- Department of Cardiology, Aerospace Center Hospital, Beijing, China
Background: Irreversible worsening of cardiac function is an adverse event associated with significant morbidity among patients with acute decompensated heart failure (ADHF). We aimed to develop a parsimonious model which is simple to use in clinical settings for the prediction of the risk of irreversible worsening of cardiac function.
Methods: A total of 871 ADHF patients were enrolled in this study. Data for each patient were collected from the medical records. Irreversible worsening of cardiac function included cardiac death within 30-days of patient hospitalization, implantation of a left ventricular assistance device, or emergency heart transplantation. We performed LASSO regression for variable selection to derive a multivariable logistic regression model. Five candidate predictors were selected to derive the final prediction model. The prediction model was verified using C-statistics, calibration curve, and decision curve.
Results: Irreversible worsening of cardiac function occurred in 7.8% of the patients. Advanced age, NYHA class, high blood urea nitrogen, hypoalbuminemia, and vasopressor use were its strongest predictors. The prediction model showed good discrimination C-statistic value, 0.866 (95% CI, 0.817–0.907), which indicated good identical calibration and clinical efficacy.
Conclusion: In this study, we developed a prediction model and nomogram to estimate the risk of irreversible worsening of cardiac function among ADHF patients. The findings may provide a reference for clinical physicians for detection of irreversible worsening of cardiac function and enable its prompt management.
Background
Approximately 4 million people in China were estimated to have heart failure, with a prevalence of 0.4–1.3% (1). Patients hospitalized with acute decompensated heart failure (ADHF) have a high risk of mortality, with 30-day mortality rates approaching 10% (2). Some patients are discharged from the hospital after treatment, while in others deterioration of heart failure occurs during hospitalization, and remaining patients show irreversible worsening of heart function. Patients who experienced in-hospital worsening heart failure had high 30-day mortality (29.7%) (3). Therefore, it is necessary to predict the clinical course of heart failure as early as possible, to enable the selection of evidence-based management strategies to improve the treatment and nursing of patients with heart failure.
Risk prediction models are often used to classify patients and simplify treatment decisions. They help physicians predict prognoses and interpret the results of prognostic studies to improve the level of care for inpatients with heart failure.
To date, many death prediction models for ADHF have been developed and verified (4–7). However, the ability of these models to predict individual patient outcomes is limited. Many of these models only show moderate effects (mortality based on C-statistics: 0.70–0.80) (4, 8). Calibration is also poor even after global recalibrations (9, 10). So their utility is limited in clinical settings.
We, therefore, aimed to develop a practical risk prediction model for irreversible worsening of cardiac function among ADHF patients.
Methods
The Transparent Reporting for Individual Prognosis or Diagnosis (TRIPOD) recommendation was used as the guideline for the development and validation of the multivariable prediction model (11).
Data Sources and Processing
This study was approved by the ethics committee of the Aerospace Center Hospital, Beijing, China. Written informed consent was waived owing to the use of anonymous retrospective data. Demographic, clinical, and laboratory data were retrieved from the electronic hospital database. A team of experienced clinical cardiologists reviewed and cross-checked the data. Each record was independently verified by two clinicians.
Patient Selection
A total of 1,222 patients diagnosed with ADHF who were admitted to the Aerospace Center Hospital (a tertiary hospital in Beijing, China) between January 2017 to December 2020 were recruited retrospectively. ADHF was diagnosed following the guidelines of the European Society of Cardiology (12).
We excluded patients according to the following criteria: <18 years old, pregnant women, sudden cardiac death before admission, those who underwent surgery, reversible cardiomyopathy (tachycardiomyopathy, alcoholic cardiomyopathy, stress cardiomyopathy, drug/toxicant cardiotoxicity, and myocarditis) (Figure 1).
Potential Predictive Variables
Consistent data for each patient were collected from the medical records, and all candidate predictors were selected based on a detailed literature review and clinical evidence within the confines of data availability.
