- Faculty of Information Technology, Monash University, Clayton, VIC, Australia
Cognitive dysfunction, and the resulting social behaviours, contribute to major social problems, ranging from polarisation to the spread of conspiracy theories. Most previous studies have explored these problems at a specific scale: individual, group, or societal. This study develops a synthesis that links models of cognitive failures at these three scales. First, cognitive limits and innate drives can lead to dysfunctional cognition in individuals. Second, cognitive biases and social effects further influence group behaviour. Third, social networks cause cascading effects that increase the intensity and scale of dysfunctional group behaviour. Advances in communications and information technology, especially the Internet and AI, have exacerbated established problems by accelerating the spread of false beliefs and false interpretations on an unprecedented scale, and have become an enabler for emergent effects hitherto only seen on a smaller scale. Finally, this study explores mechanisms used to manipulate people's beliefs by exploiting these biases and behaviours, notably gaslighting, propaganda, fake news, and promotion of conspiracy theories.
1 Introduction
Human society has long been plagued by dysfunctional behaviours that arise from faulty thinking and social interactions. Some of the most widespread cognitive failures occur within groups of people. Mackay (1841) painted a vivid picture of “popular delusions and the madness of crowds.”
A major social problem of our time is that digital media and Artificial Intelligence (AI) are exacerbating social problems that arise from dysfunctional thinking and perception. In particular, they allow conspiracy theories, and other false belief systems, to spread rapidly and create social problems (Shao et al., 2018; Bovet and Makse, 2019; Treen et al., 2020; Himelein-Wachowiak et al., 2021; Fisher, 2022; Ruffo et al., 2023).
Social media, notably Facebook and Twitter, had an immense impact on public opinion during the “Arab Spring” revolutions (Wolfsfeld et al., 2013). During the Tahrir Square protests, the regime was unable to control the information shared on social media, which played a pivotal role in shaping both the protests and their outcomes (Tufekci and Wilson, 2012). The powerful impact and pervasive influence of social media on public opinion and driving activism has been corroborated by many other researchers (Tufekci, 2017; Bessi and Ferrara, 2016; Zhuravskaya et al., 2020; Gerbaudo, 2012; Lotan et al., 2011). Conversely, Morozov (2011) described how authoritarian regimes exploit social media for distributing malign propaganda, and for monitoring public attitudes and reactions.
Many faults in social cognition and decision-making arise from the ways in which individuals process and apply information about themselves, about other individuals, and about social situations. A gap in our understanding of how major social problems arise lies in the need to identify mechanisms by which failures in cognition translate into social behaviour. This also implies that the need to identify how links occur across scales points to gaps in each of the fields of research involved.
To begin closing the above gaps, this study presents a model to show how cognitive dysfunction and biases in individuals lead to dysfunctional effects within social groups and networks. We then show how modern networking technology magnifies and accelerates these effects, leading to large-scale dysfunctional behaviour (Figure 1). The scales span individuals, small social groups, and very large social groups, which may encompass entire societies. Underlying our model are insights gained from complex network theory and multi-agent models, as well as from research into the effects of modern communications, including social media.
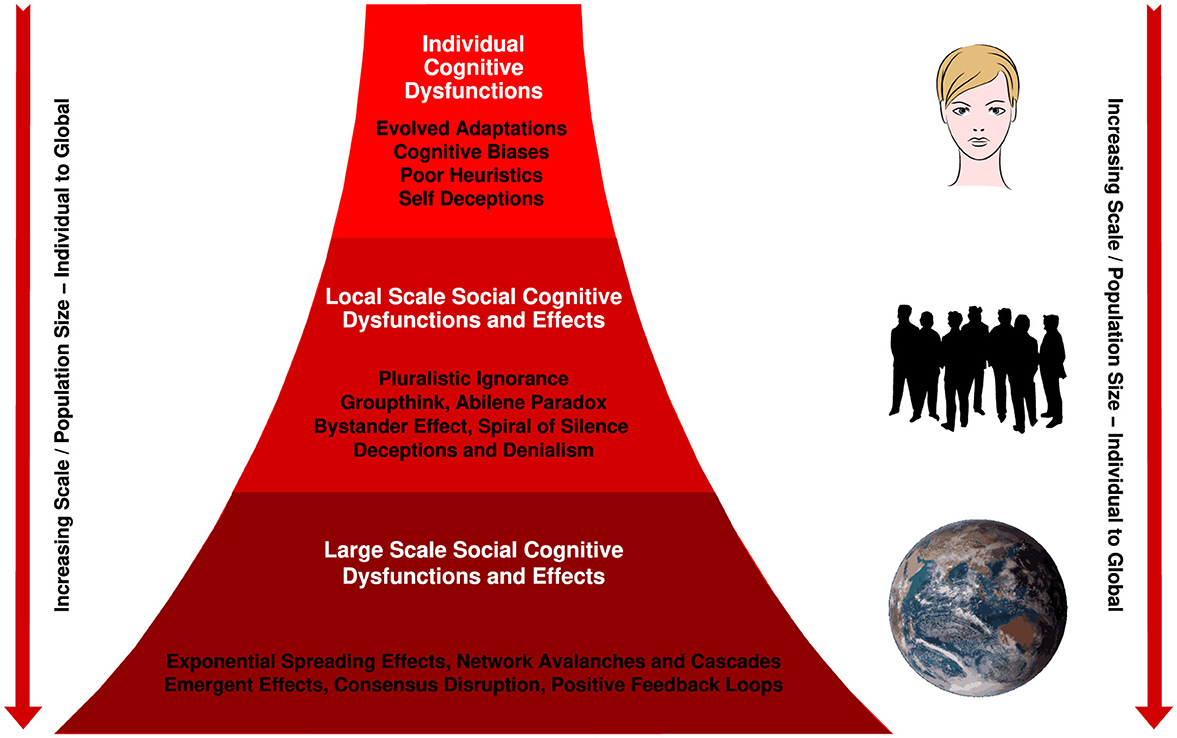
Figure 1. Digital media with their speed and global footprint expand the populations exposed to the damaging social impacts of dysfunctional thinking.
The main points in our argument are as follows:
1. Evolved drives and simplifying mechanisms combine with cognitive limitations, creating the potential for cognitive errors and biases by individuals (Section 2). Combined with cognitive dissonance, they lead to fundamental dysfunctions, notably confidence related biases (Section 2.3), confirmation bias (Section 2.4), false consensus and uniqueness bias (Section 2.5), self-deception (Section 2.6), and denial (Section 2.7).
2. Cognitive dysfunctions spread within groups, especially by peer-to-peer interactions (Section 3). Several mechanisms enable cognitive dysfunctions to propagate across social networks (Section 3.1), including percolation and cascades, and are constrained by network topology. The spread of cognitive dysfunction through social groups leads to malign social effects, such as Pluralistic Ignorance (Section 3.2), groupthink and the Abilene Paradox (Section 3.3).
3. Modern communications, especially digital networks and media, accelerate and expand the spread of false beliefs and interpretations, and enable social cognitive errors to spread across large populations (Section 4). Digital networks enable the emergence of phenomena that were previously not possible, including the exploitation of dysfunctions across large populations (Section 4.1). New technology, especially Artificial Intelligence (AI), can enhance and accelerate adverse impacts produced by digital networks (Section 4.2). These include social harms that emerge by accident such as misinformation, pluralistic ignorance and groupthink, and those that arise by intent through malign exploitation.
4. Biases and cognitive dysfunctions are vulnerabilities that have led to a plethora of methods evolving to permit malign exploitation (Section 5). These range from the distribution of disinformation, misinformation, malinformation, and false interpretations, to gaslighting, propaganda, post-truth, and fake news (Section 5.1), through to denialism, conspiracy theories, and disruptive propaganda (Section 5.2).
In summary, we argue that advances in digital technology and AI permit the formation of immense, highly connected social networks. Together they create conditions for rapid emergence of large-scale, dysfunctional group behaviours and increase the risk of malign exploitation.
2 Cognitive dysfunction and biases
Cognitive limitations, and evolved idiosyncrasies in the way humans process information, underlie social cognitive dysfunctions. In turn, they lead to many of the large scale effects observed in social networks, especially when they are interconnected via digital media.
Here, the term cognitive dysfunction means any loss or failure of ability to perceive or interpret the world in the intended or expected manner. The term cognitive effect means any psychological mechanism that alters a person's cognition and resultant behaviour, thus effecting an internal state change. Cognitive effects include limitations and evolved idiosyncrasies in the way humans process information. Behaviour is defined here to be the reactions of individuals or groups to stimuli that include the social environment as it is perceived, or misperceived.
2.1 Evolutionary origins of cognitive errors and biases
Through evolution, humans are adapted to have drives and behaviours that ensure survival. They include affiliation with a group, status and reproductive success (Maslow, 1943; Kenrick et al., 2010). These deep-seated drives were essential for survival in the environments where they evolved. However, they often lead to dysfunctional cognition and behaviour (Maner and Kenrick, 2010) and are poorly adapted to modern societies (Li et al., 2018; Del Giudice, 2018).
Expanding and updating the work of Maslow (1943), Kenrick et al. (2010) showed that behaviours and adaptions evolved to maximise the probability of genome survival within social groups, a common strategy in many species. Survival in social groups favours adaptations for fast reaction times, as well as social identity maintenance and the imperative to improve status within a group.
When processing many stimuli to interpret and react to a complex situation, a common approach is to simplify the problem by trading away reasoning accuracy for speed (Kahneman and Tversky, 1972; Tversky and Kahneman, 1974; Kahneman, 2011). But mechanisms to simplify complicated problems often lead to cognitive errors. An example is the tendency to ascribe deliberate purpose to unexplained natural events, and to anthropomorphize complex causes behind unpredictable events (Epley et al., 2008). The search for motivated causes is seen in many contexts, such as witch hunts (Mackay, 1841), accident investigations (Green, 2014), and conspiracy theories. It appears to have its origins in our animal roots. Chimpanzees, for instance, rage at storms as though they are confronting rivals (Goodall, 1999).
Below, we discuss the most problematic cognitive effects and biases, and show how these are related to survival imperatives.
2.2 Cognitive limitations
Underlying most dysfunctional cognition and behaviours are deep-rooted mechanisms that are evident both in the way humans process information, and in behaviours (Kenrick et al., 2010; Maner and Kenrick, 2010).
The human brain and senses have limited capacity for interpreting complex information (Miller, 1956; Li et al., 2022), so environmental complexity makes it impossible to analyse every scenario quickly, and in detail. This is especially important when responding to immediate threats to survival. One consequence is that people respond to issues that they can act upon immediately, but often ignore long-term problems that they have not experienced directly, such as climate change or sea-level rise (Graham et al., 2013).
As noted earlier, an important adaptation is to use simplifying mechanisms (Halford et al., 1998). Evolution often favours solutions that are adequate rather than perfect. To cope with cognitive complexity, agents achieve “fast thinking” by using heuristics and other shortcuts that skip over often complex chains of causation (Tversky and Kahneman, 1974, 1983; Kahneman and Tversky, 1996).
Recent work shows that Bayesian-like mechanisms are central to human cognition (Zhong, 2022; Pilgrim et al., 2024). In the Bayesian model, prior knowledge is updated from observed evidence, resulting in the subjective prior beliefs or probability distributions becoming posterior beliefs or probability distributions. As genuine Bayesian inference is cognitively intensive and thus slow, simplified forms of Bayesian inference are now seen as a representative model. While simplification yields a faster result accuracy is sacrificed, therefore evolution for fast reaction times produces an implicit trade-off between accuracy and cognitive load (Griffiths et al., 2008; Bowers and Davis, 2012; Lake et al., 2015).
Heuristic “fast thinking” reasoning mechanisms, often labelled “bounded rationality” or “rules of thumb” can further improve reaction times, but often with further losses in accuracy. Poor choices of heuristics can result in dysfunctional cognition (Tversky and Kahneman, 1983; Gigerenzer, 1991; Verschuere et al., 2023). For instance, Orchinik et al. (2023) found that an adaptive heuristic could be fooled, where false messages were accepted when most messages were truthful, and vice versa. From an early age, children learn to filter random signals and remember contexts with immediate impact. The chief mechanisms are schemas: cognitive frameworks (“recipes”) for interpreting and responding to the environment (Piaget, 2013). Association plays a role in the assimilation of new experiences (Takeuchi et al., 2022), so existing schemas are reinforced and become generalised. Once a context is framed by a schema, heuristics may be used to infer consequences.
When observations of reality are inconsistent with a person's internal beliefs or expectations, Cognitive Dissonance occurs. When confronting cognitive dissonance, individuals seek to avoid the resulting discomfort (Festinger, 1962). They alter either their actions, their beliefs, or their interpretations (Harmon-Jones and Mills, 2019). The resulting inconsistency, and its psychological effects, underpin a number of dysfunctional biases and effects, such as confidence related biases, self-deception, and denial behaviour (all discussed later) (Ramachandran, 1996; McGrath, 2017). Cognitive dissonance is a strong causal factor in confirmation bias (Festinger, 1964; Jonas et al., 2001; Knobloch-Westerwick et al., 2020; Miller and Cabell, 2024).
The faculty for cognitive dissonance, and its avoidance, are evolved survival mechanisms (Egan et al., 2007; Kaaronen, 2018). The ability to recognise and act upon situational changes that could be dangerous aids survival and, acting in the presence of confusing cognitions, may also aid survival (Egan et al., 2007; Harmon-Jones et al., 2017).
2.3 Confidence related biases
A well-studied problem in psychology and other literature is overconfidence, which some consider to be the most important cognitive bias (Kahneman, 2011; Moore, 2018). Moore and Healy (2008) identified three distinct definitions of overconfidence: overestimation of one's own performance (Kruger and Dunning, 1999); overestimation of one's own performance relative to others; and overconfidence in the accuracy of one own beliefs (which they termed “over-precision”). They argued that:
... On difficult tasks, people overestimate their actual performances but also mistakenly believe that they are worse than others; on easy tasks, people underestimate their actual performances but mistakenly believe they are better than others.
Several studies have argued that the overconfidence error is an artefact of human Bayesian-like belief updating when information is uncertain (Griffiths and Tenenbaum, 2006; Griffiths et al., 2008; Moore and Healy, 2008).
Overconfidence biases can explain why agents over or underestimate group opinions, and act so readily on these false perceptions of reality. They also explain how groupthink (see later) leads to wrong decisions being made with high confidence, without assessing risk, and without reviewing alternatives. In both cases, individuals fail to recognise their incompetence to perform the task and actively exacerbate a dysfunctional group interaction. Similarly, where the Dunning-Kruger effect (see later) is in play, cognitive dissonance may not arise if the afflicted individual is unable to apprehend differences between observations and expectations of reality.
Misapprehensions of own competence can manifest in other forms. A notable example is Illusory Superiority (Taylor and Brown, 1988), a cognitive bias in which people assume superiority, leading to dysfunctional behaviours that make them feel stronger. One result is that trying to argue against people's entrenched ideas can have the opposite effect of reinforcing them (Nyhan and Reifler, 2010; Nyhan, 2021).
Where manifested this problem may be exacerbated by the Echo Chamber Effect (see later), where repeated exposure to beliefs that are coherent with prior beliefs further reinforces them (Del Vicario et al., 2016a; Cinelli et al., 2021).
Using measurements of neural activity, Rollwage et al. (2020) showed that choices made with a high confidence level lead to integration of evidence that confirms the choice, unlike evidence disconfirming the choice. This shows a causal relationship between confidence related biases, and confirmation bias, discussed next.
2.4 Confirmation bias
Confirmation bias is perhaps the most problematic inferential error in human reasoning (Greenwald, 1980; Evans, 1989). Individuals experiencing confirmation bias inadvertently collect evidence selectively, accepting evidence and opinions that support their prior beliefs, and rejecting opposing evidence (Taber and Lodge, 2006). They also tend to overvalue arguments that are consistent with their prior beliefs (Nir, 2011). Avoidance of cognitive dissonance often motivates this bias (Festinger, 1957). The selection is unwitting, in contrast to litigants deliberately selecting supporting evidence to build a case (Nickerson, 1998).
Zhong (2022) argues that choices coherent with prior beliefs are optimal, thus showing that confirmation bias is survival strategy that optimises both time and resources (Dorst, 2020; Page, 2023). This is coherent with recent work, which argues that Bayesian-like belief updating underpins confirmation bias, as the high resource demands of genuine Bayesian updating force the use of approximations (Pilgrim et al., 2024).
Both conclusions align with the observation that evolution often favours solutions that minimise resource expenditures, and provide a mathematical explanation for why confirmation bias is an evolved cognitive feature.
One consequence of confirmation bias is motivated cognition. That is, prior beliefs or predispositions motivate individuals to process information and evidence in a prejudiced manner (Strickland et al., 2011). They evaluate information positively if it is consistent with their values, but reject or demean conflicting information (Eagly and Chaiken, 1993). This behaviour does not appear to depend on cognitive ability, and measuring it has presented challenges (Stagnaro et al., 2023; Tappin et al., 2021).
Another consequence is selective exposure (Stroud, 2011). Individuals selectively gather information that resonates with their attitudes and helps them to arrive at a desired conclusion, which may not be factual (Kunda, 1990). Selective exposure leads to avoiding sources of contradictory evidence, thus reinforcing their existing attitudes, values, beliefs and predispositions (Iyengar and Hahn, 2009).
Individuals also attempt to maintain an “illusion of objectivity” by establishing pseudo-rational justifications that support their predispositions. In this way, they convince themselves that the process was fair and judicious (Kunda, 1990). This is clearly an instance of self-deception (see Section 2.6), wherein individuals deceive themselves. This bias can have lethal consequences (Kassin et al., 2013; Holstein, 1985; Norman et al., 2017).
