- 1Critical Alarms Lab, Faculty of Industrial Design Engineering, Delft University of Technology, Delft, Netherlands
- 2Department of Architecture and Arts, Iuav University of Venice, Venice, Italy
- 3Department of Adult Intensive Care, Erasmus Medical Center, Rotterdam, Netherlands
There is an increased awareness of how the quality of the acoustic environment impacts the lives of human beings. Several studies have shown that sound pollution has adverse effects on many populations, from infants to adults, in different environments and workplaces. Hospitals are susceptible environments that require special attention since sound can aggravate patients' health issues and negatively impact the performance of healthcare professionals. This paper focuses on Neonatal Intensive Care Units (NICU) as an especially sensitive case representing a hostile acoustic environment in which healthcare professionals have little awareness of how unwanted sounds impact the perceived quality of the soundscape. We performed a semi-systematic review of scientific literature on sound assessment studies in NICU from 2001. A thematic analysis was performed to identify emerging themes that informed the analysis of 27 technological solutions for the assessment of sound quality in indoor and outdoor environments. Solutions were categorized by functions and evaluation methods and grouped according to the characteristics of the design components, i.e., acquisition, computation, and communication strategies. Results highlight a lack of solutions to assess the qualitative characteristics of indoor environments such as NICU and forecast the footprint that different sound sources have on the indoor soundscape. Such solutions are urgently needed to empower healthcare professionals, and especially nurses, to actively modify and prevent the negative impact of unwanted sounds on NICU and critical care soundscape.
1. Introduction
We, as a society, have developed a certain tolerance toward unwanted sounds. Yet, several studies have shown that sound pollution-defined by sound level thresholds beyond which the exposure to sound can negatively impact health (Kang et al., 2016)-has adverse effects on many populations, from infants to adults (Gupta et al., 2018; Teixeira et al., 2021), in different environments and workplaces. Within the broader spectrum of sound studies, soundscape research considers environmental sound “as resource rather than a waste” (COST TUD Action, 2013), with various actions and interventions put in place to actively improve it (Hellström et al., 2014; Moshona et al., 2022; Henze et al., 2023), instead of “silencing” it (Thibaud and Amphoux, 2013). Rather than quantitively measuring environmental sound levels, soundscape studies approach the assessment of the acoustic environment by identifying qualitative descriptors (e.g., pleasantness, perceived annoyance, appropriateness, quietness, and so on) and investigating their potential correlation with the “footprint” of different sound events (i.e., human- or machine-generated, and natural sounds) to characterize the acoustic environment as listeners perceive it (Aletta et al., 2016).
In the past decade, this approach has informed the development of novel algorithms for the automatic assessment of the acoustic environment. These algorithms are typically modeled on the outdoor soundscape and build on the growing availability of low-cost sensors for the continuous monitoring of sound levels (De Coensel and Botteldooren, 2014; De Coensel et al., 2015). This line of research aims to develop intelligent systems (Wei and Van Renterghem, 2014; Socoró et al., 2017; Quinn et al., 2022) and novel indices (Brocolini et al., 2012; Graziuso et al., 2022) to characterize, interpret, and forecast the acoustic environment beyond traditional sound level measurements and closer to how sound is perceived by humans. A recent review of 24 studies on predictive models of urban soundscapes (Lionello et al., 2020) indicates that the combination of quantitative (i.e., acoustic and psychoacoustic metrics) and qualitative analysis (i.e., subjective and perceptual information on the perceived affective quality of a soundscape) greatly improves the performance of algorithms to model and predict the outdoor soundscape, compared to algorithms that only use acoustic and psychoacoustic indicators. Additionally, more reliable results seem to be achieved when descriptors of the affective quality of the soundscape are combined with the categorization of sound sources and information on the appropriateness to a specific context.
Within professional socio-technological environments (i.e., functional settings with a specific mission that relies on time-sensitive actions and teamwork) such as the hospital, it becomes critical to identify and define the role of humans as both recipients and producers of sound events who impact the quality of the soundscape. Such identification will eventually lead to increased awareness among healthcare professionals on the impact of sound on the functionality of their shared acoustic space, and improved guidelines for the design of more “actionable” (Özcan et al., 2022b) healthcare spaces. Previous research on auditory affordances supports the claim that people understand and relate to soundscapes through their potential to induce and guide action (Rosenblum et al., 1996; Nielbo et al., 2013). How the potential for action relates to annoyance of sound events has also been recently investigated (Misdariis et al., 2019) with the goal of defining computational models for the evaluation of the quality of the urban soundscape.
In the hospital context, medical alarms, sounds from medical equipment, and the continuous human activity within units cause the typical soundscape to be perceived as poor (Bliefnick et al., 2019). However, existing studies on this topic mainly refer to a progressive and harmful increase in sound levels in hospitals (see Busch-Vishniac et al., 2005; Busch-Vishniac and Ryherd, 2019 for a review of the past 40 years). While research has shown how different sound sources (e.g., medical alarms) can create a stressful environment for medical staff (Johnson et al., 2017; Varisco et al., 2021) and how sounds from staff conversations and activities negatively impact the patients' soundscape quality (MacKenzie and Galbrun, 2007; Konkani et al., 2014; Lenzi et al., 2023), to the knowledge of the authors no comprehensive study has been conducted to assess, model, and predict the quality of the hospital soundscape beyond sound level measurements. In this paper we take a role to understand the status quo of the current technological solutions and the technological trend for near future applications for monitoring the sound of professional socio-technological environments. Our intention is to find opportunities to envision a targeted solution for hospitals at large and more specifically neonatal intensive care units in focus.
NICU soundscapes: problem statement
Of all shared spaces in society, neonatal intensive care units (NICU) are especially vulnerable environments in which patients, their families, and healthcare professionals are particularly subject to the harmful consequences of excessive sounds (Özcan et al., 2019; de Lima Andrade et al., 2021). The NICU is designed for premature neonates who are not necessarily ill upon their arrival. Nonetheless, they need special care to grow and survive, and their likelihood of getting ill inside the NICU is high since their bodies are not fully developed. Moreover, neonates are likely to experience physiological limitations, central nervous system limitations, and dependency on intensive care, which makes them more vulnerable to the whole NICU sound environment (Blackburn, 1998). Concerns regarding the impact of acoustic stimuli in the NICU were first addressed during the 1970s (Lawson et al., 1977) through direct observation of the environment. Later in the 1990s, an increased awareness of how environmental stimuli affect neonates' clinical conditions, and their neurodevelopment was recorded (Philbin et al., 2000). This led to several studies by which sound level measurements were carried out in the NICU with professional equipment such as sound level meters (Thomas, 1989; Thomas and Uran, 2007). Sound measurement studies continued throughout the years, providing recommendations for reducing the high sound level issue through room redesign (Chen et al., 2009), use of earmuffs (Duran et al., 2012), or the implementation of educational programs (Elander and Hellström, 1995; Calikusu Incekar and Balci, 2017).
Whereas, the benefits of training nurses and other hospital staff to decrease noise levels by incorporating behavioral changes is clear as an important first step (Carvalhais et al., 2015), previous research from the authors (Özcan et al., 2022a; Spagnol et al., 2022) shows that NICU occupants are often unaware of the contributors to the noisy sound environment and feel they have no control to change the sound quality of their environment. Thus, there is an urgent need to create a shared awareness about the contributors to the decreased sound quality in NICU to be able to take collective action. A recent study by the authors (Spagnol et al., 2022), that we further expand in Section Beyond sound measurements: a review of technological solutions, gaps, and opportunities of this paper, shows that current technological solutions for the assessment of indoor acoustic environments focus on collecting and measuring basic acoustic metrics such as sound pressure level (SPL, expressed in decibels). However, SPL measurements are difficult to interpret by non-experts (Alsina-Pagès et al., 2021). Additionally, they are not an appropriate indicator for describing the affective quality of sounds as perceived by humans (Aletta et al., 2016). Lastly, SPL measurements do not allow users to identify the cause of unwanted sounds (i.e., noxious sounds that can lead to harmful consequences). Sound identification is crucial to increase awareness on the NICU soundscape toward its active improvement, and it is a highly context-dependent cognitive function (Özcan and van Egmond, 2007, 2009; Axelsson, 2015). Finally, protocols for the analysis of the affective qualities of the soundscape, such as the Swedish Soundscape Protocol (Axelsson et al., 2010) were developed in the context of outdoor environments, and the application of such protocols to indoor environments is currently under development (Torresin et al., 2020). Therefore, we see a clear opportunity to explore and design intelligent solutions for the algorithmic modeling of indoor soundscapes and make it technologically viable.
Across the globe, critical care departments ranging from neonatal to adult care feel the urgency to improve their sound quality for better patient experiences and working conditions. Hospitals run exhaustive studies with extensive resources and human effort to be able to characterize their existing soundscape and plan interventions accordingly in the lack of available standardized solutions (Özcan et al., 2022b). Therefore, a sustainable solution that can automatically assess and characterize hospital soundscapes is needed and will be timely in the era of data-centric approaches employed in healthcare.
In this paper, we review the state of the art of technological solutions for the assessment of the NICU acoustic environment to highlight current technological gaps and identify opportunities for the design of novel solutions to improve the NICU soundscape. We first present a semi-systematic review of 77 publications on the topic of environmental sound in the NICU (Section Monitoring the sound quality of NICU: a semi-systematic review). We then define an evaluation framework and discuss the results of a review of 28 current technological solutions for the assessment of sound quality in NICU and, more broadly, in indoor and outdoor spaces (Section Beyond sound measurements: a review of technological solutions, gaps, and opportunities). The review supports the definition of the design requirements for a solution able to increase nurses' awareness of the impact of sounds on the NICU soundscape. The characteristics of this novel technological solution are discussed in Section Conclusions.
2. Monitoring the sound quality of NICU: a semi-systematic review
This section reports a semi-systematic literature review carried out to have an overview of the sound monitoring studies that had been conducted inside the NICU. Contrary to systematic reviews, which identify and analyze all the available empirical evidence to quantitatively answer specific research questions or hypotheses, a semi-systematic review has a broad research question, examines research areas and follows their evolution over time, and synthesizes the main themes from the literature using meta-narratives instead of quantitative methods (Snyder, 2019). The resulting themes allow collecting insights and limitations from the literature, which will guide the review of technological solutions in Section Beyond sound measurements: a review of technological solutions, gaps, and opportunities and will be later translated into opportunities for research and design in Section Conclusions.