Demographic variables included sex, age, height, and weight values. Medical history included the presence of diabetes, hypertension, coronary artery disease, previous heart failure, atrial fibrillation, previous renal dysfunction, cerebral infarction, cancer, and cirrhosis. Clinical signs and symptoms included categorical and continuous variables as follows: New York Heart Association (NYHA) functional class, paroxysmal nocturnal dyspnea, orthopnea, heart rate, systolic blood pressure, diastolic blood pressure, rales (>1/2 lung fields), jugular venous distension, peripheral edema. Imaging results consisted of left ventricular ejection fraction (LVEF) by two-dimensional transthoracic echocardiography, and laboratory findings included evaluation of levels of B-type natriuretic peptide, troponin I, hemoglobin, C-reactive protein, alanine aminotransferase, blood urea nitrogen, creatinine, albumin, serum sodium, serum potassium, uric acid, and glucose. We recorded the baseline values of these tests, with the first value taken within 2 days of onset admission. The treatment regime included administration of aldosterone antagonists, loop diuretic, angiotensin-converting enzyme inhibitors/angiotensin receptor blockers (ACE-Is/ARBs), beta-blockers, anticoagulants, aspirin, ADP-P2Y12 antagonists, and/or vasopressors. Detailed and specific definitions of the variables included are listed in Supplementary Table 1.
Characteristics of Irreversible Worsening of Cardiac Function
Irreversible worsening of cardiac function included cardiac death, implantation of a left ventricular assistance device, or emergency heart transplantation within 30-days of hospitalization.
Sample Size
We considered the events per variables (EPV) ratio between 5 and 10 acceptable, with EPV of 10 as the optimal number to minimize the overfitting of the regression model (13). According to this rule, we required a total of 50 ADHF inpatients who exhibited irreversible worsening of cardiac function to evaluate five candidate predictors. Assuming that the prevalence of 30-day mortality was ~10% among patients with ADHF (14, 15), a total sample size of at least 500 would suffice. Thus, to ensure an adequate number of events, we decided to collect data of at least 500 individuals.
Handling Missing Data
Before data analysis, predictor variables were inspected for missing values. Among the predictors, the proportion of missing data was 0.34–6.2%. To include these data in the analyses, we imputed missing data through multiple imputations using chained equations of the mice package in R, in which predictive mean matching is embedded with the cases (k) = 5 as the default. Baseline clinical characteristics before and after imputations are listed in Supplementary Table 2.
Statistical Analysis
Data were presented as frequencies (percentages) for categorical variables and as mean (standard deviation) or median (interquartile ranges [IQRs]) for continuous variables. Means for continuous variables were compared using t-tests when the data were normally distributed; else, the Mann-Whitney U test was used. Proportions of categorical variables were compared using the χ2 test; the Fisher exact probability test was used when the data were limited. The statistical significance level for all tests was set as α = 0.05; P <0.05 (two-tailed) was considered statistically significant.
Variable Selection
The least absolute shrinkage and selection operator (LASSO) regression is a compression estimation used for collinearity estimates between covariates. When there are several collinear predictors, LASSO selects only one and ignores the others or zeroes out some regression coefficients. The glmnet package in R was used for LASSO regression analysis, the lambda values were selected after 10-fold-cross-validation; the larger the lambda value, the more compact was the model. Briefly, for cross-verification, the data were divided into 10 equal parts. First, the whole data were fit to generate a lambda sequence. Second, one data point was excluded each time, and the remaining nine were used for verification. The averages and standard deviations of the deviance obtained after 10 time-verification were calculated. Finally, two models were obtained as the output. One was based on lambda.min, that is, the lambda whose deviance mean was the smallest; the other was based on lambda.1se, that is, the maximum lambda corresponding to the deviance mean within one standard deviation of the minimum value.
The results of the analysis were considered in conjunction with clinical evidence, sample size, and statistical ability (16). Finally, five candidate predictors were selected to derive the prediction model and build a nomogram based on the results of the logistic regression model.
Model Validation
We performed internal validation of the model development processes using the bootstrap resampling method (500 bootstrap samples per model) to obtain an unbiased estimate of model performance (16). Then the prediction model was verified using C-statistics, calibration curve (17), and decision curve analysis (18).
Sensitivity Analysis
Given the heterogeneity in sex (19, 20) and history of coronary artery disease (21), to investigate whether the predictive strength of the nomogram changed due to these predictors, we evaluated the C-statistics for the subgroups.
