- 1Learning Sciences and Higher Education, LSE, Behavioral Section, Department of Humanities, Social and Political Sciences, D-GESS, ETH Zurich, Zurich, Switzerland
- 2Educational Development and Technology, LET, ETH Zurich, Zurich, Switzerland
Learning is a process that leads to outcomes. The science of learning is the epistemic practice of investigating the relationship between this process and its outcomes. We propose a novel method for the study of learning: The Double Treatment experimental design. The core design for experimental studies in the Learning Sciences consists of two elements, learning activities together with assessments of their outcomes. In conventional Single Treatment experimental designs, the learning activities are subject to a controlled experimental manipulation, the outcomes of which are then evaluated in a common assessment. In the Double Treatment experimental design, both the learning activity and the assessment are subject to the experimental manipulation, leading to a basic 2 × 2 experimental design. We provide a theoretical rationale in favor of the Double Treatment design, which we illustrate and discuss in three experimental study examples implementing this method: A study on the contextual nature of memorization and recall while scuba diving, a study on invention activities as a preparation for future learning, and a study on resource-rich assessment in computer science. We argue that the Double Treatment design has the potential to enhance the epistemic power of experimental Learning Sciences research by improving the ontological coherence of experimental designs. They promise to facilitate both hypothesis testing and hypothesis generation, enable the validation of assessment methods from within corresponding studies, and reduce the need for externally validated assessments outside the control of the researchers.
Introduction
Controlled experiments constitute the gold standard scientific method in many disciplines. They induce changes in the object of study through experimental manipulation and capture resulting outcomes with appropriate measurement arrangements. Controlled experiments in the Learning Sciences consist of two core elements: Learning activities, subject to the experimental manipulation, and some form of assessment of learning as the measurement arrangement. The experimental manipulation of learning typically operationalizes contrasting and/or competing ontologies of learning and cognition. The assessment then discerns qualitative or quantitative differences in learning facilitation between these contrasting ontologies. Assessments however are not ontologically agnostic blind arbiters. Instead, they are themselves grounded in—implicit or explicit—ontologies of learning and cognition. This leads to a simple conclusion: Operationalizing competing ontologies of learning in the experimental manipulation of the learning activity alone does not suffice. Instead, the operationalization must be repeated with a second, independent, and ontologically homologous experimental manipulation in the assessment of learning. We call this the Double Treatment experimental design in contrast to conventional Single Treatment experimental designs.
We first provide a brief description of the Single Treatment experimental design in section The Conventional Single Treatment Experimental Design, followed by a description of the Double Treatment experimental design in section The Double Treatment Experimental Design. We then provide three incremental theoretical arguments, which motivate the Double Treatment design in section Rationale. Finally, we illustrate and discuss the Double Treatment design together with its motivating theoretical rationales on the basis of three empirical study examples, which implemented critical elements of the Double Treatment design in section Examples. For readers who prefer moving from the concrete to the abstract, rather than from the abstract to the concrete, we suggest reading sections Introduction through Examples in reverse order. We close the article with a brief summary and conclusion.
To facilitate reading and comprehension we will formalize key elements of the Double Treatment design through notations inspired by mathematical notation in logic and set theory. We highlight notations in bold print when we first define them.
Throughout this article, we will problematize shortcomings of conventional Single Treatment designs and argue how Double Treatment designs remediate these shortcomings. Does this mean we advocate—as one reviewer commented—throwing the baby out with the bathwater and abandoning Single Treatment designs altogether? We do not. Double Treatment designs promise an important gain: Increased epistemic power regarding foundational questions on the nature of learning. However, Double Treatment designs also incur a considerable cost: With double the experimental conditions, they also require double the number of subjects for equal statistical power as Single Treatment designs. Hence, instead of rejection we advocate that adopting Single Treatment designs requires proper justification in light of the issues highlighted in this article. More importantly however, we hope this article will encourage other researchers to try out Double Treatment designs.
The Conventional Single Treatment Experimental Design
The Single Treatment design consists of some learning activity followed by an assessment. The learning activity is subject to the experimental treatments of interest. The learning treatments reflect contrasting assumptions about the nature of learning, ontology A vs. ontology B. We call this the ontological contrast, A ≠ B. Frequently, one of the treatments is not discussed as a “treatment” at all, but instead as a benchmark or baseline condition against which the “actual” experimental treatment is compared. Since there is neither a need nor justification to discriminate between a “benchmark” and an “actual” treatment, we will simply discuss these also as learning treatments. Typically, the treatments are operationalized between subjects, leading to two corresponding experimental groups. Following the learning activities, all subjects then take a common assessment in order to determine differences in learning outcomes between the two experimental groups. To ensure validity, it is good practice to rely on assessments that have a priori been validated, often in independent validation studies. In other words, the assessments' validity is determined outside the scope of the study proper. Figure 1 illustrates the conventional Single Treatment experimental design.
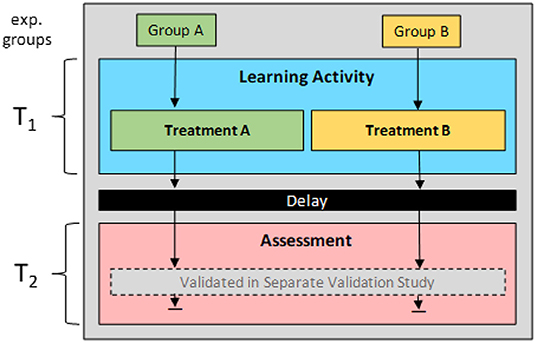
Figure 1. Illustration of the conventional Single Treatment experimental design. Two experimental groups engage in contrasting learning activities, the outcomes of which are assessed in a common, externally validated assessment.
