- Medical Physics and Digital Innovation Laboratory, School of Medicine, Faculty of Health Sciences, Aristotle University of Thessaloniki, Thessaloniki, Greece
Introduction: Children’s behavior is indicative of adaptation to early-age development. Adaptive difficulties linked to behavioral difficulties are frequent among school-aged children having a multi-level impact. Five key approaches have been used therapeutically while systematic behavior monitoring has been used to evaluate the efficacy of different interventions. The inherent barriers of traditional monitoring tools have led to the need to design and implement new approaches.
Methods: Four case study series (S1–S4) focused on vulnerable populations are described. Eight participants with different medical backgrounds underwent an intervention, either cognitive training (BrainHQ) (seven out of eight) or operant conditioning strategies. Targeted behaviors were monitored in six out of eight participants using the WHAAM application which could also measure the effectiveness of the intervention applied, while emotional fluctuations were evaluated in two out of eight participants using AffectLecture. Moreover, a 5th pilot study (S5) is included focusing on the early detection and management of ADHD using a serious game-based platform.
Results: In S1, monitoring highlighted that BrainHQ training resulted in positive changes in all but one of the behaviors in Case 1. Studies that exploited the AffectLecture application indicated that emotional state was improved after training, and it was positively correlated with performance in cognitive exercises. The performance in cognitive training was positively correlated with the emotional state before training in S2 but not in S3. A positive correlation between the change in emotional state and performance was mentioned only in S3. Educational and social benefits as well as a positive correlation between the performance and the length of training were reported in both studies. Operant conditioning strategies in S4 resulted in positive behavioral effects in most participants. Preliminary analysis of S5 indicated that the platform has a considerable capacity to discriminate users based on their in-game patterns as ADHD or non-ADHD.
Discussion: The mixture of studies presented in this article incorporates different technological solutions for monitoring or intervening in developmental disorders and behavioral difficulties. Despite the inherent inhomogeneity of such an endeavor, they all highlight the positive prospects of using technology to enhance the educational arsenal of facing behavioral and learning difficulties.
1. Introduction
Children’s behavior is indicative of adaptation to early-age development (Martineli et al., 2018). Family dynamics, among other social, biological, and environmental factors (Sutherland and Oswald, 2005), could act aggravatingly or protectively to the child’s development (Walsh, 2016) affecting the adaptation in the developmental stage. Adaptive difficulties linked to internalizing or externalizing behavioral difficulties (BDs) were found to be frequent among school-aged children (Pizato et al., 2014; Martineli et al., 2018). Behavioral difficulties have an impact on children with BD, teachers, as well as student–teacher interactions. At the individual level, BDs have been associated with weak academic achievement and higher school absenteeism (Mekonnen et al., 2020). Apart from learning, BD affects mood, family and home life, relationships, and leisure time activities (Simpson et al., 2009). As the domains commonly affected by BD represent overlapping aspects of life, children with BD usually experience difficulties in more than one domain (Simpson et al., 2009). Recently, Caslin (2019) conducted a pupil voice study describing that pupils are too aware of the stigma that comes with the label attached to them as a part of their identity. Furthermore, they internalized the labels given by others to explain their deviance from others (Caslin, 2019).
Teaching children with BD has been considered challenging (Scanlon and Barnes-Holmes, 2013), as these students are more likely to be involved in school misdemeanors (Lassen et al., 2006) and be subjected to exclusionary or restrictive practices (Kauffman, 1999) leading to negative attitudes toward students with BD (Scanlon and Barnes-Holmes, 2013). More precisely, teachers described their direct interactions with this target group as aversive and tense (Sutherland et al., 2008) leading to higher rates of burnout and depression (Hastings and Bham, 2003), as well as worsening feelings of inadequacy (Scanlon and Barnes-Holmes, 2013). Emotional labor, consisting of surface acting, deep acting, and suppression (Glomb and Tews, 2004; Lee and Brotheridge, 2011; Näring et al., 2012), seems to be involved in the teaching role (Kinman et al., 2011). Teachers experiencing surface acting seem to be vulnerable to burnout (Brackett et al., 2010) or depersonalize their students affecting negatively pupils with BD (Rae et al., 2017). On the other hand, deep acting could allow educators to establish effective relationships with their students, cultivate a relaxing environment, and manage their difficulties (Brackett et al., 2010). Positive teacher–student relationships for learning and behavior may be promoted by teachers who are emotionally nurtured in the acquisition and transfer of these skills (Koundourou, 2012; Rae, 2012). A negative relationship with teachers has been associated with a long-term exacerbation of behavioral problems (Myers and Pianta, 2008). Teachers who work in schools with high levels of suspensions are more likely to bully their students (Twemlow and Fonagy, 2005) possibly contributing to the etiology of the student’s behavioral problems (Cooper, 2011). On the other hand, teachers demonstrating emotional warmth contribute to the improvement of the student’s wellbeing expressed not only in school engagement but also in academic achievements (Cooper, 2011). Teachers’ emotional warmth seemed to promote healthier non-conflictual relationships in students expressing internalizing and externalizing behaviors (Buyse et al., 2008). Similarly, LaRusso et al. (2008) mentioned that supportive teachers used to report lower drug use, higher social inclusion, better school climate, and lower depressive symptoms than teachers lacking these qualities.
Cooper (2011) summarized five key approaches that have been used therapeutically in students with BD including the psychodynamic, behavioral, humanistic, cognitive behavioral, and systemic approach. Behavior monitoring is used to evaluate the efficacy of different intervention strategies and design or modify a plan for behavioral change (Strawhun et al., 2013). However, traditional monitoring tools commonly rely on pen-and-paper methods (Gualtieri and Johnson, 2005; Pandria et al., 2015) using expert observations and/or conducting surveys involving participants who interact with each other (Davila-Montero et al., 2021). Inherent obstacles of those methods include data loss, subjectivity bias, difficulty in synchronous monitoring at different settings, real-time recognition, and interpretation of behavior (Davila-Montero et al., 2021; Pandria et al., 2022). For addressing the limitations of existing approaches, we have designed and implemented a bundle of tools over the past few years, namely, the WHAAM application (Spachos et al., 2014), the ADHD360 (Pandria et al., 2022) platform, and the AffectLecture application (Siouli et al., 2017, 2020), in addition to exploiting the commercially available platform BrainHQ (Romanopoulou et al., 2017; Anagnostopoulou et al., 2021; Romanopoulou et al., 2021) setting different goals and targeting different populations. The WHAAM application enables the digital monitoring of Attention Deficit Hyperactivity Disorder (ADHD) behavior as well as the planning of an intervention and the statistical evaluation of its effectiveness (Spachos et al., 2014; Merlo et al., 2018) while the ADHD360 stands for an integrated platform that includes a serious game for early detection of ADHD characteristics and a mobile application for behavior monitoring. BrainHQ is a specialized, commercial software for personalized and adaptable game-based cognitive training (Romanopoulou et al., 2017) while the AffectLecture is a hybrid mobile application that assists emotional state monitoring (Siouli et al., 2017, 2020). The current manuscript aims to provide a comprehensive description of a case study series collective in which the aforementioned technologies were used either as intervention tools or as behavioral monitoring tools for vulnerable populations experiencing BD.
2. Materials and methods
2.1. Subjects and cases
Four case study series (S1–S4) were designed and implemented to focus on vulnerable populations such as participants with intellectual difficulties, ADHD combined with obsessive–compulsive disorder and oppositional defiant disorder, Williams syndrome, Simpson-Golabi-Behmel syndrome, and special learning difficulties combined with visuomotor deficits and ADHD. In total, eight participants with different medical backgrounds underwent an intervention, either cognitive training using BrainHQ software (seven out of eight participants) or operant conditioning learning strategies. Targeted behaviors were monitored in six out of eight participants using the WHAAM application while the emotional fluctuations were evaluated in two out of eight participants using the AffectLecture application.
Moreover, 43 participants were recruited to take part in the pilot study of the ADHD360 project (Pandria et al., 2022) (S5); 18 participants were diagnosed with ADHD while 25 participants were healthy controls. The ADHD360 platform and, in particular, its serious game “Pizza on Time” was explored as a tool for the early detection of ADHD characteristics and management of ADHD behavior. The mobile application ADHD360 served as the behavior monitoring tool.
The whole series of studies was approved by the Bioethics Committee of the Medical School of the Aristotle University of Thessaloniki and was conducted per the Helsinki Declaration of Human Rights. Legal guardians of both underage and adult participants signed written informed consent before their enrollment in the study.
2.2. Technology material/software and apps
2.2.1. WHAAM
WHAAM stands for Web Application for ADHD Monitoring and was developed during a project funded by the European Commission in the LLP Transversal Programme KA3—ICT Multilateral Projects (Alves et al., 2013). The main objective of the WHAAM application was to plan and facilitate digital monitoring of Attention Deficit Hyperactivity Disorder (ADHD) behavior in a SMART (Specific, Measurable, Attainable, Realistic, and Timely) way (Spachos et al., 2014; Merlo et al., 2018) involving all members of the care network of a child or young adult diagnosed with ADHD as well as provide statistical evaluation of intervention effectiveness. The aforementioned objective was fulfilled by the WHAAM application in its two main versions/components, namely, the Web and the mobile.
The Web component of the WHAAM application serves functional behavioral assessment (FBA), creation of a virtual network for data sharing, data collection, as well as planning and statistical evaluation of the intervention designed. The Web WHAAM application may be accessible through any browser, and it consists of two areas, the public and the private ones. The public area provides access to the theoretical principles of the app development and the private area through a registration procedure. The private area allows personal, school, and medical data collection, management of the user’s network by inviting people to participate, targeted behavior definition, planning of behavior monitoring, and evaluation of the intervention effectiveness through charts and statistical measures (Spachos et al., 2014). Different roles in the care system of the user are associated with different privileges (Spachos et al., 2014). Parents and personal health professionals (HPs) have access to new user creation and management of user data while teachers involved can manage school and discipline referral information. All involved roles (i.e., parents, HPs, and teachers) are allowed to target specific behaviors considered to be dysfunctional, plan an FBA, collect ABC, and define the function of a behavior while only HPs are allowed to plan a suitable intervention (Spachos et al., 2014).
The mobile component serves the data collection for FBA gathering direct observations, referring to the frequency and the duration of a behavior that occurs, or completing the information on ABC charts (Alves et al., 2013; Spachos et al., 2014; Merlo et al., 2018). The ABC charts refer to the antecedent factors, behavior topography, and consequences of the observed behavior at a personal and societal level (Spachos et al., 2014) and constitute a valuable tool in Cognitive Behavioral Therapy (Emerson, 2001). Data collection via mobile application is synchronized with the web app offering the opportunity to the HP involved in the child’s or young adult’s network to hypothesize the behavior function, design an intervention plan, and evaluate the implemented intervention (Alves et al., 2013; Spachos et al., 2014; Merlo et al., 2018).
