- 1Media Psychology, Julius-Maximilians University Würzburg, Würzburg, Germany
- 2Psychology of Intelligent Interactive Systems, Julius-Maximilians University Würzburg, Würzurg, Germany
Introduction: Modern digital devices, such as conversational agents, simulate human–human interactions to an increasing extent. However, their outward appearance remains distinctly technological. While research revealed that mental representations of technology shape users' expectations and experiences, research on technology sending ambiguous cues is rare.
Methods: To bridge this gap, this study analyzes drawings of the outward appearance participants associate with voice assistants (Amazon Echo or Google Home).
Results: Human beings and (humanoid) robots were the most frequent associations, which were rated to be rather trustworthy, conscientious, agreeable, and intelligent. Drawings of the Amazon Echos and Google Homes differed marginally, but “human,” “robotic,” and “other” associations differed with respect to the ascribed humanness, consciousness, intellect, affinity to technology, and innovation ability.
Discussion: This study aims to further elaborate on the rather unconscious cognitive and emotional processes elicited by technology and discusses the implications of this perspective for developers, users, and researchers.
Introduction
Let us start with a scenario! Who or what are you thinking about when interacting with smart devices? You may answer that smartphones, smart speakers, or voice assistants are nothing more than technological equipment so why should you be thinking of anything more—or even of anyone? From a logical point of view, there is nothing to object to these thoughts. From a psychological point of view, however, there are reasons to doubt this rational perspective. Research going back to the 1990s has shown that people tended to treat desktop computers “as social actors.” They reacted to computers they interacted with in a way only known from human–human interactions and adopted social norms of politeness or gender stereotypes (Reeves and Nass, 1996). More recent research has transferred this perspective to modern digital devices, revealing that social robots (Rosenthal-von der Pütten et al., 2013) or smartphones (Carolus et al., 2019) also elicit social reactions in their users. Looking at the phenomenon from that perspective, the scenario seems to have had an early scientific background. In addition, there are stories like the one from the hollywood movie “Her” told back in 2013, about a man falling in love with Samantha, a type of virtual assistant based on artificial intelligence. Although the technological level Samantha operates on is still futuristic, the movie draws a picture of the human-technology interaction we may experience in a not-so-distant future. Nowadays, we are interacting with devices that send increasing numbers of humanoid cues. Smart speakers like the Amazon Echo or Google Home appear more and more intelligent, respond in a more and more adaptive way, and are no longer controlled by keys or touch screens, but are directly addressed by the user. In contrast, their outward appearance is that of a simple loudspeaker and not a humanoid entity. Both Google's Home Mini and Amazon's Echo Dot resemble an oversized version of a puck; their larger devices (Echo and Home) are cylinder shaped. In contrast to social robots or recent attempts to connect a humanoid robot's torso with a voice assistant (Erol et al., 2018), the outer appearance of the Google Home and Amazon Echo is kept simple and is much removed from a humanoid appearance.
The combination of the smart speakers' humanoid capacities on the one hand and their outward technological appearance on the other hand results in new scientific challenges regarding the imagined entity users address when interacting with the device. Do they think of the loudspeaker or the hardware? Or do they imagine a specific mental representation of the device? Moreover, does this potential representation involve humanoid features? To reveal initial insights into the mental representations of intelligent voice assistants, the participants in Study 1 were presented with a smart speaker and were instructed to imagine this device to have a body. Then, they were asked to draw the image of the device they had in mind. Afterward, they answered a questionnaire, which asked for characteristics of both the participants themselves and the device. The contents of their images were analyzed, resulting in a selection of prototypical images of smart speakers. In Study 2, this selection was shown to a second set of participants who again assessed the characteristics of these images as well as of themselves.
Intelligent voice assistants adopt basic principles of humanity
Nowadays, people are moving into more and more houses offering numerous advantages which seem to justify both its purchase and the potential disadvantages that come with it: intelligent voice assistants have found their way into everyday life. In the US, every fourth household owns a smart speaker (Nielsen, 2018a); ~78% of them own an Amazon Echo and 28% own a Google Home (Statista., 2019), the most popular devices. In Germany, ~71% of persons owning smart speakers use an Amazon product and 19% a Google version (Statista., 2019), and the numbers keep rising. Although the terminology is rather inconsistent, vocal social agents, voice user interfaces, conversational agents, voice user interfaces, or intelligent voice assistants all refer to devices which can “have a conversation” with their users (Porcheron et al., 2018). The above-mentioned Amazon Echo and Google Home are mostly referred to as smart speakers: loudspeakers connected to a web-based intelligent personal assistant or intelligent virtual assistant, which are software agents performing tasks upon being prompted by verbal commands. Amazon's Alexa, Google's Google Assistant, as well as Apple's Siri, to provide another example, are activated by saying their names (Alexa, Hey Google, or Siri). Smart speakers are technological devices that run these virtual assistants, offering hands-free interaction. To avoid conceptual confusion, we will refer to the device and the operating software as an “intelligent voice assistant” (IVA), or we will be using the short form “voice assistant” throughout this article. When activated, voice assistants offer various features. The most popular are playing music or audio data, answering questions, finding the weather forecast, and setting an alarm or timer (Nielsen, 2018b). Their operation is primarily voice-based, with the user providing voice input and the devices sending voice output resulting in a humanlike conversation. In contrast, their outward appearance is far from humanlike; larger versions of both devices are cylinder shaped (Amazon Echo and Google Home) and the smaller versions resemble an oversized puck (Amazon Echo Dot and Google Home Mini). While they are clearly recognizable as technological devices, operating them by speech results in human–computer interactions that increasingly resemble human–human interactions. Speech has been regarded as a “fundamental and uniquely human propensity” (Nass and Gong, 2000). From an evolutionary perspective, Pinker (1994) assumes the universality of language as an instinct or innate ability of humans. Hearing speech, Nass and Gong (2000) argue, has been evolutionarily linked with the immediate conclusion of encountering another human being, until recently. Modern voice-based technology challenges these ancient principles of human uniqueness. Although the outward experience of intelligent voice assistants scarcely contributes to the impression of a humanoid counterpart, the way of interacting with them may elicit effects already known from research on the media equation.
Media equation: Media equals real life
Although they are consciously and unmistakably recognizable as desktop computers, research going back to the 1990s, as well as the technological equipment used then, revealed that devices elicit social reactions in their human users. Empirical studies following the Media Equation Approach showed that a media device “communicating” with its users was unconsciously met with a reaction similar to that toward a human being (Reeves and Nass, 1996). Allegedly, social cues sent by modern devices prompt their users to adopt basic social rules and norms known from human–human interactions. As this happens unconsciously, the basic principle that “media equals real life” is regarded as a universal, almost unavoidable phenomenon (Reeves and Nass, 1996, p. 5). Empirical studies in this field usually follow the experimental paradigm recognizing “Computers As Social Actors” (CASA) (Nass et al., 1994), which transfers the social dynamics of human–human interactions to human–computer interactions. Hence, to investigate if social rules and norms will still be followed, a computer replaces one human counterpart of a dyad of two humans. Studies following this approach have revealed that social rules like politeness (Nass et al., 1994), group membership (Nass et al., 1996), or gender stereotypes (Nass et al., 1997) can also be elicited by computer counterparts. Furthermore, more modern popular devices, which send considerably more alleged social cues than their ancestors studied in early CASA research, were analyzed. Recent studies focused on smartphones and revealed gender stereotypes (Carolus et al., 2018a) and reccurring effects of politeness (Carolus et al., 2018b), for example. Similarly, virtual agents and social robots were shown to elicit social responses (for an overview: Krämer et al., 2015). Studies focused on intelligent voice assistants as devices added the new feature of speech to CASA research (Carolus et al., 2021). Although there were early attempts to analyze the effects of “talking devices” (Nass et al., 1997), implementation of the speech functions was rather poor (desktop computers “talking” with pre-recorded human voices) and of low external validity as devices used at that time simply did not have these features. Consequently, modern smart speakers introduced a fundamentally new quality constituting a relevant subject for CASA studies. The contrast between their humanlike feature of being able to talk and their technological outward appearance refers to the basic conflicting cues of CASA research.
Visualization and appearance
Operating a device entirely by speech, addressing the device by a name (“Alexa” and “Hey Google”), and being addressed by the device, in return, results in new qualities of human–computer interactions and raises questions about users' mental images: What or whom do users “mentally” turn to when talking to the device? How do they “see” the device in their imagination?
Questions of mental images accompanying cognitive processing have been widely discussed throughout the history of psychology. Early academic psychologists Wundt and Titchener claimed that “images are a necessary component of all thought activities,” which constitutes a perspective reaching back to Aristotle's idea that “thought without images was not possible” (Massironi, 2002, p. 161). Consequently, drawings and graphic notations as communicative and informational acts have been observed in ancient history, with the first drawings made ~73,000 years ago (Henshilwood et al., 2018). Since that time, graphics have evolved to be “indispensable tools for social and cultural evolution [...] showing no time of having exhausted its value [sic]” (Massironi, 2002).