The Double Treatment Experimental Design
The Double Treatment design in its simplest form consists of the following four elements: Two contrasting ontologies of learning, A vs. B; a learning activity; an assessment; and a pair of contrasting experimental treatments. These experimental treatments correspond to the contrasting ontologies A vs. B and are applied to the learning activity as learning treatments A vs. B, and to the assessment as assessment treatments A′ vs. B′. Importantly, the two contrasting learning activities should only differ in the experimental treatments but otherwise remain as identical or homologous as possible, i.e., /A ≈ /B1 in the case of homology, /A = /B in the case of identity. The same holds for the two contrasting assessments, which should also only differ in their corresponding experimental treatments but otherwise remain as identical or homologous as possible, i.e., /A′ ≈ /B′ and /A′ = /B′. We will call these two requirements learning treatment comparability, /A ≈ /B, and assessment treatment comparability, /A′ ≈ /B′, or more generally as comparability of contrasting treatments, because the treatments compared are motivated by the contrasting ontologies A vs. B, respectively. Furthermore, the treatments of the learning activities should be identical or homologous with the corresponding treatments of the assessments: Learning treatment A with the corresponding assessment treatment A′, i.e., A ≈ A′ or A = A′, and learning treatment B with the corresponding assessment treatment B′, i.e., B ≈ B′ or B = B′. We will call this requirement comparability of corresponding treatments, because the compared treatments correspond to the same ontology, either A or B. The experimental treatments A and A′, as well as B and B′ are motivated from a theoretical argument grounded in the contrasting ontologies of learning A or B, respectively. The relationship between the learning treatments and their corresponding assessment treatments, i.e., (A → A′) and (B → B′), also requires grounding in their corresponding ontologies A vs. B. In other words, there needs to be a coherent theoretical rationale grounded in ontology A, which links all ontologically corresponding elements—the ontology, the learning treatment, the assessment treatment, and the relationship between the learning and assessment treatment—with each other, i.e., A → (A → A′) and B → (B → B′). We call this requirement ontological coherence. The learning treatments, A and B, and the assessment treatments, A′ and B′, are then combined independently, leading to a basic 2 × 2 (or more generally n x n) experimental design with four (or n*n) experimental conditions, A → A′, A → B′, B → A′, and B → B′. Figure 2 illustrates this most basic between-subjects Double Treatment design.
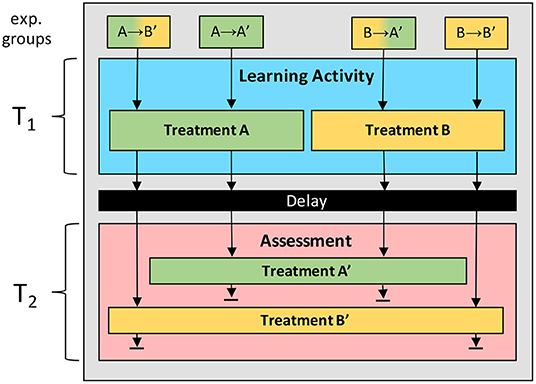
Figure 2. Illustration of the Double Treatment experimental design. Both the learning activity and the assessment are subject to identical or homologous experimental treatments A and A′, as well as B and B′.
Comparability of contrasting treatments, i.e., /A ≈ /B and /A′ ≈ /B′, ensures the reduction or complete removal of confounds extraneous to the ontological contrast, A ≠ B, from the comparison of the contrasting learning activities and assessments. Similarly, comparability of corresponding treatments, i.e., A ≈ A′ and B ≈ B′, ensures the reduction or complete removal of confounds extraneous to the theoretical rationales, A → (A → A′) and B → (B → B′), underlying ontological coherence. Hence, comparability of contrasting treatments ensures that patterns in experimental outcomes may indeed be attributed to the intended epistemic question underlying the ontological contrast, A ≠ B, while comparability of corresponding treatments ensures that the respective ontologies, A and B, are each operationalized in a coherent manner. Clearly, for all four comparability requirements, i.e., /A ≈ /B, /A′ ≈ /B′, A ≈ A′, and B ≈ B′, it will often be impossible or impractical to find actual operationalizations that are identical. In these cases, ontological homology between the respective elements is also sufficient. With ontological homology we mean a coherent theoretical rationale grounded in the corresponding ontology of learning A or B, arguing how and why two non-identical operationalizations in one of the analogous requirement pairs, in fact, reflect identical deep features of ontology A, or respectively, ontology B. In other words, what constitutes homology is entirely informed by the contrasting ontologies in question. This requires grounding in only one ontology, either A or B, for the comparability of corresponding treatments, i.e., A ≈ A′ and B ≈ B′, but conversely the same argument needs to satisfy both contrasting ontologies, A and B, for comparability of contrasting treatments, i.e., /A ≈ /B and /A′ ≈ /B′. Therefore, satisfying identity as best possible is much more important in the contrasting treatment comparisons, i.e., ensuring /A = /B and /A′ = /B′, than in the comparison of corresponding treatments, i.e., ensuring A = A′ and B = B′.
Rationale
In the following three subsections, we provide a theoretical rationale in favor of Double Treatment experimental designs. The rationale consists of three larger arguments. An ontological argument, which is grounded in the premise that learning always consists of two elements, a process and an outcome. The second argument discusses validation as an epistemic practice. The third argument builds on Hans-Jörg Rheinberger's concept of experimental systems as drivers of epistemic progress.
Ontological Argument
While there are many ontologies of what learning is, they all share a basic premise: Learning is a process, which leads to outcomes. Learning outcomes are latent. They become manifest again in the form of processes. Hence, a theory of learning is always a theory of a learning process and processes associated with corresponding learning outcomes: Acquisition and application, memorization and recall, encoding and retrieval, knowledge construction and knowledge transfer, etc. If we intend to study learning, therefore it does not suffice to only investigate the mechanisms of acquisition. We must equally investigate the corresponding mechanisms of subsequent application. Importantly, an ontology of learning, i.e., a coherent theory of what learning is, should provide a coherent account of how its proposed acquisition and application mechanisms interrelate.
This illustrates a fundamental weakness of the conventional Single Treatment experimental design. In Single Treatment designs, assessments are validated a priori and independently of the study proper. Hence, they are validated based on some ontology Z. If we are investigating contrasting ontologies of learning, A ≠ B, then the validation ontology Z can at best correspond with either A or B, but not both. If ontology Z corresponds with ontology A, then we are still evaluating learning outcomes from learning treatment B through an inadequate ontological lens corresponding to Z and A. We are breaking theoretical coherence in the assessment regarding ontology B. In the Double Treatment experimental design however, we are assessing learning outcomes from both learning treatments, A vs. B, equally through both ontological lenses, A and B, with the corresponding assessment treatments, A′ vs. B′. Hence, for both ontologies A and B equally, we are once breaking theoretical coherence, evaluating their outcomes through the lens of the competing ontology, and once maintaining theoretical coherence, evaluating their outcomes through the lens of their own ontology. This guarantees a more coherent, ontologically unbiased, and level playing field in the cross-comparison of the ontological contrast, A ≠ B. Moreover, there is still the possibility to work with externally validated assessments according to ontologies Z and Y. We simply need to provide a convincing argument, why Z corresponds to A, and why Y to B.