2.2.2. ADHD360
The ADHD360 platform was developed in the context of the ADHD360 project funded by the European Union and Greek national funds through the Operational Program Competitiveness, Entrepreneurship, and Innovation, under the call RESEARCH–CREATE–INNOVATE (grant Τ1ΕΔΚ-01680). The main focus of the project was to build an integrated platform that includes a serious game (“Pizza on Time” game) for the early detection of ADHD characteristics and a mobile application (mADHD360 app) for behavior monitoring (Pandria et al., 2022).
The “Pizza on Time” game is a running game where the player tries to deliver a pizza avoiding obstacles and collecting coins as a pizza slice is lost every time the player falls over an obstacle. The same level is played again when the pizza is lost. The game is taking place in three in-game world levels (city, jungle, and space) including 40 sublevels in each world level. Retaining the concept of pizza delivery, four mini games are integrated with the main game to enhance the game mechanics. The game design is based on diagnostic criteria and neuropsychological tools commonly used in ADHD screening. More precisely, the principles of diagnostic criteria described in the Diagnostic and Statistical Manual of Mental Disorders, Fifth Edition (DSM-V) (American Psychiatric Association, 2013) and neuropsychological tools were transferred to serious game design as game mechanics. Additionally, the early detection of ADHD was facilitated by calculating the likelihood of having ADHD after analyzing gameplay data through machine learning methodology.
Within the ADHD360 platform, the mADHD360 application (Pandria et al., 2022), as the rational descendant of the WHAAM app, constitutes a progressive web app designed to run on smartphones, mobile devices, and tablets. It is dedicated to behavioral monitoring providing observation features to HPs, parents, and teachers to conduct FBA in a unified and easy-to-use way. Additionally, it integrates a sociocentric methodology enabling the creation of a virtual network of people involved in the user’s care. mADHD360 also facilitates the evaluation of the intervention plan by providing a visual representation of gathered data in terms of duration and frequency (Pandria et al., 2022). Although the mADHD360 application builds on the knowledge gained from the WHAAM app and preserves its main principles, it is enriched with more features (e.g., asynchronous chat capabilities) and provides advanced user experience and usability (Pandria et al., 2022).
2.2.3. BrainHQ
BrainHQ designed and developed by Posit Science constitutes a specialized, commercial software for personalized and adaptable game-based cognitive training (Romanopoulou et al., 2017). In more detail, BrainHQ is an online interactive tool that incorporates six categories with more than 29 highly empowering cognitive exercises focusing on memory, attention, navigation, intelligence, brain speed, and people skills (BrainHQ, 2022). Tailored cognitive training is ensured by automatically adjusting each exercise to the level of competence of each participant. The adjustability of the cognitive training is provided by including hundred levels of difficulty (Romanopoulou et al., 2021). The proven benefits of BrainHQ are summarized as the rewiring of the brain as assessed through the improvement of brain activation, timing, and connectivity between different brain regions, the sharpening of cognitive function, and the improvement of daily living (Anagnostopoulou et al., 2021; BrainHQ, 2022).
2.2.4. AffectLecture
AffectLecture is a hybrid mobile application that serves as a self-reporting emotion-registering tool to monitor emotional states (Siouli et al., 2017, 2020). It provides a five-level Likert scale where “1” stands for “very sad” while “5” stands for “very happy” and is displayed using emoticons. The app was developed using the Ionic framework which is free and open source while the backend was developed using AngularJS which is well-suited for robust applications (Siouli et al., 2020). The application was originally designed to monitor the students’ mood before and after a university or a classroom lecture, but it was later also considered and used to monitor the emotional changes of smokers who underwent biofeedback and neurofeedback training (Pandria et al., 2017) as well as in other protocols. Once the app is activated, a list of the sessions of the day ordered by the beginning time is provided. Navigation to a specific session is limited to the users who will attend the session with a 4-digit PIN which is defined by the instructor who registers the session on the app (Siouli et al., 2020). Once the right PIN is entered, the user selects the emoticon that expresses better his/her mood at that moment by clicking on a toolbar. The user votes two times, before and after the lecture or the activity/intervention applied (Siouli et al., 2020).
2.3. Studies
2.3.1. Profile of participants
Although six participants were initially recruited, three of them were withdrawn; as a result, only three female individuals with a mean age of 19.6 ± 5.03 years completed the first study (S1). One participant was 15 years old; she was diagnosed with mild intellectual disability. The other participant was 19 years old suffering from severe intellectual disability while the last one was 25 years old and diagnosed with cerebral palsy and right hemiplegia.
The second study (S2) involved an 8-year-old male child with a body mass index (BMI) of 21.4 Kg/m2 who was diagnosed with Simpson-Golabi-Behmel syndrome. He was characterized by dysmorphia, macrostomia, and macroglossia which make it difficult to pronounce the words. He also has benign congenital hypotonia, nephromegaly, umbilical hernia, squamous chest, square palms, and two extra nipples.
A 10-year-old male child with a BMI of 24.1 Kg/m2 who was diagnosed with a special learning disorder, visual motor disorder, and ADHD characteristics participated in the third study (S3).
The fourth study (S4) involved a heterogenous group of participants including (a) a 17-year-old male diagnosed with Williams syndrome, antisocial behavior, and inattention as profound conditions, (b) a 15-year-old female diagnosed with intellectual disability and inattention, and (c) a 13-year-old female diagnosed with ADHD, obsessive–compulsive disorder (OCD), oppositional defiant disorder, and special learning difficulties.
Forty-three participants with a mean age of 11.82 ± 2.81 years were recruited in the ADHD360 pilot study (S5) forming two groups, the ADHD group (18 participants) and healthy controls (25 participants). Of the 43 participants, 28 (65%) were male and 15 were female.
A summary of participant characteristics and technologies used per study is presented in Table 1.
2.3.2. Study design
The WHAAM application and the BrainHQ software were used in S1. In more detail, the study was conducted in the Child Care Center of Parents’ Association, Guardians and Friends of People with Disability of the Western Macedonia Region (Ptolemaida, Greece), from 1st June 2017 to 31st July 2017. Two 1-month phases including baseline and intervention were defined. Before the baseline phase, Raven’s Colored Progressive Matrices (CPM), Wechsler Intelligence Scale for Children (3rd edition), and Reading the Mind in the Eyes were administered by experienced clinical psychologists to assess cognitive functioning and emotion recognition. Targeted behaviors were defined for each participant (Table 2) by the psychologists and the caregivers of the Child Care Center and they were monitored in both phases using the WHAAM application. Two observation frameworks were set for the baseline including leisure time and during activities of the Care Center, while three observation frameworks were defined for the intervention phase including leisure time, during activities of the Care Center, and during the intervention. The duration of each observation was 20 min and two observations per behavior were carried out each day. The total number of observations for each behavior was 30. Cognitive training using the BrainHQ software was applied as an intervention. Participants were trained three times per week with an average duration of training being some 30 min per session. Once the training was completed, the neuropsychological evaluation was performed following the baseline procedures.
S2 was conducted in a primary school in Chalkidiki Province, Greece. Thirty sessions of cognitive training using the BrainHQ platform were used as an intervention with a frequency of three times per week and an average duration of 45 min per session. The training was mainly performed at school as well as at the participant’s home. Before the intervention, neuropsychological and educational evaluations were performed. A neuropsychological assessment was conducted by an experienced clinical psychologist following the procedures described in the previous study (S1). The educational evaluation was performed by a (disabilities) specialist educator, and it was focused on five domains including speaking, writing, psychomotor skills, mental abilities, and emotional organization. The educational evaluation was based on direct and systematic observations as well as informal tests. Moreover, an observation diary was used to monitor the participant’s behavior daily. Additionally, the emotional state was assessed through the AffectLecture application both before and after each training session. Once training was completed, the neuropsychological and educational assessments were repeated. Moreover, a semi-structured interview between the specialist educator and the participant’s mother was conducted to assess any possible improvement in the student’s emotional state observed after the training sessions, the effect of emotional state on his school performance, as well as the effect of performance on his emotional state overall.
The third study (S3) was conducted in a primary school in Thessaloniki Province, Greece. In total, 40 training sessions through the BrainHQ platform were used as an intervention, which was performed at school as well as at the participant’s home. The frequency of training was three times per week for a total duration of 6 weeks, while the last 2 weeks of the training were performed daily with a duration of training of some 45 min per session. Before the intervention, a neuropsychological evaluation was performed by an experienced psychologist following the procedures described in the previous study (S1). Moreover, an observation diary was used to monitor the participant’s behavior daily. Emotional states were evaluated through the AffectLecture application both before and after each training session. The neuropsychological assessment was repeated after completing the training. Furthermore, a semi-structured interview was performed between the teachers and the participant’s mother to discuss any noticeable possible improvement in cognitive skills and emotional states after the intervention, the impact of emotional states on school performance, as well as the possible effect of school performance on the emotional state overall.
In the fourth study (S4), the WHAAM application was used as the monitoring tool while operant conditioning techniques were implemented as an intervention. The study was conducted in Volos Orphanage, Greece, from 15 July 2016 to 15 September 2016. The evaluation of the intervention was performed through effect size calculations by the app. Targeted behaviors defined for each participant are described in Table 2.
S5 consisted of a two-phase non-randomized controlled pilot study conducted in the context of the ADHD360 project, the objective was to explore whether the ADHD360 platform could facilitate early detection of ADHD characteristics as well as serve as an intervention for ADHD management. Due to the COVID-19 outbreak, the study was performed remotely from 1st April 2020 to 30th December 2021. A thorough description of the study protocol is available in Pandria et al. (2022).
2.3.3. Statistical analysis
In three out of five studies (S1–S3), IBM SPSS (Version 23) was used for statistical analysis at a significance level of 0.05. In more detail, descriptive statistics were calculated and presented along with effect sizes automatically produced by the WHAAM app in the first study. Studies S2 and S3 followed a similar methodology conducting descriptive statistical analysis. Additionally, continuous variables such as scores from neuropsychological evaluation and AffectLecture data were explored for normality assumption using the Kolmogorov–Smirnov test both before and after the intervention. Wilcoxon Signed Ranks Test was performed in both studies (S2, S3) to explore possible changes in continuous variables before and after the intervention. In S2, Spearman’s rho correlation index was calculated to assess the correlation between the emotional state before the intervention and the performance evaluated as collected “stars” during the game, the emotional state after the intervention and the performance, as well as the performance and the number of training sessions. Apart from the aforementioned analysis, Spearman’s rho correlation index was computed to evaluate the correlation between the difference in the emotional state (post-pre intervention) and the performance, the scores in the Double decision exercise and the Mind Bender exercise, and the participant’s performance, respectively, in the S3. S4 used effect sizes automatically produced by the WHAAM app as measures to evaluate the effectiveness of the intervention applied. Statistical analysis in the last study (S5) was performed using IBM SPSS (Version 26) at a significance level of 0.05. Continuous variables were explored for normality by the Shapiro–Wilk test and then reported either as mean ± standard deviation or as median and interquartile range Q1–Q3, where Q1 and Q3 are the first and the third distribution quartiles, respectively. Categorical data were reported as frequencies and percentages. State-of-the-art machine learning (ML) algorithms were implemented to analyze labeled gameplay data targeting to reveal discriminative patterns between the two groups (ADHD and non-ADHD) and estimate the likelihood of having ADHD characteristics. A train-test data splitting (75:25 split ratio), a k-fold cross-validation procedure with k = 3, an ML pipeline, and data analysis form a schema that is thoroughly described in Pandria et al. (2022).