To provide insight into peoples' imagination, (clinical) psychology tried to utilize drawings to overcome the limitations of verbal techniques requiring language skills. In psychotherapy, for example, children are encouraged to draw their family members as animals to gain insights into familiar structures (Petermann, 1997). Another technique requires the interpretation of drawings or graphics. For example, the Rorschach Test presents graphics resembling blots of ink, which are to be interpreted in terms of what they depict (Rorschach, 1921). The Thematic Apperception Test shows pictures of ambiguous situations and the subject is instructed to explain these situations in more detail (Revers, 1973). In sum, projective procedures represent an approach to projecting thoughts and inner cognitive processing by visualizing them (Frank, 1939; Revers, 1973; Schaipp and Plaum, 2000). However, they offer only limited diagnostic value. Any attempts to use drawings or interpretations of drawings to directly deduce characteristics of the drawer or the interpreter of the drawing are highly questionable. Nevertheless, these procedures can be regarded as a means of eliciting associations that can subsequently be discussed.
Visualization of technology
In the context of human–technology interaction, studies have illustrated that the outward appearance of technological devices influences the way users interact with them. The appearance of digital counterparts such as robots or virtual agents shapes users' expectations about their capacities (Goetz et al., 2003; Woods et al., 2004). For example, when thinking about social roles like office clerks, hospital staff, and instructors, users preferred human-looking robots over mechanical-looking robots. When referring to roles like lab assistants and security guards, mechanical-looking robots were preferred. Furthermore, the appearance of robots affected the attribution of qualities like trustworthiness (Schaefer et al., 2012). Moreover, impressions of devices were also affected by the characteristics of the devices such as perceived agency (Gray et al., 2007) and by interindividual differences such as age (Pradhan et al., 2019), experience (Taylor et al., 2020), and personality (Tharp et al., 2017).
Consequently, understanding the user's imagination of the device offers insights into emotional–cognitive processes such as expectations, attributions, and feelings toward the device, which, in the end, determine usage satisfaction and technology acceptance. While the diagnostic validity of drawings is weak, associative, spontaneous, or automatic drawings have been shown to constitute an alternative way of gaining insights into psychological concepts such as attitudes, for example, without the need for participants to assess items or answer questions verbally (Gawronski and De Houwer, 2014). Thus, they constitute a way of eliciting thoughts, sentiments, and feelings to facilitate and enhance considerations and discussions of a certain topic (www.aqr.org.uk). Accordingly, Phillips et al. (2017) asked participants to draw a certain type of robot (e.g., military, teammate, household, humanoid robots, or artificial intelligence) and showed that participants distinctly distinguished between human-like and machine-like robots depending on the area of operation. In contrast, the AI category produced highly diverse drawings, ranging from fully embodied robots to abstract networks.
In sum, previous research has shown that the appearance of technological systems shapes users' emotional–cognitive processes regarding digital devices and determines acceptance and user experience. In contrast to embodied technology such as robots, the ambiguous signals that voice-based systems send, looking like a machine but speaking like a human, resulted in visualizations that are more heterogeneous and revealed only a fragmented understanding of peoples' ideas of voice-based systems. To bridge this gap, this study adopts the core idea of “associative drawing” and again aims for the visualization of intelligent voice assistants. To allow more detailed insights into the mental representations of voice assistants, drawers and an independent second sample of participants will evaluate these drawings. Visualizations of voice assistants were generated and served as an association or projection of evaluations and characterizations of modern technologies.
Research questions
The Media Equation Approach and the CASA paradigm revealed that media devices elicit social reactions in their human users. Although consciously referred to as technological devices, desktop computers of the 1990s were shown to have a social impact on their users. Modern digital technology such as intelligent assistants are much cleverer and send more alleged social cues than their ancestors studied in early CASA research. However, intelligent voice assistants still possess the basic conflicting cues of CASA research: human-like cues (speech) on one hand and a technological appearance (loudspeaker) on the other. Additionally, their constituting feature to “speak” refers to a capability that, until recently, was unique to human beings, raising the question as to what or whom users are referring to when talking to their smart speaker.
Addressing these issues, the first research questions ask for the visual appearance of voice assistants: RQ1a: What kind of visual appearances do individuals associate with intelligent voice assistants? RQ1b: Do interindividual differences result in different visual appearances associated with intelligent voice assistants?
The second research questions address the characteristics of intelligent voice assistants:
RQ2a: Do individuals attribute human characteristics to intelligent voice assistants?
RQ2b: Do the attributed characteristics correspond to the individual's characteristics?
The final research questions ask for an interpretation of the visual associations from an external point of view:
RQ3a: What do others see in the individuals' visual appearances associated with voice assistants?
RQ3b: Do others attribute human characteristics to voice assistants?
RQ3c: Do the attributed characteristics correspond to the external raters' individual characteristics?
Overview of the studies: Visualization and characterization of intelligent voice assistants
To answer these questions, two studies were conducted. In Study 1, participants were presented with an intelligent voice assistant (Google Home or Amazon Echo) and were instructed to “imagine this device to have a body.” Then, participants were asked to “depict it” by using a computer-based drawing program (2D or 3D version). Afterward, they filled in a questionnaire that asked for the participants' characteristics (e.g., personality, gender, and affinity to technology) as well as for characteristics of the device (e.g., personality, gender, and user experience). In Study 2, a subsample of prototypical images was selected and presented to a second sample of participants who, again, analyzed the appearance of the images and assessed both their own characteristics as well as the characteristics of the illustrated devices. Figure 1 gives a detailed overview of the procedures used in Study 1 and Study 2.
Study 1—Visualization of intelligent voice assistants
Method
Participants
A total of 60 participants (19 male, 41 female) aged 18–54 (M = 22.68, SD = 4.85) took part. As they were recruited via a university recruitment system, participants were mainly students (n = 57) receiving course credits for participating. Regarding intelligent voice assistants, participants were rather inexperienced. On a Likert-Scale from 1 = never to 5 = very often, they rated their own experience between 1 and 2 (most experiences with Siri: M = 2.05; SD = 0.96; Amazon Echo/Alexa: M = 1.78; SD = 0.74; Google Home: M = 1.17; SD = 0.53).
Experimental design and task
A laboratory study was conducted adopting a 2 × 2 between-subjects factorial design. Participants were presented with a smart speaker, either the Amazon Echo Dot or the Google Home Mini (Factor 1: device). Echo Dot and Google Home were chosen as they are by far the most popular devices in Germany, the country where the study was carried out. Then, participants were instructed to “imagine that the Echo/Home has a body” and were encouraged to “depict this body” by either using the app Tayasui Sketches on an iPad or Paint3D on a Lenovo Thinkpad Yoga notebook (Factor 2: 2D vs. 3D condition). Referring to the literature on the benefits of 2D vs. 3D visualizations (Herbert and Chen, 2015), the idea was to explore potential differences between these two graphic renditions. In all conditions, participants used a digital pen to draw their pictures on the tablet screen. Afterward, participants answered the following questionnaires to assess the characteristics of themselves and the voice assistant (see Appendix for Table A summarizing the measures).
Measures: Participant's characteristics
Participants were asked to describe themselves using the following scales:
Media usage
Participants rated their usage of media (e.g., smartphone, notebook, and television) on a 9-point Likert scale (ranging from 1 = never to 9 = multiple times per hour).
Previous experience with intelligent voice assistants
On a 5-point Likert scale (1 = never to 5 = very often), participants indicated how often they have already interacted with intelligent voice assistants.
Affinity to technology
On a 6-point Likert scale (1 = strongly disagree to 6 = strongly agree), the nine items (e.g., “I like to try functions of new technological systems” or “It's enough for me to know the basic functions of a technological system.”) of the Affinity for Technology Interaction scale were answered (Attig et al., 2018). The internal reliability was good (α = 0.89).
Innovation ability
Following the method of Agarwal and Prasad (1998), participants responded to four items to indicate their Personal Innovativeness in Information Technology. Items such as “In my environment I am usually the first one to try out new technologies” or “I like trying new technologies” were answered on a 7-point Likert scale (1 = does not apply to 7 = fully applies) with good internal reliability (α = 0.84).
Interpersonal trust
The three items (“I'm convinced that most people have good intentions.”; “You can't rely on anyone these days.”; and “In general, you can trust people.”) of the Short Scale Interpersonal Trust scale (Beierlein et al., 2012) were answered on a 7-point Likert scale (1 = does not apply to 7 = fully applies) resulting in acceptable internal reliability (α = 0.77).
Institutional trust
The Scale of Online Trust by Bär et al. (2011) includes four items (“I am generally cautious about using new technologies” or “The Internet is a trustworthy environment for me”), which were answered on a 7-point Likert scale (1 = does not apply to 7 = fully applies). Internal reliability was acceptable (α = 0.75).
Personality
The Mini International Personality Item Pool (Mini-IPIP) was used to assess participants' personality (Donnellan et al., 2006). Five subscales with four items each were answered on a 5-point Likert scale (ranging from 1 = does not apply to 5 = fully applies): agreeableness (e.g., “I sympathize with other's feelings”; internal reliability α = 0.67), extroversion (“I am the life of the party”; α = 0.77), intellect (“I have a vivid imagination”; α = 0.44), consciousness (“I like order”; α = 0.72), and neuroticism (“I get upset easily”; α = 0.66). Two scales fell below the common criteria of acceptable values of Cronbach's Alpha (Cronbach, 1951). With reference to the small number of items per scale and the broad concept these scales referred to, we argue to keep the scales in their original version but to interpret results carefully (Nunnally, 1978).