Statistical Analysis Software
Data were analyzed using statistical packages in R (The R Foundation; http://www.r-project.org; version 4.0.5).
Results
In total, 871 ADHF patients were included in this study; the mean age was 75.30 years, and 412 (47.30%) patients were male. Sixty eight patients had irreversibly worsened cardiac function, which implied that the incidence was 7.80%.
Unlike other patients, those with irreversible worsening of cardiac function were older (P <0.05). Table 1 shows the comparison of the patient characteristics in the study.
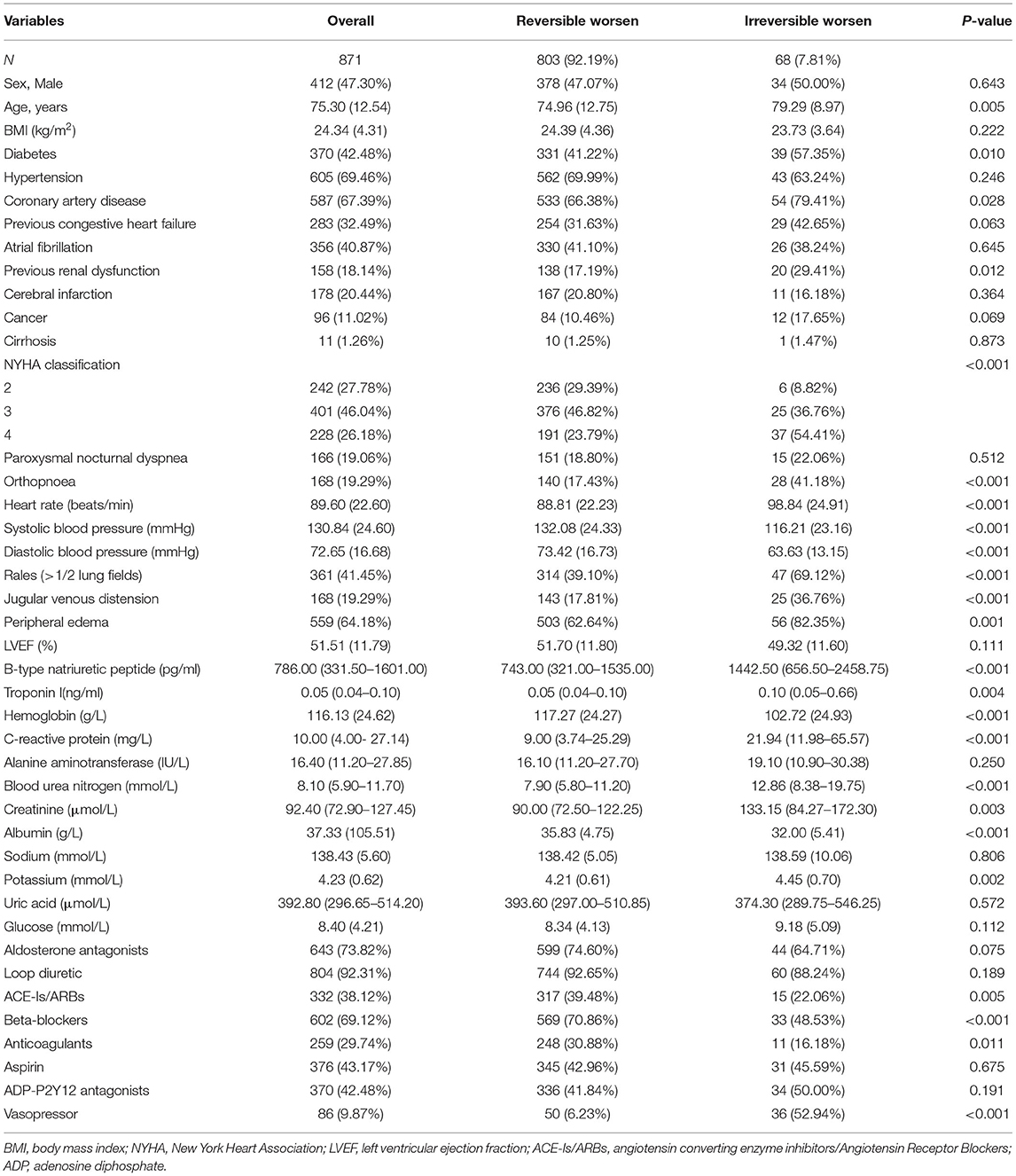
Table 1. Comparison of the characteristics between the reversible worsen and irreversible worsen groups.