2.1. Methodology
Our research started by identifying relevant studies on environmental sound in the NICU. First, to retrieve relevant titles, we queried the popular academic literature search engine PubMed with the following search string, [NICU OR (Neonat* AND “Intensive Care”)] AND (Noise OR “Sound Level*”). The query returned 77 articles. Then, we excluded (1) articles published more than 20 years ago, i.e., before 2001; (2) articles written in another language than English; (3) non-journal publications; (4) entries without a full text available; (5) duplicate entries. After this filtering phase, 59 articles were left.
Upon carefully reading all the 59 articles, we generated a table of different factors that could hint at potential research themes, such as targeted listener (e.g., neonate, nurse, family); methodologies used for assessment (e.g., measurements, questionnaires, structured interviews); devices used for sound monitoring. Finally, we further excluded those articles that did not actually report the results of environmental sound recordings inside a NICU. The final sample included 41 articles. Thematic analysis, i.e., a qualitative data analysis method, was used to code, analyze, and report patterns in the form of themes (Braun and Clarke, 2012). In particular, the coding phase consisted of highlighting sections of text and coming up with shorthand labels, or codes, to describe their content. For this task, the ATLAS.ti 9 software was used. Once codes were written for all articles, higher-level categories were formed from patterns in the codes. Lastly, categories were clustered into four main themes. When interpreting and explaining themes, insights and limitations emerged. Figure 1 schematically reports the above-described process.
2.2. Results
Table 1 reports an overview of the four themes that emerged from the thematic analysis of the literature focusing on recorded sound/noise levels in NICUs. Each theme covers several categories, which are listed below it. We now present an overview of the themes along with the corresponding insights and limitations.
Theme 1: Collecting and processing sound focuses on methods used for sound measurement and recording, along with their outcomes.
1. Measurement time spans are generally scattered, and studies hardly follow the same protocol [for instance, a 24-h period every week for a total of 44 weeks (Brandon et al., 2007), 168 consecutive hours (Aita et al., 2021), or eight separate 1-h recordings (Krueger et al., 2007)]. Currently, all we can find are studies that are episodic rather than continuous. Most of them focus on measuring sound levels to report how harmful the auditory environment can be but do not focus on implementing potentially long-lasting sound monitoring solutions.
2. Sound level meters are the most used devices for measurement, followed by sound dosimeters (Liu, 2010; Ramm et al., 2017; Smith et al., 2018). Only a few studies use available sound level monitoring solutions for healthcare (Milette, 2010; Casey et al., 2020) or smaller devices such as probe microphones (Surenthiran et al., 2003) to measure neonates' exposure to sound. Sound level meters generally provide extremely accurate yet objective measurements of the auditory environment. More intuitive interpretations are needed to give individuals a concrete means of evaluating the sound environment as they experience it.
3. The outcome variables are almost exclusively measurements expressed in decibels (dB), most often A-weighted (dBA). Only a few studies also conduct spectral analysis, therefore analyzing sound power at different frequencies (Surenthiran et al., 2003; Livera et al., 2008; Lahav, 2015). However, excluding a time-frequency analysis from a sound recording limits a complete perspective of sound events occurring in the NICU. Privacy issues might be the main reason why sound recordings are not stored and analyzed, which limits their possible use as training data for sound event detection approaches.
Theme 2: Assessing the NICU environment is about the environmental factors and experimental configurations that studies aim to assess.
4. The goal of most of the considered studies is to report sound levels exceeding the recommended thresholds. Baseline levels are established by national and international organizations such as the American Academy of Pediatrics (AAP), the US Environmental Protection Agency (EPA), or the World Health Organization (WHO) (Williams et al., 2007; Darcy et al., 2008). The general problem with these baseline levels is that they are seen as too low and therefore hard to reach within an environment that several different individuals visit or work in.
5. A substantial number of studies focus on not only reporting sound levels in a specific unit but comparing different environments: for instance, NICUs of different levels of care (Levy et al., 2003), open bay units vs. single-family rooms (Liu, 2012; Szymczak and Shellhaas, 2014), or before vs. after a structural change in the unit (Krueger et al., 2007; Aita et al., 2021). These comparisons aim to report and give evidence on the most suitable environment for the wellbeing of neonates, parents, and nurses. Unfortunately, the NICU characteristics in which every study is conducted are unique. Among the characteristics that change we can count patient census, number of beds, number of nurses working during shifts, presence of parents, to name but a few. All these factors can potentially contribute to an increase in average and/or peak sound levels. It is even more challenging to compare outcomes from different studies since they do not share the same settings.
6. In the analyzed literature, most researchers explain where measurement devices are positioned. If the goal is to measure environmental sound, devices are often positioned at the center of the room (Livera et al., 2008; Lahav, 2015). Conversely, they are placed close to neonates' heads when the goal is to measure either subjective exposure or care activities nearby the incubator area (Surenthiran et al., 2003; Liu, 2010). A few studies give a more extensive mapping by placing measurement devices in several different locations within the unit (Krueger et al., 2005; Wang et al., 2014). As single measurement devices are used, measurements in different locations are not time synchronized. The main reason for this experimental choice could be the lack of resources and/or budget. The use of a set of independent devices would give a more complete picture of sound levels and events within the unit.
Theme 3: Interactions with sound is about auditory events in the NICU and how the staff (mainly nurses) deal with them.
7. There is consensus on the most relevant sound sources in the NICU. The most cited categories are related to equipment, i.e., alarms, incubators, mechanical ventilation systems (Lasky and Williams, 2009; Liu, 2012; Restin et al., 2021), and speech (Lahav, 2015; Hernández-Salazar et al., 2020). Alarm levels can neither be set below a certain threshold, nor turned off, meaning that alarm-induced sound level issues can only be addressed through rules and regulations and with the collaboration of stakeholders involved in the manufacturing and supply chain. Furthermore, it is very difficult to avoid voice communication, especially in such a human-centered environment. The goal toward reducing sound levels must go in accordance with the care activities carried out in the unit.
8. Nurses are naturally considered as the main source for human-induced sound nuisance because of their constant presence and continuous activities within the NICU. Although nurses commit to keeping a quieter NICU environment for the wellbeing of neonates, a commonly seen issue is that they are unaware of how loud the sounds they produce can be and how susceptible their environment is (Darcy et al., 2008; Ahamed et al., 2018). The lack of (real-time) feedback in the NICU, such as a visual representation of the impact of human activity on the overall sound level, might de-prioritize individual sound awareness.
Theme 4: Beyond sound measurements includes assessments outside the domain of sound, as well as strategies aimed at reducing sound levels.
9. Subjective measurements are necessary to assess individual sound exposure. Literature generally presents two different approaches, depending on the targeted population. In the case of neonates, it is correlated to alterations in heart rate, blood pressure, and oxygen saturation (Williams et al., 2009; Smith et al., 2018). Conversely, questionnaires and interviews are the classical methods used to evaluate staff tolerance and awareness toward sound (Darcy et al., 2008; Trickey et al., 2012). However, studies focusing on the repercussions of the sound environment on parents are scarce, if not absent.
10. Alongside structural changes, behavioral change strategies and the implementation of educational programs are recurrent patterns in literature (Milette, 2010; Wang et al., 2014; Ahamed et al., 2018), although some authors already point out that they are not effective in the long term (Liu, 2010; Carvalhais et al., 2015). It is indeed uncertain to which extent behavioral strategies can be sustained long-term without periodic reinforcement. Therefore, it can be possible that the found effects are only temporary.
The results of the literature review reveal the already existing practice for sound recording and analysis in NICUs, albeit brief and for research purposes. However, there seems to be a need to understand the effect of NICU sound environment on nurses and patients (and on families, to date scarcely investigated) by psychological and physiological measurements and the need to use objective measurements for long-term behavior change through knowledge. This acknowledges that sound is an issue in NICUs worldwide and a threat to the wellbeing of its occupants. Moreover, NICU soundscapes are also found to be susceptible to human-environment interactions indicating that NICU occupants do contribute to sound levels. Yet, the nurses especially seek to understand individual sound sources and monitor the behavior of sound events over time to be able to take action to reduce sound pollution. For design purposes, these outcomes support the need for an intelligent system that enhances nurses' understanding of environmental sounds by continuously monitoring, analyzing, and explaining the acoustic environment in terms of sound sources and perceptual characteristics. In other words, we need to move beyond the often-sporadic measurement of noise levels with a physical descriptor that does not consider human perception and is difficult to make sense of for the non-expert. In the next Section, we closely look at 27 existing solutions to assess their capacity to measure, characterize and interpret (and possibly, forecast) the acoustic environment for long-term sound awareness and noise management in the NICU.
3. Beyond sound measurements: a review of technological solutions, gaps, and opportunities
To complement the literature review, we conducted a technology search to identify those products that are available on the market and could potentially serve as a solution to reducing sound pollution in the NICU. The search was initially based on sound monitoring solutions for hospitals. Given the extremely limited availability of such solutions (see Section Conclusions), our search criteria were extended. Our approach was then to start the search broad and include solutions that would cover multiple contexts (indoors and outdoors), then funnel the solutions into indoors, healthcare, and NICU, respectively. We included both commercial solutions that are marketed products and services for the assessment of the acoustic quality as well as academic response to the sound monitoring needs that would have produced concept solutions (i.e., demonstrators with prototypes). The latter is important as it showcases the trend for future applications and indicates where the technology might be best applied. Previous research from two of the co-authors on six case studies (Spagnol et al., 2022) categorized solutions by product complexity, customization options, active or passive feedback, and interpretative and predictive power. The latter two attributes define the threshold between solutions that only quantify the physical quality of sound events in terms of standard indices such as dB levels and solutions that use qualitative data to provide meaningful information that empowers the user to improve the acoustic environment. The study showed how some of the existing sound monitoring solutions can also generate some sort of reports that describe the acoustic environment at a deeper level (i.e., through the behavior of noise levels over time) but are unable to characterize perceptual properties and interpret it in terms of sound sources and even less so, to predict its dynamic nature by a well-defined model under different circumstances or over time. In this review, we increased the number of cases, and we deepened the analysis taking into account the entire design life cycle of a technological solution and its three main components: the type of data collected from the environment (Acquisition), how data are processed to gain insights into the phenomenon under study (Computation), and how information is displayed (Communication) for the final user to make sense of the sound phenomenon.
