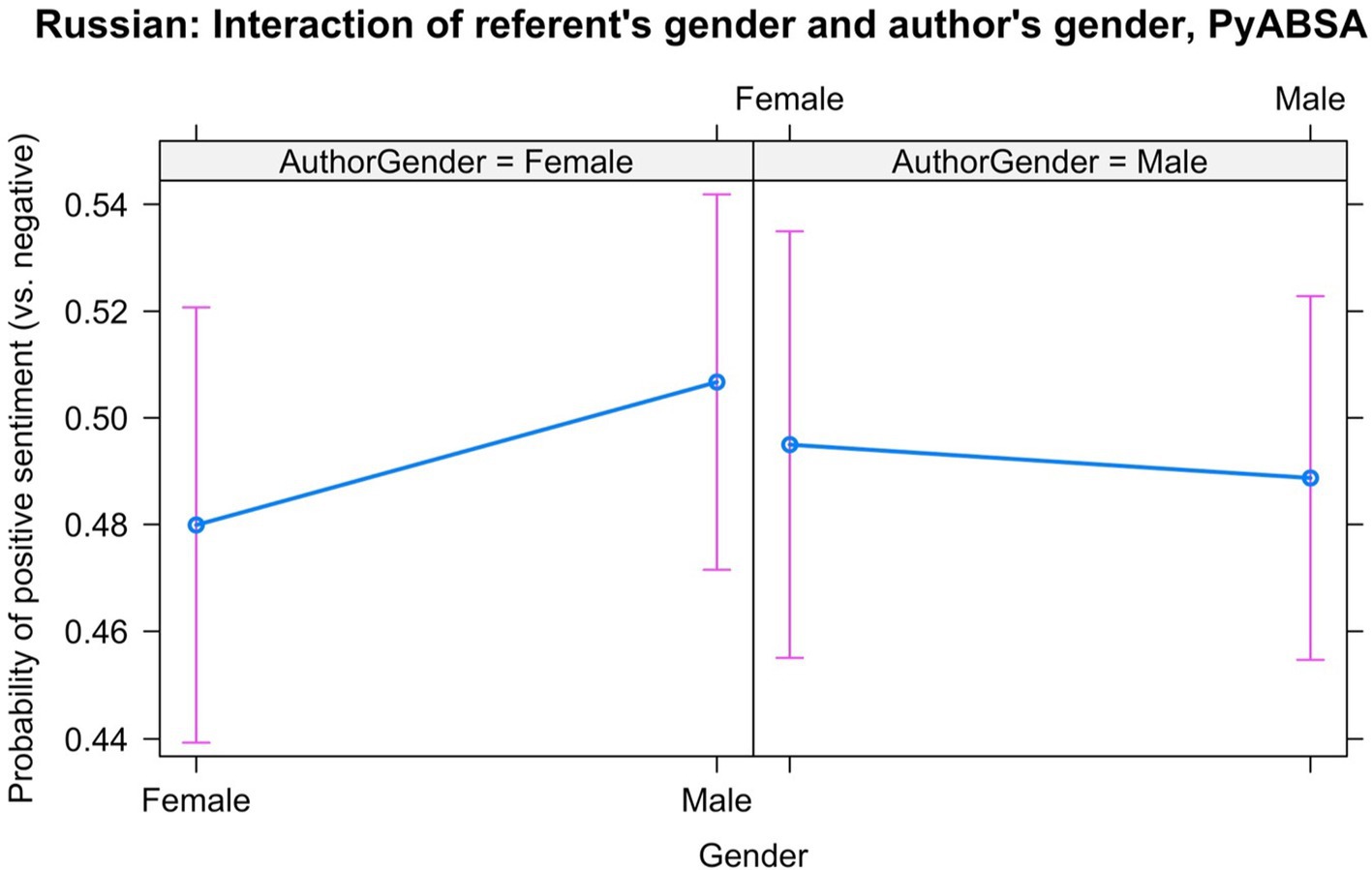
Figure 13. Interaction between Gender of the referent and Author’s Gender in the Russian data, PyABSA: Positive vs. negative sentiment.