Variable Selection and Model Development
Based on the LASSO analysis (Supplementary Figure 1), we identified model 1 consisting of four variables. The prediction model can accommodate five variables. Since age was an important factor affecting the prognosis of patients (6, 22), it was included in the model (16). Then we derived the prediction model 2 with the five variables (Table 2). We evaluated the C-statistics for each model (Table 3).
Finally, we selected model 2 with five candidate predictors to derive the prediction model and built a nomogram based on the logistic regression model (Figure 2).
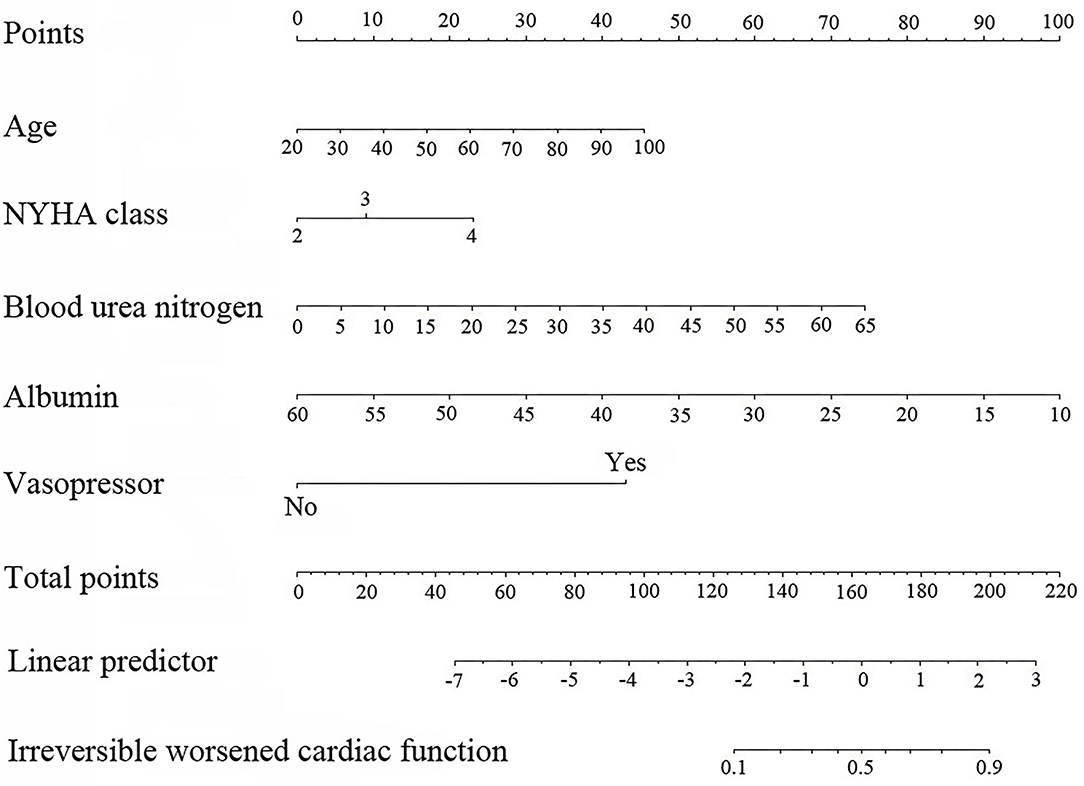
Figure 2. Nomogram based on the results of for logistic regression model (age in years, blood urea nitrogen in g/L, and albumin in g/L).
The risk score was calculated for each patient using the following formula derived from the expression of the five variables weighed by their regression coefficients: Risk score = −4.29800 +0.03388 × Age (years) +0.53645 × NYHA class +0.30738 × NYHA class = 4 +0.06832 × Blood urea nitrogen (g/L) −0.11930 × Albumin (g/L) +2.56681 × (Vasopressor = 1).