2.5 False consensus and false uniqueness bias
Under false consensus bias, an individual's perception of group preferences becomes biased by their own preferences. They tend to overestimate support for their own views. Similarity between self and others is more readily accessed from memory than dissimilarity, thus creating an illusion of consensus on the individual's preferred position (Ross et al., 1977).
Individuals under false consensus bias are prone to interact with like-minded people. These interactions can produce an “Echo Chamber Effect” (Del Vicario et al., 2016a; Cinelli et al., 2021) and shape their perception about social preferences (Fiske and Taylor, 1991). An alternative explanation is that people exaggerate support for their preferred position because they focus on their own choice, rather than alternatives (Marks and Miller, 1987).
A commonly seen shortcut in evaluating multiple sources of information is “correlation neglect” where multiple repeated accounts from a single source are considered to be independently sourced. This can contribute to confirmation bias and false consensus bias by adding undue weight to propositions that are being widely repeated (Enke and Zimmermann, 2017; Bowen et al., 2021).
The opposite bias is false uniqueness bias where individuals mistakenly underestimate the prevalence of their own attitudes. That is, they perceive themselves to be almost unique, when they are not (Suls and Wan, 1987). A person's perception of their uniqueness can be either negative or positive, but generally this bias refers to individuals' tendency to see themselves as superior to others on desirable attributes and behaviours (Goethals et al., 1991; Marks and Miller, 1987).
2.6 Deception and self-deception
Deception evolved as a means to gain a survival advantage and is widespread in nature (Trivers, 2000). The mechanics of how deceptions work have been well studied empirically, and more recently quantitatively, and can be accurately modelled using information theory (Figure 2).
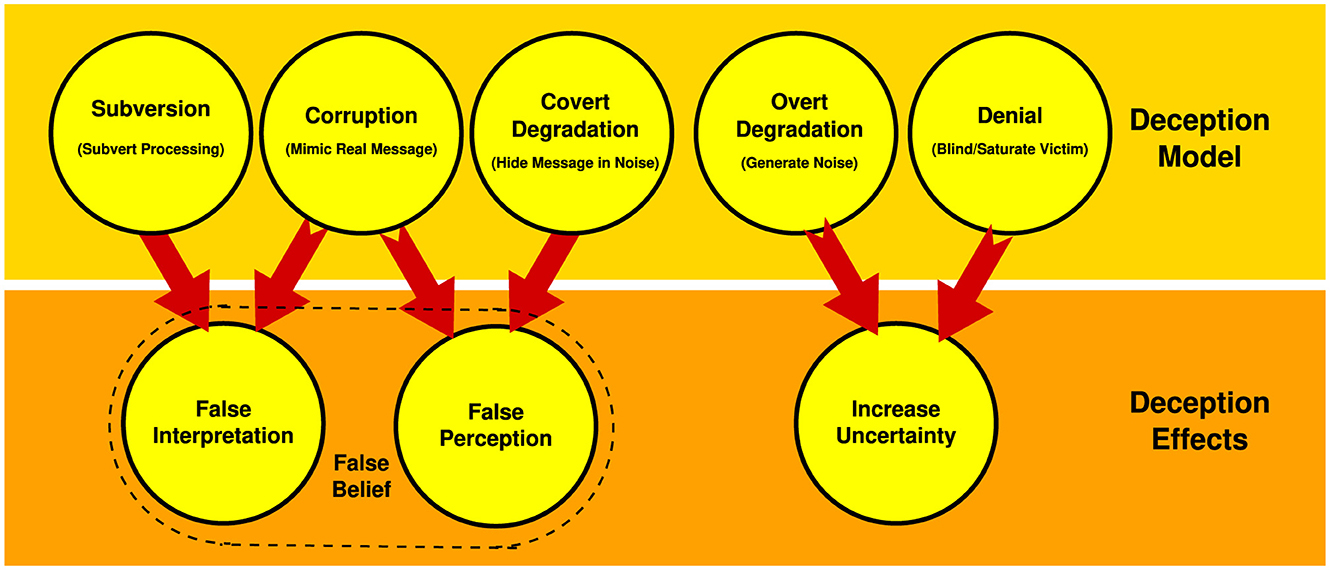
Figure 2. Information theoretic models of deception and their effects. The two prevalent deceptions in individual behaviour are degradation, where actual opinions are concealed in some fashion, and corruption, where the opinion of others is mimicked. Less frequent are denial, where the information channel is blocked, or subversion where interpretation is manipulated. The respective effects are false beliefs, or reduced confidence in true beliefs. Compound deceptions are common, mixing deception types to implant false perceptions and interpretations (Kopp et al., 2018).
Deceptive behaviours are implicit in most of the dysfunctional social behaviours explored in this paper (Kalbfleisch and Docan-Morgan, 2019). They occur when individuals create false beliefs in the minds of others by hiding or misrepresenting their actual agendas, motives, feelings, or opinions.
The four basic deception types, degradation, corruption, denial, and subversion are defined in (Kopp et al., 2018). Of these, the prevalent types in individual behaviour are degradation, where actual beliefs are concealed in some manner, and corruption, where the beliefs of others are mimicked. Individuals or groups attempting to exploit dysfunctional thinking will often employ subversion and compound deceptions, mixing deception types to implant false perceptions and interpretations (Kopp et al., 2018).
If we accept the Bayesian-like model of cognition, these four deception types target the production of a posterior belief from a prior belief. Where a prior belief is true, this is done by introducing false data or altering how true data is interpreted. Where a prior is already a false belief, this is done by reinforcing it with more false data, or by reinforcing a false interpretation with further interpretations coherent with it (Haswell, 1985; Brumley et al., 2005). Success or failure of any specific deception will depend upon the specific cognitive vulnerabilities of the targeted individual or population. Any cognitive faculty or behaviour that leads to a failure to detect and reject a deception is a vulnerability.
Deceptive behaviour is a characteristic feature of pluralistic ignorance (Section 3.2) and is often seen in groupthink (Section 3.3), where false beliefs are not internalised. However, self-deception predominates in other dysfunctional behaviours.
Three hypotheses have been proposed to explain this behaviour. Trivers (2000) argues that self-deception is an evolved function to improve an individual's ability to deceive others, while Ramachandran (1996) argues that individuals will self-deceive to avoid cognitive dissonance (Harmon-Jones and Mills, 2019). Jian et al. (2019) have shown that self-deception increases with cognitive load, as defined by Sweller (1988). This could be explained as a mechanism to reduce cognitive load and cognitive dissonance when faced with uncertainty (Kaaronen, 2018). Intentional deceivers may also become self-deceivers by believing their own falsehoods (Li, 2015). These hypotheses are not mutually exclusive. An individual internalising false beliefs can be concurrently avoiding cognitive dissonance, while also improving their ability to deceive others about their actual motivations and agendas (von Hippel and Trivers, 2011).
2.7 Denial and denialism
Bardon (2019) argues that denial and denialism are instances of motivated cognition, described as the “unconscious tendency of individuals to process information in a manner that suits some end or goal extrinsic to the formation of accurate beliefs” (Kahan, 2011). Whereas denial can be confined to a single unpalatable truth, denialism “... represents the transformation of the everyday practise of denial into a whole new way of seeing the world ...” (Kahan, 2011; Bardon, 2019). Varki (2009) argued that denial behaviours evolved to gain a survival advantage, but with a basis different from the hypothesis argued by Trivers (2000). Both hypotheses are strongly coherent with the finding by Brumley (2014) that misperception of reality can provide an evolutionary advantage.
The mechanisms that underpin denial and denialism include cognitive dissonance, the cognitive biases described above, and self-deceptions (Bardon, 2019). One driving motivation is avoidance of grief, denial being the first of the five stages in internally coping with unwanted objective truths (Kübler-Ross et al., 1972).
It is evident that self-deceptions related to denial mostly comprise denial and degradation, especially where inputs are rejected, corruption where false perceptions are accepted, and subversion where false interpretations and rationalisations are accepted. Compound self-deceptions, combining all four deceptions, are often employed (Brumley, 2014).
Both denial and denialism are important enablers of exploitation (Section 5). Notably, the contemporary digital environment characterised by ‘information overload' creates conditions highly favourable for exploitation, facilitating deception and increasing susceptibility to self-deception (Kahneman, 2011).
3 Cognitive dysfunction in groups
Interactions between individuals in social groups require communication. This results in the formation of social networks, which allow false beliefs and interpretations to propagate across the group. Properties of these networks therefore have important implications for how some social cognitive dysfunctions arise and propagate.
To explain this, we first explore social networks (Section 3.1), as distinct from digital networks providing connectivity for social networks (Section 4). We then explore the best-known group cognitive dysfunctions: pluralistic ignorance (Section 3.2), groupthink and the Abilene paradox (Section 3.3). While polarisation shares the emergent property with these cognitive dysfunctions, it is a behavioural effect rather than dysfunction (Smith et al., 2024).
3.1 Propagation of beliefs across social networks
The term social network refers here to any set of individuals who interact with one another. Ideas and beliefs typically spread across a social network via local interactions between individuals (Smith et al., 2020). Studies using agent-based models show that consensus can emerge within networks by peer-to-peer interaction (Green, 2014; Flache et al., 2017; Stocker et al., 2001; Tang and Chorus, 2019; Seeme, 2020). In contrast, social fragmentation and thus lack of consensus typically occurs when networks exceed a critical size. Even a small group propagating false beliefs can disrupt consensus forming in a large population (Dunbar, 1995; Iñiguez et al., 2014; Kopp et al., 2018; Smith et al., 2020), and spread dysfunctional thinking throughout a social network (Seeme, 2020).
Social networks have long been studied as graphs (Barnes, 1969; Scott, 2012), in which individuals are “nodes” and relationships are “edges.” Graphs exhibit well-known topologies (Figure 3), which are patterns formed by their nodes and edges. In social networks, topologies are important for opinion formation (Burt, 1987; Choi et al., 2010; Shaw-Ching Liu et al., 2005). For instance, hierarchical organisations form tree structures (Figure 3a), in which influence often flows down. A tribal leader or company CEO would be represented by a root node in the topology of a hierarchy.
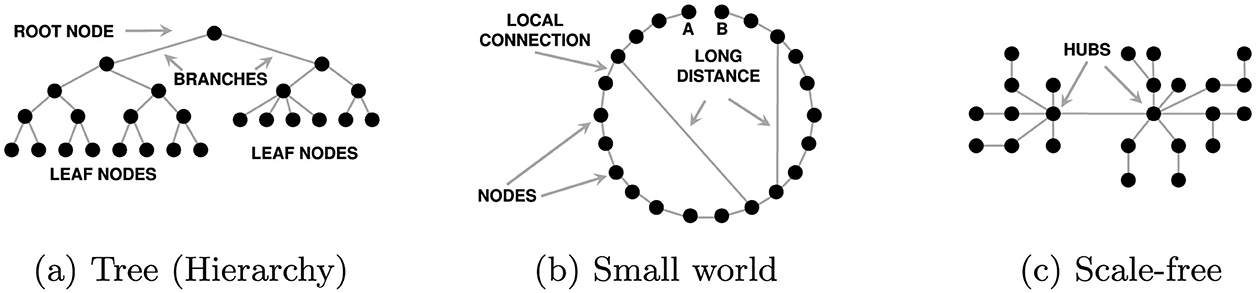
Figure 3. Common topologies in social networks. Here, circles denote nodes (individuals) and lines denote edges (links between pairs of individuals). The edges drawn here are undirected, but social interactions and influence are sometimes one-directional. (a) A hierarchy (tree graph) is common in organisations. It contains a root node and no cycles. For instance, in a typical company, the CEO is the root node and the leaf nodes are employees. (b) Small worlds are common in traditional societies. Most people interact only with others in nearby communities, shown here as local connections. However, some people travel between communities making long distance connections. Long-distance connections reduce the distance between individuals. In this example, they reduce the number of steps between A and B from 21 to 9. (c) Scale-free networks are common in online social groups. The degree of nodes (number of edges linking them to others) follows a power law and the network contains hubs of highly connected nodes. In a typical online social network, influencers would be the hubs, and their followers are the other nodes.
In traditional societies, interactions between individuals are limited by distance, so they typically form Small-Worlds (Watts and Strogatz, 1998), and long-distance travellers spread ideas between communities (Figure 3b). In small world networks, there is a critical level of connectivity, beyond which opinion drifts and a single extreme appears (Amblard and Deffuant, 2004). Long-range ties potentially connect highly dissimilar local regions or individuals, and thus can trigger repulsive influence (Flache and Macy, 2011).
The advent of online social media has enabled the formation of huge online communities. These commonly take the form of Scale-Free Networks (Barabási and Albert, 1999) (Figure 3c). That is, the distribution of the number of agents connected to an individual follows a power law. This tendency of on-line communities to form scale-free networks led to the rise of “influencers,” highly connected and trusted individuals, who are very effective at spreading ideas and opinions (Bakshy et al., 2011).
Studies of network resilience have found that scale-free networks are usually robust to random “attacks,” but can be susceptible to targeted attacks that remove hubs (Albert et al., 2000; Artime et al., 2024). This suggests that “deplatforming” influencers could be an effective way to disrupt the spread of toxic ideas from online social networks (Section 7). Classical epidemiological studies show the strong effects arising from removal of highly connected individuals in scale free networks (Keeling and Eames, 2005).
Although influencers are diffusive, they are not as persuasive as individuals with closely knitted bonds, such as friends or relatives. Rumours survive longer and reach a larger population in a randomly growing network compared to a scale-free network (Zhou et al., 2007).
In any society, individual agents are often members of all three of the above network types (and others) at different times and places. For instance, they may be part of a hierarchy in their workplace, a small world in their home or neighbourhood, and a scale-free network when using social media. In this way, individuals create overlaps between different social networks, which form a “Network of Networks” (Gao et al., 2014).
The connections between different networks are often tenuous and have no effect under normal conditions. However, an individual will sometimes relay an idea from one network into another network. In this way, an idea can be transmitted from network to network, creating a cascade in which the idea spreads across the entire society (Figure 4). This model is equally true of foot traffic between villages and online networks that encompass the globe. It shows how unexpected inputs from outside can invade and disrupt a network.
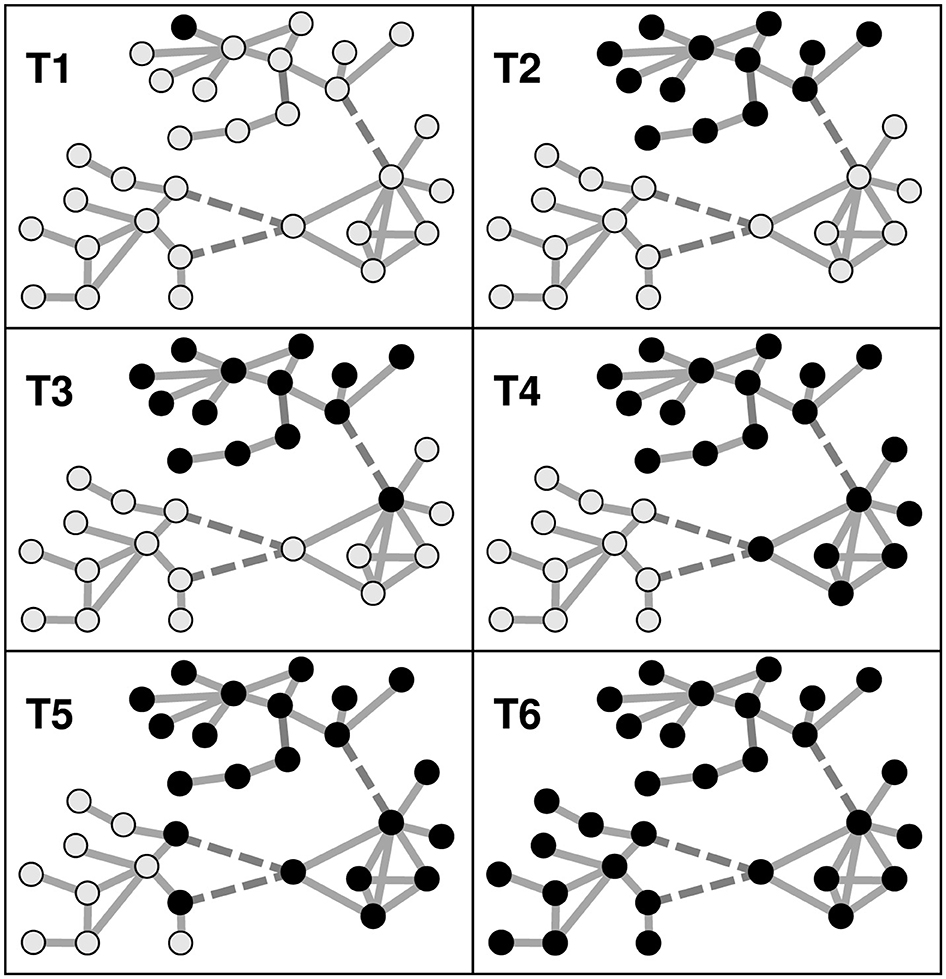
Figure 4. In this hypothetical example, a belief cascades, spreading like an epidemic across three separate social networks. The circular dots denote people and the shading denotes two different beliefs. Lines between dots denote social relationships between pairs of individuals. Dashed lines denote intermittent social relationships between individuals in different networks. The boxes (T1–T6) show the state of the community at different times. Initially (T1), a new belief (coloured in black) is held by a single individual, but quickly spreads to all others in the same network (T2). It then infiltrates another network (T3). The same pattern repeats (T3 and T4, T5 and T6) until the belief has spread to all individuals in the community. In traditional societies, the links between networks may be infrequent, requiring (say) physical movement of people from one community to another. However, in online social networks, they depend only on digital links and can be extremely fast.