3.3.2.2 GPT-3.5
The best model predicting neutral vs. non-neutral scores provided by GPT-3.5 contained random slopes for individual concept pairs, which modified the effect of the referent’s gender. The author’s gender did not play a role and was excluded from the final model. The coefficients are provided in Table 14. We observe an effect of the referent’s gender: male referents are about 1.26 times more likely to get neutral labels than female referents. This effect is displayed in Figure 14. There is also an interaction between Year and Number (not shown): plural nouns get fewer neutral labels with time, whereas singular nouns remain stable.
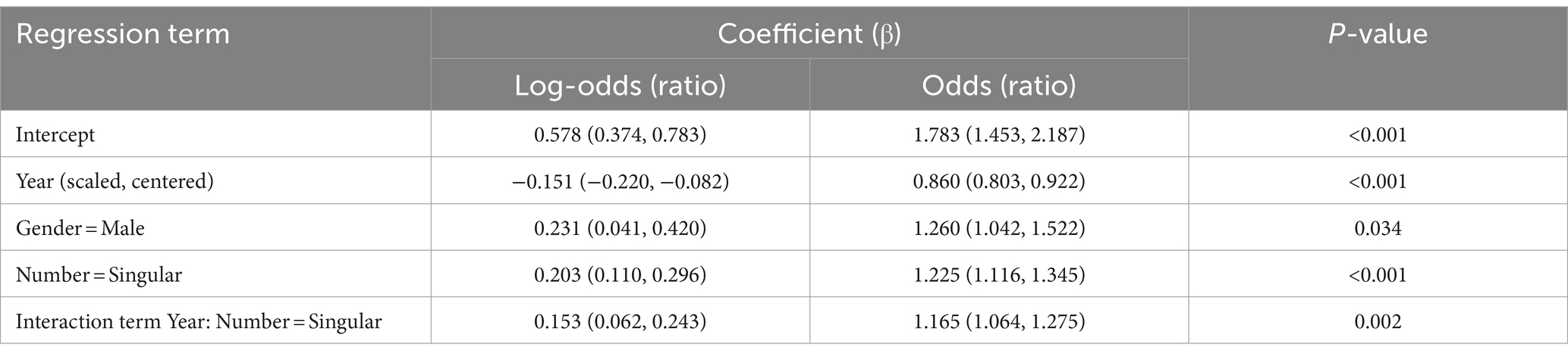
Table 14. Coefficients and their Wald 95% confidence intervals (in parentheses) of the fixed effects in the Generalized Linear Mixed-effect Model with the response variable “Neutral or Non-neutral” based on the Russian data and GPT-3.5.
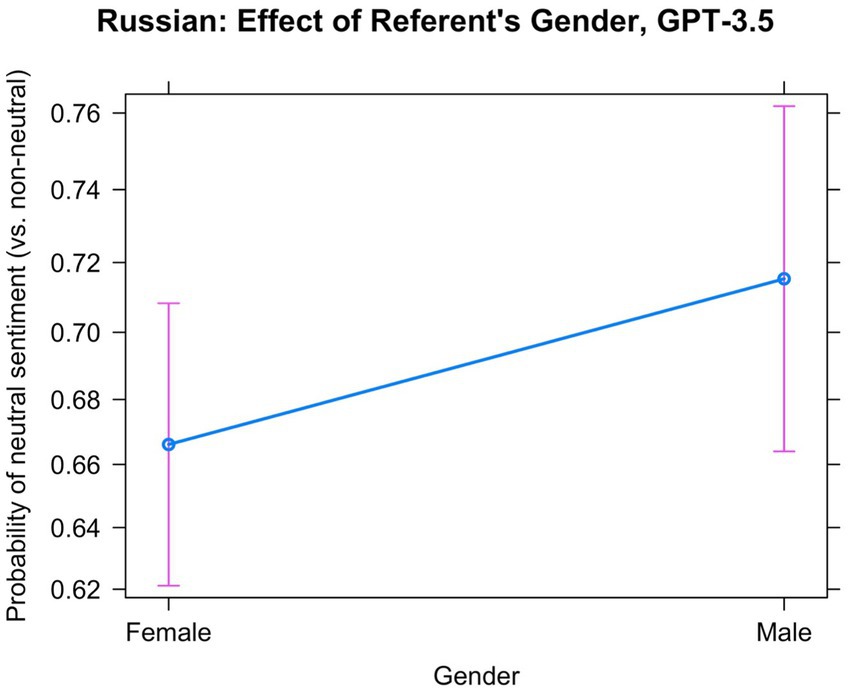
Figure 14. Main effect of the referent’s Gender in the Russian data, GPT-3.5: Neutral vs. non-neutral sentiment.