Measures: Participants' attributions to the image
Participants answered a second questionnaire stating that “the following questions refer to the graphic you just created” and asking:
User experience
Based on the concept of user experience (UX, for a definition, see ISO-Norm BS EN ISO 9241-210 or Roto et al., 2011), the perception and evaluation of the image were assessed using three out of four AttrakDiff subscales (Hassenzahl et al., 2003, 2008) which consist of seven items each. The items were presented as a semantic differential (from 3 to −3): pragmatic quality (PQ; poles: “practical–impractical”), hedonic quality–stimulation (HQS; poles: “novel–conventional”), and hedonic quality–identity (HQI; poles: “inferior–valuable”). The reliabilities of all subscales were acceptable (PQ: α = 0.76, HQS: α = 0.75, HQI: α = 0.70), where acceptable is α = 0.75.
Uncanny
To assess the eeriness of the image, the Uncanny Valley Scale was used (Ho and MacDorman, 2010). A total of 19 bipolar items (from −2 to 2) were asked for three subdomains: humanness (six items, e.g., “artificial–natural” or “synthetic–real”; α = 0.74), eeriness (eight items, “numbing–freaky” or “predictable–freaky”; α = 0.57), and attractiveness (five items, “ugly–beautiful” or “crude–stylish”; α = 0.36). The subscales eeriness and attractiveness fell below the level of acceptable internal consistency. With reference to Nunnally (1978), we decided to keep the scales and argue to tolerate low-reliability scores in the early stages of research. However, results need to be interpreted with care.
Emotional reactions
The Positive and Negative Affect Scale (PANAS) (Watson et al., 1988; Breyer and Bluemke, 2016) was implemented to assess the participants' emotional reactions toward the image. On a 5-point Likert scale (1 = not at all to 5 = extremely), participants rated 20 adjectives (e.g., active, alert, and proud). The internal reliability of the positive affect scale was good (α = 0.88) and of the negative scale acceptable (α = 0.76).
Trustworthiness
Three items (e.g., “I think the image is trustworthy”) were assessed on a 7-point Likert scale (1 = never to 4 = always; Bär et al., 2011; Backhaus, 2017). The internal reliability was acceptable (α = 0.72).
Gender and age
Participants reported the gender (male, female, or diverse) and age (in years) of the device.
Personality
As we assessed indicators of personality, we also asked participants to rate the personality of their images. Again, Mini-IPIP was used with a different instruction. Similarly, we asked for agreeableness (α = 0.77), extroversion (α = 0.75), intellect (α = 0.70), consciousness (α = 0.68), and neuroticism (α = 0.47). Across all four subscales, the internal reliabilities were acceptable or rather weak.
Procedure and instructions
The investigator welcomed all participants and briefly informed them about the broad purpose of the study as well as data protection regulations. The procedure followed the ethical guidelines laid out by the German Psychological Association. When participants agreed to the conditions laid out by the investigator, they answered the first part of the measures on an iPad Mini (demographic data, media usage, previous experience with voice assistants, and psychological characteristics). Afterward, they were introduced to one of the two drawing programs and had 10 min to familiarize themselves with it (Factor 2: 2D vs. 3D). While participants in the 2D condition tested the program autonomously, participants in the 3D condition read a brief manual first. Subsequently, they were presented with either the Amazon Echo Dot or the Google Home Mini (Factor 1: device). Note that participants were only allowed to see or touch the device but not to operate it. Participants could visually and haptically inspect the speaker but could not interact with it (e.g., test features and functions or hear the voice). We refrained from various interactions to retain as much experimental control and to avoid interindividual differences in usage experiences (e.g., usage of different features and functions, operating errors vs. correct operations, and poor vs. great user experience) and short-term effects on the participants' associations. Consequently, we argue that the pictures drawn are the result of the participants' pre-experimental impressions and attitudes or pre-existing mental images of the respective technology. Therefore, the pictures reflect the level of knowledge and awareness of rather inexperienced individuals and provide (initial) insights into their mental models of intelligent voice assistants. Then, the investigator instructed the participant to “imagine the assistant to have a body” and to “draw what this body would look like.” During the 30 min drawing session, the device remained visible on the table in front of the participants. Afterward, each participant answered the second part of the measures (participants' characteristics and the characteristics of the device they had drawn). Finally, the investigator debriefed the participants, thanked them for their participation, and said goodbye (see Figure 1).
Content analysis of the images visualizing voice assistants
To gain initial insights, content analysis of the 60 images drawn to visualize voice assistants did not aim for a complete and comprehensive analysis of every single picture, but rather for an overview summarizing the subject of the picture. Four independent raters were trained to decode the motifs using a coding system. The development of the coding system included a combination of deductive and inductive approaches (Krippendorff, 1989). Deductively, categories were derived from the literature (Krippendorff, 1989). In consideration of the coding system introduced by Phillips et al. (2017), we focused on the major motif of the picture (person or object), limiting the analysis to a minor section of the syntactic level (Faulstich, 2009). In an iterative process, these categories were adapted to our sample of pictures and inductively refined. The subsequent process of coding involved three steps: (1) An initial subsample (n = 10 out of 60 images; random sample) was coded by four independent coders. For the results, differences were discussed and the code book was improved, (2) using this revised version, the next ten images were coded. Again, coders met and discussed any ambiguities, resulting in the final version of the coding scheme which is shown in Table B in the Appendix, (3) then, the four raters were divided into two teams of two. Each team coded 20 of the 40 remaining pictures. However, they continued to meet after every tenth image coded to discuss ambiguities. Consequently, we do not report inter-rater reliabilities because every single picture was coded by two raters first and discussed afterward until all four raters reached an agreement.
Results
The brief instruction given before the drawing session resulted in heterogeneous associations and images. Consequently, the results presented aim for an overview and overall impression rather than detailed portrayals of every single aspect of the images.
Visual appearances associated with intelligent voice assistants—RQ1a
To answer RQ1a, which asked for visual appearances that are associated with intelligent voice assistants, the content analysis first concentrated on the categorization of the main image motifs. Table 1 presents the entities of the 60 images depicted, separated into four conditions (Amazon Echo vs. Google Home; 2D vs. 3D drawing). Moreover, a “confidence rating” was implemented to define how applicable the raters assessed their coding, ranging from 25% (barely applicable) to 100% (absolutely applicable). Table 1 reveals that the coders assigned a certain level of ambiguity to the entities with confidence ratings between 60 to 80%.
Most of the pictures either showed a human being (n = 32) or a robot (n = 13). Animals, abstract graphics, and images showing the original device or an object were rare (n = 15). Comparing the Amazon Echo and Google Home, the subjects of the images were quite equally distributed. However, the Amazon Echo was slightly more often drawn as a human being (18 vs. 14) and the Google Home more often as an animal (1 vs. 4). A comparison of the 2D and 3D pictures revealed no substantial differences, so we will no longer distinguish between the two conditions in the following (the only exception was that 3D drawers more often portrayed a human: 18 vs. 14). To get an idea of the entities depicted, Figure 2 presents example images and the corresponding codings.
In-depth-analysis of human and robotic images
Across all four conditions, 75% of the images showed either a human or a robotic appearance. For reasons of clarity and comprehensibility, the subsequent detailed analysis was therefore limited to three categories: (1) humans, (2) robots, and (3) others that included images coded as original devices, objects, or abstract entities. Images coded as animals were excluded from further analysis. Since the 2D vs. 3D distinction was also excluded, the subsequent analysis focused on the differentiation between the two devices visualized (Echo vs. Home), which are summarized in Figure 3.
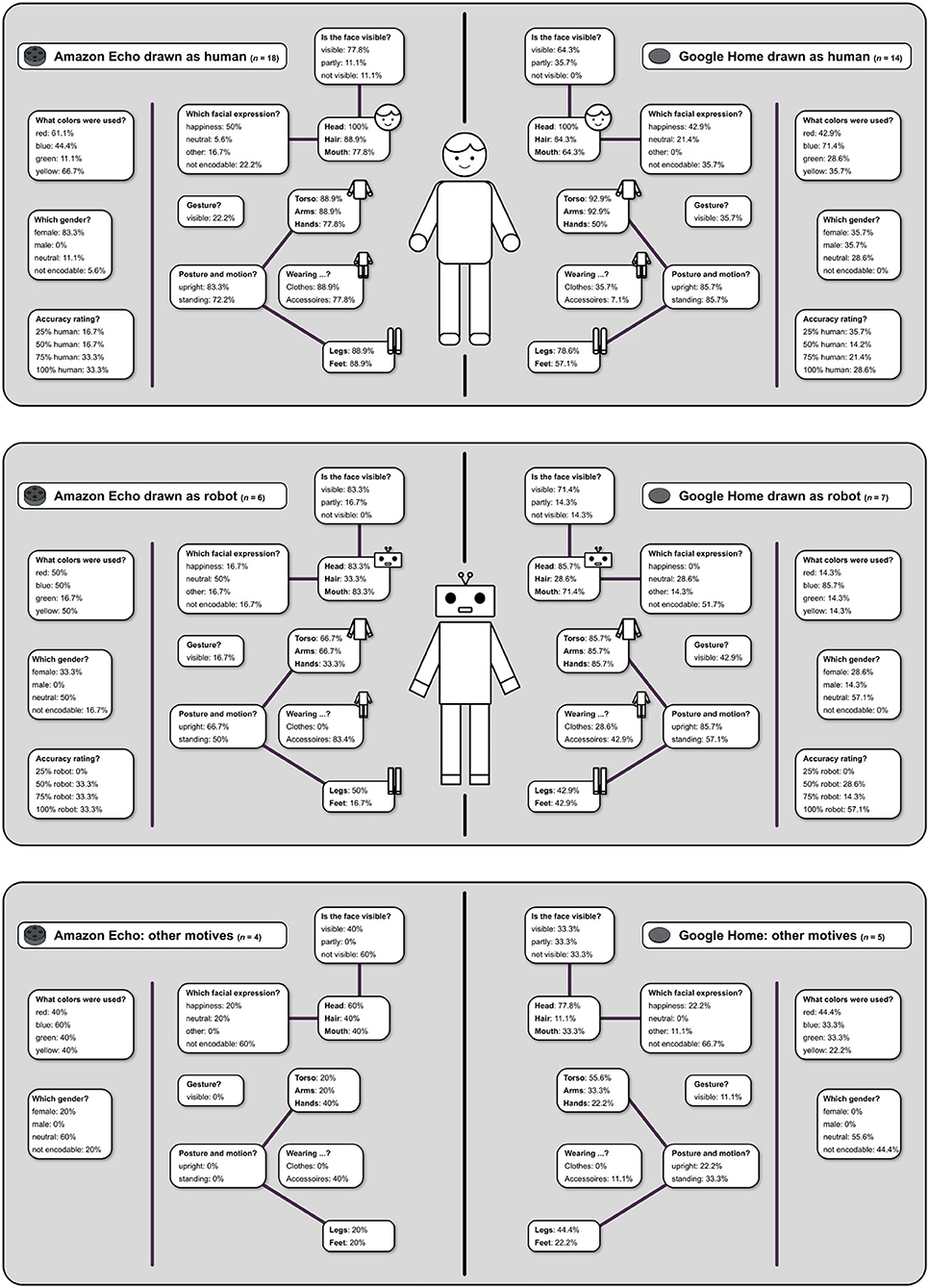
Figure 3. Summarizes the analysis on the differentiation between the two devices (Echo vs. Home). It further shows the coding categories, including the corresponding occurrences in the drawings.