The more complex and interlinked different networks are, the more susceptible they are to invasion by alien ideas and beliefs (Pastor-Satorras et al., 2015; Jalili and Perc, 2017). The complexity of overlapping social networks allows unintended consequences to emerge (Green, 2014). These can bedevil attempts to mitigate the spread of dysfunctional thinking. Connections between different networks can enable beliefs and behaviours to emerge and cascade until they become widespread (Paperin et al., 2011; Hinds et al., 2013; Tsugawa and Ohsaki, 2014; Dumitrescu et al., 2017; Shao et al., 2018). For example, after being exposed to extremist views on social media, an individual might spread them within a family or neighbourhood.
Ideas spread across, and between, networks in several ways. These include: avalanches, sudden changes in network connectivity (Paperin et al., 2011); cascades, in which a state change spreads across a system from network to network (Bikhchandani et al., 1992); and positive feedback, in which local variations grow into global patterns (Green, 2014). Network processes also mean that a small change in some widely-shared cognitive bias or dysfunction can have a massive impact on large-scale social behaviour (Green, 2014). Rafail et al. (2024) showed how feedback loops contribute to polarisation.
The complexity of overlapping social networks creates a rich array of communication pathways, which increase the likelihood of unanticipated consequences (Merton, 1936). These occur in many contexts, including accidents (Rijpma, 2019), side effects of innovations, especially new technology (Green, 2014), and cascading failures of infrastructure (Valdez et al., 2020).
The phenomenon is explained by Complexity Theory. Complex systems are composed of “agents” (in social terms, these are individual members of a group) which interact with other agents. In a poorly connected system, the agents form small isolated groups. However, as the richness of connections between agents increases, a “connectivity avalanche” occurs (Paperin et al., 2011). This avalanche causes a phase change in the network, from fragmented to connected. Instead of small, isolated groups of agents, large components emerge in which pathways of connections exist between every pair of agents. The behaviour of such a system is chaotic, which leads to unpredictable outcomes and unanticipated consequences.
3.1.1 Emergence in social networks
The advent of digital networks providing local or global connectivity between individuals has produced profound impacts. The geographical and temporal bounds on social networks that characterised human societies for millennia vanished in less than a decade. Individuals can connect locally or globally in mere seconds. This has produced opportunities for emergent behaviours to arise more frequently.
The topology of social networks emerges through behaviour of the agents involved (Wu et al., 2015; Ubaldi et al., 2021). As we saw above, trees, small-worlds and scale-free networks are well-known examples. Conversely, the topology of a social networks can affect collective cognition and behaviour, such as the formation of beliefs and norms (Momennejad, 2022). Consensus is easier to achieve in some topologies than others (Baronchelli, 2018), and changes in topology, such as a shift in centrality, can lead to emergence of new behaviours (Gower-Winter and Nitschke, 2023).
Instances of emergent behaviour in social networks are Janis' groupthink and pluralistic ignorance, detailed below (Janis, 1972; Miller and McFarland, 1987). The implicit and common antecedent for both social cognitive dysfunctions is connectivity through a social network (Heylighen, 2013; Seeme and Green, 2016). The emergence of both behaviours in small social networks connected by word of mouth, print or digital media is well studied (Schafer and Crichlow, 1996; Mendes et al., 2017).
Ruffo et al. (2023) found that polarisation, where a population divides into groups with mutually opposed viewpoints that are often impossible to reconcile, exhibited emergent properties. Confirmation bias and Bayesian reasoning have been found to be causal factors in the emergence of polarisation (Jern et al., 2014; Del Vicario et al., 2016b; Lefebvre et al., 2024).
There is a wealth of literature on emergent behaviour in very large networks (Green, 2014, 2023), but little on emergent behaviour in large, digitally connected social networks (Ubaldi et al., 2021). These implicitly allow for much larger populations to become captured by social cognitive dysfunctions like groupthink and pluralistic ignorance. More importantly, the large footprints of such networks allow for populations of individuals who meet antecedent criteria to connect, and for new groups to emerge, captured by such dysfunctions.
3.2 Pluralistic ignorance
Pluralistic Ignorance (Katz et al., 1931) is a social cognitive error where:
“..virtually every member of a group or society privately rejects a belief, opinion, or practise, yet believes that virtually every other member privately accepts it.” (Prentice and Miller, 1996)
It is a group-level phenomenon (Sargent and Newman, 2021) that stems from a shared misperception among group members that gives rise to a collective error about the true opinion(s) of their peers (Miller, 2023; Kitts, 2003). O'Gorman (1988) argued that pluralistic ignorance involves two social cognitive errors. First, individuals believe that others hold a different opinion; or they believe that they can assess others' opinions accurately.
Pluralistic ignorance appears in many forms, depending on the context, and leads to various social problems. It occurs most often in situations where people share their views about a collective position (Prentice and Miller, 1993). Pluralistic ignorance has negative impacts on health issues, education and workplace environment (Dunning et al., 2004). It also contributes to a host of social issues. These include alcohol consumption among college students (Prentice and Miller, 1993), attitudes towards dating and sex (Reiber and Garcia, 2010; van de Bongardt et al., 2015), gender bias amongst military cadets (Do et al., 2013), impediments to gender equality (Croft et al., 2021), academic underperformance by student athletes (Levine et al., 2014), avoidance of mental health services by police (Karaffa and Koch, 2016) and inaction on climate change (Geiger and Swim, 2016).
Pluralistic ignorance is usually caused by fear of rejection (Miller and McFarland, 1987, 1991), by following the herd, or by a desire to maintain group identity (Miller and Nelson, 2002). Pluralistic ignorance can lead to unpopular social norms (Prentice and Miller, 1996, 1993) and can manifest itself as other instances of dysfunctional behaviour, such as the bystander effect and the spiral of silence.
Mendes et al. (2017) found that false consensus and exclusivity biases, group polarisation, and social identity maintenance were common antecedents of pluralistic ignorance.
3.2.1 The bystander effect
The bystander effect occurs when people in groups merely observe a situation and fail to take action where they should. Despite being concerned about the victim, individuals fail to assess the need for intervention because other bystanders are also waiting for everyone else to take actions (Latané and Nida, 1981). There are many documented cases of passive bystanders witnessing violent crimes, life threatening emergencies (Banyard, 2011; Schwartz and Gottlieb, 1976; McMahon, 2015), victimisation and bullying, both in real life and online (Bauman et al., 2020; Song and Oh, 2017; Machackova et al., 2015).
The familiar pattern of pluralistic ignorance is evident in this effect. Bystanders are concerned about the victim, but wait for others to intervene. They interpret others' inaction as a sign of the situation not calling for an intervention (Miller, 2023).
3.2.2 The spiral of silence
The perception that one's opinion is counter to the popular stance on an issue inhibits one's willingness to express that opinion, leading to even less visible support for it (Koriat et al., 2016).
Examples include legal stances on abortion, preferences for a political party in national elections, addressing racial inequality (Noelle-Neumann, 1993; Moy et al., 2001; Geiger and Swim, 2016), and suppression of antiwar sentiment (Mueller, 1993).
In contrast, the perception that one's opinion is popular creates the opposite effect. Vocal expression on the one side and silence on the other side creates a “spiral of silence” on the muted topic (Noelle-Neumann, 1993).
Some studies suggest that the spiral of silence theory addresses the impact of pluralistic ignorance on public disclosure (Noelle-Neumann, 1993; Taylor, 1982). The individual misperception that their opinion is not shared by others leads to the collective error of a silent majority being suppressed by a vocal minority (Miller, 2023). The spiral of silence in social media can suppress free expression of minority opinion (Hampton et al., 2014; Gearhart and Zhang, 2015).
3.3 Groupthink and the Abilene paradox
Groupthink (Janis, 1972) is a dysfunction of group decision-making. It occurs when members of a group collectively strive to achieve consensus, even if it means ignoring their own individual views, and fail to assess alternative courses of action realistically. It leads to poor decisions, often with disastrous outcomes.
Groupthink is notorious for leading to poor decision-making and catastrophic outcomes. Infamous examples in American history include the failure to anticipate the attack on Pearl Harbour in 1941, the failed invasion of the Bay of Pigs in 1961, the escalation of the Vietnam War, NASA' s decision to launch the doomed Challenger Space Shuttle, and the Watergate cover-up (Janis, 1982). Groupthink can also lead to unethical practises within organisations (Sims, 1992) and the motivation to acquire or maintain political power may produce Groupthink in government organisations (Kramer, 1998).
Based on historic case studies of failed decisions, Janis (1982) proposed several antecedents to groupthink, including intense group cohesion, avoiding conflicts, insulation from outside influence, and external threats. A revised model of groupthink (Turner and Pratkanis, 1998) suggests that the necessary conditions are strong cohesion among the group as a social entity, and defending against a collective threat aimed at their shared positive image of the group.
Analysing many instances of groupthink, Schafer and Crichlow (1996) found that the dominant antecedents were “leadership style and patterns of group conduct,” while Turner et al. (1992); Turner and Pratkanis (1998) found that social identity maintenance was also a major factor.
In contrast, the Abilene paradox (Harvey, 1988) refers to a more passive group behaviour without any collective threat or strong group cohesion (Kim, 2001). It occurs when members of a group each make a decision that is counter to everyone's preferences, because each member seeks to avoid conflict with the group under a shared misperception of collective agreement (McAvoy and Butler, 2007; Rubin and Dierdorff, 2011).
3.4 Relationships between biases and dysfunctional group behaviours
It is apparent from the preceding discussion that many links exist between the biases and dysfunctional behaviours discussed above (Figure 5). In many cases, a prominent imperative is wanting to belong to the group (Section 2.1), which is a powerful survival imperative in humans (Brewer and Caporael, 2006). It motivates behaviours such as hiding, suppressing or misrepresenting one's real views (Cialdini and Goldstein, 2004).
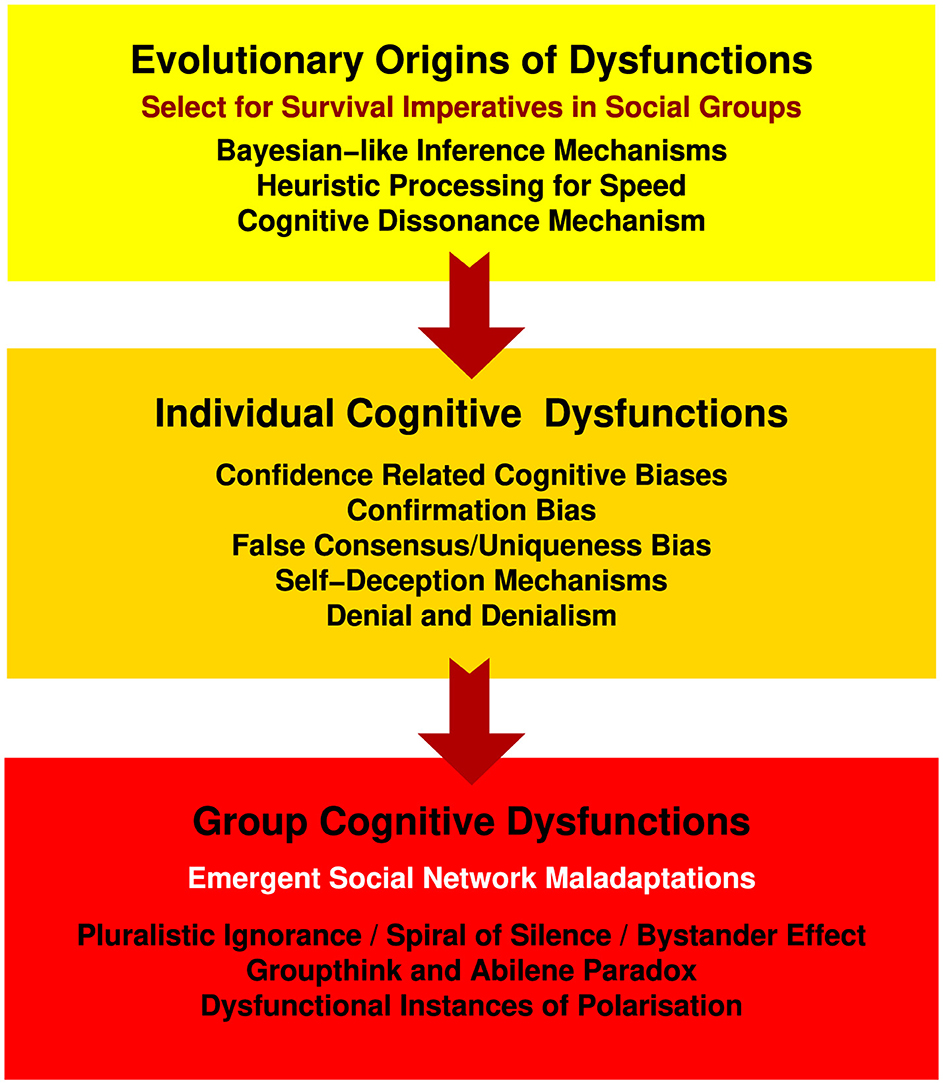
Figure 5. A model for dysfunctional cognition in groups connected by social networks. Evolved limitations result in a number of individual cognitive dysfunctions. When interacting with groups, individual cognitive dysfunctions can result in social cognitive dysfunctions. As the model shows, inherited traits and cognitive mechanisms underlie cognitive biases, which in turn drive cognitive dysfunctions within social groups. This implies that reducing dysfunctions that arise at fundamental levels can help prevent dysfunctions within social groups.
Fear of isolation or rejection from the group is the primary causal driver for many behaviours, e.g., groupthink, Abilene paradox, pluralistic ignorance (Kim, 2001), and spiral of silence. Some studies suggest that the spiral of silence theory addresses the impact of pluralistic ignorance on public disclosure (Noelle-Neumann, 1993; Taylor, 1982). Spiral of silence occurs because individuals misperceive public support for their true opinion, express a different opinion to conform to their perceived majority, which is characteristic of pluralistic ignorance.
The delusion that one's beliefs are unique and different from others is common to both false uniqueness (Section 2.5) and pluralistic ignorance. However, false uniqueness is an individual bias that may be motivated by a false sense of superiority that is not linked with group dynamics, unlike pluralistic ignorance. Similarly, individuals cannot collectively experience false uniqueness bias, whereas pluralistic ignorance does not refer to a single individual's misperception (Miller, 2023).
Mendes et al. (2017) found that the specific antecedents of pluralistic ignorance depended on whether it was defined as perceptual or inferential pluralistic ignorance, and identified both false uniqueness and false consensus biases as antecedents across the literature, while Sargent and Newman (2021) argue that the former is an antecedent.
Janis (1971) cites as antecedents a multiplicity of instances of confirmation bias in groupthink, while Schafer and Crichlow (1996) found that confidence related biases were antecedents, leading to multiple types of cognitive error in group decisions.
4 Adverse impacts of advancing technologies
Problems now arising from digital technology will be further exacerbated as the technology evolves and creates more opportunities for exploitation. Historically, new communication technologies always change the way ideas spread. For instance, Gutenberg's printing press was used heavily to distribute propaganda, an effect later observed with mass media broadcasts (Hoff, 1990; Dewar, 2000; Bagchi, 2016). The observed adverse impacts of networking technology will be amplified by the rapid evolution and adoption of Artificial Intelligence (AI), which can be used to automate and accelerate many tasks that produce malign effects (Honigberg, 2022).
4.1 Impacts of digital networking technology
Digital networking technology contributes to malign exploitation by providing increasingly pervasive, dense and fast connectivity in social networks. The cause is exponential performance growth, that multiplies performance and reduces costs over time (Kopp, 2000; Cherry, 2004).
Faster and cheaper networking technology results in ever expanding geographical coverage, and thus ever increasing exposure of a global audience to potentially malign exploitation. This problem is exacerbated by the ability of digital media to migrate digital content rapidly between media types, resulting in a complex, and sometimes chaotic, unstructured, and random mesh of connections that propagate messages across different media types (Figure 6).
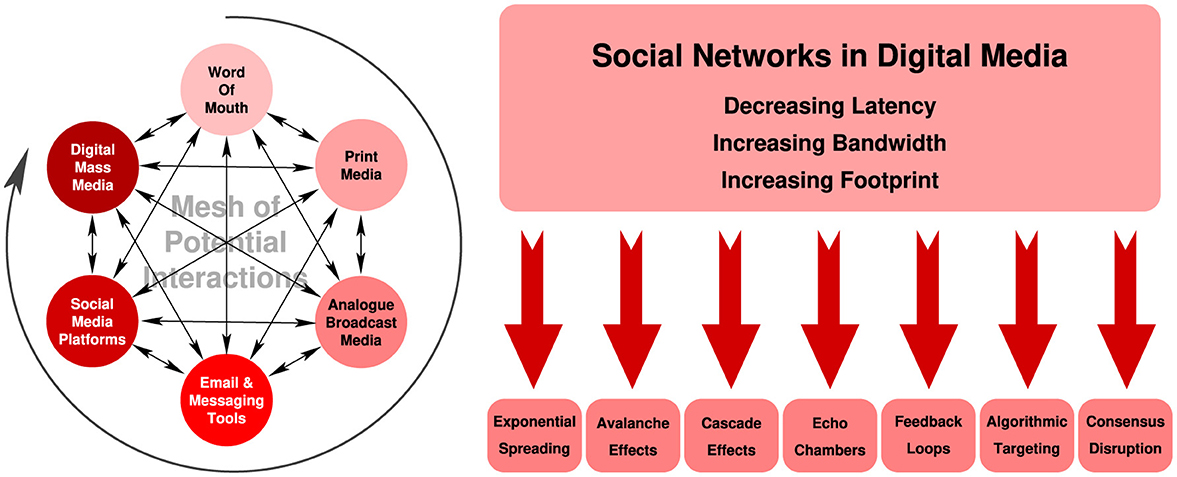
Figure 6. Left: Digital media permit content to migrate quickly between different channels, resulting in high connectivity within and between different media. Social networks residing in digital media display shorter latencies in message transmission, vastly greater footprints and connectivity, and more bandwidth than social networks predating the digital age. Right: The effects of dysfunctional social cognition are accelerated in time across orders of magnitude larger populations. Many features of digital media create effects unseen in the analogue era (Kopp, 2024).
