- 1Social Cognitive Systems Group, Centre for Cognitive Interaction Technology (CITEC), Bielefeld University, Bielefeld, Germany
- 2Social Psychology, Media and Communication, University of Duisburg-Essen, Duisburg, Germany
In this paper, we present the results of our long-term study on use of a voice assistant (Amazon Alexa via Amazon Echo Dot) in nine families with children and no previous experience with this technology. The study was conducted over the course of 5 weeks during which the families could interact with the device freely. Three house visits were made to collect empirical data from the adult participants in form of questionnaires. Additionally, conversational data from log files of the voice assistant were obtained. These data were annotated and analyzed with a focus on communication breakdowns during human-assistant interaction. We investigate user behavior for both adults and children in such situations, its reasons and consequences for user satisfaction. This article provides qualitative analysis of three particularly interesting breakdown cases, as well as statistical analysis along several hypotheses and research questions combining empirical and conversational data. Described cases of communication breakdown illustrate findings from existing literature on the topic. The statistical analysis paints a mixed picture, however, it helped us identify further avenues for research, some of which can be explored with our data set in the future. We found a significant negative effect of the number of abandoned failed requests on user satisfaction, contrary to the number of successfully repaired requests that had no influence on user satisfaction. We discovered that users are more inclined to use reformulation as repair strategy when they do not perceive the emergence of miscommunication as their fault. We could not identify a significant effect of internal reasons for the choice of other strategies, so we suggest that situational clues such as the immediate response of the voice assistant are more important for the choice of repair strategy. Our results also hint that users distinguish between repair strategies differently, as the self-perceived frequency of repetitions and abortions of requests were found to be positive predictors for the use of reformulation-based strategies. With regards to the long-term aspect of the study, use of repetition as a repair strategy by both children and adults significantly decreased with time, no other changes were found for other strategies. Additionally, no significant impact of age on the choice of repair strategy was found, as well as no interaction effect between age and time.
1. Introduction
Voice assistants (VAs) continue to become part of the daily life for more and more people. Apart from their integration in smartphones, the sales of smart speakers are high and predicted to rise even more in the future. Tenzer (2021), for instance, puts the amount of smart speakers to be sold worldwide in the year 2025 at 205 million devices. It is therefore important to understand the phenomenon of voice assistants, as for many people it is or will be their major experience in speech-based human-machine interaction that will influence their perception, expectations and behavior toward this technology. Analyzing current patterns of use, problems and shortcomings of voice assistants can also help us build truly conversational agents for a variety of tasks beyond question answering. It is also important to investigate these aspects with regards to various demographic groups, such as children or the elderly, as they have specific qualities and requirements that demand specialized approaches to technology design.
As shown in Szczuka et al. (2021), children specifically can be counted as a vulnerable group of users who might lack knowledge and understanding to be sufficiently informed about the functionality of voice assistants and various legal and ethical consequences of their use with regards to topics such as data security and processing. They also often face technical difficulties during interactions, as children's speech contains more pauses, repetitions, speaker-initiated repairs, ungrammatical utterances and other inconsistencies than adult speech. Additionally, children have higher-pitched voices due to a shorter vocal tract. All of these features decrease the performance of common speech recognition systems, as shown in Kennedy et al. (2017). While there are efforts to increase speech recognition performance to account for children's acoustic variability, such as Dubagunta et al. (2019), Gale et al. (2019), Wu et al. (2019), or Shivakumar and Georgiou (2020), other above-mentioned inconsistencies can still prevent speech-based systems from understanding or fulfilling the communicative intent of a child in spite of the low word recognition error rate, which could be observed in the study by Lovato et al. (2019). Despite all these issues, a lot of children have access to and engage with voice assistants in their everyday lives. According to a survey cited in Kats (2018), more than nine in ten children aged 4–11 have access to a VA and out of these, 26 per cent engage with it between 2 and 4 h a week and 20 per cent more than 5 h. This study also found that an overwhelming majority of children used voice assistants on smart speakers whereas only about a half of teenagers did that. These figures highlight the relevance of research on children interacting with voice assistants and the impact these interactions have on their lives.
One has to additionally consider that when things go wrong in an interaction with a voice assistant, the burden to repair the communication breakdown and to ensure the understanding of own utterances generally falls to the users. Commercially available VAs can be seen as “black boxes” that provide little indication as to the source of the breakdown and force users to rely on their own experiences, intuition and expectations in order to find a solution to the problem, which is supported by various studies such as Luger and Sellen (2016), Myers et al. (2018), Porcheron et al. (2018), Beneteau et al. (2019), Cho and Rader (2020).
We have conducted a long-term study during which families with no previous VA ownership interacted with one (Amazon Alexa) over the course of 5 weeks. Google (Kleinberg, 2018) reports that parents use smart speakers more than non-parents as part of their daily routine and for multitasking, which supports our idea that families with children are an important environment where voice assistant technology is and will be used in the future and therefore represents an important subject for scientific research. During the course of our study, we acquired both conversational data as recorded in Alexa log files and empirical data from questionnaires filled out by the participants. By combining these data we would like to understand what internal factors might influence the users' choice of behavior in miscommunication situations in the under-informed context of interaction with a “black box”, as in our opinion there is still a research gap there. Moreover, there is little literature discussing the possible differences between children and adults in the context of miscommunication in long-term interactions with VAs, something that we, too, can address with our data. This is important as the communicative experience, patterns of use and perception of voice assistants vary between children and adults, as can be seen, for example, in Garg and Sengupta (2020). They report that while adults primarily use smart speakers for listening to music and automating tasks, children are more likely to seek knowledge, play or engage in small talk or emotional conversations with the device. These latter kinds of interactions might lead to communication breakdowns more frequently, as they can require capabilities beyond simple request-response format, such as context-awareness and memory over past interactions. The authors also found younger children to have a tendency to ascribe human-like characteristics to the device which might further influence their communicative behavior.
Additionally, we are also interested in the impact that communication breakdowns and the success of their resolution have on user satisfaction with the assistant. As will be shown in the next section, over time users often reduce their interactions with a VA to a minimum (Cho et al., 2019) and one of the reasons for it may be the mismatch between the expectations of the users with regards to the conversational abilities of the devices and the reality. By understanding where exactly these capabilities fail and how communication between the user and the VA can be improved, the feeling of disappointment can be reduced in users which may lead to a better adoption of the technology in the future.
In the next section, we will explain how all these research questions tie in with the existing research on voice assistants and how our specific research questions emerge from it. Further on, we will provide a more detailed description of our study and the process of data preparation for statistical analysis and then present the results of both qualitative and quantitative analysis. Finally, we will discuss our results and their implications for the design of voice-based interaction technology.
2. Related Work and Research Questions
2.1. Expectations With Regards to Conversational Abilities of VAs and Their Influence on User Satisfaction
Based on the results of their long-term study of Amazon Echo usage in various households and pre-existing models of technology adoption, Cho et al. (2019) identified five phases of Amazon Alexa use consisting of pre-adoption, adoption, adaptation, stagnation, and acceptance. These phases describe a pattern of user's journey from having vague and mostly inaccurate ideas about the VA pre-adoption over exploration ending in disappointment, lower expectations, and negative role recognition of the assistant up to the loss of interest resulting in minimal use for simple functions or, in extreme cases, abandonment of the device. Among possible reasons for the establishment of this pattern is the mismatch between the expectations for conversational abilities of the voice assistant and its actual capabilities. Luger and Sellen (2016) and Cho et al. (2019) report that users with more experience with technology have more realistic ideas about the assistant, but those with less experience would mostly draw their expectations from familiar characteristics of human-human interaction due to insufficient information provided by the system itself, which eventually leads to disappointment at the lack of human-like conversational abilities in the assistant, such as its inability to acknowledge context across several temporally adjacent turns or understand more natural colloquial ways of communication. It could be argued, for example, based on findings of Porcheron et al. (2018) that voice assistants are currently not designed to act as conversation partners, but rather provide one-shot request-response interactions which users embed into conversational situations within human-human interaction domain, e.g., in the family context. It leads to a differing treatment of voice assistants and characterization of such interactions as can be seen from the interviews in Clark et al. (2019) where users mostly describe concepts relating to conversations with virtual agents in a functional way in contrast to human-human conversations that are described in more social terms. Along with these differences in perception, users may also change the way they speak to the assistant by, for instance, simplifying their utterances, removing excess words that do not function as keywords, or altering their prosody, a phenomenon Luger and Sellen (2016) call the “economy of language”. Siegert and Krüger (2018) investigated in their study differences in speaking style between human-human and human-assistant communication. All of their participants reported changes to their speaking style at some point during the interaction with the VA with regards to loudness, intonation and rhythm of speech. Analysis of the objective features of speech in general supported these self-assessments, yet differences in the amount of characteristics altered could be observed between types of tasks, suggesting overall variability of user speech style adaptation.
Even then, communication breakdowns still occur during interaction. Beyond the initial playful exploration phase, users are not particularly forgiving of failed interactions, which negatively affects the frequency of assistant use, as was reported in Luger and Sellen (2016). However, they also state that the more technically savvy users were more tolerant in cases of miscommunication and more persistent in their attempts to accomplish their tasks. They were also more likely to identify the causes of communication breakdowns, unlike less experienced users who were more likely to blame themselves and experience negative feelings as a result. The self-attribution of blame was also observed by Cho and Rader (2020) in cases where the voice assistant provided no clues as to the cause of miscommunication by giving the user a neutral non-understanding reaction (e.g., “Sorry, I don't know how to help with that yet, but I'm still learning”).