Starting with images coded to show humans, 18 (out of 29) Amazon Echo and 14 (out of 30) Google Home images depicted humans, which can be carefully interpreted as a conformation of Amazon's (by tendency) human-like staging, with the assistant having a human, female name: “Alexa.” Google Home, however, does not refer to a human. Also, images of the Amazon Echo were predominantly female while those of the Google Home were male, female, or neutral in equal shares. The colors point in a similar direction: while approximately two-thirds of the Echo images were colored in red and yellow (vs. Google: 40%), two-thirds of the Google pictures were predominantly blue (vs. Echo: 44%). Color schemes could also be carefully interpreted in terms of gender stereotypical colors, as, since the 1940s, pink and lighter colors have been associated with girls, while blue and primary colors with boys (for an overview see: Paoletti, 2012). Furthermore, shades of red are often interpreted as warm colors, while blue colors are referred to as cool (Bailey et al., 2006). The depicted body parts revealed only minor differences. The bodies were drawn more or less completely; the Amazon Echo was drawn in slightly more detail (face, hair, mouth, and hands) and slightly more clad (clothes and accessories). Both devices were portrayed as standing upright. When facial expressions were coded, happiness was the most frequent expression.
Six images of the Echo and seven images of the Home showed robots. Images of the Google Home were slightly more distinctly coded as robotic (see the confidence rating in Figure 3). Robotic pictures of both devices were rather human-like and gender-neutral. When facial expressions could be coded, they were most frequently coded to be neutral. In sum, these aspects reflect a more technical but still humanoid character. Body parts in the pictures of the Google Home were more complete: 80% incorporated a torso, arms, and hands. Again, the Echo was more clad (accessories). Both devices were portrayed as standing upright. Approximately 40% showed legs and feet while pictures of Amazon's Echo lacked hands (70%) and feet (80%). Again, the color schemes differed: while red, blue, and green were used in 50% of the pictures showing the Echo (vs. Google: 14%), Google pictures were predominantly blue again (80% vs. Echo: 44%). Reflecting on the causes of these differences, we carefully refer to Google's less human-like staging of their device, which might have resulted in cooler colors (colors closer to the blue end of the visible spectrum; Bailey et al., 2006).
Three images of the Echo and five images of the Home showed other motifs. The images had no distinct gender (Amazon's Echo: 60% gender neutral and Google Home: 60% not encodable). For both devices, the face was not visible in most cases, again reflecting the rather technical impression. Amazon's Echo was mostly drawn without a full body and the face was only rudimentarily depicted, whereas the Google Home tended to be drawn with a body consisting of a torso (40%), arms (40%), and legs (40%). The most common color for Amazon's Echo was blue: 75% (vs. Google: 40%), and for the Home it was red and green with 60% each (vs. Echo: 25 and 50%). Here, the explanation of the more human-like staging of Amazon compared to Google does not apply. However, the number of cases in this category was too limited to venture a reliable interpretation.
Are there differences between participants associating humans vs. robots vs. other entities with voice assistants?
RQ1b asked if interindividual differences between the drawers result in different associations of the voice assistants. Following the results of RQ1a, three groups of participants were derived: participants who drew human or humanoid entities (confidence ratings below 100%, but categorized as human) were grouped and labeled “humans” (n = 32), drawers of robot entities were labeled “robots” (n = 12), and, due to small sample sizes, we pooled the remaining image motifs (original device, object, and abstract) into “other motifs” (n = 9). Animals were excluded from further analyses due to their small number. As the sample sizes of the three groups were small, a non-parametric Kruskal–Wallis test was conducted to compare the three groups of drawers. Table 2 presents the mean values with p-values indicating the significance of the group comparison. The effect sizes (r-values) refer to the differences between the two groups differing the most. Mann–Whitney U-tests for these two groups were conducted and the resulting Z-scores were used to calculate r, with r =| Z/√n|. We report r-values instead of Cohen's d value because d is biased for small sample sizes. However, r is problematic for unequal group sizes because n is part of the denominator, directly affecting r. Thus, effect sizes need to be compared with caution. Nevertheless, the interpretation of the r-value coincides with the interpretation of Pearson's correlation coefficient (Cohen, 1988; Faulstich, 2009). Thus, small (|r| = 0.10), medium (|r| = 0.30), and large effect sizes (|r| = 0.50) indicate meaningful differences between the groups.
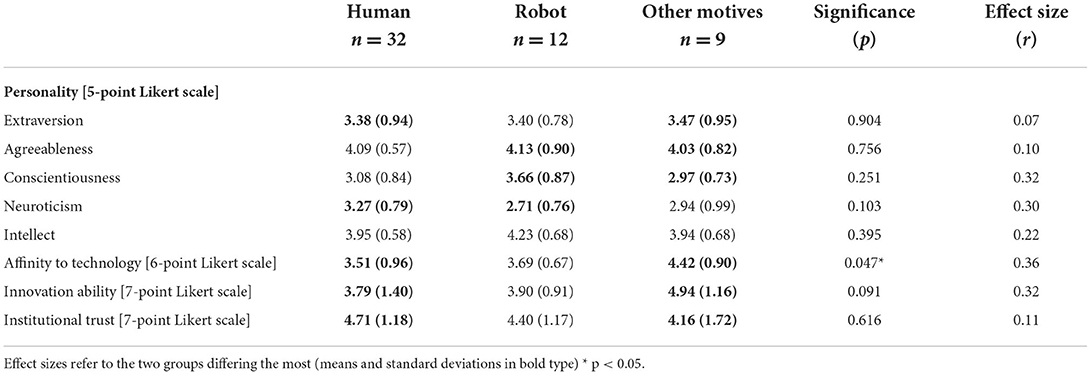
Table 2. Mean differences between the drawers of three image types, significances, and effect sizes.
Regarding personality traits, none of the five subscales exceeded the standard levels of significance (convention, e.g., alpha = 0.05 or 0.10). However, effect sizes indicate medium effects for consciousness, neuroticism, and intellect. Despite the small sample sizes, these effect sizes could be carefully interpreted as indicators of effects worth considering. For example, participants associating robots rated themselves to be the most conscious and intellectual, whereas participants associating other motifs (original device, object, and abstract) rated themselves as less conscious (robots vs. other motifs: r = 0.32) and less intellectual (robots vs. other motifs: r = 0.22). Furthermore, participants who drew humans were the most neurotic and participants who drew other motifs were less neurotic (human vs. other motifs: r = 0.30). In addition to the global perspective of the big five personality traits, more detailed concepts revealed differences that are more remarkable. Participants associating other motifs rated their affinity to technology the highest, and participants associating humans rated their affinity to technology the lowest (other motifs vs. human: r = 0.36). The same applies to innovation ability: again, participants drawing other motifs obtained the highest values and participants drawing humans the lowest (other motifs vs. human: r = 0.32). The differences of institutional trust were contrary and less substantial: values were the highest for humans and the lowest for other motifs (other motifs vs. human: r = 0.11). In sum, effect sizes indicated meaningful differences between the drawers' personalities, with those drawing robots characterizing themselves to be the most conscious and the most intellectual and those drawing humans rating themselves as the most neurotic. Participants who drew other motifs were salient, obtaining the highest affinity to technology, the highest innovation ability, and the lowest institutional trust.
Characteristics associated with intelligent voice assistants—RQ2
RQ2 asked if people attribute essentially human characteristics to intelligent voice assistants. To answer this question, participants' ratings of the pictures of the voice assistants were compared with regard to pragmatic and hedonic qualities, affect, eeriness, trustworthiness, personality, gender, and age. Again, Table 3 distinguishes between images of humans, robots, and other images as well as between images of the Amazon Echo and the Google Home, and presents the evaluations.