As for the difference between positive and negative sentiment, the best model included neither the referent’s gender, nor the author’s. They did not play any role. The only significant factor, as shown in Table 15, was Year. The negative coefficient means that the odds of positive sentiment decreased with time, negative sentiment became gradually more likely. The term Number is included, although it was not statistically significant, due to the random slopes for this variable depending on individual Conceptual Pairs.

Table 15. Coefficients and their Wald 95% confidence intervals (in parentheses) of the fixed effects in the Generalized Linear Mixed-effect Model with the response variable “Positive or Negative” based on the Russian data and GPT-3.5.
3.3.2.3 Summary
The Russian data reveal a tendency for male referents to be more often used neutrally than female referents, in both approaches. As for the PyABSA labels, this gender gap increases with time. This interaction is not observed by the GPT-3.5 data, however.
The pejoration hypothesis is supported only marginally: male referents tend to be used in more positive contexts than female referents in restricted situations (female authors and singular forms), and only for the labels provided by PyABSA. No effect of gender is found in the GPT-3.5 data.
Can these differences between the approaches be explained by the different sizes of the datasets used for the PyABSA and GPT-3.5? The answer is positive for the contrast between neutral and non-neutral sentiment. When fitted on the smaller sample, the model predicting PyABSA labels did not support the interaction between Gender and Year anymore. Instead, we observe the same tendency for male referents to get neutral labels more often than for female referents, which was observed in the model based on the GPT-3.5 labels. As for the positive vs. negative sentiment, it is interesting that the PyABSA results hold even on the small sample.
4 Discussion
In our paper we used two methods of Aspect-Based Sentiment Analysis with the help of language models. One employed the software package PyABSA and was based on zero-shot learning, whereas the other used the large language model GPT-3.5 and few-shot learning. We found differences in the results produced by the two approaches, but also many similarities.
The main result of our Aspect-Based Sentiment Analysis is that we do not find a consistent preference for female referents to be associated with more negative sentiment than for male referents. This goes against the view that highlights predominantly derogatory attitudes toward females.
However, some of our models suggest that female terms are on average less often associated with neutral sentiment than their male counterparts, supporting the analysis in Hoyle et al. (2019). This difference is found in the data annotated by PyABSA representing English fiction (only for the singular nouns, though), Chinese news and Russian fiction, and in the Russian data annotated by GPT-3.5. It remains an open question whether the absence of this effect in the English and Chinese GPT-3.5 data has to do with the very high frequency of neutral sentiment in the GPT-3.5 annotations, which makes it more difficult to discover significant effects.
If this bias is real, one could conclude that female humans are provided with more emotionally charged descriptions, positive or negative. It is quite remarkable that the results based on corpora representing three very different cultures and two registers converge in this point. At the same time, contrary to our expectations, we find no diachronic convergence in the sentiment evoked by female and male referents. In contrast, in the Russian data, the gender gap seems to be increasing with time. This can mean several things, in principle. First, it is possible that sexism is so deeply rooted that the recent progress has only affected the surface of our behavior and cognition. Alternatively, we cannot exclude that the text types examined in our study have not caught up yet with the social changes. It would be worthwhile to investigate other text sources and look into older data.