Validation as an Epistemic Practice
Assessments are not theoretically agnostic, but instead themselves grounded in—implicit or explicit—ontologies of learning and cognition. Furthermore, validity is not simply a property of an assessment. Validity is a property of an assessment's interpretation (Messick, 1990). Interpretation in turn, does not happen in an empty void, but instead in the context of some ontology of learning. Hence, the common assessment in the conventional Single Treatment design is actually interpreted in two contrasting ways, once within the framework of ontology A, and once within the framework of ontology B. Since validity is a function of assessment interpretation and since assessment interpretation occurs within the context of a given ontology of learning, it directly follows, that an assessment may be adequately valid from the point of view of ontology A, while ontology B would reject this notion. Moreover, if the assessment was validated in an independent study, the externally established validity may be reflective of ontology A or B, or it may be reflective of a completely unrelated ontology Z. Certainly, if the ontological contrast A ≠ B is substantial, we must assume that the common assessment, in fact, likely will not be valid from the point of view of both A and B. In other words, the assumption of an externally validated assessment as fair arbiter between learning outcomes from learning activity A vs. learning activity B, is in fact an illusion based on an incomplete interpretation of validity. Indeed, we can only assume interpretations of assessment outcomes to be valid with respect to ontology Z underlying the independent validation study, rather than ontologies A or B, which are actually of interest in the study. In other words, the breaking of theoretical coherence we discussed in the previous ontological argument, not only precludes a level playing field, it also necessarily compromises assessment validity with regards to at least one of the two ontologies in question. Without validity however, the epistemic value of any corresponding conclusions in the discussion of the ontological contrast A ≠ B is equally compromised. Clearly, we need to find a common ground that enables the evaluation of ontology A vs. B based on empirical findings from assessments A′ and B′. Currently, even in the Double Transfer design, we are still trapped within the ontological lenses A and B, as operationalized in the assessments A′ and B′.
Before continuing our argument, we briefly clarify how we define validity. With Messick (1989) validity is a unitary construct (i.e., there are not different “kinds” of validity), specifically: Validity is the extent to which an assessment measures what it purports to measure (Ruch, 1924). Furthermore, and crucially, validity is an epistemic construct and needs to be argued for (Kane, 2006). This definition of validity is key to finding a common ground between the contrasting ontological lenses: Validation is evidence-based argumentation for the epistemic value of test interpretations.
The Double Treatment design enables argumentation for or against the merits of ontology A and B, as operationalized in the learning treatments A and B, and as seen through the operationalized ontological lenses A′ and B′. Hence, with the Double Treatment design we have a complex set of contrasting modular components from which to develop validity arguments: The learning ontologies A vs. B, the learning treatments A vs. B, and the assessment treatments A′ vs. B′. For example, we can develop a validity argument from the point of view of ontology B for the learning outcomes of the learning treatment A as seen through the lens of assessment A′, or short VB(A′(A)). Correspondingly, the Double Treatment design enables eight different evidence based validity arguments: VA(A′(A)), VA(A′(B)), VA(B′(A)), VA(B′(B)), VB(B′(B)), VB(B′(A)), VB(A′(B)), and VB(A′(A)). Of course, one may for example formulate a validity argument VB(A′(A)) which simply rejects the validity of any conclusions based on assessment A′, because ontology B rejects the notion of assessment A′ being an adequate (i.e., valid) form of assessment of learning outcomes. This however, is not a problem but instead a feature. First, even rejection can be leveraged to formulate concrete, testable hypotheses. Second, even performance data from a suboptimal or “invalid” assessment, constitutes tangible empirical evidence, whose patterns a good theory should be able to explain. Third and crucially, since validation is an epistemic practice, the critical question is not whether some validity argument VB(A′(A)) grounded in ontology B exists. The critical question is how convincing this argument is—especially as compared to the converse validity argument VA(A′(A)) grounded in the contrasting ontology A. In sum, the contrasting and corresponding learning activity and assessment treatments lead to a complex set of empirical data, [A′(A), A′(B), B′(A), B′(B)], which demands explanation through the contrasting ontologies of learning A and B, each in its own integrated validity argument VA and VB. And these integrated validity arguments VA vs. VB each will be more or less convincing. Because the Double Treatment design renders a complex set of ontologically contrasting and corresponding data, this makes it highly likely that the competing validity arguments will differ in their ability to convincingly accommodate the data, which in turn enables the assessment of their respective epistemic value. This is the common ground for assessing ontologies A vs. B we were looking for. The integrated validity arguments VA and VB, and the epistemic arguments EA vs. EB, concerned with the epistemic merit of ontology A vs. ontology B, coalesce into one and the same argument.
Epistemic Argument
Through his exploration of scientific advances in the natural sciences of the 19th and 20th century, particularly Biology, Hans-Jörg Rheinberger (e.g., Rheinberger, 1997) came to argue against epistemic progress (in the natural sciences) being primarily theory driven. Instead, he identified “experimental systems” as the primary drivers of epistemic advances. We can think of experimental systems as the integrated sum of experimental apparatuses, the scientists that operate them, and the ontological constructs together with the social practices through which the scientists attempt to make sense of it all. Rheinberger describes experimental systems as “extremely tricky apparatuses; one has to conceive of them as sites of emergence, as structures which we have thought up for the purpose of capturing the in-imaginable. They are like a spider's web. Something has to be able to entangle itself in them, of which one does not know, what it is […]” (Rheinberger, 2007; translation ours). Crucial to his conception, experimental systems must afford sufficient leeway for the unexpected, “in-imaginable” to be able to occur and at the same time be sufficiently structured for it to register and be interpretable: “[Research] ultimately is about gaining new insights; and that which is truly new, by definition cannot be premeditated […]. The experiment is […] a sort of search machine, but of peculiar structure: It creates things, of which one can only say after the fact that one would have had to have been looking for them.” Adopting Rheinberger's view highlights a new problem of conventional Single Treatment experimental designs: They are inherently theory driven. Operationalizing the contrast between ontologies A and B only in the learning activities but not the assessments results in an unbalanced experimental system, biased toward the ontology of learning Z implemented in the assessments. We must assume that an assessment cannot see beyond its own ontology and correspondingly, the same applies to the larger experimental system these assessments are a part of. By independently operationalizing the ontological contrast manifest in the learning treatments also in the assessments, Double Treatment experimental systems span a much larger, more intricate web in which to entangle the unknown.