A comparison of the characterizations of the Amazon Echo vs. the Google Home only yielded marginal differences, with no difference exceeding standard levels of significance or meaningful effect sizes. The only difference worth reporting, in line with the preceding content analyses, was that Echos were more often rated as female than Google Homes, which were more often rated as male.
Comparing the ratings of pictures of human entities vs. robot entities vs. other motifs revealed that participants rated user experience in all three subscales above medium levels. Images showing human entities scored the highest. Meaningful effect sizes were shown for pragmatic quality (human > other motifs; r = 0.30). Uncanniness revealed medium levels in all three subscales and across all conditions. The results showed significant differences for humanness (unsurprisingly, human images were rated more human than robotic images; r = 0.37). Regarding essentially human characteristics, the results showed that participants did, in principle, assign these characteristics to the devices. Devices appeared to be rather trustworthy with ratings above medium levels. Moreover, personality ratings confirmed this impression with overall high ratings of conscientiousness, above-medium ratings of agreeableness and intellect, and low ratings of neuroticism. A comparison of the entities yielded significant results for extraversion (humans > robots; r = 0.35). Also, the non-significant results of the four personality traits seemed worth considering: agreeableness (human > robot; r = 0.26), consciousness (human > other motifs; r = 0.30), neuroticism (other motifs > robots; r = 0.30), and intellect (human > robots; r = 0.30). Furthermore, intelligent voice assistants did elicit higher levels of positive emotional reactions than negative reactions with meaningful differences noted for a negative effect (human > robots; r = 0.29). In line with the results of content analyses, most human entities were rated to be female and most other motifs were rated to be diverse.
To answer RQ2b, we focused on similarities between the drawers' self-perception and their perception of the voice assistant. Thus, correlations of the variables, which participants answered for themselves and for the voice assistants, were calculated as follows: age, personality, and gender. As Table 4 reveals, the associations were rather weak, with two exceptions: intellect/imagination correlated negatively to a significant extent, revealing that the higher participants rated their own intellect, the lower the rating of the intellect of voice assistants and vice versa. Although not significant, the positive correlation of agreeableness seems worth noting: the more agreeable participants rated themselves, the more agreeable they assessed the voice assistant. To analyze gender effects, Chi-Square Tests were conducted, testing for the effects of participants' gender and the type of device (Amazon Echo/Alexa vs. Google Home) on gender ascriptions1. Because no participants chose the self-designation “diverse,” analysis was limited to “male” and “female” gender categories. The results indicate no significant effect of participants' gender, = 2.54, p = 0.329, although significantly more women (19 out of 36) than men (1 out of 16) assigned their own gender to the devices. Also, the effect of the device was not significant, = 5.57, p = 0.061. However, the p-value indicates a nearly significant effect, highlighting the descriptive difference where 16 out of 25 Amazon Echos were rated as female, whereas 13 out of 27 Google Homes were rated as gender neutral.
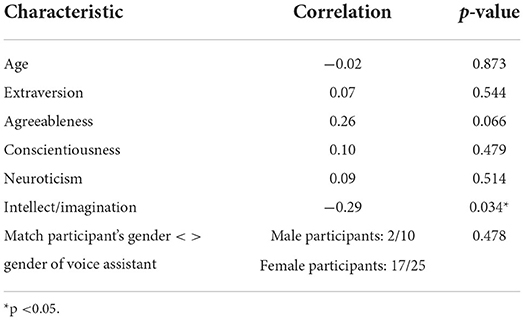
Table 4. Associations between the participants' characteristics and their visualization of the voice assistant.
To conclude, participants' self-concepts seem to be loosely linked with voice assistants, indicating that mental representations of voice assistants seem to be more than mere projections of one's own characteristics on the device.
Study 2—Widening the perspective on characteristics of voice assistants by incorporating an external perspective
Study 1 provided insights into the visualization and characterization of voice assistants. However, results were obtained from only one sample of participants, who evaluated the assistants by referring to their own picture drawn. Study 2 widened the perspective by consulting a second sample of participants, who assessed the images obtained in Study 1, and provided an external perspective. Every participant was presented with one image and was asked for his/her impression and idea of what the image showed (RQ3a). At this stage of the experiment, participants were neither informed about the origin of the image nor about the content. Then, they were debriefed and asked to assess the characteristics of the drawn voice assistants just as participants of Study 1 had done before (RQ3b). As before in Study 1, the external raters' assessments of their own characteristics were compared to their assessments of the characteristics of the smart assistant (refer to RQ3c).
Method
Participants
A total of 97 students (70 female, 27 male) aged between 18 and 29 years (M = 20.92 years, SD = 1.90) participated in Study 2. They were students of media communication (n = 79) or human computer systems (n = 18) and received course credits for their participation. Compared to Study 1, they were slightly more experienced with voice assistants in general (most experienced with Amazon Echo/Alexa: M = 2.79; SD = 1.05; Google Home: M = 1.7; SD = 1.03).
Measures: Participant's characteristics and assessment of voice assistants
The measures from Study 1 were transferred to Study 2 (see Section Measures: participant's characteristics). In line with Study 1, participants were first asked for a self-assessment. Table 5 summarizes the measures, the scales, and their reliabilities. Then, participants were shown a prototypical picture from Study 1 (see Figure 4) and were asked to associate what the picture showed (“What do you see in the picture?”). To avoid restrictions on associations, answers were allowed to be given in a free text format. After being told that the image showed a voice assistant, participants then evaluated the voice assistants by answering the measures used in Study 1 (see Section Material: Selection of prototypical images and Table C in the Appendix).
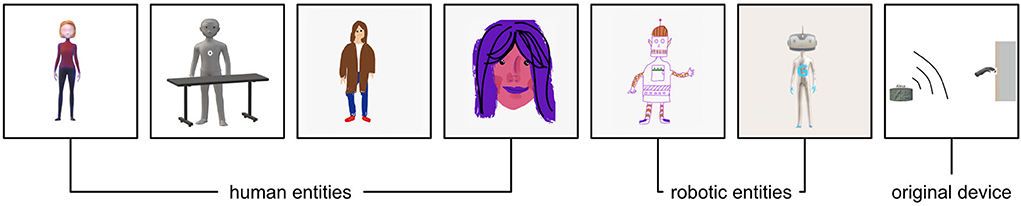
Figure 4. Shows the random chosen subsample of the prototypical pictures that have been used for Study 2. Four pictures were chosen from the most heterogeneous visualizations of human entities. Additionally, two examples of the robotic motifs and one example showing the original device (category: other motifs) were selected.
Procedure
As in Study 1, an online survey briefly introduced the broad purpose of the study and the study's procedure. Then, participants answered the first questionnaire, which asked for self-assessments. Afterward, participants were randomly assigned to one prototypical picture from Study 1. They did not know what the picture depicted. They were encouraged to make associations relating to the image (“What do you see in the picture?”). Afterward, they learned that the image showed the visualization of a voice assistant and that the following part of the questionnaire would ask for their evaluation and assessments of this specific picture. Finally, the investigator thanked them and said goodbye to the participants.
Material: Selection of prototypical images
When asked for the visual appearance of voice assistants, Study 1 showed that participants most frequently associated humans, followed by robotic entities, almost entirely independent of the device presented. Consequently, Study 2 narrowed the focus, concentrating on robotic and human content. In line with Study 1, a random subsample of the prototypical pictures was drawn (Figure 4). Four pictures were chosen from the most heterogeneous visualizations of human entities. Additionally, two examples of the robotic motifs and one example showing the original device (category: other motifs) were selected.
Results
In the following, external perspectives on the visualization of voice assistants (RQ3a), their characterizations (RQ3b), and the associations between the external raters' characterizations of both themselves and the voice assistants are presented (RQ3c).
Interpretation of the visualizations of voice assistants—RQ3a
Without knowing the drawer's intentions, participants wrote down what they saw in the picture. A comparison of their interpretations with the raters' codings in Study 1 revealed a substantial overlap: 70% of the pictures, which were coded to show human entities, were again identified as “human” (associations were words like “human,” “woman,” or “wearing clothes”). The remaining 30%, which were not clearly identified as human content, are regarded as a confirmation of the confidence ratings of Study 1, which indicated a certain level of ambiguity in the assignment. Approximately 96% of the participants assigned to a picture that was coded as showing a robot also identified it as a robot (using words such as “robot,” “artificial,” and “technology”). Finally, 80% of the participants rating an image depicting the voice assistant itself agreed with the prior rating (e.g., “Amazon Alexa,” “voice assistant,” and “voice commands”). In sum, the results of the above content analyses were confirmed.
Characteristics associated with voice assistants—RQ3b and RQ3c
After the debriefing, external raters assessed the characteristics associated with intelligent voice assistants. Table 6 presents the evaluations of the user experience, personality, and gender ascribed to voice assistants. Compared with Study 1, user experience was rated slightly lower (see Table 3). In line with the results from Study 1, the pragmatic quality of human visualizations was rated the highest again, indicating this as the only significant difference of experience aspects analyzed (humans > robots; r = 0.29). The hedonic quality stimulation and identity did not differ significantly between the visualizations. Assessments of personalities differed more distinctly. Compared with Study 1, human entities were rated the highest in all subscales except neuroticism, although only the difference in agreeableness was almost significant (humans > original device; r = 0.23). In line with Study 1, the gender of the human entities was mostly rated as female or male. Robotic entities, which were predominantly rated to be diverse in Study 1, were mostly rated to be male in Study 2.