3.1. Methodology
The 27 solutions we considered in this review were mainly retrieved through a manual search on the most popular web search engines with the keywords “sound measurement tools,” “acoustic measurements systems,” “noise evaluation tools” “noise measurement tools.” We also combined all these searches with the keywords “healthcare,” “wellbeing,” “NICU,” “intensive care,” and “critical care.” To this search, we added solutions of which we had direct knowledge and solutions retrieved through the previous literature review. We excluded off-the-shelf sound level meters such as those manufactured and commercialized by Castle or Amprobe as the technological value of these tools relies fundamentally on the quality of the integrated microphones rather than in the design of the solution as we define it in this review (Acquisition, Computation, Communication). Additionally, professional tools such as these require the presence of a trained operator both to capture the sound levels and to interpret the results, of difficult interpretation for lay people. As mentioned, we are interested in solutions that can increase nurses' awareness of the contribution of sound to an unhealthy experience in the NICU and therefore in solutions that do not need the presence of audio experts. We also excluded both freemium and premium mobile applications marketed with the same purpose. These are simplified versions of hardware sound meters that use lower-level audio equipment (i.e., the smartphone's own microphones). As such, they provide non-expert users with less reliable basic information such as an average SPL at a given time.
Because the focus of the review is on technological solutions for measuring the quality of acoustic environments, we first listed current methods that evaluate environmental sounds. These methods include (i) acoustic and psychoacoustic indices for the quantitative measurement of sound, (ii) indicators of perceived affective qualities of soundscapes (Aletta et al., 2016), and (iii) applications of machine learning (ML) to categorize sound events and forecast their behavior in a given context. For each of the 27 solutions we analyzed available public documentation (websites, scientific publications, demos, and videos) to list specific indices, indicators, and ML techniques used by manufacturers. Through an iterative process, we grouped methods by the function they fulfill. Figure 2 summarizes the assessment methods identified across the 27 solutions and the four Functions they define: Measure, Characterize, Interpret, and Forecast. As shown in Figure 2, each Function builds upon the other and integrates the previous evaluation method on a scale of complexity that goes from standard acoustic measurements to the characterization of soundscape in terms of its perceptually relevant dimensions (e.g., pleasantness, eventfulness, annoyance, monotony, homogeneity), then to the classification of sound events to be able to interpret the acoustic environment in terms of the footprint of sound events, and finally, the use of Artificial Intelligence (AI) to model soundscapes and forecast the dynamic behavior of acoustic environments. This distinction of functions will allow us to distribute the existing technological solutions (i.e., cases) in an organized way.
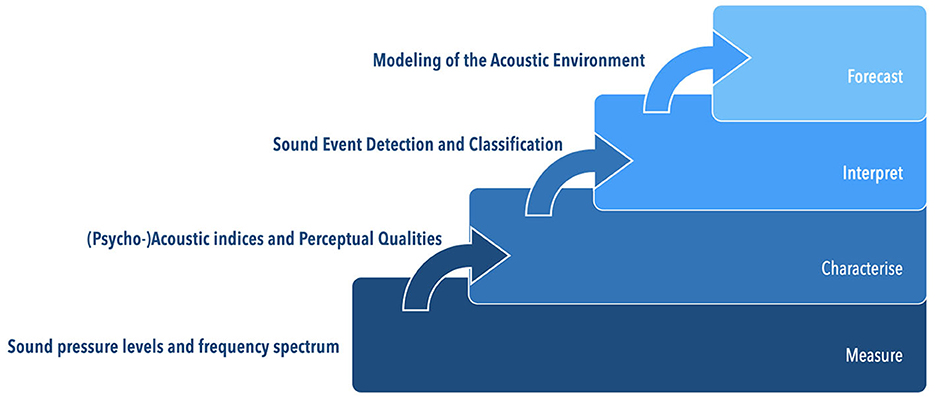
Figure 2. The four functions of technological solutions for sound quality evaluation, defined by the sound evaluation methods used. Each function builds and integrates the previous one.
The correlation of the four Functions with the three data-driven design components (Acquisition, Computation, and Communication) defines a matrix (see Table 2) that guides the analysis of the cases. Based on the matrix, we describe each component for each selected case in an iterative process to identify common features around which the cases were grouped.
Solutions that allow users to Measure the acoustic environment only extract physical features from sound (i.e., SPL) in real time. These measurements are typically used by private and public enterprises to comply with existing regulations on noise levels (such as the European Parliament., 2000/14/EC) both outdoors (e.g., in public urban spaces) and indoors (e.g., in offices, school, hospitals). Particularly interesting to this analysis are those solutions marketed for healthcare environments and specifically for NICU and/or ICU (McLennan Sound Monitoring, Sound Intelligence). In these solutions, data is collected manually by trained users with specialized equipment or automatically by sensors permanently installed at the customer's premises (Noisemote, Noisescout). Data are displayed in real-time (as in hand-held acoustic cameras like Sorama, which produces and displays real-time heatmaps of the indoor acoustic environment, see Figure 3) and sent to a centralized repository where analytics and reports are generated and regularly sent to customers (gfai tech, Norsonic and Sorama). Among the most common methods to represent and communicate findings to the end users are heatmaps (in the form of so-called noise maps), spectrograms and other basic diagrams representing the evolution of the physical descriptors of sound (e.g., frequency and amplitude) over time (see Figure 3 for an example of how data are displayed for the user). All the solutions in the Measure category are commercialized and have a high Technology Readiness Level (TRL) e.g., between 7 and 9.
Solutions that not only measure the physical characteristics of sound but aim to Characterize the soundscape as perceived by humans in context (ISO 12913-1., 2014) tend to offer both real-time measurements and historical data of dB levels for monitoring and trend analysis. Sometimes acoustic data are coupled with other information such as air quality or temperature (Quietyme, SonicU) or are scored to represent the acceptable ranges (e.g., sleep score by Quietyme). These products are commercialized to couple compliance with regulations with data intelligence that can support decision-making on noise mitigation policies both in private and public enterprises, indoors and outdoors (e.g., hospitals, construction sites). Other solutions that belong to this group try to engage users in the data acquisition process to increase awareness on the impact of noise. Typically, a mobile app is provided to collect data such as audio recordings and surveys on qualitative and perceptual characteristics of sound events (e.g., peaceful, uneventful, chaotic, pleasant) (MosART, Harmonica Project). Data is later uploaded to a central repository for analysis and, in some cases, public communication (for instance, via crowdsourced soundscape maps on the web as in HushCity, Radicchi et al., 2021). Solutions in this category are for the vast majority available on the market both for purchase and as a free download (in the case of mobile applications), with an average TRL of 7. A small percentage is still in an R&D phase (TRL 3 or 4). These solutions mainly refer to academic or publicly funded research to mitigate the impact of sound pollution in contexts such as public health (Mietlicki et al., 2014; Misdariis et al., 2019). As we discuss in Section Conclusions, we believe that the inclusion of solutions that are currently only modeled and applied to the outdoor context can provide relevant information to support the design of novel technological solutions for the assessment of the quality of the indoor soundscapes such as the NICU.
We define solutions that can Interpret the acoustic environment as products or systems that leverage big data collected through networked sensors over a longer period. These solutions use AI methods such as ML or the so-called Machine Listening (i.e., the processing of sounds through a computer in a way that mimics human auditory cognition) in combination with signal processing techniques to interpret the sound environment in terms of detection of sound events, and classification of sound sources so that the “footprint” (i.e., the impact on the quality of the acoustic environment) can be assessed. As we will further detail in the following Section, these solutions are characterized by a lower TRL (between 2 and 5). Some solutions, notably those that address the specificity of the NICU context, are currently concept solutions (Özcan et al., 2022c; Spagnol et al., 2023). These solutions pay special attention to how information is displayed to the user (see Figure 3) to support sense-making of data for long-term behavior change, and their proposed interface has been designed considering the nurses' needs and expectations as collected through qualitative research (interviews, observations). Lastly, some solutions focus on detecting and, in some cases predicting the behavior of unwanted sound events in outdoor environments as part of academic endeavors, sometimes in collaboration with industrial partners (Salamon et al., 2016; Sevillano et al., 2016; Misdariis et al., 2019). No commercially available solutions fit in this category. Authors recognize that current measurements are still “insufficiently understood by the general public and authorities” and use sound descriptors that are “complicated to explain and relatively far-removed” from human perception (Mietlicki et al., 2014). These solutions are interesting to the present study as they focus on the algorithmic modeling of an acoustic environment to inform the end-user on the auditory footprint of different sound sources, with the goal of supporting better decision-making to mitigate the negative effects of sound pollution. In Section Conclusions, we comment on how similar approaches could be applied to the NICU context in order to increase nurses' awareness with actionable knowledge on the role of different sound sources.
Solutions that can Forecast the acoustic environments in the longer term can be understood as like weather forecasts, i.e., they should provide information that we can easily consult to make daily and long-term decisions that are informed by patterns found in big and historical data used for generalization and prediction (i.e., modeling). When applied to the assessment of acoustic environments, this area of investigation is still in its infancy and despite a clear increase in the research effort (Bianco et al., 2019) no viable solutions are yet available on a large scale, let alone commercial applications. In the context of outdoor soundscapes, experimental solutions apply Deep Learning and other ML techniques on multiple data streams at the same time (e.g., geospatial data, sound recordings, traffic data, weather data related to seasonal conditions, etc.) to ultimately augment the human listening capabilities in terms of sound events recognition, classification and prediction, and perceptual interpretation of the acoustic environment (eventfulness and pleasantness) (Mitchell et al., 2021). In the authors' intention (Sharan and Moir, 2016; Pedersen et al., 2018), this augmented knowledge will allow for better management and planning to take action, both at the institutional and individual level. In the NICU context, a solution that can predict the quality of the soundscape while also assessing the impact of different sound categories (e.g., speech by nursing staff, machinery, medical equipment) could support behavioral change among nurses but also, in the long-term, guide the management in better planning for the NICU activity and inform design decisions for device manufacturers and architects.
Once defined the matrix to classify the solutions, we proceeded to analyze the selected cases in terms of Function (Measure, Characterize, Interpret and Forecast), Scope (Outdoor/Indoor) and application to the Healthcare and NICU context. In the following section, we present our findings for each category and discuss specific representative cases.
3.2. Analysis and discussion of results
Figure 4 offers an overview of the selected solutions by Function. Of the 27 cases analyzed, 12 are used to measure the acoustic environment, eight aim to characterize it, seven provide for a more sophisticated interpretation, and one only case represents the current research effort in applying various degrees of ML techniques to augment human listening capabilities to look into the future of the acoustic environment. The reader can find the complete list of cases with the metadata used in this review study and references to external resources as Supplementary material.