3. Results
3.1. Study S1
3.1.1. Case 1
Case 1 refers to a 19-year-old female participant who was suffering from a severe intellectual disability. Three behaviors were targeted as shown in Table 2. Fifty-nine frequency-based observations were performed for the behavior “Easily distracted by things around them” where 30 out of 59 were completed before the intervention while 29 out of 59 were performed after the intervention. The mean frequency of behavior occurrence was 5.80 ± 6.91 and 2.52 ± 2.38 before and after the intervention, respectively. The effect size automatically calculated by the app was −0.209 showing that the intervention had a small effect on the decrease in behavior occurrence (Figure 1). Fifty-seven frequency-based observations were performed for the behavior “She is in a good mood” where 31 out of 57 were implemented at baseline while the remaining observations were performed after the intervention. The average frequency of behavior occurrence was 5.42 ± 4.87 before the training and 5.85 ± 3.52 after the intervention. The effect size was calculated to be 0.401 showing that the intervention had a medium effect on the increase in behavior occurrence (Figure 2). Fifty-six frequency-based observations (baseline: 30, post-training: 26) were conducted for the behavior “She feels worried.” A reduction in the behavior occurrence was observed as the average frequency of behavior occurrence was 1.33 ± 2.43 at baseline and 0.15 ± 0.46 post-training. However, the effect size was calculated to be −0.099 indicating that the intervention did not affect the occurrence of behavior (Figure 3).
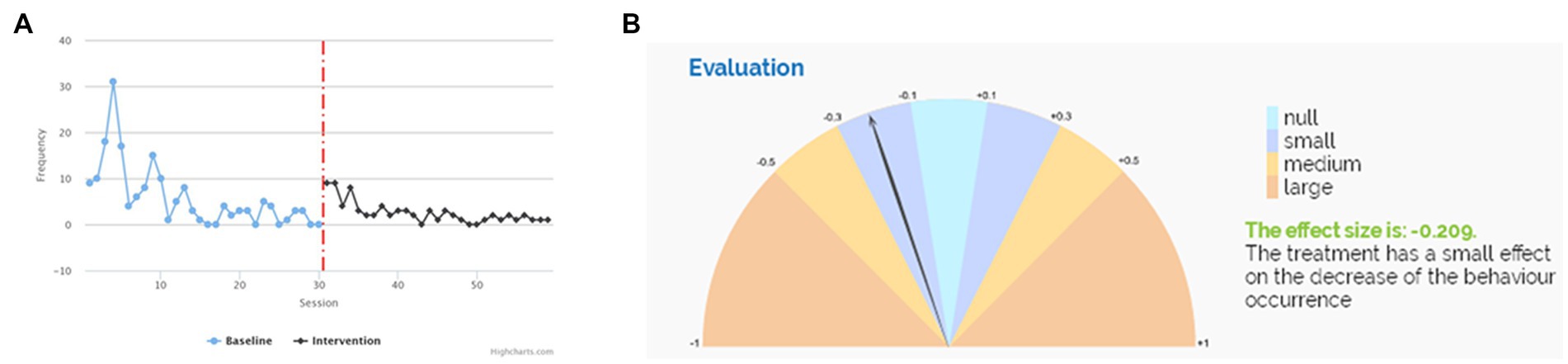
Figure 1. (A) Line graph of frequency-based observations about the behavior “Easily distracted by things around them” before and after the cognitive training through the BrainHQ platform. (B) Effect size calculated by the WHAAM application. Data displayed refer to Case 1 (S1).
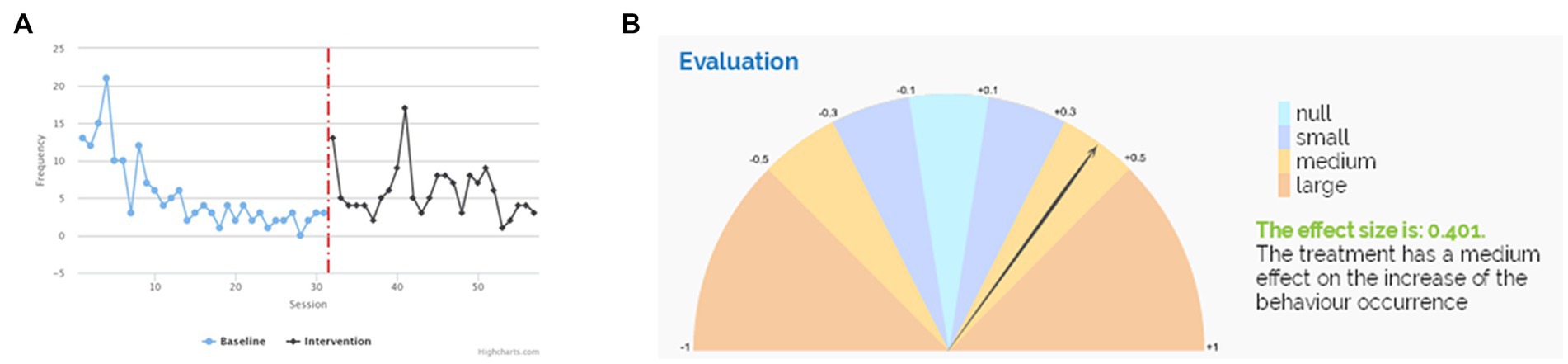
Figure 2. (A) Line graph of frequency-based observations about the behavior “She is in a good mood” before and after the cognitive training through the BrainHQ platform. (B) Effect size calculated by the WHAAM application. Data shown refer to Case 1 (S1).
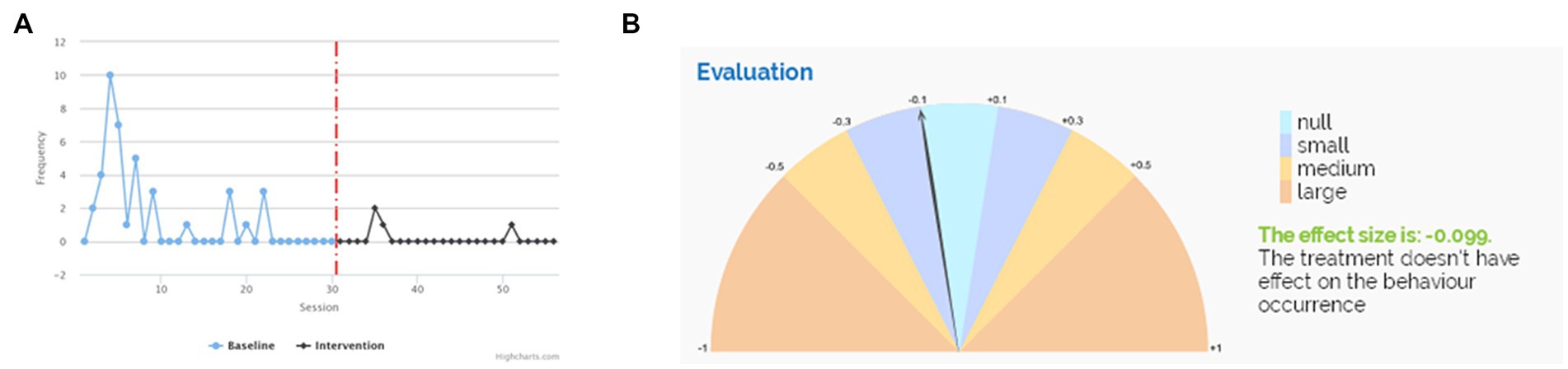
Figure 3. (A) Line graph of frequency-based observations about the behavior “She feels worried” before and after the cognitive training through the BrainHQ platform. (B) Effect size calculated by the WHAAM application. Data shown refer to Case 1 (S1).
3.1.2. Case 2
Case 2 refers to a 15-year-old female participant diagnosed with mild intellectual disability. Sixty duration-based observations were performed (30 observations before and after the intervention) about the behavior “She gets angry and sad.” The mean duration of the behavior occurrence was 12.77 ± 23.29 min at baseline and 1.00 ± 5.48 min after the intervention. The effect size was calculated to be −0.223 indicating a small effect of intervention in decreasing the behavior occurrence (Figure 4). Moreover, 59 observations were conducted (30 at baseline; 29 post-training) for the behavior “She has manifestations of shame.” The mean frequency of behavior occurrence was 0.80 ± 1.80 and 0.07 ± 0.26 before and after the intervention, respectively. Furthermore, an effect size equal to −0.15 showed that the intervention had a small effect on the reduction of behavior occurrence (Figure 5).
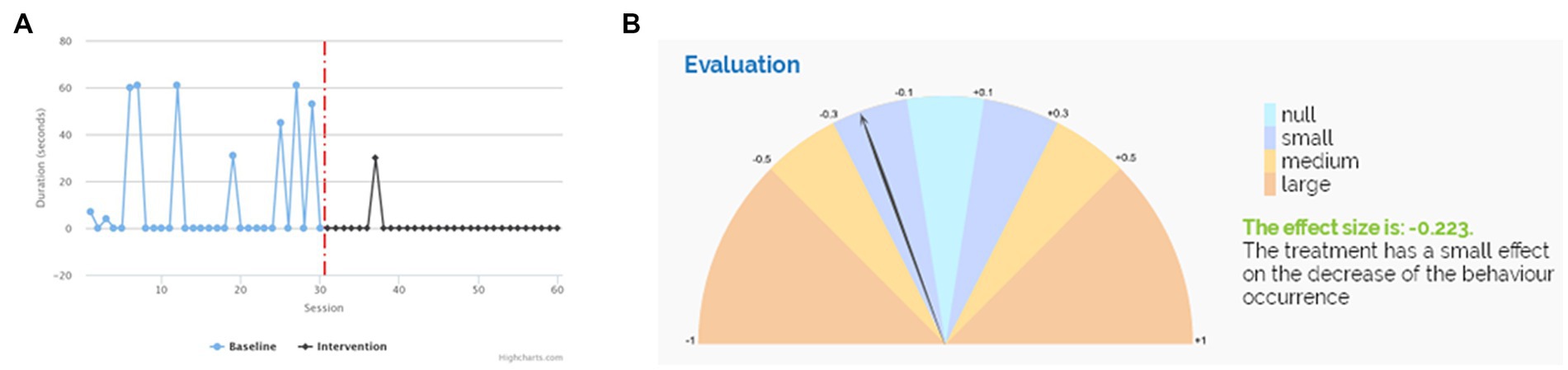
Figure 4. (A) Line graph of duration-based observations about the behavior “She gets angry and sad” before and after the cognitive training through the BrainHQ platform. (B) Effect size calculated by the WHAAM application. Data shown refer to Case 2 (S1).