The potentially very high density of very fast long-distance interconnections in social networks that are formed across digital networks has important implications (Section 3.1), which will be become worse as the technology evolves.
Spreading behaviour in large, online social networks, for instance X/Twitter, frequently follows epidemiological patterns observed with biological pathogens. Both measurements and simulations of spread display remarkably good fits to traditional compartment models used in epidemiology (Keeling and Eames, 2005; Brauer, 2008; Kopp et al., 2018; Castiello et al., 2023).
However, the large footprints and speed of digital networked media result in spreading effects many orders of magnitude greater than biological pathogens. Continuing exponential growth in digital networks will inevitably increase observed adverse effects as susceptible populations grow. Spreading effects of this magnitude implicitly increase the impact of highly connected influencers in social networks, who can propagate messages to global audiences, with increasingly large footprints over time.
Social media platforms typically rank content by popularity, that has led to the widespread use of software robots or bots to create illusory popularity and further spreading behaviour. Bots propagate content by emulating the behaviour of human users sharing content, with each bot typically employing a user account with a fake identity. Bots have been found to enhance the spreading of content that might not have otherwise propagated well (Shao et al., 2018; Vosoughi et al., 2018; Gilani et al., 2019; Bovet and Makse, 2019; Himelein-Wachowiak et al., 2021).
Bots have been supplemented by social media “troll factories” where paid personnel using fake identities participate in social media debates to promote agendas or sow discord amongst legitimate social media users (Linvill and Warren, 2020).
4.2 Impacts of Artificial Intelligence technology
Artificial Intelligence (AI) technology is rapidly maturing. It is now deployed in many applications, including chatbots, natural language translation, image, speech and text recognition, and the synthesis of text, graphics and video. A major concern is that the AI algorithms and models underlying these systems have no understanding of truth, ethics or honesty, as they typically mimic human responses.
Early AI applications have shown disturbing parallels to well-known problems in human cognition. For instance, the “hallucination” effect, in which AI systems produce nonsensical answers to trivial problems, resembles “cognitive illusions” in humans (Kahneman and Tversky, 1996; Alkaissi and McFarlane, 2023). Cognitive biases and errors inherited from human generated training sets and human labelling are well known problems in the development of AI models (Schwartz et al., 2022).
AI models exhibit behaviours akin to human confirmation bias, where they show a preference for their own generated content (Yang et al., 2024). This raises the risk of a runaway positive feedback loop, leading to “Model Collapse,” in which the AI amplifies its own erroneous outputs over many training cycles (Shumailov et al., 2024).
Peterson (2025) argues that the availability of AI content, and the tendency of AI models to ignore less frequently seen content, effectively dilutes the available base of knowledge. This leads to the “Knowledge Collapse” problem, where important content is lost. The “Model Collapse” and “Knowledge Collapse” problems could accelerate the established problem of “Truth Decay” (RAND Corporation, 2019).
Erroneous outputs and embedded bias errors, are obstacles to legitimate applications of AI systems, but they do not present obstacles in the production of propaganda and disinformation, which are not concerned with the truth. Poisoning of AI model training datasets with propaganda is now an identified problem (Bagdasaryan and Shmatikov, 2022; Nguyen et al., 2024). Sadeghi et al. (2024) found multiple AI chatbots presenting propaganda narratives as fact as a result of training dataset poisoning by a nation state actor.
The integration of generative AI with web based platforms is already being pursued as a vehicle for producing and sharing malign propaganda (Vykopal et al., 2024). The most disturbing recent instance involves training an AI model to digitally emulate a deceased ultra-nationalist propagandist (Radauskas, 2023).
Predicted a quarter of a century ago (Kopp, 2000), convincing “deep fake” imagery and video produced by generative AI now exhibit increasingly high fidelity and low production cost due to exponential growth in computer technology, and can enhance propaganda acceptance (Helmus, 2022).
Accurately assessing the effects of specific attacks is a very difficult challenge without standardised measures and detailed data showing the exposure and vulnerability of populations (Kopp, 2024). A nation-by-nation analysis of techniques employed has underscored this problem (Łabuz and Nehring, 2024). They explored the use of “deep fake” technology in election campaigns in eleven nations across the globe, specifically the USA, Turkiye, Argentina, Poland, UK, France, India, Bulgaria, Taiwan, Indonesia, and Slovakia, and also found non-election related political exploitation in Estonia, Germany, Israel, Japan, Serbia, Sudan, and other nations, showing that this technology has become a mainstream tool for malign manipulation of public opinion.
Łabuz and Nehring (2024) found the highest frequency of “deep fake” technology use in the Argentinian election of 2023, but concluded that despite intensive use by most campaigns, the election outcome was not strongly impacted. Their study shows that the use of ‘deep fake' technology in 2023 was haphazard, and techniques for maximising effects on audiences were mostly not refined. They found little evidence of precise message targeting, and in many instances the poor quality of fakes permitted easy debunking by media and political opponents.
Microtargeting, where generative AI is used to produce messages customised to the vulnerabilities of specific individuals being targeted has proven effective in political advertising (Simchon et al., 2024). The widely debated Cambridge Analytica scandal involved the abuse of personal information to enable microtargeting of voters in an election (Berghel, 2018).
The use of AI algorithms that suggest links based on a user's access history in Internet searches has caused further problems. Pariser identified the “filter bubble” effect, where users become isolated in groups that share common interests and viewpoints, and filtering hides alternative content (Pariser, 2011). Microtargeting and filtering can produce a positive feedback loop, in which existing cognitive biases are reinforced by further exposure to congruent information, thus increasing confidence in a belief that may be biased or false. For instance, after accessing a few biased items, a user may become immersed in a deluge of biased or false material (Green, 2014). Fisher (2022) details numerous cases where the spread of fake ideas and conspiracy theories in this way have spilled out into real-world violence.
Feedback loops have been shown to contribute to polarisation (Rafail et al., 2024). Recent studies have used highly polarised test populations to test hypotheses about feedback loops, echo chambers, filter bubbles and impacts of social media exposure (Asimovic et al., 2021, 2023; Guess et al., 2023; Nyhan et al., 2023; Törnberg, 2018; Cinelli et al., 2021; Dahlgren, 2021). The outcomes suggest weak impacts of these mechanisms, which in turn indicates that in a highly polarised population, mechanisms such as confirmation bias, social identity maintenance and pluralistic ignorance are dominant. Uncritical acceptance of false beliefs that appear to be coherent with prior beliefs accepted in the population is a common outcome. The paucity of studies on weakly polarised or unpolarized populations leaves the generality of claimed minor impacts only weakly tested.
Malign AI models, deep fakes, microtargeting, AI search algorithms and poisoning of training data sets exacerbate the implicit integrity problems seen in AI (Helmus, 2022; Radauskas, 2023; Cinà et al., 2023; Simchon et al., 2024). Increasing reliance on such systems for news and information are undermining sound decision-making (Winchester, 2023). AI can therefore directly contribute to dysfunctional cognition by inadvertently or intentionally injecting often persistent false beliefs, false interpretations and biases into its users (Honigberg, 2022; Vicente and Matute, 2023).
5 Malign exploitation of dysfunctional thinking
Dysfunctional thinking in individuals and groups presents opportunities for manipulation and exploitation. Such manipulation has a colourful history, involving especially propaganda, gaslighting, and political deception (Bernays, 1928; Davis and Ernst, 2019; Kahan, 2011; Bardon, 2019; MacKenzie and Bhatt, 2020).
The aim of an exploiter is to gain an advantage over victims and elicit behaviour that favours the exploiter. Opportunities arise especially where objective truth creates dissonance relative to the victim's prior beliefs or interpretations, or where understanding truth imposes a high cognitive load (Kaaronen, 2018; Sweller, 1988; Jian et al., 2019).
Gershman (2019) showed by the application of Bayesian reasoning that supporting beliefs, termed auxiliary hypotheses, can prevent disconfirmation of a belief when confronted with disconfirming evidence. Exploiters can therefore reinforce false beliefs or reduce confidence in true beliefs by focusing on supporting beliefs.
Creative exploitation of dysfunctional thinking employs tools that are all instances of well-studied and understood compound deceptions. In propaganda, gaslighting and deceptive political practises, it is a common to exploit the victim's prior false beliefs and interpretations, and to provide multiple channels of deception (Haswell, 1985; Brumley et al., 2005). Exploiters utilise false interpretations of objective truths, and if necessary, create new false posterior beliefs and interpretations (Haswell, 1985; Kalbfleisch and Docan-Morgan, 2019; Halbach and Leigh, 2020).
Once the victim is entrapped by a false belief or interpretation, the exploiter can introduce further false beliefs and interpretations. The victim then perceives an alternate reality, which is often sufficiently internally consistent to minimise cognitive dissonance (Malgin, 2014; Pies, 2017a).
Kahneman (2011) argued that humans have two modes of processing information: fast thinking, based on heuristic shortcuts, and slow thinking, based on reasoning. Empirical studies show that only some heuristics facilitate the detection of deceptions, while most are ineffective (Qin and Burgoon, 2007; Verschuere et al., 2023). Ineffective heuristics in fast thinking applied to detecting deceptions are prior false beliefs and interpretations about deceptions. This includes assumptions about the veracity of sources (Verschuere et al., 2023; Orchinik et al., 2023).
False prior beliefs are exploited often because confirmation bias makes the victim susceptible to falsehoods that are coherent with the false prior belief. Victims may accept false claims that reinforce their established bias, as this is a consequence of Bayesian-like belief updating (Pilgrim et al., 2024). Empirical studies that have shown the strong influence of prior beliefs, true or false, support the observations of Tappin et al. (2021); Orchinik et al. (2023). In both advertising and propaganda, popular techniques involve exposing audiences to large-scale, repeated messages intended to influence their beliefs. This demonstrates the effort that is required to dislodge established beliefs, whether they are true or false (Kopp, 2005, 2006; Asimovic et al., 2021, 2023; Pilgrim et al., 2024).
Beliefs that are not falsifiable, and not subject to revision, can reinforce entrapment (Friesen et al., 2015; Bardon, 2019). Where prior beliefs and interpretations are not false, an exploiter will attack both. Often they accomplish this by deceptions that increase uncertainty, and by exploiting the false consensus bias, while logical fallacies are used to disrupt proper interpretations. Once the victim is uncertain about their correct prior beliefs and interpretations, they can be seduced with false beliefs and interpretations. The diversity of known deceptions used in exploitation is empirical proof that skilled exploiters often target all vulnerabilities in a victim's cognitive functions (Bernays, 1928; Dorpat, 1996; MacKenzie and Bhatt, 2020).
5.1 Gaslighting, propaganda, post-truth, and fake news
A notable instance of exploitation is Gaslighting. This refers to the systematic practise of feeding a victim or victims with falsehoods, so that they begin to doubt their own memory, perception, or judgment (Dorpat, 1996). An exploiter will consistently deny facts and make blatantly false statements, thus undermining the victim's prior beliefs and interpretations and paving the way to promote their agenda. In effect, the exploiter is deceptively creating uncertainty in the victim's understanding.
Individuals have used gaslighting in many different contexts, for instance, to cover up extramarital affairs to their spouse (Gass and Nichols, 1988), identity-related abuse of transgender children by their parents (Riggs and Bartholomaeus, 2018), and racial gaslighting to maintain white supremacy in the United Stated (Davis and Ernst, 2019). Gaslighting has also played a part in domestic violence (Sweet, 2019) and in suppressing whistle-blowing within institutions (Ahern, 2018). Sweet (2019) suggested that gaslighting is more effective in cases where it is rooted in social inequalities, especially gender, race, and sexuality.
While the tools employed for different kinds of manipulation may be identical, the scale of the manipulated population varies widely. Like political manipulations, propaganda typically targets nation states or even global populations, while gaslighting typically targets sub-populations or individuals.
The common thread is the use of deceptions intended to inject false beliefs and false interpretations into the minds of the victims. Where successful, these deceptions put the victims into a perceptual alternate reality, where common dysfunctional thinking behaviours capture them and reinforce a system of false beliefs and interpretations.
The Nazi and Soviet propaganda systems were large, internally coherent machines for gaslighting victim populations (Krumiņš, 2018; Sinha, 2020). A notable feature of both was the intentional exclusion of external information sources. This precluded cognitive dissonance in the victim population, and enabled exploitation of prior cognitive biases and anxieties where available. By exploiting online news platforms and social media, gaslighting has also played a role in Western, especially American politics (Carpenter, 2018).
As observed above, the transition to digital media, and the advent of social media, created immense new opportunities for exploitation, by accessing millions or billions of users globally in timescales of minutes. This presented unprecedented opportunities to propagate misinformation, disinformation, propaganda, and fake news among the public (Bovet and Makse, 2019).
The nature of social media platforms, with their “influencers,” “follower networks,” “echo chambers,” “filter bubbles,” and automated ranking algorithms, present significant opportunities for exploitation. In marketing, the use of influencers and viral promotion are well-known and widely used (Miller and Lammas, 2010; Vaidya and Karnawat, 2023). Bakshy et al. (2011) showed that multiple strategies using influencers could produce cascades in social media traffic. Dubois and Blank (2018) showed that social media users who rely on a narrow range of sources are susceptible to “echo chambers,” although the prevalence was lower than earlier thought. Chitra and Musco (2020) found that for multiple kinds of social media platforms, Pariser's filter bubble effect could “greatly increase opinion polarisation in social networks.”
Fake user accounts, software bots and troll factories exploit both confirmation bias and especially false consensus bias concurrently. Both empirical studies (Vosoughi et al., 2018; Linvill and Warren, 2020) and simulations (Xiao et al., 2015) prove the effectiveness of these techniques. They show that deceptive messages propagate exponentially in a manner akin to biological pathogens, as noted earlier (Castiello et al., 2023). However, they spread much faster than biological pathogens and across much larger populations, reflecting both the well-known behaviour of connectivity avalanches (Paperin et al., 2011; Green, 2014; Akbarpour and Jackson, 2018) and the nature of the digital infrastructure (Nickerson, 1998; Pariser, 2011; Kalogeratos et al., 2018; Kopp et al., 2018; Toma et al., 2019).
For example, even if an individual's peers are not supporting an agenda, leaders could make consistent and repeated false statements about public opinion. This practise can undermine individuals' perceptions or judgments. They might begin to believe the propaganda, a situation previously explained using game theory (Press and Dyson, 2012). Thus, gaslighting a population by using propaganda, post-truth, and fake news will affect both the individual's opinion and their perception of public opinion.
Another recently popularised term is post-truth, which describes statements that appeal to public emotions, bypassing the truth and ignoring expert opinions or fact-checking. These false and misleading statements are used to gaslight the population. The intent is to make individuals ignore their own judgment, and eventually sway public opinion towards the promoted falsehood (Rocavert, 2019). While this term was popularised after the 2016 Brexit vote and the 2016 US presidential election, it was already an implicit feature of past Nazi and Soviet propaganda systems (McIntyre, 2018).
McIntyre (2018) suggested that the post-truth era is a result of people favouring “alternative facts” in place of actual facts, and of feelings having more weight than evidence:
What is striking about the idea of post-truth is not just that the truth is being challenged, but that it is being challenged as a mechanism for asserting political dominance.
Many recent studies have investigated the prevalence of post-truth in politics. It takes the form of “obfuscation of facts, abandonment of evidential standards in reasoning, and outright lying” (McIntyre, 2018; Sismondo, 2017; D'Ancona, 2017). Introducing new information with factual evidence is not enough to break this deep-seated spell of post-truth, rather it needs political intervention and sincere public incentives with sufficient motivation to become well-informed instead of staying misinformed (Lewandowsky et al., 2017).
A curious aspect of the post-truth phenomenon is that improbable falsehoods are frequently accepted without question. In part, this may reflect the potential of falsehoods to minimise cognitive dissonance and load for an audience, whereas objective truth may increase it. This could explain why falsehoods tend to propagate better in social media (Vosoughi et al., 2018). Shannon's (1948) information theory showed that improbable but true messages contain much more information than highly probable messages. This is coherent with empirical findings (Ruffo et al., 2023) that disinformation worked “through a series of cognitive hooks that make the information appealing, triggering a psychological reaction in the reader,” where disinformation employed more novelty, was easier to process, and produced a stronger emotional reaction in the audience.
If humans are instinctively drawn to the improbable, then individual and social cognitive dysfunctions create an unusually high susceptibility to improbable falsehoods.
5.2 The rise of denialism, conspiracy theories, and disruptive propaganda
As shown earlier, many kinds of dysfunctional thinking contribute to often-cited societal problems, such as the widespread influence of denialism (Section 2.7), conspiracy theories (Section 2.1), and “fake news” (Section 5.1) (Green, 2014; Beauvais, 2022). In many social contexts, especially politics, a party challenging an established belief, whether true or false, will capture attention and appear to be strong, while those defending appear weaker. This imbalance is a problem because false claims are easy to fabricate because they are unburdened by facts. Mass media and social media instantly propagate sensational claims, even if they are improbable. In contrast, disproving a false claim can take hours, days or even months. Also, disproof often requires technical evidence that is often difficult to understand, and thus poorly reported.
The increasingly esoteric, technical nature of scientific information makes the above problems worse. Science has become increasingly difficult to comprehend by lay audiences (Plavén-Sigray et al., 2017). This problem has contributed to increasing distrust in science (Rutjens et al., 2018). Instead, people often turn to sources they trust, even sources whose trustworthiness is weak.