Rheinberger's view also has interesting implications on our previous discussion of assessment validity in experimental studies. If the purpose of experimental studies is capturing the in-imaginable (rather than confirming the already imagined), then it is inherently impossible to premeditate the interpretation one “will have had to have intended” and hence it follows, that validity inherently cannot be determined a priori, but only argued for post hoc. Hence, the limitations of Single Treatment designs go much deeper, than the previously discussed faulty premise that validity is the property of an assessment, rather than its interpretation: Scientific investigation, we argue with Rheinberger, demands experimental systems, which enable argumentation for validity post hoc.
Single Treatment experimental designs do not offer much empirical evidence from which to argue for or against validity post hoc, because there is nothing meaningful against which to compare or contrast the assessment. They render a rather limited and incoherent set of empirical data: Learning treatments A vs. B as assessed through the externally validated assessment Z′, i.e., [Z′(A), Z′(B)]. Moreover, if assessments are the lens in our epistemic apparatus, then the epistemic power of our experimental systems is also limited to only what this lens can see, i.e., the ontology Z. An assessment cannot see beyond its own ontology. The Double Treatment design on the other hand generates a much richer, more coherent and contrasting, set of empirical data, i.e., [A′(A), A′(B), B′(A), B′(B)]. And the coherence and contrasts in the data are precisely “located” around the epistemic question of interest, the ontological contrast A ≠ B. This provides a rich basis from which to develop post hoc validity arguments and in turn epistemic arguments relating to the ontologies of learning in question.
Examples
We now illustrate the Double Treatment design by the example of three empirical studies that implemented critical features of this design. We discuss fidelity with the Double Treatment design by highlighting where these studies followed the according design principles and where they did not. We discuss how fidelity to the Double Treatment design was instrumental to the studies' findings, and hence epistemic contributions. We first discuss the scuba diver study on the context dependency of memory by Godden and Baddeley (1975), which is highly illustrative of a number of older foundational studies on memory and recall. This is followed by the Inventing to Prepare for Future Learning study by Schwartz and Martin (2004), which strongly inspired our original thoughts that ultimately led us to the proposition of the Double Treatment design. We conclude this section with a discussion of a recent study of ours on resource-rich assessment, which constitutes our first implementation of the Double Treatment experimental design in an empirical study.
Memorization and Recall While Scuba Diving
In their study on the context dependency of memory, Godden and Baddeley (1975) asked subjects to memorize and recall lists of words in one of two natural environments, either underwater or on dry land. The study's goal was to validate the assumption that “what is learnt in a given environment is best recalled in that environment.” Participants memorized lists of 36 unrelated words presented to them in the form of audio recordings, either under water or on land. Subsequently, they were instructed to recall as many words as possible, again either under water or on land. This resulted in a 2 × 2 between-subjects experimental design analogous to the Double Treatment design.
In the underwater condition, subjects were sitting on the seafloor in full scuba diving gear, 20 ft. underwater on the coast near Oban, Scotland. Instructions, as well as the word lists for the memorization task were presented to the subjects as audio recordings delivered through Diver Underwater Communication sets. In the subsequent recall tasks, subjects recorded their responses in pencil on formica boards. In the dry land conditions, subjects did exactly the same as in the underwater conditions, in full scuba gear, at the same site, but sitting at the edge of the water on dry land, “masks tipped back, breathing tubes removed, and receivers in place.”
The study findings confirmed the hypothesis: What is learnt underwater is best recalled underwater and what is learnt on land, on land. While there were no significant main effects of either the learning or the recall environment on recall performance, the interaction between the learning and recall environment was highly significant. Lists learnt underwater were better recalled underwater than on land, and vice-versa, lists learnt on land were better recalled on land than underwater. Moreover, lists learnt underwater were better recalled under water than were lists learnt on land, and vice-versa, lists learnt on land were better recalled on land than were lists learnt underwater. Hence, the authors concluded, “[r]ecall is better if the environment of original learning is reinstated.”
The scuba diver study relates to the Double Treatment design in several ways. First, more as a side note, its findings on the contextual nature of recall are empirical evidence in support of our ontological argument, that in the research of learning, we must pay equal attention to the process of learning and the processes associated with its outcome—in this case memorization and recall. Second and more importantly, the study illustrates how identical experimental treatments in both the learning activity and the assessment can be possible, even in messy real world settings. In this case, the experimental treatments are “underwater” vs. “dry land” and their operationalization as learning treatment and as assessment treatment was (almost) identical. The only arguable difference between the learning and assessment treatments lies in the observation that listening underwater differs from listening on dry land in a different manner, than writing underwater differs from writing on dry land. These arguable differences aside, the study ensures identity in the comparability of corresponding treatments. The underwater treatment is identical in both the learning activity and the assessment, i.e., A = A′, and the dry land treatment is identical in both the learning activity and the assessment, i.e., B = B′. Furthermore, the two learning activities, as well as the two assessments are also (near) identical in all features, save for the experimental treatments proper. Hence, the study also ensures identity in the comparability of contrasting treatments. The learning activities are identical, except that one took place underwater and the other on dry land, i.e., /A = /B, and the same is true for the assessments, i.e., /A′ = /B′. Since the study ensures identity in comparability both of contrasting and of corresponding treatments, the study has eliminated potential confounds to the operationalization of its epistemic question. Hence, any patterns in the empirical data may safely be attributed to the learning and assessment treatments proper, i.e., A, A′, B, and B′. This is particularly noteworthy, since the study was conducted under messy, real world conditions outside the laboratory. Finally, the study beautifully illustrates the inherent epistemic power of Double Treatment designs. There is no need for the study to externally establish, whether memorization and recall of lists of words render valid assessments of learning. The results speak for themselves. In fact, the study results themselves validate the assessment method: Whatever kind of learning the assessment measures, it is difficult to argue that this learning is not context dependent, and hence, it is difficult to argue that this assessment method is not valid for assessing the context dependency of learning. The validity argument for the assessments, V, and the epistemic argument relating to the study itself, E, originate from one and the same argument.