In the Measure group, Noise Aware represents a baseline solution that provides customers with a real-time alarm whenever it detects a breach of the established noise threshold. It comes with a noise level detector installed on mostly real-estate properties for one's “peace of mind” and “protection of profits” (Noise Aware website., 2022). NIOSH app is a representative of a broad category of hardware and software devices for the real-time measurement and display of noise levels in decibels (dB). We consider it a special case as it is released by a public authority, the United States National Institute for Occupational Safety and Health “to help workers make informed decisions about their noise environment and promote better hearing health and prevention efforts” (NIOSH website., 2022). Like NIOSH, the Noise Capture app stands out as a crowdsourced project that encourages citizens to capture the dB level in the urban space with their mobile phones and upload the measurements to a shared web map, in the context of the European Union effort on sensitizing the population on the negative effects of urban noise on health. The solutions of Noisemote, Noisescout and McLennan Sound Monitoring leverage permanently installed networks of noise sensors, mainly in an outdoor context, to continuously measure sound levels. Next to real-time alerts, they also provide access to a dashboard for continuous monitoring of dB average and peak levels along the network. Finally, solutions provided by Sorama, Brüel and Kjaer and CAE systems are at the forefront of this market as they leverage holographic technology (an increasingly popular technique to estimate sound wave propagation for better source identification and localization) to provide a more accurate estimation of sound events' SPL and source localization through handheld and hand-moved acoustic cameras.
Solutions that Characterize the acoustic environment, move beyond noise measurement to inform users on the psychoacoustical and perceptually relevant dimensions of sounds. Off-the-shelf tools such as Quietyme, SonicU, Pulsar Safe Ear, and Sound Ear are particularly relevant since, as we will discuss below, they target the healthcare sector and have been applied to the context of NICU. Both Quietyme and SonicU provide integrated solutions that rely on permanent installations of networked sensors and store data for analysis, forensic investigations of incidents and correlation with other information sources over time. Reports with historical data on average noise levels and peak noise events over a certain time are regularly sent to customers to support a deeper awareness of the acoustic environment and decision-making processes. Both products integrate different data sources, such as temperature and air quality. In an effort to get closer to the human perception of sound and characterize noise level in terms of the effects it has on people, Pulsar Safe Ear and Sound Ear provide their customers with an artifact: a physical display that is permanently installed on the premises. In the case of Sound Ear, the display is ear-shaped to attract the attention of users, both listeners and producers of sound events, on the perceptual effects of noise levels. The display uses a simple visual cue to communicate information in real-time: similarly, to a traffic light, the color changes from green (“good” noise level) to red (“critical,” “harmful” noise level). The exact dB measurement, represented by a number, is also displayed.
Cases such as MosART, Hush City and the Harmonica and Mouvie projects deserve special attention as their aim is to provide users with meaningful information on the acoustic environment as mediated by human perception. MosART (Mobile Soundscape Appraisal and Recording Technology) is a smartphone application prototyped in the context of the MosART+ intervention (Kosters et al., 2022), a research and commercial endeavor that aims “to increase auditory awareness in healthcare professionals, to research the experience of music festivals by residents and visitors, and to study possible effects on sound annoyance of different constructions techniques” (Soundappraisal website., 2022). The MosART app prompts users to sample their acoustic environment collecting short recordings then labeled according to perceptual qualitative characteristics such as calm, boring, chaotic, and lively. It is also possible to label sound sources that the user identifies while recording. It is particularly relevant to this review that the main goal of the project is to increase awareness of nursing staff on the impact of the acoustic environment on healthcare professionals and, conversely, the impact of our own actions as sound producers. The increased awareness acts as a facilitator to support more informed institutional decision-making and individual action-taking to contrast the negative impact of noise in the healthcare space (Kosters et al., 2022). Hush City (Radicchi, 2021) also proposes a smartphone app that citizens can use to record, geotag, and share on an open web map quiet places in their cities. It is a solution framed within the citizen science movement that wants to empower people in collectively assessing the quietness of the urban soundscape “with the potential of orientating plans and policies for healthier living” (Hushcity website., 2022). While it shares some characteristics with the Noise Capture app described above (it provides the user with real-time dB measurements), it also prompts users to make a subjective judgement on the perceived quietness of the place thus interpreting a physical dimension such as SPL through the lenses of human listening in context.
The Mouvie (Mobility and Quality of Life in Urban Areas) project ran from 2014 to 2019 to develop new metrics for the assessment of urban noise generated from vehicles traffic (Misdariis et al., 2019). Researchers explicitly aimed to move from a “normative” approach to the evaluation of the urban acoustic environment, based on “objective measurements, sound level thresholds and operational solutions” to a “sensitive” approach based on subjective metrics that consider the psychoacoustical, cognitive, and social dimensions of sound events (Misdariis et al., 2019, p. 2). In an experimental validation, several psychoacoustic metrics along with automatic sound event classification are used in combination with listeners annotations to characterize the urban acoustic space (notably, traffic sounds) in terms of its annoyance level in relation to potential for action. The combined metric forms the basis for the definition of a computational method to automatically evaluate the annoyance level of traffic noise and its impact on human activity in urban space. Finally, the Harmonica Index developed in the context of the Harmonica Project (Mietlicki et al., 2014) defines a novel approach to noise measurements that interprets and displays the physical features of sound (such as average and peak SPL) in a way that is closer to what people perceive. The Index combines two existing metrics, the background noise level, and the peak noise level (that refers to salient sound events such as aircrafts, rail traffic, trucks passing by). The quantitative information extracted from these two metrics is presented to users on a simple 0 to 10 scale, thus favoring the interpretation by non-experts both at citizen and institutional level. This representation, explicitly inspired by current consumer solutions for air quality monitoring, provides listeners with information on the “real feeling” of sound (analogous to the real feeling of temperature provided by weather forecasts), rather than analytical, accurate information on SPL. Thanks to an engaging data visualization display, the Harmonica Index wants to increase awareness on the role of different sound sources and their impact at different times and in different locations in the urban context.
In all solutions, Characterization seems to have been achieved by making the perceptual quality of the sounds explicit for the non-expert user by combining quantitative data on sound pressure levels with information on how it affects listeners i.e., how annoying, pleasant, quiet, harmful, or peaceful the soundscape can be. Thus, an overall quality assessment is the result of these sound evaluation methods.
Solutions that aim to Interpret the acoustic environment replicating the cognitive process of the human ear can be clustered around two main areas. DYNAMAP (Sevillano et al., 2016), Noise Modeling (Le Bescond et al., 2021), BirdVox (Salamon et al., 2016), and SONYC (Dove et al., 2022) rely on low-cost sensor networks already deployed in cities to collect sound data later used to train ML algorithms for sound events classification, with the ultimate goal of designing reliable models of the urban and natural soundscapes. Except for BirdVox, which is targeted to the identification of bird calls for scientific and ecological purposes, all other solutions address, once again, the issue of urban noise and the harmful impact it can have on public health by providing advanced real-time monitoring systems and noise mapping. All solutions are academic research projects at various degrees of validation, in general characterized by a lower TRL (between 4 and 5). They are all designed for the outdoor acoustic environment, which highlights a lack of research in sound events detection and source classification in indoor spaces. DYNAMAP's goal is to develop low-cost solutions for the real-time update of noise maps. Its algorithm builds a “sound layer” on general purpose Geographical Information System (GIS) platforms (used to manage geographical data for research, land management, and urban planning). According to the authors, this strategy reduces the need of public institutions to rely on expensive dedicated acoustic software and hardware (Sevillano et al., 2016) to produce mandatory municipal noise maps, while at the same time it provides a more accurate description of the impact of urban noise (mainly, traffic sounds). Similarly, SONYC (Sounds of New York City) leverages existing low-cost sensors within the city of New York to understand the urban soundscape in terms of noise pollution. Machine Listening algorithms were developed (Salamon and Bello, 2017) to predict noise levels and identify patterns and outliers in the propagation of urban noise from specific sound sources. As an additional layer, SONYC also promotes citizen participation for the collection of data. Samples of urban noise recordings can be collected by individuals and added to the database. BirdVox applies ML and automatic sound recognition to the natural soundscape with the goal of cataloging free-flying birds calls. The algorithm will be deployed in natural environments in conjunction with audio sensor networks for the monitoring and interpretation of birds' migrations paths to support and promote actions for the protection of avian species. Two prototypal solutions, Doplor Sleep and SOUNDscapes are of relevance to this study as they are designed for healthcare and, in one case, NICU. Doplor Sleep addresses the issue of sound-induced sleep disturbance in hospitals. Through a smartphone app, the system captures sound events to visualize, through a friendly and attractive interface that targets non-expert users, critical information such as sound levels but also classification of sound sources (alarm, speech, incidental sounds, or snore) for increased awareness on sleep disturbances (Özcan et al., 2022c). Doplor Sleep also has a nurse interface and displays the analysis of the sound events occurring at night. Both the patient and nurse solutions also use characterization method for displaying the acoustical quality of the nighttime sound environment. SOUNDscapes is a digital platform that detects, localizes and classifies sound events occurring at the NICU. Data is then displayed to inform nurses on real-time sound levels, trends (e.g., during day or nighttime), type of sound sources and their localization (Spagnol et al., 2023), to provide healthcare professionals and, over time, hospital management, with specific knowledge to address the issue of noise in NICU. Like Doplor Sleep, SOUNDscapes uses a metaphorical and visual description to provide the listener with an overall evaluation of the quality of the acoustic environment.
The Interpretation of the acoustic environment is achieved by combining data and information from all previous stages. The Measurement of quantitative metrics i.e., sound levels, and the Characterization of perceptual characteristics such as annoyance and pleasure are combined with information on the footprint (i.e., the impact on the overall soundscape's quality) that different sound events have. The goal of these solutions is to present the user with a comprehensive understanding of the acoustic environment so that they can take action to improve it, both by individually changing their behavior (e.g., producing less “noise”) and working toward better management of the space and work activity. Thus, users have access to measurement data, its perceptual characterization, and the footprint of the different sound categories.
We see Forecast solutions as guided by the ambition of providing human users with augmented capabilities to make-sense and predict the behavior of acoustic environments at a previously unimaginable scale. In this sense, these solutions will be “empowered hearing systems” that, while they face “the same challenge that biological hearing systems have evolved to solve-to make sense of sound and thereby infer the state of the world” (Sharan and Moir, 2016), aspire to overcome the limits of human listening and cognition. Ultimately, these solutions could be used to “answer specific biological, ecological, and management” (Bianco et al., 2019) questions. In the NICU context, these questions might include: How does sound influence the sleeping pattern of premature babies? How do decisions on personnel shifts (e.g., when nurses change shift, when food is delivered, when cleaning service is administered) impact the sound quality of a NICU unit? How does the design of the rooms impact the perception of different sound sources? The capability to answer these questions would provide both individuals and institutions with usable knowledge to take action toward a healthier sound environment in critical care, both in the short and in the long term.