Our hypothesis H1 assumes the existence of these observations in our data. With the help of statistical methods, we would like to investigate how a set of particular internal characteristics of the user may influence user's success in the resolution of communication breakdowns.
H1: User's affinity for technology, the party they attribute the emergence of a communication breakdown to, and the emotions they experience when such a breakdown occurs serve as predictors for the number of abandoned failed requests (H1a) and successfully repaired requests (H1b). Hereby, we expect users with lower affinity for technology, users that attribute communication breakdowns to own mistakes and users experiencing negative emotions during breakdowns to abandon requests more frequently and achieve successful resolution of miscommunication less frequently.
Other studies with adult participants such as Jiang et al. (2015), Kiseleva et al. (2016) and the analysis of Amazon Echo reviews by Purington et al. (2017) show that various aspects connected to communication breakdowns, such as the quality of speech recognition, occurrence of technical errors or effort required to accomplish a task have an effect on user satisfaction with speech-based human-machine interactions. Purington et al. (2017) report that users who mentioned technical issues with the device in their reviews were significantly less satisfied with it. Jiang et al. (2015) found a significant positive correlation between intent recognition quality and user satisfaction across various tasks and a lower, but also a significant positive correlation between speech recognition quality and user satisfaction for certain types of tasks, such as device function and web search. The article of Kiseleva et al. (2016) also suggests that impact of certain aspects on user satisfaction varies based on the type of the underlying task, as they report a low correlation between user satisfaction and task accomplishment in use cases of information seeking, but high correlation in use cases of device control. Additionally, they found a strong negative correlation between perceived effort spent accomplishing a task and user satisfaction. Correspondingly, our hypothesis H2 assumes an impact of communication breakdown resolutions on user's satisfaction with the voice assistant.
H2: The number of abandoned failed requests negatively (H2a) and successfully repaired requests positively (H2b) influence user's satisfaction with the voice assistant.
Hereby, we assume abandoned requests to have a negative influence on user satisfaction as they correspond with unresolved issues in VA's understanding of user intent and potentially mean that the user deemed the cost of repairing the breakdown too high. We also hypothesize that successfully repaired requests have a positive effect on user satisfaction as they correspond with successfully accomplished tasks, especially if the user deemed the effort of repairing the breakdown acceptable in these situations.
2.2. Types of Communication Breakdowns in Speech-Based Human-Machine Interaction and Corresponding User Behavior
Various papers have addressed communication breakdowns in speech-based human-machine interaction in general and voice assistants in particular, for example, Stent et al. (2008), Jiang et al. (2013), Myers et al. (2018), Beneteau et al. (2019), Cho and Rader (2020), Motta and Quaresma (2022). These studies investigate the relationship between different strategies that users employ in the event of miscommunication and the types of underlying errors or system's responses to the user. System's responses are widely recognized as an important resource for the users to identify communication breakdowns and a fitting course of action.
The results of these studies are often consistent with each other, as Stent et al. (2008), Jiang et al. (2013), Myers et al. (2018) and Motta and Quaresma (2022) observe hyperarticulation or prosodic changes as one of the most common strategies employed by users, especially when they are faced with errors in speech recognition. Other commonly seen strategies mentioned in Jiang et al. (2013), Myers et al. (2018), Porcheron et al. (2018), Beneteau et al. (2019) and Motta and Quaresma (2022) include simplification of utterances, variations of the amount of information given to the system, semantic and syntactical adjustments to queries, repetition of commands. Users tend to explore these varied strategies when faced with errors seemingly not directly related to speech recognition, such as the system being unable to recognize the communicative intent of the user or to follow through on the recognized intent or performing a task that is different from the requested one.
Two of the above-mentioned studies have a unique focus. Beneteau et al. (2019) investigate interactions with a voice assistant as a joint family activity and look at various types of discourse scaffolding employed by family members to support each other during resolution of communication breakdowns, such as giving instructions or redirecting the interaction back to the desired conversation topic. Unfortunately, we cannot systematically examine this phenomenon with the data available through our study, since it requires extra recordings of conversations between family members that are not documented by the assistant. However, we could observe some cases of joint miscommunication repair in the conversational data, examples of which will be shown in the section 4.1.
Cho and Rader (2020) investigate the responses Google Home gives in miscommunication situations with regards to their advancement of conversational grounding between the system and the user and their helpfulness for the achievement of user goals. For this, they do not focus on specific repair strategies, but rather categorize user utterances into “advancing” and “backtracking” depending on whether the utterance seems to move the conversation closer to task completion or not. They find that the most common type of response observed from the Google Home, which can be categorized as the “Cannot Help” response (e.g., “Sorry, I don't know how to help with that yet, but I'm still learning”), provides the least clues to the user for the resolution of the communication breakdown, despite being a correct response in case of an error. On the contrary, “Unrelated” responses that are not connected to user's request and often occur when the system acts on own misunderstanding may provide more clues to the user for the cause of miscommunication or invite experimentation with regards to repair strategies.
While out of scope of this paper, another type of miscommunication can occur with the voice assistant, namely, accidental activation of the device and, subsequently, a false assumption about an unrelated user utterance being a request. This may lead to issues, especially, as modern VAs have extensive capabilities to make purchases, contact people via calls, etc. We have seen examples of falsely assumed requests that Alexa acted upon in our data, however, we do not focus on the analysis of such situations here. Work by other researchers is concerned with identifying potential reasons for accidental activation of the VA and how the amount of such cases can be decreased through improved addressee detection mechanisms. For example, Siegert (2021) analyzed examples of audio recordings of utterances incorrectly triggering Alexa and found that higher variety in intonation leads to more accidental activations.
Researchers are in agreement that users require better guidance from voice assistants in order to successfully accomplish tasks and resolve communication breakdowns, for example, Porcheron et al. (2018), Beneteau et al. (2019), Cho and Rader (2020) and Motta and Quaresma (2022), that currently available state-of-the-art systems are often unhelpful and act as “black boxes”, leaving users under-informed. We pose our first research question RQ1 to investigate the impact of internal factors such as personal estimation of causes for communication breakdowns and perception of own preferred cause of action in these situations on the actual behavior of users. While different papers mentioned in this section use slightly different ways to describe repair strategies in interaction with VAs, in our research, we decided to base our classification on the system used in Beneteau et al. (2019). Hereby, we grouped breakdown repair strategies into three categories, namely reformulation (group A), repetition (group B) and changes in articulation (group C) that are used for the research questions RQ1a-c respectively. More information on the repair strategies we identified in the conversational data can be found in section 3.2.
RQ1: Do user's attribution of communication breakdowns to specific factors and issues, along with their perception of their own behavior when such situations occur predict their choice of repair strategy (RQ1a-c) or abandonment of the query before attempting any repair in the first place (RQ1d)?
2.3. Children and Communication Breakdowns in Interactions With Voice Assistants
Prior research such as Gallagher (1977) suggests that children in general are persistent when it comes to establishing understanding and attempting communicative repairs. While adults were not the primary users in the study conducted by Cheng et al. (2018), but rather supported their children and thus did not have an immediate interest in pursuing task accomplishment, they gave up on the repair attempts faster than the children, perhaps because they were quicker to attribute the communication breakdown to a technical failure that could not be resolved. Further research is required to study potential differences in repair persistence between children and adults in equal scenarios.
When it comes to interactions with voice assistants, children employ a variety of repair strategies. Studies such as Lovato and Piper (2015), Druga et al. (2017), Cheng et al. (2018) and Yarosh et al. (2018) agree that the most commonly employed strategies are repetition of the initial query and increase in volume. However, children will also reword their requests or supplement them with additional context, especially if their initial repair attempts have failed. Lovato and Piper (2015), Druga et al. (2017), Cheng et al. (2018), Beneteau et al. (2019) and Garg and Sengupta (2020) also discuss the importance of discourse scaffolding mentioned in the previous subsection with regards to children and their repair strategies, as adults can encourage children to try out different approaches through suggestion or modeling and reinforce specific behaviors by giving their approval. However, Garg and Sengupta (2020) report that in their long-term study children learnt the interaction principles and required less help when using voice assistants after 2–3 months. It is also suggested that some of these scaffolding functions could be relegated to the assistant itself, the successful realization of which can be seen in Xu and Warschauer (2019) where the system was able to provide re-prompts that would constrain children's response options in case of miscommunication or to use follow-up questions to scaffold correct pronunciation of words and prevent communication breakdowns in the future.
Our research question RQ2 ties in with this research and investigates the effect of age (children vs. adults) and time spent using a voice assistant on user behavior and choice of repair strategies in case of communication breakdowns. Here again, we operate within the three categories of repair strategies A to C for research questions RQ2a-c respectively.
RQ2: Does user's age and the length of time they have interacted with a voice assistant predict their choice of repair strategy (RQ2a-c) or abandonment of the query before attempting repair (RQ2d)?