Model Validation
The bootstrap analysis showed a good discriminative ability for the prediction model (C-statistics: 0.866 [95% CI, 0.817–0.907]) (Figure 3A). The calibration plots of the model based on the bootstrap method showed good performance (Figure 3B). Decision curve analysis showed moderate clinical efficacy of the model (Figure 3C).
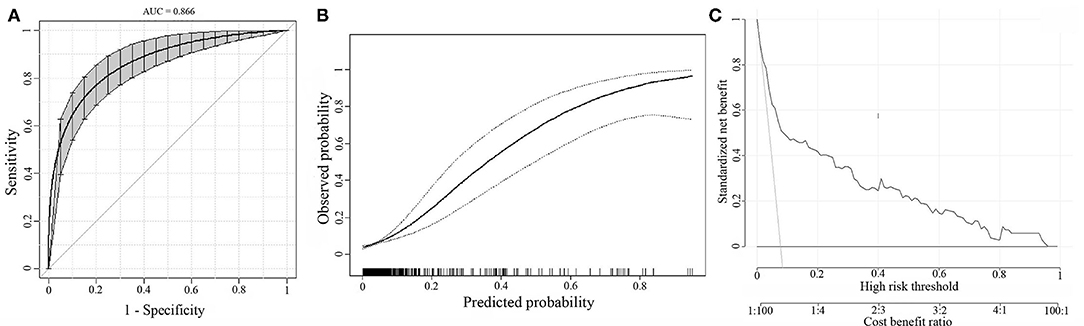
Figure 3. Internal model validation. (A) The discrimination of the prediction model by the bootstrap method. (B) Calibration plots of the model by the bootstrap method. (C) Decision curve analysis for the model.
Sensitivity Analysis
The discrimination of the prediction model was consistent for the sex and coronary artery disease history subgroups (C-statistics for the male subgroup: 0.827 [95% CI, 0.748–0.906], C-statistics for the female subgroup: 0.912 [95% CI, 0.868–0.956], C-statistics for the coronary artery disease subgroup: 0.859 [95% CI, 0.802–0.916], C-statistics for without coronary artery disease subgroup: 0.907 [95% CI, 0.846–0.967]) (Supplementary Table 3).
Nomogram Interpretation
The point in Figure 2 was the selected scoring standard or scale. For each independent variable, a straight line perpendicular to the point's axis (through a ruler) was made. The intersection point represented the score for the value of the independent variable. For example, age at 60 meant 22.5 points and NYHA class at (IV) meant 22.5 points. The corresponding points of these independent variables for each patient were calculated and the total points were estimated; thus the perpendicular line location to the axis was estimated. This indicated the risk of irreversible worsening of cardiac function in the corresponding patient.
Discussion
Based on the LASSO regression, we found four predictive risk factors for irreversible worsening of cardiac function, including NYHA class, high blood urea nitrogen, hypoalbuminemia, and use of vasopressor, in this retrospective study. Since age was an important factor affecting the prognoses of patients, this variable was also added (23).
We took many steps to minimize the potential bias (24). This model applies to a broad spectrum of patients with heart failure, including those with preserved left ventricular systolic function, the ones diagnosed with ADHF, or newly diagnosed with acute heart failure, and those previously diagnosed with heart failure.
The discussion on variable screening has been an ongoing one. The analysis results, clinical reasons, sample size, and statistical power are simultaneously considered. The predictive variables in the nomogram model are convenient for clinical acquisition and thus, the construction of these models is feasible. We performed LASSO regression analysis to select variables. This parsimonious model showed sufficiently stable applicability. Sometimes machine learning algorithms are used to construct models and most of them are non-parametric. However, owing to the absence of parameters like regression coefficients, the clinical interpretation of such non-parametric models is difficult (23). Therefore, we did not use machine learning algorithms for variable filtering.