Along the course of this study, we identified several research efforts that move toward this direction exploring applications of ML techniques (for a recent review see Bianco et al., 2019) and the development of new acoustic, psychoacoustic and bioacoustics computational models (Brocolini et al., 2012; Sueur et al., 2014; Alsina-Pagès et al., 2021). Although none of these studies focus on indoor environments, we include them in this review as they constitute a possible scenario for a solution that can forecast the behavior of the NICU environment, as described above. In particular, here we consider a case that-albeit sharing some of the characteristics of embryonic research and low TRL (3)-has been empirically validated as an integrated solution, rather than an isolated set of novel indexes. The study by Pedersen et al. (2018, 2021) leverages ML to predict ambient sound levels across the United States. Sound samples from more than 600 locations were correlated with GIS measurements from more than 100 sites in the U.S. to train a model to predict the impact on the acoustic environment of changes in land management, such as the introduction or the removal of an airport or a high-traffic road. This project is of particular interest to this review as the authors acknowledge the importance of accurate soundscape modeling for “public health studies and urban development,” potential “commercial applications for real estate and urban development” and “implications for social justice” (Pedersen et al., 2018, p. 2). In the context of NICU soundscape, a similar algorithm that can forecast the impact of different auditory footprints and display it for nurses would support both short-term increased awareness and longer-term improved decision-making toward a healthier acoustic environment in critical care.
As part of the next step in our analysis, we categorized the solutions as a function of their Technology Readiness Levels (TRLs). The definition of the TRL is based on the official description adopted by the European Union and can be found here https://ec.europa.eu/research/participants/data/ref/h2020/wp/2014_2015/annexes/h2020-wp1415-annex-g-trl_en.pdf. The TRL analysis by Function (Figure 5) shows that solutions in the Measure and Describe function are characterized by a higher TRL (between seven and nine). As we move toward Interpret and Forecast, the TRL level decreases. Specifically, the TRL level of solutions that Interpret the acoustic environment starts at two, with a maximum of five points, while the TRL level of the solution representative of the Forecast function is three.
As shown in Figure 6, of the 27 cases considered in this review 12 are solutions for the evaluation of the outdoor soundscape while only eight are presented as indoor solutions. Eight are described as applicable to both outdoor and indoor contexts. This might be due to several factors including critical technical aspects (e.g., the relative lack of variety of sound sources and the higher complexity of the acoustic space) that are made more complex by the difficulty to access and collect the needed data in indoor spaces due to privacy concerns. For instance, in the context of NICU, the limitations imposed by privacy rules in an extremely sensitive environment make the development of algorithms that can identify and categories different sound sources difficult to achieve. Additionally, social aspects such as the citizens' perception and public discourse around noise facilitate the advancement of research in the context of urban environment while leaving the indoor soundscape relatively under-investigated.
Our findings highlight a clear gap in the research, development, and commercialization of solutions that address the needs of indoor environments. However, functional socio-technological environments such as control rooms, workspaces, healthcare environments, where sound is conducive to social, environmental, and instrumental interactions (Özcan et al., 2022a) greatly impact the everyday life of people and therefore, innovation in this area is urgently needed. Critical care environments, and especially the NICU, represent a unique case of a particularly self-contained functional environment with peculiar design characteristics that greatly differentiate it from other indoor environments, notably the strict control of interchanges with the external world. In fact, only a handful of solutions among those we consider in this study are applied to healthcare environments (Figure 7). The majority belongs to the Measure and Characterize functions and are marketed by manufacturers as off-the-shelf solutions to monitor noise levels. Some of them (SonicU, Quietyme) offer integrated environmental monitoring of critical environments that couple the measurement of sound levels with temperature and air quality. Others, like McLennan Sound Monitoring and SoundIntel provide healthcare personnel with real-time alerts of unusual sound events that might represent a threat to patients' safety. However, the definition of what constitutes a “threat” is based solely on the detection of sound levels (e.g., the sudden peak in environmental sound level caused by a body falling) that does not provide any insight into the cause of the event. Among the solutions considered in this study, only MosART+ (described above, Kosters et al., 2022) engages nursing staff in the qualitative categorization of sound events in the hospital environment by means of a dedicated mobile application. While this system collects data on the perceived qualities of different sound sources, it does not automatically inform users on the footprint of hospital sounds so that they can take action to improve it.
Of the five solutions that explicitly target NICU (sometimes along with other critical and intensive care environments such as PICU and ICU), the only interpretative solution (in green in Figure 6), SOUNDscapes, is currently in the state of concept design (i.e., it has not been prototyped yet). Only three solutions-Sound Ear, Pulsar Safe Ear, and SonicU-are commercialized to be applied in NICU. This finding highlights the smaller market represented by NICU as compared to healthcare in general (NICU solutions are < 50% of all solutions for healthcare). NICUs, as reminded on various occasions in this article, are extremely delicate acoustic environments where patients are particularly vulnerable to sounds and where extra care is required when it comes to the introduction of new technology and the collection of soundscape data. At the same time, patients of NICU are unique in that they are exposed to the negative effect of unwanted sounds, but they rarely have a role in producing sounds that can negatively impact the acoustic environment (as premature babies are in general extremely quiet and cannot express verbally). For the very same reasons, we see NICU as an opportunity for the design of novel solutions that want to move beyond a descriptive approach to support a more informed sense-making of the sound quality. These novel solutions should, as Doplor Sleep and SOUNDscapes, assess the impact that sound events have on the quality of the NICU soundscape, and provide nurses with actionable information so that they can actively contribute to increase it. Solutions that prove to be efficient in the NICU could be scalable to other critical care contexts such as PICU and adult ICU.
4. Conclusions
Our semi-systematic review of more than 70 scientific publications on the acoustic environment of the NICU shows that there is an urgent need for solutions that can provide nurses with comprehensive, holistic information on how different categories of sounds (e.g., speech, medical alarms, machinery) impact the quality of the soundscape. This information should include quantitative measurements of sound levels but also increase awareness of how qualitative characteristics of sound-which are subjective and highly context-dependent (Axelsson, 2015)-are perceived (e.g., annoying, pleasant, chaotic, calm).
The review of technological solutions highlights that existing products marketed for critical care tend to monitor the acoustic environment by measuring the overall sound level episodically and in real time. While this measurement strategy complies with privacy regulations that limit the collection and identification of personal data (such as data contained in speech), it greatly limits the possibilities for nurses (i.e., the guardians of NICU patients and their wellbeing) of understanding how different sound sources impact the perceived quality of critical care soundscape and consequently, the possibility to take immediate action to improve it. Current research that leverages low-cost and networked acoustic sensors to collect long-term data and algorithmically model the soundscape characteristics only focuses on outdoor spaces, where such networks exist as part of a public effort in mitigating noise pollution. Additionally, existing protocols to assess the affective quality of soundscapes were designed for the outdoor context and their applicability for indoors is still debated (Torresin et al., 2020). Consequently, we see a clear opportunity for the design and development of new solutions that focus on the collection of rich soundscape data in the NICU environment to allow for quantitative (i.e., based on physical properties of sound), qualitative (i.e., based on the perceived characteristics), and categorical (i.e., based on the sources of different sound events) analysis. Such rich data should be used to extract the appropriate soundscape descriptors (Aletta et al., 2016) to model the NICU soundscape and develop novel algorithms to characterize, interpret, and predict its behavior over time in a holistic perspective. Particular attention should be given to the design of how nurses interact with the soundscape data, i.e., how data are translated into usable information that becomes actionable knowledge (Masud et al., 2010). A technological solution that integrates these algorithms with a user-centered display of information on the auditory footprint and quality of sound events in NICU, would provide nurses with actionable information to actively improve the soundscape. However, critical ethical and privacy concerns are faced to collect the appropriate soundscape data from the indoor environment, especially in the context of critical care. These concerns are recognized by providers of commercial solutions for audio data collection for AI (Javahid, 2023) as well as by researchers (Nautsch et al., 2019). The exploration of automatic data cancellation (for instance regarding speech) and technological solutions such as on edge data collection where acquisition and processing happen on the device is necessary although beyond the scope of this article.
4.1. Design implications
We commenced this review study not only to assess the state-of-the-art of the measurement of the acoustic quality of NICU, but also to understand what characteristics future solutions should present. Awareness that current acoustic measurement systems are neither facilitating radical change in the quality of the auditory experience in NICU, nor preventing health and cognitive risks connected with the exposure to dangerous noise levels is widespread. Therefore, with our accumulated knowledge and insights, we reflected on the critical elements of a system that aims to reduce the negative impact of sound in NICU in the long-term while raising awareness to the root causes and magnitude of the impact generated. Ultimately, a combination of longer-term data collection, the development of new soundscape indices and ML techniques for the quantitative and qualitative evaluation of the sound environment, and a human-centered design of informative displays seems a promising combination of design factors for solutions that can help critical care professionals to drastically improve the quality of the NICU acoustic environment.
Figure 8 summarizes our findings and consequently highlights a vision for a future solution that integrates several main functions and provides a holistic analysis of the NICU soundscape with the aim to better inform nurses with respect to its perceived qualities and the footprint of specific sound categories. The Figure highlights the past and established efforts with sound measurement methods but also a future vision for a system that can model soundscapes and forecast the perceptual impact of sound events. However, the intermediate level of solutions (captured in blue background in the Figure) provides the sweet spot for state-of-the-art data-centric design for soundscape improvement as the current knowledge and technologies would be suitable to characterize the perceptual qualities of the sound and interpret the acoustic environment in terms of its sound sources. Yet, the solutions we have found that fit the NICU and would incorporate these functions only represent conceptual designs with low TRLs. The NICU context urgently needs an industrial and scientific effort to support their sonic needs.