3. Study Description and Data Analysis
In order to investigate our hypotheses and research questions, we conducted a study during which families with children and no previous ownership of voice assistants interacted with an Amazon Alexa over the course of 5 weeks. This section describes the exact qualities of the data sample we obtained and the measures that were calculated from both empirical and conversational data.
3.1. Sample
In our study, ten families with twelve children received an Alexa Echo Dot device for 5 weeks. The study was conducted in Germany between mid-January and end of February 2020. Unfortunately, for one family, no log file data were retrieved. Therefore, calculations were done with nine complete datasets. Recruiting was carried out via local Facebook groups, the distribution of flyers and personal contacts. Families who wanted to participate must not have had previous voice assistant experience and must at least have one child between 6 and 12 years of age living in the household. Out of the nine families, one father was a single parent, whereas the remaining eight lived in heterosexual relationships, which adds up to a total of 17 adults. On average, the parents were 41.17 years old (SD = 5.37, Range: 31–48). In total, seven people had a degree below the German Abitur (A-levels, formerly 9 years of secondary school), whereas nine people finished their Abitur. One person had obtained a university degree. Thus, gender and education amongst adults were well-balanced and largely representative of the German population. Across the families, there was a total of 11 children, who were on average 8.91 years old (SD = 1.70, Range: 6–11). Further information on the age and gender of the children can be found in Table 1.
Throughout the 5 weeks, three home visits were carried out. The first one included the device's installation, encouragement of a first interaction, and running of the first questionnaire. Here, the families also provided their consent for participation and log file retrieval after they had been briefed appropriately. Afterwards, the parents filled in an online questionnaire including sociodemographic data and affinity for technology. In the second and third session they rated questions regarding situations when misunderstandings with the voice assistant occurred as well as their satisfaction with the device. If not differently stated, participants were asked to rate the questions as a mean for the entire household. These questionnaires can be found in Supplementary Materials to this paper. Further questionnaires which are of no relevance to this work were included but will not be described here in more detail. The study procedure was approved by the University of Duisburg-Essen ethics committee.
3.2. Annotation of Conversational Data
With participants' consent, we acquired access to the log files of their Amazon Echo devices. These files provide audio recordings of user queries, textual representations of these queries as recognized by the voice assistant and system's responses to the queries. These data were annotated using ELAN 6.0 annotation software (Hellwig and Sloetjes, 2021), a screenshot of which can be found in Figure 1. There was a total of two annotation cycles (done by one and two annotators, respectively).
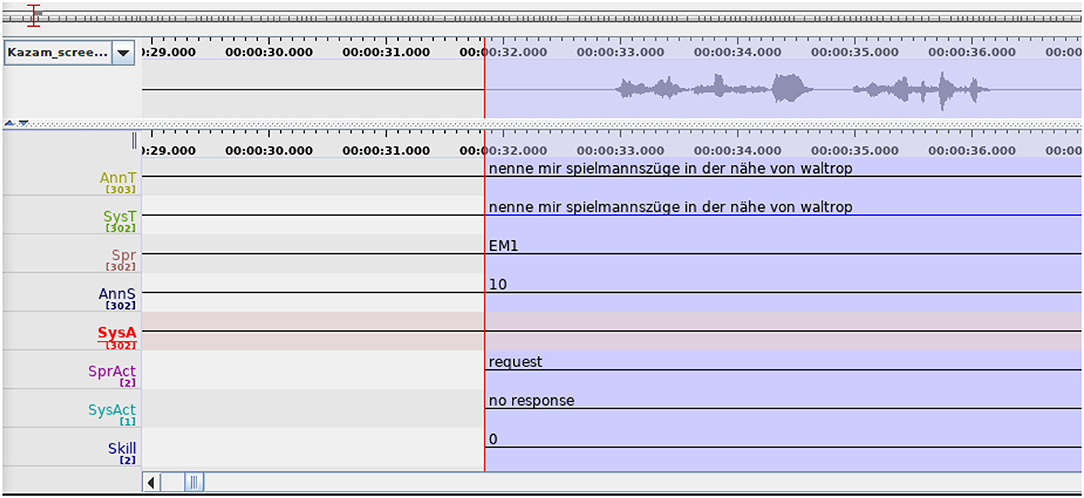
Figure 1. Screenshot of ELAN annotation software from the second annotation cycle. All layers mentioned in section 3.2 can be seen here.
In the first annotation cycle, the audio recordings of user queries were manually transcribed to facilitate the comparison with the textual representation given by Alexa, and then each request was annotated with the information about the speaker as perceived from the audio. Thus, the annotation tier “AnnT”contains the transcription of the annotator and the tier “SysT”—the transcription of the same query as provided by Amazon Alexa. Regarding the speaker, the tier “Spr” contains the perceived gender of the person, represented by M (male) or W (female), and the age distinction between an adult (E) and a child (K). The speakers are also numbered within their gender-age category, e.g., KW1 and KW2 for “female child #1” and “female child #2”. In addition to this, the tier “AnnS” was used to indicate the certainty of the annotator regarding the classification of the speaker on a scale from 1 (least certain) to 10 (absolutely certain). Alexa's responses were recorded in the tier “SysA”. The tier “TS” was used to annotate the timestamp of the interaction.
The second annotation cycle was focused solely on communication breakdowns. For this, conversational data were segmented into temporally adjacent and thematically consistent interaction episodes (blocks consisting of one or more request-response pairs). Out of these, only episodes containing communication breakdowns were selected. Each of these episodes started with a request-response pair where miscommunication occurred and ended with a request-response pair corresponding to either a successful resolution of the breakdown and fulfillment of the user's request or the abandonment of repair attempts by the user. These episodes were then subjected to the second cycle of annotation. The paper by Beneteau et al. (2019) served as basis for the annotation scheme here, as it provided a comprehensive overview over repair strategies seen in conversational data of an experiment with conditions similar to ours.
Again, multiple tiers were defined during the annotation. The tier “SprAct” was used to annotate the characteristics of the speaker's request. A full overview can be found in Table 2. Characteristics describing conversational repair strategies were partially taken from Beneteau et al. (2019) and additional ones were defined to accurately represent the observations in our data. To facilitate calculations concerning our research questions, we then combined repair strategies into three supercategories, namely reformulation (including lexical, syntactical and semantic adjustments, termed “group A”), repetition (termed “group B”), and changes in articulation (including increased volume, prosodic changes and overarticulation, termed “group C”). These groups are denoted by different colors in Table 2. The tier “SysAct” was used to annotate how the Echo Dot responded to the speaker. For this tier the response types “acting on misunderstanding,” “neutral clarification response,” and “specific clarification response” from Beneteau et al. (2019) were used. Two more response types were added: “no response” and “proper response.” A description of these labels can be found in Table 3. The tier “Skill” was used to indicate whether the user was interacting with a third-party Alexa skill or not.
As the second annotation cycle involved two annotators, inter-annotator agreement had to be determined. For this, a set of conversational data including two families was annotated by both annotators and then average Fleiss' κ for these families was calculated, once for the annotation of speaker's speech acts (κ = 0.685) and once for the annotation of system response types (κ = 0.815). According to Viera and Garrett (2005), these values indicate substantial agreement between annotators.
3.3. Measures
3.3.1. Failed and Successfully Repaired Request Blocks
First, we assessed the number of requests a family made during the 5 weeks of the study by investigating the respective log files (M = 518.90, SD = 275.08, Range: 166–1,087). Then, as mentioned in the section 3.2, these requests were grouped into interaction episodes, consisting of one or many request-response pairs connected by time and topic. For hypotheses H1a and H1b, we defined successfully repaired request blocks as the number of successful interaction episodes wherein at least one repair attempt has been done. Hence, requests which were successful directly are not included. Failed blocks were defined as those interaction episodes where a request block ends with an unsuccessful request whereupon the user abandons (further) repair attempts. To make the number of failed and successfully repaired blocks comparable among the families, we calculated the number of request blocks per 100 requests (successfully repaired blocks: M = 1.95, SD = 0.85; failed blocks: M = 10.82, SD = 3.55).
3.3.2. Repair Strategies
For research questions RQ1a-c we wanted to investigate the repair strategies and the way in which they may be influenced by the perceived causes of errors and participants' reactions to them. For these calculations, repair strategies were not considered separately, but in three groups described in section 3.2 and Table 2. Hereby research question RQ1a corresponds to the repair strategy group A and so on, respectively. For the research question RQ1d we consider failed interaction episodes where no repair was undertaken by the user in the first place. Again, to make the number of times a specific strategy was used more comparable, we calculated a number of uses for each strategy per 100 “miscommunication and repair requests.” These include all unsuccessful requests which required a repair strategy (M = 197.78, SD = 110.74, Range: 90–405). The descriptive values reveal that strategy A was most frequently used (M = 11.21, SD = 7.89, Range: 2.13–27.78), followed by strategy B (M = 1.92, SD = 1.25, Range: 0–3.39) and strategy C (M = 1.49, SD = 1.91, Range: 0–4.92). On average, 1.95 out of 100 miscommunication situations were left unrepaired (SD = 1.08, Range: 0.26–4.13). For research questions RQ2a-d we furthermore made a distinction between children and adults as well as the two phases of measurement, i.e., between the first and second (MP1), and between the second and third (MP2) house visit (for descriptive values see Table 4).