Interestingly, although B-type natriuretic peptide is a widely recognized prognostic factor for patients with heart failure (25), it has not been included in the risk prediction models. The B-type natriuretic peptide is affected by several factors; for example, B-type natriuretic peptide levels are lower in obese people (25), thus, it is only available in some of the enrolled patients. Although B-type natriuretic peptide has an accurate prognostic ability in inpatients with heart failure, other clinical factors may also play a key role in influencing prognosis. Further analysis showed that B-type natriuretic peptide does not contribute significantly to the nomogram to indicate the poor score. Therefore, considering the major risk factors in the risk scoring system is a necessary condition for predicting important outcomes (23).
Consistent with previous studies, the renal function also was an important predictor of outcomes (26). Serum urea nitrogen level was a stronger predictor than creatinine level. The greater prognostic power of serum urea nitrogen level may be attributed to its incorporation in both prerenal and renal function statuses (27). Serum urea nitrogen level is a factor incorporated in other predictive models, such as blood urea nitrogen level for predicting in-hospital mortality (6, 22) and post-discharge clinical outcomes (4). Heart and kidney interactions are complex, and the subject is of immense clinical and scientific interest and debate. The coexistence of acute cardiac and renal dysfunction, termed acute cardiorenal syndrome, is correlated with increased mortality and results in adverse outcomes (27).
Limitations
In addition to these findings, some limitations exist in this study which should be addressed in the future. First, this was an observational study, and thus, we could not draw direct causal conclusions. Second, we excluded the patients with reversible cardiomyopathy and those who underwent surgery. Hence, the findings of this study cannot be extrapolated to the general population. Third, the non-linearity and interaction of variables were not analyzed. This may need to be investigated in the future. Finally, as this study was based on patients from a single center in China, there existed an inevitable sample selection bias, and external validation of the findings was lacking. As such, prospective validation to examine model stability, reproducibility, and external verification in independent samples is needed.
We evaluated ADHF cardiac function in patients using the irreversible worsening prediction model. This would allow for early prediction of the patient's clinical course, and allocation of appropriate resources, including transplantation and mechanical circulation auxiliary equipment.
Conclusion
In conclusion, an irreversible worsening of cardiac function is an adverse event associated with significant morbidity among patients with ADHF. Currently, there is no effective practical tool for estimating its occurrence likelihood. In this study, we developed a risk score-based prediction model and nomogram to estimate the risk of irreversible worsening of cardiac function among ADHF patients. The findings may provide a reference for clinical physicians to detect irreversible worsening of cardiac function and manage it promptly.
Data Availability Statement
The original contributions presented in the study are included in the article/Supplementary Material, further inquiries can be directed to the corresponding author/s.
Ethics Statement
The studies involving human participants were reviewed and approved by the Ethics Committee of the Aerospace Center Hospital, Beijing, China. Written informed consent for participation was not required for this study in accordance with the national legislation and the institutional requirements.
Author Contributions
Y-TZ and LW made contributions to data collection, drafted the manuscript, and made contributions to the analysis and interpretation of the data. All authors contributed to the article and approved the submitted version.
Conflict of Interest
The authors declare that the research was conducted in the absence of any commercial or financial relationships that could be construed as a potential conflict of interest.
Publisher's Note
All claims expressed in this article are solely those of the authors and do not necessarily represent those of their affiliated organizations, or those of the publisher, the editors and the reviewers. Any product that may be evaluated in this article, or claim that may be made by its manufacturer, is not guaranteed or endorsed by the publisher.