This brings us to the sole feature of this study, which diverges from our proposition of the Double Treatment design. The study is not interested in a direct comparison of learning underwater with learning on land. In other words, the experimental treatments, A, A′, B, and B′, are not motivated by underlying ontologies “learning on land,” A, vs. “learning underwater,” B. Instead the study is interested in competing ontologies of learning which, statistically speaking, relate to the interaction between the treatments, i.e., learning and recall as context dependent—there is an interaction—vs. learning and recall as context independent—there is no interaction. This indicates that the Double Treatment design has applicability beyond our original proposition of operationalizing an ontological contrast A ≠ B through experimental treatments that reflect direct ontological coherence with one of either ontology A vs. B, i.e., A → (A → A′) and B → (B → B′). It does however leave the question unanswered, whether the original proposition of operationalizing ontological contrasts A ≠ B through ontologically coherent operationalizations has any merit. This of course does not diminish the original study in any way, whose elegantly simple design we find uniquely beautiful.
Preparation for Future Learning
In their seminal study “Inventing to Prepare for Future Learning” Schwartz and Martin (2004) investigated whether invention-based learning activities would lead to superior learning outcomes as compared to more conventional “tell-and-practice” learning activities. The core methodological element of the study consisted of what the authors called an assessment experiment where they combined two between-subjects learning conditions, “invention” vs. “tell-and-practice,” with two between-subjects variations of a target transfer assessment task. They called these two transfer task variations the “standard transfer paradigm” vs. the “double transfer paradigm.” In the learning activities, students studied mean deviation (“Topic X”). Before the assessment experiment proper, all students engaged in two preparatory “inventing to prepare for learning” (IPL) instructional cycles. Students were first presented with invention tasks emphasizing central tendency followed by a brief lecture on the mean (first IPL cycle), and then tasks emphasizing variability followed by a short presentation and discussion of the formula for mean deviation by the instructor (second IPL cycle). The formula used is as follows:
In the tell-and-practice learning condition, the teacher then introduced a procedure for grading on a curve, which students subsequently practiced on new datasets. In contrast, in the invention learning condition, students were instructed to invent ways in which to compare the merit of a new world record in one sport (long jump) vs. another (pole vault) based on a list of prior world records in each sport. The students in the invention condition received neither an introduction to a solution procedure for the problem, nor feedback on their inventions, nor a canonical solution. The authors' theoretical rationale in favor of the invention learning condition, in short, was that inventive production would “help […] let go of old interpretations and see new structures,” thus facilitating the construction of new understanding and also future learning (for example future learning from direct instruction).
In the assessment following the learning activities, students received a target transfer problem, which was “structurally similar to Topic X,” mean deviation, but had different surface features. The target transfer problem concerned standardized scores, i.e.,:
Students in both assessment conditions worked on identical target transfer problems. However, students in the double transfer condition received a learning resource embedded in their assessment. The resource was presented in the form of a worked example on standardized scores in the guise of another assessment task. The authors argued that this double transfer task was uniquely suitable for assessing students' “preparedness for learning”: First, students need to “transfer in” what they have learned in the learning activity to make sense of the worked example, and subsequently they need to “transfer out” what they have learned in the worked example in order to apply it successfully to the target transfer problem. In contrast, the students in the standard transfer assessment condition did not receive the worked example task in their assessment. The authors called this “sequestered” problem solving, because students did not receive an opportunity to learn. Based on their theoretical rationale for invention-based learning, the authors then proposed the following two core hypotheses. First, the invention-based learning activity uniquely prepares students for successful learning from the worked example in the assessment, whereas the tell-and-practice learning activity does not. Second, the assessment of preparedness for learning with the double transfer paradigm is more suitable for comparing differences in the learning outcomes from the invention-based learning activity vs. the tell-and-practice learning activity, than is the assessment of sequestered problem solving with the standard transfer paradigm. The study results confirmed both hypotheses: The invention-based learning activity led to superior performance in the target transfer problem, but only for students in the double transfer assessment condition with the embedded worked example. In the standard transfer assessment condition, there was no performance difference between the two learning activity experimental groups.
The assessment experiment of the Preparation for Future Learning study implements most critical features of the Double Transfer design. The authors proposed an ontology of learning A: Inventive production as a facilitator of (future) constructivist learning. They developed an operationalization of ontology A as learning treatment A—the IPS instructional cycles—motivated by a theoretical argument informed by ontology A—invention activities facilitate future learning because they facilitate appropriate evaluation of both prior knowledge and novel information in relation to the novel learning topic. Finally, they developed a suitable assessment method A′—the assessment of “preparedness for learning” with the double transfer paradigm—on the basis of a theoretical rationale informed by ontology A and treatment A. “[S]tudents need to transfer what they learned from the instructional method to learn from the resource [i.e., the worked example], and they need to transfer what they learned from the resource to solve the target problem.” This grounds the assessment treatment A′ in the ontology A. The rationale furthermore continues with the proposition that the invention-based learning activities create a unique preparedness for this (future) learning from the resource operationalized in the assessment treatment A′. This links the learning treatment A with the assessment treatment A′, i.e., (A → A′). Clearly, the invention-based learning treatment A and the corresponding double transfer assessment treatment A′ are not identical in any meaningful sense of the word. They are however ontologically homologous, ensuring comparability, i.e., A ≈ A′, because they are both motivated by a coherent theoretical rationale grounded in the common theory of learning A: Inventive production as a facilitator of future learning. Hence, the authors provide a theoretical rationale which coherently links ontology A, learning treatment A, assessment treatment A′, and the relationship between A and A′, (A → A′), with each other, i.e., A → (A → A′), ensuring ontological coherence. Correspondingly, the authors propose a contrasting ontology of learning B: Constructivist learning as disparate from preparatory (inventive) production activities. They present a corresponding learning treatment B: Tell-and-practice learning activities. And finally, an assessment treatment B′: Sequestered problem solving in standard transfer tasks. Furthermore, the authors used homologous learning activities for the two learning treatments—grading on a curve and world records—satisfying learning treatment comparability, i.e., /A ≈ /B. Finally, in the two assessment conditions students worked on identical transfer tasks, save for the treatment proper, hence we also have assessment treatment comparability, i.e., /A′ = /B′.