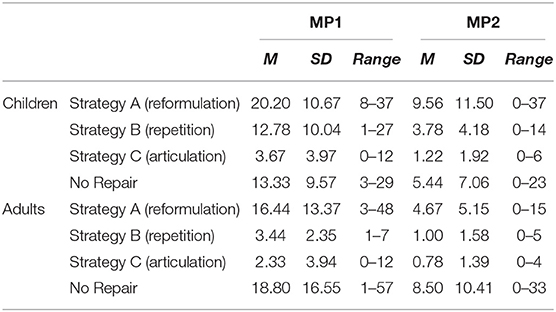
Table 4. Descriptive statistics of repair strategies by time and age group (MP1, first phase of the study; MP2, second phase of the study).
3.3.3. Affinity for Technology
To measure participants' affinity for technology, we used three items inspired by two subscales of the TA-EG by Karrer et al. (2009), namely enthusiasm for technology and technical competencies: “We like integrating new electronic devices into our everyday family life”, “Members of my family know most of the functions of the electronic devices we own (to the extent they can understand them based on their age)”, and “Compared to our social environment, we are more open to the use of electronic devices in everyday family life”. Testing for reliability, Cronbach's Alpha yielded a score of 0.74, which indicates a good scale reliability (Streiner, 2003). Participants rated the items on a five-point Likert scale during the first home visit, ranging from 1 = not true at all to 5 = fully true. To have a value for hypothesis testing, we calculated a mean score (M = 3.67, SD = 0.82).
3.3.4. Reasons for Errors
In the questionnaire, to describe possible reasons for errors during interaction with the VA six items concerned with common problems were generated: “I/The other person spoke too unclearly or too quietly”, “I expressed myself/The other person expressed themself too ambiguously (e.g., by using a word that was misunderstood by the voice assistant)”, “I/The other person did not activate the voice assistant correctly”, “I have/The other person has made a request incorrectly”, “My language skills/The language skills of the other person were not sufficient” and “I/The other person used a formulation/dialect which the voice assistant did not understand”. On a five-point Likert scale, participants rated how often these problems were reason for miscommunication during the second and third home visit (from 1 = never to 5 = very often). We first calculated a mean across the two sessions before we ran a factor analysis which indicated a one-factor solution including all items with a very good reliability (Cronbach's α = 0.87). A scale mean was retrieved for further calculations (M = 2.04, SD = 0.68).
3.3.5. Error Attribution
Furthermore, we used Nass and Moon's (Nass and Moon, 2000) consideration that people can either direct their social reactions to a system directly or to a person behind the system (such as a programmer) to generate three items concerning the attribution of blame when an error occurs. The participants were asked to think of an error that had occurred multiple times, and in what way they would attribute that error to the following parties: themself (“I have made an error”), the programmer (“The voice assistant has not been satisfactorily programmed”) and the voice assistant (“The voice assistant itself has committed an error”). Participants rated the items on a five-point Likert scale (from 1 = not true at all to 5 = fully true) during the second and third home visit. We calculated mean scores for each item across both sessions for further computations (self: M = 2.40, SD = 0.70; programmer: M = 3.40, SD = 1.13; voice assistant: M = 2.55, SD = 0.93).
3.3.6. Emotions When Errors Occur
Based on the Differential Emotions Scale [DES; Izard et al. (1974)] we extracted emotions that may occur due to a communication breakdown with a VA and set up a scale of nine items: anger, disappointment, sadness, surprise, desperation, interest, motivation, annoyance, and amusement. Participants rated to what extent they experienced the respective emotions when an error occurred on a five-point Likert scale (1 = not at all to 5 = very strongly) both in the second and third home visit. For a factor analysis, mean scores of both sessions were calculated. Factor analysis revealed a two-factor solution, one including anger (M = 1.55, SD = 0.80), sadness (M = 1.45, SD = 0.80), and desperation (M = 1.25, SD = 0.43), and one encompassing the remaining six items. Since the first subscale was more unambiguous in its meaning, we decided to use it for further computations by calculating a mean value for each participant (M = 1.42, SD = 0.56).
3.3.7. Reaction to Errors
For RQ1 we also wanted to investigate the predictive power of the reaction to errors for the repair strategy a user picks. Therefore, we generated four distinct items, each rated on a five-point Likert scale from 1 = never to 5 = very often, asking the participants how often they reacted to an error in the respective way: repetition (“I repeat the request”), rephrasing (“I rephrase the request”), getting help (“I get help”, e.g., from another person or the internet) and abortion (“I abort the request”). Again, participants rated these items during the second and third home visit, which is why for further calculations, a mean score was retrieved for each item (repetition: M = 3.05, SD = 1.14; rephrase: M = 3.00, SD = 1.03; get help: M = 1.90, SD = 0.78; abortion: M = 2.70, SD = 1.18).
3.3.8. Satisfaction With the Voice Assistant
Lastly, we wanted to explore the connection between satisfaction with the device and the number of aborted and successful request blocks. Participants rated their satisfaction with the device on a five-point scale, where a very sad emoji indicated absolute dissatisfaction and a very happy emoji indicated absolute satisfaction with the voice assistant. The scale was rated at the end of the experiment to measure the final level of satisfaction (M = 3.56, SD = 1.01, Range: 2–5).
4. Results
In this section, we present the results of our study. First, three interesting cases of communication breakdown (translated into English from German) that occurred during the interactions within our study will be considered to illustrate the repair strategies and VA responses described in section 3.2. Then, quantitative results of our statistical analysis with regards to the hypotheses and research questions presented in section 2 will be shown.
4.1. Examples of Communication Breakdowns
Many interaction episodes involving miscommunication observed in the conversational data are rather simple and do not follow many steps until either resolution or abandonment. However, these examples were selected because they showcase some of the more complex behaviors, both on the side of the user and the assistant and invite informed speculation about possible reasons for such behaviors.
Consider the following example where an adult male speaker during his second week as a participant in the study attempts various reformulation-based repair strategies, such as semantic, lexical and syntactical adjustment (defined in Table 2) in order to get the desired information and accomplish his task.
Example 1
EM1: “Alexa, name me the member countries of the WHO”
A: “I am sorry, I don't know anything about that”
EM1: “Alexa, what is the WHO?”
A: “Browser Helper Objects are computer programmes that extend the functionality of the Internet Explorer. BHOs are used since version 4 of the Internet Explorer. They have direct access to the Document Object Model”
EM1: “Alexa, what is the World Human Organisation?”
A: “The World Human Vehicle Association was founded in 1976 in the US under the name of the International Powered Vehicle Association”
EM1: “Alexa, name me the member countries of the World Human Organisation”
A: no response
EM1: “Alexa, name me the member states of the World Human Organisation”
A: no response
EM1: “Alexa, what is the WHO?”
A: “Browser Helper Objects are computer programmes that extend the functionality of the Internet Explorer. BHOs are used since version 4 of the Internet Explorer. They have direct access to the Document Object Model”
EM1: “Alexa, the Health Organisation what, Alexa”
A: no response
EM1: “Alexa, name me the member states of the World Health Organisation”
A: “Unfortunately, I don't know anything about that”
EM1: “Alexa, what is the World Health Organisation?”
A: no response
Here, we can observe how the speaker tries to get information from Alexa with regards to the World Health Organisation (WHO) and its member states. Considering that the WHO currently has 194 member states, as is stated on its official website (World Health Organisation, 2021), this request is not well-answerable via a speech-based interface and a voice assistant would only be able to provide a fraction of the requested information within the interaction and perhaps a link to a website that could be followed by the user on a device such as a mobile phone. However, judging by the transcription of the user request provided in Alexa log files, it seems that the assistant could not fulfil the request due to speech recognition errors as two words, including the actual abbreviation “WHO,” were mistranscribed. Yet Alexa only provides a neutral clarification response here, not indicating to the user what the problem might have been.
The first repair attempt the user undertakes is a semantic adjustment of his initial request, showing his willingness to settle for a more generalized type of information that might be easier to retrieve for Alexa. The request is misunderstood by the assistant and it acts on this misunderstanding by providing a definition to an object with a name similar to the requested one. This acting on misunderstanding might have provided additional clues to the speaker, which would be consistent with the findings of Cho and Rader (2020) described in section 2.2, and so the user decides to expand the abbreviation to eliminate it as a possible source of the communication breakdown. However, he gets the term wrong, calling the WHO the “World Human Organisation” and Alexa acts on this incorrect request as best it can, yet the moment the user reformulates his request to be more specific again while keeping the wrong name of the organization, the assistant fails to provide any kind of response.
After the repetition of the first repair attempt with the same result, the user corrects himself and uses the exact full name of the WHO in his next repair attempt. He also briefly tries dropping the sentence structure almost entirely and focusing his request on the keyword, though it could be questioned whether it was a deliberate syntactical adjustment at this point. From the log files we can see that out of the last three repair attempts of this block only the second one (“Alexa, name me the member states of the World Health Organisation”) was correctly transcribed by the speech recognition. However, Alexa probably cannot deliver a response to query that specific and so this is the only request out of these three that it reacts to with a neutral clarification response. The other two failed requests are not reacted upon, since speech recognition errors made them into gibberish according to the log files. After these failed breakdown resolution attempts, the user gives up and abandons the request.