To conclude, our data lend tentative and partial support to Glick and Fiske’s (1996), p. 491 claim that “women have been revered as well as reviled” throughout human history. The deep ambivalence leads to constant fluctuation between hostile sexism and benevolent sexism. This may also have to do with the society’s constant scrutiny and evaluation of women. As Tannen (1993) wrote in her eponymous 1993 essay, “[t]here is no unmarked woman”, in the sense that all choices that women make – be that a hairstyle or choosing the name after marriage – are perceived as marked, or carrying additional social meaning. In our case, we see that a woman is less likely to be described neutrally or unmarked in the emotional sense than a man. Although these findings can be still interpreted as evidence of prejudice, they do not support the idea that the roots of linguistic pejoration toward females have to do with a generally more negative attitude toward female referents, which is not found in our data. The causes of the diachronic processes leading to the semantic biases, which were outlined in the Introduction, should probably be searched for elsewhere.
It is necessary to mention some limitations of our study. First of all, our study is limited to seven pairs of gendered concepts in each of the three languages – a limitation by necessity shared by most of the studies on gender in corpora mentioned in the Introduction. We are also aware that more additional factors need to be controlled for. For example, in the English data we were not able to control for the author’s gender. We hope that follow-up studies will address this issue.
Second, our conclusions are based on pre-trained models for sentiment analysis. In other words, we have not fine-tuned the existing model on fiction and media texts. The feelings expressed about consumer goods and services, which most PyABSA sentiment analysis models are trained on, should be different from the feelings expressed about humans. However, we find partial support of the PyABSA results in the data annotated by GPT-3.5. Our study also supports previous findings based on a different approach and set of words. PyABSA is a smaller and more specialized model than GPT-3, and is likely to pay more attention to shallow linguistic features such as vocabulary use, while GPT-3 has the capability of performing a deeper semantic analysis. Whether it actually does this remains to be seen, as analyzing this in detail is a difficult but interesting question that goes beyond the scope of our work.
Finally, one cannot exclude that the sentiment evaluation of different contexts could be different at the time when the text was written and now. In other words, when we classify sentiment of a sentence published, let us say, in 1970, the model estimates the sentiment from a contemporary language user’s perspective, and not from a perspective of someone who wrote or read this sentence in 1970. Unfortunately, it would be in principle impossible to estimate that sentiment with full certainty in all contexts.
Yet, our results dovetail with the conclusions reported in a recent study by Morehouse et al. (2023), who find that language representations from word embeddings based on different large corpora of English strongly correlate with people’s implicit attitudes toward diverse topics measured experimentally. This correlation is remarkably stable, persisting across two centuries, and being found in different text registers.3 Implicit attitudes are strongly anchored in our culture, and are less malleable than explicit attitudes, which depend on new norms and cultural demands (Ibid.). Their existence below the radar of consciousness can explain, at least partly, why social prejudice, such as the pro-White racial bias in the United States (Payne et al., 2019), is so persistent and difficult to eradicate.
Despite all above-mentioned limitations, we hope that our study opens a new direction for research in diachronic lexical typology and in lexical typology in general. Lexical typology, defined as the “systematic study of cross-linguistic variation in words and vocabularies, i.e., the cross-linguistic and typological branch of lexicology” (Koptjevskaja-Tamm, 2012: 373), has to a large extent ignored the issue of semantic prosody and connotation. Also, while changes in connotations and pejoration/meliorization are frequently discussed in the context of semantic change and lexical replacement in particular languages and richly illustrated in textbooks on historical semantics, these are rarely taken into account in the more systematic comparative research in diachronic lexical typology. The latter instead often focuses on the more “conceptual” side of semantic shifts such as metonymy, metaphor, broadening, etc. (e.g., the contributions in Juvonen and Koptjevskaja-Tamm, 2016, Georgakopoulos and Polis, 2021, but see Vejdemo and Hörberg, 2016 for including arousal in the model predicting the rate of lexical replacement across languages). We look forward toward other lexico-typological studies in which semantic prosody is taken as a noteworthy aspect of comparison.