As in the scuba diver study, the results mostly speak for themselves. Notably, there is no apparent need for the assessments to have been a priori validated externally. Indeed, the authors motivated, designed, and validated a novel assessment method all within the first iteration of their assessment experiment. The standard vs. double transfer experimental design in itself renders sufficient evidence to validate the assessments a posteriori. If the double transfer paradigm were not suitable for assessing preparedness for learning, why then do we only see performance differences between students from the two learning conditions when they receive an assessment embedded learning resource? Conversely, if the invention activities did not facilitate preparedness for future learning, why then are only the students from this experimental condition able to profit from the embedded learning resource, while the students in the tell-and-practice condition performed equally well (or poorly), regardless of whether the worked example was present or not? This again illustrates the epistemic power of the Double Treatment design. The data at once enable the formulation of validity arguments V with regards to the assessments, as well as an epistemic argument E with regards to the comparison of the contrasting learning ontologies A ≠ B. More specifically, ontology A, inventive production as a facilitator of future learning, is able to provide a coherent account of the observed data [A′(A), A′(B), B′(A), B′(B)] in the form of an integrated validity argument VA, whereas the contrasting ontology B does not. We observe that ontological coherence through the strong theoretical rationale linking ontology A with its corresponding experimental treatments, A → (A → A′), is crucial to the formulation of the integrated validity argument VA. The integrated validity argument VA validates both the assessment A′ and the ontology A, because it constitutes a convincing and ontologically coherent integrated account of the entire observed data [A′(A), A′(B), B′(A), B′(B)]. In other words, VA is at once an empirically grounded validity argument for A′ and an empirically validated epistemic argument EA for ontology A.
We have illustrated how the Inventing to Prepare for Future Learning assessment experiment implements the concerns from both our ontological argument for the Double Treatment design, as well as from our discussion of validation as an epistemic practice. The Preparation for Future Learning assessment experiment however, arguably falls short of implementing an epistemic web suitable for the capture of the in-imaginable, in Rheinberger's sense. In fact, the study is fundamentally hypothesis driven. The entire design of the study intends to confirm (or alternatively refute) the proposed hypotheses. It is not at all concerned with alternate hypotheses, should the original ones fail to hold. In this sense, it constitutes an experimental system designed for the purpose of substantiating the already imagined, rather than capturing the not yet imaginable. Indeed, the authors successfully and elegantly confirm their hypotheses, but beyond that, the study does not generate many new questions or epistemic insights. Since this presumably was never the intention of the original study, there is nothing inherently wrong with this. Arguably, the Learning Sciences do not suffer from a paucity in theories and an overabundance of dependable empirical results from controlled experiments. It is however instructive to take a closer look at the assessment experiment, in order to determine which changes to its design might enhance its power as an experimental system that drives epistemic progress toward the not yet imagined. We have argued that the authors ensured ontological coherence through a strong theoretical rationale linking ontology A with its operationalizations, i.e., A → (A → A′). Arguably however, the same cannot be said for the converse elements of the study relating to ontology B. Instead, ontology B, its learning treatment B, and its assessment treatment B′ seem to be more strongly motivated by not being their ontologically contrasting counterparts, A, A, and A′. That is, instead of a coherent rationale linking ontology B with its operationalizations, i.e., B → (B → B′), we actually have ontology B, which is primarily motivated by not being ontology A, i.e., B := !(A), learning treatment B as not learning treatment A, i.e., B := !(A), and assessment treatment B′ as not assessment treatment A′, i.e., B′ := !(A′). Indeed, if we look at how the authors define B, B, and B′, they are actually either ontological or methodological precursors of A, A, and A′. Invention-based learning A is an extension of its ontological precursor active constructivist learning B. Tell-and-practice instruction B is simply an established, orthodox form of instruction. The double transfer paradigm A′, again, is an extension of its precursor, the standard transfer paradigm B′. Hence, there is in fact no equally coherent ontology B present in the study. Instead, we only have one coherent ontology present in the study, A, together with its negation, !(A). We have argued that experimental systems cannot see beyond their own ontology. Arguably, this is precisely what we see in the Preparation for Future Learning assessment experiment, due to its grounding in only a single coherent ontology of learning.
Resource-Rich Assessment
In our study on resource-rich vs. resource-poor assessment in computer science, we investigated whether and how the availability or absence of a disciplinary tool—a programming environment with a fully functional compiler—would impact the validity of corresponding assessments of learning. We have recently published select results of the study in the Proceedings of the 41st Annual Meeting of the Cognitive Science Society (Halbherr et al., 2019). A more detailed and extensive analysis of the study results is currently in writing. The study investigated two core questions. First, the pragmatic question whether “resource-rich” (RR) or “resource-poor” (Rp) assessments are more suitable for the valid assessment of learning outcomes. Second, the epistemic question, whether a rigidly “situated” ontology of cognition and learning vs. a rigidly “mindbased” ontology of cognition and learning would be more suitable for explaining the learning and assessment practices under investigation. In the study, students participated in a learning activity in the form of a self-study tutorial, either in a RR condition or in a Rp condition. Subsequently they performed either a RR assessment or a Rp assessment. All assessments were scored manually with scoring rubrics that emphasized “conceptual correctness.” The assessment consisted of three tasks, a direct replication of one of the tutorial tasks, a standard transfer task, and a double transfer task with an embedded information resource. In the RR conditions students worked on the programming tasks in a web-based programming environment with a fully functional compiler. In the Rp conditions students worked on the identical programming tasks in the identical web-based programming environment, except that we had deactivated the compiler. We chose the availability of the compiler as the key resource for operationalizing the RR vs. Rp conditions, because the compiler is the essential element for sustaining the practice of programming: It generates running programs out of written code. We then defined the mindbased ontology as follows: Cognition and learning are manifestly located in the “mind,” with mindbased processes mediating the relationship between stimuli and response. Fundamentally, the mindbased ontology regards mindbased processing (i.e., cognition) as dissociable from environmental interaction. The situated ontology on the other hand defines cognition and learning as situated action that is emergent from the dynamical interaction between the cognitive agent and the environment with which he/she interacts. Fundamentally, the situated ontology regards cognitive action as indissociable from corresponding environmental interaction. Since programming is a resource-mediated cognitive practice—the actual practice of programming requires working with a programming environment with a fully functional compiler—the situated vs. mindbased ontologies led to contrasting hypotheses regarding expected experimental results. Because it assumes indissociability, the situated perspective would predict that the RR learning activity leads to superior learning outcomes, including transfer tasks intended to assess “deep” conceptual learning, and that the RR assessment is particularly sensitive to this difference, again including the transfer tasks. The mindbased ontology on the other hand, rejecting the indissociability assumption, does not assume superior learning in the RR learning activity as long as students in the Rp learning activity also receive adequate feedback. More importantly, the mindbased ontology would expect higher sensitivity of the Rp assessment, particularly in the transfer tasks, because the Rp assessment removes construct-irrelevant variance related to “superficial” details in coordinating with the resource and unburdens the cognitive apparatus from coordinating with the resource.