Next one is an example of parental help for resolution of miscommunication situations. The importance of such help for child-assistant interaction was mentioned in section 2.3. There are two speakers here in their second week of the experiment, a male child that is aided by a female adult speaker (presumably, his mother).
Example 2
KM1: “Alexa, what can I do together with my mom and sister?”
A: no response
EW1: “Alexa, events in [city name] today”
A: “Unfortunately, I am not sure how to respond”
EW1: “Alexa, what is happening today in [city name]?”
A: “‘Today’ means the present, the current times (the times in which we live)”
EW1: “Alexa, events in [city name]”
A: starts a skill offering event recommendations in [city name]
Here, the child expresses his request in a rather colloquial fashion, more appropriate for human-human conversation where additional context is either known to the conversation partner or can be acquired through additional questions. Alexa does not provide a response to this request, despite the fact that it was correctly transcribed by the speech recognition. That is when the adult person comes to help and attempts a semantic adjustment of the original request by creating a more precisely-worded query-style utterance and providing required context such as the name of the city and the fact that they are looking for events specifically and not, for example, ideas on what to do at home. It is not clear why Alexa cannot provide a response to this, as it only gives a neutral clarification to the user, though the request is transcribed correctly. Considering the last request-response pair in this block, this request might be overspecified and cannot be matched to the corresponding skill due to the use of the word “today”. The user tries further repair strategies by adjusting the lexical content and the syntactic form of the request, yet it is misunderstood by Alexa and this misunderstanding is acted upon. In the next step, the user returns back to the query-style utterance consisting only of keywords which is ultimately successful and resolves the communication breakdown. This ties in with the phenomenon of the “economy of language” which is a special modification of linguistic qualities of speech employed by users of voice assistants as mentioned in Luger and Sellen (2016).
And here is the third example of two children, male and female, who are collaborating to receive information as to which animal on Earth is the most agile. This example comes from the third week of the experiment.
Example 3
KM1: “Alexa, what is the most agile animal in the world?”
A: “The sea wasp which is a kind of box jelly is the most venomous animal in the world”
KW1: “Alexa, what is the most agile animal in the entire world?”
A: “The smartest animal in the world is a dolphin. They can recognize emotions, plan own actions in advance and thus, solve complex problems. The capacity of their brains can potentially equal that of the human brain”
KW1: “Alexa, who is the most agile?”
A: “I am sorry, I don't know anything about that”
KW1: “Alexa, is there alien alien something?”
A: “On WELT, there is a documentary 'Aliens, the Message' tomorrow night at 1 a.m. and two episodes on N24 Doku starting from 1:45. By the way, you can ask me questions such as 'What movies are on tonight?' or 'Is there a crime series on today?' More information can be found in your Alexa app”
KM1: “Alexa, what is the most agile animal in the world?”
A: “The longest animal on Earth is a blue whale that is 27.5 m long”
KM1: “Alexa, what is the most agile animal in the world?”
A: “The smallest known animals in the world are the Myxozoa that belong to the phylum of Cnidaria and do not grow bigger than 20 μm. It is a parasitic lifeform living in the waters. The smallest of the Myxozoa is the Myxabolus shekel with the average size of 8.5 μm. The smallest vertebrate is the frog Paedophryne amauensis with an average size of approximately 7.7 mm”
KM1: “Alexa, what are the most agile animals in the world?”
A: “Hmm, I don't know, but I am still learning”
KM1: “Alexa, what is the most agile animal in the world?”
A: “I like seahorses”
In this example, we see two children supporting each other in the resolution of a communication breakdown without intervention or scaffolding from an adult. Apart from the third request that is unclear and may be incomplete or unintended, the rest are repair attempts utilizing various strategies, such as lexical and syntactical adjustments (although they are minor), speaker change and a repetition at an increased volume as could be recognized from the audio files (the sixth request).
Alexa for the most part, however, keeps providing the wrong kind of information with regards to animals. It appears to be mostly an issue of speech recognition, as in the first, the fifth and the last request-response pairs the transcription substitutes the word “gelenkigste” (“the most agile” in German) with similar-sounding “giftigste” (“the most venomous”), “längste” (“the longest”), and “beliebteste” (“the most beloved”), respectively, and Alexa acts on these misunderstandings by providing the corresponding answers. The seventh request is also mistranscribed, but the resulting utterance is non-sensical, and while the third one features the correct adjective, it lacks the necessary context that animals are the subject of this request. In both cases, Alexa provides a neutral clarification response. The second and the sixth request are transcribed more or less accurately by the system and feature at least the correct adjective, yet Alexa seems to have misunderstood the intention of the users again and delivered wrong results. After all these unsuccessful repair attempts, the children have abandoned their task.
These examples can be seen as an illustration of some of the aspects known from previous research as described in section 2. Sometimes family members help each other resolve communication breakdowns in interactions with voice assistants which happened in examples 2 and 3. Sometimes the assistant acting on misunderstanding instead of delivering a neutral message of non-understanding can provide clues as to what the reason for the breakdown might be, which could have been the case in example 1. Children in these interactions seem to have more difficulty to be understood correctly by the system, either due to errors in speech recognition or lack of context in their requests or unclear communication of their intent. However, these are just a few examples the reconstruction of which is limited by the characteristics of the conversational data that was collected during this study.
4.2. Quantitative Results
4.2.1. Predictive Power of Affinity for Technology, Error Attribution and Emotions on the Number of Failed and Successfully Repaired Requests
H1: User's affinity for technology, the party they attribute the emergence of a communication breakdown to, and the emotions they experience when such a breakdown occurs serve as predictors for the number of abandoned failed requests (H1a) and successfully repaired requests (H1b). Hereby, we expect users with lower affinity for technology, users that attribute communication breakdowns to own mistakes and users experiencing negative emotions during breakdowns to abandon requests more frequently and achieve successful resolution of miscommunication less frequently.
In our study, we were able to combine the results in form of the empirical and conversational data in order to investigate the connections between various variables present in these data sets. For this hypothesis specifically, we analyse the relationship of the measures described in sections 3.3.1, 3.3.3, 3.3.5, and 3.3.6. In order to do that, we calculate two multiple linear regressions where the affinity for technology scale's mean, the error attribution items and the subscale for negative emotions are used as predictors, whereas the number of failed and successfully repaired request blocks per 100 requests serve as criteria. For the first regression (H1a), homoscedasticity was not given, which is why a multiple linear regression with bootstrapping (1,000 samples) was calculated. Results revealed that there was no significant relation [F(5, 3) = 1.883, p = 0.319]. For H1b, all prerequisites were fulfilled, yet the calculations yielded no significance either [F(5, 3) = 2.272, p = 0.266]. Therefore, H1a and H1b need to be rejected.
4.2.2. Predictive Power of the Number of Failed and Successfully Repaired Requests on the Satisfaction With the VA
H2: The number of abandoned failed requests negatively (H2a) and successfully repaired requests positively (H2b) influence user's satisfaction with the voice assistant.
To investigate to what extent the satisfaction with the voice assistant (measure described in section 3.3.8) can be predicted from the number of abandoned failed and successfully repaired requests (measure described in section 3.3.1), we conducted a multiple linear regression. The number of failed and successfully repaired requests per 100 requests served as predictors, whereas the satisfaction with the device was the criterion. Heteroscedasticity was given, hence the regression was calculated using bootstrapping (1,000 samples). The model became significant with F(2, 6) = 11.798 and p = 0.013. According to Cohen (1988), the model had a high goodness-of-fit (adjusted R = 0.76). Taking a closer look at the predictors, only the number of abandoned failed requests was significant for the prediction of satisfaction (p = 0.005, β = −0.309), whereas the number of successfully repaired requests was not (p = 0.361). Thus, we can accept H2a: the number of abandoned failed requests has a negative effect on user's satisfaction with the VA.
4.2.3. Predictive Power of Reasons for Errors and Reactions to Errors on the Choice of Repair Strategy and Abortion of Requests
RQ1: Do user's attribution of communication breakdowns to specific factors and issues, along with their perception of their own behavior when such situations occur predict their choice of repair strategy (RQ1a-c) or abandonment of the query before attempting any repair in the first place (RQ1d)?
Combining our empirical and conversational data, we were able to investigate the relationship between the internal perception of reasons for breakdowns (measure described in section 3.3.4), user's own reactions to them (described in section 3.3.7) and the repair strategies they actually used during these situations (described in section 3.3.2). Here, we calculated four multiple regression analyses. For each of them, the mean of all “reasons for errors”-items as well as the four items regarding reactions to errors were included as predictors, whereas the criterion was the number of choices of specific repair behavior per 100 requests. Hereby, reformulation corresponds to the research question RQ1a, repetition to RQ1b, changes in articulation to RQ1c and absence of attempted repairs to RQ1d.
RQ1a lacked homoscedasticity, which is why the regression was calculated with bootstrapping (1,000 samples). It yielded significance [F(5, 3) = 17.926, p = 0.019] with an adjusted R of 0.91, which, according to Cohen (1988), indicates a high goodness-of-fit. Looking at the individual predictors, three out of five proved to be significant in predicting the frequency of making reformulations, namely the reasons for errors mean (p = 0.015, β = −9.043), “I repeat the request” (p = 0.024, β = 3.173) and “I abort the request” (p = 0.010, β = 5.480). The remaining two were not significant (“I rephrase the request”: p = 0.953; “I get help”: p = 0.051). RQ1c was the only other research question which lacked homoscedasticity, which is why here, too, bootstrapping was used (1,000 samples). For RQ1b, RQ1c and RQ1d, no significant relationship could be shown [RQ1b: F(5, 3) = 0.337, p = 0.864; RQ1c: F(5, 3) = 3.709, p = 0.155; RQ1d: F(5, 3) = 0.200, p = 0.942].