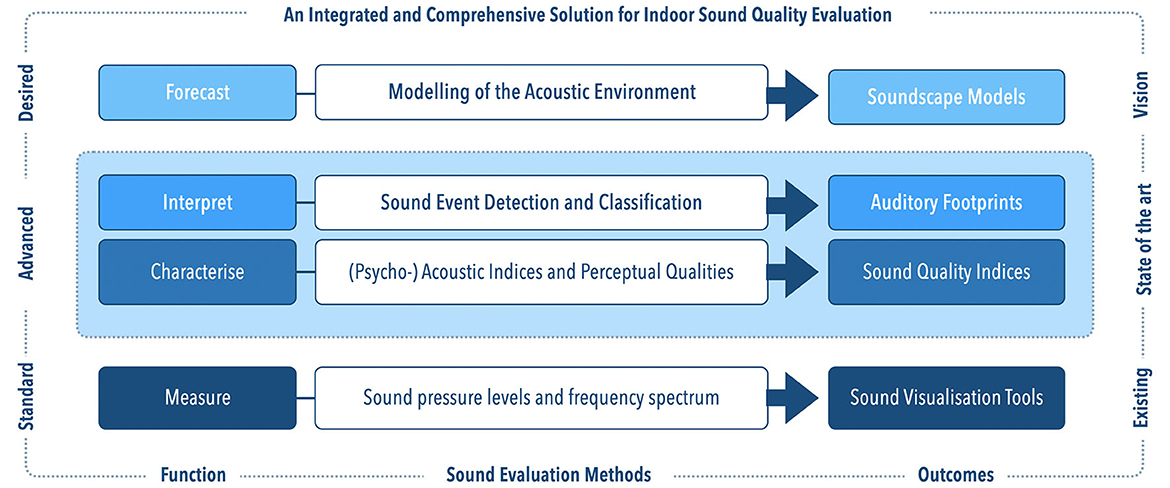
Figure 8. The four functions of technological solutions for sound quality evaluation and the outcome they generate for the user.
A sound quality assessment solution for NICU should answer the following questions:
• What is the relationship between the physical and the perceived properties of sound, and the footprint of different sound categories in the NICU soundscape?
• What are the appropriate indicators to algorithmically model the relationship between the physical (quantitative) and the perceived (qualitative) footprint of different sound categories of the NICU soundscape?
• How does a technological solution for nurses holistically represent the quantitative, qualitative and categorical properties of the NICU soundscape in a context-relevant, human-centered way?
Ideally, a sound quality evaluation system fit for NICUs could have the following functions. First, a general scan of the acoustic environment could result in traditional and well accepted measurement and display of sound levels. This stage is also essential to collect the sound input properly and carefully to be used by more advanced functionalities (Characterize, Interpret, and Forecast). Purposely developed sound quality indices would further complement the sound measurements and build toward a holistic evaluation of the NICU soundscape for nurses to make quick judgements and take immediate action to improve it. Such an evaluation would imply the development of new soundscape descriptors and indicators based on affective qualities of sound (e.g., pleasantness, annoyance, eventfulness) and modeled on the indoor NICU environment. The automatic assessment of sound quality would represent a great advancement in the study of indoor soundscapes and could help listeners further train their listening skills and be aware of the perceptual impact of sound. The Interpret function will provide nurses with insights into the root causes of the sound quality by making sound sources (e.g., speech, alarms, support devices) and their footprint over time explainable. Finally, the Forecast function will provide a holistic overview of the NICU soundscape based on big, trained data for any given time or situation and will allow for predictions and early diagnosis for possible threats to the quality of the acoustic environment. Not only nurses but most likely unit managers and hospital technology scouts will make use of this function to make well-informed choices for structural change (e.g., purchasing decisions, workflow analysis that can cause unwanted noise). Accordingly, all these functions will augment the listener's perceptual, cognitive, and affective skills enhancing their sense making of the NICU soundscape.
The future holistic solution envisaged in Figure 8 will build on a quantitative, qualitative, and categorical approach to sound analysis with the goal to computationally model “the relationship between the physical and the perceived properties of the acoustic environment” (Aletta et al., 2016, p. 68) in the context of the NICU indoor soundscape. The quantitative analysis would be based on audio signal processing and psychoacoustics and inform the conventional metrics which represent the prior art sound measurements. The qualitative analysis would be based on perceived affective quality and inform the modeling of the descriptive qualities of the NICU soundscape. The categorical analysis would be based on the classification of sound sources and their context relevance and inform the computational modeling of classification of sound events and their appropriateness. The combination of these three approaches would provide nurses with a comprehensive understanding of the NICU soundscape thus facilitating its active improvement both in the short term–through behavior changes, and long term–by supporting informed decisions on the organization of NICU activities and design of its infrastructure.
Author contributions
SL conceived the review study of technological solutions and wrote the Section 3. SS and EÖ conceived the semi-systematic literature review and wrote the Section 2. SL, SS, and EÖ equally contributed to Sections 1 and 4, Figures, and Tables. All authors approved the submitted version.
Funding
This study was conducted with fundings from Delft University of Technology, Faculty of Industrial Design Engineering, Department of Human-Centered Design and Erasmus Medical Center Rotterdam.
Conflict of interest
The authors declare that the research was conducted in the absence of any commercial or financial relationships that could be construed as a potential conflict of interest.
Publisher's note
All claims expressed in this article are solely those of the authors and do not necessarily represent those of their affiliated organizations, or those of the publisher, the editors and the reviewers. Any product that may be evaluated in this article, or claim that may be made by its manufacturer, is not guaranteed or endorsed by the publisher.
Supplementary material
The Supplementary Material for this article can be found online at: https://www.frontiersin.org/articles/10.3389/fcomp.2023.1156693/full#supplementary-material
Supplementary Material 1. List of the technological solutions analyzed, with metadata.
References
Ahamed, M. F., Campbell, D., Horan, S., and Rosen, O. (2018). Noise reduction in the neonatal intensive care unit: a quality improvement initiative. Am. J. Med. Qual. 33, 177–184. doi: 10.1177/1062860617711563
Aita, M., Robins, S., Charbonneau, L., Doray-Demers, P., and Feeley, N. (2021). Comparing light and noise levels before and after a NICU change of design. J. Perinatol. 41, 2235–2243. doi: 10.1038/s41372-021-01007-8
Aletta, F., Kang, J., and Axelsson, Ö. (2016). Soundscape descriptors and a conceptual framework for developing predictive soundscape models. Landsc. Urban Plan. 149, 65–74. doi: 10.1016/j.landurbplan.2016.02.001
Alsina-Pagès, R. M., Benocci, R., Brambilla, G., and Zambon, G. (2021). Methods for noise event detection and assessment of the sonic environment by the harmonica index. Appl. Sci. 11:8031. doi: 10.3390/app11178031
Axelsson, Ö. (2015). “How to measure soundscape quality,” in Proceedings of the Euronoise 2015 conference, 1477–1481.
Axelsson, Ö., Nilsson, M. E., and Berglund, B. (2010). A principal components model of soundscape perception. J. Acoust. Soc. Am. 128, 2836–2846. doi: 10.1121/1.3493436
Bianco, M. J., Gerstoft, P., Traer, J., Ozanich, E., Roch, M. A., Gannot, S., et al. (2019). Machine learning in acoustics: theory and applications. J. Acoust. Soc. Am. 146, 3590–3628. doi: 10.1121/1.5133944
Blackburn, S. (1998). Environmental impact of the NICU on developmental outcomes. J. Pediatr. Nurs. 13, 279–289. doi: 10.1016/S0882-5963(98)80013-4
Bliefnick, J. M., Ryherd, E. E., and Jackson, R. (2019). Evaluating hospital soundscapes to improve patient experience. J. Acoust. Soc. Am., 145, 1117. doi: 10.1121/1.5090493
Brandon, D. H., Ryan, D. J., and Barnes, A. H. (2007). Effect of environmental changes on noise in the NICU. Neonatal Netw. 26, 213–218. doi: 10.1891/0730-0832.26.4.213
Braun, V., and Clarke, V. (2012). “Thematic analysis,” in APA Handbook of Research Methods in Psychology, Vol. 2. Research Designs: Quantitative, Qualitative, Neuropsychological, and Biological, eds H. Cooper, P. M. Camic, D. L. Long, A. T. Panter, D. Rindskopf, and K. J. Sher (American Psychological Association), 57–71.
Brocolini, L., Lavandier, C., Marquis-Favre, C., Quoy, M., and Lavandier, M. (2012). Prediction and Explanation of Sound Quality Indicators by Multiple Linear Regressions and Artificial Neural Networks. Nantes: Acoustics, 2121–2126.
Busch-Vishniac, I., and Ryherd, E. (2019). Hospital soundscapes: characterization, impacts, and interventions. Acoust. Today, 15, 11–18. doi: 10.1121/AT.2019.15.3.11
Busch-Vishniac, I. J., West, J. E., Barnhill, C., Hunter, T., Orellana, D., and Chivukula, R. (2005). Noise levels in Johns Hopkins Hospital. J. Acoust. Soc. Am., 118, 3629–3645. doi: 10.1121/1.2118327
Calikusu Incekar, M., and Balci, S. (2017). The effect of training on noise reduction in neonatal intensive care units. J. Spec. Pediatr. Nurs. 22:e12181. doi: 10.1111/jspn.12181
Carvalhais, C., Santos, J., da Silva, M. V., and Xavier, A. (2015). Is there sufficient training of health care staff on noise reduction in neonatal intensive care units? a pilot study from Neonoise project. J. Toxicol. Environ. Health, 78, 897–903. doi: 10.1080/15287394.2015.1051204
Casey, L., Fucile, S., Flavin, M., and Dow, K. (2020). A two-pronged approach to reduce noise levels in the neonatal intensive care unit. Early Hum. Dev. 146, 105073. doi: 10.1016/j.earlhumdev.2020.105073
Chen, H. L., Chen, C. H., Wu, C. C., Huang, H. J., Wang, T. M., and Hsu, C. C. (2009). The influence of neonatal intensive care unit design on sound level. Pediatr. Neonatol., 50, 270–274. doi: 10.1016/S.1875-9572(09)60076-0
COST TUD Action (2013). TD-804 March 22, Publications. (J. Kang, K. Chourmouziadou, K. Sakantamis, B. Wang, and Y. Hao, Eds.) from COST TUD Action TD-0804 Soundscape of European Cities and Landscapes. Available online at: http://soundscape-cost.org/index.php?option=com_contentandview=articleandid=62:final-e-book-publishedandcatid=32:publicationsandItemid=9 (accessed April 15, 2016).
Darcy, A. E., Hancock, L. E., and Ware, E. J. (2008). A descriptive study of noise in the neonatal intensive care unit. ambient levels and perceptions of contributing factors. Adv. Neonatal Care, 8, 165–175. doi: 10.1097/01.ANC.0000324341.24841.6e
De Coensel, B., and Botteldooren, D. (2014). “Smart sound monitoring for sound event detection and characterisation,” in Proceedings of the INTERNOISE (Melbourne).