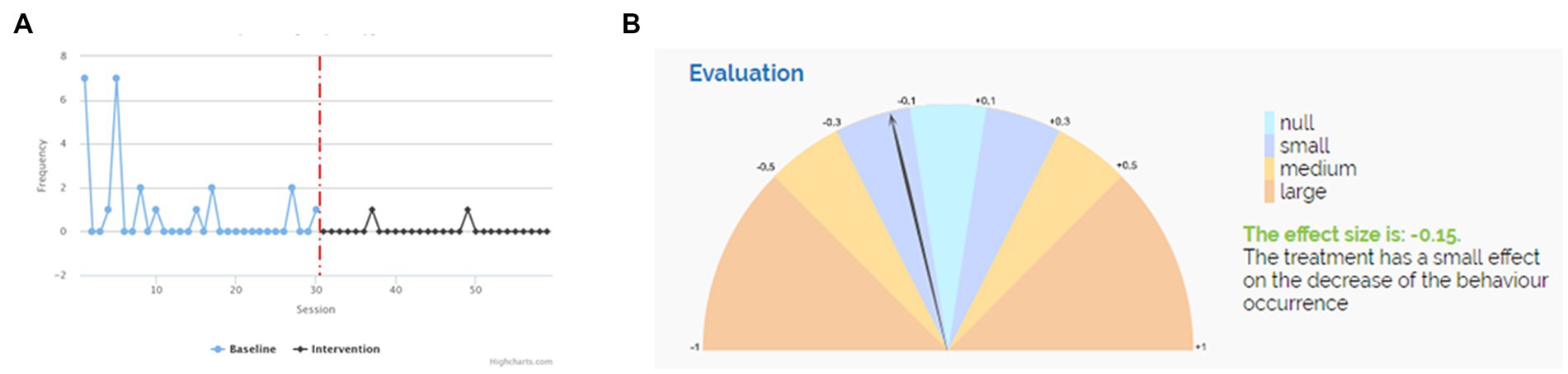
Figure 5. (A) Line graph of frequency-based observations about the behavior “She has manifestations of shame” before and after the cognitive training through the BrainHQ platform. (B) Effect size calculated by the WHAAM application. Data reported refer to Case 2 (S1).
3.1.3. Case 3
Case 3 refers to a 25-year-old female participant diagnosed with cerebral palsy and right hemiplegia. Fifty-eight frequency-based observations were focused on the behavior “She is cooperative and easy-going.” Thirty observations were conducted at baseline whereas 28 observations were conducted after the intervention. The average frequency of behavior occurrence was changed from 4.53 ± 3.55 before training to 5.86 ± 2.26 after training. An effect size equal to 0.462 showed that the intervention had a medium effect on the increase in behavior occurrence (Figure 6).
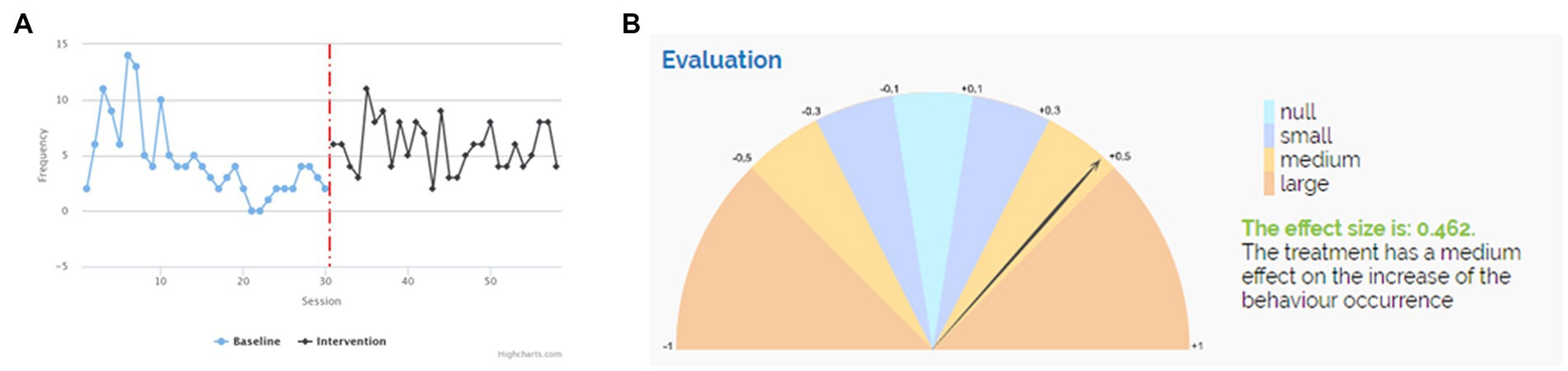
Figure 6. (A) Line graph of frequency-based observations about the behavior “She is cooperative and easy-going” before and after the cognitive training through the BrainHQ platform. (B) Effect size calculated by the WHAAM application. Data shown refer to Case 3 (S1).
3.2. Study S2
The participant in S2 scored higher in the neuropsychological evaluation after training (Table 3) compared to baseline (W = −3.070; p = 0.002). Furthermore, the emotional state improved after the intervention compared to the baseline (W = −3.000; p = 0.003). The participant performance in BrainHQ measured as the number of stars gained during the training was positively correlated to the emotional state both before (r = 0.768; p < 0.001) and after (r = 0.896; p < 0.001) the intervention (Figure 7). Additionally, the performance was positively associated with the number of training sessions (r = 0.650; p < 0.001; Figure 8). Educational evaluation in the two phases showed improvement in reading speed, phonological awareness, vocabulary, and handwriting. Moreover, interpersonal peer relationships improved after the training.
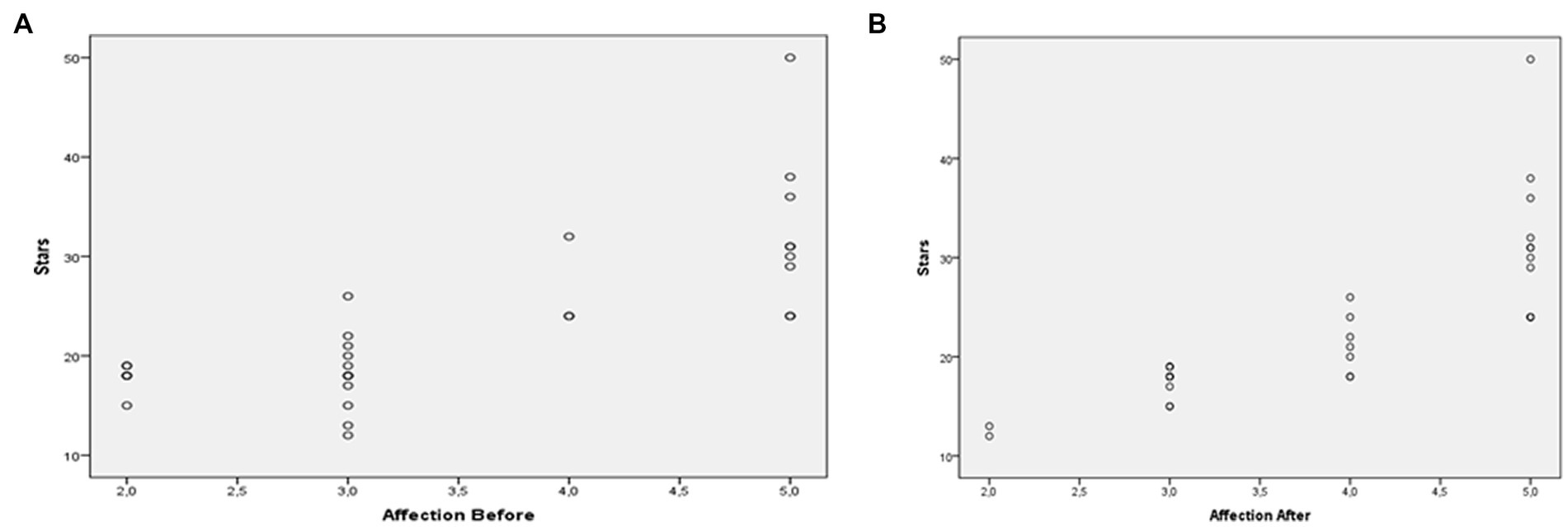
Figure 7. (A) Correlation of performance in BrainHQ measured as the number of stars gained during the training with the emotional state reported in AffectLecture app before the intervention. (B) Correlation of performance in BrainHQ measured as the number of stars gained during the training with the emotional state reported in AffectLecture app after the intervention. Data shown refer to S2.
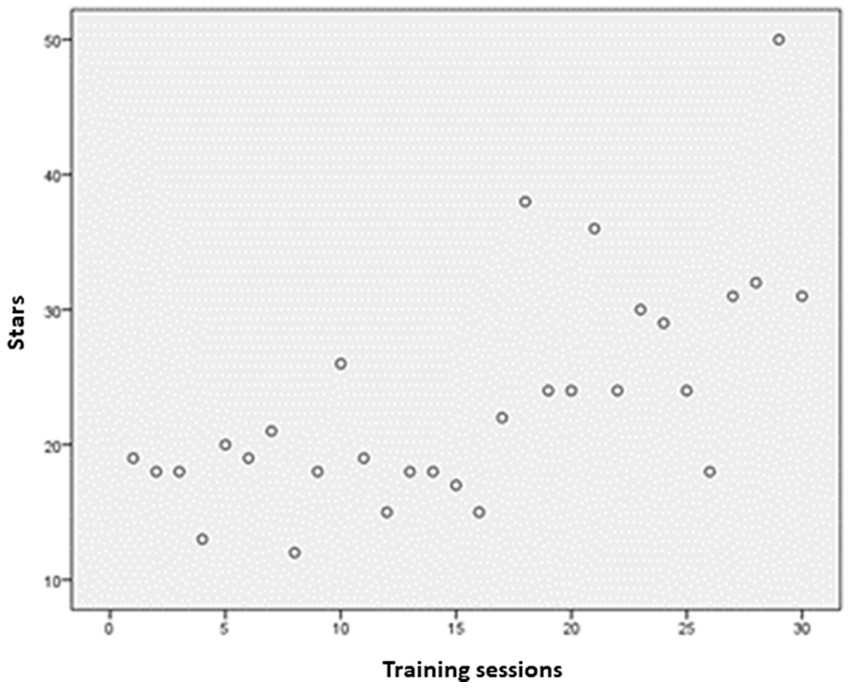
Figure 8. Correlation of performance in BrainHQ measured as the number of stars gained during the training with the number of training sessions. Data shown refer to S2.