Thus, we could only find a significant relationship regarding RQ1a here. The questions about the reasons for miscommunication with a VA are a negative predictor for the number of reformulations that users applied during repairs. These questions are described in section 3.3.4 and can be seen to refer to certain actions of the speaker that might have led to a communication breakdown, such as “I spoke too unclearly or too quietly”. Meanwhile, the user's perception of them repeating their requests or aborting them in cases of miscommunication was found to be a positive predictor of them using reformulation.
4.2.4. Impact of Time and Age on Choice of Repair Strategy and Abortion of Requests
RQ2: Does user's age and the length of time they have interacted with a voice assistant predict their choice of repair strategy (RQ2a-c) or abandonment of the query before attempting repair (RQ2d)?
One of the advantages of our study is that we could observe developments over time due to two measuring points (MPs) and one of our goals was to investigate the influence of time on the choice/lack of repair strategy (as described in section 3.3.2), including the distinction between children and adults. Therefore, we calculated four repeated measures ANOVAs, each with the age group (adult/child) as within-subjects factor, and the respective strategy (reformulation: RQ2a; repetition: RQ2b; changes in articulation: RQ2c; no repair attempt: RQ2d) per 100 requests as dependent variable. Testing for normal distribution with the Shapiro-Wilk test (α = 0.05), we yielded significant results for reformulation (MP1: p = 0.027; MP2: p < 0.001) and changes in articulation (MP1: p = 0.009; MP2: p < 0.001). As studies have shown, though, that repeated measures ANOVAs are largely robust against effects of normal distribution violations (Berkovits et al., 2000), we proceeded with our calculations as usual.
For reformulation (RQ2a), no significant development could be observed over time [F(1) = 0.202, p = 0.659], as was the case for changes in articulation (RQ2c) [F(1) = 0.018, p = 0.896] and the absence of repair attempts (RQ2d) [F(1) = 0.160, p = 0.694]. Regarding time's effect on the frequency of repetition as a repair strategy (RQ4b), the ANOVA became significant with F(1) = 4.947 and p = 0.047. For descriptive values, see Table 5. Age did not play a significant role [F(1) = 0.294, p = 0.595]. It needs to be noted that for two research questions, Levene's Test for Equality of Variances revealed a significance, namely for the first measuring point regarding repetition (RQ2b) (p = 0.001) and the second measuring point regarding changes in articulation (RQ2c) (p = 0.025), which means that equality of variances is only partially given here. Therefore, RQ2a, RQ2c, and RQ2d could not be answered, whereas the calculations could give us some insight regarding RQ2b, namely that the amount of time the user has interacted with the VA has a negative impact on the number of repetitions they use during repairs.
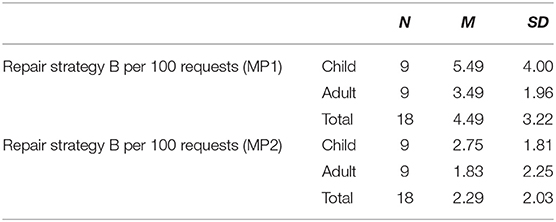
Table 5. Descriptive statistics for repair strategy B by time and age (MP1, first phase of the study; MP2, second phase of the study).
5. Discussion
The study presented in this paper aimed at investigating the situations in which communication breakdowns occur in interactions with a voice assistant in the family context. Hereby we address research gaps concerning (1) the behavior of both child and adult users in situations with communication breakdowns, (2) users' perception of such situations and their repair strategies, and (3) the consequences for user satisfaction with the assistant. In the previous section the results of our investigation were presented. Now we will reflect on these findings with regard to their implications for the state of research on the topic and their meaning for the design of future voice assistants or speech-based agents, more generally.
The examples presented in section 4.1 illustrate the user and VA behavior in situations when communication breakdowns occur. Instances of different repair strategies and system responses could be seen there, including some that seem rather baffling and can be explained only by looking at actual speech recognition results in the conversational data. The users of VAs, however, do not have access to this information, unless they are actively monitoring the history of their interactions online at that moment. The feedback that the assistant uses to signal a miscommunication is usually fourfold, as presented in Table 3. Generally, this feedback is considered unhelpful, as most of it puts the burden of finding a solution on the user. However, unexpected system behavior in cases of misunderstanding might still provide the users with clues to finding an adequate repair strategy, as suggested by Cho and Rader (2020). One such case could be seen in the first example in section 4.1.
In the conversational data we occasionally saw instances of family members helping each other, both within and across the two age groups (adults and children). Two examples of such situations were also presented in section 4.1. Unfortunately, as we did not have access to interactions between family members outside of Alexa log files, we could not identify whether more instances of such scaffolding took place during our study, and we were unable to gather sufficient data on this repair strategy for statistical analysis. In the second and the third examples, some of the children's requests lacked crucial contextual information and were therefore not successful. Further, children probably have difficulty recognizing the missing information due to an inaccurate mental model of the capabilities of the VA. In the second example, the adult was able to help the child by providing the missing contextual information to the assistant. In the third example, the children were unable to succeed with the request on their own as the system gave them no direct clues on how to fix the breakdown and their own knowledge about the VA may not have been sufficient to find the cause of the problem and an adequate repair strategy.
The statistical results give a mixed picture with regards to our research questions and hypotheses. Contrary to our expectations, H1a and H1b had to be rejected as we could find no significant effect of user's affinity for technology, their attribution of blame for communication breakdowns, and emotions they experience in miscommunication situations on either user's abandonment of failed requests or their success in accomplishing communicative repairs. This result suggests that other factors may contribute to the successful resolution of communication breakdowns, for example, the users' level of detail of knowledge about the VA or their speech quality. As mentioned in the literature overview, Siegert (2021) was able to find that accidental activation of Alexa was connected to the intonation variety of the speaker, so perhaps similar features could also be a factor here. Still, user characteristics such as affinity for technology may have an effect on the amount of effort the users are willing to invest into resolution of communication breakdowns or the variety of repair strategies they employ. These questions could be investigated in further research.
With regards to H2 we could confirm that the number of abandoned failed requests was a significant negative predictor for the satisfaction of the user with the voice assistant (H2a) which connects to related findings presented in section 2.1. Errors in speech and intent recognition were found to have a negative impact on user satisfaction and they also can prevent communication breakdowns from being resolved, which might cause the user to abandon their request. Concerning H2b, however, no effect of successfully repaired requests on user satisfaction was identified. This might be connected to findings of Kiseleva et al. (2016) that show that correlation between task completion and satisfaction is task-dependent, so no general significant impact could be found across all tasks. The positive impact of task completion might also have been removed by the negative effect of effort to be spent when repairing the communication breakdown.
We were able to gain insights regarding the research question RQ1a where three predictors became significant for the choice of reformulation-based strategies: the mean of perceived reasons for errors as a negative predictor (the reasons for errors as can be seen in their description in section 3.3.4 all refer to user's linguistic and communicative shortcomings) and the self-perceived frequency of repetitions and abortions of requests as positive predictors. This suggests that the users tend to vary the semantic, syntactical and lexical contents of their utterance when they do not find reasons for errors in their own communicative behavior, reverting to a general strategy of varying their utterances to overcome internal errors or shortcomings of the VA. The positive predictors here are especially interesting and may suggest that the classification of repair strategies used in this study is not experienced as such by the users, e.g., they might see minor lexical or syntactical adjustments as repetitions of the old request and major semantic adjustments as presenting a new request after the abortion of the old one.
Additionally, we found a significant effect of the time spent interacting with the voice assistant on the amount of repetitions used to repair communication breakdowns (RQ2b). In the second half of our experiment, the mean amount of repetitions used by both adults and children per 100 requests decreased significantly. It would be interesting to further investigate whether repetitions were the least successful repair strategy and were therefore used less over time. Unexpectedly, we could not find a significant impact of age and time on other choices of behavior. Perhaps our study was still too short to see any real changes in children's interaction style, as Garg and Sengupta (2020) suggest it might take 2–3 months for them to become more independent in voice assistant use.
Overall, we can summarize our findings and identify avenues for further research as follows:
• As we could not support H1, we believe that other factors might contribute to the failure or success of repair attempts, for example, user's speech style. Instead, the internal characteristics such as affinity for technology could have an effect on the amount of effort the users are prepared to spend on resolution of communication breakdowns or the variety of repair strategies they employ.
• We could confirm H2a and found a significant negative effect of the number of abandoned requests on user satisfaction.
• We could not confirm H2b and believe that further investigation of communication breakdown situations distinguished by task and effort spent on repairs might be necessary to understand the impact of successfully repaired dialogues on user satisfaction. This kind of research may give insight into the interaction quality necessary in various use contexts and identify critical types of tasks where miscommunication is associated with the highest cost for the user. Being able to mitigate these costs can help reduce the risk of abandonment of the device in the long run due to disappointment of the user in conversational capabilities of the system.