Data availability statement
The original contributions presented in the study are included in the article/Supplementary material, further inquiries can be directed to the corresponding author.
Author contributions
NL: Conceptualization, Formal analysis, Investigation, Methodology, Project administration, Resources, Validation, Visualization, Writing – original draft, Writing – review & editing. MK: Conceptualization, Project administration, Validation, Writing – original draft, Writing – review & editing. RÖ: Data curation, Methodology, Resources, Software, Validation, Writing – original draft, Writing – review & editing.
Funding
The author(s) declare financial support was received for the research, authorship, and/or publication of this article. Natalia Levshina’s research was partly funded by the Netherlands Organisation for Scientific Research (NWO) under Gravitation grant “Language in Interaction”, grant number 024.001.006. Robert Östling’s research was partly funded by the Swedish Research Agency (VR), grant number 2019-04129.
Conflict of interest
The authors declare that the research was conducted in the absence of any commercial or financial relationships that could be construed as a potential conflict of interest.
Publisher’s note
All claims expressed in this article are solely those of the authors and do not necessarily represent those of their affiliated organizations, or those of the publisher, the editors and the reviewers. Any product that may be evaluated in this article, or claim that may be made by its manufacturer, is not guaranteed or endorsed by the publisher.
Supplementary material
The Supplementary material for this article can be found online at: https://www.frontiersin.org/articles/10.3389/fcomm.2024.1266407/full#supplementary-material
Footnotes
1. ^Online demo: https://huggingface.co/spaces/yangheng/PyABSA-APC; Github repository: https://github.com/yangheng95/PyABSA
2. ^Conceptually, this variable should be treated as random effects, but with the low number of groups (only two), there is no practical difference between treating it as fixed or random effects (Gelman and Hill, 2007, p. 247).
3. ^The conclusion that the same correlation is found across different text registers harmonizes well with our finding that the results for the three languages in our study are very similar, in spite of the fact that the Chinese corpus represents a different text genre than the Russian and the English ones.
References
Borkowska, P., and Kleparski, G. (2007). It befalls words to fall down: pejoration as a type of semantic change. Stud Anglica Resoviensia 47, 33–50.
Brown, T., Mann, B., Ryder, N., Subbiah, M., Kaplan, J. D., Dhariwal, P., et al. (2020). Language models are few-shot learners. Adv. Neural Inf. Proces. Syst. 33, 1877–1901. doi: 10.48550/arXiv.2005.14165
Caldas-Coulthard, C. R., and Moon, R. (2010). ‘Curvy, hunky, kinky’: using corpora as tools for critical analysis. Discourse Soc. 21, 99–133. doi: 10.1177/0957926509353843
Charlesworth, T. E. S., Yang, V., Mann, T. C., Kurdi, B., and Banaji, M. R. (2021). Gender stereotypes in natural language: word Embeddings show robust consistency across Child and Adult language corpora of more than 65 million words. Psychol. Sci. 32, 218–240. doi: 10.1177/0956797620963619
Davies, M. (2010). The Corpus of Historical American English (COHA). Available at: https://www.english-corpora.org/coha/.
DeFranza, D., Mishra, H., and Mishra, A. (2020). How language shapes prejudice against women: an examination across 45 world languages. J. Pers. Soc. Psychol. 119, 7–22. doi: 10.1037/pspa0000188
Dovidio, J. F., Samuel, G. P., and Laurie, A. R. (2005). On the nature of prejudice fifty years after Allport. Malden, MA: Blackwell Publishing.
Fiske, S. T. (2018). Stereotype content: warmth and competence endure. Curr. Dir. Psychol. Sci. 27, 67–73. doi: 10.1177/0963721417738825
Fiske, S. T., Cuddy, A. J. C., Glick, P., and Jun, X. (2002). A model of (often mixed) stereotype content: competence and warmth respectively follow from perceived status and competition. J. Pers. Soc. Psychol. 82, 878–902. doi: 10.1037/0022-3514.82.6.878
Garg, N., Schiebinger, L., Jurafsky, D., and Zou, J. (2018). Word Embeddings quantify 100 years of gender and ethnic stereotypes. Proc. Natl. Acad. Sci. USA 115, E3635–E3644. doi: 10.1073/pnas.1720347115
Gelman, A., and Hill, J. (2007). Data analysis using regression and multilevel/hierarchical models. Cambridge: Cambridge University Press.