3.3. Study S3
Improvement was shown in neuropsychological tests (W = −1.901; p = 0.057; Table 3) and the emotional state (W = −3.382; p = 0.001; Figure 9) compared to baseline. A significant positive correlation was observed between the participant performance in BrainHQ training and the change in the emotional state (r = 0.415; p = 0.008; Figure 10), as well as between the performance and the emotional state after the training (r = 0.433; p = 0.005; Figure 10). However, performance in BrainHQ training was not associated with the emotional state before the training (r = −0.255; p = 0.112). Moreover, the performance in training was positively correlated with the number of training sessions (r = 0.436; p = 0.005; Figure 11). The training performance in Double decision (r = 0.675; p = 0.032) and Mind Bender (r = 0.629; p = 0.016) exercises was positively correlated with the number of training sessions (Figure 12). Exercises focused on attention, intelligence, social skills, and brain speed or exercises enriched with audio feedback were the most preferred while memory and navigation exercises were considered challenging. The semi-structured interview revealed that improvement was shown in phonological awareness, reading, writing, concentration, descriptive reasoning, and social interactions. Moreover, his attitude toward school and home changed positively and his school performance was improved.
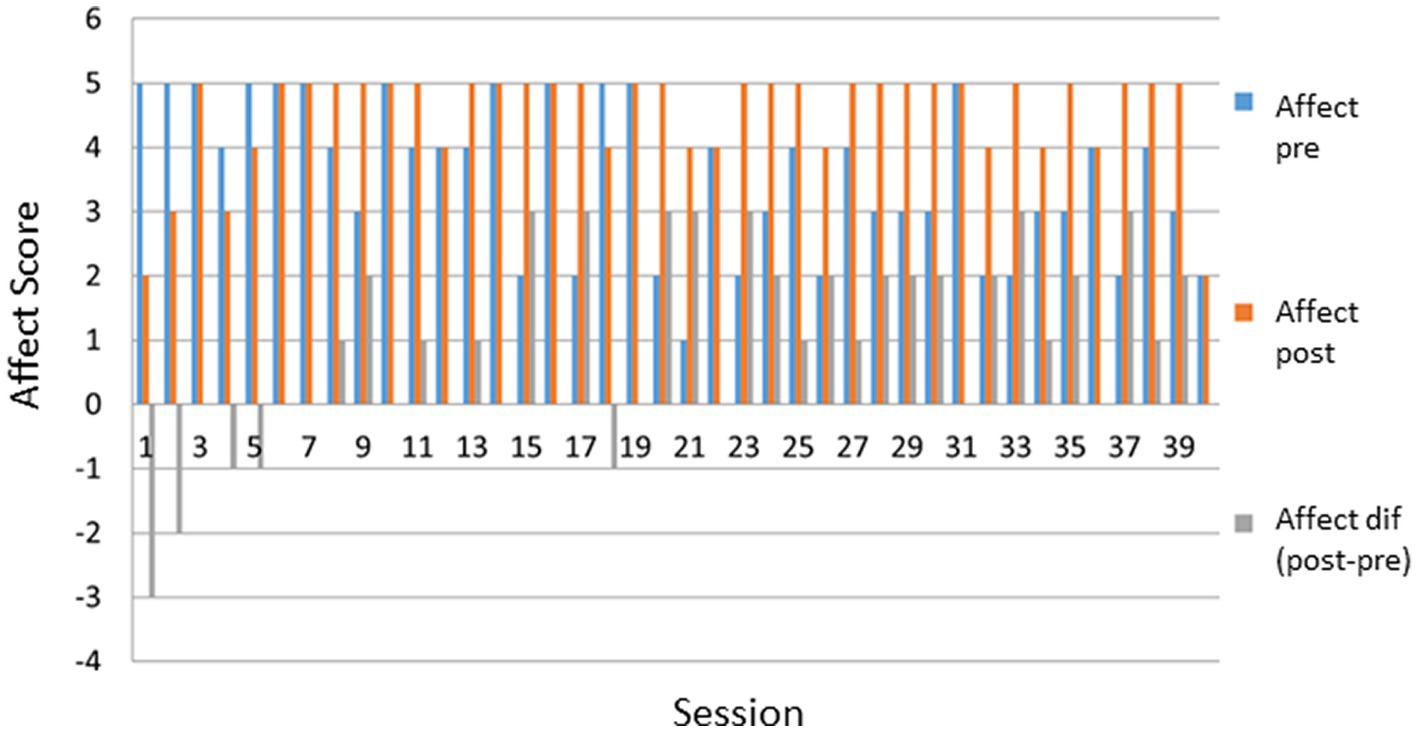
Figure 9. Emotional state of the participant in S3 before and after the intervention and the change in the emotional state (post-pre intervention). The emotional state was reported in the AffectLecture app.
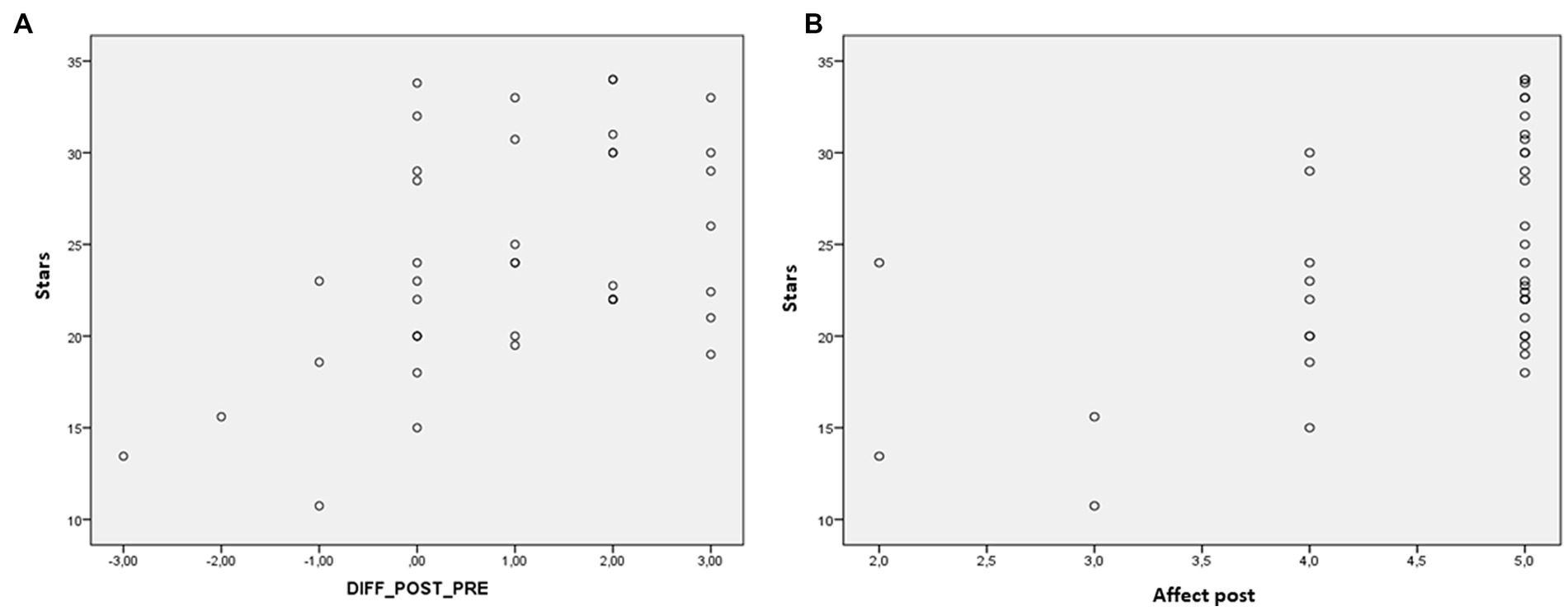
Figure 10. (A) Correlation of performance measured as the number of stars gained during the training with the change in the emotional state (post-pre intervention) reported in the AffecLecture app. (B) Correlation of performance measured as the number of stars gained during the training with the emotional state reported in the AffectLecture app after the intervention. Data shown refer to S3.
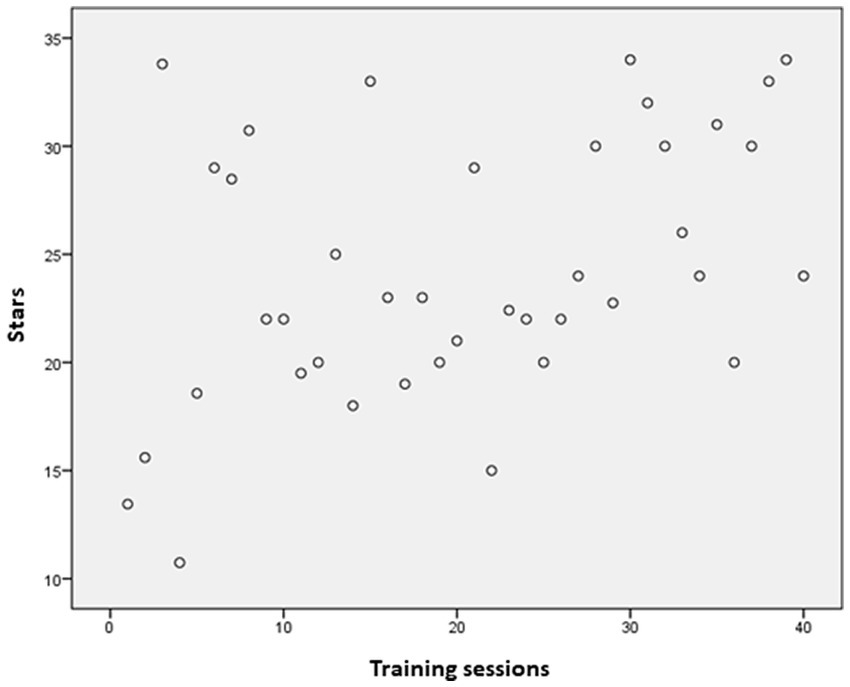
Figure 11. Correlation of performance measured as the number of stars gained during the training with the number of training sessions. Data displayed refer to S3.