To answer RQ3c, we focused on similarities between the participants' self-perception and their perception of the voice assistant. Table 7 shows that participants' self-assessment of personality traits or gender did not correlate with their assessment of the personalities or gender of the voice assistants. Similar to Study 1, self-description and the description of voice assistants barely overlapped (see Table 4). Again, these results were taken as evidence that mental representations of the devices seem to be more than mere projections of one's own characteristics on the device.
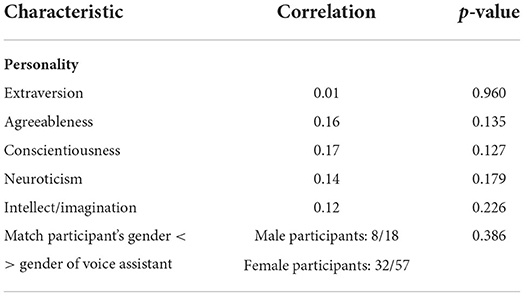
Table 7. Associations between the participants' characteristics and their visualization of the voice assistant.
Discussion
Based on the idea of media equation and the conceptualization of technology as “social actors” effects (e.g., Reeves and Nass, 1996), and on research that revealed that the appearance of technology shapes users' emotional-cognitive processes and determines the acceptance and experience of usage (Schaipp and Plaum, 2000; Woods et al., 2004; Phillips et al., 2017), this study focuses on participants' mental image of intelligent voice assistants. These devices exhibit the basic conflicting cues of media equation research such as human-like cues (speech) on the one hand and a distinctly technological appearance (loudspeaker) on the other, raising the question about potential psychological effects; e.g., what or whom users mentally refer to when interacting with their smart speaker. To bridge this gap, the present study adopts the core idea of “associative drawing” and aims for the visualization of intelligent voice assistants (Amazon Echo vs. Google Home). To allow more detailed insights into the mental representations of voice assistants, drawers and an independent second sample of participants (i.e., external raters) evaluated the associative drawings. First, the appearances of the visualizations were investigated (RQ1a, external perspective: RQ3a), and second, their characteristics were examined (RQ2a, external perspective: RQ3b) and associated with intelligent voice assistants. In addition, potential links between the participant's characteristics and those associated with the intelligent voice assistants were investigated (RQ1b, RQ2b, external perspective: RQ3c).
Summarizing the main results, the content analyses revealed that most participants associated a human being (~50%), a humanoid, or an anthropomorphic robot (~20%) with the device. The Amazon Echo, or “Alexa,” was slightly more often drawn as a human being (18 vs. 14). Animals, abstract graphics, and the depiction of the original device or an object were rare. Drawings rated as human revealed differences between the Amazon Echo and the Google Home. Echos were predominantly female, Google Homes were male, female, or neutral in equal shares. While Echo images were predominantly colored in red and yellow, Google images were predominantly blue. The results seem to confirm the rather human-like staging of Amazon's Echo in contrast to Google's neutral presentation of the device. Robots were the second most common motif. Six images of the Echo and seven images of the Home showed rather human-like and gender-neutral robots. Again, colors were cooler when a Google Home was drawn. After they had visualized the device, participants were asked to rate its characteristics. Comparisons of pictures showing human and robot entities (vs. other motifs) revealed that user experience, which was rated above average for all three motifs, was the highest for pictures showing a human. Moreover, the results showed that participants ascribed essentially human characteristics to the devices. They described devices to be rather trustworthy, conscious, agreeable, and intellectual, but not neurotic. A comparison of the motifs resulted in the following differences: human motifs were rated to be significantly more extraverted, agreeable, and intellectual than robots, as well as more conscious than other motifs. In general, higher levels of positive emotional reactions than negative reactions were observed. However, pictures showing a human motif were characterized more negatively than robots.
In Study 2, a selection of prototypical pictures was reassessed by a second group of participants who were not informed about what the drawers of the images intended to visualize. Their interpretations of the images substantially overlapped with the results of the content analyses: pictures, which the raters of Study 1 coded as showing human or robotic entities, were again interpreted as humans and robots, respectively. Pictures showing human entities were rated the highest in terms of extraversion, agreeableness, conscientiousness, and intellect and the lowest in neuroticism.
To conclude, the associative drawing of technological devices can be considered as a fruitful approach. Conceivable concerns, where participants could refuse the experimental task due to inappropriateness or incomprehension, were unfounded. To gain more detailed insights, future research could build upon the method presented, offering a promising approach to analyze a range of mental representations, to which explicit measures (questionnaires) barely have access to. Furthermore, non-verbal measures offer opportunities to survey participants limited in their reading capabilities (e.g., children). To validate the method, future research should explore non-student samples and a larger variety of technological devices. In addition, the computer-based drawing procedure used in our study could be complemented by analogous approaches using paper and pencil to address rather less technology-savvy subsamples (e.g., elderly people).
In the next step and with reference to the pictures drawn or presented before, participants of both studies were instructed to rate a list of characteristics describing the voice assistant. Most remarkably, both groups of participants ascribed human characteristics to the devices, which were rated as rather trustworthy, conscientious, agreeable, and intelligent. Particularly, when the image drawn before showed a human entity, voice assistants were assessed to be more extroverted, more agreeable, more conscious, and more intelligent. However, this was true only for participants who had drawn a picture themselves. Participants who were merely presented with the picture only rated the agreeableness and the intellect of human entities the highest. When focusing on potential causes of different visualizations of the device, the type of device (Echo vs. Google Home) explained very little variance. Only the ascription of gender was affected, with Echos (Alexas) rated to be female more often than Google Homes, which were more often rated to be male.
Analysis of the interindividual differences between the participants drawing humans vs. robots vs. other motifs revealed a greater variance. In sum, meaningful differences were found for conscientiousness and intellect (participants who drew robots were the most conscientious and the most intellectual) and neuroticism (participants who drew humans were the most neurotic). Furthermore, participants who drew “other motifs” rated their affinity to technology and their innovation ability the highest and their institutional trust the lowest. Interestingly, participants who drew robots characterized themselves to be the most conscientious and the most intellectual, and participants who drew humans rated themselves as the most neurotic. Moreover, those who drew other motifs were salient, exhibiting the highest affinity to technology, the highest innovation ability, and the lowest institutional trust. These results indicate that different individuals have different associations when it comes to intelligent voice assistants. Different associations may be linked to different expectations and could have different consequences for the acceptance of devices, for the way the device is used, or for the cognitive, emotional, and behavioral consequences of its use (e.g., Phillips et al., 2017). To be diagnostically more conclusive, future work should consider a more differentiated analysis of potential interindividual differences and an investigation of potential corresponding consequences for different user groups.
Concluding interpretation of the results
The results indicate that participants did not associate a mere technological device with voice assistants, but rather an allegedly social counterpart with human-like characteristics. Asking for the origin of these associations, we asked for potential projections of the participants' self-image onto the device.
The idea of a mere projection can be ruled out by our findings, which show that participants' characteristics on the one hand, and characteristics associated with voice assistants on the other, did not correlate meaningfully, neither for the drawers themselves (Study 1) nor for the external raters (Study 2). Reviewing this result critically, people may regard similarities or overlaps between themselves and a technological device as rather absurd. However, the small sample sizes and the resulting weak statistical power make inference statistical analyses difficult. Nevertheless, the attribution of human characteristics and the variety of associated appearances suggest the interpretation that drawers and external raters associate a separate entity, which seems to be rather independent of their own personalities. Moreover, these entities feature human or humanoid characteristics, arguing for the Media Equation approach and unconscious reactions similar to human–human interactions. Carrying on the work of Woods et al. (2004) who showed that different usage scenarios are associated with different expectations, future research should further investigate how different contexts of use and different usage experiences (which could be manipulated in an experimental setup) shape mental representations. For example, studies may analyze how a private social context of use differs from an industrial or military context: will the same device be associated with a human entity if the context is social? And what will people associate with a military context? Consequently, beyond the mere appearance, the context of use, the cues, and the function of the device used should be considered when users' expectations toward technology and mental representations of the devices are investigated.
Although our results clearly demonstrated human-like attributions to intelligent voice assistants, we can only speculate about their meaning and their importance. Future research should address the potential consequences of attributions in detail. One way to do so could be through the experimental approaches CASA studies have established. This may be considered by adopting social psychological concepts and theories referring to human–human interaction transferred to human–device interaction to analyze what kind of human attributes are associated with voice assistants and how they affect the users' cognitive, emotional, and behavioral reactions to the device, in imaginary, short-term, or long-term interactions. The rather subliminal, human-centered representations, which seem to be part of our understanding of technology, should result in a more distinct focus on the “human” in human–computer interactions, particularly regarding the current discussion on artificial intelligence.
Limitations
Starting with methodological limitations, the experimental instruction needs to be reviewed critically. Participants were only allowed to visually and haptically inspect the device but not to use it, test its features, or hear its voice. We argued that our idea was to elicit well-established mental images or concepts of the technology in participants. However, as most of them were rather inexperienced, one could question the profoundness of their associations, and ask what the associations are based on. By preventing interactions, we could control the interindividual variance during the experiment, but some questions remain to be answered. Thus, future research needs to account for this limitation by giving participants the opportunity to interact with the device so that they can have their own experiences. Then, drawings would reflect impressions based on personal experiences and not so much on prior (limited) knowledge based on media reports or hearsay.