Supplementary Material
The Supplementary Material for this article can be found online at: https://www.frontiersin.org/articles/10.3389/fcvm.2021.785587/full#supplementary-material
References
1. Ariely R, Evans K, Mills T. Heart failure in China: a review of the literature. Drugs. (2013) 73:689–701. doi: 10.1007/s40265-013-0057-8
2. Lagu T, Pekow PS, Shieh M-S, Stefan M, Pack QR, Kashef MA, et al. Validation and comparison of seven mortality prediction models for hospitalized patients with acute decompensated heart failure. Circulation Heart Fail. (2016) 9:e002912. doi: 10.1161/CIRCHEARTFAILURE.115.002912
3. Clark AL, Cherif M, McDonagh TA, Squire IB. In-hospital worsening heart failure: a clinically relevant endpoint? ESC Heart Fail. (2018) 5:9–18. doi: 10.1002/ehf2.12195
4. Demissei BG, Valente MA, Cleland JG, O'Connor CM, Metra M, Ponikowski P, et al. Optimizing clinical use of biomarkers in high-risk acute heart failure patients. Eur J Heart Fail. (2016) 18:269–80. doi: 10.1002/ejhf.443
5. Eapen ZJ, Liang L, Fonarow GC, Heidenreich PA, Curtis LH, Peterson ED, et al. Validated, electronic health record deployable prediction models for assessing patient risk of 30-day rehospitalization and mortality in older heart failure patients. JACC Heart Fail. (2013) 1:245–51. doi: 10.1016/j.jchf.2013.01.008
6. Peterson PN, Rumsfeld JS, Liang L, Albert NM, Hernandez AF, Peterson ED, et al. A validated risk score for in-hospital mortality in patients with heart failure from the American Heart Association get with the guidelines program. Circ Cardiovasc Qual Outcomes. (2010) 3:25–32. doi: 10.1161/CIRCOUTCOMES.109.854877
7. Sahle BW, Owen AJ, Chin KL, Reid CM. Risk prediction models for incident heart failure: a systematic review of methodology and model performance. J Card Fail. (2017) 23:680–7. doi: 10.1016/j.cardfail.2017.03.005
8. Ketchum ES, Levy WC. Establishing prognosis in heart failure: a multimarker approach. Prog Cardiovasc Dis. (2011) 54:86–96. doi: 10.1016/j.pcad.2011.03.003
9. Wessler BS, Ruthazer R, Udelson JE, Gheorghiade M, Zannad F, Maggioni A, et al. Regional validation and recalibration of clinical predictive models for patients with acute heart failure. J Am Heart Assoc. (2017) 6:e006121. doi: 10.1161/JAHA.117.006121
10. Nagai T, Sundaram V, Shoaib A, Shiraishi Y, Kohsaka S, Rothnie KJ, et al. Validation of US mortality prediction models for hospitalized heart failure in the United Kingdom and Japan. Eur J Heart Fail. (2018) 20:1179–90. doi: 10.1002/ejhf.1210
11. Collins GS, Reitsma JB, Altman DG, Moons KG, Group T. Transparent reporting of a multivariable prediction model for individual prognosis or diagnosis (TRIPOD): the TRIPOD statement. The TRIPOD Group. Circulation. (2015) 131:211–9. doi: 10.1161/CIRCULATIONAHA.114.014508
12. Ponikowski P, Voors AA, Anker SD, Bueno H, Cleland JGF, Coats AJS, et al. 2016 ESC Guidelines for the diagnosis and treatment of acute and chronic heart failure: the Task Force for the diagnosis and treatment of acute and chronic heart failure of the European Society of Cardiology (ESC) developed with the special contribution of the Heart Failure Association (HFA) of the ESC. Eur Heart J. (2016) 37:2129–200. doi: 10.1093/eurheartj/ehw128
13. Riley RD, Ensor J, Snell KIE, Harrell FE Jr, Martin GP, Reitsma JB, et al. Calculating the sample size required for developing a clinical prediction model. BMJ. (2020) 368:m441. doi: 10.1136/bmj.m441
14. Doshi R, Dhawan T, Rendon C, Rodriguez M, Al-Khafaji J, Taha M, et al. Incidence and implications of acute kidney injury in patients hospitalized with acute decompensated heart failure. Intern Emerg Med. (2019) 15:421–8. doi: 10.1007/s11739-019-02188-z
15. Thorvaldsen T, Claggett BL, Shah A, Cheng S, Agarwal SK, Wruck LM, et al. Predicting risk in patients hospitalized for acute decompensated heart failure and preserved ejection fraction: the atherosclerosis risk in communities study heart failure community surveillance. Circ Heart Fail. (2017) 10:e003992. doi: 10.1161/CIRCHEARTFAILURE.117.003992