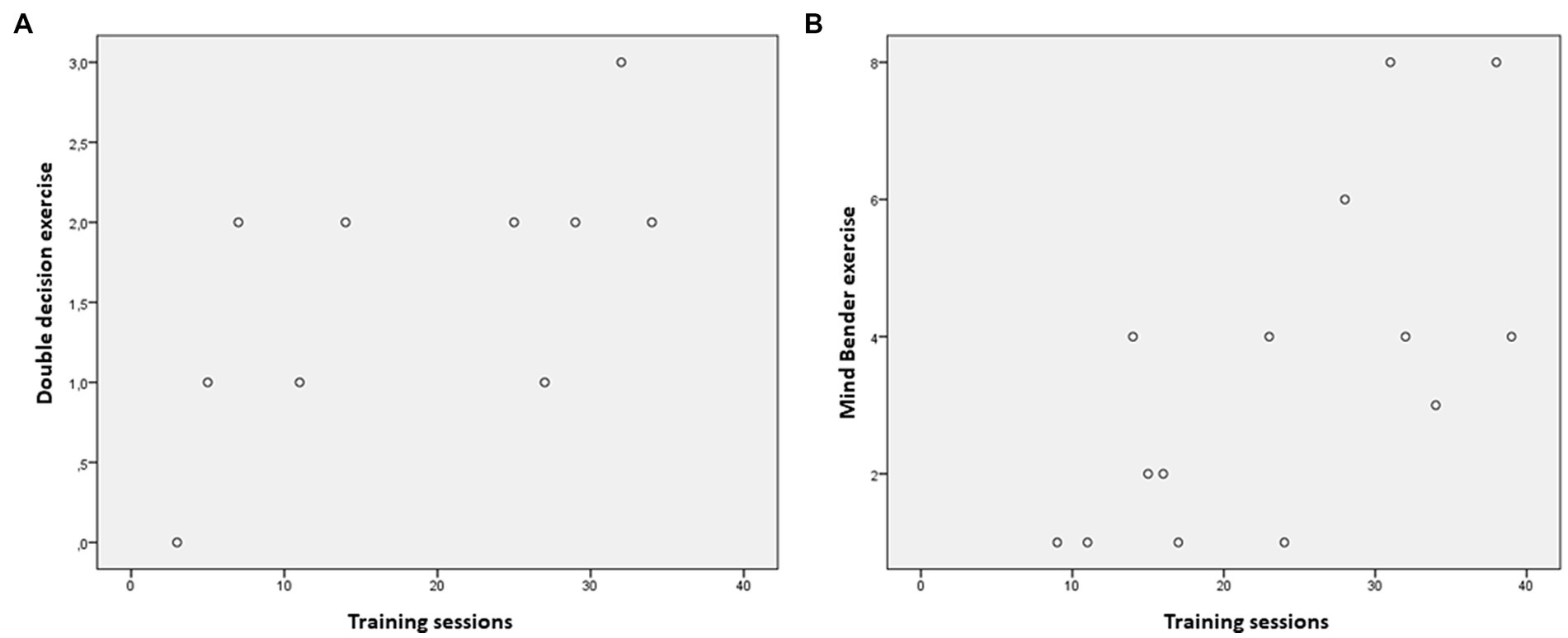
Figure 12. (A) Correlation of performance in Double decision exercise (BrainHQ platform) with the number of training sessions. (B) Correlation of performance in Mind Bender exercise (BrainHQ platform) with the number of training sessions. Data reported refer to S3.
3.4. Study S4
3.4.1. Case 1
Case 1 stands for a 17-year-old male participant diagnosed with Williams syndrome, antisocial behavior, and inattention. Five observations were performed in the reading room (three out of five), in the yard (one out of five), and in the living room (one out of five) focusing on his antisocial behavior before the intervention was applied. Going out for shopping was used as operant conditioning rewarding method when he showed more sociability. Four observations were performed after the intervention. The effect size was calculated to be 0.537 indicating that the intervention had a large effect on the increase of the behavior occurrence. Furthermore, four observations were conducted focusing on the inattention before and after the intervention was applied. In this case, a walk was used as operant conditioning rewarding method when the desired behavior (increased attention) occurred. The effect size was calculated to be −0.462 showing that the intervention had a medium effect on the decrease of the behavior occurrence.
3.4.2. Case 2
Case 2 refers to a 15-year-old diagnosed with intellectual disability and inattention. Four observations were performed focusing on her inattention both before and after the intervention was used. As an intervention, another child gained the stickers intended to be given to her. The effect size was found to be −0.167 showing that the method used had a small effect on reducing the behavior occurrence.
3.4.3. Case 3
Case 3 refers to a 13-year-old diagnosed with ADHD, obsessive–compulsive disorder (OCD), oppositional defiant disorder, and special learning difficulties. Four observations were conducted focusing on reducing occurrence of the rude behavior toward peers and caregivers both before and after the intervention was applied. A walk was used as operant conditioning rewarding method every 5 days the behavior did not occur. The effect size was computed to be 0.139 showing that the method applied had a small effect on increasing the behavior occurrence. Furthermore, eight observations were performed targeting the decrease of the behavior “Destruction of objects belonging either to herself or to others” before the implementation of the intervention. Photocopies of her favorite game were used as operant conditioning rewarding method. Four observations were performed after the intervention was applied. The calculated effect size was 0.305 indicating that the method used moderately affected the increase of behavior occurrence. Targeting the reduction of physical aggression, the loss of caregivers’ attention due to her behavior was used as an operant conditioning approach. Four observations were conducted through the WHAAM application both before and after the intervention was applied. The computed effect size was −0.483 showing that the approach applied had a moderate effect on decreasing the behavior occurrence. Focusing on reducing interruption or interference in conversation with others, her favorite stickers were given as an operant conditioning method. Four observations were conducted both before and after the intervention was applied. A small effect on behavior occurrence reduction was revealed by an effect size equal to −0.204.
3.5. Study S5
Neuropsychological and gameplay data were available from 30 out of 43 participants. Healthy controls (non-ADHD group) scored higher in WISC-V subscales and lower in ADHD Rating Scale-IV (see reference Pandria et al., 2022). Applying the machine learning methodology described in Pandria et al. (2022), Support Vector Machine emerged to be the most suitable algorithm as it features the best tradeoff between validation performance and goodness of fit while achieving the highest validation F1 score. Furthermore, the evaluation of the data analysis system showed that it has a notable capacity to classify gameplay behavior as either ADHD or non-ADHD.
4. Discussion
The inherent but paramount obstacles of existing traditional monitoring tools relying on pen-and-paper methods are related to data loss, difficulty in synchronous monitoring at different settings, the need for real-time recognition and interpretation of behavior, as well as subjectivity biases. To address these challenges, a set of technological tools were either on purpose developed or repurposed to enhance the educational and interventional arsenal regarding behavioral difficulties, developmental disorders, and special education. More specifically, this study explains how the WHAAM (Spachos et al., 2014), the AffectLecture application (Siouli et al., 2017, 2020), the ADHD360 (Pandria et al., 2022), and BrainHQ platforms (Romanopoulou et al., 2021) were designed and implemented or deployed as pilot intervention. Four case series studies (S1–S4) along with a pilot study (S5) were presented integrating different technological solutions for monitoring, thereby highlighting multiple aspects of behavioral monitoring and its benefits in special education and behavioral difficulties.
The importance of behavioral monitoring has been described as a crucial step in providing efficient interventions to students including those with BD (Lane and Walker, 2015). Among the available behavioral monitoring tools, systematic behavioral monitoring (SBM) is considered to be the “gold standard” as it provides a precise snapshot of behavior when it occurs (Riley-Tillman et al., 2005; Lane and Walker, 2015). This facilitates the identification and monitoring of targeted behaviors providing information about the frequency, duration, and intensity of a behavior during a definite time period (Merlo et al., 2018). Although its feasibility in school settings is limited by resource drain, it has been used as a screening, diagnostic, and monitoring tool (Riley-Tillman et al., 2005). SBM is integrated into Functional Behavioral Analysis (FBA) which is a descriptive behavioral assessment tool that includes direct and indirect observations and behavior measurements (Hanley, 2012). FBA focuses on identifying the function of targeted behavior by collecting information about the antecedents, the consequences, and the behavior itself (Gresham et al., 2001). It is recognized as a best practice approach in designing effective interventions for individuals with BD (Pelham et al., 2005).
During the last few years, different technological systems were developed to facilitate behavioral evaluation (Hser et al., 2001) providing selectively one feature of the FBA process (i.e., behavioral charts, counters, and video recording) (Merlo et al., 2018). However, the WHAAM application supports the entire FBA process from identifying a targeted behavior to evaluating an intervention that targets it. In parallel, it enables the creation of a virtual network of people involved in the user’s care promoting cooperation between them (Merlo et al., 2018). It constitutes a paradigm of how technological solutions can apply the FBA approach supporting the application of different interventions and investigating their effectiveness as described in S1 and S4. By integrating the WHAAM application into studies S1 and S4, it was possible to evaluate the effect of the intervention applied, namely, cognitive training through BrainHQ and operant conditioning strategies, respectively, thereby highlighting the positive outcomes in most monitored behaviors. Behavior, cognition, decision-making, memory, and learning outcomes are affected by emotional state (Pierre and Oughton, 2007; Shephard, 2008; Siouli et al., 2017) pointing out the need of having tools to monitor the emotional state and use this information to further assess its association with intervention effectiveness. The proliferation of sensor-based technologies and smartphones has favored the development of systems that can recognize, process, interpret, and stimulate human emotions (Picard et al., 2004; Kanjo et al., 2015). Self-monitoring, physiological measurements and behavioral observations can be used to reflect affective sensing (Mauss and Robinson, 2009). Self-monitoring is the most traditional and explicit way to collect information related to a person’s emotional state (Kanjo et al., 2015). For this purpose, verbal scales, animated cartoons, or pictograms have been used (Kanjo et al., 2015) and systems such as the Self-Assessment Manikin (Bradley and Lang, 1994) and the Emocards (Desmet et al., 2001) have been developed. More recently, self-monitoring was employed by smartphone applications such as the Mappiness (Mac, 2011) and WiMo (Mody et al., 2009) as well as by the most of commercially available and research-based systems (Kanjo et al., 2015). Similarly, we developed a hybrid mobile application, the AffectLecture app, which serves as self-reporting emotion-registering tool to monitor emotional states (Siouli et al., 2017, 2020). It provides a five-level Likert scale which is displayed using emoticons. The AffectLecture app was used to evaluate the emotional state of the participants in the studies S2 and S3 and using this information to further assess the association of emotional state with intervention effectiveness. Both studies indicated that emotional state was improved after cognitive training performed through BrainHQ and revealed a positive correlation between the performance in cognitive exercises and emotional state after the intervention. The performance in cognitive training was positively correlated with the emotional state before training in S2 but not in S3. Furthermore, a positive correlation between the change in emotional state and performance was mentioned only in S3.