Georgakopoulos, T., and Polis, S. (2021). Lexical diachronic semantic maps: mapping the evolution of time-related lexemes. J. Hist. Linguist. 11, 367–420. doi: 10.1075/jhl.19018.geo
Glick, P., and Fiske, S. T. (1996). The ambivalent sexism inventory: differentiating hostile and benevolent sexism. J. Pers. Soc. Psychol. 70, 491–512. doi: 10.1037/0022-3514.70.3.491
Gough, H. G., and Heilbrun, A. B. (1965). The adjective check list manual. Palo Alto, CA: Consulting Psychologists Press.
Grzega, J. (2004). A qualitative and quantitative presentation of the forces for lexemic change in the history of English. Onomasiology Online 51, 1–55.
Herdağdelen, A., and Baroni, M. (2011). Stereotypical gender actions can be extracted from web text. J. Am. Soc. Inf. Sci. Technol. 62, 1741–1749. doi: 10.1002/asi.21579
Hosseini-Asl, E., Liu, W., and Xiong, C. (2022). A generative language model for few-shot aspect-based sentiment analysis. arXiv 2022:5356. doi: 10.48550/arXiv.2204.05356
Hoyle, A. M., Wolf-Sonkin, L., Wallach, H., Augenstein, I., and Cotterell, R. (2019). Unsupervised discovery of gendered language through latent-variable modeling. In: Proceedings of the 57th annual meeting of the Association for Computational Linguistics, pp. Florence, Italy: Association for Computational Linguistics, pp. 1706–1716. Available at: https://www.aclweb.org/anthology/P19-1167.
Jackson, L. M. (2011). Defining prejudice. The psychology of prejudice: From attitudes to social action; the psychology of prejudice: From attitudes to social action. Washington, DC: American Psychological Association, American Psychological Association, pp. 7–28.
Jakobson, R. (1971[1932]). “Zur structur des russischen Verbums,” in Selected writings. Vol. II. Word and Language. ed. R. Jakobson. (Berlin: De Gruyter Mouton), 3–15.
Juvonen, P., and Koptjevskaja-Tamm, M. (2016). The lexical typology of semantic shifts. Cognitive linguistics research. 58. Berlin, New York: De Gruyter Mouton.
Kim, M. (2008). On the semantic derogation of terms for women in Korean, with parallel developments in Chinese and Japanese. Korean Stud. 32, 148–176.
Kleparski, G. (1997). Theory and practice of historical semantics: the case of middle English and Early Modern English synonyms of girl/young women. Lublin: University Press of the Catholic University of Lublin.
Koptjevskaja-Tamm, M. (2012). New directions in lexical typology. Linguistics 50, 373–394. doi: 10.1515/ling-2012-0013
Macalister, J. (2011). Flower-girl and bugler-boy no more: changing gender representation in writing for children. Corpora 6, 25–44. doi: 10.3366/cor.2011.0003
Morehouse, K., Rouduri, V., Cunningham, W., and Charlesworth, T. (2023). Traces of human attitudes in contemporary and historical word embeddings (1800–2000). Preprint (Version 1) Research Square.
Moscovici, S. (1988). Notes towards a description of social representations. Eur. J. Soc. Psychol. 18, 211–250. doi: 10.1002/ejsp.2420180303
Norberg, C. (2016). Naughty boys and sexy girls: the representation of young individuals in a web-based Corpus of English. J. Engl. Linguist. 44, 291–317. doi: 10.1177/0075424216665672
Nosek, B. A., Smyth, F. L., Hansen, J. J., Devos, T., Lindner, N. M., Ranganath, K. A., et al. (2007). Pervasiveness and correlates of implicit attitudes and stereotypes. Eur. Rev. Soc. Psychol. 18, 36–88. doi: 10.1080/10463280701489053
Osgood, C. E., May, W. H., and Miron, M. S. (1975). Cross-cultural universals of affective meaning. Urbana, Illinois: University of Illinois Press.