De Coensel, B., Sun, K., Wei, W., Van Renterghem, T., Sineau, M., Ribeiro, C., et al. (2015). “Dynamic noise mapping based on fixed and mobile sound measurements,” in Proceedings of the Euronoise (Maastrich), 2339–2344.
de Lima Andrade, E., da Cunha E Silva, D. C., de Lima, E. A., de Oliveira, R. A., Zannin, P., and Martins, A. (2021). Environmental noise in hospitals: a systematic review. Environ. Sci. Pollut. Res. Int., 28, 19629–19642. doi: 10.1007/s11356-021-13211-2
Dove, G., Mydlarz, C., Bello, J. P., and Oded, N. (2022). Sounds of New York city. Interactions 29, 32–35. doi: 10.1145/3527726
Duran, R., Ciftdemir, N. A., Ozbek, U. V., Berberoglu, U., Duranku,ş, F., Süt, N., et al. (2012). The effects of noise reduction by earmuffs on the physiologic and behavioral responses in very low birth weight preterm infants. Int. J. Pediatr. Otorhinolaryngol. 76, 1490–1493. doi: 10.1016/j.ijporl.2012.07.001
Elander, G., and Hellström, G. (1995). Reduction of noise levels in intensive care units for infants: evaluation of an intervention program. Heart Lung, 24, 376–379. doi: 10.1016/S0147-9563(05)80058-5
European Parliament. (2000). Directive 2000/14/EC of the European Parliament and of the Council of 8 May 2000 on the Approximation of the Laws of the Member States Relating to the Noise Emission in the Environment by Equipment for Use Outdoors. Available online at: https://eur-lex.europa.eu/legal-content/EN/TXT/?uri=celex:32000L0014
Graziuso, G., Francavilla, A. B., Mancini, S., and Guarnaccia, C. (2022). “Application of the harmonica Index for noise assessment in different spatial contexts,” in Journal of Physics: Conference Series, Volume 2162, 5th. International Conference on Applied Physics, Simulation and Computing (APSAC 2021) (Salerno). doi: 10.1088/1742-6596/2162/1/012006
Gupta, A., Gupta, A., Jain, K., and Gupta, S. (2018). Noise pollution and impact on children health. Indian J. Pediatr., 85, 300–306. doi: 10.1007/s12098-017-2579-7
Hellström, B., Nilsson, M. E., Axelsson, Ö., and Lundén, P. (2014). Acoustic design artifacts and methods for urban soundscapes: a case study on the qualitative dimensions of sounds. J. Archit. Plann. Res. 31, 57–71. Available online at: http://www.jstor.org/stable/43031024
Henze, H., Moshona, C., and Fiebig, A. (2023). “Katalogisierung von Lärmschutzmaßnahmen mit Gestaltungsaspekten,” in Fortschritte der Akustik – DAGA 2023, 49. Jahrestagung für Akustik, Deutsche Gesellschaft für Akustik e.V. (DEGA).
Hernández-Salazar, A. D., Gallegos-Martínez, J., and Reyes-Hernández, J. (2020). Level and noise sources in the neonatal intensive care unit of a reference hospital. Invest. Educac. Enferm. 38:e13. doi: 10.17533/udea.iee.v38n3e13
Hushcity website. (2022). Available online at: https://map.opensourcesoundscapes.org (accessed January 10, 2023).
ISO 12913-1. (2014). Acoustics—Soundscape—Part 1: Definition and Conceptual Framework. Geneva: International Organization for Standardization.
Javahid, S. (2023). Audio Data Collection for AI: Challenges and Best Practices in 2023. Available online at: https://research.aimultiple.com/audio-data-collection/ (accessed January 27, 2023).
Johnson, K. R., Hagadorn, J. I., and Sink, D. W. (2017). Alarm safety and alarm fatigue. Clin. Perinatol. 44, 713–728. doi: 10.1016/j.clp.2017.05.005
Kang, J., Aletta, F., Gjestland, T. T., Brown, L. A., Botteldooren, D., Schulte-Fortkamp, B., et al. (2016). Ten questions on the soundscapes of the built environment. Build. Environ. 108, 284–294. doi: 10.1016/j.buildenv.2016.08.011
Konkani, A., Oakley, B., and Penprase, B. (2014). Reducing hospital ICU noise: a behavior-based approach. J. Healthc. Eng. 5, 229–246. doi: 10.1260/2040-2295.5.2.229
Kosters, J., Janus, S. I. M., Van Den Bosch, K. A., Zuidema, S., Luijendijk, H. J., and Andringa, T. C. (2022). Soundscape optimization in nursing homes through raising awareness in nursing staff with MoSART. Front. Psychol. 13:871647. doi: 10.3389/fpsyg.2022.871647
Krueger, C., Schue, S., and Parker, L. (2007). Neonatal intensive care unit sound levels before and after structural reconstruction. MCN Am. J. Matern. Child Nurs. 32, 358–362. doi: 10.1097/01.NMC.0000298131.55032.76
Krueger, C., Wall, S., Parker, L., and Nealis, R. (2005). Elevated sound levels within a busy NICU. Neonatal Netw. 24, 33–37. doi: 10.1891/0730-0832.24.6.33
Lahav, A. (2015). Questionable sound exposure outside of the womb: frequency analysis of environmental noise in the neonatal intensive care unit. Acta. Paediatr. 104, e14–e19. doi: 10.1111/apa.12816
Lasky, R. E., and Williams, A. L. (2009). Noise and light exposures for extremely low birth weight newborns during their stay in the neonatal intensive care unit. Pediatrics, 123, 540–546. doi: 10.1542/peds.2007-3418
Lawson, K., Daum, C., and Turkewitz, G. (1977). Environmental characteristics of a neonatal intensive-care unit. Child Dev. 48, 1633–1639. doi: 10.2307/1128527
Le Bescond, V., Can, A., Aumond, P., and Gastineau, P. (2021). Open-source modeling chain for the dynamic assessment of road traffic noise exposure. Transp. Res. D. Transp. Environ. 94:102793. doi: 10.1016/j.trd.2021.102793
Lenzi, S., Lindborg, P. M., Han, N., Spagnol, S., Kamphuis, D., and Özcan, E. (2023). “Disturbed sleep: estimating night-time sound annoyance at a hospital ward,” in Proceedings of Forum Acusticum 2023 (Torino).
Levy, G. D., Woolston, D. J., and Browne, J. V. (2003). Mean noise amounts in level II vs level III neonatal intensive care units. Neonatal Netw. 22, 33–38. doi: 10.1891/0730-0832.22.2.33
Lionello, M., Aletta, F., and Kang, J. (2020). A systematic review of prediction models for the experience of urban soundscapes. Appl. Acoust. Volume 170:107479. doi: 10.1016/j.apacoust.2020.107479
Liu, W. F. (2010). The impact of a noise reduction quality improvement project upon sound levels in the open-unit-design neonatal intensive care unit. J. Perinatol. 30, 489–496. doi: 10.1038/jp.2009.188
Liu, W. F. (2012). Comparing sound measurements in the single-family room with open-unit design neonatal intensive care unit: the impact of equipment noise. J. Perinatol. 32, 368–373. doi: 10.1038/jp.2011.103
Livera, M. D., Priya, B., Ramesh, A., Suman Rao, P. N., Srilakshmi, V., Nagapoornima, M., et al. (2008). Spectral analysis of noise in the neonatal intensive care unit. Indian J. Pediatr., 75, 217–222. doi: 10.1007/s12098-008-0048-z
MacKenzie, D., and Galbrun, L. (2007). Noise levels and noise sources in acute care hospital wards. Build. Serv. Eng. Res. Technol. 28, 117–131. doi: 10.1177/0143624406074468
Masud, L., Valsecchi, F., Ciuccarelli, P., Ricci, D., and Caviglia, G. (2010). From data to knowledge: visualizations as transformation processes within the data-information-knowledge continuum. In Proc. Int. Conf. Inf. Vis. 2010, 445–449. doi: 10.1109/IV.2010.68
Mietlicki, C., Mietlicki, F., Ribeiro, C., Gaudibert, P., and Vincent, B. (2014). “The HARMONICA project, new tools to assess environmental noise and better inform the public,” in Proceedings of the Forum Acusticum 2014 (Kraków).
Milette, I. (2010). Decreasing noise level in our NICU: the impact of a noise awareness educational program. Adv. Neonatal Care, 10, 343–351. doi: 10.1097/ANC.0b013e3181fc8108
Misdariis, N., Marignier, L., Dianoux, C., and Leiba, R. (2019). “Experimental validation of an urban traffic noise annoyance model,” in ICSV - International Congress on Sound and Vibration (Montreal).
Mitchell, A., Oberman, T., Aletta, F., Kachlicka, M., Lionello, M., Erfanian, M., et al. (2021). (2021). Investigating urban soundscapes of the COVID-19 lockdown: a predictive soundscape modeling approach. J. Acoust. Soc. Am. 150, 4474. doi: 10.1121/10.0008928
Moshona, C., Aletta, F., Henze, H., Chen, X., Mitchell, A., Oberman, T., et al. (2022). “What is a soundscape intervention? exploring definitions and identification criteria and a platform to gather real-world examples,” in Internoise, 51st International Congress and Exposition on Noise Control Engineering.
Nautsch, A., Jiménez, A., Treiber, A., Kolberg, J., Jasserand, C., Kindt, E., et al. (2019). Preserving privacy in speaker and speech characterisation. Comput. Speech Lang. 58, 441–480. doi: 10.1016/j.csl.2019.06.001
Nielbo, F. L., Steele, D., and Guastavino, C. (2013). Investigating soundscape affordances through activity appropriateness. Proc. Mtgs. Acoust. 19, 040059. doi: 10.1121/1.4800502
NIOSH website. (2022). Available online at: https://www.cdc.gov/niosh/topics/noise/app.html (accessed January 10, 2023).
Noise Aware website. (2022). Available online at: https://noiseaware.com/ (accessed January q0, 2023).
Özcan, E., Broekmeulen, C. L. H., Luck, Z. A., van Velzen, M., Stappers, P. J., and Edworthy, J. R. (2022b). Acoustic biotopes, listeners and sound-induced action: a case study of operating rooms. Int. J. Environ. Res. Public Health. 19:16674. doi: 10.3390/ijerph192416674
Özcan, E., Kerimoglu, E., Müler, B. J., and Spagnol, S. (2022a). Exploring the Soundscape of the NICU: A Semi-Systematic Review With a Human-Centric Approach, (Technical Report).