16. Zhou ZR, Wang WW Li Y, Jin KR, Wang XY, Wang ZW, et al. In-depth mining of clinical data: the construction of clinical prediction model with R. Ann Transl Med. (2019) 7:796. doi: 10.21037/atm.2019.08.63
17. Alba AC, Agoritsas T, Walsh M, Hanna S, Iorio A, Devereaux PJ, et al. Discrimination and calibration of clinical prediction models: users' guides to the medical literature. JAMA. (2017) 318:1377–84. doi: 10.1001/jama.2017.12126
18. Steyerberg EW, Vergouwe Y. Towards better clinical prediction models: seven steps for development and an ABCD for validation. Eur Heart J. (2014) 35:1925–31. doi: 10.1093/eurheartj/ehu207
19. Lawson CA, Zaccardi F, Squire I, Ling S, Davies MJ, Lam CSP, et al. 20-year trends in cause-specific heart failure outcomes by sex, socioeconomic status, and place of diagnosis: a population-based study. Lancet Public Health. (2019) 4:e406–20. doi: 10.1016/S2468-2667(19)30108-2
20. Kajimoto K, Sato N. Sex differences in New York Heart Association functional classification and survival in acute heart failure patients with preserved or reduced ejection fraction. Can J Cardiol. (2020) 36:30–6. doi: 10.1016/j.cjca.2019.08.020
21. Rusinaru D, Houpe D, Szymanski C, Lévy F, Maréchaux S, Tribouilloy C. Coronary artery disease and 10-year outcome after hospital admission for heart failure with preserved and with reduced ejection fraction. Eur J Heart Fail. (2014) 16:967–76. doi: 10.1002/ejhf.142
22. Voors AA, Ouwerkerk W, Zannad F, van Veldhuisen DJ, Samani NJ, Ponikowski P, et al. Development and validation of multivariable models to predict mortality and hospitalization in patients with heart failure. Eur J Heart Fail. (2017) 19:627–34. doi: 10.1002/ejhf.785
23. Agrawal SK, Shrank WH. Clinical and social risk adjustment - reconsidering distinctions. N Engl J Med. (2020) 382:1581–3. doi: 10.1056/NEJMp1913993
24. Wolff RF, Moons KGM, Riley RD, Whiting PF, Westwood M, Collins GS, et al. PROBAST: a tool to assess the risk of bias and applicability of prediction model studies. Ann Intern Med. (2019) 170:51–8. doi: 10.7326/M18-1376
25. Mueller C, McDonald K, de Boer RA, Maisel A, Cleland JGF, Kozhuharov N, et al. Heart Failure Association of the European Society of Cardiology practical guidance on the use of natriuretic peptide concentrations. Eur J Heart Fail. (2019) 21:715–31. doi: 10.1002/ejhf.1494
26. O'Connor CM, Whellan DJ, Wojdyla D, Leifer E, Clare RM, Ellis SJ, et al. Factors related to morbidity and mortality in patients with chronic heart failure with systolic dysfunction: the HF-ACTION predictive risk score model. Circ Heart Fail. (2012) 5:63–71. doi: 10.1161/CIRCHEARTFAILURE.111.963462
Keywords: acute decompensated heart failure, irreversible worsened cardiac function, prediction model, development and validation, nomogram
Citation: Wang L and Zhao Y-T (2021) Development and Validation of a Prediction Model for Irreversible Worsened Cardiac Function in Patients With Acute Decompensated Heart Failure. Front. Cardiovasc. Med. 8:785587. doi: 10.3389/fcvm.2021.785587
Received: 29 September 2021; Accepted: 19 November 2021;
Published: 10 December 2021.
Edited by:
Alexander E. Berezin, Zaporizhia State Medical University, UkraineReviewed by:
Julie K. K. Vishram-Nielsen, Rigshospitalet, DenmarkMahmoud Hassanein, Alexandria University, Egypt
Copyright © 2021 Wang and Zhao. This is an open-access article distributed under the terms of the Creative Commons Attribution License (CC BY). The use, distribution or reproduction in other forums is permitted, provided the original author(s) and the copyright owner(s) are credited and that the original publication in this journal is cited, in accordance with accepted academic practice. No use, distribution or reproduction is permitted which does not comply with these terms.
*Correspondence: Yun-Tao Zhao, raas@hsc.pku.edu.cn