Ouyang, L., Wu, J., Xu, J., Almeida, D., Wainwright, C., Mishkin, P., et al. (2022). Training language models to follow instructions with human feedback. Adv. Neural Inf. Proces. Syst. 35, 27730–27744. doi: 10.48550/arXiv.2203.02155
Payne, B. K., Vuletich, H. A., and Brown-Iannuzzi, J. L. (2019). Historical roots of implicit bias in slavery. PNAS 116, 11693–11698. doi: 10.1073/pnas.1818816116
Pearce, M. (2008). Investigating the collocational behaviour of man and woman in the BNC using sketch engine. Corpora 3, 1–29. doi: 10.3366/E174950320800004X
Potts, A., and Weare, S. (2018). Mother, monster, Mrs, I: a critical evaluation of gendered naming strategies in English sentencing remarks of women who kill. Int. J. Semiot. Law - Rev. Int. Sémiot. Jurid. 31, 21–52. doi: 10.1007/s11196-017-9523-z
Romaine, S. (2000). Language in society: An introduction to sociolinguistics. 2nd Edn. Oxford: Oxford University Press.
Salmons, J. (1990). “The context of language change,” in Research guide on language change. ed. E. C. Polomé (Berlin, New York: De Gruyter Mouton), 71–96.
Schulz, M. (1975). “The semantic derogation of women,” in Language and sex: difference and dominance. eds. B. Thome and N. Henley (Newbury Hall).
Stern, G. (1931). Meaning and change of meaning, with special reference to the English language. Bloomington, London: Indiana University Press.
Tannen, D. (1993). Wears jump suits. Sensible shoes. Uses husband’s last name. New York Times Magazine. pp. 52–54.
Taylor, C. (2013). Searching for similarity using Corpus-assisted discourse studies. Corpora 8, 81–113. doi: 10.3366/cor.2013.0035
Ullmann, S. (1957). The principles of semantics. 2nd Edn. Glasgow, Oxford: Jackson, Son, and Co., Basil Blackwell.
Vejdemo, S., and Hörberg, T. (2016). Semantic factors predict the rate of lexical replacement of content words. PLoS One 11:e0147924. doi: 10.1371/journal.pone.0147924
Williams, J. E., and Best, D. L. (1990). Measuring sex stereotypes: A multination study. Newbury Park, London: Sage Publications, Inc.
Yang, H., and Li, K. (2023). PyABSA: A modularized framework for reproducible Aspect-Based Sentiment Analysis. arXiv 2023:01368. doi: 10.48550/arXiv.2208.01368
Zasina, A. J. (2019). Gender-specific adjectives in Czech newspapers and magazines. J. Linguist. 70, 299–312. doi: 10.2478/jazcas-2019-0060
Keywords: semantic derogation, pejoration, sentiment analysis, diachronic corpora, semantic change, semantic prosody, gender stereotypes, prejudice
Citation: Levshina N, Koptjevskaja-Tamm M and Östling R (2024) Revered and reviled: a sentiment analysis of female and male referents in three languages. Front. Commun. 9:1266407. doi: 10.3389/fcomm.2024.1266407
Edited by:
Gerd Carling, Goethe University Frankfurt, GermanyReviewed by:
Gisela Redeker, University of Groningen, NetherlandsKate Bellamy, Leiden University, Netherlands
Copyright © 2024 Levshina, Koptjevskaja-Tamm and Östling. This is an open-access article distributed under the terms of the Creative Commons Attribution License (CC BY). The use, distribution or reproduction in other forums is permitted, provided the original author(s) and the copyright owner(s) are credited and that the original publication in this journal is cited, in accordance with accepted academic practice. No use, distribution or reproduction is permitted which does not comply with these terms.
*Correspondence: Maria Koptjevskaja-Tamm, tamm@ling.su.se