The analyses revealed low internal reliabilities for some scales (e.g., personality scales, eeriness, or attractiveness in Study 1). Since we wanted to use well-established scales, we did not eliminate certain items to increase the Cronbach's Alpha value of the scales (Cortina, 1993). Nevertheless, the limited quality of the scales therefore influences the measurement accuracy and the results. Although scale development was not the focus of this study, it should be considered in future studies. Beyond reliability problems, fundamental questions regarding the validity of the measures should be investigated more thoroughly to prove the appropriateness of scales that are well-established in social science, but transferred to the context of human–computer interaction research. Additionally, while the sample size for qualitative analysis was relatively high, possibilities for inference statistical analyses were limited. Both samples were limited to young, higher-educated, and rather technology-savvy participants from western culture. Since our results indicated that different visualizations of potentially different mental representations are accompanied by individual differences, a more diverse sample may result in more informative insights. Another limitation results from the unspecified voice assistant we presented to our participants. The context of use, its features or functions, and the accompanying cues were not specified in the experimental instruction. Consequently, the concrete aspects of the wide range of contexts, features, and usage could have been rather heterogeneous, resulting in an uncontrolled variance in our data. Thus, future studies need to ask participants for their thoughts and beliefs linked with their associations. Additionally, different user groups need to be distinguished and compared (e.g., users vs. non-users; experts vs. non-experts; younger vs. older users). Finally, the approaches implemented to investigate the implicit associations were limited to a computer-based drawing program. As we have already stated, analog approaches (paper and pencil) seem to be promising, especially when it comes to less technology-savvy participants. Besides, other methodological approaches such as implicit measures (Fazio and Olson, 2003) are worth considering.
Conclusion
To conclude, when we asked whom or about what you may think about when interacting with smart devices, our study has revealed the following: You most likely think of a human or a humanoid entity to which you accordingly ascribe a human visual appearance and characteristics. If others were confronted with your associations, they would not realize that the origin of the drawing is actually a technological device. They would rather identify the drawing as a human or robotic being. Also, it is most likely that others would also ascribe originally distinct human characteristics to what somebody else imagines a smart device to “look” like, even though they knew that the picture shows a technological device and not a real human being. Furthermore, the idea that these ascriptions may be mere projections of one's own characteristics could not be confirmed: both the way participants imagined the devices and their interpretations from an external point of view do not simply mirror self-concepts. Instead, there are only marginal overlaps, and participants seem to ascribe a distinct identity, gender, and personality to the devices. The boundaries between technology on the one hand, and humanity on the other, seem to diminish when it comes to the mental representation of modern technologies. In line with assumptions in the Media Equation approach, this “essential humanness” seems to be attributed to voice assistants. Beyond the outward appearance of smart speakers, which is distinctly technological, there seems to be a mental representation of technology, which assigns both outer and inner concepts and characteristics, well established in human–human interactions. We carefully interpret these results as indicators that the actual outward appearance of a device or its functions determine the understanding and consideration of technological entities, but that particular features which simulate essential characteristics such as speech are highly relevant.
Consequently, the conceptualization of modern technology, its development and implementation, as well as the research approaches deployed to analyze its use and its consequences need to be widened substantially. It is probably neither the mere hardware that users think of, nor the software or algorithms “behind” the device. Instead, it could rather be an entity with concrete characteristics, a certain personality, or a certain gender or age that people have in mind when using certain devices and applications. What we know about human beings; how they think, feel and act, on their own as well as within social structures, becomes even more relevant than the conceptualization of human–computer interactions that have mostly been accounted for to date.
Theoretical and methodological approaches need to be scrutinized regarding how well they account for their adopters' mental processes. The link between computer science and social science needs to be strengthened even more. Particularly regarding the current discussion on artificial intelligence, the focus seems to be too limited to technological, mathematical, or computational reasoning. Of course, these aspects are of constituting importance. However, already at this stage of the process, “human biases” may occur: computational scientists, developers, and designers, for example, may (unconsciously) incorporate their mental representations into their work (research on the media equation has shown that technological expertise does not automatically mean that one does not have this tendency). Also, the sample of this study was rather experienced with digital media and technology in general, and they nevertheless illustrated the visual appearance with only a small minority drawing the actual device they had been presented with. For the average end consumer using the device within private surroundings and for everyday tasks, the effects may be even greater. By examining associations toward voice assistants, our study argues for a more elaborate understanding of unconscious cognitive and emotional processes elicited by technology. The humanness individuals see in their technological counterparts needs to be accounted for to further develop human–computer interactions and to responsibly shape them regarding psychological, societal, as well as ethical needs and standards for our shared digital future.
Data availability statement
The raw data supporting the conclusions of this article will be made available by the authors, without undue reservation.
Ethics statement
Ethical review and approval was not required for the study on human participants in accordance with the local legislation and institutional requirements. The patients/participants provided their written informed consent to participate in this study.
Author contributions
AC: conceptualization, data curation, formal analysis, funding acquisition, investigation, methodology, project administration, resources, software, supervision, validation, visualization, writing—original draft, and writing—review and editing. CW: conceptualization, formal analysis, investigation, methodology, validation, visualization, and writing—review and editing. All authors contributed to the article and approved the submitted version.
Funding
This publication was supported by the Open Access Publication Fund of the University of Wuerzburg.
Acknowledgments
We would like to thank Anna-Maria Toerke and Felix Ebner for their support.
Conflict of interest
The authors declare that the research was conducted in the absence of any commercial or financial relationships that could be construed as a potential conflict of interest.
Publisher's note
All claims expressed in this article are solely those of the authors and do not necessarily represent those of their affiliated organizations, or those of the publisher, the editors and the reviewers. Any product that may be evaluated in this article, or claim that may be made by its manufacturer, is not guaranteed or endorsed by the publisher.
Supplementary material
The Supplementary Material for this article can be found online at: https://www.frontiersin.org/articles/10.3389/fcomp.2022.981435/full#supplementary-material
Footnotes
1. ^Images where the gender was not codable (Amazon: 4; Google: 3) were excluded from the analyses.
References
Agarwal, R., and Prasad, J. (1998). A conceptual and operational definition of personal innovativeness in the domain of information technology. Inform. Syst. Res. 9, 204–215. doi: 10.1287/isre.9.2.204
Attig, C., Mach, S., Wessel, D., Franke, T., Schmalfuß, F., and Krems, J. F. (2018). Technikaffinität als Ressource für die Arbeit in Industrie 4.0. Innteract 3. doi: 10.14464/awic.v3i0.251
Backhaus, N. (2017). Nutzervertrauen und –erleben im Kontext technischer Systeme: Empirische Untersuchungen am Beispiel von Webseiten und Cloudspeicherdiensten. Berlin: Technische Universität Berlin.
Bailey, R. J., Grimm, C. M., and Davoli, C. (2006). The real effect of warm-cool colors. Comput. Sci. Eng. Res. doi: 10.7936/K7736P3B
Bär, N., Hoffmann, A., and Krems, J. (2011). “Entwicklung von Testmaterial zur experimentellen Untersuchung des Einflusses von Usability auf Online-Trust,” in Reflexionen und Visionen der Mensch-Maschine-Interaktion – Aus der Vergangenheit lernen, Zukunft Gestalten, Eds S. Schmid, M. Elepfandt, J. Adenauer, and A. Lichtenstein (Düsseldorf: VDI Verlag) 627–631.
Beierlein, C., Kemper, C. J., Kovaleva, A., and Rammstedt, B. (2012). Kurzskala zur Messung des zwischenmenschlichen Vertrauens: Die Kurzskala Interpersonales Vertrauen (KUSIV3). Mannheim: GESIS – Leibniz-Institut für Sozialwissenschaften.
Breyer, B., and Bluemke, M. (2016). Deutsche Version der Positive and Negative Affect Schudule PANAS (GESIS Panel): Zusammenstellung Sozialwissenschaftlicher Items und Skalen. Mannheim: GESIS – Leibniz-Institut für Sozialwissenschaften.
Carolus, A., Muench, R., Schmidt, C., and Schneider, F. (2019). Impertinent mobiles - effects of politeness and impoliteness in human-smartphone interaction. Comput. Human Behav. 93, 290–300. doi: 10.1016/j.chb.2018.12.030
Carolus, A., Schmidt, C., Muench, R., Mayer, L., and Schneider, F. (2018a). “Pink Stinks - at least for men,” in Human-Computer Interaction. Interaction in Context, Eds M. Kurosu (Cham: Springer), 512–525.
Carolus, A., Schmidt, C., Schneider, F., Mayr, J., and Muench, R. (2018b). “Are people polite to smartphones?,” in Human-Computer Interaction. Interaction in Context, Eds M. Kurosu (Cham: Springer), 500–511.
Carolus, A., Wienrich, C., Toerke, A., Friedel, T., and Schwietering, C. (2021). ‘Alexa, I feel for you!'-Observers' empathetic reactions towards a conversational agent. Front. Comput. Sci. 3, 46. doi: 10.3389/fcomp.2021.682982
Cohen, J. (1988). Statistical Power Analysis for the Behavioral Sciences: Statistical Power Analysis for the Behavioral Sciences, 2 Edn. Hillsdale, NJ: Lawrence Eribaum Associates.