• We found that users are more inclined to use reformulation, i.e., variation of semantic, syntactical or lexical content of their requests when they do not perceive the emergence of miscommunication as their fault. We could not find a significant effect of internal reasons for the choice of other strategies, so we suggest that situational clues such as the immediate response of the VA are more important for the choice of repair strategy (RQ1) which could be investigated based on our conversational data set in the future.
• Our results suggest that the classification of repair strategies that was created for this paper based on other approaches in the field may be differently perceived by users, as we found the self-perceived frequency of repetitions and abortions of requests to be positive predictors for the use of reformulation-based strategies (RQ1). This raises a plethora of questions concerning studies of communication breakdowns in interactions with VA in general, which should be addressed in the future. On what basis should the classification of repair strategies be constructed? Should there be an empirical evaluation of strategy categories? How much does self-perception of own repair behavior matter and what factors is it influenced by? How does it correspond with mental models about the functionality of the VA?
• In our data, use of repetition as repair strategy by both children and adults significantly decreases over time. No changes were found for other strategies (RQ2). To understand this finding, further analysis is needed. We hypothesize that repetitions might not lead to successful resolutions of breakdowns and, therefore, are used less with time. Our data set can be used to investigate the relationship between the used repair strategy and the following Alexa response.
• No significant impact of age on the choice of repair strategy was found, as well as no interaction effect between age and time (RQ2). We suggest that a study over the course of several months is needed to investigate changes in children's interaction style and the success of their communication with the device over time.
Finally, some limitations of our study need to be pointed out. Our sample of nine families is quite small and despite good balance with regards to the representation of gender and education amongst the demographic in Germany, it may not be representative in terms of voice-assistant-related behavior. Additionally, we have only used the data sets of families that interacted with Amazon Echo Dot due to the availability of audio log files. While the results of Berdasco et al. (2019) show Alexa and Google Assistant both as significantly better than Cortana and Siri with regards to the quality and correctness of their responses, but not significantly different among each other, inclusion of various voice assistants into the study might have provided a fuller picture and potentially more robust results. Further, the conversational data might have missed some of the dynamics within a family concerning the use of the voice assistant, such as discourse scaffolding between family members. We were able to detect only those instances when the other family member actively addressed the voice assistant to help in miscommunication repair, such as the examples shown in section 4.1.
As far as consequences for the development of voice assistants are concerned, we support the view previously advocated by other researchers that VAs need to give better feedback to users in cases of miscommunication. Based on our results, we suggest that situational factors may play a major role in the choice of repair strategy as opposed to internal characteristics of the user. Finding appropriate repair strategies might reduce the amount of requests that users abandon and thus increase the level of satisfaction with the device. Hereby, the assistant could also gain awareness of the cost of repair a particular user associates with a particular type of task. The VA also could use information about critical and non-critical tasks in terms of repair cost to be more efficient. In the long run, all of these adjustments could help alleviate the problem of device abandonment or reduced usage shown in Luger and Sellen (2016) and Cho et al. (2019).
The clues that the assistant gives in cases of miscommunication, however, should be adapted to specific user groups, accounting for their mental models with regards to VA functionality, e.g., children. Here, specific discourse scaffolding strategies could be employed by the system to address unique challenges presented by children's speech, for example, building up on the research of Xu and Warschauer (2019). Children already are active users of speech-based technology (Yarosh et al., 2018), especially as it can alleviate the limitations presented by the lack of reading and writing skills in younger children, and the design of voice assistants or other speech-based agents has to address their specific needs. In general, however, one has to consider whether this sort of proactive or cooperative system behavior is possible under the paradigm of one-shot request-response interactions that can be seen in commercially available voice assistants today (Porcheron et al., 2018). We thus conjecture that future speech-based agents will require additional capabilities that would allow understanding the current interaction context and the mental states and knowledge level of the user, through some sort of joint co-construction and mentalizing occurring incrementally over the course of the interaction (Kopp and Krämer, 2021).
Data Availability Statement
The raw data supporting the conclusions of this article will be made available by the authors, without undue reservation.
Ethics Statement
The studies involving human participants were reviewed and approved by University of Duisburg-Essen, Germany. Written informed consent to participate in this study was provided by the participants' legal guardian/next of kin.
Author Contributions
LM responsible for the writing and editing of the most chapters, the general structure of this work and its presentation. LM and JS were additionally responsible for the formulation of the hypotheses and research questions and the direction of the data analysis process. CS was responsible for writing sections 3.1, 3.3, and 4.2. LMB was responsible for writing section 3.2. NK and SK were principal investigators of the project and are responsible for the overall scientific and organizational supervision as well as editing and revision of this article. All authors participated in either the design and execution of the study or in the data analysis process or both.
Funding
This research was part of the project IMPACT (The implications of conversing with intelligent machines in everyday life for people's beliefs about algorithms, their communication behavior and their relationship-building) and was funded by the Volkswagen Foundation.
Conflict of Interest
The authors declare that the research was conducted in the absence of any commercial or financial relationships that could be construed as a potential conflict of interest.
Publisher's Note
All claims expressed in this article are solely those of the authors and do not necessarily represent those of their affiliated organizations, or those of the publisher, the editors and the reviewers. Any product that may be evaluated in this article, or claim that may be made by its manufacturer, is not guaranteed or endorsed by the publisher.
Acknowledgments
The authors would like to thank Benedict Schneider, a former student researcher at the Social Cognitive Systems Group who greatly contributed to the data analysis process necessary for this article.
Supplementary Material
The Supplementary Material for this article can be found online at: https://www.frontiersin.org/articles/10.3389/fcomp.2022.791704/full#supplementary-material
Data Sheet S1. Questionnaire for the first home visit.
Data Sheet S2. Questionnaire for the second and third home visit.
References
Beneteau, E., Richards, O. K., Zhang, M., Kientz, J. A., Yip, J., and Hiniker, A. (2019). “Communication breakdowns between families and Alexa,” in Proceedings of the 2019 CHI Conference on Human Factors in Computing Systems (Glasgow). doi: 10.1145/3290605.3300473
Berdasco, A., Lopez, G., Diaz, I., Quesada, L., and Guerrero, L. A. (2019). “User experience comparison of intelligent personal assistants: Alexa, Google Assistant, Siri and Cortana,” in Proceedings of 13th International Conference on Ubiquitous Computing and Ambient Intelligence (UCAmI 2019) (Toledo). doi: 10.3390/proceedings2019031051
Berkovits, I., Hancock, G. R., and Nevitt, J. (2000). Bootstrap resampling approaches for repeated measure designs: relative robustness to sphericity and normality violations. Educ. Psychol. Measure. 60, 877–892. doi: 10.1177/00131640021970961
Cheng, Y., Yen, K., Chen, Y., Chen, S., and Hiniker, A. (2018). “Why doesn't it work?: Voice-driven interfaces and young children's communication repair strategies,” in Proceedings of the IDC 2018: The 17th International Conference on Interaction Design and Children (Trondheim). doi: 10.1145/3202185.3202749
Cho, J., and Rader, E. (2020). “The role of conversational grounding in supporting symbiosis between people and digital assistants,” in Proceedings of the ACM on Human-Computer Interaction. 4, 1–28. doi: 10.1145/3392838
Cho, M., Lee, S.-S., and Lee, K.-P. (2019). “Once a kind friend is now a thing: Understanding how conversational agents at home are forgotten,” in Proceedings of the 2019 ACM Designing Interactive Systems Conference (DIS 2019) (San Diego, CA). doi: 10.1145/3322276.3322332
Clark, L., Pantidi, N., Cooney, O., Doyle, P., Garaialde, D., Edwards, J., et al. (2019). “What makes a good conversation? Challenges in designing truly conversational agents,” in Proceedings of the 2019 CHI Conference on Human Factors in Computing Systems (Glasgow). doi: 10.1145/3290605.3300705
Cohen, J. (1988). Statistical Power Analysis for the Behavioral Sciences, 2nd Edn. Hillsdale, NJ: Lawrence Erlbaum Associates.
Druga, S., Breazeal, C., Williams, R., and Resnick, M. (2017). ““Hey Google, is it OK if I eat you?”: initial explorations in child-agent interaction,” in Proceedings of the IDC 2017: The 16th International Conference on Interaction Design and Children (Stanford, CA). doi: 10.1145/3078072.3084330
Dubagunta, S. P., Kabil, S. H., and Magimai-Doss, M. (2019). “Improving children recognition through feature learning from raw speech signal,” in Proceedings of the 2019 IEEE International Conference on Acoustics, Speech and Signal Processing (ICASSP) (Brighton), 5736–5740. doi: 10.1109/ICASSP.2019.8682826
Gale, R., Chen, L., Dolata, J., van Santen, J., and Asgari, M. (2019). “Improving ASR systems for children with autism and language impairment using domain-focused DNN transfer techniques,” in Proceedings of Interspeech 2019 (Graz). doi: 10.21437/Interspeech.2019-3161
Gallagher, T. M. (1977). Revision behaviors in the speech of normal children developing language. J. Speech Hear. Res. 20, 303–318. doi: 10.1044/jshr.2002.303
Garg, R., and Sengupta, S. (2020). “He is just like me”: a study of the long-term use of smart speakers by parents and children. Proc. ACM Interact. Mobile Wearable Ubiquit. Technol. 4, 3381002. doi: 10.1145/3381002
Hellwig, B., and Sloetjes, H. (2021). ELAN - Linguistic Annotator. Available online at: https://www.mpi.nl/corpus/html/elan/ (accessed September 28, 2021).