Kahneman (2011) showed that intuitive, fast thinking favours cognitive biases over reason (Kahneman and Tversky, 1996). Where “information overload” arises (Toffler, 1970), susceptibility to fast thinking and avoidance of cognitive dissonance are inevitably increased. Such cognitive ‘shortcuts” can lead to errors in perception and interpretation. For instance, people are prone to search for patterns, and may mistake random arrangements for deliberate design (see Section 2.1). They often confuse correlation with causation (Altman and Krzywinski, 2015) and are prone to mistake random events for deliberate intent (Waytz et al., 2010; Green, 2014).
In exploiting these vulnerabilities, conspiracy theorists often employ well-tried educational methods to spread falsehoods. For instance, trying to argue against entrenched ideas only reinforces them (Nyhan and Reifler, 2010). Leading people to discover ideas for themselves is far more effective (Bruner, 1961).
False interpretations of truthful accounts can be very persuasive, Allen et al. (2024) found that such reports had 46-fold more effect than reports identified as misinformation by a social media platform.
Susceptibility to malign messaging has been identified as a factor in the spread of conspiracy theories. Bowes et al. (2023) found a close correlation between subjective beliefs in threats and powerlessness, intolerance of ambiguity, receptivity to misinformation, as well as collective narcissism, and conspiratorial ideation.
Social pressures also reinforce the acceptance of falsehoods. Pluralistic ignorance can drive members of social groups to believe that everyone in their group holds to a claim, so they advertise their membership by spreading the claim themselves. If an assertive advocate of the falsehood is present, groupthink may also arise.
Finally, digital technology exacerbates these problems by virtue of its pervasive coverage, dense network interconnections, and speed, as observed earlier. Falsehoods can be rapidly constructed using AI tools, rapidly and widely disseminated using digital mass media, social media and messaging platforms, and reinforced using “bots” and “troll farms” as observed above (Section 4). Algorithmic filtering may produce ‘filter bubbles” and positive feedback ‘echo chambers' that further reinforce prior biases and polarisation, acting in effect as passive censorship (Pariser, 2011).
These problems are exacerbated by absent or biased fact-checking, which legitimises falsehoods, whereby fact-checkers become proxy deceivers, thus supporting exploiters (Soprano et al., 2024).
Denialism, which amounts to reflexive rejection of objective truths, reinforces the previous problems. Denialists and exploiters of denialism employ the full gamut of traditional deception tactics to self-deceive, or recruit others to share their deceptions. These tactics include false allegations of conspiracy, fabrication of false evidence, cherry-picking objective truths, demanding unattainable proofs for counterargument, exploiting logical fallacies, and using deception to increase uncertainty (Hoofnagle, 2007; Diethelm and McKee, 2009; Green, 2014). The problem of denialism, exacerbated by the Dunning-Kruger effect, is central to the problem of pervasive public rejection of expert insights (Nichols, 2017).
In summary, exploiters possess multiple asymmetric advantages when individuals and groups are victims of cognitive dysfunctions.
6 Measures to defeat malign exploitation
Given the immense diversity of methods and techniques in use to effect malign exploitation, there is no simple panacea solution to address this problem, and any such expectation is wishful thinking (Kopp, 2024).
As noted earlier, the way malign messages spread closely reflects models used in epidemiology (Castiello et al., 2023). Biological pathogens are typically defeated or controlled by measures analogous to those discussed below: (1) suppress the source, (2) control or suppress propagation, and (3) increase the immunity of the exposed population. There is a case to be made for this approach in dealing with malign exploitation (Kopp, 2024, 2025).
Malign messaging intended for exploitation is typically designed for desired effects. It must be produced, delivered (directly or by proxies), and its effects must be assessed to learn whether it achieved the desired effects. Counter-measures can therefore target one or more phases in this process: the means of creating a malign message; its distribution to targeted individuals; or its processing by a targeted individual. The latter provides the option of making a target population more resistant to malign messages (Roozenbeek et al., 2022).
In practical terms, each phase of the production and delivery cycle of malign messaging is susceptible to defeat or to measures that degrade its effects (Kopp, 2024, 2025).
Law enforcement can halt production in a persistent, or non-persistent, manner. Nation state producers present the biggest challenges as they typically employ national resources to both produce and protect their means of production and distribution (Kopp, 2025). They can be countered by cyber-attacks (Nakashima, 2019) or other means, such as regime change.
The distribution of malign messaging presents further opportunities for defeat or mitigation. The means of distribution are now primarily digital. In nation states subjected to malign messaging. the entities that operate digital distribution fall under the footprint of regulatory bodies, or of law enforcement.
Social media platforms, websites, and encrypted messaging platforms can be blocked to deny access. This approach has be widely employed, but can often be overcome by technological means. Effectiveness has varied widely, often polarised populations will seek the denied content via other channels (Golovchenko, 2022; Okholm et al., 2024).
Another option to reduce the spread of disinformation is to “deplatform” malign influencers. However, such parties may simply migrate to another platform, if possible in another jurisdiction (Jhaver et al., 2021; Ribeiro et al., 2024). Removal of proxies who share malign messages could present legal challenges (Law Council of Australia, 2023), as they may believe the false messages.
Using fact checking to filter content presents practical challenges, especially the potential for bias and limitations on competency. These constraints also impact the use of AI for fact checking (Korb, 2022; Kopp, 2025). Fact checking frequently fails due to bias in a polarised audience (Hameleers and van der Meer, 2020).
Finally, immunisation or inoculation strategies aim to temporarily or permanently equip potential victims with the capability to identify malign messaging (Roozenbeek et al., 2022). However, recognising that a message contains malign disinformation does not guarantee it will be rejected (Pies, 2017b; Ecker et al., 2022). Persistence of effects is yet to be proven, and experience with the “forgetting curve” indicates that retention will be a challenge for this approach (Sarno et al., 2022; Murre and Dros, 2015).
7 Conclusion
This study proposed a synthesis of models that trace cognitive dysfunction from individuals to groups, and societies. It also argued that advances in digital technology and AI permit the formation of immense, highly connected social networks. These create conditions for the rapid emergence of large-scale, dysfunctional group behaviours.
Most previous studies have focused on processes at a single scale. This account began by surveying a range of these models, which deal with cognitive dysfunction at each of the above scales. Individuals are affected by limitations on cognition, and by innate drives. Cognitive biases of individuals lead to dysfunctional effects in behaviour of groups. In social networks, peer to peer interactions can propagate false beliefs. New technologies, especially the Internet, enable these interactions to cascade widely and rapidly. Finally, the study showed how malicious agents exploit cognitive dysfunctions.
The impacts of exponentially growing digital technologies present a daunting picture of the future. They have accelerated and expanded the scale of dysfunctional social cognition and its effects (Shao et al., 2018; Bovet and Makse, 2019; Treen et al., 2020; Himelein-Wachowiak et al., 2021; Fisher, 2022; Ruffo et al., 2023).
The future will see increasing global coverage by systems that integrate pervasive networking with AI systems capable of producing convincing content, including material that is false, misleading or malicious. The commodification of AI will further exacerbate such challenges, by providing effective tools for malign exploitation. AI generated content will rapidly displace human generated content, and nonsensical and malign messaging will become pervasive unless controlled. Opportunities for malign exploitation will abound unless controlled, and there is immense potential for commercial and political abuse of such an environment.
The challenge ahead is abundantly clear. If these technologies continue their rapid advance, so will the potential for malign social impacts.
Further study is needed to better understand individual and collective vulnerabilities to the multiplicity of highly refined techniques for the malign exploitation of cognitive dysfunctions (Lazer et al., 2018; Kopp et al., 2018).
Many measures as noted earlier can be shown to mitigate or reduce malign exploitation.
Bak-Coleman et al. (2021) argue that emergent effects are already producing pervasive damage to societies and conclude that the study of collective behaviour should be elevated to a “crisis discipline.” Their conclusions support the argument that recent research involving modelling of these effects in social systems provides the essential tools for analysis and management of both of these damage effects and policy measures to mitigate them.
Author contributions
FS: Conceptualization, Investigation, Writing – original draft, Writing – review & editing. DG: Conceptualization, Formal analysis, Investigation, Methodology, Visualization, Writing – original draft, Writing – review & editing. CK: Conceptualization, Formal analysis, Investigation, Methodology, Visualization, Writing – original draft, Writing – review & editing.
Funding
The author(s) declare that financial support was received for the research and/or publication of this article. This study was sponsored by Monash University.
Conflict of interest
The authors declare that the research was conducted in the absence of any commercial or financial relationships that could be construed as a potential conflict of interest.
Generative AI statement
The author(s) declare that no Gen AI was used in the creation of this manuscript.
Publisher's note
All claims expressed in this article are solely those of the authors and do not necessarily represent those of their affiliated organizations, or those of the publisher, the editors and the reviewers. Any product that may be evaluated in this article, or claim that may be made by its manufacturer, is not guaranteed or endorsed by the publisher.
References
Ahern, K. (2018). Institutional betrayal and gaslighting. J. Perin. Neonatal Nurs. 32, 59–65. doi: 10.1097/JPN.0000000000000306
Akbarpour, M., and Jackson, M. O. (2018). Diffusion in networks and the virtue of burstiness. Proc. Nat. Acad. Sci. 115, E6996–E7004. doi: 10.1073/pnas.1722089115
Albert, R., Jeong, H., and Barabási, A.-L. (2000). Error and attack tolerance of complex networks. Nature 406, 378–382. doi: 10.1038/35019019
Alkaissi, H., and McFarlane, S. I. (2023). Artificial hallucinations in ChatGPT: implications in scientific writing. Cureus 15:35179. doi: 10.7759/cureus.35179
Allen, J., Watts, D. J., and Rand, D. G. (2024). Quantifying the impact of misinformation and vaccine-skeptical content on facebook. Science 384, 1–8. doi: 10.1126/science.adk3451
Altman, N., and Krzywinski, M. (2015). Association, correlation and causation. Nat. Methods 12, 899–900. doi: 10.1038/nmeth.3587
Amblard, F., and Deffuant, G. (2004). The role of network topology on extremism propagation with the relative agreement opinion dynamics. Phys. A 343, 725–738. doi: 10.1016/j.physa.2004.06.102
Artime, O., Grassia, M., De Domenico, M., Gleeson, J. P., Makse, H. A., Mangioni, G., et al. (2024). Robustness and resilience of complex networks. Nat. Rev. Phys. 6, 114–131. doi: 10.1038/s42254-023-00676-y
Asimovic, N., Nagler, J., Bonneau, R., and Tucker, J. A. (2021). Testing the effects of Facebook usage in an ethnically polarized setting. Proc. Nat. Acad. Sci. 118:e2022819118. doi: 10.1073/pnas.2022819118
Asimovic, N., Nagler, J., and Tucker, J. A. (2023). Replicating the effects of Facebook deactivation in an ethnically polarized setting. Res. Polit. 10:20531680231205157. doi: 10.1177/20531680231205157
Bagchi, D. (2016). Printing, Propaganda, and Public Opinion in the Age of Martin Luther. Oxford: Oxford Research Encyclopedia of Religion. doi: 10.1093/acrefore/9780199340378.013.269
Bagdasaryan, E., and Shmatikov, V. (2022). “Spinning language models: risks of propaganda-as-a-service and countermeasures,” in 2022 IEEE Symposium on Security and Privacy (SP), 769–786. doi: 10.1109/SP46214.2022.9833572
Bak-Coleman, J. B., Alfano, M., Barfuss, W., Bergstrom, C. T., Centeno, M. A., Couzin, I. D., et al. (2021). Stewardship of global collective behavior. Proc. Nat. Acad. Sci. 118:e2025764118. doi: 10.1073/pnas.2025764118
Bakshy, E., Hofman, J. M., Mason, W. A., and Watts, D. J. (2011). “Everyone's an influencer: quantifying influence on twitter,” in Proceedings of the Fourth ACM International Conference on Web Search and Data Mining, WSDM '11 (New York, NY, USA: Association for Computing Machinery), 65–74. doi: 10.1145/1935826.1935845
Banyard, V. L. (2011). Who will help prevent sexual violence: creating an ecological model of bystander intervention. Psychol. Violence 1:216. doi: 10.1037/a0023739
Barabási, A.-L., and Albert, R. (1999). Emergence of scaling in random networks. Science 286, 509–512. doi: 10.1126/science.286.5439.509
Bardon, A. (2019). The Truth About Denial: Bias and Self-Deception in Science, Politics, and Religion. Oxford: Oxford University Press. doi: 10.1093/oso/9780190062262.001.0001
Barnes, J. A. (1969). Graph theory and social networks: a technical comment on connectedness and connectivity. Sociology 3, 215–232. doi: 10.1177/003803856900300205
Baronchelli, A. (2018). The emergence of consensus: a primer. R. Soc. Open Sci. 5:172189. doi: 10.1098/rsos.172189
Bauman, S., Yoon, J., Iurino, C., and Hackett, L. (2020). Experiences of adolescent witnesses to peer victimization: the bystander effect. J. Sch. Psychol. 80, 1–14. doi: 10.1016/j.jsp.2020.03.002
Beauvais, C. (2022). Fake news: why do we believe it? Joint Bone Spine 89:105371. doi: 10.1016/j.jbspin.2022.105371
Berghel, H. (2018). Malice domestic: the Cambridge analytica dystopia. Computer 51, 84–89. doi: 10.1109/MC.2018.2381135
Bessi, A., and Ferrara, E. (2016). Social bots distort the 2016 US Presidential election online discussion. First Monday 21:7090. doi: 10.5210/fm.v21i11.7090
Bikhchandani, S., Hirshleifer, D., and Welch, I. (1992). A theory of fads, fashion, custom, and cultural change as informational cascades. J. Polit. Econ. 100, 992–1026. doi: 10.1086/261849
Bovet, A., and Makse, H. A. (2019). Influence of fake news in Twitter during the 2016 US presidential election. Nat. Commun. 10, 1–14. doi: 10.1038/s41467-018-07761-2
Bowen, R., Dmitriev, D., and Galperti, S. (2021). Learning from Shared News: When Abundant Information Leads to Belief Polarization. Working Paper 28465, National Bureau of Economic Research. doi: 10.3386/w28465
Bowers, J., and Davis, C. (2012). Bayesian just-so stories in psychology and neuroscience. Psychol. Bull. 138, 389–414. doi: 10.1037/a0026450
Bowes, S. M., Costello, T. H., and Tasimi, A. (2023). The conspiratorial mind: a meta-analytic review of motivational and personological correlates. Psychol. Bull. 149, 259–293. doi: 10.1037/bul0000392
Brauer, F. (2008). “Compartmental models in epidemiology,” in Mathematical Epidemiology, eds. F. Brauer, P. Van den Driessche, and J. Wu (Berlin, Heidelberg: Springer), 19–79. doi: 10.1007/978-3-540-78911-6_2
Brewer, M. B., and Caporael, L. R. (2006). “An evolutionary perspective on social identity: revisiting groups,” in Evolution and Social Psychology, eds. M. Schaller, J. A. Simpson, and D. T. Kenrick (New York: Psychology Press), 143–161.
Brumley, L. (2014). Misperception and its evolutionary value. PhD thesis, Faculty of Information Technology, Monash University.
Brumley, L., Kopp, C., and Korb, K. B. (2005). “Misperception, self-deception and information warfare,” in Proceedings of the 6th Australian Information Warfare and Security Conference 2005, eds. G. Pye, and M. Warren (Geelong, Australia: School of Information Systems, Deakin University), 71–79.
Burt, R. S. (1987). Social contagion and innovation: cohesion versus structural equivalence. Am. J. Sociol. 92, 1287–1335. doi: 10.1086/228667
Castiello, M., Conte, D., and Iscaro, S. (2023). Using epidemiological models to predict the spread of information on Twitter. Algorithms 16:391. doi: 10.3390/a16080391
Cherry, S. (2004). Edholm's Law of Bandwidth: telecommunications data rates are as predictable as Moore's Law. IEEE Spectrum 41, 58–60. doi: 10.1109/MSPEC.2004.1309810
Chitra, U., and Musco, C. (2020). “Analyzing the impact of filter bubbles on social network polarization,” in Proceedings of the 13th International Conference on Web Search and Data Mining, WSDM '20 (New York, NY, USA: Association for Computing Machinery), 115–123. doi: 10.1145/3336191.3371825
Choi, H., Kim, S.-H., and Lee, J. (2010). Role of network structure and network effects in diffusion of innovations. Ind. Market. Manag. 39, 170–177. doi: 10.1016/j.indmarman.2008.08.006
Cialdini, R. B., and Goldstein, N. J. (2004). Social influence: compliance and conformity. Annu. Rev. Psychol. 55, 591–621. doi: 10.1146/annurev.psych.55.090902.142015
Ciná, A. E., Grosse, K., Demontis, A., Vascon, S., Zellinger, W., Moser, B. A., et al. (2023). Wild patterns reloaded: a survey of machine learning security against training data poisoning. ACM Comput. Surv. 55, 1–39. doi: 10.1145/3585385
Cinelli, M., Morales, G. D. F., Galeazzi, A., Quattrociocchi, W., and Starnini, M. (2021). The echo chamber effect on social media. Proc. Nat. Acad. Sci. 118:e2023301118. doi: 10.1073/pnas.2023301118
Croft, A., Atkinson, C., Sandstrom, G., Orbell, S., and Aknin, L. (2021). Loosening the grip (gender roles inhibiting prosociality) to promote gender equality. Person. Soc. Psychol. Rev. 25, 66–92. doi: 10.1177/1088868320964615
Dahlgren, P. M. (2021). A critical review of filter bubbles and a comparison with selective exposure. Nordicom Rev. 42, 15–33. doi: 10.2478/nor-2021-0002
Davis, A. M., and Ernst, R. (2019). Racial gaslighting. Polit. Groups, Ident. 7, 761–774. doi: 10.1080/21565503.2017.1403934
Del Giudice, M. (2018). Evolutionary psychopathology: a unified approach. Oxford University Press. doi: 10.1093/med-psych/9780190246846.001.0001
Del Vicario, M., Bessi, A., Zollo, F., Petroni, F., Scala, A., Caldarelli, G., et al. (2016a). The spreading of misinformation online. Proc. Nat. Acad. Sci. 113, 554–559. doi: 10.1073/pnas.1517441113
Del Vicario, M., Scala, A., Caldarelli, G., Stanley, H., and Quattrociocchi, W. (2016b). Modeling confirmation bias and polarization. Sci. Rep. 7:40391. doi: 10.1038/srep40391
Dewar, J. A. (2000). The information age and the printing press: looking backward to see ahead. Ubiquity 8:348784. doi: 10.1145/347634.348784
Diethelm, P., and McKee, M. (2009). Denialism: what is it and how should scientists respond? Eur. J. Public Health 19, 2–4. doi: 10.1093/eurpub/ckn139
Do, J. J., Samuels, S. M., Adkins, D. J., Clinard, M. E., and Koveleskie, A. J. (2013). Gender bias and pluralistic ignorance in perceptions of fitness assessments. Milit. Psychol. 25, 23–35. doi: 10.1037/h0094754
Dorpat, T. L. (1996). Gaslighthing, the Double Whammy, Interrogation and Other Methods of Covert Control in Psychotherapy and Analysis. Jason Aronson Inc.