The game performance has also been employed as a behavioral monitoring tool leading to meaningful information such as digital biomarkers in cognitive impairment (Gielis et al., 2019), motor signs in Autism Spectrum Disorder (Bondioli et al., 2021), and ADHD-related discriminative gameplay patterns (Pandria et al., 2022) supporting early diagnosis, disease monitoring, and management. Toward this direction, preliminary analysis of the ADHD360 pilot study (S5) indicated that the platform was considered to have a notable capacity to classify users either as ADHD or non-ADHD based on their in-game patterns. It is believed that this could potentially serve as a complementary screening tool providing benefits to people diagnosed with ADHD or their associated educators and healthcare professionals.
All studies reported here support the argument that technology may have multiple roles in monitoring behavior and may enhance our capacity in confronting behavioral difficulties in education. However, none of the studies is free of limitations. Two out of five studies were case studies, two out of five studies were case series, and one out of five studies referred to a two-phase non-randomized controlled pilot study. Heterogeneity in study design and participant profile is another limitation. Additionally, only one out of five studies (S5) recruited healthy controls. However, all studies take advantage of tools for different monitoring purposes. The COVID-19 outbreak highlighted the necessity of continuous monitoring of behavior along with other psychological challenges such as herd behavior, misinformation, and stigmatization (Betsch et al., 2020). Furthermore, the pandemic has accelerated the transition to remote monitoring and treatment according to users’ (unmet) needs and risks improving health-related outcomes (Pronovost et al., 2022). Remote monitoring at home has been considered to provide potential safety benefits contributing to the transformation of care (Pronovost et al., 2022). It is therefore anticipated that technological tools related to monitoring may be considered valuable for the field of education and health as they enable the evaluation of the effectiveness of an intervention, the outcomes related to education, health, and emotional states, the associations between the emotional state with intervention effectiveness, as well as the early detection of ADHD characteristics through discriminative in-game patterns.
To conclude, this study is mostly a collage of unpublished and unreported studies (S1–S4), which despite their inhomogeneous designs, possess a common underlier, which is the empowerment offered by technology in alleviating some of the burden associated with behavioral difficulties in special education. Obviously, one needs to expand this case study series into a proper set of interventional clinical/educational trials, to create solid evidence and produce recommendations. However, the feasibility of using the technological tools described herein as well as their positive findings and the lessons learned should be considered as useful motivators for knowledge building and should not be underestimated.
Data availability statement
The original contributions presented in the study are included in the article/supplementary material, further inquiries can be directed to the corresponding author.
Ethics statement
The studies involving human participants were reviewed and approved by the Bioethics Committee of the Medical School of the Aristotle University of Thessaloniki. Written informed consent to participate in this study was provided by the participants’ legal guardian/next of kin. Written informed consent was obtained from the minor(s)’ legal guardian/next of kin for the publication of any potentially identifiable images or data included in this article.
Author contributions
PDB and NP contributed to the conception and design of this study, authored the manuscript, undertook the manuscript revision and proofreading, and approved the submitted version. Interventions and data collection were conducted by several contributors/facilitators, namely, Evangelia Romanopoulou, Maria Karagianni, Styliani Siouli, Stylianos Makris, Anastasia Koniali, and Victoria Mouratoglou, all of them supervised by PDB. All authors contributed to the article and approved the submitted version.
Funding
This whole research and case series did not receive any specific grant from funding agencies in the public, commercial, or not-for-profit sectors. However, this work was supported by the LLM Care (www.llmcare.gr/en) self-funded initiative that emerged as the not-for-profit business exploitation of the Long-Lasting Memories (LLM Project) originally funded by the ICT-CIP-PSP (Information and Communication Technologies–Competitiveness and Innovation Framework Programme–Policy Support Programme) Program of the European Commission (grant agreement ID: 238904). Parts of this research have been partially supported by the Web Health Application for ADHD Monitoring (WHAAM) project that was funded with the support of the European Commission, in the context of the Lifelong Learning Programme Key Activity 3 Multilateral Projects, Call EAC/27/11. Reference project number: 531244-LLP-2012-IT-KA3MP. Moreover, parts of this work were supported by the ADHD360 project that was funded by the European Union and Greek national funds through the Operational Program Competitiveness, Entrepreneurship, and Innovation, under the call RESEARCH–CREATE–INNOVATE (grant Τ1ΕΔΚ-01680).
Conflict of interest
The authors declare that the whole research series was conducted in the absence of any commercial or financial relationships that could be construed as a potential conflict of interest. However, there may be potential conflicts of interest (other, not financial, outside the scope of the submitted work) for the author PDB in respect of PositScience and the Aristotle University of Thessaloniki. There is a co-marketing agreement between PositScience and the Aristotle University of Thessaloniki to exploit BrainHQ within the LLM Care self-funded initiative that emerged as the non-for-profit business exploitation of the Long LastingMemories (LLM Project) (www.longlastingmemories.eu) originally funded by the ICT-CIP-PSP Program of the European Commission. BrainHQ now forms part of the LLM Care, a technology transfer/self-funded initiative that emerged as the not-for-profit business exploitation of LLM.
Publisher’s note
All claims expressed in this article are solely those of the authors and do not necessarily represent those of their affiliated organizations, or those of the publisher, the editors and the reviewers. Any product that may be evaluated in this article, or claim that may be made by its manufacturer, is not guaranteed or endorsed by the publisher.
References
Alves, S., Bamidis, P., Bilbow, A., Callahan, A., Chiazzese, G., Chifari, A., et al. (2013). WHAAM: Context-Driven Framework. 1–148.
American Psychiatric Association. Diagnostic and statistical manual of mental disorders. 5th. Washington, VA: American Psychiatric Publishing. (2013).
Anagnostopoulou, A., Styliadis, C., Kartsidis, P., Romanopoulou, E., Zilidou, V., Karali, C., et al. (2021). Computerized physical and cognitive training improves the functional architecture of the brain in adults with down syndrome: a network science EEG study. Netw. Neurosci. 5, 274–294. doi: 10.1162/netn_a_00177
Betsch, C., Wieler, L. H., and Habersaat, K. (2020). Monitoring behavioural insights related to COVID-19. Lancet 395, 1255–1256. doi: 10.1016/S0140-6736(20)30729-7
Bondioli, M., Chessa, S., Narzisi, A., Pelagatti, S., and Zoncheddu, M. (2021). Towards motor-based early detection of autism red flags: enabling technology and exploratory study protocol. Sensors 21:1971. doi: 10.3390/s21061971
Brackett, M. A., Palomera, R., Mojsa-Kaja, J., Reyes, M. R., and Salovy, P. (2010). Emotion-regulation ability, burnout, and job satisfaction among British secondary-school teachers. Psychol. Sch. 47, 406–417. doi: 10.1002/pits.20478
Bradley, M. M., and Lang, P. J. (1994). Measuring emotion: the self-assessment manikin and the semantic differential. J. Behav. Ther. Exp. Psychiatry 25, 49–59. doi: 10.1016/0005-7916(94)90063-9
Brain, HQ. (2022). Homepage Posit Science. Available at: www.brainhq.com
Buyse, E., Verschueren, K., Doumen, S., Van Damme, J., and Maes, F. (2008). Classroom problem behavior and teacher-child relationships in kindergarten: the moderating role of classroom climate. J. Sch. Psychol. 46, 367–391. doi: 10.1016/j.jsp.2007.06.009
Caslin, M. (2019). ‘I have got too much stuff wrong with me’ – an exploration of how young people experience the social, emotional and behavioural difficulties (SEBD) label within the confines of the UK education system. Emot. Behav. Difficulties 24, 167–180. doi: 10.1080/13632752.2019.1587899
Cooper, P. (2011). Teacher strategies for effective intervention with students presenting social, emotional and behavioural difficulties: an international review. Eur. J. Spec. Needs Educ. 26, 71–86. doi: 10.1080/08856257.2011.543547
Davila-Montero, S., Dana-Le, J. A., Bente, G., Hall, A. T., and Mason, A. J. (2021). Review and challenges of technologies for real-time human behavior monitoring. IEEE Trans. Biomed. Circuits Syst. 15, 2–28. doi: 10.1109/TBCAS.2021.3060617
Desmet, P., Overbeeke, K., and Tax, S. (2001). Designing products with added emotional value: development and Appllcation of an approach for research through design. Des. J. 4, 32–47. doi: 10.2752/146069201789378496
Emerson, E. Challenging behaviour: analysis and intervention in people with severe intellectual disabilities. Cambridge, MA: Cambridge University Press. (2001).
Gielis, K, Kennes, J, De, Dobbeleer C, Puttemans, S, and Vanden, Abeele V. Collecting digital biomarkers on cognitive health through computer vision and gameplay: an image processing toolkit for card games. In 2019 IEEE International Conference on Healthcare Informatics (ICHI). Xi'an, China: IEEE. (2019). p. 1–12.
Glomb, T. M., and Tews, M. J. (2004). Emotional labor: a conceptualization and scale development. J. Vocat. Behav. 64, 1–23. doi: 10.1016/S0001-8791(03)00038-1
Gresham, F., Watson, T., and Skinner, C. (2001). Functional behavioral assessment: principles, procedures, and future direction. Sch. Psych. Rev. 30, 156–172. doi: 10.1080/02796015.2001.12086106
Gualtieri, C. T., and Johnson, L. G. (2005). ADHD: is objective diagnosis possible? Psychiatry 2, 44–53.
Hanley, G. P. (2012). Functional assessment of problem behavior: dispelling myths, overcoming implementation obstacles, and developing new lore. Behav. Anal. Pract. 5, 54–72. doi: 10.1007/BF03391818
Hastings, R. P., and Bham, M. S. (2003). The relationship between student behaviour patterns and teacher burnout. Sch Psychol Int 24, 115–127. doi: 10.1177/0143034303024001905
Hser, Y. I., Shen, H., Chou, C. P., Messer, S. C., and Anglin, M. D. (2001). Analytic approaches for assessing long-term treatment effects. Examples of empirical applications and findings. Eval. Rev. 25, 233–262. doi: 10.1177/0193841X0102500206
Kanjo, E., Al-Husain, L., and Chamberlain, A. (2015). Emotions in context: examining pervasive affective sensing systems, applications, and analyses. Pers. Ubiquitous Comput. 19, 1197–1212. doi: 10.1007/s00779-015-0842-3
Kauffman, J. M. (1999). The role of science in behavioral disorders. Behav. Disord. 24, 265–272. doi: 10.1177/019874299902400403
Kinman, G., Wray, S., and Strange, C. (2011). Emotional labour, burnout and job satisfaction in UK teachers: the role of workplace social support. Educ. Psychol. 31, 843–856. doi: 10.1080/01443410.2011.608650
Koundourou, C. (2012). “Developing staff skills through emotional literacy to enable better practices with children with social emotional and behavioural difficulties (SEBD)” in Transforming troubled lives: Strategies and interventions for children with social, emotional and behavioural difficulties. eds. J. Visser, H. Daniels, and T. Cole, International Perspectives on Inclusive Education, vol. 2 (Bingley, West Yorkshire: Emerald Group Publishing Limited), 93–106.