Izard, C. E., Dougherty, F. E., Bloxom, B. M., and Kotsch, N. E. (1974). The Differential Emotions Scale: a Method of Measuring the Subjective Experience of Discrete Emotions. Nashville, TN: Vanderbilt University Press.
Jiang, J., Awadallah, A. H., Jones, R., Ozertem, U., Zitouni, I., Kulkarni, R. G., et al. (2015). “Automatic online evaluation of intelligent assistants,” in Proceedings of the 24th International Conference on World Wide Web (WWW '15) (Florence). doi: 10.1145/2736277.2741669
Jiang, J., Jeng, W., and He, D. (2013). “How do users respond to voice input errors? Lexical and phonetic query reformulation in voice search,” in Proceedings of the 36th International ACM SIGIR Conference on Research and Development in Information Retrieval (SIGIR '13) (Dublin). doi: 10.1145/2484028.2484092
Karrer, K., Glaser, C., Clemens, C., and Bruder, C. (2009). Technikaffinität erfassen - Der Fragebogen TA-EG. Der Mensch im Mittelpunkt technischer Systeme 8, 196–201.
Kats, R. (2018). Are Kids and Teens Using Smart Speakers? The Smart Speaker Series Infographic. Available online at: https://www.emarketer.com/content/the-smart-speaker-series-kids-teens-infographic (accessed December 18, 2021).
Kennedy, J., Lemaignan, S., Montassier, C., Lavalade, P., Irfan, B., Papadopoulos, F., et al. (2017). “Child speech recognition in human-robot interaction: evaluations and recommendations,” in Proceedings of the 2017 ACM/IEEE International Conference on Human-Robot Interaction (HRI '17) (Vienna), 82–90. doi: 10.1145/2909824.3020229
Kiseleva, J., Williams, K., Jiang, J., Awadallah, A. H., Crook, A. C., Zitouni, I., et al. (2016). “Understanding user satisfaction with intelligent assistants,” in Proceedings of the ACM SIGIR Conference on Human Information Interaction and Retrieval (CHIIR) (Chapel Hill, NC). doi: 10.1145/2854946.2854961
Kleinberg, S. (2018). Why Parents Are Voice-Assistance Power Users. Available online at: https://www.thinkwithgoogle.com/marketing-strategies/app-and-mobile/voice-assistance-parent-users/ (accessed December 18, 2021).
Kopp, S., and Krämer, N. (2021). Revisiting human-agent communication: the importance of joint co-construction and understanding mental states. Front. Psychol. 12, 580955. doi: 10.3389/fpsyg.2021.580955
Lovato, S., and Piper, A. M. (2015). ““Siri, is this you?”: understanding young children's interactions with voice input systems,” in Proceedings of the IDC 2015: The 14th International Conference on Interaction Design and Children (Medford, MA). doi: 10.1145/2771839.2771910
Lovato, S., Piper, A. M., and Wartella, E. A. (2019). ““Hey Google, do unicorns exist?”: conversational agents as a path to answers to children's questions,” in Proceedings of the IDC 2019: The 18th International Conference on Interaction Design and Children (Boise, ID). doi: 10.1145/3311927.3323150
Luger, E., and Sellen, A. (2016). ““Like having a really bad PA”: the gulf between user expectation and experience of conversational agents,” in Proceedings of the 2016 CHI Conference on Human Factors in Computing Systems (San Jose, CA), 5286–5297. doi: 10.1145/2858036.2858288
Motta, I., and Quaresma, M. (2022). “Users' error recovery strategies in the interaction with voice assistants (VAs),” in Proceedings of the 21st Congress of the International Ergonomics Association (IEA 2021), eds N. L. Black, W. P. Neumann, and I. Noy (Springer International Publishing), 658–666. doi: 10.1007/978-3-030-74614-8_82
Myers, C., Furqan, A., Nebolsky, J., Caro, K., and Zhu, J. (2018). “Patterns for how users overcome obstacles in voice user interfaces,” in Proceedings of the 2018 CHI Conference on Human Factors in Computing Systems (Montreal, QC). doi: 10.1145/3173574.3173580
Nass, C., and Moon, Y. (2000). Machines and mindlessness: social responses to computers. J. Soc. Issues 56, 81–103. doi: 10.1111/0022-4537.00153
Porcheron, M., Fischer, J. E., Reeves, S., and Sharples, S. (2018). “Voice interfaces in everyday life,” in Proceedings of the 2018 CHI Conference on Human Factors in Computing Systems (Montreal, QC). doi: 10.1145/3173574.3174214
Purington, A., Taft, J. G., Sannon, S., Bazarova, N. N., and Taylor, S. H. (2017). ““Alexa is my new BFF”: Social roles, user satisfaction, and personification of the Amazon Echo,” in Proceedings of the 2017 CHI Conference Extended Abstracts on Human Factors in Computing Systems (Denver, CO). doi: 10.1145/3027063.3053246
Shivakumar, P. G., and Georgiou, P. (2020). Transfer learning from adult to children for speech recognition: evaluation, analysis and recommendations. Comput. Speech Lang. 63, 101077. doi: 10.1016/j.csl.2020.101077
Siegert, I. (2021). “Effects of prosodic variations on accidental triggers of a commercial voice assistant,” in Proceedings of INTERSPEECH 2021 (Brno). doi: 10.21437/Interspeech.2021-1354
Siegert, I., and Krüger, J. (2018). How do we speak with ALEXA - Subjective and objective assessments of changes in speaking style between HC and HH conversations. Kognitive Systeme 1. doi: 10.17185/duepublico/48596
Stent, A. J., Huffman, M. K., and Brennan, S. E. (2008). Adapting speaking after evidence of misrecognition: local and global hyperarticulation. Speech Commun. 50, 163–178. doi: 10.1016/j.specom.2007.07.005
Streiner, D. L. (2003). Starting at the beginning: an introduction to coefficient alpha and internal consistency. J. Pers. Assess. 80, 99–103. doi: 10.1207/S15327752JPA8001_18
Szczuka, J., Artelt, A., Geminn, C., Hammer, B., Kopp, S., Krämer, N., et al. (2021). Konnen Kinder aufgeklärte Nutzer*innen von Sprachassistenten sein?: Rechtliche, psychologische, ethische und informatische Perspektiven. University of Duisburg-Essen, University Library, Essen.
Tenzer, F. (2021). Prognose zum Absatz von intelligenten Lautsprechern weltweit bis 2025. Available online at: https://de.statista.com/statistik/daten/studie/1079997/umfrage/prognose-zum-absatz-von-intelligenten-lautsprechern-weltweit/ (accessed September 28, 2021).
Viera, A. J., and Garrett, J. M. (2005). Understanding interobserver agreement: the kappa statistic. Family Med. 37, 360–363.
World Health Organisation (2021). World Health Organisation - Countries. Available online at:https://www.who.int/countries (accessed September 29, 2021).
Wu, F., Garcia-Perera, L. P., Povey, D., and Khudanpur, S. (2019). “Advances in automatic speech recognition for child speech using factored time delay neural network,” in Proceedings of Interspeech 2019 (Graz). doi: 10.21437/Interspeech.2019-2980
Xu, Y., and Warschauer, M. (2019). “Young children's reading and learning with conversational agents,” in Proceedings of the 2019 CHI Conference on Human Factors in Computing Systems (Glasgow). doi: 10.1145/3290607.3299035
Yarosh, S., Thompson, S., Watson, K., Chase, A., Senthilkumar, A., Yuan, Y., et al. (2018). “Children asking questions: speech interface reformulations and personification preferences,” in Proceedings of the IDC 2018: The 17th International Conference on Interaction Design and Children (Trondheim). doi: 10.1145/3202185.3202207
Keywords: voice assistants, conversation analysis, miscommunication, communication breakdowns, human-machine interaction, repair strategies
Citation: Mavrina L, Szczuka J, Strathmann C, Bohnenkamp LM, Krämer N and Kopp S (2022) “Alexa, You're Really Stupid”: A Longitudinal Field Study on Communication Breakdowns Between Family Members and a Voice Assistant. Front. Comput. Sci. 4:791704. doi: 10.3389/fcomp.2022.791704
Received: 08 October 2021; Accepted: 04 January 2021;
Published: 27 January 2022.
Edited by:
Roberto Therón, University of Salamanca, SpainReviewed by:
Ronald Böck, Otto von Guericke University Magdeburg, GermanyMichela Patrizi, Sapienza University of Rome, Italy
Copyright © 2022 Mavrina, Szczuka, Strathmann, Bohnenkamp, Krämer and Kopp. This is an open-access article distributed under the terms of the Creative Commons Attribution License (CC BY). The use, distribution or reproduction in other forums is permitted, provided the original author(s) and the copyright owner(s) are credited and that the original publication in this journal is cited, in accordance with accepted academic practice. No use, distribution or reproduction is permitted which does not comply with these terms.
*Correspondence: Lina Mavrina, bHZhcm9uaW5hQHRlY2hmYWsudW5pLWJpZWxlZmVsZC5kZQ==