Dorst, K. (2020). Confirmation Bias Maximizes Expected Accuracy. Stranger Apologies newsletter. Available online at: https://www.kevindorst.com/stranger_apologies/confirmation-bias-as-avoiding-ambiguity (accessed May 6, 2025).
Dubois, E., and Blank, G. (2018). The echo chamber is overstated: the moderating effect of political interest and diverse media. Inf. Commun. Soc. 21, 729–745. doi: 10.1080/1369118X.2018.1428656
Dumitrescu, A.-T., Oltean, E., Merezeanu, D., and Dobrescu, R. (2017). “Emergence in hierarchical complex systems structured as social networks,” in 2017 21st International Conference on Control Systems and Computer Science (CSCS), 426–431. doi: 10.1109/CSCS.2017.66
Dunbar, R. (1995). Neocortex size and group size in primates: a test of the hypothesis. J. Hum. Evol. 28, 287–296. doi: 10.1006/jhev.1995.1021
Dunning, D., Heath, C., and Suls, J. M. (2004). Flawed self-assessment: implications for health, education, and the workplace. Psychol. Sci. Public Interest 5, 69–106. doi: 10.1111/j.1529-1006.2004.00018.x
Eagly, A. H., and Chaiken, S. (1993). The Psychology of Attitudes. San Diego: Harcourt Brace Jovanovich College Publishers.
Ecker, U. K. H., Lewandowsky, S., Cook, J., Schmid, P., Fazio, L. K., Brashier, N., et al. (2022). The psychological drivers of misinformation belief and its resistance to correction. Nat. Rev. Psychol. 1, 13–29. doi: 10.1038/s44159-021-00006-y
Egan, L. C., Santos, L. R., and Bloom, P. (2007). The origins of cognitive dissonance: evidence from children and monkeys. Psychol. Sci. 18, 978–983. doi: 10.1111/j.1467-9280.2007.02012.x
Enke, B., and Zimmermann, F. (2017). Correlation neglect in belief formation. Rev. Econ. Stud. 86, 313–332. doi: 10.1093/restud/rdx081
Epley, N., Waytz, A., Akalis, S., and Cacioppo, J. T. (2008). When we need a human: motivational determinants of anthropomorphism. Soc. Cogn. 26, 143–155. doi: 10.1521/soco.2008.26.2.143
Evans, J. S. B. (1989). Bias in Human Reasoning: Causes and Consequences. Mahwah: Lawrence Erlbaum Associates, Inc.
Festinger, L. (1957). A Theory of Cognitive Dissonance. Redwood City: Stanford University Press. doi: 10.1515/9781503620766
Festinger, L. (1962). A Theory of Cognitive Dissonance, volume 2. Redwood City: Stanford University Press.
Festinger, L. (1964). Conflict, Decision, and Dissonance, volume 3. Redwood City: Stanford University Press.
Fisher, M. (2022). The Chaos Machine: the Inside Story of How Social Media Rewired Our Minds and Our World. London: Hachette UK.
Flache, A., and Macy, M. W. (2011). Small worlds and cultural polarization. J. Math. Sociol. 35, 146–176. doi: 10.1080/0022250X.2010.532261
Flache, A., Mäs, M., Feliciani, T., Chattoe-Brown, E., Deffuant, G., Huet, S., et al. (2017). Models of social influence: towards the next frontiers. J. Artif. Soc. Soc. Simulat. 20:2. doi: 10.18564/jasss.3521
Friesen, J. P., Campbell, T. H., and Kay, A. C. (2015). The psychological advantage of unfalsifiability: the appeal of untestable religious and political ideologies. J. Pers. Soc. Psychol. 108, 515–529. doi: 10.1037/pspp0000018
Gao, J., Li, D., and Havlin, S. (2014). From a single network to a network of networks. Natl. Sci. Rev. 1, 346–356. doi: 10.1093/nsr/nwu020
Gass, G. Z., and Nichols, W. C. (1988). Gaslighting: a marital syndrome. Contemp. Fam. Ther. 10, 3–16. doi: 10.1007/BF00922429
Gearhart, S., and Zhang, W. (2015). “Was it something I said?” “No, it was something you posted!” A study of the spiral of silence theory in social media contexts. Cyberpsychol. Behav. Soc. Networ. 18, 208–213. doi: 10.1089/cyber.2014.0443
Geiger, N., and Swim, J. K. (2016). Climate of silence: Pluralistic ignorance as a barrier to climate change discussion. J. Environ. Psychol. 47, 79–90. doi: 10.1016/j.jenvp.2016.05.002
Gerbaudo, P. (2012). Tweets and the Streets: Social Media and Contemporary Activism. London: Pluto Press.
Gershman, S. J. (2019). How to never be wrong. Psychon. Bull. Rev. 26, 13–28. doi: 10.3758/s13423-018-1488-8
Gigerenzer, G. (1991). How to make cognitive illusions disappear: beyond “heuristics and biases”. Eur. Rev. Soc. Psychol. 2, 83–115. doi: 10.1080/14792779143000033
Gilani, Z., Farahbakhsh, R., Tyson, G., and Crowcroft, J. (2019). A large-scale behavioural analysis of bots and humans on Twitter. ACM Trans. Web 13, 1–23. doi: 10.1145/3298789
Goethals, G. R., Messick, D. M., and Allison, S. T. (1991). “The uniqueness bias: studies of constructive social comparison,” in Social Comparison: Contemporary Theory and Research, eds. J. Suls, and T. A. Wills (Lawrence Erlbaum Associates, Inc.), 149–176. doi: 10.4324/9781003469490-8
Golovchenko, Y. (2022). Fighting propaganda with censorship: a study of the Ukrainian ban on Russian Social Media. J. Polit. 84, 639–654. doi: 10.1086/716949
Gower-Winter, B., and Nitschke, G. (2023). “Using graph theory to produce emergent behaviour in agent-based systems,” in 2023 IEEE Symposium Series on Computational Intelligence (SSCI) (IEEE), 1690–1695. doi: 10.1109/SSCI52147.2023.10371866
Graham, S., Barnett, J., Fincher, R., Hurlimann, A., Mortreux, C., and Waters, E. (2013). The social values at risk from sea-level rise. Environ. Impact Assess. Rev. 41, 45–52. doi: 10.1016/j.eiar.2013.02.002
Green, D. G. (2014). Of Ants and Men-The Unexpected Side Effects of Complexity in Society. Cham: Springer. doi: 10.1007/978-3-642-55230-4
Green, D. G. (2023). Emergence in complex networks of simple agents. J. Econ. Inter. Coord. 18, 419–462. doi: 10.1007/s11403-023-00385-w
Greenwald, A. G. (1980). The totalitarian ego: Fabrication and revision of personal history. Am. Psychol. 35, 603–618. doi: 10.1037/0003-066X.35.7.603
Griffiths, T. L., Kemp, C., and Tenenbaum, J. B. (2008). “Bayesian models of cognition,” in The Cambridge Handbook of Computational Psychology, ed. R. Sun (Cambridge: Cambridge University Press), 59–100. doi: 10.1017/CBO9780511816772.006
Griffiths, T. L., and Tenenbaum, J. B. (2006). Optimal predictions in everyday cognition. Psychol. Sci. 17, 767–773. doi: 10.1111/j.1467-9280.2006.01780.x
Guess, A. M., Malhotra, N., Pan, J., Barberá, P., Allcott, H., Brown, T., et al. (2023). How do social media feed algorithms affect attitudes and behavior in an election campaign? Science 381, 398–404. doi: 10.1126/science.abp9364
Halbach, V., and Leigh, G. E. (2020). “Axiomatic theories of truth,” in The Stanford Encyclopedia of Philosophy. Metaphysics Research Lab, Stanford University, spring 2020 edition. Available online at: https://plato.stanford.edu/entries/truth-axiomatic/ (accessed May 6, 2025).
Halford, G. S., Wilson, W. H., and Phillips, S. (1998). Processing capacity defined by relational complexity: Implications for comparative, developmental, and cognitive psychology. Behav. Brain Sci. 21, 803–831. doi: 10.1017/S0140525X98001769
Hameleers, M., and van der Meer, T. G. L. A. (2020). Misinformation and polarization in a high-choice media environment: how effective are political fact-checkers? Communic. Res. 47, 227–250. doi: 10.1177/0093650218819671
Hampton, K. N., Rainie, H., Lu, W., Dwyer, M., Shin, I., and Purcell, K. (2014). Social media and the 'spiral of silence'. PewResearchCenter. Available online at: https://www.pewresearch.org/internet/2014/08/26/social-media-and-the-spiral-of-silence/ (accessed May 6, 2025).
Harmon-Jones, C., Haslam, N., and Bastian, B. (2017). Dissonance reduction in nonhuman animals: implications for cognitive dissonance theory. Animal Senti. 1:4. doi: 10.51291/2377-7478.1191
Harmon-Jones, E., and Mills, J. (2019). “An introduction to cognitive dissonance theory and an overview of current perspectives on the theory,” in Cognitive dissonance: Reexamining a pivotal theory in psychology, ed. E. Harmon-Jones (New York: American Psychological Association), 3–24. doi: 10.1037/0000135-001
Harvey, J. B. (1988). The abilene paradox: The management of agreement. Organ. Dyn. 17, 17–43. doi: 10.1016/0090-2616(88)90028-9
Haswell, J. (1985). The Tangled Web: The Art of Tactical And Strategic Deception (1st ed.). John Goodchild, Wendover.
Helmus, T. C. (2022). Artificial Intelligence, Deepfakes, and Disinformation: A Primer. Santa Monica, CA: RAND Corporation.
Heylighen, F. (2013). “Self-organization in communicating groups: the emergence of coordination, shared references and collective intelligence,” in Complexity Perspectives on Language, Communication and Society, eds. À. Massip-Bonet, and A. Bastardas-Boada (Berlin, Heidelberg: Springer), 117–149. doi: 10.1007/978-3-642-32817-6_10
Himelein-Wachowiak, M., Giorgi, S., Devoto, A., Rahman, M., Ungar, L., Schwartz, H. A., et al. (2021). Bots and misinformation spread on social media: implications for COVID-19. J. Med. Internet Res. 23:e26933. doi: 10.2196/26933
Hinds, J., Calderon, A., and Johnson, P. (2013). “Emergent behaviour and social media in large-scale disasters,” in IADIS Multi Conference on Computer Science and Information Systems 2013.
Hoff, P. (1990). German Television (1935–1944) as subject and medium of National Socialist Propaganda. Historical J. Film, Radio Telev. 10, 227–240. doi: 10.1080/01439689000260181
Holstein, J. A. (1985). Jurors' interpretations and jury decision making. Law Hum. Behav. 9, 83–100. doi: 10.1007/BF01044291
Honigberg, B. (2022). The existential threat of AI-enhanced disinformation operations. Technical report, Reiss Center on Law and Security, New York University School of Law.
Hoofnagle, C. (2007). Denialists' deck of cards: an illustrated taxonomy of rhetoric used to frustrate consumer protection efforts. Working paper, Public Choice and Political Economy eJournal. doi: 10.2139/ssrn.962462
Iñiguez, G., Govezensky, T., Dunbar, R., Kaski, K., and Barrio, R. A. (2014). Effects of deception in social networks. Proc. R. Soc. B Biol. Sci. 281:20141195. doi: 10.1098/rspb.2014.1195
Iyengar, S., and Hahn, K. S. (2009). Red media, blue media: evidence of ideological selectivity in media use. J. Commun. 59, 19–39. doi: 10.1111/j.1460-2466.2008.01402.x
Jalili, M., and Perc, M. (2017). Information cascades in complex networks. J. Complex Netw. 5, 665–693. doi: 10.1093/comnet/cnx019
Janis, I. L. (1972). Victims of Groupthink: A Psychological Study of Foreign-Policy Decisions and Fiascoes. Boston: Houghton Mifflin.
Janis, I. L. (1982). Groupthink: Psychological Studies of Policy Decisions and Fiascoes. Boston: Houghton Mifflin.
Jern, A., min Chang, K., and Kemp, C. (2014). Belief polarization is not always irrational. Psychol. Rev. 121, 206–224. doi: 10.1037/a0035941
Jhaver, S., Boylston, C., Yang, D., and Bruckman, A. (2021). Evaluating the effectiveness of deplatforming as a moderation strategy on Twitter. Proc. ACM Hum.-Comput. Interact. 5:3479525. doi: 10.1145/3479525
Jian, Z., Zhang, W., Tian, L., Fan, W., and Zhong, Y. (2019). Self-deception reduces cognitive load: the role of involuntary conscious memory impairment. Front. Psychol. 10:1718. doi: 10.3389/fpsyg.2019.01718
Jonas, E., Schulz-Hardt, S., Frey, D., and Thelen, N. (2001). Confirmation bias in sequential information search after preliminary decisions: An expansion of dissonance theoretical research on selective exposure to information. J. Pers. Soc. Psychol. 80, 557–571. doi: 10.1037/0022-3514.80.4.557
Kaaronen, R. O. (2018). A theory of predictive dissonance: Predictive processing presents a new take on cognitive dissonance. Front. Psychol. 9:2218. doi: 10.3389/fpsyg.2018.02218
Kahan, D. (2011). Neutral principles, motivated cognition, and some problems for constitutional law. Harvard Law Rev. 125, 1–77. doi: 10.2139/ssrn.1910391
Kahneman, D., and Tversky, A. (1972). Subjective probability: a judgment of representativeness. Cogn. Psychol. 3, 430–454. doi: 10.1016/0010-0285(72)90016-3
Kahneman, D., and Tversky, A. (1996). On the reality of cognitive illusions. Psychol. Rev. 103, 582–591. doi: 10.1037/0033-295X.103.3.582
Kalbfleisch, P. J., and Docan-Morgan, T. (2019). “Defining truthfulness, deception, and related concepts,” in The Palgrave Handbook of Deceptive Communication, eds. T. Docan-Morgan (Cham: Springer International Publishing), 29–39. doi: 10.1007/978-3-319-96334-1_2
Kalogeratos, A., Scaman, K., Corinzia, L., and Vayatis, N. (2018). “Chapter 24 - information diffusion and rumor spreading,” in Cooperative and Graph Signal Processing, eds. P. M. Djuric, and C. Richard (New York: Academic Press), 651–678. doi: 10.1016/B978-0-12-813677-5.00024-9
Karaffa, K. M., and Koch, J. M. (2016). Stigma, pluralistic ignorance, and attitudes toward seeking mental health services among police officers. Crim. Justice Behav. 43, 759–777. doi: 10.1177/0093854815613103
Kassin, S. M., Dror, I. E., and Kukucka, J. (2013). The forensic confirmation bias: Problems, perspectives, and proposed solutions. J. Appl. Res. Mem. Cogn. 2, 42–52. doi: 10.1016/j.jarmac.2013.01.001
Katz, D., Allport, F. H., and Jenness, M. B. (1931). Students' Attitudes: A Report of the Syracuse University Reaction Study. London: Craftsman Press.
Keeling, M. J., and Eames, K. T. (2005). Networks and epidemic models. J. R. Soc. 2, 295–307. doi: 10.1098/rsif.2005.0051
Kenrick, D. T., Griskevicius, V., Neuberg, S. L., and Schaller, M. (2010). Renovating the pyramid of needs: contemporary extensions built upon ancient foundations. Persp. Psychol. Sci. 5, 292–314. doi: 10.1177/1745691610369469
Kim, Y. (2001). A comparative study of the “Abilene paradox” and “Groupthink”. Public Admin. Quart. 25, 168–189. doi: 10.1177/073491490102500204
Kitts, J. A. (2003). Egocentric bias or information management? Selective disclosure and the social roots of norm misperception. Soc. Psychol. Quart. 66, 222–237. doi: 10.2307/1519823
Knobloch-Westerwick, S., Mothes, C., and Polavin, N. (2020). Confirmation bias, ingroup bias, and negativity bias in selective exposure to political information. Communic. Res. 47, 104–124. doi: 10.1177/0093650217719596
Kopp, C. (2000). “Moore's law and its implications for information warfare,” in Proceedings of the 3rd International Association of Old Crows (AOC) Electronic Warfare Conference, Zurich (Alexandria, Virginia: Association of Old Crows), 1–23. doi: 10.26180/27193632
Kopp, C. (2005). “Classical deception techniques and perception management vs. the four strategies of information warfare,” in Proceedings of the 6th Australian Information Warfare and Security Conference 2005 (IWAR 2005), eds. G. Pye, and M. Warren (Geelong, VIC: School of Information Systems, Deakin University), 81–89. doi: 10.26180/271938421172
Kopp, C. (2006). “Considerations on deception techniques used in political and product marketing,” in Proceedings of the 7th Australian Information Warfare and Security Conference 2006 (IWAR 2006), eds. C. Valli, and A. Woodward (Perth, WA: School of Computer and Information Science, Edith Cowan University), 62–71. doi: 10.4225/75/57a80fdfaa0cc1176
Kopp, C. (2024). “Defining measures of effect for disinformation attacks,” in Human Aspects of Information Security and Assurance, eds. N. Clarke, and S. Furnell (Cham: Springer Nature Switzerland), 180–199. doi: 10.1007/978-3-031-72559-3_13
Kopp, C. (2025). A Rationale for a Disinformation Defeat Capacity Maturity Model for Nations. White Paper. Monash University, Department of Software, Systems and Cybersecurity, Faculty of IT, Clayton, Australia.