The study results confirmed neither the mindbased nor the situated ontology. Instead, the results confirmed some hypotheses of both ontologies while rejecting others. Regarding the pragmatic question of assessment validity, the results supported the conclusion that both RR and Rp assessments are valid methods for differentiating between the RR and Rp learning conditions. The core findings are the following. There was a robust learning facilitation effect for the RR learning activity. Across all tasks and experimental conditions, the RR learning activity led to superior outcomes. On the other hand, there was a robust performance facilitation effect for the Rp assessment. Again, across all tasks and experimental conditions, students in the Rp assessment outperformed students in the RR assessments. Furthermore, the effect size of the performance difference between the students from the RR learning activity and the Rp learning activity was larger in the Rp assessment than in the RR assessment in the transfer tasks (but not in the direct replication task). At the same time, the effect size of the performance difference between the students from the RR learning activity and the Rp learning activity for both assessment conditions was larger in the transfer tasks vs. the direct replication task. In other words, the Rp assessment vs. the RR assessment, as well as independently the transfer tasks vs. the direct replication task, were more sensitive to the greater learning facilitation of the RR learning activity, and sensitivity to this RR learning facilitation was highest in the Rp transfer tasks. Since both the RR and the Rp assessment successfully differentiated between the RR and the Rp learning activity, and since furthermore, the scores from both assessments were in principle coherent with each other, we concluded that both the RR and the Rp assessment render in principal valid assessments of learning. Since, the students from RR learning activity consistently had the largest performance difference to the students from the Rp learning activity in the transfer tasks, and since furthermore, this performance difference was largest in the Rp transfer tasks, we concluded that RR learning in particular facilitates deep conceptual learning, rather than the memorization of “superficial” resource-specific details. Since RR learning was strongly associated with deep conceptual learning, we rejected the mindbased ontology. Since Rp assessment facilitated performance—especially since it facilitated performance also for the students from the RR learning activity, which had no practice in dealing with the absence of the compiler—we also rejected the situated ontology. Particularly intriguing was the pattern of the Rp assessment transfer tasks showing the largest performance difference between the RR and the Rp learners. How could the absence of the very tool that so robustly and consistently facilitated learning—the availability of a fully functional compiler—moreover in the more difficult transfer tasks, which require some form of novel learning, lead to the greatest performance facilitation for the RR learners as compared to the performance of the Rp learners? We concluded that the best way to make sense of this pattern was that we saw a form of “resource internalization,” which strongly facilitated deep conceptual learning. In other words, on the one hand resource-interaction appeared uniquely instrumental to this form of conceptual learning. On the other, the Rp assessment was most sensitive to this resource-mediated conceptual learning. Furthermore, we observed that the RR assessment corresponded more closely with the actual disciplinary practice of programming than the Rp assessment (i.e., it has higher “ecological validity”). Finally, students performed worse in the RR assessment than in the Rp assessment, meaning that they clearly had to demonstrate “more” competency in order to achieve similar scores. Thus, we concluded that the RR assessment rendered results that reflected a more exhaustive and complete representation of the intended measurement construct, programming competency related to the topics covered in the tutorial. Conversely, we concluded, that the Rp assessment results underrepresented the competency construct as it relates to the resource-mediated practice, while being differentially more sensitive to a subconstruct, namely resource-mediated conceptual learning.
Our study on resource-rich assessment illustrates how we envision the implementation of the Double Treatment design. At the outset, we were interested in an epistemic comparison of the mindbased ontology of learning A vs. the situated ontology of learning B. We were also interested in contrasting teaching, learning, and assessment practices motivated by these two ontologies, particularly Rp assessment A′ vs. RR assessment B′. The experimental learning and assessment treatments A and A′ and conversely B and B′ in this study are identical. Hence comparability of corresponding treatments is satisfied, i.e., A = A′ and B = B′. Furthermore, the contrasting learning conditions A vs. B, as well as the contrasting assessment conditions A′ vs. B′, are identical, save for the experimental treatments proper. Hence, comparability of contrasting treatments is also satisfied, i.e., /A = /B and /A′ = /B′. Therefore, there is no confound to the ontological contrast A ≠ B and any patterns in the data [A′(A), A′(B), B′(A), B′(B)] can safely be attributed to the experimental treatments alone. Furthermore, there is a coherent theoretical rationale tying both ontologies together with their respective operationalizations, i.e., A → (A → A′) and B → (B → B′), ensuring ontological coherence. As in previously discussed studies, we were hence able to convincingly validate the assessments post hoc, solely through argumentation from the study data proper. In contrast to the previous study however, our study design was not informed by strong a priori hypotheses A and B, which we intended to confirm. Instead, our design was motivated by creating a strong contrast A ≠ B between two currently debated, alternative ontologies of learning and cognition. While our rigid interpretation of these alternate ontologies led to the (perhaps unsurprising) rejection of both, the strong theoretical contrast between the two arguably generated sufficiently salient data patterns in the study's experimental outcomes, to facilitate delimiting the epistemic space “in between” ontology A and ontology B through post hoc sense-making and hypothesis generation. Hence, our contrasting original ontologies A and B functioned like two foundational threads between which we span our epistemic web, covering uncharted space, from which something in-imaginable might entangle itself. Indeed, we seem to have captured an unpremeditated ontology C, “learning as resource internalization.” Certainly, we did not even remotely anticipate our quite surprising empirical results.