Cortina, J. M. (1993). What is coefficient alpha? An examination of theory and applications. J. Appl. Psychol. 78, 98–104. doi: 10.1037/0021-9010.78.1.98
Cronbach, L. J. (1951). Coefficient alpha and the internal structure of tests. Psychometrika 16, 297–334. doi: 10.1007/BF02310555
Donnellan, M. B., Oswald, F. L., Baird, B. M., and Lucas, R. E. (2006). The mini-IPIP scales: tiny-yet-effective measures of the big five factors of personality. Psychol. Assess. 18, 192. doi: 10.1037/1040-3590.18.2.192
Erol, B. A., Wallace, C., Benavidez, P., and Jamshidi, M. (2018). “Voice activation and control to improve human robot interactions with IoT perspectives,” in 2018 World Automation Congress (WAC). (Stevenson, WA), 322–327.
Fazio, R. H., and Olson, M. A. (2003). Implicit measures in social cognition research: their meaning and use. Annu. Rev. Psychol. 54, 297–327. doi: 10.1146/annurev.psych.54.101601.145225
Frank, L. K. (1939). Projective methods for the study of personality. J. Psychol. Interdiscip. Appl. 8, 389–413. doi: 10.1080/00223980.1939.9917671
Gawronski, B., and De Houwer, J. (2014). “Implicit measures in social and personality psychology,” in Handbook of Research Methods in Social and Personality Psychology, 2 Edn, Eds H. T. Reis and C. M. Judd (New York, NY: Cambridge University Press), 283–310.
Goetz, J., Kiesler, S., and Powers, A. (2003). “Matching robot appearance and behavior to tasks to improve human-robot cooperation,” in RO-MAN 2003. 12th IEEE International Workshop on Robot and Human Interactive Communication, (Millbrae, CA), 55–60.
Gray, H. M., Gray, K., and Wegner, D. M. (2007). Dimensions of mind perception. Science 315, 619–619. doi: 10.1126/science.1134475
Hassenzahl, M., Burmester, M., and Koller, F. (2003). “AttrakDiff: Ein Fragebogen zur Messung wahrgenommener hedonischer und pragmatischer Qualität,” in Mensch and Computer 2003 (Wiesbaden: Vieweg+ Teubner Verlag), 187–196.
Hassenzahl, M., Burmester, M., and Koller, F. (2008). Der User Experience (UX) auf der Spur: Zum Einsatz von www.attrakdiff.de. Available online at: www.attrakdiff.de
Henshilwood, C. S., D'Errico, F., van Niekerk, K. L., Dayet, L., Queffelec, A., and Pollarolo, L. (2018). An abstract drawing from the 73,000-year-old levels at Blombos Cave, South Africa. Nature 562, 115–118. doi: 10.1038/s41586-018-0514-3
Herbert, G., and Chen, X. (2015). A comparison of usefulness of 2D and 3D representations of urban planning. Cartogr. Geogr. Inf. Sci. 42, 22–32,. doi: 10.1080/15230406.2014.987694
Ho, C.-C., and MacDorman, K. F. (2010). Revisiting the uncanny valley theory: developing and validating an alternative to the Godspeed indices. Comput. Human Behav. 26, 1508–1518. doi: 10.1016/j.chb.2010.05.015
Krämer, N. C., Rosenthal-von der Pütten, A. M., and Hoffmann, L. (2015). “Social effects of virtual and robot companions, in The Handbook of the Psychology of Communication Technology, Ed S. S. Sundar (Chichster: Wiley), 137–159.
Krippendorff, K. (1989). “Content analysis,” in International Encyclopedia of Communication, 1. Available online at: http://repository.upenn.edu/asc_papers/226
Massironi, M. (2002). The Psychology of Graphic Images: Seeing, Drawing, Communicating. New York, NY: Psychology Press.
Nass, C., Fogg, B. J., and Moon, Y. (1996). Can computers be teammates? Int. J. Hum. Comput. Stud. 45, 669–678. doi: 10.1006/ijhc.1996.0073
Nass, C., and Gong, L. (2000). Speech interfaces from an evolutionary perspective. Commun. ACM 43, 36–43. doi: 10.1145/348941.348976
Nass, C., Moon, Y., and Green, N. (1997). Are machines gender neutral? Gender-stereotypic responses to computers with voices. J. Appl. Soc. Psychol. 27, 864–876. doi: 10.1111/j.1559-1816.1997.tb00275.x
Nass, C., Steuer, J., and Tauber, E. R. (1994). “Computer are social actors,” in CHI ‘94: Proceedings of the SIGCHI Conference on Human Factors in Computing Systems (New York, NY: ACM Press), 72–78.
Nielsen (2018a). Nielsen Launches New MediaTech Trender Survey to Uncover Consumer Sentiment on Emerging Technologies.
Nielsen (2018b). (Smart) Speaking My Language: Despite Their Vast Capabilities, Smart Speakers Are All About the Music. In.
Paoletti, J. B. (2012). Pink and Blue: Telling the Boys From the Girls in America. Bloomington, IN: Indiana University Press.
Petermann, F. (1997). Die Familiensituation im Spiegel der Kinderzeichnung. Zeitschr. Differ. Diagn. Psychol. 18, 90–92.
Phillips, E., Ullman, D., de Graaf, M. M. A., and Malle, B. F. (2017). What does a robot look like?: A multi-site examination of user expectations about robot appearance. Proc. Hum. Fact. Ergon. Soc. Ann. Meet. 61, 1215–1219. doi: 10.1177/1541931213601786
Porcheron, M., Fischer, J. E., Reeves, S., and Sharples, S. (2018). Voice Interfaces in Everyday Life, Vol. 2018–April. New York, NY: Association for Computing Machinery.
Pradhan, A., Findlater, L., and Lazar, A. (2019). “Phantom Friend” or “Just a Box with Information”: personification and ontological categorization of smart speaker-based voice assistants by older adults. Proc. ACM Hum. Comput. Interact. 3, 214. doi: 10.1145/3359316
Reeves, B., and Nass, C. (1996). The Media Equation: How People Treat Computers, Television, and New Media Like Real People and Places. Cambridge University Press. Available online at: https://psycnet.apa.org/record/1996-98923-000
Revers, W. J. (1973). Der Thematische Apperzeptionstest (TAT): Handbuch zur Verwendung des TAT in der Psychologischen Persönlichkeitsdiagnostik. Bern: Huber.
Rorschach, H. (1921). Psychodiagnostik: Methodik und Ergebnisse Eines Warhrnehmungsdiagnostischen Experiments (Deutenlassen von Zufallsformen), Vol. 2. E. Leipzig: Bircher.
Rosenthal-von der Pütten, A. M., Krämer, N. C., Hoffmann, L., Sobieraj, S., and Eimler, S. C. (2013). An experimental study on emotional reactions towards a robot. Int. J. Soc. Robot. 5, 17–34. doi: 10.1007/s12369-012-0173-8
Roto, V., Law, E., Vermeeren, A. P. O. S., and Hoonhout, J. (2011). “User experience white paper: bringing clarity to the concept of user experience,” in Dagstuhl Seminar on Demarcating User Experience, 12. Available online at: http://www.allaboutux.org/files/UX-WhitePaper.pdf
Schaefer, K. E., Sanders, T. L., Yordon, R. E., Billings, D. R., and Hancock, P. A. (2012). Classification of robot form: Factors predicting perceived trustworthiness. Proceed. Human Fact. Ergon. Soc. Ann. Meet. 56, 1548–1552. doi: 10.1177/1071181312561308
Schaipp, C., and Plaum, E. (2000). Sogenannte projektive techniken: verfahren zwischen psychometrie, hermeneutik und qualitativer heuristik. J. Psychol. 8, 29–44. https://nbn-resolving.org/urn:nbn:de:0168-ssoar-28558
Taylor, J., Weiss, S. M., and Marshall, P. J. (2020). “Alexa, how are you feeling today?”: mind perception, smart speakers, and uncanniness. Interact. Stud. 21, 329–352. doi: 10.1075/is.19015.tay
Tharp, M., Holtzman, N. S., and Eadeh, F. R. (2017). Mind perception and individual differences: a replication and extension. Basic Appl. Soc. Psych. 39, 68–73. doi: 10.1080/01973533.2016.1256287
Watson, D., Clark, L. A., and Tellegen, A. (1988). Development and validation of brief measures of positive and negative affect: the PANAS scales. J. Pers. Soc. Psychol. 54, 1063–1070. doi: 10.1037/0022-3514.54.6.1063
Keywords: media equation, conversational agents, smart speakers, visualization of technology, embodiment
Citation: Carolus A and Wienrich C (2022) “Imagine this smart speaker to have a body”: An analysis of the external appearances and the characteristics that people associate with voice assistants. Front. Comput. Sci. 4:981435. doi: 10.3389/fcomp.2022.981435
Received: 29 June 2022; Accepted: 24 October 2022;
Published: 24 November 2022.
Edited by:
Mark Billinghurst, University of South Australia, AustraliaReviewed by:
Peter J. Marshall, Temple University, United StatesJebediah Taylor, University of Michigan Law School, Unites States, in collaboration with reviewer PM
Yuqing Mao, National Institutes of Health (NIH), United States
Copyright © 2022 Carolus and Wienrich. This is an open-access article distributed under the terms of the Creative Commons Attribution License (CC BY). The use, distribution or reproduction in other forums is permitted, provided the original author(s) and the copyright owner(s) are credited and that the original publication in this journal is cited, in accordance with accepted academic practice. No use, distribution or reproduction is permitted which does not comply with these terms.
*Correspondence: Astrid Carolus, YXN0cmlkLmNhcm9sdXNAdW5pLXd1ZXJ6YnVyZy5kZQ==