Kopp, C., Korb, K. B., and Mills, B. I. (2018). Information-theoretic models of deception: Modelling cooperation and diffusion in populations exposed to “fake news”. PLoS ONE 13, 1–35. doi: 10.1371/journal.pone.0207383
Korb, K. B. (2022). Reliable Sources. Technical report, BayesianWatch - Bayesian argument analysis in action. Available online at: https://bayesianwatch.wordpress.com/2022/07/27/rs/ (accessed May 6, 2025).
Koriat, A., Adiv, S., and Schwarz, N. (2016). Views that are shared with others are expressed with greater confidence and greater fluency independent of any social influence. Person. Soc. Psychol. Rev. 20, 176–193. doi: 10.1177/1088868315585269
Kramer, R. M. (1998). Revisiting the Bay of Pigs and Vietnam decisions 25 years later: how well has the groupthink hypothesis stood the test of time? Organ. Behav. Hum. Decis. Process. 73, 236–271. doi: 10.1006/obhd.1998.2762
Kruger, J., and Dunning, D. (1999). Unskilled and unaware of it how difficulties in recognizing one's own incompetence lead to inflated self-assessments. J. Pers. Soc. Psychol. 77, 1121–1134. doi: 10.1037/0022-3514.77.6.1121
Krumiņš, G. (2018). Soviet economic gaslighting of Latvia and the Baltic States. Defence Strat. Commun. 4, 49–78. doi: 10.30966/2018.riga.4.2.
Kübler-Ross, E., Wessler, S., and Avioli, L. V. (1972). On death and dying. JAMA 221, 174–179. doi: 10.1001/jama.1972.03200150040010
Kunda, Z. (1990). The case for motivated reasoning. Psychol. Bull. 108, 480–498. doi: 10.1037/0033-2909.108.3.480
Łabuz, M., and Nehring, C. (2024). On the way to deep fake democracy? Deep fakes in election campaigns in 2023. Eur. Polit. Sci. 23, 454–473. doi: 10.1057/s41304-024-00482-9
Lake, B. M., Salakhutdinov, R., and Tenenbaum, J. B. (2015). Human-level concept learning through probabilistic program induction. Science 350, 1332–1338. doi: 10.1126/science.aab3050
Latané, B., and Nida, S. (1981). Ten years of research on group size and helping. Psychol. Bull. 89, 308. doi: 10.1037/0033-2909.89.2.308
Law Council of Australia (2023). Submission to the Department of Infrastructure, Transport, Regional Development, Communications and the Arts in relation to the Exposure Draft of the Communications Legislation Amendment (Combatting Misinformation and Disinformation) Bill 2023. Available online at: https://lawcouncil.au/publicassets/5b25938f-d346-ee11–948a-005056be13b5/4410%20-%20S%20-%20Combatting%20Misinformation%20and%20Disinformation.pdf (accessed May 6, 2025).
Lazer, D. M., Baum, M. A., Benkler, Y., Berinsky, A. J., Greenhill, K. M., Menczer, F., et al. (2018). The science of fake news. Science 359, 1094–1096. doi: 10.1126/science.aao2998
Lefebvre, G., Deroy, O., and Bahrami, B. (2024). The roots of polarization in the individual reward system. Proc. R. Soc. B 291:2011. doi: 10.1098/rspb.2023.2011
Levine, J., Etchison, S., and Oppenheimer, D. M. (2014). Pluralistic ignorance among student-athlete populations: a factor in academic underperformance. Higher Educ. 68, 525–540. doi: 10.1007/s10734-014-9726-0
Lewandowsky, S., Ecker, U. K., and Cook, J. (2017). Beyond misinformation: Understanding and coping with the “post-truth”? era. J. Appl. Res. Mem. Cogn. 6, 353–369. doi: 10.1016/j.jarmac.2017.07.008
Li, D. (2015). Do liars come to believe their own lies? The effect of deception on memory. PhD thesis, Psychology, University of New South Wales. doi: 10.26190/unsworks/18865
Li, N. P., Van Vugt, M., and Colarelli, S. M. (2018). The evolutionary mismatch hypothesis: Implications for psychological science. Curr. Dir. Psychol. Sci. 27, 38–44. doi: 10.1177/0963721417731378
Li, T., Zheng, Y., Wang, Z., Zhu, D. C., Ren, J., Liu, T., et al. (2022). Brain information processing capacity modeling. Sci. Rep. 12:2174. doi: 10.1038/s41598-022-05870-z
Linvill, D. L., and Warren, P. L. (2020). Troll factories: manufacturing specialized disinformation on Twitter. Polit. Commun. 37, 447–467. doi: 10.1080/10584609.2020.1718257
Lotan, G., Graeff, E., Ananny, M., Gaffney, D., and Pearce, I. (2011). The Arab Spring| the revolutions were tweeted: information flows during the 2011 Tunisian and Egyptian revolutions. Int. J. Commun. 5:31.
Machackova, H., Dedkova, L., and Mezulanikova, K. (2015). Brief report: The bystander effect in cyberbullying incidents. J. Adolesc. 43, 96–99. doi: 10.1016/j.adolescence.2015.05.010
Mackay, C. (1841). Extraordinary Popular Delusions and the Madness of Crowds. London: Richard Bentley. Reprinted 1980.
MacKenzie, A., and Bhatt, I. (2020). Opposing the power of lies, bullshit and fake news: the value of truth. Postdigital Sci. Educ. 2, 217–232. doi: 10.1007/s42438-019-00087-2
Malgin, A. (2014). Putin's Media Lives in an Alternate Reality. The Moscow Times. Available online at: https://www.themoscowtimes.com/2014/07/30/putins-media-lives-in-an-alternate-reality-a37849 (accessed May 6, 2025).
Maner, J. K., and Kenrick, D. T. (2010). When adaptations go awry: functional and dysfunctional aspects of social anxiety. Soc. Issues Policy Rev. 4, 111–142. doi: 10.1111/j.1751-2409.2010.01019.x
Marks, G., and Miller, N. (1987). Ten years of research on the false-consensus effect: an empirical and theoretical review. Psychol. Bull. 102, 72–90. doi: 10.1037/0033-2909.102.1.72
McAvoy, J., and Butler, T. (2007). The impact of the Abilene paradox on double-loop learning in an agile team. Inf. Softw. Technol. 49, 552–563. doi: 10.1016/j.infsof.2007.02.012
McGrath, A. (2017). Dealing with dissonance: a review of cognitive dissonance reduction. Soc. Personal. Psychol. Compass 11:e12362. doi: 10.1111/spc3.12362
McMahon, S. (2015). Call for research on bystander intervention to prevent sexual violence: the role of campus environments. Am. J. Commun. Psychol. 55, 472–489. doi: 10.1007/s10464-015-9724-0
Mendes, A., Lopez-Valeiras, E., and Lunkes, R. J. (2017). Pluralistic ignorance: conceptual framework, antecedents and consequences. Intangible Capital 13, 781–804. doi: 10.3926/ic.1063
Merton, R. K. (1936). The unanticipated consequences of purposive social action. Am. Sociol. Rev. 1, 894–904. doi: 10.2307/2084615
Miller, D. T. (2023). A century of pluralistic ignorance: what we have learned about its origins, forms, and consequences. Front. Soc. Psychol. 1:1260896. doi: 10.3389/frsps.2023.1260896
Miller, D. T., and McFarland, C. (1987). Pluralistic ignorance: when similarity is interpreted as dissimilarity. J. Pers. Soc. Psychol. 53, 298–305. doi: 10.1037/0022-3514.53.2.298
Miller, D. T., and McFarland, C. (1991). “When social comparison goes awry: the case of pluralistic ignorance,” in Social Comparison: Contemporary Theory and Research, eds. J. Suls, and T. A. Wills (Lawrence Erlbaum Associates, Inc.), 287–313. doi: 10.4324/9781003469490-14
Miller, D. T., and Nelson, L. D. (2002). Seeing approach motivation in the avoidance behavior of others: implications for an understanding of pluralistic ignorance. J. Pers. Soc. Psychol. 83, 1066–1075. doi: 10.1037/0022-3514.83.5.1066
Miller, G. A. (1956). The magical number seven, plus or minus two: Some limits on our capacity for processing information. Psychol. Rev. 63:81. doi: 10.1037/h0043158
Miller, M. K., and Cabell, J. J. (2024). “Confirmation bias, cognitive dissonance, and the COVID-19 pandemic,” in The Social Science of the COVID-19 Pandemic: A Call to Action for Researchers (Oxford University Press). doi: 10.1093/oso/9780197615133.003.0006
Miller, R., and Lammas, N. (2010). Social media and its implications for viral marketing. Asia Pacific Public Relat. J. 11, 1–9.
Momennejad, I. (2022). Collective minds: social network topology shapes collective cognition. Philos. Trans. R. Soc. B 377:20200315. doi: 10.1098/rstb.2020.0315
Moore, D. (2018). Overconfidence: The mother of all biases. Psychology Today. Available online at: https://www.psychologytoday.com/intl/blog/perfectly-confident/201801/overconfidence (accessed May 6, 2025).
Moore, D., and Healy, P. (2008). The trouble with overconfidence. Psychol. Rev. 115, 502–517. doi: 10.1037/0033-295X.115.2.502
Moy, P., Domke, D., and Stamm, K. (2001). The spiral of silence and public opinion on affirmative action. Journal. Mass Commun. Quart. 78, 7–25. doi: 10.1177/107769900107800102
Mueller, J. (1993). “American public opinion and the Gulf War,” in The Political Psychology of the Gulf War: Leaders, Publics, and the Process of Conflict, ed. S. Renshon (Pittsburgh: University of Pittsburgh Press), 199–226. doi: 10.2307/j.ctv25m88qk.16
Murre, J. M. J., and Dros, J. (2015). Replication and analysis of ebbinghaus”? forgetting curve. PLoS ONE 10, 1–23. doi: 10.1371/journal.pone.0120644
Nakashima, E. (2019). US Cyber Command operation disrupted Internet access of Russian troll factory on day of 2018 midterms. Washington Post, 27. Available online at: https://www.washingtonpost.com/world/national-security/us-cyber-command-operation-disrupted-internet-access-of-russian-troll-factory-on-day-of-2018-midterms/2019/02/26/1827fc9e-36d6-11e9-af5b-b51b7ff322e9_story.html (accessed May 6, 2025).
Nguyen, T. T., Quoc Viet hung, N., Nguyen, T. T., Huynh, T. T., Nguyen, T. T., Weidlich, M., et al. (2024). Manipulating recommender systems: a survey of poisoning attacks and countermeasures. ACM Comput. Surv. 57, 1–39. doi: 10.1145/3677328
Nichols, T. (2017). The Death of Expertise, The Campaign Against Established Knowledge and Why it Matters. Oxford University Press. Available online at: https://global.oup.com/academic/product/the-death-of-expertise-9780190865979 (accessed May 6, 2025).
Nickerson, R. S. (1998). Confirmation bias: a ubiquitous phenomenon in many guises. Rev. General Psychol. 2, 175–220. doi: 10.1037/1089-2680.2.2.175
Nir, L. (2011). Motivated reasoning and public opinion perception. Public Opin. Q. 75, 504–532. doi: 10.1093/poq/nfq076
Noelle-Neumann, E. (1993). The Spiral of Silence: Public Opinion, Our Social Skin. University of Chicago Press. Available online at: https://press.uchicago.edu/ucp/books/book/chicago/S/bo3684069.html (accessed May 6, 2025).
Norman, G. R., Monteiro, S. D., Sherbino, J., Ilgen, J. S., Schmidt, H. G., and Mamede, S. (2017). The causes of errors in clinical reasoning: cognitive biases, knowledge deficits, and dual process thinking. Acad. Med. 92, 23–30. doi: 10.1097/ACM.0000000000001421
Nyhan, B. (2021). Why the backfire effect does not explain the durability of political misperceptions. Proc. Nat. Acad. Sci. 118:e1912440117. doi: 10.1073/pnas.1912440117
Nyhan, B., and Reifler, J. (2010). When corrections fail: the persistence of political misperceptions. Polit. Behav. 32, 303–330. doi: 10.1007/s11109-010-9112-2
Nyhan, B., Settle, J., Thorson, E., Wojcieszak, M., Barberá, P., Annie, Y., et al. (2023). Like-minded sources on Facebook are prevalent but not polarizing. Nature 620, 137–144. doi: 10.1038/s41586-023-06297-w
O'Gorman, H. J. (1988). “The uniqueness bias: studies of constructive social comparison,” in Surveying social life: Papers in honor of Herbert H. Hyman (Wesleyan University Press), 149–176.
Okholm, C. S., Fard, A. E., and ten Thij, M. (2024). Blocking the information war? Testing the effectiveness of the EU's censorship of Russian state propaganda among the fringe communities of Western Europe. Internet Policy Rev. 13, 1–21. doi: 10.14763/2024.3.1788
Orchinik, R., Martel, C., Rand, D. G., and Bhui, R. (2023). Uncommon Errors: Adaptive Intuitions in High-Quality Media Environments Increase Susceptibility to Misinformation. Technical report, Center for Open Science. doi: 10.31234/osf.io/q7r58
Page, L. (2023). Reassessing the Confirmation Bias. Is it a flaw or an efficient strategy? Optimally Irrational newsletter. Available online at: https://www.optimallyirrational.com/p/reassessing-the-confirmation-bias (accessed May 6, 2025).
Paperin, G., Green, D. G., and Sadedin, S. (2011). Dual-phase evolution in complex adaptive systems. J. R. Soc. Interf. 8, 609–629. doi: 10.1098/rsif.2010.0719
Pariser, E. (2011). The filter bubble: What the Internet is hiding from you. Penguin, United Kingdom. Available online at: https://www.penguin.com.au/books/the-filter-bubble-9780141969923 (accessed May 6, 2025).
Pastor-Satorras, R., Castellano, C., Van Mieghem, P., and Vespignani, A. (2015). Epidemic processes in complex networks. Rev. Mod. Phys. 87, 925–979. doi: 10.1103/RevModPhys.87.925
Peterson, A. J. (2025). AI and the problem of knowledge collapse. AI Soc. 2025, 1–21. doi: 10.1007/s00146-024-02173-x
Piaget, J. (2013). Play, Dreams and Imitation in Childhood. London: Routledge. doi: 10.4324/9781315009698
Pies, R. W. (2017a). ‘Alternative facts': A Psychiatrist's Guide to Distorted Reality. Live Science. Available online at: https://www.livescience.com/58320-psychiatrist-guide-to-distorted-reality.html (accessed May 6, 2025).
Pies, R. W. (2017b). ”Alternative facts”: A psychiatrist's guide to twisted relationships to truth. The Conversation. Available online at: https://theconversation.com/alternative-facts-a-psychiatrists-guide-to-twisted-relationships-to-truth-72469 (accessed May 6, 2025).
Pilgrim, C., Sanborn, A., Malthouse, E., and Hills, T. T. (2024). Confirmation bias emerges from an approximation to Bayesian reasoning. Cognition 245:105693. doi: 10.1016/j.cognition.2023.105693
Plavén-Sigray, P., Matheson, G. J., Schiffler, B. C., and Thompson, W. H. (2017). The readability of scientific texts is decreasing over time. Elife 6:e27725. doi: 10.7554/eLife.27725
Prentice, D. A., and Miller, D. T. (1993). Pluralistic ignorance and alcohol use on campus: some consequences of misperceiving the social norm. J. Pers. Soc. Psychol. 64, 243–256. doi: 10.1037/0022-3514.64.2.243
Prentice, D. A., and Miller, D. T. (1996). “Pluralistic ignorance and the perpetuation of social norms by unwitting actors,” in Advances in Experimental Social Psychology, ed. M. P. Zanna (New York: Academic Press), 161–209. doi: 10.1016/S0065-2601(08)60238-5
Press W. H. Dyson F. J. (2012). Iterated prisoner's dilemma contains strategies that dominate any evolutionary opponent. Proc. Nat. Acad. Sci. 109, 10409–10413. doi: 10.1073/pnas.1206569109
Qin, T., and Burgoon, J. K. (2007). “An investigation of heuristics of human judgment in detecting deception and potential implications in countering social engineering,” in 2007 IEEE Intelligence and Security Informatics, 152–159. doi: 10.1109/ISI.2007.379548
Rafail, P., O'Connell, W. E., and Sager, E. (2024). Polarizing Feedback Loops on Twitter: Congressional Tweets during the 2022 Midterm Elections. Socius 10:23780231241228924. doi: 10.1177/23780231241228924
Ramachandran, V. S. (1996). The evolutionary biology of self-deception, laughter, dreaming and depression: some clues from anosognosia. Med. Hypotheses 47, 347–362. doi: 10.1016/S0306-9877(96)90215-7
RAND, Corporation. (2019). Fighting Disinformation Online: A Database of Web Tools. Technical report, Countering Truth Decay Initiative, RAND Corporation. Available online at: https://www.rand.org/research/projects/truth-decay/fighting-disinformation.html (accessed May 6, 2025).