Conclusion
We have problematized fundamental shortcomings of conventional Single Treatment experimental designs. By applying experimental treatments only to learning activities, they divorce the assessments from the original research question. Accordingly, we have argued Single Treatment designs constitute incomplete operationalizations of ontologies of learning. We questioned the validity of exclusive reliance on externally “validated” tests in the context of epistemic investigations into ontologies of learning. Last not least, we discussed why Single Treatment designs constitute experimental systems, that cannot see beyond their a priori hypotheses.
We have argued a detailed theoretical rationale in favor of Double Treatment experimental designs and we have illustrated this rationale in three empirical study examples. Double Treatment designs take into account the nature of learning as a process with an outcome, by operationalizing experimental treatments in both the learning activities and the assessments. They regard validation as an argument-based epistemic practice, rather than an externally certifiable, stable property of a test. Finally, they regard scientific research as an epistemic practice, which should strive beyond confirming the imagined, toward also facilitating the discovery of the previously in-imaginable.
We propose that studies implementing the Double Treatment design will both necessitate and facilitate a more thorough discussion of the ontologies of learning in which both learning interventions and assessments are grounded. As such, they are a design suitable for both the research of learning and the validation of assessments. Indeed, one of our core arguments in this article is that the two are an epistemically inseparable pair. We expect that this intimate interlinking of learning and assessment can help bootstrap both intervention and validation studies toward an epistemically more profound penetration into foundational questions of the Learning Sciences. We furthermore propose that adopting Double Treatment designs will require a reconceptualization of assessments as (learner) activities rather than static task objects. This may help rebalance the focus from a current bias for assessment data, toward more equal attention also for the task-activity space of assessments. Finally, we see Double Treatment designs as a call for an approach to assessment validation that is more strongly inspired by experimental research methodologies.
Double Treatment designs promise great methodological flexibility. They are compatible with both quantitative and qualitative research methods, with behavioral, cognitivist, situated, socio-cultural, or complexity science ontologies of learning and cognition. While we have discussed the Double Treatment design in the context of between-subjects designs, it can equally accommodate within-subjects designs. As we have illustrated with the three study examples, the Double Treatment design is also well-suited for researching learning outside the safe confines of laboratories, in in-class settings, or more generally for the study of learning in the wild. We believe it can complement and enhance research practices grounded in Single Treatment designs in productive ways. Notably, the Double Treatment design offers the promise of closing the gap between hypothesis testing and hypothesis generation. Indeed, the ability of Double Treatment studies to generate unpremeditated epistemic and validity arguments out of their own data, promises findings that are both more robust and more surprising. A bold claim. It is time to build new webs and find out.
Data Availability Statement
All datasets generated for this study are included in the article/supplementary material or directly referenced in the article.
Author Contributions
TH devised the original ideas presented and authored the manuscript. MK consulted the project and reviewed the manuscript.
Funding
This work was supported by ETH resources.
Conflict of Interest
The authors declare that the research was conducted in the absence of any commercial or financial relationships that could be construed as a potential conflict of interest.
Footnote
1. ^Here the notation “/” is intended to express the exclusion of “A” or respectively “B”. The notation “/A ≈ /B” then reads as: “[everything in the learning activity] except learning treatment A corresponds to [everything in the other learning activity] except learning treatment B.”
References
Godden, D. R., and Baddeley, A. D. (1975). Context-dependent memory in two natural environments: on land and underwater. Br. J. Psychol. 66, 325–331. doi: 10.1111/j.2044-8295.1975.tb01468.x
Halbherr, T., Lehner, H., and Kapur, M. (2019). “Resource-rich versus resource-poor assessment in introductory computer science and its implications on models of cognition: an in-class experimental study,” in Proceedings of the 41st Annual Conference of the Cognitive Science Society, eds A. K. Goel, C. M. Seifert, and C. Freksa (Montreal, QC: Cognitive Science Society), 1873–1879.
Kane, M. T. (2006). Validation in Educational Measurement. Washington, DC: American Council on Education, (S. 17–64).
Messick, S. (1989). Validity in Educational Measurement. Washington, DC: American Council on Education.
Messick, S. (1990). Validity of test interpretation and use. ETS Res. Rep. Ser. 1990, 1487–1495. doi: 10.1002/j.2333-8504.1990.tb01343.x
Rheinberger, H.-J. (1997). Toward a History of Epistemic Things: Synthesizing Proteins in the Test Tube. Stanford, CA: Stanford University Press.
Rheinberger, H.-J. (2007). Man weiss nicht genau, was man nicht weiss. Über die Kunst, das Unbekannte zu erforschen. Neue Zürcher Zeitung. Available online at: https://www.nzz.ch/articleELG88-1.354487
Keywords: double treatment, learning science, assessment, validity, validation, methods, experimental design
Citation: Halbherr T and Kapur M (2020) Validation Reconceived: The Double Treatment Experimental Design. Front. Educ. 4:156. doi: 10.3389/feduc.2019.00156
Received: 31 August 2019; Accepted: 17 December 2019;
Published: 22 January 2020.
Edited by:
Philipp Sonnleitner, University of Luxembourg, LuxembourgReviewed by:
April Lynne Zenisky, University of Massachusetts Amherst, United StatesRosemary Hipkins, New Zealand Council for Educational Research, New Zealand
Copyright © 2020 Halbherr and Kapur. This is an open-access article distributed under the terms of the Creative Commons Attribution License (CC BY). The use, distribution or reproduction in other forums is permitted, provided the original author(s) and the copyright owner(s) are credited and that the original publication in this journal is cited, in accordance with accepted academic practice. No use, distribution or reproduction is permitted which does not comply with these terms.
*Correspondence: Tobias Halbherr, dG9iaWFzLmhhbGJoZXJyQGdlc3MuZXRoei5jaA==