Özcan, E., Liu, Y., Vroon, J., Kamphuis, D., and Spagnol, S. (2022c). “Doplor sleep: monitoring hospital soundscapes for better sleep hygiene,” in Proceedings of the 6th International Conference on Medical and Health Informatics (ICMHI '22). Association for Computing Machinery (New York, NY), 69–77. doi: 10.1145/3545729.3545745
Özcan, E., Rietdijk, W. J. R., and Gommers, D. (2019). Shaping critical care through sound-driven innovation: introduction, outline, and research agenda. Intensive Care Med. 46, 542–543. doi: 10.1007/s00134-019-05832-6
Özcan, E., and van Egmond, R. (2007). Memory for product sounds: the effect of sound and label type. Acta Psychol. 126, 196–215. doi: 10.1016/j.actpsy.2006.11.008
Özcan, E., and van Egmond, R. (2009). The effect of visual context on the identification of ambiguous environmental sounds. Acta Psychol. 131, 110–119. doi: 10.1016/j.actpsy.2009.03.007
Pedersen, K., Gee, K. L., Transtrum, M. K., and Butler, B. A. (2018). Machine learning-based prediction of outdoor ambient sound levels: ensemble averaging and feature reduction. J. Acoust. Soc. Am. 144, 1791–1791. doi: 10.1121/1.5067908
Pedersen, K., Transtrum, M. K., Gee, K. L., Lympany, S. V., James, M. M., and Salton, A. R. (2021). Validating two geospatial models of continental-scale environmental sound levels. JASA Express Lett. 1, 122401. doi: 10.1121/10.0007368
Philbin, M. K., Lickliter, R., and Graven, S. N. (2000). Sensory experience and the developing organism: a history of ideas and view to the future. J. Perinatol. 20, S2–S5. doi: 10.1038/sj.jp.7200434
Quinn, C. A., Burns, P., Gill, G., Baligar, S., Snyder, R. L., Salas, L., et al. (2022). Soundscape classification with convolutional neural networks reveals temporal and geographic patterns in ecoacoustic data. Ecol. Indic. 138:108831. doi: 10.1016/j.ecolind.2022.108831
Radicchi, A. (2021). “Citizen science mobile apps for soundscape research and public spaces studies: lessons from the Hush City project,” in Geographic Citizen Science Design: No one left behind, eds A. Skarlatidou, and M. Haklay (UCL Press), 130–148. doi: 10.2307/j.ctv15d8174.14
Radicchi, A., Cevikayak Yelmi, P., Chung, A., Jordan, P., Stewart, S., Tsaligopoulos, A., et al. (2021). Sound and the healthy city. Cities Health, 5, 1–2. doi: 10.1080/23748834.2020.1821980
Ramm, K., Mannix, T., Parry, Y., and Gaffney, M. P. (2017). A comparison of sound levels in open plan versus pods in a neonatal intensive care unit. Health Environ. Res. Design J. 10, 30–39. doi: 10.1177/1937586716668636
Restin, T., Gaspar, M., Bassler, D., Kurtcuoglu, V., Scholkmann, F., and Haslbeck, F. B. (2021). Newborn incubators do not protect from high noise levels in the neonatal intensive care unit and are relevant noise sources by themselves. Children, 8:704. doi: 10.3390/children8080704
Rosenblum, L. D., Wuestefeld, A. P., and Anderson, K. L. (1996). Auditory reachability: an affordance approach to the perception of sound source distance. Ecol. Psychol. 8, 1–24. doi: 10.1207/s15326969eco0801_1
Salamon, J., and Bello, J. P. (2017). Deep convolutional neural networks and data augmentation for environmental sound classification. IEEE Signal Process. Lett. 24, 279–283. doi: 10.1109/LSP.2017.2657381
Salamon, J., Bello, J. P., Farnsworth, A., Robbins, M., Keen, S., et al. (2016). Towards the automatic classification of avian flight calls for bioacoustic monitoring. PLoS ONE 11:e0166866. doi: 10.1371/journal.pone.0166866
Sevillano, X., Socoró, J. C., Alías, F., Bellucci, P., Peruzzi, L., Radaelli, S., et al. (2016). DYNAMAP–development of low cost sensors networks for real time noise mapping. Noise Mapp. 3, 172–189. doi: 10.1515/noise-2016-0013
Sharan, R. V., and Moir, T. J. (2016). An overview of applications and advancements in automatic sound recognition. Neurocomput. 200, 22–34. doi: 10.1016/j.neucom.2016.03.020
Smith, S. W., Ortmann, A. J., and Clark, W. W. (2018). Noise in the neonatal intensive care unit: a new approach to examining acoustic events. Noise Health, 20, 121–130. doi: 10.4103/nah.NAH_53_17
Snyder, H. (2019). Literature review as a research methodology: an overview and guidelines. J. Bus. Res. 104, 333–339. doi: 10.1016/j.jbusres.2019.07.039
Socoró, J. C., Alías, F., and Alsina-Pagès, R. M. (2017). An anomalous noise events detector for dynamic road traffic noise mapping in real-life urban and suburban environments. Sensors. 17:2323. doi: 10.3390/s17102323
Soundappraisal website. (2022). Available online at: https://soundappraisal.eu/mosart.html (accessed January 10, 2023).
Spagnol, S., Viñas Vila, N., Akdag Salah, A., Goos, T. G., and Özcan, E. (2023). “SOUNDscapes: a dashboard for promoting a healthy sound environment in the Neonatal Intensive Care Unit,” in Proceedings of Forum Acusticum 2023 (Torino).
Spagnol, S., Viñas Vila, N., Akdag Salah, A., Goos, T. G., Reiss, I., and Özcan, E. (2022). “Towards a quieter neonatal intensive care unit: current approaches and design opportunities,” in Proc. 2022 Int. Conf. of the Design Research Society (DRS 2022) (Bilbao). doi: 10.21606/drs.2022.258
Sueur, J., Farina, A., Gasc, A., Pieretti, N., and Pavoine, S. (2014). Acoustic indices for biodiversity assessment and landscape investigation. Acta Acustica united Acust. 100, 772–781. doi: 10.3813/AAA.918757
Surenthiran, S. S., Wilbraham, K., May, J., Chant, T., Emmerson, A. J., and Newton, V. E. (2003). Noise levels within the ear and post-nasal space in neonates in intensive care. Arch. Dis. Child. Fetal Neonatal Ed. 88, F315–F318. doi: 10.1136/fn.88.4.F315
Szymczak, S. E., and Shellhaas, R. A. (2014). Impact of NICU design on environmental noise. J. Neonatal Nurs. 20, 77–81. doi: 10.1016/j.jnn.2013.07.003
Teixeira, L. R., Pega, F., Dzhambov, A. M., Bortkiewicz, A., da Silva, D., de Andrade, C., et al. (2021). The effect of occupational exposure to noise on ischaemic heart disease, stroke and hypertension: a systematic review and meta-analysis from the WHO/ILO Joint Estimates of the work-related burden of disease and injury. Environ. Int. 154:106387. doi: 10.1016/j.envint.2021.106387
Thibaud, J. P., and Amphoux, P. (2013). Silencing the city?. SoundEffects Interdiscip. J. Sound Sound Exp. 3, 62–70. doi: 10.7146/se.v3i3.18441
Thomas, K. A. (1989). How the NICU environment sounds to a preterm infant. MCN Am. J. Matern. Child Nurs. 14, 249–251. doi: 10.1097/00005721-198907000-00007
Thomas, K. A., and Uran, A. (2007). How the NICU environment sounds to a preterm infant: update. MCN Am. J. Matern. Child Nurs. 32, 250–253. doi: 10.1097/01.NMC.0000281966.23034.e9
Torresin, S., Albatici, R., Aletta, F., Babich, F., Oberman, T., Siboni, S., et al. (2020). Indoor soundscape assessment: a principal components model of acoustic perception in residential building. Build. Environ. 182:107152. doi: 10.1016/j.buildenv.2020.107152
Trickey, A. W., Arnold, C. C., Parmar, A., and Lasky, R. E. (2012). Sound levels, staff perceptions, and patient outcomes during renovation near the neonatal intensive care unit. Health Environ. Res. Design J. 5, 76–87. doi: 10.1177/193758671200500407
Varisco, G., van de Mortel, H., Cabrera-Quiros, L., Atallah, L., Hueske-Kraus, D., Long, X., et al. (2021). Optimisation of clinical workflow and monitor settings safely reduces alarms in the NICU. Acta Paediatr. 110, 1141–1150. doi: 10.1111/apa.15615
Wang, D., Aubertin, C., Barrowman, N., Moreau, K., Dunn, S., and Harrold, J. (2014). Examining the effects of a targeted noise reduction program in a neonatal intensive care unit. Arch. Dis. Child. Fetal Neonatal Ed. 99, F203–F208. doi: 10.1136/archdischild-2013-304928
Wei, W., and Van Renterghem, T. (2014). “Monitoring sound exposure by real time measurement and dynamic noise map,” in Forum Acusticum 2014 Proceedings (Krakow EAA).
Williams, A. L., Sanderson, M., Lai, D., Selwyn, B. J., and Lasky, R. E. (2009). Intensive care noise and mean arterial blood pressure in extremely low-birth-weight neonates. Am. J. Perinatol. 26, 323–329. doi: 10.1055/s-0028-1104741
Keywords: neonatal intensive care unit (NICU), acoustic environment evaluation, indoor sound quality, soundscape perception, Machine Listening, indoor soundscape assessment, indoor soundscape modelling
Citation: Lenzi S, Spagnol S and Özcan E (2023) Improving the quality of the acoustic environment in neonatal intensive care units: a review of scientific literature and technological solutions. Front. Comput. Sci. 5:1156693. doi: 10.3389/fcomp.2023.1156693
Received: 01 February 2023; Accepted: 18 September 2023;
Published: 05 October 2023.
Edited by:
Arianna Astolfi, Polytechnic University of Turin, ItalyReviewed by:
Marcia Epstein, University of Calgary, CanadaVeronica Amodeo, Università di Firenze, Italy
Kjetil Falkenberg, Royal Institute of Technology, Sweden
Martin Ljungdahl Eriksson, University West Trollhättan, Sweden, in collaboration with reviewer [KF]
Copyright © 2023 Lenzi, Spagnol and Özcan. This is an open-access article distributed under the terms of the Creative Commons Attribution License (CC BY). The use, distribution or reproduction in other forums is permitted, provided the original author(s) and the copyright owner(s) are credited and that the original publication in this journal is cited, in accordance with accepted academic practice. No use, distribution or reproduction is permitted which does not comply with these terms.
*Correspondence: Sara Lenzi, cy5sZW56aUB0dWRlbGZ0Lm5s; c2FyYS5sZW56aUBkZXVzdG8uZXM=