Reiber, C., and Garcia, J. R. (2010). Hooking up: gender differences, evolution, and pluralistic ignorance. Evol. Psychol. 8, 390–404. doi: 10.1177/147470491000800307
Ribeiro, M. H., Jhaver, S., i Martinell, J. C., Reignier-Tayar, M., and West, R. (2024). Deplatforming norm-violating influencers on social media reduces overall online attention toward them. arXiv:2401.01253.
Riggs, D. W., and Bartholomaeus, C. (2018). Gaslighting in the context of clinical interactions with parents of transgender children. Sexual Relationship Ther. 33, 382–394. doi: 10.1080/14681994.2018.1444274
Rijpma, J. A. (2019). “Complexity, tight-coupling and reliability: Connecting normal accidents theory and high reliability theory,” in Risk Management (Routledge), 149–157. doi: 10.4324/9780429282515-10
Rocavert, C. (2019). Retrieving truth in a post-truth world: drama in the age of reality entertainment. Int. J. Crit. Cult. Stud. 17, 11–26. doi: 10.18848/2327-0055/CGP/v17i01/11-26
Rollwage, M., Loosen, A., Hauser, T., Moran, R., Dolan, R., and Fleming, S. (2020). Confidence drives a neural confirmation bias. Nat. Commun. 11:2634. doi: 10.1038/s41467-020-16278-6
Roozenbeek, J., van der Linden, S., Goldberg, B., Rathje, S., and Lewandowsky, S. (2022). Psychological inoculation improves resilience against misinformation on social media. Sci. Adv. 8:eabo6254. doi: 10.1126/sciadv.abo6254
Ross, L., Greene, D., and House, P. (1977). The “false consensus effect”: an egocentric bias in social perception and attribution processes. J. Exp. Soc. Psychol. 13, 279–301. doi: 10.1016/0022-1031(77)90049-X
Rubin, R. S., and Dierdorff, E. C. (2011). On the road to Abilene: time to manage agreement about MBA curricular relevance. Acad. Manag. Learn. Educ. 10, 148–161. doi: 10.5465/AMLE.2011.59513280
Ruffo, G., Semeraro, A., Giachanou, A., and Rosso, P. (2023). Studying fake news spreading, polarisation dynamics, and manipulation by bots: a tale of networks and language. Comput. Sci. Rev. 47:100531. doi: 10.1016/j.cosrev.2022.100531
Rutjens, B. T., Heine, S. J., Sutton, R. M., and van Harreveld, F. (2018). Attitudes towards science. Adv. Exp. Soc. Psychol. 57, 125–165. doi: 10.1016/bs.aesp.2017.08.001
Sadeghi, M., Dimitriadis, D., and Wollen, M. (2024). Top 10 Generative AI Models Mimic Russian Disinformation Claims A Third of the Time, Citing Moscow-Created Fake Local News Sites as Authoritative Sources. Tech Report 18, NewsGuard Technologies. Available online at: https://www.newsguardtech.com/special-reports/generative-ai-models-mimic-russian-disinformation-cite-fake-news/ (accessed May 6, 2025).
Sargent, R. H., and Newman, L. S. (2021). Pluralistic ignorance research in psychology: a scoping review of topic and method variation and directions for future research. Rev. General Psychol. 25, 163–184. doi: 10.1177/1089268021995168
Sarno, D. M., McPherson, R., and Neider, M. B. (2022). Is the key to phishing training persistence? Developing a novel persistent intervention. J. Exper. Psychol. 28:85. doi: 10.1037/xap0000410
Schafer, M., and Crichlow, S. (1996). Antecedents of groupthink: a quantitative study. J. Conflict Resol. 40, 415–435. doi: 10.1177/0022002796040003002
Schwartz, R., Vassilev, A., Greene, K., Perine, L., Burt, A., and Hall, P. (2022). Towards a standard for identifying and managing bias in artificial intelligence, volume 3. US Department of Commerce, National Institute of Standards and Technology. doi: 10.6028/NIST.SP.1270
Schwartz, S. H., and Gottlieb, A. (1976). Bystander reactions to a violent theft: Crime in Jerusalem. J. Pers. Soc. Psychol. 34, 1188–1199. doi: 10.1037/0022-3514.34.6.1188
Scott, J. (2012). What is Social Network Analysis? London: Bloomsbury Academic. doi: 10.5040/9781849668187
Seeme, F. (2020). Agent Based Modelling of Pluralistic Ignorance in Social Systems. PhD thesis, Faculty of Information Technology, Monash University. Available online at: https://bridges.monash.edu/articles/thesis/Agent_Based_Modelling_of_Pluralistic_Ignorance_in_Social_Systems/14714775 (accessed May 6, 2025).
Seeme, F. B., and Green, D. G. (2016). “Pluralistic ignorance: emergence and hypotheses testing in a multi-agent system,” in 2016 International Joint Conference on Neural Networks (IJCNN), 5269–5274. doi: 10.1109/IJCNN.2016.7727896
Shannon, C. E. (1948). A mathematical theory of communication. Bell Syst. Tech. J. 27, 379–423. doi: 10.1002/j.1538-7305.1948.tb01338.x
Shao, C., Ciampaglia, G. L., Varol, O., Yang, K.-C., Flammini, A., and Menczer, F. (2018). The spread of low-credibility content by social bots. Nat. Commun. 9, 1–9. doi: 10.1038/s41467-018-06930-7
Shaw-Ching Liu, B., Madhavan, R., and Sudharshan, D. (2005). DiffuNET: the impact of network structure on diffusion of innovation. Eur. J. Innov. Manage. 8, 240–262. doi: 10.1108/14601060510594701
Shumailov, I., Shumaylov, Z., Zhao, Y., Papernot, N., Anderson, R., and Gal, Y. (2024). AI models collapse when trained on recursively generated data. Nature 631, 755–759. doi: 10.1038/s41586-024-07566-y
Simchon, A., Edwards, M., and Lewandowsky, S. (2024). The persuasive effects of political microtargeting in the age of generative artificial intelligence. PNAS Nexus 3:35. doi: 10.1093/pnasnexus/pgae035
Sims, R. R. (1992). Linking groupthink to unethical behavior in organizations. J. Bus. Ethics 11, 651–662. doi: 10.1007/BF01686345
Smith, E. B., Brands, R. A., Brashears, M. E., and Kleinbaum, A. M. (2020). Social networks and cognition. Annu. Rev. Sociol. 46, 159–174. doi: 10.1146/annurev-soc-121919-054736
Smith, L., Thomas, E., Bliuc, A.-M., and McGarty, C. (2024). Polarization is the psychological foundation of collective engagement. Commun. Psychol. 2:41. doi: 10.1038/s44271-024-00089-2
Song, J., and Oh, I. (2017). Investigation of the bystander effect in school bullying: Comparison of experiential, psychological and situational factors. Sch. Psychol. Int. 38, 319–336. doi: 10.1177/0143034317699997
Soprano, M., Roitero, K., La Barbera, D., Ceolin, D., Spina, D., Demartini, G., et al. (2024). Cognitive biases in fact-checking and their countermeasures: a review. Inf. Proc. Manag. 61:103672. doi: 10.1016/j.ipm.2024.103672
Stagnaro, M. N., Tappin, B. M., and Rand, D. G. (2023). No association between numerical ability and politically motivated reasoning in a large US probability sample. Proc. Nat. Acad. Sci. 120:e2301491120. doi: 10.1073/pnas.2301491120
Stocker, R., Green, D., and Newth, D. (2001). Consensus and cohesion in simulated social networks. J. Artif. Soc. Soc. Simul. 4, 1–8.
Strickland, A. A., Taber, C. S., and Lodge, M. (2011). Motivated reasoning and public opinion. J. Health Polit. Policy Law 36, 935–944. doi: 10.1215/03616878-1460524
Stroud, N. J. (2011). Niche News: The Politics of News Choice. Oxford: Oxford University Press. doi: 10.1093/acprof:oso/9780199755509.001.0001
Suls, J., and Wan, C. K. (1987). In search of the false-uniqueness phenomenon: fear and estimates of social consensus. J. Pers. Soc. Psychol. 52, 211–217. doi: 10.1037/0022-3514.52.1.211
Sweet, P. L. (2019). The sociology of gaslighting. Am. Sociol. Rev. 84, 851–875. doi: 10.1177/0003122419874843
Sweller, J. (1988). Cognitive load during problem solving: effects on learning. Cogn. Sci. 12, 257–285. doi: 10.1207/s15516709cog1202_4
Taber, C. S., and Lodge, M. (2006). Motivated skepticism in the evaluation of political beliefs. Am. J. Polit. Sci. 50, 755–769. doi: 10.1111/j.1540-5907.2006.00214.x
Takeuchi, T., Tamura, M., Tse, D., Kajii, Y., Fernández, G., and Morris, R. G. (2022). Brain region networks for the assimilation of new associative memory into a schema. Mol. Brain 15:24. doi: 10.1186/s13041-022-00908-9
Tang, T., and Chorus, C. G. (2019). Learning opinions by observing actions: simulation of opinion dynamics using an action-opinion inference model. J. Artif. Societ. Soc. Simulat. 22:2. doi: 10.18564/jasss.4020
Tappin, B. M., Pennycook, G., and Rand, D. G. (2021). Rethinking the link between cognitive sophistication and politically motivated reasoning. J. Exp. Psychol. 150, 1095–1114. doi: 10.1037/xge0000974
Taylor, D. G. (1982). Pluralistic ignorance and the spiral of silence: a formal analysis. Public Opin. Q. 46, 311–335. doi: 10.1086/268729
Taylor, S. E., and Brown, J. D. (1988). Illusion and well-being: a social psychological perspective on mental health. Psychol. Bull. 103, 193–210. doi: 10.1037/0033-2909.103.2.193
Toma, C. L., Bonus, J. A., and Van Swol, L. M. (2019). “Lying online: Examining the production, detection, and popular beliefs surrounding interpersonal deception in technologically-mediated environments,” in The Palgrave Handbook of Deceptive Communication, eds. T. Docan-Morgan (Cham: Springer International Publishing), 583–601. doi: 10.1007/978-3-319-96334-1_31
Törnberg, P. (2018). Echo chambers and viral misinformation: modeling fake news as complex contagion. PLoS ONE 13, 1–21. doi: 10.1371/journal.pone.0203958
Treen, K. M. d, Williams, H. T. P., and O'Neill, S. J. (2020). Online misinformation about climate change. WIREs Clim. Change 11:e665. doi: 10.1002/wcc.665
Trivers, R. (2000). The elements of a scientific theory of self-deception. Ann. N. Y. Acad. Sci. 907, 114–131. doi: 10.1111/j.1749-6632.2000.tb06619.x
Tsugawa, S., and Ohsaki, H. (2014). “Emergence of fractals in social networks: analysis of community structure and interaction locality,” in 2014 IEEE 38th Annual Computer Software and Applications Conference, 568–575. doi: 10.1109/COMPSAC.2014.80
Tufekci, Z. (2017). Twitter and tear gas: The power and fragility of networked protest. Yale University Press. Available online at: https://yalebooks.yale.edu/book/9780300234176/twitter-and-tear-gas/ (accessed May 6, 2025).
Tufekci, Z., and Wilson, C. (2012). Social media and the decision to participate in political protest: observations from Tahrir Square. J. Commun. 62, 363–379. doi: 10.1111/j.1460-2466.2012.01629.x
Turner, M. E., and Pratkanis, A. R. (1998). A social identity maintenance model of groupthink. Organ. Behav. Hum. Decis. Process. 73, 210–235. doi: 10.1006/obhd.1998.2757
Turner, M. E., Pratkanis, A. R., Probasco, P., and Leve, C. (1992). Threat, cohesion, and group effectiveness: testing a social identity maintenance perspective on groupthink. J. Pers. Soc. Psychol. 63:781. doi: 10.1037//0022-3514.63.5.781
Tversky, A., and Kahneman, D. (1974). Judgment under uncertainty: heuristics and biases: Biases in judgments reveal some heuristics of thinking under uncertainty. Science 185, 1124–1131. doi: 10.1126/science.185.4157.1124
Tversky, A., and Kahneman, D. (1983). Extensional versus intuitive reasoning: the conjunction fallacy in probability judgment. Psychol. Rev. 90, 293–315. doi: 10.1037/0033-295X.90.4.293
Ubaldi, E., Burioni, R., Loreto, V., and Tria, F. (2021). Emergence and evolution of social networks through exploration of the adjacent possible space. Commun. Physics.4:28. doi: 10.1038/s42005-021-00527-1
Vaidya, R., and Karnawat, T. (2023). Conceptualizing influencer marketing: a literature review on the strategic use of social media influencers. Int. J. Manag. Public Policy Res. 2, 81–86. doi: 10.55829/ijmpr.v2iSpecialIssue.140
Valdez, L. D., Shekhtman, L., La Rocca, C. E., Zhang, X., Buldyrev, S. V., Trunfio, P. A., et al. (2020). Cascading failures in complex networks. J. Complex Netw. 8:cnaa013. doi: 10.1093/comnet/cnaa013
van de Bongardt, D., Reitz, E., Sandfort, T., and Deković, M. (2015). A meta-analysis of the relations between three types of peer norms and adolescent sexual behavior. Person. Soc. Psychol. Rev. 19, 203–234. doi: 10.1177/1088868314544223
Verschuere, B., Lin, C.-C., Huismann, S., Kleinberg, B., Willemse, M., Mei, E., et al. (2023). The use-the-best heuristic facilitates deception detection. Nat. Hum. Behav. 7, 718–728. doi: 10.1038/s41562-023-01556-2
Vicente, L., and Matute, H. (2023). Humans inherit artificial intelligence biases. Nat. Sci. Rep. 13:15737. doi: 10.1038/s41598-023-42384-8
von Hippel, W., and Trivers, R. (2011). The evolution and psychology of self-deception. Behav. Brain Sci. 34, 1–16. doi: 10.1017/S0140525X10001354
Vosoughi, S., Roy, D., and Aral, S. (2018). The spread of true and false news online. Science 359, 1146–1151. doi: 10.1126/science.aap9559
Vykopal, I., Pikuliak, M., Srba, I., Moro, R., Macko, D., and Bielikova, M. (2024). “Disinformation capabilities of large language models,” in Proceedings of the 62nd Annual Meeting of the Association for Computational Linguistics (Volume 1: Long Papers), eds. L.-W. Ku, A. Martins, and V. Srikumar (Bangkok, Thailand: Association for Computational Linguistics), 14830–14847. doi: 10.18653/v1/2024.acl-long.793
Watts, D. J., and Strogatz, S. H. (1998). Collective dynamics of “small-world” networks. Nature 393, 440–442. doi: 10.1038/30918
Waytz, A., Epley, N., and Cacioppo, J. T. (2010). Social cognition unbound: Insights into anthropomorphism and dehumanization. Curr. Dir. Psychol. Sci. 19, 58–62. doi: 10.1177/0963721409359302
Winchester, S. (2023). Knowing What We Know: The Transmission of Knowledge: From Ancient Wisdom to Modern Magic. Dublin: Harper Collins.
Wolfsfeld, G., Segev, E., and Sheafer, T. (2013). Social media and the Arab Spring: Politics comes first. Int. J. Press/Politics 18, 115–137. doi: 10.1177/1940161212471716
Wu, Z., Menichetti, G., Rahmede, C., and Bianconi, G. (2015). Emergent complex network geometry. Sci. Rep. 5:10073. doi: 10.1038/srep10073
Xiao, C., Freeman, D. M., and Hwa, T. (2015). “Detecting clusters of fake accounts in online social networks,” in Proceedings of the 8th ACM Workshop on Artificial Intelligence and Security, AISec'15 (New York, NY, USA: Association for Computing Machinery), 91–101. doi: 10.1145/2808769.2808779
Yang, S., Ali, M. A., Yu, L., Hu, L., and Wang, D. (2024). MONAL: model autophagy analysis for modeling human-AI interactions. arXiv preprint arXiv:2402.11271.
Zhong, W. (2022). Optimal dynamic information acquisition. Econometrica 90, 1537–1582. doi: 10.3982/ECTA17787
Zhou, J., Liu, Z., and Li, B. (2007). Influence of network structure on rumor propagation. Phys. Lett. A 368, 458–463. doi: 10.1016/j.physleta.2007.01.094
Zhuravskaya, E., Petrova, M., and Enikolopov, R. (2020). Political effects of the internet and social media. Annu. Rev. Econom. 12, 415–438. doi: 10.1146/annurev-economics-081919-050239
Appendix-I: Common dysfunctional biases and behaviours
Keywords: cognition, cognitive error, social cognitive error, social network, artificial intelligence, emergence, complex systems, gaslighting
Citation: Seeme F, Green D and Kopp C (2025) Ignorance of the crowd: dysfunctional thinking in social networks. Front. Commun. 10:1547489. doi: 10.3389/fcomm.2025.1547489
Received: 18 December 2024; Accepted: 22 April 2025;
Published: 19 May 2025.
Edited by:
Tobias Eberwein, Austrian Academy of Sciences (OeAW), AustriaReviewed by:
Thseen Nazir, Ibn Haldun University, TürkiyeGabriela Zago, Federal University of Pelotas, Brazil
Copyright © 2025 Seeme, Green and Kopp. This is an open-access article distributed under the terms of the Creative Commons Attribution License (CC BY). The use, distribution or reproduction in other forums is permitted, provided the original author(s) and the copyright owner(s) are credited and that the original publication in this journal is cited, in accordance with accepted academic practice. No use, distribution or reproduction is permitted which does not comply with these terms.
*Correspondence: Carlo Kopp, Q2FybG8uS29wcEBNb25hc2guZWR1