Lane, K. L., and Walker, H. (2015). “The connection between assessment and intervention: how can screening lead to better intervention” in Enduring issues in special education personal perspectives. eds. B. Bateman, M. Tankersley, and J. Lloyd (New York: Routledge), 285–302.
LaRusso, M. D., Romer, D., and Selman, R. L. (2008). Teachers as builders of respectful school climates: implications for adolescent drug use norms and depressive symptoms in high school. J. Youth Adolesc. 37, 386–398. doi: 10.1007/s10964-007-9212-4
Lassen, S. R., Steele, M. M., and Sailor, W. (2006). The relationship of school-wide positive behavior support to academic achievement in an urban middle school. Psychol. Sch. 43, 701–712. doi: 10.1002/pits.20177
Lee, R. T., and Brotheridge, C. M. (2011). Words from the heart speak to the heart. Career Dev. Int. 16, 401–420. doi: 10.1108/13620431111158805/full/html
Mac, KG. (2011). Mappiness. Available at: http://www.mappiness.org.uk/
Martineli, A. K. B., Pizeta, F. A., and Loureiro, S. R. (2018). Behavioral problems of school children: impact of social vulnerability, chronic adversity, and maternal depression. Psicol Reflexão e Crítica 31:11. doi: 10.1186/s41155-018-0089-9
Mauss, I. B., and Robinson, M. D. (2009). Measures of emotion: a review. Cogn Emot 23, 209–237. doi: 10.1080/02699930802204677
Mekonnen, H., Medhin, G., Tomlinson, M., Alem, A., Prince, M., and Hanlon, C. (2020). Impact of child emotional and behavioural difficulties on educational outcomes of primary school children in Ethiopia: a population-based cohort study. Child Adolesc. Psychiatry Ment. Health 14:22. doi: 10.1186/s13034-020-00326-6
Merlo, G., Chiazzese, G., Sanches-Ferreira, M., Chifari, A., Seta, L., McGee, C., et al. (2018). The WHAAM application: a tool to support the evidence-based practice in the functional behaviour assessment. J. Innov. Heal. Informat. 25, 63–70. doi: 10.14236/jhi.v25i2.919
Mody, RN, Willis, KS, and Kerstein, R. WiMo: location-based emotion tagging. In Proceedings of the 8th International Conference on Mobile and Ubiquitous Multimedia New York, NY, USA: ACM. (2009). pp. 1–4
Myers, S. S., and Pianta, R. C. (2008). Developmental commentary: individual and contextual influences on student–teacher relationships and children’s early problem behaviors. J. Clin. Child Adolesc. Psychol. 37, 600–608. doi: 10.1080/15374410802148160
Näring, G., Vlerick, P., and Van de Ven, B. (2012). Emotion work and emotional exhaustion in teachers: the job and individual perspective. Educ. Stud. 38, 63–72. doi: 10.1080/03055698.2011.567026
Pandria, N., Petronikolou, V., Lazaridis, A., Karapiperis, C., Kouloumpris, E., Spachos, D., et al. (2022). Information system for symptom diagnosis and improvement of attention deficit hyperactivity disorder: protocol for a nonrandomized controlled pilot study. JMIR Res. Protoc. 11:e40189. doi: 10.2196/40189
Pandria, N, Spachos, D, Athanasiou, A, and Bamidis, PD. Assessing emotional impact of biofeedback and neurofeedback training in smokers during a smoking cessation project. In 2017 IEEE 30th International Symposium on Computer-Based Medical Systems (CBMS). Thessaloniki, Greece: IEEE. (2017). p. 486–491.
Pandria, N, Spachos, D, and Bamidis, PD. The future of mobile health ADHD applications: projecting WHAAM application on future mobile health. Proceedings of 2015 International Conference on Interactive Mobile Communication Technologies and Learning, IMCL. Thessaloniki, Greece. (2015).
Pelham, W. E. Jr., Fabiano, G. A., and Massetti, G. M. (2005). Evidence-based assessment of attention deficit hyperactivity disorder in children and adolescents. J. Clin. Child Adolesc. Psychol. 34, 449–476. doi: 10.1207/s15374424jccp3403_5
Picard, R. W., Papert, S., Bender, W., Blumberg, B., Breazeal, C., Cavallo, D., et al. (2004). Affective learning — a manifesto. BT Technol. J. 22, 253–269. doi: 10.1023/B:BTTJ.0000047603.37042.33
Pizato, E. C. G., Marturano, E. M., and Fontaine, A. M. G. V. (2014). Trajetórias de habilidades sociais e problemas de comportamento no ensino fundamental: influência da educação infantil. Psicol Reflexão e Crítica 27, 189–197. doi: 10.1590/S0102-79722014000100021
Pronovost, P. J., Cole, M. D., and Hughes, R. M. (2022). Remote patient monitoring during COVID-19. JAMA 327, 1125–1126. doi: 10.1001/jama.2022.2040
Rae, T. (2012). “Developing emotional literacy – approaches for staff and students developing an approach in the SEBD school” in Transforming troubled lives: strategies and interventions for children with social, emotional and behavioural difficulties. eds. J. Visser, H. Daniels, and T. Cole, International Perspectives on Inclusive Education, vol. 2 (Bingley, West Yorkshire Emerald Group Publishing Limited), 1–18.
Rae, T., Cowell, N., and Field, L. (2017). Supporting teachers’ well-being in the context of schools for children with social, emotional and behavioural difficulties. Emot. Behav. Difficulties 22, 200–218. doi: 10.1080/13632752.2017.1331969
Riley-Tillman, T. C., Kalberer, S. M., and Chafouleas, S. M. (2005). Selecting the right tool for the job: a review of behavior monitoring tools used to assess student response to intervention. Calif. Sch. Psychol. 10, 81–91. doi: 10.1007/BF03340923
Romanopoulou, E. D., Zilidou, V. I., and Bamidis, P. D. (2017). Creating and sustaining a social health care ecosystem: the case of LLM care services in Greece. Hell. J. Nucl. Med. 20, 40–48.
Romanopoulou, E. D., Zilidou, V. I., Gilou, S., Dratsiou, I., Varella, A., Petronikolou, V., et al. (2021). Technology enhanced health and social care for vulnerable people during the COVID-19 outbreak. Front. Hum. Neurosci. 15:15. doi: 10.3389/fnhum.2021.721065/full
Scanlon, G., and Barnes-Holmes, Y. (2013). Changing attitudes: supporting teachers in effectively including students with emotional and behavioural difficulties in mainstream education. Emot. Behav. Difficulties 18, 374–395. doi: 10.1080/13632752.2013.769710
Shephard, K. (2008). Higher education for sustainability: seeking affective learning outcomes. Int. J. Sustain. High. Educ. 9, 87–98. doi: 10.1108/14676370810842201
Simpson, G. A., Cohen, R. A., Bloom, B., and Blumberg, S. J. (2009). The impact of children’s emotional and behavioural difficulties on their lives and their use of mental health services. Paediatr Perinat Epidemiol 23, 472–481. doi: 10.1111/j.1365-3016.2009.01043.x
Siouli, S, Dratsiou, I, Tsitouridou, M, Kartsidis, P, Spachos, D, and Bamidis, PD. Evaluating the AffectLecture Mobile App within an Elementary School Class Teaching Process. In 2017 IEEE 30th International Symposium on Computer-Based Medical Systems (CBMS). Thessaloniki, Greece: IEEE; (2017). p. 481–485.
Siouli, S., Makris, S., Romanopoulou, E., and Bamidis, P. P. D. (2020). “Living with learning difficulties: two case studies exploring the relationship between emotion and performance in students with learning difficulties” in Addressing global challenges and quality education. EC-TEL 2020. Lecture notes in computer science. eds. C. Alario-Hoyos, M. J. Rodríguez-Triana, M. Scheffel, I. Arnedillo-Sánchez, and S. M. Dennerlein, vol. 12315 (Cham: Springer), 131–143.
Spachos, D, Chifari, A, Chiazzese, G, Merlo, G, Doherty, G, and Bamidis, P. WHAAM: a mobile application for ubiquitous monitoring of ADHD behaviors. In 2014 International Conference on Interactive Mobile Communication Technologies and Learning (IMCL2014). Thessaloniki, Greece: IEEE. (2014). p. 305–309.
Strawhun, J, Norris, L, and Peterson, RL. (2013). Student behavior monitoring. Available at: https://www.researchgate.net/profile/Student-Engagement/publication/292147655_Behavior_Monitoring/links/56aa8c9708aeadd1bdcad56d/Behavior-Monitoring.pdf
Sutherland, K. S., Lewis-Palmer, T., Stichter, J., and Morgan, P. L. (2008). Examining the influence of teacher behavior and classroom context on the behavioral and academic outcomes for students with emotional or behavioral disorders. J. Spec. Educ. 41, 223–233. doi: 10.1177/0022466907310372
Sutherland, K. S., and Oswald, D. P. (2005). The relationship between teacher and student behavior in classrooms for students with emotional and Behavioral disorders: transactional processes. J Child Fam Stud 14, 1–14. doi: 10.1007/s10826-005-1106-z
Twemlow, S. W., and Fonagy, P. (2005). The prevalence of teachers who bully students in schools with differing levels of behavioral problems. Am. J. Psychiatry 162, 2387–2389. doi: 10.1176/appi.ajp.162.12.2387
Keywords: assistive technologies, eHealth, serious games, disability, Attention Deficit Hyperactivity Disorder, BrainHQ
Citation: Pandria N and Bamidis PD (2023) Technology-based solutions for behavior monitoring: lessons learned. Front. Educ. 8:1150239. doi: 10.3389/feduc.2023.1150239
Edited by:
George DuPaul, Lehigh University, United StatesReviewed by:
Stella Nicolaou, University of Nicosia, CyprusRania Petro, University of Piraeus, Greece
Copyright © 2023 Pandria and Bamidis. This is an open-access article distributed under the terms of the Creative Commons Attribution License (CC BY). The use, distribution or reproduction in other forums is permitted, provided the original author(s) and the copyright owner(s) are credited and that the original publication in this journal is cited, in accordance with accepted academic practice. No use, distribution or reproduction is permitted which does not comply with these terms.
*Correspondence: Panagiotis D. Bamidis, YmFtaWRpc0BhdXRoLmdy